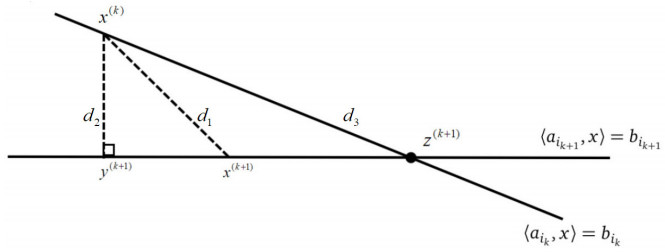
This paper presents a method called MCADFusion, a feature decomposition technique specifically designed for the fusion of infrared and visible images, incorporating target radiance and detailed texture. MCADFusion employs an innovative two-branch architecture that effectively extracts and decomposes both local and global features from different source images, thereby enhancing the processing of image feature information. The method begins with a multi-scale feature extraction module and a reconstructor module to obtain local and global feature information from rich source images. Subsequently, the local and global features of different source images are decomposed using the the channel attention module (CAM) and the spatial attention module (SAM). Feature fusion is then performed through a two-channel attention merging method. Finally, image reconstruction is achieved using the restormer module. During the training phase, MCADFusion employs a two-stage strategy to optimize the network parameters, resulting in high-quality fused images. Experimental results demonstrate that MCADFusion surpasses existing techniques in both subjective visual evaluation and objective assessment on publicly available TNO and MSRS datasets, underscoring its superiority.
Citation: Wangwei Zhang, Menghao Dai, Bin Zhou, Changhai Wang. MCADFusion: a novel multi-scale convolutional attention decomposition method for enhanced infrared and visible light image fusion[J]. Electronic Research Archive, 2024, 32(8): 5067-5089. doi: 10.3934/era.2024233
[1] | Wenya Shi, Xinpeng Yan, Zhan Huan . Faster free pseudoinverse greedy block Kaczmarz method for image recovery. Electronic Research Archive, 2024, 32(6): 3973-3988. doi: 10.3934/era.2024178 |
[2] | Ranran Li, Hao Liu . On global randomized block Kaczmarz method for image reconstruction. Electronic Research Archive, 2022, 30(4): 1442-1453. doi: 10.3934/era.2022075 |
[3] | Yimou Liao, Tianxiu Lu, Feng Yin . A two-step randomized Gauss-Seidel method for solving large-scale linear least squares problems. Electronic Research Archive, 2022, 30(2): 755-779. doi: 10.3934/era.2022040 |
[4] | Jun Guo, Yanchao Shi, Weihua Luo, Yanzhao Cheng, Shengye Wang . Exponential projective synchronization analysis for quaternion-valued memristor-based neural networks with time delays. Electronic Research Archive, 2023, 31(9): 5609-5631. doi: 10.3934/era.2023285 |
[5] | Yun Ni, Jinqing Zhan, Min Liu . Topological design of continuum structures with global stress constraints considering self-weight loads. Electronic Research Archive, 2023, 31(8): 4708-4728. doi: 10.3934/era.2023241 |
[6] | Yanlong Zhang, Rui Zhang, Li Wang . Oblique impact dynamic analysis of wedge friction damper with Dankowicz dynamic friction. Electronic Research Archive, 2024, 32(2): 962-978. doi: 10.3934/era.2024047 |
[7] | Dongmei Yu, Yifei Yuan, Yiming Zhang . A preconditioned new modulus-based matrix splitting method for solving linear complementarity problem of H+-matrices. Electronic Research Archive, 2023, 31(1): 123-146. doi: 10.3934/era.2023007 |
[8] | Haoqing Wang, Wen Yi, Yannick Liu . Optimal assignment of infrastructure construction workers. Electronic Research Archive, 2022, 30(11): 4178-4190. doi: 10.3934/era.2022211 |
[9] | Yu Wang . Bi-shifting semantic auto-encoder for zero-shot learning. Electronic Research Archive, 2022, 30(1): 140-167. doi: 10.3934/era.2022008 |
[10] | Yaguo Guo, Shilin Yang . Projective class rings of the category of Yetter-Drinfeld modules over the 2-rank Taft algebra. Electronic Research Archive, 2023, 31(8): 5006-5024. doi: 10.3934/era.2023256 |
This paper presents a method called MCADFusion, a feature decomposition technique specifically designed for the fusion of infrared and visible images, incorporating target radiance and detailed texture. MCADFusion employs an innovative two-branch architecture that effectively extracts and decomposes both local and global features from different source images, thereby enhancing the processing of image feature information. The method begins with a multi-scale feature extraction module and a reconstructor module to obtain local and global feature information from rich source images. Subsequently, the local and global features of different source images are decomposed using the the channel attention module (CAM) and the spatial attention module (SAM). Feature fusion is then performed through a two-channel attention merging method. Finally, image reconstruction is achieved using the restormer module. During the training phase, MCADFusion employs a two-stage strategy to optimize the network parameters, resulting in high-quality fused images. Experimental results demonstrate that MCADFusion surpasses existing techniques in both subjective visual evaluation and objective assessment on publicly available TNO and MSRS datasets, underscoring its superiority.
Consider to solve a large-scale consistent linear system
Ax=b, | (1.1) |
where the matrix A∈Rm×n, b∈Rm. One of the solutions of the system (1.1) is x∗=A†b, which is the least Euclidean norm solution. Especially, when the coefficient matrix A is full column rank, x∗ is the unique solution of the system (1.1).
There are many researches on solving the system (1.1) through iterative methods, among which the Kaczmarz method is a representative and efficient row-action method. The Kaczmarz method [1] selects the rows of the matrix A by using the cyclic rule and in each iteration, the current iteration point is orthogonally projected onto the corresponding hyperplane. In 1970, Gordon et al. [2] first applied the Kaczmarz method, also known as algebraic reconstruction technique (ART), to the field of computed tomography (CT). In the development of CT field, representative methods include filtered-back projection (FBP) method [3], ART and Maximum entropy method [4,5], etc. However, if the collected data are incomplete, the FBP method performs very poorly and the ART method is widely used in this field [6,7] due to its superior anti-interference performance, implicity and low storage characteristics. Kaczmarz method is also widely applied in image reconstruction [8,9,10,11], distributed computing [12] and signal processing [13]; and so on [14,15,16,17]. In 1971, Tanabe [18] analyzed the theoretical convergence of the Kaczmarz method and obtained the conclusion that when initial vector x(0)∈N(A), the Kaczmarz method converges to the minimum norm solution x∗ of the problem (1.1). In recent years, Kang et al. [19,20] obtained the theoretical convergence rate proof of the Kaczmarz method.
Since the Kaczmarz method cycles through the rows of A, the performance may depend heavily on the ordering of these rows. A poor ordering may result in a very slow convergence rate. McCormick [21] proposed a maximal weighted residual Kaczmarz (MWRK) method and proved its convergence. In recent work, a new theoretical convergence estimate was proposed for the MWRK method in [22]. Strohmer and Vershynin [23] proposed a randomized Kaczmarz (RK) method which selects a given row with proportional to the Euclidean norm of the rows of the coefficient matrix A and proved its convergence. After the above work, research on the Kaczmarz-type methods was reignited recently, see for example, the randomized block Kaczmarz-type methods [24,25,26,27], the greedy version of Kaczmarz-type methods [28,29,30,31,32], the extended version of Kaczmarz-type methods [33,34,35] and many others [36,37,38,39,40,41]. The related works of Kaczmarz also accelerated the development of column action iterative methods represented by the coordinate descent (CD) method [42] (see [43,44,45,46,47,48,49,50], etc.).
Recently, Bai and Wu [28] proposed a new randomized row index selection strategy, which is aimed at grasping larger entries of the residual vector at each iteration and constructed a greedy randomized Kaczmarz (GRK) method. They proved that the convergence of the GRK method is faster than that of the RK method. In [38,51], Popa gave the definition of oblique projection, which broke the limitation of orthogonal projection. In [52], Lorenez and Rose et al. used oblique projection to construct random Kaczmarz methods with mismatched adjoint (RKMA). Recently, Li and Wang et al. [53] proposed a Kaczmarz method with oblique projection (KO). This method continuously projects the current iteration point to the intersection of two hyperplanes and can solve the problem that the rows of the coefficient matrix A are highly linearly correlated as well as the algorithms proposed in [36,54]. They also proposed a uniform random version of KO method—random Kaczmarz method with oblique projection (RKO) and proved theoretically and numerically that KO method and RKO method are faster than Kaczmarz method [1] and RK method [23] respectively. In this paper, we first briefly introduce the oblique projection and give the relationship between the KO method and the CD method. Based on the row index selection rules of two representative randomized and non-randomized Kaczmarz-type methods—the GRK method and the MWRK method, we propose two new Kaczmarz-type methods with oblique projection (KO-type)—the greedy randomized Kaczmarz method with oblique projection (GRKO) and the maximal weighted residual Kaczmarz method with oblique projection (MWRKO) respectively and prove their convergence theoretically and numerically. We emphasize the efficiency of our proposed methods when the rows of the matrix A are highly linearly correlated and find that Kaczmarz-type methods based on orthogonal projection performed poorly when applied to this kind of matrices.
The organization of this paper is as follows. In Section 2, we introduce the KO-type method and give its two lemmas. In Section 3, we propose the GRKO method and MWRKO method naturally and prove the convergence of the two methods. In Section 4, some numerical examples are provided to illustrate the efficiency of our new methods. Finally, some brief concluding remarks are described in Section 5.
In this paper, ⟨⋅,⋅⟩ stands for the scalar product. ‖x‖ is the Euclidean norm of x∈Rn. For a given matrix G=(gij)∈Rm×n, gTi, GT, G†, R(G), N(G), ‖G‖F and λmin(G), are used to denote the ith row, the transpose, the Moore-Penrose pseudoinverse [55], the range space, the null space, the Frobenius norm and the smallest nonzero eigenvalue of G respectively. PC(x) is the orthogonal projection of x onto C, ˜x is any solution of the system (1.1). Let Ek denote the expected value conditonal on the first k iterations, that is,
Ek[⋅]=E[⋅|j0,j1,⋯,jk−1], |
where js(s=0,1,⋯,k−1) is the column chosen at the sth iteration.
In this chapter, we first briefly introduce the definition of oblique projection and analyze the relationship between CD method [42] and KO-type method [53]. Finally, we give two lemmas of KO-type method, which provide a theoretical guarantee for the two oblique projection methods proposed in the next chapter.
