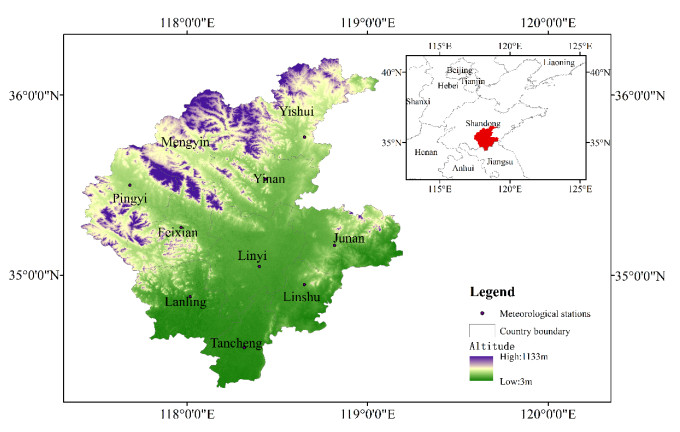
Evaporation is a key element of the water and energy cycle and is essential in determining the spatial and temporal variations of meteorological elements. In particular, evaporation is crucial for thoroughly understanding the climate variations of a region. In this study, we discussed evaporation, precipitation, and temperature by adopting Linyi City in Shandong Province, China, which is an important agricultural region, as a research case. Linear regression analysis, the empirical orthogonal decomposition function, and the Morlet wavelet function were used to reveal the trends, spatiotemporal modes, and multi-time scale characteristics of the three climate factors and provide a theoretical basis for the efficient use of climate resources in the future development of regional agriculture. Results showed that the precipitation (2.09 mm/a) and temperature (0.04 ℃/a) in Linyi City exhibited a synchronous growth trend. Conversely, evaporation (−6.47 mm/a) showed a decreasing trend and the evaporation paradox because of the considerable decrease in evaporation energy. Regional development of water-consuming agriculture in consideration of global warming is a key point for improving water use efficiency in Linyi City. In terms of spatial distribution, precipitation was dominated by the first mode wherein low precipitation was observed at the early stage, and high precipitation occurred at the late stage. The first mode was supplemented by the second mode wherein an inverse phase change occurred in the southeast-northwest direction. Large interannual fluctuations were observed only in Yinan County. Temperature exhibited a pattern of warming change with high homogeneity. Evaporation demonstrated obvious heterogeneity and was dominated by two major modes, and the difference in evaporation between Junan County and the other regions of Linyi City was large. Therefore, the local regional climate changes in Yinan and Junan should be given attention. All three meteorological elements showed interannual and interdecadal variations in the short (5 a), medium (16 a), and long (25 a) terms, with precipitation, temperature, and evaporation dominated by 16 a, 24 a, and 31 a, respectively. In the short-term future, the regional precipitation and temperature in Linyi will experience decrements that are above the multiyear average, and evaporation will increase to above the multiyear average. Given the changing trends of precipitation, temperature, and evaporation, urgent requirements for the regional development of efficient water-saving irrigation and the promotion of digital agriculture should be proposed.
