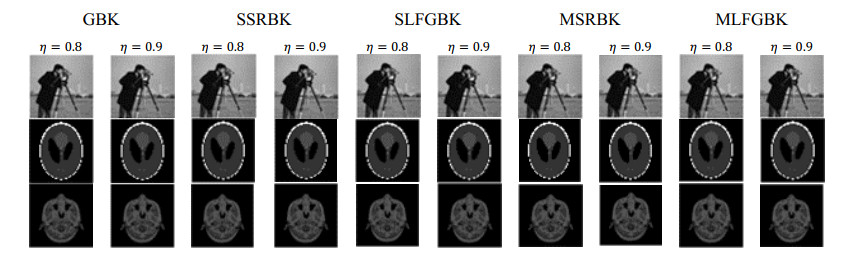
The issue of non-fragile sampled-data control for synchronizing Markov jump Lur'e systems (MJLSs) with time-variant delay was investigated. The time-variant delay allowed for uncertainty that was constrained to an interval with defined upper and lower boundaries. The components of the nonlinear function within the MJLSs were considered to satisfy either Lipschitz continuity or non-decreasing monotonicity. Numerically tractable conditions that ensured stochastic synchronization with a predefined L2−L∞ disturbance attenuation level for the drive-response MJLSs were established, utilizing time-dependent two-sided loop Lyapunov-Krasovskii functionals, together with integral and matrix inequalities. An exact mathematical expression of the desired controller gains can be obtained based on these conditions. Finally, an example with numerical simulation was employed to demonstrate the effectiveness of the proposed control strategies.
Citation: Dandan Zuo, Wansheng Wang, Lulu Zhang, Jing Han, Ling Chen. Non-fragile sampled-data control for synchronizing Markov jump Lur'e systems with time-variant delay[J]. Electronic Research Archive, 2024, 32(7): 4632-4658. doi: 10.3934/era.2024211
[1] | Ravi Agarwal, Snezhana Hristova, Donal O'Regan . Integral presentations of the solution of a boundary value problem for impulsive fractional integro-differential equations with Riemann-Liouville derivatives. AIMS Mathematics, 2022, 7(2): 2973-2988. doi: 10.3934/math.2022164 |
[2] | Hasanen A. Hammad, Hassen Aydi, Manuel De la Sen . The existence and stability results of multi-order boundary value problems involving Riemann-Liouville fractional operators. AIMS Mathematics, 2023, 8(5): 11325-11349. doi: 10.3934/math.2023574 |
[3] | Ymnah Alruwaily, Lamya Almaghamsi, Kulandhaivel Karthikeyan, El-sayed El-hady . Existence and uniqueness for a coupled system of fractional equations involving Riemann-Liouville and Caputo derivatives with coupled Riemann-Stieltjes integro-multipoint boundary conditions. AIMS Mathematics, 2023, 8(5): 10067-10094. doi: 10.3934/math.2023510 |
[4] | Md. Asaduzzaman, Md. Zulfikar Ali . Existence of positive solution to the boundary value problems for coupled system of nonlinear fractional differential equations. AIMS Mathematics, 2019, 4(3): 880-895. doi: 10.3934/math.2019.3.880 |
[5] | Khalid K. Ali, K. R. Raslan, Amira Abd-Elall Ibrahim, Mohamed S. Mohamed . On study the fractional Caputo-Fabrizio integro differential equation including the fractional q-integral of the Riemann-Liouville type. AIMS Mathematics, 2023, 8(8): 18206-18222. doi: 10.3934/math.2023925 |
[6] | Veliappan Vijayaraj, Chokkalingam Ravichandran, Thongchai Botmart, Kottakkaran Sooppy Nisar, Kasthurisamy Jothimani . Existence and data dependence results for neutral fractional order integro-differential equations. AIMS Mathematics, 2023, 8(1): 1055-1071. doi: 10.3934/math.2023052 |
[7] | Ugyen Samdrup Tshering, Ekkarath Thailert, Sotiris K. Ntouyas . Existence and stability results for a coupled system of Hilfer-Hadamard sequential fractional differential equations with multi-point fractional integral boundary conditions. AIMS Mathematics, 2024, 9(9): 25849-25878. doi: 10.3934/math.20241263 |
[8] | Thabet Abdeljawad, Sabri T. M. Thabet, Imed Kedim, Miguel Vivas-Cortez . On a new structure of multi-term Hilfer fractional impulsive neutral Levin-Nohel integrodifferential system with variable time delay. AIMS Mathematics, 2024, 9(3): 7372-7395. doi: 10.3934/math.2024357 |
[9] | Pinghua Yang, Caixia Yang . The new general solution for a class of fractional-order impulsive differential equations involving the Riemann-Liouville type Hadamard fractional derivative. AIMS Mathematics, 2023, 8(5): 11837-11850. doi: 10.3934/math.2023599 |
[10] | Bashir Ahmad, Ahmed Alsaedi, Sotiris K. Ntouyas . Nonlinear Langevin equations and inclusions involving mixed fractional order derivatives and variable coefficient with fractional nonlocal-terminal conditions. AIMS Mathematics, 2019, 4(3): 626-647. doi: 10.3934/math.2019.3.626 |
The issue of non-fragile sampled-data control for synchronizing Markov jump Lur'e systems (MJLSs) with time-variant delay was investigated. The time-variant delay allowed for uncertainty that was constrained to an interval with defined upper and lower boundaries. The components of the nonlinear function within the MJLSs were considered to satisfy either Lipschitz continuity or non-decreasing monotonicity. Numerically tractable conditions that ensured stochastic synchronization with a predefined L2−L∞ disturbance attenuation level for the drive-response MJLSs were established, utilizing time-dependent two-sided loop Lyapunov-Krasovskii functionals, together with integral and matrix inequalities. An exact mathematical expression of the desired controller gains can be obtained based on these conditions. Finally, an example with numerical simulation was employed to demonstrate the effectiveness of the proposed control strategies.
In the modern fields of scientific research and medical diagnostics [1,2,3], there is an increasing reliance on image restoration techniques [4,5,6,7], which are particularly prominent in the field of medical imaging. Ensuring image quality is paramount for the authenticity of data [8]. By processing X-ray projection data obtained from CT scans, we can reconstruct clear tomographic images with a resolution of n×n pixels. This technology encompasses two main schools: mathematical theoretical analysis [9] and iterative methods [10]. The former, like the filtered back projection method [11], is widely used in fields such as CT imaging, while the latter excels in dealing with noise interference and data loss. With technological advancements, image restoration is moving toward greater precision and efficiency.
To reconstruct images, we must solve a large-scale system of linear equations with multiple righthand sides, which is presented as follows:
AX=B | (1) |
In contemporary mathematics and engineering, solving systems of equations stands as a pivotal task, particularly in scientific research and industrial applications where efficiency and precision are of paramount importance. A variety of numerical methods have been widely adopted [12,13,14,15], among which the Kaczmarz algorithm [16] is renowned for its iterative projection approach in approximating the true solution. The algorithm's simplicity has facilitated its application across various domains, including image reconstruction [12,17], medical imaging [11,18], and signal processing [19,20]. Advances in technology have given rise to multiple enhanced versions of the Kaczmarz algorithm [21,22,23,24,25,26,27,28,29,30,31], improving performance in large-scale parallel computing and noisy data environments. Notably, with the development of free pseudo-inverse techniques, Du and Sun [24] further extended the randomized extended average block Kaczmar (REABK) method, proposing a class of pseudo-inverse-free stochastic block iterative methods for solving both consistent and inconsistent systems of linear equations, Free pseudo-inverse can accelerate convergence speed. Pseudo-inverse approximation is a specific case of generalized inverse techniques. For more theoretical analysis and applications, refer to literature [32]. Inspired by references [33,34], this paper introduces a faster lazy free greedy block Kaczmarz (LFGBK) method, exploring matrix sketching techniques as a key tool for accelerating matrix operations. This method employs sampling based on an approximate maximum distance criterion, excelling at selecting small, representative samples from large datasets for more efficient computation. The adaptive randomized block Kaczmarz (ARBK) [35] algorithm integrates adaptive and randomized block selection strategies. However, under certain specific matrix structures, ARBK may experience slow convergence or even fail to converge. Additionally, when dealing with large-scale sparse matrices, the ARBK algorithm incurs significant memory overhead. Our improved algorithm successfully overcomes these drawbacks, offering a more stable and efficient solution.
