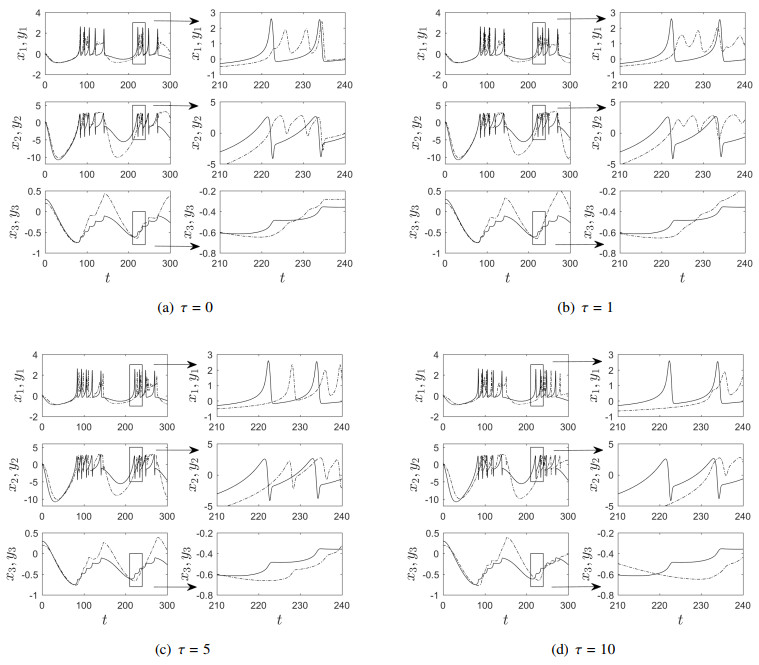
This research presents an adaptive synchronization approach crafted to facilitate exact lag synchronization between a pair of unidirectionally linked Hindmarsh-Rose (HR) neurons, taking into account both explicit propagation delays and the existence of uncertain parameters. The precise condition for lag synchronization is deduced analytically, utilizing the Laplace transform and convolution theorem, alongside the iterative approach within the framework of Volterra integral equations theory. The established criterion guarantees robust stability irrespective of the propagation delay's magnitude, facilitating the realization of approximate lag and anticipating synchronization in a pair of HR neurons. The approximate synchronizations are realized in the absence of direct time-delay coupling, with the Taylor series expansion serving as an alternative to the precise time-delay component. Numerical simulations are executed to validate the effectiveness of the suggested approximate synchronization approach. The research demonstrates that employing the current state of an HR neuron, despite having uncertain parameters, enables the accurate prediction of future states and the reconstruction of past states. This study provides a novel perspective for comprehending neural processes and the advantageous attributes inherent in nonlinear and chaotic systems.
Citation: Bin Zhen, Ya-Lan Li, Li-Jun Pei, Li-Jun Ouyang. The approximate lag and anticipating synchronization between two unidirectionally coupled Hindmarsh-Rose neurons with uncertain parameters[J]. Electronic Research Archive, 2024, 32(10): 5557-5576. doi: 10.3934/era.2024257
[1] | Xuerong Shi, Zuolei Wang, Lizhou Zhuang . Spatiotemporal pattern in a neural network with non-smooth memristor. Electronic Research Archive, 2022, 30(2): 715-731. doi: 10.3934/era.2022038 |
[2] | Moutian Liu, Lixia Duan . In-phase and anti-phase spikes synchronization within mixed Bursters of the pre-Bözinger complex. Electronic Research Archive, 2022, 30(3): 961-977. doi: 10.3934/era.2022050 |
[3] | Bin Zhen, Wenwen Liu, Lijun Pei . An analytic estimation for the largest Lyapunov exponent of the Rössler chaotic system based on the synchronization method. Electronic Research Archive, 2024, 32(4): 2642-2664. doi: 10.3934/era.2024120 |
[4] | Ariel Leslie, Jianzhong Su . Modeling and simulation of a network of neurons regarding Glucose Transporter Deficiency induced epileptic seizures. Electronic Research Archive, 2022, 30(5): 1813-1835. doi: 10.3934/era.2022092 |
[5] | Huijun Xiong, Chao Yang, Wenhao Li . Fixed-time synchronization problem of coupled delayed discontinuous neural networks via indefinite derivative method. Electronic Research Archive, 2023, 31(3): 1625-1640. doi: 10.3934/era.2023084 |
[6] | Yu-Jing Shi, Yan Ma . Finite/fixed-time synchronization for complex networks via quantized adaptive control. Electronic Research Archive, 2021, 29(2): 2047-2061. doi: 10.3934/era.2020104 |
[7] | Jiaqi Chang, Xiangxin Yin, Caoyuan Ma, Donghua Zhao, Yongzheng Sun . Estimation of the time cost with pinning control for stochastic complex networks. Electronic Research Archive, 2022, 30(9): 3509-3526. doi: 10.3934/era.2022179 |
[8] | Jun Guo, Yanchao Shi, Weihua Luo, Yanzhao Cheng, Shengye Wang . Exponential projective synchronization analysis for quaternion-valued memristor-based neural networks with time delays. Electronic Research Archive, 2023, 31(9): 5609-5631. doi: 10.3934/era.2023285 |
[9] | Yuzhi Zhao, Honghui Zhang, Zilu Cao . The important role of astrocytes in activity pattern transition of the subthalamopallidal network related to Parkinson's disease. Electronic Research Archive, 2024, 32(6): 4108-4128. doi: 10.3934/era.2024185 |
[10] | Shuang Liu, Tianwei Xu, Qingyun Wang . Effect analysis of pinning and impulsive selection for finite-time synchronization of delayed complex-valued neural networks. Electronic Research Archive, 2025, 33(3): 1792-1811. doi: 10.3934/era.2025081 |
This research presents an adaptive synchronization approach crafted to facilitate exact lag synchronization between a pair of unidirectionally linked Hindmarsh-Rose (HR) neurons, taking into account both explicit propagation delays and the existence of uncertain parameters. The precise condition for lag synchronization is deduced analytically, utilizing the Laplace transform and convolution theorem, alongside the iterative approach within the framework of Volterra integral equations theory. The established criterion guarantees robust stability irrespective of the propagation delay's magnitude, facilitating the realization of approximate lag and anticipating synchronization in a pair of HR neurons. The approximate synchronizations are realized in the absence of direct time-delay coupling, with the Taylor series expansion serving as an alternative to the precise time-delay component. Numerical simulations are executed to validate the effectiveness of the suggested approximate synchronization approach. The research demonstrates that employing the current state of an HR neuron, despite having uncertain parameters, enables the accurate prediction of future states and the reconstruction of past states. This study provides a novel perspective for comprehending neural processes and the advantageous attributes inherent in nonlinear and chaotic systems.