Citation: Li Li, Xiaoning Lu, Wu Jun. Spatiotemporal variation of the major meteorological elements in an agricultural region: A case study of Linyi City, Northern China[J]. Electronic Research Archive, 2024, 32(4): 2447-2465. doi: 10.3934/era.2024112
[1] | Li Li, Xiaoning Lu, Wu Jun . Correction: Spatiotemporal variation of the major meteorological elements in an agricultural region: A case study of Linyi City, Northern China. Electronic Research Archive, 2024, 32(5): 3313-3315. doi: 10.3934/era.2024152 |
[2] | Huimin Bai, Li Li, Yongping Wu, Chen Liu, Zhiqiang Gong, Guolin Feng, Gui-Quan Sun . Study on the influence of meteorological elements on growing season vegetation coverage in Xinjiang, China. Electronic Research Archive, 2022, 30(9): 3463-3480. doi: 10.3934/era.2022177 |
[3] | Jieqiong Yang, Panzhu Luo . Study on the spatial correlation network structure of agricultural carbon emission efficiency in China. Electronic Research Archive, 2023, 31(12): 7256-7283. doi: 10.3934/era.2023368 |
[4] | Ruo Jia, Richard Chamoun, Alexander Wallenbring, Masoomeh Advand, Shanchuan Yu, Yang Liu, Kun Gao . A spatio-temporal deep learning model for short-term bike-sharing demand prediction. Electronic Research Archive, 2023, 31(2): 1031-1047. doi: 10.3934/era.2023051 |
[5] | Hongze Zhu, Chenguang Zhou, Nana Sun . A weak Galerkin method for nonlinear stochastic parabolic partial differential equations with additive noise. Electronic Research Archive, 2022, 30(6): 2321-2334. doi: 10.3934/era.2022118 |
[6] | Ruohan Cao, Jin Su, Jinqian Feng, Qin Guo . PhyICNet: Physics-informed interactive learning convolutional recurrent network for spatiotemporal dynamics. Electronic Research Archive, 2024, 32(12): 6641-6659. doi: 10.3934/era.2024310 |
[7] | Jongho Kim, Woosuk Kim, Eunjeong Ko, Yong-Shin Kang, Hyungjoo Kim . Estimation of spatiotemporal travel speed based on probe vehicles in mixed traffic flow. Electronic Research Archive, 2024, 32(1): 317-331. doi: 10.3934/era.2024015 |
[8] | Gang Cheng, Changliang He . Analysis of bus travel characteristics and predictions of elderly passenger flow based on smart card data. Electronic Research Archive, 2022, 30(12): 4256-4276. doi: 10.3934/era.2022217 |
[9] | Dongbao Jia, Zhongxun Xu, Yichen Wang, Rui Ma, Wenzheng Jiang, Yalong Qian, Qianjin Wang, Weixiang Xu . Application of intelligent time series prediction method to dew point forecast. Electronic Research Archive, 2023, 31(5): 2878-2899. doi: 10.3934/era.2023145 |
[10] | Noelia Bazarra, José R. Fernández, Ramón Quintanilla . Numerical analysis of a problem in micropolar thermoviscoelasticity. Electronic Research Archive, 2022, 30(2): 683-700. doi: 10.3934/era.2022036 |
Evaporation is a key element of the water and energy cycle and is essential in determining the spatial and temporal variations of meteorological elements. In particular, evaporation is crucial for thoroughly understanding the climate variations of a region. In this study, we discussed evaporation, precipitation, and temperature by adopting Linyi City in Shandong Province, China, which is an important agricultural region, as a research case. Linear regression analysis, the empirical orthogonal decomposition function, and the Morlet wavelet function were used to reveal the trends, spatiotemporal modes, and multi-time scale characteristics of the three climate factors and provide a theoretical basis for the efficient use of climate resources in the future development of regional agriculture. Results showed that the precipitation (2.09 mm/a) and temperature (0.04 ℃/a) in Linyi City exhibited a synchronous growth trend. Conversely, evaporation (−6.47 mm/a) showed a decreasing trend and the evaporation paradox because of the considerable decrease in evaporation energy. Regional development of water-consuming agriculture in consideration of global warming is a key point for improving water use efficiency in Linyi City. In terms of spatial distribution, precipitation was dominated by the first mode wherein low precipitation was observed at the early stage, and high precipitation occurred at the late stage. The first mode was supplemented by the second mode wherein an inverse phase change occurred in the southeast-northwest direction. Large interannual fluctuations were observed only in Yinan County. Temperature exhibited a pattern of warming change with high homogeneity. Evaporation demonstrated obvious heterogeneity and was dominated by two major modes, and the difference in evaporation between Junan County and the other regions of Linyi City was large. Therefore, the local regional climate changes in Yinan and Junan should be given attention. All three meteorological elements showed interannual and interdecadal variations in the short (5 a), medium (16 a), and long (25 a) terms, with precipitation, temperature, and evaporation dominated by 16 a, 24 a, and 31 a, respectively. In the short-term future, the regional precipitation and temperature in Linyi will experience decrements that are above the multiyear average, and evaporation will increase to above the multiyear average. Given the changing trends of precipitation, temperature, and evaporation, urgent requirements for the regional development of efficient water-saving irrigation and the promotion of digital agriculture should be proposed.