Additionally, it not only addresses single righthand side linear equations but also extends to multiple righthand side linear equations, solving the memory overflow issues that traditional algorithms face when dealing with image processing vectors. A comprehensive theoretical framework supports the convergence of these methods. Numerical experiments validate its effectiveness, demonstrating improved computational efficiency and laying a solid foundation for further optimization of matrix sketching techniques.
The structure of this paper is as follows: Section 2 introduces the necessary background knowledge. Section 3 presents the faster free pseudo-inverse GBK method for solving single righthand side linear equation systems. Section 4 proposes the leverage score sampling free pseudo-inverse GBK method for solving multiple righthand side linear equation systems. Section 5 details the numerical experiments, and Section 6 concludes the paper.
In this article, we adopt the same notation as in reference [27]. For example, A(i),AT,A†,||A||,||A||F, and [n], respectively, represent the i-th row of the coefficient matrix A, transpose, generalized inverse, spectral norm, F-norm, and the set {1,2,…,n}.
Recently, Niu and Zheng combined greedy strategy with the block Kaczmarz method, proposing the GBK [29] method for solving large-scale consistent linear equation systems. See Algorithm 1 for the specific process.
Algorithm1 GBK method |
1: Input: A,b,l,x0∈range(AT)andη∈(0,1). 2: Output: xl. 3: for k=0,1,…l−1 do 4: Compute εk=ηmax1≤i≤m{|bi−A(i)xk|2||A(i)||22}. 5: Determine the sequence of indicator sets. Tk={ik:|bik−A(ik)xk|2≥εk||A(ik)||22}. 6: Compute xk+1=xk+A†Tk(bTk−ATkxk). 7: end for |
Convergence analysis of the GBK method is described as follows:
Theorem 1 ([29]) If the linear system of Eq (1) is consistent, then the iterative sequence {xk}∞k=0 generated by algorithm 1 converges to the minimum norm solution x∗=A†b of the system of equations, and satisfies for any k≥0,
||xk+1−x∗||22≤(1−γk(η)σ2min(A)||A||2F)k+1||x0−x∗||22. |
The formula for γk(η) is defined as follows: γk(η)=η||A||2F||A||2F−||ATk−1||2F||ATk||2Fσ2max(ATk). Here, γ0(η) is defined as η||AT0||2Fσ2max(AT0), where η is in the range (0,1], and σmin(A) and σmax(A) represent the nonzero minimum singular value and maximum singular value of matrix A.
In the matrix sketching technique, as described in leverage score sampling [36,37], we select samples based on the leverage score of each row. Specifically, we will choose each row with a probability proportional to its leverage score. Therefore, rows with higher scores (i.e., rows with greater influence in the dataset) will have a greater chance of being selected.
Algorithm2 Leverage score sampling method based on manifold |
1: Input:A∈Rm×n. 2: Initialize C as a d×n zero matrix. 3: Initialize the variable sum to 0. 4: Calculate the singular value decomposition of A as U, S, and V. 5: for k=1,…,m 6: Calculate the sum of the squares of each row in U and add it to the sum. 7: Calculate the probability of each data point being sampled: Calculate the sum of squares for each row of U, then divide by the total sum. 8: Based on the calculated probabilities, sampling is conducted to obtain the sampling indices. 9: Utilize C=[indices,:] for indexing. 10: end for 11: Return C∈Rd×n. |
The advantage of this sampling method lies in its ability to select a small, representative subset of samples from a large dataset, enabling more efficient computation. The resulting sample set S∈Rd×m, where d is the chosen number of samples, can be utilized to estimate various properties of the original matrix A, such as singular value decomposition, principal component analysis, and so on. The LFGBK algorithm is similar to the derivation process in reference [38].
Remark: In addition to the method proposed in this paper, there are other sampling techniques such as random Gaussian matrices, subsampled randomized Hadamard transform (SRHT), and uniform sampling. In previous experiments, we also tried other methods like random sparse sampling. Through comparison, we found that the method proposed in this paper excels in extracting the main features of matrices. Other methods not only have higher computational complexity but may also extract all-zero vectors during actual image processing, rendering calculations impossible. Our method effectively avoids these issues, which is why we chose to adopt it.
This chapter introduces an algorithm, namely, the leverage score sampling free pseudo-inverse GBK method for single righthand side, and provides a proof of the corresponding convergence theory for the algorithm.
First, each step follows the approximate maximum illustration principle.
|bik−A(ik)xk|2||A(ik)||22≥ηmax1≤i≤m{|bi−A(i)xk|2||A(i)||22}, |
Second, select the index set Tk for the block matrix ATk; then, project the current estimate onto each row forming the block matrix ATk; finally, calculate the average of the projections to determine the next iteration.
xk+1=xk+(∑i∈Tkwibi−A(i)xk||A(i)||22AT(i)). |
Before presenting the convergence theory for Algorithm 3, let us first introduce a lemma.
Algorithm 3 SLFGBK method |
1: Let A,b,l,x0 be within the range of (AT), parameter η within (0,1), and consider the sequences of step sizes (αk)k≥0 and weights (wk)k≥0. 2: Output:xl. 3: Initialization: Leverage score sampling enables the selection of a small, representative subset from large datasets, facilitating more efficient computation. Algorithm 2 generates a leverage score sampling transformation matrix ˜A=SA∈Rd×n and vector ˜b=Sb, where d≪m. 4: for k=0,1,…l−1 do 5: Calculation ˜εk=ηmax1≤i≤m{|˜bi−˜A(i)xk|2||˜A(i)||22}. 6: Define the index set sequence Tk={ik:|˜biK−˜A(ik)xk|2≥εk||˜A(ik)||22}. 7: Computation xk+1=xk+(∑i∈Tkwi˜bi−˜A(i)xk||˜A(i)||22˜AT(i)). 8: end for |
Lemma 1 ([27]): If any vector u belongs to the range of AT, then
||Au||22≥λmin(ATA)||u||22 |
Theorem 2: The leverage score transformation S satisfies d=O(n2/δθ2), and x∗=A†b is the minimum norm solution of the single righthand side linear equation systems, for any k ≥ 0.
||xk+1−x∗||22≤(1−˜ψk(η)σ2min(˜A)||˜A||2F)||xk−x∗||22 | (2) |
The function ˜ψk(η)=ηt(2t−1t2σ2max(ˆATTk))σ2min,η∈(0,1], where range(A),σmin(A), and σmax(A) represent the range, nonzero minimum singular value, and maximum singular value of matrix A, respectively.
Proof. With algorithm 3 and ˜rk=˜b−˜Axk, we can obtain
xk+1=xk+(∑i∈Tk1|Tk|˜rik˜AT(i)||˜A(i)||22). | (3) |
Expand Eq (3) with the set |Tk|=t and Tk={jk1,…,jkt}.
xk+1=xk+∑i∈Tk1t˜AT(i)eTi˜rk||˜A(i)||22=xk+1t(˜AT(jk1)eTjk1˜rk||˜A(jk1)||22+⋯+˜AT(jkt)eTjkt˜rk||˜A(jkt)||22)=xk+1tˆATTkˆITk˜rk. | (4) |
Among them
ˆATTk=[˜AT(jk1)||˜A(jk1)||2,˜AT(jk2)||˜A(jk2)||2,…,˜AT(jkt)||˜A(jkt)||2]∈Rn×t, | (5) |
and
ˆITk=[e(jk1)||˜A(jk1)||2,e(jk2)||˜A(jk2)||2,…,e(jkt)||˜A(jkt)||2]T∈Rt×d. | (6) |
Subtracting x∗ from Eq (4) simultaneously yields
xk+1−x∗=xk−x∗−1tˆATTkˆITk˜A(xk−x∗)=(I−1tˆATTkˆATk)(xk−x∗) | (7) |
Taking the spectral norm and squaring both sides of Eq (7), and for any positive semi-definite matrix Q, satisfying Q2≼λmax(Q)Q, we can obtain
||xk+1−x∗||22=||(xk−x∗)−1tˆATTkˆATk(xk−x∗)||22=||(xk−x∗)||22−2t||ˆATk(xk−x∗)||22+1t2||ˆATTkˆATk((xk−x∗)||22≤||(xk−x∗)||22−(2t−1t2σ2max(ˆATTk))||ˆATk(xk−x∗)||22 | (8) |
Using Eq (5) and the inequality |˜bjk−˜A(jk)xk|2≥˜εk||˜A(jk)||22, a straightforward calculation yields.