The sets Hi={x∈Rn | ⟨ai,x⟩=bi} (i=1,2,⋯,m) are the hyperplanes which associated to the ith equation of the system (1.1). To project the current iteration point x(k) to one of the hyperplanes, the oblique projection [38,51] can be expressed as follows:
x(k+1)=PdHi(x(k))=x(k)−⟨ai,x(k)⟩−bi⟨d,ai⟩d, | (2.1) |
where d∈Rn is a given direction. In Figure 1, x(k+1) is obtained by oblique projection of the current iteration point x(k) to the hyperplane Hik+1 along the direction d1, i.e., x(k+1)=Pd1Hik+1(x(k)). y(k+1) is the iteration point obtained when the direction d2=aik+1, i.e., y(k+1)=Paik+1Hik+1(x(k)). When the direction d=ai(i=mod(k,m)+1), it is the classic Kaczmarz method. However, when the hyperplanes are close to linearly parallel, the Kaczmarz method based on orthogonal projection has a slow iteration speed. In this paper, we use a iteration direction d3=wik=aik+1−⟨aik,aik+1⟩‖ai‖2aik mentioned in [53], to make the current iteration point approach to the intersection of two hyperplanes, i.e., z(k+1)=PwikHik+1(x(k)).
The framework of KO-type method is given as follows:
Algorithm 1 Kaczmarz-type method with oblique projection (KO-type) |
Require:
A∈Rm×n, b∈Rm, x(0)∈Rn, K
1: For i=1:m, M(i)=‖ai‖2 2: Choose i1 based on a certain selection rule 3: Compute x(1)=x(0)+bi1−⟨ai1,x(0)⟩M(i1)ai1 4: for k=1,2,⋯,K do 5: Choose ik+1 based on a certain selection rule 6: Compute Dik=⟨aik,aik+1⟩ and r(k)ik+1=bik+1−⟨aik+1,x(k)⟩ 7: Compute wik=aik+1−DikM(ik)aik and hik(=‖wik‖2)=M(ik+1)−DikM(ik)Dik 8: α(k)ik=r(k)ik+1hik and x(k+1)=x(k)+α(k)ikwik 9: end for 10: Output x(K+1) |
For KO-type method, the residual satisfies
r(k+1)=b−Ax(k+1)=b−A(x(k)+α(k)ik(aik+1−⟨aik,aik+1⟩||aik||2aik))=r(k)−α(k)ik(Aaik+1−⟨aik,aik+1⟩||aik||2Aaik). | (2.2) |
In the next section, the certain selection rules in step 2 and step 5 of algorithm 1 will be given.
In order to get the relationship between the CD method and the KO-type method, we need to explain the construction idea of the CD method [42]. Consider a linear system
˜Ax=b, | (2.3) |
where the coefficient matrix ˜A∈Rn×n is a positive semidefinite matrix and b∈Rn is a real m dimensional vector. In this case, solving the system (2.3) is equivalent to the following strict convex quadratic minimization problem
f(x)=12xT˜Ax−bTx. |
From [42], the next iteration point x(k+1) is the solution to mint∈Rf(x(k)+td), i.e.,
x(k+1)=x(k)+(b−˜Ax(k))TddT˜Add, | (2.4) |
where d is a nonzero direction and x(k) is a current iteration point.
Since the requirement that matrix ˜A is positive semidefinite is not general, problem (2.3) is usually transformed into the following two regularizing linear systems:
ATAx=ATb, | (2.5) |
and
{AATy=b,x=ATy, | (2.6) |
where A∈Rm×n in (2.5) and (2.6) is an arbitrary matrix. Obviously, both ATA and AAT are positive semidefinite matrices, so we can apply systems (2.5) and (2.6) to iteration (2.4).
One natural choice of a set of easily computable search directions is to choose d by successively cycling through the set of canonical unit vectors {e1,⋯,en}, where ei∈Rn (i=1, ⋯, n). Applying system (2.5) to iteration (2.4), we can get:
x(k+1)=x(k)+⟨r(k),Ai⟩||Ai||2ei, |
where i=mod(k,n)+1, Ai is the i-th column of matrix A. This is the iterative formula of CD method [42], also known as Gauss-Seidel method. When d=ei, where ei∈Rm (i=1, ⋯, m), applying system (2.6) to iteration (2.4), we can get:
x(k+1)=x(k)+bi−aTix(k)‖ai‖2ai, |
where i=mod(k,m)+1. This is the iterative formula of Kaczmarz method [1].
Next, we will prove that the KO-type method is an iterative form in the new direction d=eik+1−⟨aik+1,aik⟩||aik||2eik=eik+1−DikM(ik)eik, where ei∈Rm (i=1, ⋯, m). Applying system (2.6) to iteration (2.4), we get:
y(k+1)=y(k)+(b−AATy(k))T(eik+1−DikM(ik)eik)‖AT(eik+1−DikM(ik)eik)‖2(eik+1−DikM(ik)eik)=y(k)+(b−Ax(k))T(eik+1−DikM(ik)eik)hik(eik+1−DikM(ik)eik)=y(k)+r(k)ik+1−DikM(ik)r(k)ikhik(eik+1−DikM(ik)eik). |
Multiply left by AT on both sides of the above equation, we get
x(k+1)=x(k)+r(k)ik+1−DikM(ik)r(k)ikhikwik=x(k)+α(k)ikwik−Dikr(k)ikM(ik)hikwik, k=1,2,⋯. | (2.7) |
Now we prove that r(k)ik=0. In fact,
r(k)ik=bik−⟨aik,x(k)⟩=bik−⟨aik,x(k−1)+r(k−1)ik−Dik−1M(ik−1)r(k−1)ik−1hik−1wik−1⟩=r(k−1)ik−r(k−1)ik+Dik−1M(ik−1)r(k−1)ik−1=Dik−1M(ik−1)r(k−1)ik−1, k=2,3,⋯. |
Therefore, the following formula holds:
r(k)ik=k−1∏s=1DisM(is)r(s)is. |
By the step 3 of Algorithm 1, r(1)i1=0, so
r(k)ik=0(∀k>0). | (2.8) |
Thus the iteration (2.7) becomes
x(k+1)=x(k)+α(k)ikwik, k=1,2,⋯. |
From the above deduction, we have confirmed our idea.
Remark 1. When d=eik+1−⟨Aik+1, Aik⟩‖Aik‖2eik, where ei∈Rn (i=1, ⋯, n), applying system (2.5) to iteration (2.4), we can get the Gauss-Seidel method with oblique direction, see [56] for details.
Remark 2. Formally, iteration (2.1) is the same as RKMA method [52]. Algorithm 1 can be regarded as a special case of vik=aik+1−⟨aik,aik+1⟩‖ai‖2aik in RKMA method. However, the oblique projection vik in RKMA method is more defined in a way of mismatched adjoint, which is different from the oblique projection concept proposed here, see [52] for details.
In this section, we will give two very important lemmas of Algorithm 1 to serve the algorithms mentioned in Chapter 3. The selection rules of row indices i1 in step 2 and ik+1 (k>0) in step 5 of Algorithm 1 do not affect the lemmas.
Lemma 2.1. For the Kaczmarz-type method with oblique projection, the residual satisfies the following equations:
r(k)ik−1=0(∀k>1). | (2.9) |
Proof. From the definition of the KO-type method, since k>1,
x(k)=x(k−1)+α(k−1)ik−1wik−1. |
We get
(b−Ax(k))ik−1=(b−Ax(k−1))ik−1−(Aα(k−1)ik−1wik−1)ik−1, |
that is,
r(k)ik−1=r(k−1)ik−1−α(k−1)ik−1⟨aik−1,wik−1⟩(i)=α(k−1)ik−1⟨aik−1,aik−⟨aik−1,aik⟩||aik−1||2aik−1⟩=0. |
The equality (i) holds due to the Eq (2.8). Thus, the Eq (2.9) holds.
Lemma 2.2. The iteration sequence {x(k)}∞k=0 generated by the Kaczmarz-type method with oblique projection, satisifies the following equations:
||x(k+1)−˜x||2=||x(k)−˜x||2−||x(k+1)−x(k)||2(∀k≥0), | (2.10) |
where ˜x is an arbitrary solution of the system (1.1). Especially, when PN(A)(x(0))=PN(A)(˜x), x(k)−˜x∈R(AT).
Proof. For k=0, the iteration in step 3 of Algorithm 1 is the classical Kaczmarz iteration, so we have
⟨ai1,x(1)−˜x⟩=⟨ai1,x(0)−˜x+bi1−⟨ai1,x(0)⟩M(i1)ai1⟩=⟨ai1,x(0)⟩−bi1+⟨ai1,bi1−⟨ai1,x(0)⟩M(i1)ai1⟩=0, |
which shows that x(1)−˜x is orthogonal to ai1. Therefore, we know
(x(1)−x(0))T(x(1)−˜x)=0. |
It follows that
||x(1)−˜x||2=||x(0)−˜x||2−||x(1)−x(0)||2. |
For k>0, we have
⟨wik,x(k+1)−˜x⟩=⟨wik,x(k)−˜x+α(k)ikwik⟩=⟨aik+1−DikM(ik)aik,x(k)−˜x⟩+⟨wik,r(k)ik+1hikwik⟩(ii)=−r(k)ik+1+DikM(ik)r(k)ik+r(k)ik+1(iii)=0. |
The equality (ii) and equality (iii) hold due to hik=‖wik‖2 and the Eq (2.8) respectively. Thus we get that x(k+1)−˜x is orthogonal to wik. Therefore, we get that
(x(k+1)−x(k))T(x(k+1)−˜x)=0. |
It follows that
||x(k+1)−˜x||2=||x(k)−˜x||2−||x(k+1)−x(k)||2(∀k>0). | (2.11) |
Thus, from the above proof, the Eq (2.10) holds.
According to the iterative formula
{x(1)=x(0)+bi1−⟨ai1,x(0)⟩M(i1)ai1,x(k+1)=x(k)+α(k)ikwik(∀k>0), |
we can get PN(A)(x(k))=PN(A)(x(k−1))=⋯=PN(A)(x(0)), and by the fact that PN(A)(x(0))=PN(A)(˜x), we can deduce that x(k)−˜x∈R(AT).
In this section, we combine the oblique projection with the GRK method [28] and the MWRK method [21] to obtain the GRKO method and the MWRKO method and prove their convergence. Theoretical results show that the KO-type method can accelerate the convergence when there are suitable row index selection strategies.
The core of the GRK method [28] is a new probability criterion, which can grasp the large items of the residual vector in each iteration and randomly select the item with probability in proportion to the retained residual norm. Theories and experiments prove that it can speed up convergence speed. This paper uses the row index selection rule in combination with the Algorithm 1 to obtain the GRKO method and the algorithm is as follows:
Algorithm 2 Greedy randomized Kaczmarz method with oblique projection (GRKO) |
Require: A∈Rm×n, b∈Rm, x(0)∈Rn, K
1: For i=1:m, M(i)=‖ai‖2 2: Uniformly randomly select select i1 and compute x(1)=x(0)+bi1−⟨ai1,x(0)⟩M(i1)ai1 3: for k=1,2,⋯,K−1 do 4: Compute εk=12(1||b−Ax(k)||2max1≤ik+1≤m{|bik+1−⟨aik+1,x(k)⟩|2||aik+1||2}+1||A||2F) 5: Determine the index set of positive integers Uk={ik+1||bik+1−⟨aik+1,x(k)⟩|2≥εk||b−Ax(k)||2||aik+1||2} 6: Compute the ith entry ˜r(k)i of the vector ˜r(k) according to ˜r(k)i={bi−⟨ai,x(k)⟩,if i∈Uk0otherwise 7: Select ik+1∈Uk with probability Pr(row=ik+1)=|˜r(k)ik+1|2||˜r(k)||2 8: Compute Dik=⟨aik,aik+1⟩ 9: Compute wik=aik+1−DikM(ik)aik and hik(=‖wik‖2)=M(ik+1)−DikM(ik)Dik 10: α(k)ik=˜r(k)ik+1hik(=r(k)ik+1hik) and x(k+1)=x(k)+α(k)ikwik 11: end for 12: Output x(K) |
The convergence of the GRKO method is provided as follows.