The Hindmarsh-Rose (HR) neuron model [1] continues to be among the most widely used mathematical representations for characterizing the dynamics of an individual neuron. It is an enhancement of Fitzhugh's B.V.P. model [2], distinguished by the feature that each neuronal firing is followed by an extended interspike interval, a characteristic that closely mirrors the behavior observed in actual neurons. The HR model adeptly encapsulates the quintessential features of neuronal activity, encompassing both the genesis of action potentials and the manifestation of oscillations. Additionally, the model incorporates nonlinear components that elucidate the gradual recovery and adaptive processes witnessed in authentic neuronal functioning. Rosa et al. [3] examined a series of unidirectional coupled HR neurons, each exhibiting periodic spiking behavior. They observed that the initial periodic oscillations of certain neurons evolved into localized spiking-bursting chaos along the network, which later transitioned into regular slow oscillations, despite the ongoing chaotic nature of the spiking activity. The HR neuron model can exhibit complex dynamical behaviors, and therefore it has been broadly applied to investigate the dynamics of individual neurons and their reciprocal influences within neural networks [4,5].
Synchronization is characterized by the alignment or unison of oscillations or behaviors within separate systems or components. It materializes when a pair or more of oscillators or dynamical systems synchronize their operations to achieve a state of phase-locking or to maintain a coherent relationship as time progresses. Synchronization is a key phenomenon that manifests in a variety of natural and man-made systems, with a wide array of applications spanning diverse domains [6]. The synchronization of neuronal activity is integral to the processing of information and the execution of cognitive functions within the brain. Grasping the principles of synchronization in neural systems offers significant insights into brain functionality, cognitive mechanisms, and the nature of neurological disorders. It holds significance for the innovation of new therapeutic approaches, the evolution of brain-inspired computational models, and the progression of brain-machine interface technologies [7].
Lag synchronization and anticipating synchronization are two specialized types of synchronization phenomena that emerge in the context of dynamical systems. Traditional synchronization is characterized by the real-time concordance of dynamical behaviors, whereas lag synchronization and anticipating synchronization entail a temporal offset in the synchronization between the systems involved. Lag synchronization is characterized by the synchronization of two interconnected systems' states, albeit with a temporal delay or lag in their alignment. In this scenario, one system precedes or follows the other by a consistent time delay [8]. The synchronization observed is not instantaneous, but maintains a reliable relationship characterized by a constant phase discrepancy. The phenomenon of lag synchronization is evident in a wide array of both natural and engineered systems, ranging from systems of coupled oscillators and chaotic systems to biological systems, including but not limited to neural networks [9,10,11]. Anticipating synchronization is distinguished by the capacity of one system to project or foretell the forthcoming dynamics of its counterpart [12,13]. In this instance, the synchronizing system replicates the dynamics of the reference system, albeit with a temporal lead. The system engaged in anticipating synchronization not only tracks the target system, but also projects its forthcoming states. The occurrence of anticipating synchronization has been identified in setups that incorporate time-delayed feedback within the context of neural networks, as well as in predictive control systems. In the field of neuroscience, the study of anticipating synchronization has been pursued to shed light on the brain's neural coding processes and the mechanisms underlying prediction [14,15]. The phenomena of lag synchronization and anticipating synchronization exhibit captivating behaviors and have been harnessed in a multitude of fields. They offer profound understanding into the inner workings of intricate systems and pave the way for the creation of innovative technologies that leverage synchronization principles.
Exact synchronization, both in lag and anticipation forms, between a pair of oscillators can be achieved through the application of explicit time delay coupling [11,14]. Alternatively, approximate lag synchronization can be instigated in reciprocally coupled oscillators that exhibit a disparity in parameters [16]. Specifically, both intermittent and continuous lag synchronizations have manifested as transitional stages en route from phase synchronization to full synchronization as the coupling strength is augmented [17,18,19]. Anticipating synchronization has also been detected in systems that do not incorporate time delays. This type of phenomenon was initially documented through the classical Voss scheme, which substitutes the actual time-delay term with its first-order approximation [20]. The research has substantiated the feasible existence of a real-time predictor for chaotic dynamics in systems devoid of inherent delays. The Voss scheme facilitates the adjustment of the anticipation time without necessitating any changes to the dynamics of the drive system. However, the synchronization achieved in this manner is not perfectly precise. Pyragienė and Pyragas [21] demonstrated that anticipating synchronization can emerge in unidirectionally coupled chaotic systems that are not identical, possess significantly disparate parameters, and operate without self-feedback of time-delay in the slave system. To transcend the constraint of a brief anticipation time, Pyragienė and Pyragas introduced an innovative coupling scheme devoid of time-delay. This scheme comprises a master system and two slave systems arranged in series, with the use of switching parameters to accomplish anticipating synchronization over an extended anticipation period [22]. In each of the aforementioned scenarios, it is imperative to have prior knowledge of all the parameters of the interconnected systems to ascertain the conditions for lag and anticipating synchronization. Ji et al. [23], Liu et al. [24], and Sun et al. [25] developed adaptive control mechanisms aimed at realizing lag and anticipating synchronization in systems with time delays and uncertain parameters, leveraging the Lyapunov stability theory. However, the synchronization conditions obtained using the Lyapunov method are usually too conservative, which is not conducive to their practical implementation in real-world applications. Actually, relatively few studies have been made available in the literature concerning the approximate lag and anticipating synchronization of interconnected oscillators that encompass uncertain parameters in the absence of time delay.
In this manuscript, we introduce an innovative coupling strategy devoid of time delays tailored for a pair of HR neurons characterized by uncertain parameters, facilitating the achievement of approximate lag and anticipating synchronization. Initially, we devise an adaptive control mechanism and formulate the precise condition for lag synchronization between two HR neurons, factoring in time-delayed coupling and the presence of uncertain parameters. Divergent from other studies, lag synchronization is regarded here as a distinct variant of generalized synchronization, which can be explored through the application of the auxiliary system methodology [26]. We did not use the Lyapunov method, even though there are unknown parameters in the coupled systems. Numerical simulations are conducted to affirm the stability of the synchronization condition across a range of time delay values. A broad spectrum of approximate lag and anticipating synchronization can be attained by substituting the actual time delay term with its Taylor series expansion. This research provides a method that utilizes the present condition of an HR neuron with uncertain parameters and facilitates the precise forecasting of subsequent states and the reconstitution of antecedent states.