||ˆATk(xk−x∗)||22=∑jk∈Tk1||˜A(jk)||22|˜rjkk|2 | (9) |
≥∑jk∈Tk1||˜A(jk)||22˜εk||˜A(jk)||2 | (10) |
Substituting ˜εk=ηmax1≤i≤d{|˜bi−˜A(i)xk|2||˜A(i)||22} into the above equation yields the following result:
||ˆATk(xk−x∗)||22≥∑jk∈Tkηmax1≤i≤d{|˜rik|2||˜A(i)||22}≥ηtd∑i=1|˜rik|2||A(i)||22||˜A(i)||22||A||2F=ηt||˜rk||2||˜A||2F | (11) |
Given x0∈range(AT) and x∗=A†b, we have xk−x∗∈range(AT). Therefore, by Lemma 1, we can conclude that
||˜rk||2=||˜A(xk−x∗)||22 |
≥λ2min(˜AT˜A)||xk−x∗||22 Combining the above equation, we can obtain the following
||ˆATk(xk−x∗)||22≥ηtσ2min(˜A)||˜A||2F||xk−x∗||22 | (12) |
From Eqs (8) and (12), we can obtain Eq (2), and the iterative sequence {xk}∞k=0 converges to the minimum norm solution x∗=A†b of the system of equations. Hence, Theorem 2 is proved.
In this chapter, we introduce an algorithm known as the multi-righthand-side leverage score sampling free pseudo-inverse GBK method, and we provide a proof for the corresponding convergence theory of the algorithm.
For most image reconstruction, the system of equations is formulated as follows:
AX=B | (13) |
In the context, A∈Rm×n,X=[x1,x2,…,xd]∈Rn×d,B=[b1,b2,…,bd]∈Rm×d,d>1. This paper addresses the solution of multiple righthand side linear equation systems and initially presents the index set for each iteration selection in the multiple righthand side linear equation systems.
max1≤i≤m{|b(i)−A(i)xk|||A(i)||22} | (14) |
Iterate through the following steps:
xk+1=xk+b(i)−A(i)xk||A(i)||22AT(i) |
To solve systems of linear equations with multiple righthand sides, our approach involves working with the j righthand side vector bj by selecting the working row Tkj according to the criterion established in Eq (15).
max1≤i≤m{|B(i,j)−A(i)x(k)j|||A(i)||22},j=1,2,…,kb. | (15) |
The iterative formula corresponding to the solution at the j right endpoint is as follows:
x(k+1)j=xkj+˜B(Tkj,j)−˜A(Tkj)x(k)j||˜A(Tkj)||22˜ATTkj | (16) |
According to the criterion for selecting the index set in Eq (15), each term on the righthand side corresponds to the selection of a working row. For the system of linear equations with multiple righthand terms as in Eq (13), the Kaczmarz method can be extended to an iterative format that simultaneously solves for the righthand side system of linear equations.
In this context, X(k+1)=(x(k+1)1,x(k+1)2,…,x(k+1)d) represents the approximate solution at the k+1 iteration.
Xk+1=Xk+(˜ATjk1,…,˜ATjkd)Tdiag(˜B(jk1,1)−˜Ajk1x(k)1||˜Ajk1||22,…,˜B(jkd,d)−˜Ajkdx(k)d||˜Ajkd||22) |
To reduce computational effort, Algorithm 2 is employed to extract certain rows from matrix A corresponding to the index set Tkj. The sub-matrix formed by these rows is denoted as ˜A,, while the sub-matrix of B corresponding to the index set Tk is denoted as ˜B. The specific configurations of ˜A and ˜B are as follows.
˜BTTkj=(BTTkj1,BTTkj2,…,BTTkjt) | (17) |
and
˜ATTkj=(ATTk1,ATTk2,…,ATTkt) | (18) |
The transposed matrix BTTkji is represented as (B(Tkji,1),B(Tkji,2),…,B(Tkji,d)), where Tkji is an element of the index set Tkj. Here, B(Tkji,t) denotes the element in the i row and t column of the sub-matrix ˜B, and |Tkj| denotes the number of row indices contained in the index set Tkj with |Tkj| equal to t.
The multi-righthand-side method proposed in this paper is a specialized block approach for solving large-scale righthand side linear equations using a leverage-based pseudo-inverse GBK method. We provide a detailed framework for this method, which involves simultaneous computation of multiple righthand sides, with each iteration involving a matrix B∈Rm×t containing t righthand sides, resulting in the selection of t row indices.
This article exclusively discusses the scenario where αk equals 1. The convergence theory for solving large-scale righthand side linear equation groups with the LFGBK method is presented below:
Theorem 3: Assume that the linear equation system (13) is consistent, and so are its extracted subsystems. The iterative sequence X(k) from k=0 to infinity, generated by Algorithm 4 starting from the initial value x0, converges to the least squares solution X∗=A†B. The expected error for the iterative sequence solutions X(k) from k=0 to infinity is:
E||X(k+1)−X∗||2F≤(1−σ2min(˜A(Tkj))||˜A(Tkj)||2F)E||Xk−X∗||2F | (19) |
Algorithm 4 MLFGBK Method |
1: Let A,b,l,x0 be within the range of (AT), parameter η within (0,1), and consider the sequences of step sizes (αk)k≥0 and weights (wk)k≥0. 2: Output:x. 3: Initialization: Algorithm 2 generates a leverage score sampling transformation matrix ˜A=SA∈Rd×n and matrix ˜b=Sb, where d≪m. 4: for k=0,1,…l−1 do 5: Calculation ˜εkj=ηmax1≤i≤m{|B(i,j)−A(i)X(k)j|||A(i)||22},j=1,2,…,d. 6: Establish the sequence of indicator sets. Tkj={ikj:|˜bikj−˜A(ikj)xkj|2≥˜εkj||˜A(ikj)||22}. 7: Calculation Xk+1=Xk+(˜ATjk1,…,˜ATjkd)Tdiag{˜B(jk1,1)−˜Ajk1x(k)1||˜Ajk1||22,…,˜B(jkd,d)−˜Ajkdx(k)d||˜Ajkd||22}. 8: end for |
The tilde-decorated A and B, denoted as ˜A and ˜B, respectively, represent the sub-matrices formed by the rows corresponding to the index set Tkj within matrices A and B.
Proof. According to Algorithm 4 and reference [39], we have
x(k+1)j=xkj+˜B(Tkj,j)−˜A(Tkj)x(k)j||˜A(Tkj)||22˜ATTkj |
Since X∗=A†B is the least squares solution, we infer that x∗j=A†B(:,j) for j=1,2,…,d, where B(:,j) denotes the j column of matrix B.
Further,
E||X(k+1)−X∗||2F=E∑tj=1||x(k+1)j−x∗j||22=∑tj=1E||x(k+1)j−x∗j||22 | (20) |
For j=1,2,…,d, it can be deduced that
||x(k+1)j−x∗j||22=||x(k)j−x∗j||22−E||x(k+1)j−xkj||22 |
Then
E||x(k+1)j−xkj||22=|˜B(Tkj,j)−˜A(Tkj)x(k)j|2||˜A(Tkj)||22=maxTkji∈Tkj{|˜B(Tkji,j)−˜A(Tkji)x(k)j|2||˜A(Tkji)||22} |
The specific details of the term E||x(k+1)j−xkj||22 are elaborated in the proof of Theorem 3.2 in [39].
Due to
maxTkji∈Tkj{|˜B(Tkji,j)−˜A(Tkji)x(k)j|2||˜A(Tkji)||22}=maxTkji∈Tkj{|˜B(Tkji,j)−˜A(Tkji)x(k)j|2||˜A(Tkji)||22}||Akx(k)j−˜B(:,j)||22||Akx(k)j−˜B(:,j)||22=maxTkji∈Tkj{|˜B(Tkji,j)−˜A(Tkji)x(k)j|2||˜A(Tkji)||22}||Akx(k)j−˜B(:,j)||22∑ti=1||˜A(Tkji)||22||˜B(Tkji,j)−ATkjix(k)j||22||˜A(Tkji)||22≥||˜A(Tkj)x(k)j−˜B(:,j)||22∑ti=1||˜A(Tkji)||22≥σ2min(˜A(Tkj))||˜A(Tkj)||22||x(k)j−x∗j||22 | (21) |
In this context, ˜B(:,j) denotes the j column of the matrix ˜B.