Theorem 3.1. Consider the consistent linear system (1.1), where the coefficient matrix A∈Rm×n, b∈Rm. Let x(0)∈Rn be an arbitrary initial approximation, ˜x is a solution of system (1.1) such that PN(A)(˜x)=PN(A)(x(0)). Then the iteration sequence{x(k)}∞k=1 generated by the GRKO method obeys
E||x(k)−˜x||2≤k−1Πs=0ζs||x(0)−˜x||2. | (3.1) |
where ζ0=1−(λmin(ATA))m||A||2F,ζ1=1−12(1γ1||A||2F+1)λmin(ATA)Δ⋅||A||2F,ζk=1−12(1γ2||A||2F+1)λmin(ATA)Δ⋅||A||2F (∀k>1), which
γ1=max1≤i≤mm∑s=1s≠i||as||2, | (3.2) |
γ2=max1≤i,j≤mi≠jm∑s=1s≠i,j||as||2, | (3.3) |
Δ=maxj≠ksin2⟨aj,ak⟩(∈(0,1]). | (3.4) |
In addition, if x(0)∈R(AT), the sequence {x(k)}∞k=1 converges to the least-norm solution of the system (1.1), i.e., limk→∞x(k)=x∗=A†b.
Proof. When k=1, we can get
ε1||A||2F=max1≤i2≤m{|bi2−⟨ai2,x(1)⟩|2||ai2||2}2m∑i2=1||ai2||2||A||2F.|bi2−⟨ai2,x(1)⟩|2||ai2||2+12(iv)=max1≤i2≤m{|bi2−⟨ai2,x(1)⟩|2||ai2||2}2m∑i2=1i2≠i1||ai2||2||A||2F.|bi2−⟨ai2,x(1)⟩|2||ai2||2+12≥12(||A||2Fm∑i2=1i2≠i1||ai2||2+1)≥12(1γ1||A||2F+1). | (3.5) |
The equality (iv) holds due to the Eq (2.8).
When k>1, we get
εk||A||2F=max1≤ik+1≤m(|bik+1−⟨aik+1,x(k)⟩|2||aik+1||2)2m∑ik+1=1||aik+1||2||A||2F.|bik+1−⟨aik+1,x(k)⟩|2||aik+1||2+12(v)=max1≤ik+1≤m(|bik+1−⟨aik+1,x(k)⟩|2||aik+1||2)2m∑ik+1=1ik+1≠ik,ik−1||aik+1||2||A||2F.|bik+1−⟨aik+1,x(k)⟩|2||aik+1||2+12≥12( ||A||2Fm∑ik+1=1ik+1≠ik,ik−1||aik+1||2+1)≥12(1γ2||A||2F+1). | (3.6) |
The equality (v) holds due to the Eqs (2.8) and (2.9).
Under the GRKO method, Lemma 2.2 still holds, so we can take the full expectation on both sides of the Eq (2.10) and get that for k=0,
E||x(1)−˜x||2=||x(0)−˜x||2−E||x(1)−x(0)||2=||x(0)−˜x||2−1mm∑i1=1||bi1−⟨ai1,x(0)⟩M(i1)ai1||2(vi)≤||x(0)−˜x||2−1m||b−Ax(0)||2||A||2F(vii)≤(1−λmin(ATA)m||A||2F)||x(0)−˜x||2=ζ0||x(0)−˜x||2, | (3.7) |
and for k>0,
Ek||x(k+1)−˜x||2=||x(k)−˜x||2−Ek||x(k+1)−x(k)||2=||x(k)−˜x||2−∑ik+1∈Uk|bik+1−⟨aik+1,x(k)⟩|2∑ik+1∈Uk|bik+1−⟨aik+1,x(k)⟩|2.|r(k)ik+1|2||wik||2(viii)≤||x(k)−˜x||2−∑ik+1∈Uk|bik+1−⟨aik+1,x(k)⟩|2∑ik+1∈Uk|bik+1−⟨aik+1,x(k)⟩|2.|r(k)ik+1|2Δ⋅||aik+1||2(ix)≤||x(k)−˜x||2−εkΔ||b−Ax(k)||2=||x(k)−˜x||2−εkΔ||A(˜x−x(k))||2(vii)≤(1−εkλmin(ATA)Δ)||x(k)−˜x||2. | (3.8) |
The inequality (vi) of the Eq (3.7) is achieved with the use of the fact that |b1||a1|+|b2||a2|≥|b1|+|b2||a1|+|a2| (if |a1|>0, |a2|>0) and the inequality (viii) of the Eq (3.8) is achieved with the use of the fact that
||wik||2=||aik+1||2−⟨aik,aik+1⟩2||aik||2=sin2⟨aik,aik+1⟩||aik+1||2≤Δ⋅||aik+1||2, | (3.9) |
and the inequality (ix) of the Eq (3.8) is achieved with the use of the definition of Uk which lead to
|bik+1−⟨aik+1,x(k)⟩|2≥εk||b−Ax(k)||2||aik+1||2,∀ik+1∈Uk. |
Here in the last inequalities (vii) of the Eqs (3.7) and (3.8), we have used the estimate ||Au||22≥λmin(ATA)||u||2, which holds true for any u∈Cn belonging to the column space of AT. According to the lemma 2.2, it holds.
By making use of the Eqs (3.5), (3.6) and (3.8), we get
E1||x(2)−˜x||2≤[1−12(1γ1||A||2F+1)λmin(ATA)Δ⋅||A||2F]||x(1)−˜x||2=ζ1||x(1)−˜x||2, |
Ek||x(k+1)−˜x||2≤[1−12(1γ2||A||2F+1)λmin(ATA)Δ⋅||A||2F]||x(k)−˜x||2=ζk||x(k)−˜x||2(∀k>1). |
Finally, by recursion and taking the full expectation, the inequality (3.1) holds.
Remark 3. In the GRKO method, hik is not zero. Suppose hik=0, which means ∃λ>0, λaik=aik+1. Due to the system is consistent, it holds ⟨aik+1,x∗⟩=λ⟨aik,x∗⟩=λbik=bik+1. According to the Eq (2.8), it holds r(k)ik+1=λr(k)ik=0. From step 5 of Algorithm 1, we can know that such index ik+1 will not be selected.
Remark 4. Set ~ζk=1−12(1γ1||A||2F+1)λmin(ATA)||A||2F(∀k>0) and the convergence of GRK method in [28] meets:
Ek‖x(k+1)−x∗‖2≤~ζk‖x(k)−x∗‖2. |
Obviously, ∀Δ∈(0,1], ζ1≤~ζ1,ζk<~ζk(∀k>1) is satisfied, so the convergence speed of GRKO method is faster than GRK method.
Remark 5. In fact, in each iteration, the most computationally expensive part is computing the residual r(k). If B=AAT is calculated before iteration, the GRK method [28] costs 7m+2n+2 flopping operations and the GRKO method costs 9m+3n+6 flopping opeartions, where the residual r(k) is calculated according to Eq (2.2).
The selection strategy for the index ik used in the maximal weighted residual Kaczmarz (MWRK) method [21] is: Set
ik=argmaxi∈{1,2,⋯,m}|aTix(k)−bi|‖ai‖. |
Mccormick proved the exponential convergence of the MWRK method. In [22], a new convergence conclusion of the MWRK method is given. We use its row index selection rule combined with KO-type method to obtain MWRKO method and the algorithm is as follows:
Algorithm 3 Maximal Weighted Residual Kaczmarz Method with Oblique Projection (MWRKO) |
Require: A∈Rm×n, b∈Rm, x(0)∈Rn, K
1: For i=1:m, M(i)=‖ai‖2 2: Compute i1=argmaxi∈{1,2,⋯,m}|aTix(0)−bi|‖ai‖ and x(1)=x(0)+bi1−⟨ai1,x(0)⟩M(i1)ai1 3: for k=1,2,⋯,K do 4: Compute ik+1=argmaxi∈{1,2,⋯,m}|aTix(k)−bi|‖ai‖ 5: Compute Dik=⟨aik,aik+1⟩ and r(k)ik+1=bik+1−⟨aik+1,x(k)⟩ 6: Compute wik=aik+1−DikM(ik)aik and hik(=‖wik‖2)=M(ik+1)−DikM(ik)Dik 7: α(k)ik=r(k)ik+1hik and x(k+1)=x(k)+α(k)ikwik 8: end for 9: Output x(K+1) |
The convergence of the MWRKO method is provided as follows.
Theorem 3.2. Consider the consistent linear system (1.1), where the coefficient matrix A∈Rm×n, b∈Rm. Let x(0)∈Rn be an arbitrary initial approximation, ˜x is a solution of system (1.1) such that PN(A)(˜x)=PN(A)(x(0)). Then the iteration sequence{x(k)}∞k=1 generated by the MWRKO method obeys
||x(k)−˜x||2≤k−1Πs=0ρs||x(0)−˜x||2, | (3.10) |
where ρ0=1−λmin(ATA)‖A‖2F,ρ1=1−λmin(ATA)Δ⋅γ1,ρk=1−λmin(ATA)Δ⋅γ2(∀k>1),which γ1, γ2 and Δ are defined by Eqs (3.2), (3.3) and (3.4) respectively.
In addition, if x(0)∈R(AT), the sequence {x(k)}∞k=1 converges to the least-norm solution of the system (1.1), i.e., limk→∞x(k)=x∗=A†b.