Currently, Earth's climate is undergoing considerable changes characterized by warming. The Sixth Assessment Report of the Intergovernmental Panel on Climate Change states that from 1850–1900, the global average surface temperature increased by about 1 ℃, and it is expected to increase even further by more than 1.5 ℃ in the next 20 years [1]. Global warming affects the earth's hydrological cycle, thus increasing the frequency of abnormal weather patterns [2,3]. The effects of climate change on agriculture and food security are also expected to be substantial, albeit differing across regions and crops. Coupled with the burgeoning global population, there exists an imperative for agricultural adaptation to safeguard forthcoming food security. In China, extreme weather events, such as the strong sandstorms that swept across most of the northern regions in 2021 and the 7.20 Henan torrential rain that took place in the same year, frequently occur [4,5]. In addition, prolonged high-temperature abnormal weather was observed in the upper and middle reaches of the Yangtze River in 2022. Different regions have different response mechanisms to global warming, and these mechanisms involve various climate change characteristics [6]. Therefore, climate variations must be determined at the regional scale.
Linyi is located in the southeast of Shandong Province and has a complex topography. Its northern part is Yimeng Mountain, and its southern part is a vast plain, which is an important commodity grain base in Shandong Province. Against the background of global warming, extreme precipitation occurs frequently in summer in Shandong Province, especially in Linyi City, which is located in southeastern Shandong [7,8]. Extreme climate variation poses serious challenges to the sustainable development of agricultural production in this city.
Numerous studies have been conducted on meteorological changes, especially climate element variations. However, most of them were limited to two main elements, namely, precipitation and temperature. Ren et al. [9] analyzed the characteristics of precipitation and air temperature changes in Shandong Province. Dong et al. [10] examined the trend, mutation, periodicity, and other characteristics of precipitation in Shandong Province in the past 50 years. Cheng et al. [11] predicted future precipitation and temperature through the R/S method by analyzing climate characteristics. Wu et al. [12] investigated the climate change trend and mutation characteristics of Linyi in the past 44 years. Xu et al. [13] studied the periodic changes in temperature and precipitation at different time scales in Linyi and predicted future trends. Only a few of the abovementioned studies in Linyi City considered the evaporation element. Evaporation is a key element for the water and energy cycle, and its long-term trends have become an increasingly important topic in modern hydrology. Increased attention should be devoted to the evaporation element in climate change research.
Various methods of meteorological analysis have been employed in recent studies. For instance, Zhang et al. [14] used ensemble empirical mode decomposition and empirical orthogonal function (EOF) decomposition to analyze the spatiotemporal characteristics of meteorological droughts worldwide from 1901 to 2020. Yetik et al. [15] investigated the precipitation trends in Turkey from 1980 to 2019 using Spearman rank correlation, Mann–Kendall tests, and Sen's slope. They analyzed spatiotemporal variations and identified potential change points. Zhao and Luo [16] utilized wavelet analysis to examine the changes in temperature and precipitation in Dabie Mountain of West Anhui. Compared with the Mann–Kendall test and Sen's slope, linear trend analysis is a simpler calculation method but is equally effective in analyzing trends in meteorological data [17]. EOF analysis can transform a high-dimensional data set into a set of orthogonal patterns, where each pattern represents a different variation pattern of the original data [18]. In this way, the dimensions of the data can be greatly reduced, making it easier to analyze and interpret [19]. Wavelet analysis is relatively flexible in processing signal boundaries, and does not have the problem of spectrum leakage as the traditional Fourier transform, it has a strong ability to analyze non-stationary signals, and can effectively capture the time-varying characteristics of signals, so as to better understand and predict meteorological phenomena [20]. Meteorological data usually have changes on multiple scales, from meteorological events on short time scales to climate changes on long time scales [21]. Wavelet analysis can decompose and analyze data in different time scales, making the analysis results more multi-scale. With the support of meteorological monitoring data (precipitation and temperature data in 1972–2021, especially evaporation data in 1972–2013) from 10 meteorological stations in Linyi City, we analyze the spatiotemporal characteristics of the three major meteorological elements via linear regression analysis, EOF, and the complex-value Morlet wavelet function. We focus on revealing the trends, modes, and periodicity characteristics of the three major meteorological elements to effectively guide regional agricultural production.