The structure of the paper is outlined as follows. In Section 2, we elucidate our proposed coupling scheme, while Section 3 is dedicated to the derivation of analytical conditions for precise lag synchronization. Section 4 focuses on the formulation of parameter update laws, along with numerical illustrations of exact lag synchronization between a pair of HR neurons featuring time delay coupling and an examination of its resilience to variations in time delay. Section 5 delves into the achievements of approximate lag and anticipating synchronization. Finally, Section 6 presents our concluding remarks.
The form of the HR neuron system is given by
˙u=v−au3+bu2−w+Iext,˙v=c−du2−v,˙w=rs0(u−x0)−rw, | (2.1) |
where u is the membrane potential, v is a recovery variable associated with fast current of N+a or K+ ions, and w represents a slowly changing adaptation current of, for example, C+a ions. a, b, c, d, s0, r, and x0 are uncertain parameters to be identified, and Iext is the external current input.
The HR neuronal model is a widely utilized mathematical framework for depicting the intricate dynamics of neuronal activity. It employs a set of three interconnected first-order differential equations to mimic the fluctuating membrane potentials of neurons, which can result in diverse neuronal activities such as quiescence, spiking, and bursting. However, in real-world scenarios, the HR model's parameters can be subject to uncertainty due to a range of influences, including errors in measurement, simplifications made in the model, and the natural variation present within biological systems. Investigating the synchronization of HR neuronal systems with uncertain parameters is essential for gaining insights and exerting control over the chaotic dynamics of neurons. For system (2.1), we consider all parameters to be uncertain. By implementing an adaptive control strategy and establishing the parameter update laws, we aim to realize in this section exact lag synchronization between a pair of HR neurons, all without the need for precise knowledge of the system's parameters.
If the true values of the uncertain parameters of system (2.1) are chosen as a=1.0, b=3.0, c=1.0, d=5.0, r=0.006, s0=4.0, x0=−1.6, and Iext=3.0, system (2.1) is chaotic bursting. For convenience, by moving the equilibrium of system (2.1) to the origin, it can be rewritten as
˙x1=−ϕ1x31+ϕ5x21+ϕ6x1+x2−x3,˙x2=−ϕ2x21+ϕ7x1−x2,˙x3=ϕ3(ϕ4x1−x3), | (2.2) |
where ϕ1=a, ϕ2=d, ϕ3=r, ϕ4=s0, ϕ5=−3au0+b, ϕ6=−3au20+2bu0, ϕ7=−2du0, and u0 is the unique real root to equation
au30+(d−b)u20+s0u0−(s0x0+c+Iext)=0. | (2.3) |
In the following, we consider ϕj, j=1,2,⋯, 7, as uncertain parameters in system (2.2) to be identified. The true values of the uncertain parameters ϕj, j=1,2,⋯, 7, are taken as (ϕ4,5,6 are obtained on basis of Eq (2.3))
ϕ01=1.0,ϕ02=5.0,ϕ03=0.006,ϕ04=4.0,ϕ05=5.3646,ϕ06=−6.5931,ϕ07=7.8822. | (2.4) |
System (2.2) is chosen as the drive system, and the response HR neuron system is described as follows:
˙y1=−ψ11y31+ψ15y21+ψ16y1+y2−y3+kδ1,˙y2=−ψ12y21+ψ17y1−y2,˙y3=ψ13(ψ14y1−y3), | (2.5) |
where ψ1j, j=1,2,⋯, 7, are the estimated values of uncertain parameters ϕj, respectively, k is the control parameter, and δ1=x1(t−τ)−y1, τ is the time delay in the signal transmission. If there exists
limt→∞||xi(t−τ)−yi||=0,i=1,2,3, | (2.6) |
then lag synchronization is said to be reached for two HR neurons in systems (2.2) and (2.5).
To determine the uncertain parameters in systems (2.2) and (2.5), the parameter update laws are designed as follows:
˙ψ1j=gj(ψ1j−ϕj,δ1),j=1,2,⋯,7, | (2.7) |
where gj:R→R are functions satisfying gj(0,0)=0, j=1,2,⋯, 7. Our objective is to identify suitable expressions for gj, j=1,2,⋯, 7, in Eq (2.7) and to select an appropriate range for k within Eq (2.5). This selection is intended to facilitate lag synchronization between the systems described by Eqs (2.2) and (2.5). Concurrently, ψ1j in Eqs (2.5) and (2.7) should converge to their respective values ϕj, j=1,2,⋯, 7.
Indeed, the lag synchronization occurring between systems (2.2) and (2.5) can be classified as a distinct variant of generalized synchronization. This phenomenon can be identified by employing the method of an auxiliary system, as referenced in [26]. Let us contemplate the scenario where systems (2.5) and (2.7) are identical replicas, both being influenced by an identical signal emanating from system (2.2),
˙z1=−ψ21z31+ψ25z21+ψ26z1+z2−z3+kδ2,˙z2=−ψ22z21+ψ27z1−z2,˙z3=ψ23(ψ24z1−z3),˙ψ2j=gj(ψ2j−ϕj,δ2),j=1,2,⋯,7, | (2.8) |