Proof. Under the MWRKO method, Lemma 2.2 still holds. For k=1, we have
‖x(1)−˜x‖2=‖x(0)−˜x‖2−‖x(1)−x(0)‖2=‖x(0)−˜x‖2−|bi1−⟨ai1,x(0)⟩|2M(i1)=‖x(0)−˜x‖2−|bi1−⟨ai1,x(0)⟩|2M(i1)⋅‖b−Ax(0)‖2m∑i=1|bi−⟨ai,x(0)⟩|2M(i)⋅M(i)≤‖x(0)−˜x‖2−‖A(˜x−x(0))‖2‖A‖2F(i0)≤‖x(0)−˜x‖2−λmin(ATA)‖A‖2F‖x(0)−˜x‖2=(1−λmin(ATA)‖A‖2F)‖x(0)−x∗‖2=ρ0‖x(0)−x∗‖2. | (3.11) |
For k=1, we have
‖x(2)−˜x‖2=‖x(1)−˜x‖2−‖x(2)−x(1)‖2=‖x(1)−˜x‖2−|bi2−⟨ai2,x(1)⟩|2‖wi1‖2(i1)≤|x(1)−˜x‖2−|bi2−⟨ai2,x(1)⟩|2Δ⋅M(i2)⋅‖b−Ax(1)‖2m∑i=1,i≠i1|bi−⟨ai,x(1)⟩|2M(i)⋅M(i)(i2)≤‖x(1)−˜x‖2−‖A(˜x−x(1))‖2Δ⋅γ1(i0)≤‖x(1)−˜x‖2−λmin(ATA)Δ⋅γ1‖x(1)−˜x‖2=(1−λmin(ATA)Δ⋅γ1)‖x(1)−˜x‖2=ρ1‖x(1)−˜x‖2, | (3.12) |
where the inequality (xi) can be obtained by using Eqs (3.9) and (2.8). For inequality (xii), using the row index selection rule of the MWRKO method, we get:
|bi2−⟨ai2,x(1)⟩|2Δ⋅M(i2)⋅‖b−Ax(1)‖2m∑i=1,i≠i1|bi−⟨ai,x(1)⟩|2M(i)⋅M(i)=maxi∈{1,2,⋯,m}|bi−⟨ai,x(1)⟩|2Δ⋅M(i)⋅‖b−Ax(1)‖2m∑i=1,i≠i1|bi−⟨ai,x(1)⟩|2M(i)⋅M(i)≥‖b−Ax(1)‖2Δ⋅m∑i=1,i≠i1M(i)≥‖b−Ax(1)‖2Δ⋅γ1. | (3.13) |
For k>1, we have
‖x(k+1)−˜x‖2=‖x(k)−˜x‖2−‖x(k+1)−x(k)‖2=‖x(k)−˜x‖2−|bik+1−⟨aik+1,x(k)⟩|2‖wik‖2(i3)≤‖x(k)−˜x‖2−|bik+1−⟨aik+1,x(k)⟩|2Δ⋅M(ik+1)⋅‖b−Ax(k)‖2m∑i=1,i≠ik,ik−1|bi−⟨ai,x(k)⟩|2M(i)⋅M(i)(i4)≤‖x(k)−˜x‖2−‖A(˜x−x(k))‖2Δ⋅γ2(i0)≤‖x(k)−˜x‖2−λmin(ATA)Δ⋅γ2‖x(k)−˜x‖2=(1−λmin(ATA)Δ⋅γ2)‖x(k)−˜x‖2=ρk‖x(k)−˜x‖2, | (3.14) |
where the inequality (xiii) can be obtained by using Eqs (3.9), (2.8) and (2.9). For the inequality (xiv), it can be easily obtained by using a derivation similar to Eq (3.13). In the inequalities (x) of the Eqs (3.11), (3.12) and (3.14), we have used the estimate
||Au||22≥λmin(ATA)||u||2, |
which holds true for any u∈Cn belonging to the column space of AT. According to the lemma 2.2, it holds.
From the Eqs (3.11), (3.12) and (3.14), the Eq (3.10) holds.
Remark 6. When multiple indicators ik+1 are met in Step 2 of Algorithm 2 in the iterative process, we randomly select any one of them.
Remark 7. In the MWRKO method, the reason of hik≠0 is similar to Remark 3.
Remark 8. Set ˜ρ0=1−λmin(ATA)‖A‖2F, ˜ρk=1−λmin(ATA)γ1(∀k>0), and the convergence of MWRK method in [22] meets:
||x(k)−x∗||2≤k−1Πs=0~ρs||x(0)−x∗||2. |
Obviously, ∀Δ∈(0,1], ρk<~ρk(∀k>1), ρ1≤~ρ1 and ρ0=~ρ0, so the convergence speed of MWRKO method is faster than MWRK method. Note that ˜ρk<˜ζk, ρk<ζk(∀k>0,∀Δ∈(0,1]), that is VMWRK<VMWRKO, VGRK<VGRKO, VGRK<VMWRK, VGRKO<VMWRKO, where V represents the convergence speed.
Remark 9. If B=AAT is calculated before iteration, the MWRK method costs 4m+2n flopping operations and the MWRKO method costs 6m+3n+4 flopping opeartions, where the residual r(k) is calculated according to Eq (2.2).
In this section, some numerical examples are provided to illustrate the effectiveness of the greedy randomized Kaczmarz (GRK) method, the greedy randomized Kaczmarz method with oblique projection (GRKO), the maximal weighted residual Kaczmarz method (MWRK) and the maximal weighted residual Kaczmarz method (MWRKO). All experiments are carried out using MATLAB (version R2019b) on a personal laptop with 1.60 GHz central processing unit (Intel(R) Core(TM) i5-10210U CPU), 8.00 GB memory and Windows operating system (64 bit Windows 10).
In our implementations, the right vector b=Ax∗ such that the exact solution x∗∈Rn is a vector generated by the rand function. Define the relative residual error (RRE) at the kth iteration as follows:
RRE=‖b−Ax(k)‖2‖b‖2. |
The initial point x(0)∈Rn is set to be a zero vector and the iterations are terminated once the relative solution error satisfies RRE<ω or the number of iteration steps exceeds 100,000. If the number of iteration steps exceeds 100,000, it is denoted as "-".
We will compare the numerical performance of these methods in terms of the number of iteration steps (denoted as "IT") and the computing time in seconds (denoted as "CPU"). Here the CPU and IT mean the arithmetical averages of the elapsed running times and the required iteration steps with respect to 50 trials repeated runs of the corresponding method.
The random matrix collection in [0,1] is randomly generated by using the MATLAB function rand and the numerical results are reported in Tables 1 and 2 and Figures 2 and 3. In this subsection, we let ω=0.5×10−8. According to the characteristics of the matrix generated by MATLAB function rand, Tables 1 and 2 are the experiments for the overdetermined consistent linear systems, underdetermined consistent linear systems respectively. Under the premise of convergence, all methods can find the unique least Euclidean norm solution.
m | IT | CPU | ||||||
GRK | GRKO | MWRK | MWRKO | GRK | GRKO | MWRK | MWRKO | |
1000 | 12,072 | 2105 | 11,265 | 1913 | 1.2824 | 0.2099 | 0.7192 | 0.1089 |
2000 | 4726 | 1088 | 4292 | 898 | 1.4792 | 0.3413 | 1.1107 | 0.2157 |
3000 | 3362 | 897 | 3234 | 771 | 1.7550 | 0.5172 | 1.5711 | 0.3575 |
4000 | 2663 | 859 | 2517 | 668 | 1.9415 | 0.6396 | 1.6634 | 0.4807 |
5000 | 2398 | 826 | 2282 | 605 | 2.4134 | 0.8160 | 2.1528 | 0.5801 |
6000 | 2100 | 772 | 2018 | 586 | 2.6235 | 0.8912 | 2.0975 | 0.6486 |
7000 | 1970 | 752 | 1829 | 562 | 2.6019 | 1.0720 | 2.5441 | 0.7822 |
8000 | 1861 | 747 | 1703 | 555 | 3.1035 | 1.2421 | 2.4987 | 0.8390 |
9000 | 1750 | 747 | 1612 | 530 | 3.0223 | 1.3055 | 2.6148 | 0.8730 |
m | IT | CPU | ||||||
GRK | GRKO | MWRK | MWRKO | GRK | GRKO | MWRK | MWRKO l | |
100 | 802 | 286 | 848 | 272 | 0.0496 | 0.0223 | 0.0258 | 0.0165 |
200 | 1968 | 523 | 1948 | 481 | 0.1648 | 0.0496 | 0.0831 | 0.0276 |
300 | 3104 | 759 | 3148 | 709 | 0.3982 | 0.1090 | 0.2404 | 0.0664 |
400 | 4586 | 1002 | 4612 | 930 | 1.0539 | 0.2594 | 0.8433 | 0.1920 |
500 | 6233 | 1250 | 6336 | 1215 | 1.9528 | 0.4409 | 1.6836 | 0.3576 |
600 | 8671 | 1576 | 8882 | 1497 | 3.6363 | 0.7493 | 3.1625 | 0.5957 |
700 | 11,895 | 2063 | 11,575 | 1879 | 5.8642 | 1.1078 | 5.0029 | 0.9087 |
800 | 14,758 | 2451 | 14,888 | 2394 | 8.4280 | 1.5350 | 7.7007 | 1.6405 |
900 | 18,223 | 3250 | 18,608 | 2945 | 12.0469 | 2.2750 | 10.9511 | 1.8884 |
From Table 1 and Figure 2, we can see that when the linear system is overdetermined, with the increase of m, the IT of all methods decreases, but the CPU shows an increasing trend. Our new methods—the GRKO method and the MWRKO method, perform better than the GRK method and the MWRK method respectively in both iteration steps and running time. Among the four methods, the MWRKO method performs best. From Table 2 and Figure 3, we can see that in the case of underdetermined linear system, with the increase of m, the IT and CPU of all methods decrease.
In this group of experiments, whether it is an overdetermined or underdetermined linear system, whether in terms of the IT or CPU, the GRKO method and the MWRKO method perform very well compared with the GRK method and the MWRK method. These experimental phenomena are consistent with the theoretical convergence conclusions we got.
In this subsection, the entries of our coefficient matrix are randomly generated in the interval [c,1]. This set of experiments was also done in [36] and [54] and pointed out that when the value of c is close to 1, the rows of matrix A are more linearly correlated. Theorems 3.1 and 3.2 have shown the effectiveness of the GRKO method and the MWRKO method in this case. In order to verify this phenomenon, we construct several 1000×500 and 500×1000 matrices A, which entries is independent identically distributed uniform random variables on some interval [c,1]. Note that there is nothing special about this interval and other intervals yield the same results when the interval length remains the same. In the experiment of this subsection, we take ω=0.5×10−8.