E||X(k+1)−Xkj||22=||x(k)j−x∗j||22−E||x(k+1)j−xkj||22≤(1−σ2min(˜A(Tkj))||˜A(Tkj)||22)||x(k)j−x∗j||22 | (22) |
Thus, by combining Eqs (20) and (22), we can derive inequality (19).
The proof of Theorem 3 reveals that the convergence rate of Algorithm 4 is related to the sub-matrix ˜A sampled during each leveraged iteration.
In this section, we evaluate the effectiveness of large-scale image restoration equations using several algorithms through comparative examples: the GBK method [29], the semi-stochastic block Kaczmarz method with simple random sampling for single righthand sides [40], the LFGBK method for single righthand sides, the semi-stochastic block Kaczmarz method with simple random sampling for multiple righthand sides [40], and the LFGBK method for multiple righthand sides. All experiments are implemented in MATLAB, with metrics such as peak signal-to-noise ratio (PSNR), structural similarity index (SSIM), mean squared error (MSE), and computational time (CPU in seconds) reported. Following the approach of [27], the PSNR, SSIM, MSE, and CPU metrics reflect the average iterations and computational time needed for 50 repeated calculations. During all computations, we initialize the matrix x=zeros(n,m) and set the righthand side B=AX, where X represents images from MATLAB's Cameraman, Phantom, and Mri datasets, each sized 100×100. Matrix A is constructed with elements corresponding to the product of the number of X-ray beams (4), the range of scanning angles t (0° to 179°), and the image pixels. The stopping criterion is set as RSE=||Xk−X∗||22||X∗||22≤10−6. In practice, the condition O(n2/δθ2) is quite stringent, but in many real-world computations, a sketching factor of d=n2 yields satisfactory results. We consider matrices A∈R(4∗180∗n,m) of two types: type a, generated by radon(), and type b, generated by randn().
Based on the numerical results from Tables 1 and 2, when variable A is generated using radon, we can draw the following conclusions: ⅰ) The GBK method, single righthand side SRBK (single RHS SRBK) method, single RHS LFGBK method, multiple righthand side SRBK (multiple RHS SRBK) method, and multiple RHS LFGBK method all demonstrate effectiveness in solving equation systems for image restoration. ⅱ) The GBK method, single RHS SRBK method, single RHS LFGBK method, multiple RHS SRBK method, and multiple RHS LFGBK method show similar restoration quality; however, the single RHS LFGBK and multiple RHS LFGBK methods significantly outperform the GBK, Single RHS SRBK, and Multiple RHS SRBK methods in terms of time efficiency. ⅲ) The LFGBK method exhibits superior acceleration effects at η = 0.9 compared to η = 0.8. ⅳ) The MLFGBK method displays more pronounced acceleration effects at η = 0.9 than at η = 0.8.
GBK | SSRBK | SLFGBK | |||||
data | Metrics | η=0.8 | η=0.9 | η=0.8 | η=0.9 | η=0.8 | η=0.9 |
Cameraman | PSNR | 19.595 | 17.996 | 19.076 | 17.640 | 17.595 | 17.519 |
SSIM | 0.999 | 0.998 | 0.999 | 0.998 | 0.999 | 0.999 | |
MSE | 0.011 | 0.015 | 0.012 | 0.017 | 0.017 | 0.017 | |
CPU | 234.78 | 335.37 | 55.00 | 94.755 | 11.581 | 7.543 | |
Phantom | PSNR | 73.636 | 72.694 | 73.639 | 73.655 | 72.377 | 72.430 |
SSIM | 1.000 | 1.000 | 1.000 | 1.000 | 1.000 | 1.000 | |
MSE | 0 | 0 | 0 | 0 | 0 | 0 | |
CPU | 266.67 | 279.43 | 56.186 | 75.168 | 12.496 | 8.556 | |
Mri | PSNR | 29.319 | 28.936 | 28.068 | 29.023 | 28.057 | 28.112 |
SSIM | 0.969 | 0.975 | 0.924 | 0.919 | 0.962 | 0.978 | |
MSE | 0.001 | 0.001 | 0.001 | 0.001 | 0.001 | 0.001 | |
CPU | 259.86 | 375.48 | 60.963 | 162.06 | 19.340 | 15.563 |
SLFGBK | MSRBK | MLFGBK | |||||
data | Metrics | η=0.8 | η=0.9 | η=0.8 | η=0.9 | η=0.8 | η=0.9 |
Cameraman | PSNR | 17.595 | 17.519 | 17.611 | 17.732 | 17.894 | 17.876 |
SSIM | 0.999 | 0.999 | 0.999 | 0.999 | 0.999 | 0.999 | |
MSE | 0.017 | 0.017 | 0.017 | 0.016 | 0.016 | 0.016 | |
CPU | 11.581 | 7.543 | 18.027 | 14.207 | 3.196 | 2.880 | |
Phantom | PSNR | 72.377 | 72.430 | 72.492 | 72.532 | 72.486 | 72.760 |
SSIM | 1.000 | 1.000 | 1.000 | 1.0000 | 1.000 | 1.000 | |
MSE | 0 | 0 | 0 | 0 | 0 | 0 | |
CPU | 12.496 | 8.556 | 43.106 | 38.075 | 20.874 | 20.023 | |
Mri | PSNR | 28.057 | 28.112 | 28.081 | 28.234 | 28.161 | 28.506 |
SSIM | 0.962 | 0.978 | 0.901 | 0.899 | 0.895 | 0.891 | |
MSE | 0.001 | 0.001 | 0.001 | 0.001 | 0.001 | 0.001 | |
CPU | 19.340 | 15.563 | 42.535 | 37.575 | 18.237 | 17.539 |
Based on the numerical results from Tables 3 and 4, when variable A is generated using randn, we can draw the following conclusions: ⅰ) The GBK method, single RHS SRBK method, single RHS LFGBK method, multiple RHS SRBK method, and multiple RHS LFGBK method all demonstrate effectiveness in solving equation systems for image restoration. ⅱ) The GBK method, single RHS SRBK method, single RHS LFGBK method, multiple RHS SRBK method, and multiple RHS LFGBK method show similar restoration quality; however, the Single RHS LFGBK and Multiple RHS LFGBK methods significantly outperform the GBK, single RHS SRBK, and multiple RHS SRBK methods in terms of time efficiency. ⅲ) The LFGBK method exhibits superior acceleration effects at η = 0.9 compared to η = 0.8. ⅳ) The MLFGBK method displays more pronounced acceleration effects at η = 0.9 than at η = 0.8.
GBK | SSRBK | SLFGBK | |||||
data | Metrics | η=0.8 | η=0.9 | η=0.8 | η=0.9 | η=0.8 | η=0.9 |
Cameraman | PSNR | 20.122 | 19.195 | 18.419 | 17.594 | 17.519 | 17.552 |
SSIM | 0.999 | 0.998 | 0.998 | 0.998 | 0.999 | 0.999 | |
MSE | 0.009 | 0.012 | 0.014 | 0.017 | 0.017 | 0.017 | |
CPU | 3.651 | 6.916 | 1.433 | 3.596 | 1.698 | 0.723 | |
Phantom | PSNR | 73.904 | 72.475 | 74.040 | 73.704 | 72.619 | 72.464 |
SSIM | 1.000 | 1.000 | 1.000 | 1.000 | 1.000 | 1.000 | |
MSE | 0 | 0 | 0 | 0 | 0 | 0 | |
CPU | 68.434 | 129.151 | 19.739 | 35.465 | 7.776 | 6.878 | |
Mri | PSNR | 30.615 | 29.072 | 29.289 | 28.899 | 28.080 | 28.169 |
SSIM | 0.912 | 0.900 | 0.893 | 0.884 | 0.892 | 0.905 | |
MSE | 0 | 0.001 | 0.001 | 0.001 | 0.001 | 0.001 | |
CPU | 63.758 | 122.983 | 28.132 | 31.338 | 9.451 | 8.641 |
SLFGBK | MSRBK | MLFGBK | |||||
data | Metrics | η=0.8 | η=0.9 | η=0.8 | η=0.9 | η=0.8 | η=0.9 |
Cameraman | PSNR | 17.519 | 17.552 | 17.748 | 17.762 | 17.860 | 17.805 |
SSIM | 0.999 | 0.999 | 0.999 | 0.999 | 0.999 | 0.999 | |
MSE | 0.017 | 0.017 | 0.016 | 0.016 | 0.016 | 0.016 | |
CPU | 1.698 | 0.723 | 14.582 | 11.879 | 2.005 | 1.672 | |
Phantom | PSNR | 72.619 | 72.464 | 72.398 | 72.629 | 72.695 | 72.353 |
SSIM | 1.000 | 1.000 | 1.000 | 1.000 | 1.000 | 1.000 | |
MSE | 0 | 0 | 0 | 0 | 0 | 0 | |
CPU | 7.776 | 6.878 | 47.513 | 45.431 | 13.824 | 13.757 | |
Mri | PSNR | 28.080 | 28.169 | 28.241 | 28.093 | 28.241 | 28.328 |
SSIM | 0.892 | 0.905 | 0.904 | 0.904 | 0.902 | 0.897 | |
MSE | 0.001 | 0.001 | 0.001 | 0.001 | 0.001 | 0.001 | |
CPU | 9.451 | 8.641 | 54.802 | 38.773 | 11.533 | 10.332 |
This study aims to explore a new image reconstruction algorithm, the LFGBK method, which utilizes a greedy strategy. The core of this method lies in transforming the image reconstruction problem into solving a linear system problem with multiple righthand sides. In traditional Kaczmarz algorithms, the iterative process of gradually approaching the solution is often inefficient and susceptible to the influence of initial value selection. However, the LFGBK method introduces leverage score sampling and extends from solving single righthand side linear equations to solving multiple righthand side linear equations, which is crucial for handling large-scale problems due to the time-consuming and resource-intensive nature of pseudo-inverse computation on large matrices. By avoiding pseudo-inverse calculation, the LFGBK method significantly improves the algorithm's computational efficiency. To validate the effectiveness of the proposed algorithm, this study conducts in-depth research through theoretical analysis and simulation experiments. The theoretical analysis confirms the convergence of the LFGBK method, ensuring the reliability and stability of the algorithm. The simulation experiments, compared with traditional Filtered Back Projection (FBP) methods, demonstrate the advantages of LFGBK in terms of image reconstruction quality. The experimental results show that the LFGBK method significantly preserves image details and reduces noise, proving its practicality and superiority in image reconstruction tasks.