Linyi is located in the southeastern part of Shandong Province, China. It borders the Yellow Sea to the east and is crossed by Yi River. Linyi has an area of 17,191.2 km2, and its geographic coordinates are 34°22′–36°13′ N and 117°24′–119°11′ E. It has jurisdiction over three districts, including Lanshan, and nine counties, such as Tancheng. The distribution of meteorological stations in Linyi City and other counties is shown in Figure 1. The terrain in Linyi City is complex and diverse, with high elevations in the northwest and low ones in the southeast. Three major mountain ranges, namely, Yishan, Mengshan, and Nishan, extend northwest to southeast and control the flow of Yi River, Shu River, and their major tributaries. With Yi and Shu Rivers as the center, the city is surrounded by mountains on the west, north, and east, forming a fan-shaped alluvial plain to the south.
The Linyi Meteorological Bureau provides access to a long series of meteorological data at a monthly scale. Given the limitation in the validity of different data, a difference exists in the observed length of the series. The series length of precipitation and evaporation was up to 49 years from 1972 to 2021, whereas that of evaporation was 41 years from 1972 to 2013. The missing information is the annual evaporation of Lanling and Linshu Stations from 1994 to 1998, which accounts for 2.38% of the total evaporation. The moving average interpolation method was used to fill the missing value caused by individual missing values in this series [17], Missing values were replaced by mean values of meteorological data for the same period from bordering stations.
The annual variation of each meteorological element can be expressed by a 1D linear regression model as follows [17]:
x=a+bt, | (1) |
where x is the meteorological variable, t represents time, a is the regression constant, and b is the regression coefficient used to evaluate the trend of each meteorological variable, namely, the tendency rate.
EOF is a commonly used method in meteorological diagnosis that separates temporal and spatial variations. It decomposes the variable field, which changes over time, into a sum of spatial and temporal functions; the former varies with time, and the latter shows the opposite. The main purpose of EOF is to project the normally high-dimensional physical variables x(t) onto a few dominant EOF patterns [18].
Continuous meteorological observation data at various gauges are regarded as an m-dimensional random vector X, where xij is the observation data of the i th gauge station in year j. Meteorological data field X can be decomposed into the linear combination of feature vector matrix V and corresponding time coefficient matrix T as follows [19]:
X=VT=[v11⋯v1n⋮⋱⋮vm1⋯vmn][t11⋯t1n⋮⋱⋮tm1⋯tmn]. | (2) |
A random test is required to verify the random property of the spatial mode in the observation data because any random number or false data will be decomposed into a suite of spatial eigenvectors and principal components. The error range of characteristic roots, Δλ is used to measure the significance. At the 95% confidence level, the error range of a characteristic root can be expressed as follows [20]:
Δλ=λ√2N∗, | (3) |
where λ is the characteristic root and N* is the freedom degree of the data. Each characteristic root λ is inputted to Eq (3) to obtain error range Δλ. If the Δλ caused by two characteristic roots shows some type of overlap before and after, then it fails the significance test.
The time coefficient represents the time variation characteristic of the distribution, which is represented by the feature vector. If the time coefficient is positive, then the same variation direction as that in the spatial mode will be obtained and vice versa. The larger the absolute value of the time coefficient is, the more significant this type of distribution is.