From Table 3 and Figure 4, it can be seen that when the linear system is overdetermined, with c getting closer to 1, the GRK method and the MWRK method have a significant increase in the number of iterations and running time. When c increases to 0.7, the GRK method and the MWRK method exceeds the maximum number of iterations. But the IT and CPU of the GRKO method and the MWRKO method have decreasing trends. From Figure 5, we can get that the numerical experiment of the coefficient matrix A in the underdetermined case has similar trends to the numerical experiment in the overdetermined case in Figure 4. In this group of experiments, it can be observed that when the rows of the matrix are more linearly correlated, the GRKO method and the MWRKO method can find the least Euclidean norm solution more quickly than the GRK method and the MWRK method.
c | IT | CPU | ||||||
GRK | GRKO | MWRK | MWRKO | GRK | GRKO | MWRK | MWRKO | |
0.1 | 14,757 | 2036 | 14,594 | 1830 | 1.5811 | 0.2180 | 0.9419 | 0.0969 |
0.2 | 21,103 | 1840 | 20,717 | 1714 | 2.1684 | 0.2287 | 1.1828 | 0.1003 |
0.3 | 27,375 | 1708 | 26,986 | 1569 | 3.5926 | 0.1789 | 1.5865 | 0.1195 |
0.4 | 36,293 | 1708 | 35,595 | 1394 | 3.6751 | 0.1802 | 2.0682 | 0.0885 |
0.5 | 53,485 | 1428 | 52,853 | 1310 | 5.3642 | 0.1486 | 3.0024 | 0.0847 |
0.6 | 84,204 | 1353 | 81,647 | 1185 | 9.0879 | 0.1388 | 4.5468 | 0.0767 |
0.7 | - | 1227 | - | 1036 | - | 0.1298 | - | 0.0564 |
0.8 | - | 1080 | - | 926 | - | 0.1107 | - | 0.0580 |
0.9 | - | 715 | - | 583 | - | 0.0707 | - | 0.0324 |
c | IT | CPU | ||||||
GRK | GRKO | MWRK | MWRKO | GRK | GRKO | MWRK | MWRKO | |
0.1 | 16,828 | 1968 | 16,913 | 1795 | 1.7612 | 0.2103 | 0.9353 | 0.1083 |
0.2 | 23,518 | 2003 | 23,234 | 1857 | 2.3037 | 0.2066 | 1.3119 | 0.1230 |
0.3 | 30,875 | 1661 | 31,017 | 1688 | 2.9310 | 0.1635 | 1.7373 | 0.0997 |
0.4 | 41,242 | 1511 | 40,986 | 1515 | 4.3004 | 0.1726 | 2.2899 | 0.1025 |
0.5 | 60,000 | 1399 | 59,750 | 1349 | 5.4754 | 0.1252 | 2.8920 | 0.0727 |
0.6 | 97,045 | 1270 | 95,969 | 1264 | 8.5229 | 0.1173 | 4.8380 | 0.0688 |
0.7 | - | 1082 | - | 1022 | - | 0.1168 | - | 0.0646 |
0.8 | - | 858 | - | 863 | - | 0.0960 | - | 0.0585 |
0.9 | - | 549 | - | 598 | - | 0.0582 | - | 0.0353 |
In this subsection, we will give three examples to illustrate the effectiveness of our new methods applied to sparse matrix. The coefficient matrices A of these three examples are the practical problems from [57] and the two test problems from [58].
Example 1. We solve the problem (1.1) with the coefficient matrix A∈Rm×n chosen from the University of Florida sparse matrix collection [57]. the matrices are divorce, photogrammetry, Ragusa18, Trec8, Stranke94 and well1033. In Table 5, we list some properties of these matrices, where density is defined as follows:
density=number of nonzeros of m-by-n matrixmn. |
A | divorce | photogrammetry | Ragusa18 | Trec8 | Stranke94 | well1033 |
m × n | 50 × 9 | 1388 × 390 | 23 × 23 | 23 × 84 | 10 × 10 | 1033 × 320 |
rank | 9 | 390 | 15 | 23 | 10 | 320 |
cond(A) | 19.3908 | 4.35e+8 | 3.48e+35 | 26.8949 | 51.7330 | 166.1333 |
density | 50.00% | 2.18% | 12.10% | 28.42% | 90.00% | 1.43% |
In this group of experiments, we set ω=0.5×10−5. In order to solve Example 1, we list the IT, CPU and historical convergence of the GRK, GRKO, MWRK and MWRKO methods in Figure 6 and Table 6, respectively. It can be seen that IT and CPU of the MWRKO method are the least. Although the GRKO method is not faster than the MWRK method for most of the experiments in Table 6, it is always faster than the GRK method.
A | IT | CPU | ||||||
GRK | GRKO | MWRK | MWRKO | GRK | GRKO | MWRK | MWRKO | |
divorce | 51 | 28 | 54 | 22 | 0.0053 | 0.0037 | 0.0017 | 0.0013 |
photogrammetry | 85,938 | 48,933 | 90,480 | 27,084 | 9.9917 | 8.0424 | 3.9809 | 2.5026 |
Ragusa18 | 744 | 262 | 727 | 280 | 0.0577 | 0.0270 | 0.0121 | 0.0098 |
Trec8 | 465 | 152 | 538 | 139 | 0.0382 | 0.0168 | 0.0111 | 0.0062 |
Stranke94 | 1513 | 197 | 1453 | 181 | 0.1291 | 0.0187 | 0.0208 | 0.0082 |
well1033 | 22,924 | 9825 | 25,250 | 8655 | 2.4278 | 1.5112 | 0.8491 | 0.5827 |
Example 2. We consider fancurvedtomo(N,θ,P) test problem from the MATLAB package AIR Tools [58], which generates sparse matrix A, an exact solution x∗ and b=Ax∗. We set N=60, θ=0:0.5:179.5∘, P=90, then resulting matrix is of size 32400×3600. We test RRE every 10 iterations and run these four methods until RRE <ω is satisfied, where ω=0.5×10−5.
We first remove the rows of A where the entries are all 0 and perform row unitization processing on A and b. We emphasize that this will not cause a change in x∗. In Figure 7, we give 60×60 images of the exact phantom and the approximate solutions obtained by the GRK, GRKO, MWRK, MWRKO methods. In Figure 7, we can see that the GRK, GRKO, MWRK and MWRKO method can all converge to the exact solution successfully. In the subgraph (f) of Figure 7, we can see that the MWRKO method needs the least iterative steps and the GRKO method has less iterative steps than the GRK method. It can be observed from Table 7 that the MWRKO method is the best in terms of IT and CPU.
method | IT | CPU |
GRK | 13,550 | 581.17 |
GRKO | 12,750 | 538.82 |
MWRK | 12,050 | 504.83 |
MWRKO | 10,790 | 452.86 |
Example 3. We use an example from 2D seismic travel-time tomography reconstruction, implemented in the function seismictomo(N,s,p) in the MATLAB package AIR Tools [58], which generates sparse matrix A, an exact solution x∗ and b=Ax∗. We set N=50, s=150, p=120, then resulting matrix is of size 18000×2500. We test RRE every 50 iterations and run these four methods until RRE <ω is satisfied, where ω=0.5×10−6.
We first remove the rows of A where the entries are all 0 and perform row unitization processing on A and b. In Figure 8, we give 50×50 images of the exact phantom and the approximate solutions obatined by the GRK, GRKO, MWRK, MWRKO methods. From the subgraph (f) of Figure 8 and Table 8, we can see that the MWRKO method is the best in terms of IT and CPU.
method | IT | CPU |
GRK | 21,500 | 378.6629 |
GRKO | 15,850 | 324.9338 |
MWRK | 19,250 | 334.3446 |
MWRKO | 14,400 | 262.1489 |
In this subsection, we compare the classical methods—Landweber method [59] and generalized minimum residual (GMRES) method [60] with our proposed methods—GRKO and MWRKO. The iterative expression of Landweber is:
x(k+1)=x(k)+ηAT(b−Ax(k)), |
where η is a real parameter satisfying 0<η<2λmax(ATA). For convenience, we take η=2‖A‖2F(<2λmax(ATA)) in the following numerical experiments. There are also other new improvements to the Landweber method. Interested readers can refer [61,62] and the references therein.
For the GMRES method, we use the matlab function gmres(A,b,n,RRE∗,n), where RRE∗=||b−Ax(k)||||b||=√RRE and n is the dimension of matrix A. In this group of experiments, we take the parameter ω=0.5×10−8 and sparse matrices A∈R600×600 are generated by the MATLAB function sprand(600,600,density,0.75) and then perform row unitization processing. Obviously, the biggest computational cost of the GRKOmethod and the MWRKO method is the update of the residualr. Therefore, we firstcalculate B=AAT and then calculate the residual r accordingto Eq (2.2), which can improve CPU to a certain extent. Note that the calculation time of B is included in the CPU of the GRKO method and the MWRKO method.
In Table 9, we compare IT and CPU of MWRKO, GRKO, Landweber and GMRES methods at different densities. Obviously as density increases, the CPU increases for all methods. Among them, the best CPU performance is still the MWRKO method, followed by the GMRES method.
density | IT | CPU | ||||||
MWRKO | GRKO | Landweber | GMRES | MWRKO | GRKO | Landweber | GMRES | |
0.15 | 1423 | 1432 | 3177 | 600 | 0.1285 | 0.2557 | 0.4897 | 0.2522 |
0.3 | 1501 | 1518 | 3236 | 600 | 0.1808 | 0.3484 | 1.0245 | 0.2839 |
0.45 | 1546 | 1557 | 3221 | 600 | 0.2299 | 0.3708 | 3.3660 | 0.3239 |
0.6 | 1573 | 1593 | 3245 | 600 | 0.2633 | 0.4202 | 4.5986 | 0.3423 |
0.75 | 1581 | 1599 | 3236 | 600 | 0.2782 | 0.4282 | 5.7157 | 0.3671 |
0.9 | 1632 | 1652 | 3231 | 600 | 0.3132 | 0.4734 | 6.9041 | 0.4021 |
Combined with the representative randomized and non-randomized row index selection strategies, two Kaczamrz-type methods with oblique projection for solving large-scale consistent linear systems are proposed, namely the GRKO method and the MWRKO method. The exponential convergences of the GRKO method and the MWRKO method are deduced. Theoretical and experimental results show that the convergence rates of the GRKO method and the MWRKO method are better than GRK method and MWRK method respectively. Numerical experiments show the effectiveness of these two methods, especially when the rows of the coefficient matrix A are highly linearly correlated.
This work was supported by the Fundamental Research Funds for the Central Universities [grant number 18CX02041A, 19CX05003A-2, 19CX02062A], the National Key Research and Development Program of China [grant number 2019YFC1408400] and the Science and Technology Support Plan for Youth Innovation of University in Shandong Province [No.YCX2021151].
The authors declare that there is no conflict of interests regarding the publication of this paper.