where ψ2j, j=1,2,⋯,7, are the estimated values of uncertain parameters ϕj, respectively, and δ2=x1(t−τ)−z1. From the auxiliary system approach, lag synchronization in systems (2.2) and (2.5) can be reached, and ψ1j adapt themselves to the true values ψ1j=ϕj, j=1,2,⋯,7, respectively, if the following conditions are satisfied:
limt→∞||yi−zi||=0,i=1,2,3,limt→∞||ψ1j−ψ2j||=0,j=1,2,⋯,7. | (2.9) |
By letting
e1=y1−z12,e2=y2−z22,e3=y3−z32,e4j=ψ1j−ψ2j2,e5=y1+z12,e6=y2+z22,e7=y3+z32,e8j=ψ1j+ψ2j2,j=1,2,⋯,7, |
systems (2.2), (2.5), (2.7), and (2.8) are given as
˙e1=(e86−k)e1+e2−e3+(2e85e5−3e81e25)e1+(e45−3e41e5)e21−e81e31+e46e5+e45e25−e41e35,˙e2=(e87−2e82e5)e1−e2+e47e5−e42(e21+e25),˙e3=(e43e44+e83e84)e1−e83e3+(e43e84+e44e83)e5−e43e7,˙e4j=12[gj(e8j+e4j−ϕj,x1(t−τ)−e5−e1)−gj(e8j−e4j−ϕj,x1(t−τ)−e5+e1)],˙e5=e46e1+e85e21−e41e31+(e86+2e1e45−3e81e21)e5+(e85−3e1e41)e25−e81e35+e6−e7+k(x1(t−τ)−e5),˙e6=e47e1+(e87−2e42e1)e5−e6−e82(e21+e25),˙e7=(e43e44+e83e84)e5+(e84e1−e3)e43+e83(e44e1−e7),˙e8j=12[gj(e8j+e4j−ϕj,x1(t−τ)−e5−e1)+gj(e8j−e4j−ϕj,x1(t−τ)−e5+e1)],j=1,2,⋯,7. | (3.1) |
Condition (2.9) becomes
limt→∞||ei||=0,i=1,2,3,limt→∞||e4j||=0,j=1,2,⋯,7. | (3.2) |
If condition (3.2) is satisfied, then it is evident that e8j→ϕj, j=1,2,⋯,7, holds. For sufficiently small e1,2,3 and e4j, j=1,2,⋯,7, e8j can be expressed as e8j=ϕj+U8j(t), j=1,2,⋯,7. Simultaneously, near the origin, the right-hand side of the first four equations in system (3.1) can be written as
˙e1=(ϕ6−k)e1+e2−e3+P1,˙e2=ϕ7e1−e2+P2,˙e3=ϕ3ϕ4e1−ϕ3e3+P3,˙e4j=γ1je1+γ2je4j+Fj,j=1,2,⋯,7, | (3.3) |
where
P1=(2e85e5−3e81e25)e1+(e45−3e41e5)e21−e81e31+e46e5+e45e25−e41e35+U86(t)e1,P2=−2e82e5e1+e47e5−e42(e21+e25)+U87(t)e1,P3=e43e44e1+(e43e84+e44e83)e5−e43e7+U83(t)U84(t)−U83(t)e3,γ1j=−∂gj(0,0)∂δ1,γ2j=∂gj(0,0)∂(ψ1j−ϕj),Fj=1(2n+1)!∞∑n=1[∂(2n+1)gj(0,0)∂(ψ1j−ϕj)(2n+1)e(2n+1)4j−∂(2n+1)gj(0,0)∂δ(2n+1)1e(2n+1)1],j=1,2,⋯,7. |
Consider the following definition of the Laplace transform pair:
Ei(s)=L[ei]=∫+∞0ei(t)e−stdt,E4j(s)=L[e4j]=∫+∞0e4j(t)e−stdt,ei(t)=L−1[Ei]=12πi∫σ+i∞σ−i∞Ei(s)estds,e4j(t)=L−1[E4j]=12πi∫σ+i∞σ−i∞E4j(s)estds,i=1,2,3;j=1,2,⋯,7. | (3.4) |
Taking the Laplace transforms on both sides of system (3.3), one has
(S−M)[E1,E2,E3,E4j]T=[e10,e20,e30,e4j0]T+[W1,W2,W3,W4j]T, | (3.5) |
where
S=[s0000s0000s0000sI7],M=[ϕ6−k1−10ϕ7−100ϕ3ϕ40−ϕ30γ100γ2]≡[M1M2M3γ2], | (3.6) |
I7 is the 7×7 real identity matrix; γ1=[γ11,γ12,⋯,γ17]T, γ2=diag{γ21,γ22,⋯,γ27}; ei0, i=1,2,3, and e4j0, j=1,2,⋯,7, are given initial values of system (3.3); and W1,2,3 and W4j, j=1,2,⋯,7, are the Laplace transforms of the nonlinear parts P1,2,3 and Fj, j=1,2,⋯,7, in Eq (3.3), respectively, i.e.,
Wi=L[Pi],i=1,2,3;W4j=L[Fj],j=1,2,⋯,7. |
Solving Eq (3.5) using Cramer's rule yields
E1=e10s2+β4s+β5D1(s)+[s2+(ϕ3+1)s+ϕ3]W1D1(s)+(s+ϕ3)W2D1(s)−(s+1)W3D1(s),E2=e20s2+β6s+β7D1(s)+ϕ7(s+ϕ3)W1D1(s)+[s2+(β1−1)s+β8]W2D1(s)−ϕ7W3D1(s),E3=e30s2+β9s+β10D1(s)+ϕ3ϕ4(s+1)W1D1(s)+ϕ3ϕ4W2D1(s)+[s2+(β1−ϕ3)s+(k−ϕ6−ϕ7)]W3D1(s),E4j=e4j0s−γ2j+γ1jE1+W4js−γ2j,j=1,2,⋯,7, | (3.7) |
where D1(s)=s3+β1s2+β2s+β3, which is the characteristic polynomial of matrix M1 in Eq (3.6); β1=k+ϕ3+1−ϕ6, β2=(k+ϕ4+1−ϕ6)ϕ3+k−ϕ6−ϕ7, β3=(k+ϕ4−ϕ6−ϕ7)ϕ3, β4=e10(ϕ3+1)+e20−e30, β5=ϕ3(e10+e20)−e30, β6=e10ϕ7+e20(k+ϕ3−ϕ6), β7=e20ϕ3(k+ϕ4−ϕ6)+(e10ϕ3−e30)ϕ7, β8=ϕ3(k+ϕ4−ϕ6), β9=e30(k+1−ϕ6)+e10ϕ3ϕ4, β10=e30(k−ϕ6−ϕ7)+ϕ3ϕ4(e10+e20).
Taking the inverse Laplace transforms of Eq (3.7) and applying the convolution theorem produces
e1=Γ1(t)+∫t0Γ2(t−τ)P1(τ)dτ+∫t0Γ3(t−τ)P2(τ)dτ−∫t0Γ4(t−τ)P3(τ)dτ,e2=Γ5(t)+ϕ7∫t0Γ3(t−τ)P1(τ)dτ+∫t0Γ6(t−τ)P2(τ)dτ−ϕ7∫t0Γ7(t−τ)P3(τ)dτ,e3=Γ8(t)+ϕ3ϕ4∫t0Γ4(t−τ)P1(τ)dτ+ϕ3ϕ4∫t0Γ7(t−τ)P2(τ)dτ+∫t0Γ9(t−τ)P3(τ)dτ,e4j=e4j0eγ2jt+γ1j∫t0eγ2j(t−τ)e1(τ)dτ+∫t0eγ2j(t−τ)Fj(τ)dτ,j=1,2,⋯,7, | (3.8) |
in which
Γ1(t)=L−1[e10s2+β4s+β5D1(s)],Γ2(t)=L−1[s2+(ϕ3+1)s+ϕ3D1(s)],Γ3(t)=L−1[s+ϕ3D1(s)],Γ4(t)=L−1[s+1D1(s)],Γ5(t)=L−1[e20s2+β6s+β7D1(s)],Γ6(t)=L−1[s2+(β1−1)s+β8D1(s)],Γ7(t)=L−1[1D1(s)],Γ8(t)=L−1[e30s2+β9s+β10D1(s)],Γ9(t)=L−1[s2+(β1−ϕ3)s+(k−ϕ6−ϕ7)D1(s)]. |
Theorem 3.1. The necessary condition for ei→0, e4j→0, i=1,2,3, j=1,2,⋯,7, with t→∞ in Eq (3.8) is that all eigenvalues of matrix M1 defined in Eq (3.6) have negative real parts, and
γ2j=∂gj(0,0)∂(ψ1j−ϕj)<0,j=1,2,⋯,7. | (3.9) |
Proof. Without loss of generality, consider the inverse Laplace transform of the true fraction
A1s2+A2s+A3D1(s) |
where Ai, i=1,2,3, are constants, and D1(s) is the characteristic polynomial of matrix M1 in Eq (3.6). There exist the following four cases:
● D1(s) has 3 distinct real roots: s1,s2,s3
A1s2+A2s+A3D1(s)=B1s−s1+B2s−s2+B3s−s3,
where Bi=A1s2+A2s+A3D1(s)(s−si)|s=si, i=1,2,3.