Wavelets can characterize the local features of time series in time and frequency domains, and they have been widely used in meteorological and hydrological studies in recent years [21,22,23,24]. Wavelet transform has been studied for many years and used to achieve a good fit to meteorological time-scale data. In terms of 1D time series, which can be delineated as f(t)∈L2(R), the wavelet transform function Wf(a,b) is the shifted ψ(t) with b displacement, and the inner product between the shifted ψ(t) and the function f(t) is calculated at different scales of a, as expressed in Eq (4). A π/2 bit-phase difference exists between the real and imaginary parts of the complex Morlet wavelet, and it can eliminate the oscillation of the wavelet transform coefficients in real form and separate the mode and phase of the wavelet transform coefficients [25]. The expression of the complex Morlet wavelet is given as:
Wf(a,b)=|a|−12∫+∞−∞f(t)¯ψ(t−ba)dt, | (4) |
where ¯ψ(t)−ψ(t) is the complex conjugate function and Wf(a,b) denotes the wavelet transform coefficients.
ψ(t)=eicte−t22, | (5) |
where c is a constant and i is the imaginary part.
In this study, complex Morlet wavelet transform was utilized to explore the periodic variations of three main meteorological elements in Linyi City. According to wavelet transform theory, the positive or negative magnitudes of the real part of the wavelet coefficients indicate the fluctuations of each meteorological element around the multiyear averages in the wavelet variation domain, which in turn can reflect the energy strength of temporal variation. In the contour map of the mode square, that is, the energy map, the energy of the fluctuating strength of each climate element in the wavelet change domain is described to reveal the fluctuating changes in the energy aggregation center in the time domain.
Wavelet variance is the integrated square of all wavelet coefficients at different scales in the time domain, and it is used to determine the main time scales that exist in a certain time series as follows [25]:
var(a)=∫+∞−∞|Wf(a,b)|2db. | (6) |
The average annual precipitation in Linyi is 809.04 mm, with the south and the north having high and low spatial distributions, respectively (Figure 2). The average annual temperature is 13.65 ℃ and exhibits a general decrease from the southwest to the northeast. The average annual evaporation is 1638.91 mm, which increases with latitude. Linyi City has a temperate monsoon climate with four distinct seasons and sufficient rainfall. Under these climate conditions, the three major elements have undergone considerable spatiotemporal changes because of global warming.
Figure 3 displays the temporal variation curves of the overall annual average of the three major meteorological elements from 10 meteorological stations in Linyi City. The average annual precipitation in Linyi is 809.04 mm. The maximum precipitation (1178.64 mm) occurred in 1974, and it was 2.29 times greater than the minimum precipitation in 2002 (514.92 mm), indicating a large interannual difference in precipitation. A weak upward trend with an annual variation rate of 2.09 mm/a (R2 = 0.03) was observed in the precipitation series. The multiyear average annual temperature was 13.65 ℃; the highest temperature of 14.83 ℃ was recorded in 2017, and the lowest temperature of 12.39 ℃ was recorded in 1980. Temperature exhibited a similar upward trend as precipitation, but its trend was more significant than that of precipitation, with an R2 value of 0.67 and an annual variation rate of 0.04 ℃. By contrast, evaporation showed a downward trend of −6.47 mm/a (R2 = 0.32), which was the complete opposite of the trend of precipitation. The highest evaporation value of 2007.01 mm occurred in 1978, and the lowest value of 1313.99 mm was found in 2003.
The analysis above revealed that precipitation and temperature showed an increasing trend, whereas evaporation presented a decreasing trend. Table 1 shows considerable differences in the magnitude of the trends among stations, indicating a lack of spatial homogeneity. Temperature exhibited the strongest spatial homogeneity among the three meteorological elements, and the spatial heterogeneity in the evaporation series was the highest. Precipitation showed moderate spatial heterogeneity, and the largest decrease was observed in Mengyin County, where the annual variation rate was 1.76 times that of the regional trend. Pingyi, Linshu, and Feixian had the lowest precipitation trend among the counties. The minimum value was 1.14 mm/a, and the maximum value was only 1.16 mm/a, accounting for only 55.50% of the annual change rate of the regional trend.