[1] |
B. Meher, S. Agrawal, R. Panda, A. Abraham, A survey on region based image fusion methods, Inf. Fusion, 48 (2019), 119–132. https://doi.org/10.1016/j.inffus.2018.07.010 doi: 10.1016/j.inffus.2018.07.010
![]() |
[2] |
S. Karim, G. Tong, J. Li, A. Qadir, U. Farooq, Y. Yu, Current advances and future perspectives of image fusion: A comprehensive review, Inf. Fusion, 90 (2023), 185–217. https://doi.org/10.1016/j.inffus.2022.09.019 doi: 10.1016/j.inffus.2022.09.019
![]() |
[3] |
K. Ma, Z. Duanmu, H. Yeganeh, Z. Wang, Multi-exposure image fusion by optimizing a structural similarity index, IEEE Trans. Comput. Imaging, 4 (2018), 60–72. https://doi.org/10.1109/TCI.2017.2786138 doi: 10.1109/TCI.2017.2786138
![]() |
[4] |
X. Zhang, Deep learning-based multi-focus image fusion: A survey and a comparative study, IEEE Trans. Pattern Anal. Mach. Intell., 44 (2022), 4819–4838. https://doi.org/10.1109/TPAMI.2021.3078906 doi: 10.1109/TPAMI.2021.3078906
![]() |
[5] |
J. Liu, X. Fan, J. Jiang, R. Liu, Z. Luo, Learning a deep multi-scale feature ensemble and an edge-attention guidance for image fusion, IEEE Trans. Circuits Syst. Video Technol., 32 (2022), 105–119. https://doi.org/10.1109/TCSVT.2021.3056725 doi: 10.1109/TCSVT.2021.3056725
![]() |
[6] |
H. Xu, J. Ma, J. Jiang, X. Guo, H. Ling, U2Fusion: A unified unsupervised image fusion network, IEEE Trans. Pattern Anal. Mach. Intell., 44 (2022), 502–518. https://doi.org/10.1109/TPAMI.2020.3012548 doi: 10.1109/TPAMI.2020.3012548
![]() |
[7] | Z. Zhao, S. Xu, C. Zhang, J. Liu, J. Zhang, P. Li, DIDFuse: Deep image decomposition for infrared and visible image fusion, in Proceedings of the Twenty-Ninth International Joint Conference on Artificial Intelligence, (2021), 970–976. http://doi.org/10.24963/ijcai.2020/135 |
[8] | W. G. C. Bandara, V. M. Patel, Hypertransformer: A textural and spectral feature fusion transformer for pansharpening, in Proceedings of the IEEE/CVF Conference on Computer Vision and Pattern Recognition (CVPR), (2022), 1767–1777. https://doi.org/10.1109/CVPR52688.2022.00181 |
[9] | S. Xu, J. Zhang, Z. Zhao, K. Sun, J. Liu, C. Zhang, Deep gradient projection networks for pan-sharpening, in Proceedings of the IEEE/CVF Conference on Computer Vision and Pattern Recognition (CVPR), (2021), 1366–1375. https://doi.org/10.1109/CVPR46437.2021.00142 |
[10] | Z. Zhao, J. Zhan, S. Xu, K. Sun, L. Huang, J. Liu, et al., FGF-GAN: A lightweight generative adversarial network for pansharpening via fast guided filter, in 2021 IEEE International Conference on Multimedia and Expo (ICME), (2021), 1–6. https://doi.org/10.1109/ICME51207.2021.9428272 |
[11] | D. Cheng, R. Liao, S. Fidler, R. Urtasun, DARNet: Deep active ray network for building segmentation, in Proceedings of the IEEE/CVF Conference on Computer Vision and Pattern Recognition (CVPR), (2019), 7431–7439. https://doi.org/10.1109/CVPR.2019.00761 |
[12] | H. Qin, M. Zhang, Y. Ding, A. Li, Z. Cai, Z. Liu, et al., BiBench: Benchmarking and analyzing network binarization, in Proceedings of the 40th International Conference on Machine Learning, (2023), 28351–28388. |
[13] | C. He, K. Li, Y. Zhang, Y. Zhang, Z. Guo, X. Li, et al., Strategic preys make acute predators: Enhancing camouflaged object detectors by generating camouflaged objects, preprint, arXiv: 2308.03166. |
[14] | C. He, K. Li, Y. Zhang, L. Tang, Y. Zhang, Z. Guo, et al., Camouflaged object detection with feature decomposition and edge reconstruction, in Proceedings of the IEEE/CVF Conference on Computer Vision and Pattern Recognition (CVPR), (2023), 22046–22055. https://doi.org/10.1109/CVPR52729.2023.02111 |
[15] | C. He, K. Li, Y. Zhang, G. Xu, L. Tang, Y. Zhang, et al., Weakly-supervised concealed object segmentation with sam-based pseudo labeling and multi-scale feature grouping, in Advances in Neural Information Processing Systems, 36 (2023). |
[16] | J. Wang, Z. Yin, P. Hu, A. Liu, R. Tao, H. Qin, et al., Defensive patches for robust recognition in the physical world, in Proceedings of the IEEE/CVF Conference on Computer Vision and Pattern Recognition (CVPR), (2022), 2456–2465. https://doi.org/10.1109/CVPR52688.2022.00249 |
[17] | H. Chung, J. Kim, M. T. Mccann, M. L. Klasky, J. C. Ye, Diffusion posterior sampling for general noisy inverse problems, preprint, arXiv: 2209.14687. |
[18] |
X. Deng, P. L. Dragotti, Deep convolutional neural network for multi-modal image restoration and fusion, IEEE Trans. Pattern Anal. Mach. Intell., 43 (2021), 3333–3348. https://doi.org/10.1109/TPAMI.2020.2984244 doi: 10.1109/TPAMI.2020.2984244
![]() |
[19] |
A. Ben Hamza, Y. He, H. Krim, A. Willsky, A multiscale approach to pixel-level image fusion, Integr. Comput.-Aided Eng., 12 (2005), 135–146. https://doi.org/10.3233/ICA-2005-12201 doi: 10.3233/ICA-2005-12201
![]() |
[20] | J. Liu, X. Fan, Z. Huang, G. Wu, R. Liu, W. Zhong, et al., Target-aware dual adversarial learning and a multi-scenario multi-modality benchmark to fuse infrared and visible for object detection, in Proceedings of the IEEE/CVF Conference on Computer Vision and Pattern Recognition (CVPR), (2022), 5802–5811. https://doi.org/10.1109/CVPR52688.2022.00571 |
[21] |
L. Tang, Y. Deng, Y. Ma, J. Huang, J. Ma, Superfusion: A versatile image registration and fusion network with semantic awareness, IEEE/CAA J. Autom. Sin., 9 (2022), 2121–2137. https://doi.org/10.1109/JAS.2022.106082 doi: 10.1109/JAS.2022.106082
![]() |
[22] |
H. Li, X. J. Wu, J. Kittler, RFN-Nest: An end-to-end residual fusion network for infrared and visible images, Inf. Fusion, 73 (2021), 72–86. https://doi.org/10.1016/j.inffus.2021.02.023 doi: 10.1016/j.inffus.2021.02.023
![]() |
[23] |
X. Li, X. Guo, P. Han, X. Wang, H. Li, T. Luo, Laplacian redecomposition for multimodal medical image fusion, IEEE Trans. Instrum. Meas., 69 (2020), 6880–6890. https://doi.org/10.1109/TIM.2020.2975405 doi: 10.1109/TIM.2020.2975405
![]() |
[24] | H. Li, X. J. Wu, J. Kittler, Infrared and visible image fusion using a deep learning framework, in 2018 24th International Conference on Pattern Recognition (ICPR), (2018), 2705–2710. https://doi.org/10.1109/ICPR.2018.8546006 |
[25] |
H. Li, X. J. Wu, DenseFuse: A fusion approach to infrared and visible images, IEEE Trans. Image Process., 28 (2019), 2614–2623. https://doi.org/10.1109/TIP.2018.2887342 doi: 10.1109/TIP.2018.2887342
![]() |
[26] |
S. Li, X. Kang, J. Hu, Image fusion with guided filtering, IEEE Trans. Image Process., 22 (2013), 2864–2875. https://doi.org/10.1109/TIP.2013.2244222 doi: 10.1109/TIP.2013.2244222
![]() |
[27] | M. Li, Y. Dong, X. Wang, Pixel level image fusion based the wavelet transform, in 2013 6th International Congress on Image and Signal Processing (CISP), 2 (2013), 995–999. https://doi.org/10.1109/CISP.2013.6745310 |
[28] |
Y. Liu, X. Chen, H. Peng, Z. Wang, Multi-focus image fusion with a deep convolutional neural network, Inf. Fusion, 36 (2017), 191–207. https://doi.org/10.1016/j.inffus.2016.12.001 doi: 10.1016/j.inffus.2016.12.001
![]() |
[29] |
J. Ma, H. Zhang, Z. Shao, P. Liang, H. Xu, GANMcC: A generative adversarial network with multiclassification constraints for infrared and visible image fusion, IEEE Trans. Instrum. Meas., 70 (2021), 1–14. https://doi.org/10.1109/TIM.2020.3038013 doi: 10.1109/TIM.2020.3038013
![]() |
[30] |
H. Li, X. J. Wu, T. Durrani, NestFuse: An infrared and visible image fusion architecture based on nest connection and spatial/channel attention models, IEEE Trans. Instrum. Meas., 69 (2020), 9645–9656. https://doi.org/10.1109/TIM.2020.3005230 doi: 10.1109/TIM.2020.3005230
![]() |
[31] |
S. Huang, Y. Yang, X. Jin, Y. Zhang, Q. Jiang, S. Yao, Multi-sensor image fusion using optimized support vector machine and multiscale weighted principal component analysis, Electronics, 9 (2020), 1531. https://doi.org/10.3390/electronics9091531 doi: 10.3390/electronics9091531