L−1[A1s2+A2s+A3D1(s)]=B1es1t+B2es2t+B3es3t.
● D1(s) has a pair of conjugate complex roots s1,2=ω1±jω2 and a real root s3=ω3
A1s2+A2s+A3D1(s)=A1s2+A2s+A3(s−ω1−jω2)(s−ω1+jω2)(s−ω3)=B1s−ω1−jω2+B2s−ω1+jω2+B3s−ω3,
where B1,2=A1s2+A2s+A3D′1(s)|s=ω1±jω2, B3=A1s2+A2s+A3D1(s)(s−ω3)|s=ω3,
L−1[A1s2+A2s+A3D1(s)]=B1e(ω1+jω2)t+B2e(ω1−jω2)t+B3eω3t.
● D1(s) has two repeated real roots s1,2=s0 and one simple real root s3=sk
A1s2+A2s+A3D1(s)=B1s−s0+B2(s−s0)2+B3s−sk,
where B1=12d2ds2[A1s2+A2s+A3D1(s)(s−s0)2]|s=s0, B2=A1s2+A2s+A3D1(s)(s−s0)2|s=s0,
B3=A1s2+A2s+A3D1(s)(s−sk)|s=sk,
L−1[A1s2+A2s+A3D1(s)]=(B1+B2t)es0t+B3eskt.
● D1(s) has a triple real root: s1,2,3=s0
A1s2+A2s+A3D1(s)=B1s−s0+B2(s−s0)2+B3(s−s0)3,
where B(3−i)=1i!didsi[A1s2+A2s+A3D1(s)(s−s0)3]|s=s0, i=1,2,
B3=[A1s2+A2s+A3D1(s)(s−s0)3]|s=s0,
L−1[A1s2+A2s+A3D1(s)]=(B1+B2t+B3t2)es0t.
Clearly, from the first three equations in Eq (3.8), the necessary condition for ei→0, i=1,2,3, is that all roots of D1(s)=0 have negative real parts. That is, all eigenvalues of matrix M1 defined in Eq (3.6) have negative real parts. From the fourth equation in Eq (3.8), it is obvious that γ2j<0, j=1,2,⋯,7, is necessary for e4j→0, j=1,2,⋯,7.
Under the conditions given in Theorem 3.1, system (3.8) becomes
e1=∫t0Γ2(t−τ)P1(τ)dτ+∫t0Γ3(t−τ)P2(τ)dτ−∫t0Γ4(t−τ)P3(τ)dτ,e2=ϕ7∫t0Γ3(t−τ)P1(τ)dτ+∫t0Γ6(t−τ)P2(τ)dτ−ϕ7∫t0Γ7(t−τ)P3(τ)dτ,e3=ϕ3ϕ4∫t0Γ4(t−τ)P1(τ)dτ+ϕ3ϕ4∫t0Γ7(t−τ)P2(τ)dτ+∫t0Γ9(t−τ)P3(τ)dτ,e4j=γ1j∫t0eγ2j(t−τ)e1(τ)dτ+∫t0eγ2j(t−τ)Fj(τ)dτ,j=1,2,⋯,7, | (3.10) |
Theorem 3.2. ei=0, e4j=0, i=1,2,3, j=1,2,⋯,7, are the unique continuous solutions to Eq (3.10).
Proof. In fact, system (3.10) comprises a collection of Volterra integral equations which can be resolved through the method of successive approximations, as outlined in [27]. Consider the integral equation of the form
y(t)=Φ(t)+∫t0H(t−τ)f(τ,y(τ))dτ, | (3.11) |
where y(t)∈Rn, H is an n×n matrix, and Φ(t) and f(t,y(t)) are vectors with n components. Moreover, the following conditions are satisfied:
● |y(t)|<∞;
● Φ(t) and f are continuous for 0<t<t0, in which 0<t0<+∞;
● |H|∈L[0,ϵ] holds for any 0<ϵ<t0;
● For any η>0, there must exist a constant ρ(η)>0 such that
|f(t,y1)−f(t,y2)|≤ρ(η)|y1−y2|,(|y1|,|y2|≤η). |
From the results given by Nohel [27], the successive approximations
ξ0(t)=0,ξn+1(t)=Φ(t)+∫t0H(t−τ)f(τ,ξn(τ))dτ,n=0,1,2,⋯, |
will uniformly converge to the unique continuous solution y(t)=ξ(t) of Eq (3.11).
Upon juxtaposing equations (3.10) and (3.11), it becomes straightforward to verify that ei=0, e4j=0, i=1,2,3, j=1,2,⋯,7, are the unique continuous solutions to Eq (3.10).
If condition (3.2) is met, it implies that condition (2.6) is also fulfilled. Meanwhile, ψ1j in Eqs (2.5) and (2.7) converge to their respective values ϕj, j=1,2,⋯,7, respectively. From Theorems 3.1 and 3.2, we have the following result.
Theorem 3.3. Lag synchronization can be accomplished between systems (2.2) and (2.5) with parameter update laws (2.7), and the variables ψ1j within Eqs (2.5) and (2.7) will adjust to align with the values of ϕj for j=1,2,⋯,7, provided that every eigenvalue of the matrices γ2 and M1 (as specified in Eq (3.6)) possesses a negative real component.
Remark 1. The condition stipulated in Theorem (3.3) is independent of the time delay τ, indicating its universal applicability regardless of the time delay's magnitude. As a result, the stability of lag synchronization between systems (2.2) and (2.5), when governed by the parameter update laws (2.7), is maintained for any time delay value.