The authors declare they have not used Artificial Intelligence (AI) tools in the creation of this article.
The authors declare there is no conflict of interest.
[1] |
U. Parlitz, L. O. Chua, L. Kocarev, K. S. Halle, A. Shang, Transmission of digital signals by chaotic synchronization, Int. J. Bifurcation Chaos, 2 (1992), 973–977. https://doi.org/10.1142/S0218127492000562 doi: 10.1142/S0218127492000562
![]() |
[2] |
L. Kocarev, U. Parlitz, General approach for chaotic synchronization with applications to communication, Phys. Rev. Lett., 74 (1995), 5028. https://doi.org/10.1103/PhysRevLett.74.5028 doi: 10.1103/PhysRevLett.74.5028
![]() |
[3] |
Q. Xie, G. Chen, E. M. Bollt, Hybrid chaos synchronization and its application in information processing, Math. Comput. Modell., 35 (2002), 145–163. https://doi.org/10.1016/S0895-7177(01)00157-1 doi: 10.1016/S0895-7177(01)00157-1
![]() |
[4] | X. Liao, P. Yu, Absolute Stability of Nonlinear Control Systems, Springer-Verlag, New York, 2008. https://doi.org/10.1007/978-1-4020-8482-9 |
[5] |
L. M. Pecora, T. L. Carroll, Synchronization in chaotic systems, Phys. Rev. Lett., 64 (1990), 821. https://doi.org/10.1103/PhysRevLett.64.821 doi: 10.1103/PhysRevLett.64.821
![]() |
[6] |
Z. Tang, J. H. Park, Y. Wang, J. Feng, Distributed impulsive quasi-synchronization of Lur'e networks with proportional delay, IEEE Trans. Cybern., 49 (2019), 3105–3115. https://doi.org/10.1109/TCYB.2018.2839178 doi: 10.1109/TCYB.2018.2839178
![]() |
[7] |
D. Xuan, Z. Tang, J. Feng, J. H. Park, Cluster synchronization of nonlinearly coupled Lur'e networks: Delayed impulsive adaptive control protocols, Chaos, Solitons Fractals, 152 (2021), 111337. https://doi.org/10.1016/j.chaos.2021.111337 doi: 10.1016/j.chaos.2021.111337
![]() |
[8] |
S. Shao, J. Cao, Y. Hu, X. Liu. Prespecified-time distributed synchronization of Lur'e networks with smooth controllers, Asian J. Control, 24 (2022), 125–136. https://doi.org/10.1002/asjc.2422 doi: 10.1002/asjc.2422
![]() |
[9] |
J. Yang, J. Huang, X. He, W. Yang, Bipartite synchronization of Lur'e network with signed graphs based on intermittent control, ISA Trans., 135 (2023), 290–298. https://doi.org/10.1016/j.isatra.2022.10.002 doi: 10.1016/j.isatra.2022.10.002
![]() |
[10] |
R. Kavikumar, R. Sakthivel, O. M. Kwon, B. Kaviarasan, Reliable non-fragile memory state feedback controller design for fuzzy Markov jump systems, Nonlinear Anal. Hybrid Syst., 35 (2020), 100828. https://doi.org/10.1016/j.nahs.2019.100828 doi: 10.1016/j.nahs.2019.100828
![]() |
[11] |
H. Ji, Y. Li, X. Ding, J. Lu, Stability analysis of boolean networks with Markov jump disturbances and their application in apoptosis networks, Electron. Res. Arch., 30 (2022), 3422–3434. https://doi.org/10.3934/era.2022174 doi: 10.3934/era.2022174
![]() |
[12] |
R. Sakthivel, H. Divya, A. Parivallal, V. T. Suveetha, Quantized fault detection filter design for networked control system with Markov jump parameters, Circuits Syst. Signal Process., 40 (2021), 4741–4758. https://doi.org/10.1007/s00034-021-01693-x doi: 10.1007/s00034-021-01693-x
![]() |
[13] |
H. Liu, J. Cheng, J. Cao, I. Katib, Preassigned-time synchronization for complex-valued memristive neural networks with reaction–diffusion terms and Markov parameters, Neural Networks, 169 (2024), 520–531. https://doi.org/10.1016/j.neunet.2023.11.011 doi: 10.1016/j.neunet.2023.11.011
![]() |
[14] | X. Zhou, S. Zhong, Delay-range-dependent exponential synchronization of Lur'e systems with Markovian switching, Int. J. Math. Comput. Sci., 4 (2010), 407–412. |
[15] | J. Zhou, B. Zhang, Master-slave synchronization of singular Lur'e time-delay systems with Markovian jumping parameters, in 2020 7th International Conference on Information, Cybernetics, and Computational Social Systems (ICCSS), Guangzhou, China, (2020), 164–169. https://doi.org/10.1109/ICCSS52145.2020.9336877 |
[16] |
X. Huang, Y. Zhou, M. Fang, J. Zhou, S. Arik, Finite-time H∞ synchronization of semi-Markov jump Lur'e systems, Mod. Phys. Lett. B, 35 (2021), 2150168. https://doi.org/10.1142/S0217984921501682 doi: 10.1142/S0217984921501682
![]() |
[17] |
J. Zhou, J. Dong, S. Xu, Asynchronous dissipative control of discrete-time fuzzy Markov jump systems with dynamic state and input quantization, IEEE Trans. Fuzzy Syst., 31 (2023), 3906–3920. https://doi.org/10.1109/TFUZZ.2023.3271348 doi: 10.1109/TFUZZ.2023.3271348
![]() |
[18] |
X. Li, X. Qin, Z. Wan, W. Tai, Chaos synchronization of stochastic time-delay Lur'e systems: An asynchronous and adaptive event-triggered control approach, Electron. Res. Arch., 31 (2023), 5589–5608. https://doi.org/10.3934/era.2023284 doi: 10.3934/era.2023284
![]() |
[19] |
S. Santra, M. Joby, M. Sathishkumar, S. M. Anthoni, LMI approach-based sampled-data control for uncertain systems with actuator saturation: application to multi-machine power system, Nonlinear Dyn., 107 (2022), 967–982. https://doi.org/10.1007/s11071-021-06995-y doi: 10.1007/s11071-021-06995-y
![]() |
[20] |
Y. Zhou, X. Chang, W. Huang, Z. Li, Quantized extended dissipative synchronization for semi-Markov switching Lur'e systems with time delay under deception attacks, Commun. Nonlinear Sci. Numer. Simul., 117 (2023), 106972. https://doi.org/10.1016/j.cnsns.2022.106972 doi: 10.1016/j.cnsns.2022.106972
![]() |
[21] |
Q. Li, X. Liu, Q. Zhu, S. Zhong, J. Cheng, Stochastic synchronization of semi-Markovian jump chaotic Lur'e with packet dropouts subject to multiple sampling periods, J. Franklin Inst., 356 (2019), 6899–6925. https://doi.org/10.1016/j.jfranklin.2019.06.005 doi: 10.1016/j.jfranklin.2019.06.005
![]() |