![]() |
[32] |
R. Liu, J. Liu, Z. Jiang, X. Fan, Z. Luo, A bilevel integrated model with data-driven layer ensemble for multi-modality image fusion, IEEE Trans. Image Process., 30 (2021), 1261–1274. https://doi.org/10.1109/TIP.2020.3043125 doi: 10.1109/TIP.2020.3043125
![]() |
[33] |
J. Ma, L. Tang, M. Xu, H. Zhang, G. Xiao, STDFusionNet: An infrared and visible image fusion network based on salient target detection, IEEE Trans. Instrum. Meas., 70 (2021), 1–13. https://doi.org/10.1109/TIM.2021.3075747 doi: 10.1109/TIM.2021.3075747
![]() |
[34] | D. Wang, J. Liu, X. Fan, R. Liu, Unsupervised misaligned infrared and visible image fusion via cross-modality image generation and registration, preprint, arXiv: 2205.11876. |
[35] | J. Wang, Z. Wei, T. Zhang, W. Zeng, Deeply-fused nets, preprint, arXiv: 1605.07716. |
[36] | C. Szegedy, W. Liu, Y. Jia, P. Sermanet, S. Reed, D. Anguelov, et al., Going deeper with convolutions, in Proceedings of the IEEE Conference on Computer Vision and Pattern Recognition (CVPR), (2015), 1–9. https://doi.org/10.1109/CVPR.2015.7298594 |
[37] | A. Vaswani, N. Shazeer, N. Parmar, J. Uszkoreit, L. Jones, A. N. Gomez, et al., Attention is all you need, preprint, arXiv: 1706.03762. |
[38] | A. Dosovitskiy, L. Beyer, A. Kolesnikov, D. Weissenborn, X. Zhai, T. Unterthiner, et al., An image is worth 16x16 words: Transformers for image recognition at scale, preprint, arXiv: 2010.11929. |
[39] | Z. Wu, Z. Liu, J. Lin, Y. Lin, S. Han, Lite transformer with long-short range attention, preprint, arXiv: 2004.11886. |
[40] | S. W. Zamir, A. Arora, S. Khan, M. Hayat, F. S. Khan, M. H. Yang, restormer: Efficient transformer for high-resolution image restoration, in Proceedings of the IEEE/CVF Conference on Computer Vision and Pattern Recognition (CVPR), (2022), 5728–5739. https://doi.org/10.1109/CVPR52688.2022.00564 |
[41] |
X. Lin, S. Sun, W. Huang, B. Sheng, P. Li, D. D. F. Feng, EAPT: Efficient attention pyramid transformer for image processing, IEEE Trans. Multimedia, 25 (2023), 50–61. https://doi.org/10.1109/TMM.2021.3120873 doi: 10.1109/TMM.2021.3120873
![]() |
[42] |
L. Tang, J. Yuan, J. Ma, Image fusion in the loop of high-level vision tasks: A semantic-aware real-time infrared and visible image fusion network, Inf. Fusion, 82 (2022), 28–42. https://doi.org/10.1016/j.inffus.2021.12.004 doi: 10.1016/j.inffus.2021.12.004
![]() |
[43] | Z. Zhao, H. Bai, J. Zhang, Y. Zhang, S. Xu, Z. Lin, et al., CDDFuse: Correlation-driven dual-branch feature decomposition for multi-modality image fusion, in Proceedings of the IEEE/CVF Conference on Computer Vision and Pattern Recognition (CVPR), (2023), 5906–5916. https://doi.org/10.1109/CVPR52729.2023.00572 |
[44] |
L. Tang, J. Yuan, H. Zhang, X. Jiang, J. Ma, PIAFusion: A progressive infrared and visible image fusion network based on illumination aware, Inf. Fusion, 83 (2022), 79–92. https://doi.org/10.1016/j.inffus.2022.03.007 doi: 10.1016/j.inffus.2022.03.007
![]() |
[45] |
A. Toet, M. A. Hogervorst, Progress in color night vision, Opt. Eng., 51 (2012), 010901. https://doi.org/10.1117/1.OE.51.1.010901 doi: 10.1117/1.OE.51.1.010901
![]() |
[46] |
H. Zhang, J. Ma, SDNet: A versatile squeeze-and-decomposition network for real-time image fusion, Int. J. Comput. Vision, (2021), 1–25. https://doi.org/10.1007/s11263-021-01501-8 doi: 10.1007/s11263-021-01501-8
![]() |
[47] | P. Liang, J. Jiang, X. Liu, J. Ma, Fusion from decomposition: A self-supervised decomposition approach for image fusion, in European Conference on Computer Vision (ECCV), (2022), 719–735. https://doi.org/10.1007/978-3-031-19797-0_41 |
[48] | Z. Huang, J. Liu, X. Fan, R. Liu, W. Zhong, Z. Luo, Reconet: Recurrent correction network for fast and efficient multi-modality image fusion, in European Conference on Computer Vision, Springer, (2022), 539–555. https://doi.org/10.1007/978-3-031-19797-0_31 |
[49] |
W. Tan, H. Zhou, J. Song, H. Li, Y. Yu, J. Du, Infrared and visible image perceptive fusion through multi-level gaussian curvature filtering image decomposition, Appl. Opt., 58 (2019), 3064–3073. https://doi.org/10.1364/AO.58.003064 doi: 10.1364/AO.58.003064
![]() |
[50] |
W. Tang, F. He, Y. Liu, Y. Duan, T. Si, DATFuse: Infrared and visible image fusion via dual attention transformer, IEEE Trans. Circuits Syst. Video Technol., 33 (2023), 3159–3172. https://doi.org/10.1109/TCSVT.2023.3234340 doi: 10.1109/TCSVT.2023.3234340
![]() |
[51] | W. Wang, U. Neumann, Depth-aware CNN for RGB-D segmentation, in Proceedings of the European Conference on Computer Vision (ECCV), (2018), 135–150. https://doi.org/10.1007/978-3-030-01252-6_9 |
[52] | D. Lin, G. Chen, D. Cohen-Or, P. A. Heng, H. Huang, Cascaded feature network for semantic segmentation of RGB-D images, in Proceedings of the IEEE International Conference on Computer Vision (ICCV), (2017), 1311–1319. https://doi.org/10.1109/ICCV.2017.147 |
[53] |
G. Qi, Y. Zhang, K. Wang, N. Mazur, Y. Liu, D. Malaviya, Small object detection method based on adaptive spatial parallel convolution and fast multi-scale fusion, Remote Sens., 14 (2022), 420. https://doi.org/10.3390/rs14020420 doi: 10.3390/rs14020420
![]() |
[54] |
Z. Zhu, H. Yin, Y. Chai, Y. Li, G. Qi, A novel multi-modality image fusion method based on image decomposition and sparse representation, Inf. Sci., 432 (2018), 516–529. https://doi.org/10.1016/j.ins.2017.09.010 doi: 10.1016/j.ins.2017.09.010
![]() |
[55] |
K. Wang, M. Zheng, H. Wei, G. Qi, Y. Li, Multi-modality medical image fusion using convolutional neural network and contrast pyramid, Sensors, 20 (2020), 2169. https://doi.org/10.3390/s20082169 doi: 10.3390/s20082169
![]() |
[56] |
C. Wang, C. Wang, W. Li, H. Wang, A brief survey on RGB-D semantic segmentation using deep learning, Displays, 70 (2021), 102080. https://doi.org/10.1016/j.displa.2021.102080 doi: 10.1016/j.displa.2021.102080
![]() |
[57] | Y. Chen, L. Wan, Z. Li, Q. Jing, Z. Sun, Neural feature search for RGB-infrared person re-identification, in Proceedings of the IEEE/CVF Conference on Computer Vision and Pattern Recognition (CVPR), (2021), 587–597. https://doi.org/10.1109/CVPR46437.2021.00065 |
[58] |
C. Wang, X. Ning, W. Li, X. Bai, X. Gao, 3D person re-identification based on global semantic guidance and local feature aggregation, IEEE Trans. Circuits Syst. Video Technol., 34 (2024), 4698–4712. https://doi.org/10.1109/TCSVT.2023.3328712 doi: 10.1109/TCSVT.2023.3328712
![]() |
1. | Andreas Frommer, Daniel B. Szyld, On the convergence of randomized and greedy relaxation schemes for solving nonsingular linear systems of equations, 2023, 92, 1017-1398, 639, 10.1007/s11075-022-01431-7 | |
2. | Yansheng Su, Deren Han, Yun Zeng, Jiaxin Xie, On greedy multi-step inertial randomized Kaczmarz method for solving linear systems, 2024, 61, 0008-0624, 10.1007/s10092-024-00621-0 |
m | IT | CPU | ||||||
GRK | GRKO | MWRK | MWRKO | GRK | GRKO | MWRK | MWRKO | |
1000 | 12,072 | 2105 | 11,265 | 1913 | 1.2824 | 0.2099 | 0.7192 | 0.1089 |
2000 | 4726 | 1088 | 4292 | 898 | 1.4792 | 0.3413 | 1.1107 | 0.2157 |
3000 | 3362 | 897 | 3234 | 771 | 1.7550 | 0.5172 | 1.5711 | 0.3575 |
4000 | 2663 | 859 | 2517 | 668 | 1.9415 | 0.6396 | 1.6634 | 0.4807 |
5000 | 2398 | 826 | 2282 | 605 | 2.4134 | 0.8160 | 2.1528 | 0.5801 |
6000 | 2100 | 772 | 2018 | 586 | 2.6235 | 0.8912 | 2.0975 | 0.6486 |
7000 | 1970 | 752 | 1829 | 562 | 2.6019 | 1.0720 | 2.5441 | 0.7822 |
8000 | 1861 | 747 | 1703 | 555 | 3.1035 | 1.2421 | 2.4987 | 0.8390 |
9000 | 1750 | 747 | 1612 | 530 | 3.0223 | 1.3055 | 2.6148 | 0.8730 |
m | IT | CPU | ||||||
GRK | GRKO | MWRK | MWRKO | GRK | GRKO | MWRK | MWRKO l | |
100 | 802 | 286 | 848 | 272 | 0.0496 | 0.0223 | 0.0258 | 0.0165 |
200 | 1968 | 523 | 1948 | 481 | 0.1648 | 0.0496 | 0.0831 | 0.0276 |
300 | 3104 | 759 | 3148 | 709 | 0.3982 | 0.1090 | 0.2404 | 0.0664 |