According to the expressions of γ1j and Fj, j=1,2,⋯,7, given in Eq (3.3), if one chooses appropriate parameter update laws so that
∂ngj(0,0)∂δn1=0,n=1,3,5,⋯, | (4.1) |
then under the above circumstances, e4j, j=1,2,⋯,7, is independent of e1,2,3. At this juncture, provided that condition (3.9) is met, the estimated values of the uncertain parameters, denoted by ψ1j for j=1,2,⋯,7 in Eq (2.5), will invariably converge to their respective true values of ϕj over time. This convergence is assured, irrespective of whether lag synchronization is achieved between the systems represented by Eqs (2.2) and (2.5). To elucidate the aforementioned discoveries, we consider that the parameter update laws in Eq (2.7) have the following form:
˙ψ1j=gj(ψ1j−ϕj,δ1)=−(ψj−ϕj)+1−cos(δ1),j=1,2,⋯,7. | (4.2) |
From Eq (3.3), one can check that γ2j=−1<0, for j=1,2,⋯,7. Additionally,
∂ngj(ψ1j−ϕj,δ1)∂δn1={(−1)n−12sin(δ1),n=1,3,5,⋯(−1)n+22cos(δ1),n=2,4,6,⋯. | (4.3) |
Obviously, the parameter update laws designed in Eq (4.2) satisfy condition (4.1). Let us assume that in system (4.2) the values of ψ1j individually converge to ψ1j0 for each j ranging from 1 to 7 after an adequate duration has passed. Based on Theorem 3.3, lag synchronization between systems (2.2) and (2.5) will be achieved if all eigenvalues of the following matrix have negative real parts
M1=[ψ160−k1−1ψ170−10ψ130ψ1400−ψ130]. | (4.4) |
On the basis of the Routh-Hurwitz criterion, the range of k can be determined through the following inequality:
k>ψ160−ψ130−1,k>(−ψ140+ψ160−1)ψ130+ψ160+ψ1701+ψ130,k>−ψ140+ψ160+ψ170,(ψ130+1)k2+θ1k+θ2>0, | (4.5) |
where
θ1=ψ1302+(ψ140−2ψ160+2)ψ130−2ψ160−ψ170+1,θ2=(ψ140−ψ160+1)ψ1302+(ψ1602−(ψ140+2)ψ160)+1)ψ130+ψ1602+(ψ170−1)ψ160−ψ170. |
We conduct numerical simulations on systems (2.2) and (2.5) employing the parameter update laws specified in Eq (4.2) to validate the accuracy of the aforementioned analytical findings. The true values of the uncertain parameters ϕj, j=1,2,⋯,7, are presented in Eq (2.4). The initial conditions are chosen as x1(t)=0.1, x2(t)=0.2, x3(t)=0.3 for t∈(−τ,0] and y1(0)=0.1, y2(0)=y3(0)=0.2, ψ1i(0)=2.0, i=1,2,⋯,7. The control parameter is arbitrarily set to k=0.5. Despite the absence of lag synchronization between systems (2.2) and (2.5), as depicted in Figure 1, the estimated parameters ψ1j within these systems continue to align with the true values of ϕj, for j=1,2,⋯,7, i.e., ψ110=1.0, ψ120=5.0, ψ130=0.006, ψ140=4.0, ψ150=5.3646, ψ160=−6.5931, and ψ170=7.8822, as shown in Figure 2.
To fulfill the requirement of condition (4.5), we opt for k=1.5 and proceed with the numerical simulations for systems (2.2) and (2.5), utilizing the parameter update laws as outlined in Eq (4.2). The parameters and initial conditions, aside from the value of k being adjusted, are consistent with those depicted in Figure 1. The numerical findings, as illustrated in Figure 3, substantiate the effectiveness of the lag synchronization criteria established in the preceding section.
In this section, we delve into the investigation of approximate lag and anticipating synchronization phenomena between systems (2.2) and (2.5), considering a scenario without an explicit time delay. In the preceding section, we examined the stability of lag synchronization between systems (2.2) and (2.5) under parameter update laws (4.2), which is maintained for all values of τ. To achieve approximate lag and anticipating synchronization, we propose substituting the actual time-delay term x1(t−τ) with its Taylor series expansion. Near τ=0, x1(t−τ) in system (2.5) can be approximately expanded as
x1(t−τ)=x1−dx1dtτ+12!d2x1dt2τ2−13!d3x1dt3τ3+⋯, | (5.1) |
where dix1/dti, i=1,2,3, can be determined by taking the derivatives with respect to t on both sides of the first equation in system (2.2), in which the uncertain parameters ϕi are replaced by using their estimated values ψ1j, i=1,2,⋯,7, respectively,
dx1dt=−ψ110x31+ψ150x21+ψ160x1+x2−x3,d2x1dt2=(−3ψ110x21+2ψ150x1+ψ160)dx1dt−ψ120x21+ψ170x1−x2−ψ130(ψ140x1−x3),d3x1dt3=(−3ψ110x21+2ψ150x1+ψ160)d2x1dt2+2(ψ150−3ψ110x1)(dx1dt)2+(ψ170−2ψ120x1−ψ130ψ140)dx1dt+ψ2130(ψ140x1−x3)+ψ120x21−ψ170x1+x2. | (5.2) |
Define the following functions of τ:
Q1(τ)=x1−dx1dtτ,Q2(τ)=x1−dx1dtτ+12!d2x1dt2τ2,Q3(τ)=x1−dx1dtτ+12!d2x1dt2τ2−13!d3x1dt3τ3. | (5.3) |
Q1,2,3 are the first, second, and third-order approximate expressions of x1(t−τ), respectively. From the analysis in previous sections, for small time delay τ the response of system (2.5) with x1(t−τ)=Qi, i=1,2,3, allows an approximate time-shifted synchronization that lags or anticipates the drive of system (2.2) with the lag τ. Actually, system (2.5) with x1(t−τ)=Qi, i=1,2,3, is an intentionally mismatched response system that yields lag (τ>0) and anticipating (τ<0) synchronization. Numerical simulations are conducted for systems (2.2) and (2.5), incorporating parameter update laws (4.2) and the approximations x1(t−τ)=Qi, for i=1,2,3. These simulations are presented in Figures 4 and 5, showcasing the approximate time-shifted synchronizations achieved without the use of time delay coupling. The control parameter is taken as k=1.5 and the initial conditions are chosen as x1(0)=y1(0)=0.1, x2(0)=y2(0)=y3(0)=0.2, x3(0)=0.3, and ψ1j(0)=2.0, j=1,2,⋯,7.