Areas | Precipitation variation rate (mm/a) | Temperature variation rate (℃/a) | Evaporation variation rate (mm/a) |
Mengyin | 3.67 | 0.04 | −8.04 |
Pingyi | 1.14 | 0.04 | −11.89 |
Feixian | 1.16 | 0.04 | −8.41 |
Yishui | 2.26 | 0.04 | −7.80 |
Yinan | 2.22 | 0.03 | −8.48 |
Linyi | 2.83 | 0.04 | −3.14 |
Junan | 1.37 | 0.05 | −6.19 |
Lanling | 2.62 | 0.04 | −9.71 |
Linshu | 1.14 | 0.04 | −0.75 |
Tancheng | 2.53 | 0.04 | −0.32 |
As shown in Figure 4 and Table 2, the cumulative contribution rate of the first two modes in the annual precipitation field in Linyi City is about 84%. Thus, the first and second modes are suitable for expressing the spatial distribution characteristics of precipitation in Linyi City. The first mode had a variance contribution rate of 75% (Table 1), which was considerably higher than the variance contribution rates of the other modes. It was the main distribution form of precipitation in this region (Figure 4(a)). The first mode's characteristic vectors were all positive, indicating consistent precipitation changes in Linyi City. The increasing trend of the first-mode eigenvalues from southwest to northeast revealed a considerable precipitation fluctuation in northeastern Linyi City, with the highest value (indicating the greatest annual precipitation variation) being found in Yinan County.
Model | Precipitation | Temperature | Evaporation |
1 | 75% | 96% | 70% |
2 | 9% | 1% | 12% |
3 | 4% | 1% | 7% |
4 | 3% | 0.6% | 3% |
Given the dominance of the first mode of the precipitation field, excessive precipitation appeared in the entire region in 16 years, and it was concentrated in the latter half of the study period, especially after 2002. In the period between 2002 and 2021, excessive precipitation was observed for about nine years, accounting for 45% of the total for this period. Additionally, insufficient precipitation was observed in 20 years. The precipitation was concentrated mostly in the first half of the study period (13 years) in the years 1976–2001, resulting in a frequency of 46%. Between 2002 and 2021, this mode occurred only seven times. Under the dominance of the first mode, the precipitation in Linyi City was mainly characterized by insufficient precipitation at the early stages and excessive precipitation at the late stages, which resulted in an increasing tendency in regional precipitation over time. This conclusion can be verified by the upward trend of the first-mode time coefficient, which was consistent with the overall upward trend of regional precipitation obtained by the linear trend analysis.
The variance contribution of the second mode was 9%, which represents a secondary spatiotemporal distribution pattern of precipitation in Linyi City, as shown in Figure 4(b). A southeast-northwest variation was observed, with the characteristic vectors showing positive and negative values that are contrary to the dominant variation direction of the first mode. The northern region of Feixian and Yinan Counties had positive values, whereas the south region of Lanling and Linyi Counties had negative ones. This distribution mode indicated a heterogeneous precipitation pattern in Linyi City, with minimal precipitation in the southeast and abundant precipitation in the northwest or abundant precipitation in the southeast and minimal precipitation in the northwest. The mode of minimal precipitation in the southeast and abundant precipitation in the northwest occurred seven times; five occurred before 2000, and only 2 happened in 2009 and 2011. The distribution pattern of abundant precipitation in the southeast and minimal precipitation in the northwest was mainly observed in seven years, four of which were before 2000 (1972, 1979, 1982, and 1987) and three of which were after 2000 (2000, 2013, and 2018). We found that the jumping characteristic had little influence on the overall increasing trend of precipitation in the region regardless of the mode.
The variance contribution of the first mode in the temperature field was as high as 96%, which showed the dominant temperature distribution form in Linyi City (Figure 4(c)). The characteristic vectors of the first mode were all positive, indicating a strong consistency in the temperature variation trends. This result is consistent with the previous conclusion on the highest spatial homogeneity of temperature variation. The lowest value of the characteristic vector of the first mode, namely, 0.26, was found in Yinan County, and the highest value of 0.38 was in Junan County. The difference between the two regions was only about 0.12, which confirms the spatiotemporal consistency in the temperature variation of Linyi City. A remarkably increasing trend was found in the time coefficients of the first mode (Figure 5), and it was consistent with the interannual variation trend of annual mean temperature. During the early study period, 75.86% of the years before 2000 exhibited low temperatures across the region. However, after 2000, 85.71% of the years showed high temperatures throughout the period.