400 | 4586 | 1002 | 4612 | 930 | 1.0539 | 0.2594 | 0.8433 | 0.1920 |
500 | 6233 | 1250 | 6336 | 1215 | 1.9528 | 0.4409 | 1.6836 | 0.3576 |
600 | 8671 | 1576 | 8882 | 1497 | 3.6363 | 0.7493 | 3.1625 | 0.5957 |
700 | 11,895 | 2063 | 11,575 | 1879 | 5.8642 | 1.1078 | 5.0029 | 0.9087 |
800 | 14,758 | 2451 | 14,888 | 2394 | 8.4280 | 1.5350 | 7.7007 | 1.6405 |
900 | 18,223 | 3250 | 18,608 | 2945 | 12.0469 | 2.2750 | 10.9511 | 1.8884 |
c | IT | CPU | ||||||
GRK | GRKO | MWRK | MWRKO | GRK | GRKO | MWRK | MWRKO | |
0.1 | 14,757 | 2036 | 14,594 | 1830 | 1.5811 | 0.2180 | 0.9419 | 0.0969 |
0.2 | 21,103 | 1840 | 20,717 | 1714 | 2.1684 | 0.2287 | 1.1828 | 0.1003 |
0.3 | 27,375 | 1708 | 26,986 | 1569 | 3.5926 | 0.1789 | 1.5865 | 0.1195 |
0.4 | 36,293 | 1708 | 35,595 | 1394 | 3.6751 | 0.1802 | 2.0682 | 0.0885 |
0.5 | 53,485 | 1428 | 52,853 | 1310 | 5.3642 | 0.1486 | 3.0024 | 0.0847 |
0.6 | 84,204 | 1353 | 81,647 | 1185 | 9.0879 | 0.1388 | 4.5468 | 0.0767 |
0.7 | - | 1227 | - | 1036 | - | 0.1298 | - | 0.0564 |
0.8 | - | 1080 | - | 926 | - | 0.1107 | - | 0.0580 |
0.9 | - | 715 | - | 583 | - | 0.0707 | - | 0.0324 |
c | IT | CPU | ||||||
GRK | GRKO | MWRK | MWRKO | GRK | GRKO | MWRK | MWRKO | |
0.1 | 16,828 | 1968 | 16,913 | 1795 | 1.7612 | 0.2103 | 0.9353 | 0.1083 |
0.2 | 23,518 | 2003 | 23,234 | 1857 | 2.3037 | 0.2066 | 1.3119 | 0.1230 |
0.3 | 30,875 | 1661 | 31,017 | 1688 | 2.9310 | 0.1635 | 1.7373 | 0.0997 |
0.4 | 41,242 | 1511 | 40,986 | 1515 | 4.3004 | 0.1726 | 2.2899 | 0.1025 |
0.5 | 60,000 | 1399 | 59,750 | 1349 | 5.4754 | 0.1252 | 2.8920 | 0.0727 |
0.6 | 97,045 | 1270 | 95,969 | 1264 | 8.5229 | 0.1173 | 4.8380 | 0.0688 |
0.7 | - | 1082 | - | 1022 | - | 0.1168 | - | 0.0646 |
0.8 | - | 858 | - | 863 | - | 0.0960 | - | 0.0585 |
0.9 | - | 549 | - | 598 | - | 0.0582 | - | 0.0353 |
A | divorce | photogrammetry | Ragusa18 | Trec8 | Stranke94 | well1033 |
m × n | 50 × 9 | 1388 × 390 | 23 × 23 | 23 × 84 | 10 × 10 | 1033 × 320 |
rank | 9 | 390 | 15 | 23 | 10 | 320 |
cond(A) | 19.3908 | 4.35e+8 | 3.48e+35 | 26.8949 | 51.7330 | 166.1333 |
density | 50.00% | 2.18% | 12.10% | 28.42% | 90.00% | 1.43% |
A | IT | CPU | ||||||
GRK | GRKO | MWRK | MWRKO | GRK | GRKO | MWRK | MWRKO | |
divorce | 51 | 28 | 54 | 22 | 0.0053 | 0.0037 | 0.0017 | 0.0013 |
photogrammetry | 85,938 | 48,933 | 90,480 | 27,084 | 9.9917 | 8.0424 | 3.9809 | 2.5026 |
Ragusa18 | 744 | 262 | 727 | 280 | 0.0577 | 0.0270 | 0.0121 | 0.0098 |
Trec8 | 465 | 152 | 538 | 139 | 0.0382 | 0.0168 | 0.0111 | 0.0062 |
Stranke94 | 1513 | 197 | 1453 | 181 | 0.1291 | 0.0187 | 0.0208 | 0.0082 |
well1033 | 22,924 | 9825 | 25,250 | 8655 | 2.4278 | 1.5112 | 0.8491 | 0.5827 |
method | IT | CPU |
GRK | 13,550 | 581.17 |
GRKO | 12,750 | 538.82 |
MWRK | 12,050 | 504.83 |
MWRKO | 10,790 | 452.86 |
method | IT | CPU |
GRK | 21,500 | 378.6629 |
GRKO | 15,850 | 324.9338 |
MWRK | 19,250 | 334.3446 |
MWRKO | 14,400 | 262.1489 |
density | IT | CPU | ||||||
MWRKO | GRKO | Landweber | GMRES | MWRKO | GRKO | Landweber | GMRES | |
0.15 | 1423 | 1432 | 3177 | 600 | 0.1285 | 0.2557 | 0.4897 | 0.2522 |
0.3 | 1501 | 1518 | 3236 | 600 | 0.1808 | 0.3484 | 1.0245 | 0.2839 |
0.45 | 1546 | 1557 | 3221 | 600 | 0.2299 | 0.3708 | 3.3660 | 0.3239 |
0.6 | 1573 | 1593 | 3245 | 600 | 0.2633 | 0.4202 | 4.5986 | 0.3423 |
0.75 | 1581 | 1599 | 3236 | 600 | 0.2782 | 0.4282 | 5.7157 | 0.3671 |
0.9 | 1632 | 1652 | 3231 | 600 | 0.3132 | 0.4734 | 6.9041 | 0.4021 |
m | IT | CPU | ||||||
GRK | GRKO | MWRK | MWRKO | GRK | GRKO | MWRK | MWRKO | |
1000 | 12,072 | 2105 | 11,265 | 1913 | 1.2824 | 0.2099 | 0.7192 | 0.1089 |
2000 | 4726 | 1088 | 4292 | 898 | 1.4792 | 0.3413 | 1.1107 | 0.2157 |
3000 | 3362 | 897 | 3234 | 771 | 1.7550 | 0.5172 | 1.5711 | 0.3575 |
4000 | 2663 | 859 | 2517 | 668 | 1.9415 | 0.6396 | 1.6634 | 0.4807 |
5000 | 2398 | 826 | 2282 | 605 | 2.4134 | 0.8160 | 2.1528 | 0.5801 |
6000 | 2100 | 772 | 2018 | 586 | 2.6235 | 0.8912 | 2.0975 | 0.6486 |
7000 | 1970 | 752 | 1829 | 562 | 2.6019 | 1.0720 | 2.5441 | 0.7822 |
8000 | 1861 | 747 | 1703 | 555 | 3.1035 | 1.2421 | 2.4987 | 0.8390 |
9000 | 1750 | 747 | 1612 | 530 | 3.0223 | 1.3055 | 2.6148 | 0.8730 |
m | IT | CPU | ||||||
GRK | GRKO | MWRK | MWRKO | GRK | GRKO | MWRK | MWRKO l | |
100 | 802 | 286 | 848 | 272 | 0.0496 | 0.0223 | 0.0258 | 0.0165 |
200 | 1968 | 523 | 1948 | 481 | 0.1648 | 0.0496 | 0.0831 | 0.0276 |
300 | 3104 | 759 | 3148 | 709 | 0.3982 | 0.1090 | 0.2404 | 0.0664 |
400 | 4586 | 1002 | 4612 | 930 | 1.0539 | 0.2594 | 0.8433 | 0.1920 |
500 | 6233 | 1250 | 6336 | 1215 | 1.9528 | 0.4409 | 1.6836 | 0.3576 |
600 | 8671 | 1576 | 8882 | 1497 | 3.6363 | 0.7493 | 3.1625 | 0.5957 |
700 | 11,895 | 2063 | 11,575 | 1879 | 5.8642 | 1.1078 | 5.0029 | 0.9087 |
800 | 14,758 | 2451 | 14,888 | 2394 | 8.4280 | 1.5350 | 7.7007 | 1.6405 |
900 | 18,223 | 3250 | 18,608 | 2945 | 12.0469 | 2.2750 | 10.9511 | 1.8884 |
c | IT | CPU | ||||||
GRK | GRKO | MWRK | MWRKO | GRK | GRKO | MWRK | MWRKO | |
0.1 | 14,757 | 2036 | 14,594 | 1830 | 1.5811 | 0.2180 | 0.9419 | 0.0969 |
0.2 | 21,103 | 1840 | 20,717 | 1714 | 2.1684 | 0.2287 | 1.1828 | 0.1003 |
0.3 | 27,375 | 1708 | 26,986 | 1569 | 3.5926 | 0.1789 | 1.5865 | 0.1195 |
0.4 | 36,293 | 1708 | 35,595 | 1394 | 3.6751 | 0.1802 | 2.0682 | 0.0885 |
0.5 | 53,485 | 1428 | 52,853 | 1310 | 5.3642 | 0.1486 | 3.0024 | 0.0847 |
0.6 | 84,204 | 1353 | 81,647 | 1185 | 9.0879 | 0.1388 | 4.5468 | 0.0767 |
0.7 | - | 1227 | - | 1036 | - | 0.1298 | - | 0.0564 |
0.8 | - | 1080 | - | 926 | - | 0.1107 | - | 0.0580 |
0.9 | - | 715 | - | 583 | - | 0.0707 | - | 0.0324 |
c | IT | CPU | ||||||
GRK | GRKO | MWRK | MWRKO | GRK | GRKO | MWRK | MWRKO | |
0.1 | 16,828 | 1968 | 16,913 | 1795 | 1.7612 | 0.2103 | 0.9353 | 0.1083 |
0.2 | 23,518 | 2003 | 23,234 | 1857 | 2.3037 | 0.2066 | 1.3119 | 0.1230 |
0.3 | 30,875 | 1661 | 31,017 | 1688 | 2.9310 | 0.1635 | 1.7373 | 0.0997 |
0.4 | 41,242 | 1511 | 40,986 | 1515 | 4.3004 | 0.1726 | 2.2899 | 0.1025 |
0.5 | 60,000 | 1399 | 59,750 | 1349 | 5.4754 | 0.1252 | 2.8920 | 0.0727 |
0.6 | 97,045 | 1270 | 95,969 | 1264 | 8.5229 | 0.1173 | 4.8380 | 0.0688 |
0.7 | - | 1082 | - | 1022 | - | 0.1168 | - | 0.0646 |
0.8 | - | 858 | - | 863 | - | 0.0960 | - | 0.0585 |
0.9 | - | 549 | - | 598 | - | 0.0582 | - | 0.0353 |
A | divorce | photogrammetry | Ragusa18 | Trec8 | Stranke94 | well1033 |
m × n | 50 × 9 | 1388 × 390 | 23 × 23 | 23 × 84 | 10 × 10 | 1033 × 320 |
rank | 9 | 390 | 15 | 23 | 10 | 320 |
cond(A) | 19.3908 | 4.35e+8 | 3.48e+35 | 26.8949 | 51.7330 | 166.1333 |
density | 50.00% | 2.18% | 12.10% | 28.42% | 90.00% | 1.43% |
A | IT | CPU | ||||||
GRK | GRKO | MWRK | MWRKO | GRK | GRKO | MWRK | MWRKO | |
divorce | 51 | 28 | 54 | 22 | 0.0053 | 0.0037 | 0.0017 | 0.0013 |
photogrammetry | 85,938 | 48,933 | 90,480 | 27,084 | 9.9917 | 8.0424 | 3.9809 | 2.5026 |
Ragusa18 | 744 | 262 | 727 | 280 | 0.0577 | 0.0270 | 0.0121 | 0.0098 |
Trec8 | 465 | 152 | 538 | 139 | 0.0382 | 0.0168 | 0.0111 | 0.0062 |
Stranke94 | 1513 | 197 | 1453 | 181 | 0.1291 | 0.0187 | 0.0208 | 0.0082 |
well1033 | 22,924 | 9825 | 25,250 | 8655 | 2.4278 | 1.5112 | 0.8491 | 0.5827 |
method | IT | CPU |
GRK | 13,550 | 581.17 |
GRKO | 12,750 | 538.82 |
MWRK | 12,050 | 504.83 |
MWRKO | 10,790 | 452.86 |
method | IT | CPU |
GRK | 21,500 | 378.6629 |
GRKO | 15,850 | 324.9338 |
MWRK | 19,250 | 334.3446 |
MWRKO | 14,400 | 262.1489 |
density | IT | CPU | ||||||
MWRKO | GRKO | Landweber | GMRES | MWRKO | GRKO | Landweber | GMRES | |
0.15 | 1423 | 1432 | 3177 | 600 | 0.1285 | 0.2557 | 0.4897 | 0.2522 |
0.3 | 1501 | 1518 | 3236 | 600 | 0.1808 | 0.3484 | 1.0245 | 0.2839 |
0.45 | 1546 | 1557 | 3221 | 600 | 0.2299 | 0.3708 | 3.3660 | 0.3239 |
0.6 | 1573 | 1593 | 3245 | 600 | 0.2633 | 0.4202 | 4.5986 | 0.3423 |
0.75 | 1581 | 1599 | 3236 | 600 | 0.2782 | 0.4282 | 5.7157 | 0.3671 |
0.9 | 1632 | 1652 | 3231 | 600 | 0.3132 | 0.4734 | 6.9041 | 0.4021 |