[22] |
T. Yang, Z. Wang, X. Huang, J. Xia, Sampled-data exponential synchronization of Markovian jump chaotic Lur'e systems with multiple time delays, Chaos, Solitons Fractals, 160 (2022), 112252. https://doi.org/10.1016/j.chaos.2022.112252 doi: 10.1016/j.chaos.2022.112252
![]() |
[23] |
C. Ge, X. Liu, Y. Liu, C. Hua, Synchronization of inertial neural networks with unbounded delays via sampled-data control, IEEE Trans. Neural Networks Learn. Syst., 35 (2024), 5891–5901. https://doi.org/10.1109/TNNLS.2022.3222861 doi: 10.1109/TNNLS.2022.3222861
![]() |
[24] |
Y. Ni, Z. Wang, X. Huang, Q. Ma, H. Shen, Intermittent sampled-data control for local stabilization of neural networks subject to actuator saturation: A work-interval-dependent functional approach, IEEE Trans. Neural Networks Learn. Syst., 35 (2024), 1087–1097. https://doi.org/10.1109/TNNLS.2022.3180076 doi: 10.1109/TNNLS.2022.3180076
![]() |
[25] |
Y. Liu, Y. Zhang, L. Liu, S. Tong, C. L. P. Chen, Adaptive finite-time control for half-vehicle active suspension systems with uncertain dynamics, IEEE/ASME Trans. Mechatron., 26 (2021), 168–178. https://doi.org/10.1109/TMECH.2020.3008216 doi: 10.1109/TMECH.2020.3008216
![]() |
[26] |
Y. Wang, C. Hua, P Shi, Improved admissibility criteria for Takagi-Sugeno fuzzy singular systems with time-varying delay, IEEE Trans. Fuzzy Syst., 31 (2023), 2966–2974. https://doi.org/10.1109/TFUZZ.2023.3240250 doi: 10.1109/TFUZZ.2023.3240250
![]() |
[27] | G. Yang, X. Guo, W. Che, W. Guan, Linear Systems: Non-Fragile Control and Filtering, CRC Press, 2013. https://doi.org/10.1201/b14766 |
[28] |
X. Chang, G. Yang, Nonfragile H∞ filter design for T–S fuzzy systems in standard form, IEEE Trans. Ind. Electron., 61 (2014), 3448–3458. https://doi.org/10.1109/TIE.2013.2278955 doi: 10.1109/TIE.2013.2278955
![]() |
[29] |
K. Liu, A. Seuret, Y. Xia, Stability analysis of systems with time-varying delays via the second-order Bessel–Legendre inequality, Automatica, 76 (2017), 138–142. https://doi.org/10.1016/j.automatica.2016.11.001 doi: 10.1016/j.automatica.2016.11.001
![]() |
[30] |
W. Tai, D. Zuo, J. Han, J. Zhou, Fuzzy resilient control for synchronizing chaotic systems with time-variant delay and external disturbance, Int. J. Mod. Phys. B, 35 (2021), 2150177. https://doi.org/10.1142/S0217979221501770 doi: 10.1142/S0217979221501770
![]() |
[31] |
N. T. T. Huyen, M. V. Thuan, N. T. Thanh, T. N. Binh, Guaranteed cost control of fractional-order switched systems with mixed time-varying delays, Comput. Appl. Math., 42 (2023), 370. https://doi.org/10.1007/s40314-023-02505-5 doi: 10.1007/s40314-023-02505-5
![]() |
[32] |
M. Sathishkumar, R. Sakthivel, C. Wang, B. Kaviarasan, S. M. Anthoni, Non-fragile filtering for singular Markovian jump systems with missing measurements, Signal Process., 142 (2018), 125–136. https://doi.org/10.1016/j.sigpro.2017.07.012 doi: 10.1016/j.sigpro.2017.07.012
![]() |
[33] |
X. Qin, J. Dong, X. Zhang, T. Jiang, J. Zhou, H∞ control of time-delayed Markov jump systems subject to mismatched modes and interval conditional probabilities, Arab. J. Sci. Eng., 49 (2024), 7471–7486. https://doi.org/10.1007/s13369-023-08332-4 doi: 10.1007/s13369-023-08332-4
![]() |
[34] |
D. Tong, B. Ma, Q. Chen, Y. Wei, P. Shi, Finite-time synchronization and energy consumption prediction for multilayer fractional-order networks, IEEE Trans. Circuits Syst. II Express Briefs, 70 (2023), 2176–2180. https://doi.org/10.1109/TCSII.2022.3233420 doi: 10.1109/TCSII.2022.3233420
![]() |
[35] |
L. Yao, Z. Wang, X. Huang, Y. Li, Q. Ma, H. Shen, Stochastic sampled-data exponential synchronization of Markovian jump neural networks with time-varying delays, IEEE Trans. Neural Networks Learn. Syst., 34 (2023), 909–920. https://doi.org/10.1109/TNNLS.2021.3103958 doi: 10.1109/TNNLS.2021.3103958
![]() |
[36] |
G. Yang, D. Tong, Q. Chen, W. Zhou, Fixed-time synchronization and energy consumption for Kuramoto-oscillator networks with multilayer distributed control, IEEE Trans. Circuits Syst. II Express Briefs, 70 (2023), 1555–1559. https://doi.org/10.1109/TCSII.2022.3221477 doi: 10.1109/TCSII.2022.3221477
![]() |
[37] |
L. Shanmugam, Y. H. Joo, Design of interval type-2 fuzzy-based sampled-data controller for nonlinear systems using novel fuzzy Lyapunov functional and its application to PMSM, IEEE Trans. Syst. Man Cybern.: Syst., 51 (2021), 542–551. https://doi.org/10.1109/TSMC.2018.2875098 doi: 10.1109/TSMC.2018.2875098
![]() |
[38] |
M. Sathishkumar, R. Sakthivel, F. Alzahrani, B. Kaviarasan, Y. Ren, Mixed H∞ and passivity-based resilient controller for nonhomogeneous Markov jump systems, Nonlinear Anal. Hybrid Syst., 31 (2019), 86–99. https://doi.org/10.1016/j.nahs.2018.08.003 doi: 10.1016/j.nahs.2018.08.003
![]() |
[39] |
X. Jiang, G. Xia, Z. Feng, T. Li, Non-fragile H∞ consensus tracking of nonlinear multi-agent systems with switching topologies and transmission delay via sampled-data control, Inf. Sci., 509 (2020), 210–226. https://doi.org/10.1016/j.ins.2019.08.078 doi: 10.1016/j.ins.2019.08.078
![]() |
[40] |
Z. Yan, D. Zuo, T. Guo, J. Zhou, Quantized H∞ stabilization for delayed memristive neural networks, Neural Comput. Appl., 35 (2023), 16473–16486. https://doi.org/10.1007/s00521-023-08510-3 doi: 10.1007/s00521-023-08510-3
![]() |
[41] |
T. H. Lee, J. H. Park, Improved criteria for sampled-data synchronization of chaotic Lur'e systems using two new approaches, Nonlinear Anal. Hybrid Syst., 24 (2017), 132–145. https://doi.org/10.1016/j.nahs.2016.11.006 doi: 10.1016/j.nahs.2016.11.006
![]() |
[42] |