The first two modes of the evaporation field passed the north test, and the cumulative variance contribution rate reached 82%, which could characterize the spatial distribution of evaporation in most areas of Linyi City. The variance contribution of the first mode was 69.73%, and the characteristic vectors had both positive and negative values (Figure 4(d)). The evaporation trends in all the regions, except for Junan, showed positive values. The relatively low values in the central region showed a slight fluctuation in evaporation. Dominated by the first mode, 16 years experienced a spatial distribution of abundant evaporation in Junan County and minimal evaporation in the other regions. This phenomenon was observed before 2002, indicating an occurrence frequency of about 51.61%. The opposite spatial distribution only appeared 15 times, with a frequency that was less than that of the former mode. This characteristic was concentrated in the late study period and considerably influenced the overall trend of regional evaporation.
The second mode of evaporation had a variance contribution rate of 12% (Figure 4(e)). The characteristic vector in the time coefficient of this mode had positive and negative values. The positive values were mainly observed in the central and western regions, and the negative values were found in the northeastern and southeastern regions. The mode of minimal evaporation in central and western Linyi occurred in seven years. However, the opposite was mainly observed after 2000, specifically in 2004, 2005, 2006, and 2009. Therefore, according to the two models, an overall increasing trend of evaporation occurred in Linyi City.
Linyi City had a 10–20-year-scale interdecadal oscillation and a 3–7 year scale interannual oscillation in precipitation. It also had a relatively weak oscillation center on the 27-year scale that was limited to local time scales (Figures 6 and 7(a)). The 10–20-year-scale interdecadal oscillation was strong (the peak value of wavelet variance reached 42), with the scale center being at 16 years and the modular square of the wavelet transformation coefficient reaching a peak value of 2.0. However, its influence could only be seen in the limited 30 years from 1992 to 2021. Influenced by such periodic variation at this scale, Linyi City showed a season of wet, dry, wet, dry, and wet again. After a short-term increase in the last wet season, the precipitation in Linyi City exhibited a decreasing variation, which was above the multiyear average. The 3–7 year scale of the interannual period showed a center of 5 years and a relatively low level of oscillation energy, with the maximum value of the wavelet coefficient modular square being only 0.8 (Figure 7(a)). The periodic variation of precipitation at this scale was observed in the whole study period, which could be indirectly proven by the wavelet variance's peak value of 24. However, a weakening oscillation trend was observed over time. The abundance and depletion of precipitation variation in Linyi City at this scale is shown in Figure 7(a), (d). The synthetic analysis of the periodic variation of precipitation in Linyi City showed an increasing trend at the decadal scale, which could be soon replaced by a period of decreasing precipitation at the medium and short time scales. Although the amount of precipitation is higher than the multiyear average, the severe reduction trend poses a major challenge to the economic development of Linyi City. The reason is that water consumption in agriculture accounts for more than 70% of the total water consumption of this major agricultural city. This situation is expected to worsen under the current agricultural planting structure involving the sustained growth of water-intensive crops, such as vegetables and fruits. Promoting regional water-saving irrigation development can effectively address this challenge.