Figures 4 and 5 demonstrate that a broad spectrum of approximate lag and anticipation synchronization is attainable in the two HR neurons, even in the absence of time delay coupling. Overall, employing a higher-order approximation for the actual time delay x1(t−τ) can enhance the precision of the approximation. Consequently, the scope of approximate lag and anticipation can be expanded by incorporating additional higher-order derivatives from the Taylor series expansion. The investigation presented in this section suggests that an HR neuron is capable of precisely approximating both past and future signals based solely on its current signal.
In this manuscript, we introduce an adaptive control scheme and a synchronization technique aimed at achieving lag synchronization between two unidirectionally coupled HR neurons. This approach is applicable even with explicit propagation delays and in the presence of complete uncertainty regarding the system parameters. Utilizing this approach, the response system can accurately track the driver system with a time lag, while simultaneously enabling the identification of the unknown parameters. Distinguished from prior research, the lag synchronization condition is derived here by employing the auxiliary system methodology, recognizing that lag synchronization can be considered a unique form of generalized synchronization. Ultimately, an analytical criterion is formulated to ascertain the emergence of lag synchronization in the two HR neurons. This formulation is grounded in the Laplace transform and the convolution theorem, alongside the iterative techniques within Volterra integral equation theory. The principal benefit of the lag synchronization scheme introduced in this paper is the ability to devise straightforward controllers and parameter update laws, circumventing the need to construct Lyapunov functions, which simplifies its physical implementation. Numerical simulations are employed to substantiate the efficacy of the proposed lag synchronization strategy.
The precise lag synchronization criteria established herein demonstrate robust stability for any magnitude of propagation delay. This breakthrough introduces a pioneering strategy that harnesses the Taylor series expansion for the intrinsic time-delay component. By employing this technique, it becomes feasible to achieve approximate lag synchronization and predictive synchronization in a pair of HR neurons that are interconnected in a one-way manner, eliminating the necessity for direct time-delay linkage. Numerical simulations confirm the feasibility of attaining a broad spectrum of approximate lag and anticipation synchronization in two unidirectionally coupled HR neurons through the application of this approach. Furthermore, the precision of the approximation can be enhanced by incorporating higher-order derivatives from the Taylor series expansion of the actual time-delay term. This paper introduces a straightforward, memoryless method for achieving approximate lag and anticipating synchronization between two unidirectionally coupled HR neurons, even in the presence of uncertain parameters. For an HR neuron characterized by uncertain parameters, it is feasible to accurately anticipate future states or reconstruct past states based solely on the current state information. The approach outlined herein offers an innovative perspective on understanding the dynamics of neural processes, as well as the beneficial attributes of systems that exhibit nonlinearity and chaos. Our findings additionally present a straightforward method to mitigate the adverse impacts of time delays in signal transmission between the two interconnected HR neurons.
Biological neural networks consist of an extensive array of neurons intricately linked together, which underscores the significance of studying neuronal synchronization phenomena at the network scale. The self-organizing capability of multiple neurons refers to the ability of a group of neurons to spontaneously form patterns and structures without external guidance. This phenomenon is a key aspect of complex systems in neuroscience, where the interactions between neurons can lead to emergent properties that are not present in individual neurons. One of our future research priorities is to explore how our proposed method can be adapted for analyzing synchronization among a large ensemble of neurons. It has been observed that anticipating synchronization can sometimes be characterized by the more fundamental notion of negative group delay in a filter, which could potentially be generalized to lag synchronization or positive group delay. In this context, the driven system or its linear approximation serves as a filter, and every stable filter exhibits both positive and negative group delays for specific frequencies. It would be intriguing to interpret the large scale system in this broader framework, especially considering that the group delay approach does not inherently require explicit coupling delays.
The authors declare they have not used Artificial Intelligence (AI) tools in the creation of this article.
The authors gratefully acknowledge the financial support from the National Natural Science Foundation of China grant numbers 11672185 and 11972327.
The authors declare there are no conflicts of interest.
[1] |
J. L. Hindmarsh, R. M. Rose, A model of neuronal bursting using three coupled first-order differential equations, Proc. Roy. Soc. Lond. B, 221 (1984), 87–102. https://doi.org/10.1098/rspb.1984.0024 doi: 10.1098/rspb.1984.0024
![]() |
[2] |
R. Fitzhugh, Impulses and physiological states in theoretical models of nerve membrane, Biophys. J., 1 (1961), 445–466. https://doi.org/10.1016/S0006-3495(61)86902-6 doi: 10.1016/S0006-3495(61)86902-6
![]() |
[3] |
M. L. Rosa, M. I. Rabinovich, R. Huerta, H. D. I. Abarbanel, L. Fortuna, Slow regularization through chaotic oscillation transfer in an unidirectional chain of Hindmarsh-Rose models, Phys. Lett. A, 266 (2000), 88–93. https://doi.org/10.1016/S0375-9601(00)00015-3 doi: 10.1016/S0375-9601(00)00015-3
![]() |
[4] |