W. Tai, D. Zuo, Z. Xuan, J. Zhou, Z. Wang, Non-fragile L2−L∞ filtering for a class of switched neural networks, Math. Comput. Simul., 185 (2021), 629–645. https://doi.org/10.1016/j.matcom.2021.01.014 doi: 10.1016/j.matcom.2021.01.014
![]() |
[43] |
J. Zhou, X. Ma, Z. Yan, S. Arik, Non-fragile output-feedback control for time-delay neural networks with persistent dwell time switching: A system mode and time scheduler dual-dependent design, Neural Networks, 169 (2024), 733–743. https://doi.org/10.1016/j.neunet.2023.11.007 doi: 10.1016/j.neunet.2023.11.007
![]() |
[44] |
G. Chen, J. Xia, J. H. Park, H. Shen, G. Zhuang, Sampled-data synchronization of stochastic Markovian jump neural networks with time-varying delay, IEEE Trans. Neural Networks Learn. Syst., 33 (2022), 3829–3841. https://doi.org/10.1109/TNNLS.2021.3054615 doi: 10.1109/TNNLS.2021.3054615
![]() |
[45] |
D. A. Wilson, Convolution and Hankel operator norms for linear systems, IEEE Trans. Autom. Control, 34 (1989), 94–97. https://doi.org/10.1109/9.8655 doi: 10.1109/9.8655
![]() |
[46] |
A. Seuret, F. Gouaisbaut, Hierarchy of LMI conditions for the stability analysis of time-delay systems, Syst. Control Lett., 81 (2015), 1–7. https://doi.org/10.1016/j.sysconle.2015.03.007 doi: 10.1016/j.sysconle.2015.03.007
![]() |
[47] |
H. Zeng, Y. He, M. Wu, J. She, New results on stability analysis for systems with discrete distributed delay, Automatica, 60 (2015), 189–192. https://doi.org/10.1016/j.automatica.2015.07.017 doi: 10.1016/j.automatica.2015.07.017
![]() |
[48] |
A. Seuret, K. Liu, F. Gouaisbaut, Generalized reciprocally convex combination lemmas and its application to time-delay systems, Automatica, 95 (2018), 488–493. https://doi.org/10.1016/j.automatica.2018.06.017 doi: 10.1016/j.automatica.2018.06.017
![]() |
[49] |
K. Zhou, P. P. Khargonekar, Robust stabilization of linear systems with norm-bounded time-varying uncertainty, Syst. Control Lett., 10 (1988), 17–20. https://doi.org/10.1016/0167-6911(88)90034-5 doi: 10.1016/0167-6911(88)90034-5
![]() |
[50] | S. Boyd, L. El. Ghaoui, E. Feron, V. Balakrishnan, Linear Matrix Inequalities in System and Control Theory, SIAM, Philadelphia, PA, USA, 1994. https://doi.org/10.1137/1.9781611970777 |
[51] | M. Wu, Y. He, J. She, Stability Analysis and Robust Control of Time-Delay Systems, Springer, New York, 2010. https://doi.org/10.1007/978-3-642-03037-6 |
1. | Narges Peykrayegan, Mehdi Ghovatmand, Mohammad Hadi Noori Skandari, Dumitru Baleanu, An approximate approach for fractional singular delay integro-differential equations, 2022, 7, 2473-6988, 9156, 10.3934/math.2022507 | |
2. | Mehboob Alam, Akbar Zada, Implementation of q-calculus on q-integro-differential equation involving anti-periodic boundary conditions with three criteria, 2022, 154, 09600779, 111625, 10.1016/j.chaos.2021.111625 | |
3. | Binlin Zhang, Rafia Majeed, Mehboob Alam, On Fractional Langevin Equations with Stieltjes Integral Conditions, 2022, 10, 2227-7390, 3877, 10.3390/math10203877 | |
4. | Mehboob Alam, Akbar Zada, Usman Riaz, On a Coupled Impulsive Fractional Integrodifferential System with Hadamard Derivatives, 2022, 21, 1575-5460, 10.1007/s12346-021-00535-0 | |
5. | Mehboob Alam, Dildar Shah, Hyers–Ulam stability of coupled implicit fractional integro-differential equations with Riemann–Liouville derivatives, 2021, 150, 09600779, 111122, 10.1016/j.chaos.2021.111122 | |
6. | Akbar Zada, Mehboob Alam, Khansa Hina Khalid, Ramsha Iqbal, Ioan-Lucian Popa, Analysis of Q-Fractional Implicit Differential Equation with Nonlocal Riemann–Liouville and Erdélyi-Kober Q-Fractional Integral Conditions, 2022, 21, 1575-5460, 10.1007/s12346-022-00623-9 | |
7. | Ravi Agarwal, Snezhana Hristova, Donal O'Regan, Integral presentations of the solution of a boundary value problem for impulsive fractional integro-differential equations with Riemann-Liouville derivatives, 2022, 7, 2473-6988, 2973, 10.3934/math.2022164 | |
8. | Mehboob Alam, Aftab Khan, Muhammad Asif, Analysis of implicit system of fractional order via generalized boundary conditions, 2023, 0170-4214, 10.1002/mma.9139 | |
9. | Mehboob Alam, Khansa Hina Khalid, Analysis of q ‐fractional coupled implicit systems involving the nonlocal Riemann–Liouville and Erdélyi–Kober q ‐fractional integral conditions , 2023, 0170-4214, 10.1002/mma.9208 | |
10. | Rafia Majeed, Binlin Zhang, Mehboob Alam, Fractional Langevin Coupled System with Stieltjes Integral Conditions, 2023, 11, 2227-7390, 2278, 10.3390/math11102278 | |
11. | Mehboob Alam, Akbar Zada, Sumbel Begum, Usman Riaz, Analysis of Fractional Integro-differential System with Impulses, 2023, 9, 2349-5103, 10.1007/s40819-023-01584-6 | |
12. | Akbar Zada, Usman Riaz, Junaid Jamshed, Mehboob Alam, Afef Kallekh, Analysis of impulsive Caputo fractional integro‐differential equations with delay, 2024, 0170-4214, 10.1002/mma.10426 |
GBK | SSRBK | SLFGBK | |||||
data | Metrics | η=0.8 | η=0.9 | η=0.8 | η=0.9 | η=0.8 | η=0.9 |
Cameraman | PSNR | 19.595 | 17.996 | 19.076 | 17.640 | 17.595 | 17.519 |
SSIM | 0.999 | 0.998 | 0.999 | 0.998 | 0.999 | 0.999 | |
MSE | 0.011 | 0.015 | 0.012 | 0.017 | 0.017 | 0.017 | |
CPU | 234.78 | 335.37 | 55.00 | 94.755 | 11.581 | 7.543 | |
Phantom | PSNR | 73.636 | 72.694 | 73.639 | 73.655 | 72.377 | 72.430 |
SSIM | 1.000 | 1.000 | 1.000 | 1.000 | 1.000 | 1.000 | |
MSE | 0 | 0 | 0 | 0 | 0 | 0 | |
CPU | 266.67 | 279.43 | 56.186 | 75.168 | 12.496 | 8.556 | |
Mri | PSNR | 29.319 | 28.936 | 28.068 | 29.023 | 28.057 | 28.112 |
SSIM | 0.969 | 0.975 | 0.924 | 0.919 | 0.962 | 0.978 | |
MSE | 0.001 | 0.001 | 0.001 | 0.001 | 0.001 | 0.001 | |
CPU | 259.86 | 375.48 | 60.963 | 162.06 | 19.340 | 15.563 |
SLFGBK | MSRBK | MLFGBK | |||||
data | Metrics | η=0.8 | η=0.9 | η=0.8 | η=0.9 | η=0.8 | η=0.9 |
Cameraman | PSNR | 17.595 | 17.519 | 17.611 | 17.732 | 17.894 | 17.876 |
SSIM | 0.999 | 0.999 | 0.999 | 0.999 | 0.999 | 0.999 | |
MSE | 0.017 | 0.017 | 0.017 | 0.016 | 0.016 | 0.016 | |
CPU | 11.581 | 7.543 | 18.027 | 14.207 | 3.196 | 2.880 | |
Phantom | PSNR | 72.377 | 72.430 | 72.492 | 72.532 | 72.486 | 72.760 |
SSIM | 1.000 | 1.000 | 1.000 | 1.0000 | 1.000 | 1.000 | |
MSE | 0 | 0 | 0 | 0 | 0 | 0 | |
CPU | 12.496 | 8.556 | 43.106 | 38.075 | 20.874 | 20.023 | |
Mri | PSNR | 28.057 | 28.112 | 28.081 | 28.234 | 28.161 | 28.506 |
SSIM | 0.962 | 0.978 | 0.901 | 0.899 | 0.895 | 0.891 | |
MSE | 0.001 | 0.001 | 0.001 | 0.001 | 0.001 | 0.001 | |
CPU | 19.340 | 15.563 | 42.535 | 37.575 | 18.237 | 17.539 |
GBK | SSRBK | SLFGBK | |||||
data | Metrics | η=0.8 | η=0.9 | η=0.8 | η=0.9 | η=0.8 | η=0.9 |
Cameraman | PSNR | 20.122 | 19.195 | 18.419 | 17.594 | 17.519 | 17.552 |
SSIM | 0.999 | 0.998 | 0.998 | 0.998 | 0.999 | 0.999 | |
MSE | 0.009 | 0.012 | 0.014 | 0.017 | 0.017 | 0.017 | |
CPU | 3.651 | 6.916 | 1.433 | 3.596 | 1.698 | 0.723 | |
Phantom | PSNR | 73.904 | 72.475 | 74.040 | 73.704 | 72.619 | 72.464 |
SSIM | 1.000 | 1.000 | 1.000 | 1.000 | 1.000 | 1.000 | |
MSE | 0 | 0 | 0 | 0 | 0 | 0 | |
CPU | 68.434 | 129.151 | 19.739 | 35.465 | 7.776 | 6.878 | |
Mri | PSNR | 30.615 | 29.072 | 29.289 | 28.899 | 28.080 | 28.169 |
SSIM | 0.912 | 0.900 | 0.893 | 0.884 | 0.892 | 0.905 | |
MSE | 0 | 0.001 | 0.001 | 0.001 | 0.001 | 0.001 | |
CPU | 63.758 | 122.983 | 28.132 | 31.338 | 9.451 | 8.641 |
SLFGBK | MSRBK | MLFGBK | |||||
data | Metrics | η=0.8 | η=0.9 | η=0.8 | η=0.9 | η=0.8 | η=0.9 |
Cameraman | PSNR | 17.519 | 17.552 | 17.748 | 17.762 | 17.860 | 17.805 |
SSIM | 0.999 | 0.999 | 0.999 | 0.999 | 0.999 | 0.999 | |
MSE | 0.017 | 0.017 | 0.016 | 0.016 | 0.016 | 0.016 | |
CPU | 1.698 | 0.723 | 14.582 | 11.879 | 2.005 | 1.672 | |
Phantom | PSNR | 72.619 | 72.464 | 72.398 | 72.629 | 72.695 | 72.353 |
SSIM | 1.000 | 1.000 | 1.000 | 1.000 | 1.000 | 1.000 | |
MSE | 0 | 0 | 0 | 0 | 0 | 0 | |
CPU | 7.776 | 6.878 | 47.513 | 45.431 | 13.824 | 13.757 | |
Mri | PSNR | 28.080 | 28.169 | 28.241 | 28.093 | 28.241 | 28.328 |
SSIM | 0.892 | 0.905 | 0.904 | 0.904 | 0.902 | 0.897 | |
MSE | 0.001 | 0.001 | 0.001 | 0.001 | 0.001 | 0.001 | |
CPU | 9.451 | 8.641 | 54.802 | 38.773 | 11.533 | 10.332 |
GBK | SSRBK | SLFGBK | |||||
data | Metrics | η=0.8 | η=0.9 | η=0.8 | η=0.9 | η=0.8 | η=0.9 |
Cameraman | PSNR | 19.595 | 17.996 | 19.076 | 17.640 | 17.595 | 17.519 |
SSIM | 0.999 | 0.998 | 0.999 | 0.998 | 0.999 | 0.999 | |
MSE | 0.011 | 0.015 | 0.012 | 0.017 | 0.017 | 0.017 | |
CPU | 234.78 | 335.37 | 55.00 | 94.755 | 11.581 | 7.543 | |
Phantom | PSNR | 73.636 | 72.694 | 73.639 | 73.655 | 72.377 | 72.430 |
SSIM | 1.000 | 1.000 | 1.000 | 1.000 | 1.000 | 1.000 | |
MSE | 0 | 0 | 0 | 0 | 0 | 0 | |
CPU | 266.67 | 279.43 | 56.186 | 75.168 | 12.496 | 8.556 | |
Mri | PSNR | 29.319 | 28.936 | 28.068 | 29.023 | 28.057 | 28.112 |
SSIM | 0.969 | 0.975 | 0.924 | 0.919 | 0.962 | 0.978 | |
MSE | 0.001 | 0.001 | 0.001 | 0.001 | 0.001 | 0.001 | |
CPU | 259.86 | 375.48 | 60.963 | 162.06 | 19.340 | 15.563 |
SLFGBK | MSRBK | MLFGBK | |||||
data | Metrics | η=0.8 | η=0.9 | η=0.8 | η=0.9 | η=0.8 | η=0.9 |
Cameraman | PSNR | 17.595 | 17.519 | 17.611 | 17.732 | 17.894 | 17.876 |
SSIM | 0.999 | 0.999 | 0.999 | 0.999 | 0.999 | 0.999 | |
MSE | 0.017 | 0.017 | 0.017 | 0.016 | 0.016 | 0.016 | |
CPU | 11.581 | 7.543 | 18.027 | 14.207 | 3.196 | 2.880 | |
Phantom | PSNR | 72.377 | 72.430 | 72.492 | 72.532 | 72.486 | 72.760 |
SSIM | 1.000 | 1.000 | 1.000 | 1.0000 | 1.000 | 1.000 | |
MSE | 0 | 0 | 0 | 0 | 0 | 0 | |
CPU | 12.496 | 8.556 | 43.106 | 38.075 | 20.874 | 20.023 | |
Mri | PSNR | 28.057 | 28.112 | 28.081 | 28.234 | 28.161 | 28.506 |
SSIM | 0.962 | 0.978 | 0.901 | 0.899 | 0.895 | 0.891 | |
MSE | 0.001 | 0.001 | 0.001 | 0.001 | 0.001 | 0.001 | |
CPU | 19.340 | 15.563 | 42.535 | 37.575 | 18.237 | 17.539 |
GBK | SSRBK | SLFGBK | |||||
data | Metrics | η=0.8 | η=0.9 | η=0.8 | η=0.9 | η=0.8 | η=0.9 |
Cameraman | PSNR | 20.122 | 19.195 | 18.419 | 17.594 | 17.519 | 17.552 |
SSIM | 0.999 | 0.998 | 0.998 | 0.998 | 0.999 | 0.999 | |
MSE | 0.009 | 0.012 | 0.014 | 0.017 | 0.017 | 0.017 | |
CPU | 3.651 | 6.916 | 1.433 | 3.596 | 1.698 | 0.723 | |
Phantom | PSNR | 73.904 | 72.475 | 74.040 | 73.704 | 72.619 | 72.464 |
SSIM | 1.000 | 1.000 | 1.000 | 1.000 | 1.000 | 1.000 | |
MSE | 0 | 0 | 0 | 0 | 0 | 0 | |
CPU | 68.434 | 129.151 | 19.739 | 35.465 | 7.776 | 6.878 | |
Mri | PSNR | 30.615 | 29.072 | 29.289 | 28.899 | 28.080 | 28.169 |
SSIM | 0.912 | 0.900 | 0.893 | 0.884 | 0.892 | 0.905 | |
MSE | 0 | 0.001 | 0.001 | 0.001 | 0.001 | 0.001 | |
CPU | 63.758 | 122.983 | 28.132 | 31.338 | 9.451 | 8.641 |
SLFGBK | MSRBK | MLFGBK | |||||
data | Metrics | η=0.8 | η=0.9 | η=0.8 | η=0.9 | η=0.8 | η=0.9 |
Cameraman | PSNR | 17.519 | 17.552 | 17.748 | 17.762 | 17.860 | 17.805 |
SSIM | 0.999 | 0.999 | 0.999 | 0.999 | 0.999 | 0.999 | |
MSE | 0.017 | 0.017 | 0.016 | 0.016 | 0.016 | 0.016 | |
CPU | 1.698 | 0.723 | 14.582 | 11.879 | 2.005 | 1.672 | |
Phantom | PSNR | 72.619 | 72.464 | 72.398 | 72.629 | 72.695 | 72.353 |
SSIM | 1.000 | 1.000 | 1.000 | 1.000 | 1.000 | 1.000 | |
MSE | 0 | 0 | 0 | 0 | 0 | 0 | |
CPU | 7.776 | 6.878 | 47.513 | 45.431 | 13.824 | 13.757 | |
Mri | PSNR | 28.080 | 28.169 | 28.241 | 28.093 | 28.241 | 28.328 |
SSIM | 0.892 | 0.905 | 0.904 | 0.904 | 0.902 | 0.897 | |
MSE | 0.001 | 0.001 | 0.001 | 0.001 | 0.001 | 0.001 | |
CPU | 9.451 | 8.641 | 54.802 | 38.773 | 11.533 | 10.332 |