D. M. Semenov, A. L. Fradkov, Adaptive synchronization in the complex heterogeneous networks of Hindmarsh-Rose neurons, Chaos, Solitons Fractals, 150 (2021), 111170. https://doi.org/10.1016/j.chaos.2021.111170 doi: 10.1016/j.chaos.2021.111170
![]() |
[5] |
D. N. Hajian, J. Ramadoss, H. Natiq, F. Parastesh, K. Rajagopal, S. Jafari, Dynamics of Hindmarsh–Rose neurons connected via adaptive memristive synapse, Chin. J. Phys., 87 (2024), 311–329. https://doi.org/10.1016/j.cjph.2023.11.001 doi: 10.1016/j.cjph.2023.11.001
![]() |
[6] |
X. Q. Wu, X. Q. Wu, C. Y. Wang, B. Mao, J. A. Lu, J. H. L¨u, et al., Synchronization in multiplex networks, Phys. Rep., 1060 (2024), 1–54. https://doi.org/10.1016/j.physrep.2024.01.005 doi: 10.1016/j.physrep.2024.01.005
![]() |
[7] |
S. Ansarinasab, F. Parastesh, F. Ghassemi, K. Rajagopal, S. Jafari, D. Ghosh, Synchronization in functional brain networks of children suffering from ADHD based on Hindmarsh-Rose neuronal model, Comput. Biol. Med., 152 (2023), 106461. https://doi.org/10.1016/j.compbiomed.2022.106461 doi: 10.1016/j.compbiomed.2022.106461
![]() |
[8] |
E. M. Shahverdiev, S. Sivaprakasam, K. A. Shore, Lag synchronization in time-delayed systems, Phys. Lett. A, 292 (2002), 320–324. https://doi.org/10.1016/S0375-9601(01)00824-6 doi: 10.1016/S0375-9601(01)00824-6
![]() |
[9] |
Z. L. Wang, X. R. Shi, Chaotic bursting lag synchronization of Hindmarsh–Rose system via a single controller, Appl. Math. Comput., 215 (2009), 1091–1097. https://doi.org/10.1016/j.amc.2009.06.039 doi: 10.1016/j.amc.2009.06.039
![]() |
[10] |
R. Smidtaite, L. Saunoriene, M. Ragulskis, Detection of lag synchronization based on matrices of delayed differences, Commun. Nonlinear Sci. Numer. Simul., 116 (2023), 106864. https://doi.org/10.1016/j.cnsns.2022.106864 doi: 10.1016/j.cnsns.2022.106864
![]() |
[11] |
Q. Y. Wang, Q. S. Lu, G. R. Chen, Ordered bursting synchronization and complex wave propagation in a ring neuronal network, Physica A, 374 (2007), 869–878. https://doi.org/10.1016/j.physa.2006.08.062 doi: 10.1016/j.physa.2006.08.062
![]() |
[12] |
H. U. Voss, Anticipating Chaotic synchronization, Phys. Rev. E, 61 (2000), 5115–5119. https://doi.org/10.1103/physreve.61.5115 doi: 10.1103/physreve.61.5115
![]() |
[13] |
H. U. Voss, Dynamic long-term anticipation of chaotic states, Phys. Rev. Lett., 87 (2001), 014102. https://doi.org/10.1103/PhysRevLett.87.014102 doi: 10.1103/PhysRevLett.87.014102
![]() |
[14] |
M. Ciszak, C. Mayol, C. R. Mirasso, R. Toral, Anticipated synchronization in coupled complex Ginzburg-Landau systems, Phys. Rev. E, 92 (2015), 032911. https://doi.org/10.1103/PhysRevE.92.032911 doi: 10.1103/PhysRevE.92.032911
![]() |
[15] |
E. E. Mahmoud, M. Higazy, T. M. Al-Harthi, A new nine-dimensional chaotic lorenz system with quaternion variables: Complicated dynamics, electronic circuit design, anti-anticipating synchronization, and chaotic masking communication application, Mathematics, 7 (2019), 877. https://doi.org/10.3390/math7100877 doi: 10.3390/math7100877
![]() |
[16] |
K. Srinivasan, G. Sivaganesh, T. F. Fozin, I. R. Mohamed, Analytical studies on complete, lag and anticipation synchronization in cascaded circuits with numerical and experimental confirmation, AEU Int. J. Electron. Commun., 159 (2023), 154491. https://doi.org/10.1016/j.aeue.2022.154491 doi: 10.1016/j.aeue.2022.154491
![]() |
[17] |
M. G. Rosenblum, A. S. Pikovsky, J. Kurths, From phase to lag synchronization in coupled chaotic oscillators, Phys. Rev. Lett., 78 (1997), 4193–4196. https://doi.org/10.1103/PhysRevLett.78.4193 doi: 10.1103/PhysRevLett.78.4193
![]() |
[18] |
T. Heil, I. Fischer, W. Els¨asser, J. Mulet, C. R. Mirasso, Chaos synchronization and spontaneous symmetry-breaking in symmetrically delay-coupled semiconductor lasers, Phys. Rev. Lett., 86 (2001), 795–798. https://doi.org/10.1103/PhysRevLett.86.795 doi: 10.1103/PhysRevLett.86.795
![]() |
[19] |
C. Masoller, Anticipation in the synchronization of chaotic semiconductor lasers with optical feedback, Phys. Rev. Lett., 86 (2001), 2782–2785. https://doi.org/10.1103/PhysRevLett.86.2782 doi: 10.1103/PhysRevLett.86.2782
![]() |
[20] |
N. J. Corron, J. N. Blakely, S. D. Pethel, Lag and anticipating synchronization without time-delay coupling, Chaos, 15 (2005), 023110. https://doi.org/10.1063/1.1898597 doi: 10.1063/1.1898597
![]() |
[21] |
T. Pyragien˙e, K. Pyragas, Anticipating spike synchronization in nonidentical chaotic neurons, Nonlinear Dyn., 74 (2013), 297–306. https://doi.org/10.1007/s11071-013-0968-7 doi: 10.1007/s11071-013-0968-7
![]() |
[22] |
T. Pyragien˙e, K. Pyragas, Anticipating synchronization in a chain of chaotic oscillators with switching parameters, Phys. Lett. A, 379 (2015), 3084–3088. https://doi.org/10.1016/j.physleta.2015.10.030 doi: 10.1016/j.physleta.2015.10.030
![]() |
[23] |
D. H. Ji, S. C. Jeong, J. H. Park, S. M. Lee, S. C. Won, Adaptive lag synchronization for uncertain complex dynamical network with delayed coupling, Appl. Math. Comput., 218 (2012), 4872–4880. https://doi.org/10.1016/j.amc.2011.10.051 doi: 10.1016/j.amc.2011.10.051
![]() |
[24] |
Y. C. Liu, C. D. Li, T. W. Huang, X. Wang, Robust adaptive lag synchronization of uncertain fuzzy memristive neural networks with time-varying delays, Neurocomputing, 190 (2016), 188–196. https://doi.org/10.1016/j.neucom.2016.01.018 doi: 10.1016/j.neucom.2016.01.018
![]() |
[25] |
Z. K. Sun, W. Xu, X. L. Yang, Adaptive scheme for time-varying anticipating synchronization of certain or uncertain chaotic dynamical systems, Math. Comput. Modell., 48 (2008), 1018–1032. https://doi.org/10.1016/j.mcm.2007.12.009 doi: 10.1016/j.mcm.2007.12.009
![]() |
[26] |
H. D. I. Abarbanel, N. F. Rulkov, M. M. Sushchik, Generalized synchronization of chaos: The auxiliary system approach, Phys. Rev. E, 53 (1996), 4528–4535. https://doi.org/10.1103/PhysRevE.53.4528 doi: 10.1103/PhysRevE.53.4528
![]() |
[27] |
J. A. Nohel, Some problems in nonlinear Volterra integral equations, Bull. Amer. Math. Soc., 68 (1962), 323–329. https://doi.org/10.1090/S0002-9904-1962-10790-3 doi: 10.1090/S0002-9904-1962-10790-3
![]() |
1. | Shuang Liu, Tianwei Xu, Qingyun Wang, Effect analysis of pinning and impulsive selection for finite-time synchronization of delayed complex-valued neural networks, 2025, 33, 2688-1594, 1792, 10.3934/era.2025081 |