From 1972 to 2021, the temperature in Linyi City exhibited a decadal oscillation, with a 24-year scale as the dominant center; this oscillation in temperature weakened compared with that in precipitation, and the peak value of wavelet variance decreased from 42 to 3 (Figure 8). Two weak cyclic oscillations were also observed at the scales of 3–7 and 10–20 years. The modulus square of the wavelet coefficient with a 24-year scale as the decadal cyclic center reached 1.2. Determined by the periodic variation at the 24-year scale, the temperature in Linyi City showed a periodic variation of high, low, high, low, and high over time, and a strengthened trend was observed at this scale of periodic variation (Figure 8). In 2021, one-quarter of a cycle was completed at this scale, and the temperature gradually decreased from a value above the long-term average to one below it. The 3–7 years cyclic oscillation with 5 years as the scale center showed not only weak oscillation energy but also a decreasing tendency with time. The maximum energy center of this scale was only 0.64, which was mainly concentrated in the period from 1972 to 2009. The 10–20 years cyclic oscillation had a scale center of 16 years and mainly appeared at the early stage of the study period (1972–1987). The maximum energy center at this scale was only 0.46, which was only 37.10% of that at the 24-year scale (1.24; Figure 7(b), (e)). Therefore, the short-term temperature could, to a certain degree, alleviate the water consumption stress of agricultural production caused by short-term precipitation reduction; however, it could not invert the global warming-related heat stress exerted on agricultural production.
The three dominant periodic oscillations in evaporation in Linyi had scales of 6, 16, and 31 years. Although the 42-year scale also showed high wavelet variance, due to the limitation in the length of the study period, the periodic variation at this scale has some uncertainty (Figure 9). A remarkable 31-year-scale decadal oscillation was observed throughout the entire region and had a wavelet variance of 37, which was only 6 lower than the maximum value for precipitation. The periodic oscillations of evaporation in Linyi City at the scale of 31 years are shown in Figure 9, which predicts that the evaporation in Linyi City will continue to increase in the next 15 years. The oscillating energy of the 16-year scale was higher than that of the 6-year scale, but both were far lower than that of the 31-year scale. In addition, the periodic oscillation of the 16-year scale showed a strengthening tendency over the whole study period, whereas that of the 6-year scale only manifested from 1972 to 2010 and exhibited a weakening tendency over time (Figure 7(c), (f)). In the short term, the evaporation in Linyi City will continue to increase, accompanied with a decreasing tendency in precipitation in the coming period, which can result in poor soil moisture. Thus, agricultural production will be affected in terms of survival, income, and ecology. The promotion of water-saving irrigation strategies and the development of digital agriculture are urgently needed.
Complex Morlet wavelet transform revealed that the main time scales of the periodic oscillations in precipitation, temperature, and evaporation in most stations were consistent with those of the regional overall characteristics and will not be explained any more. Only the difference is discussed here. Specifically, Pingyi Station showed a decadal periodic oscillation in precipitation centered at a 35-year scale, whereas Mengyin and Yinan Stations exhibited a decadal periodic oscillation in temperature at a 10-year scale. Remarkable 42-year periodic oscillations in evaporation were also observed in the multiple stations.
The trend of annual precipitation and temperature changes is consistent with the findings of Yan et al. [26], and other researchers [27,28]. Temperature variability is more stable than precipitation variability, which is in line with Sun's research on this basin [29]. High temperatures can cause rapid surface and water evaporation, resulting in increased evaporation. In contrast to the research in Linyi City, we found a different phenomenon involving a decreasing trend in evaporation, namely, the evaporation paradox [30]. This phenomenon exists in most regions of Linyi City. The decrease in evaporation is mainly due to a considerable decrease in the energy available for evaporation [31]. This reduction is caused by the increase in vapor pressure, decrease in wind speed, and decrease in solar radiation, which compensate for the increase in evaporation caused by rising temperatures [32].
The spatial distributions of the first modes of precipitation and temperature in Linyi City were consistent because of the influence of large-scale weather systems. Linyi City is situated in a warm temperate monsoon climate zone, which is influenced by the subtropical high in summer and the prevailing westerly winds in winter [33]. In addition to the dominant mode of precipitation, a second mode with a southeast–northwest phase difference distribution was presented, and it was mainly influenced by terrain. Warm and humid airflow from the ocean enters the central and southern low hills of Shandong Province and then moves northward, leading to differences in precipitation in different terrain areas of Linyi City. The heterogeneity of the evaporation of different modes is due to various local factors, including wind speed, radiation, sunshine duration, relative humidity, temperature, and precipitation [31,34], and the complex topography and vegetation cover of Linyi City exacerbate this variability [31]. The spatiotemporal analysis of EOF revealed that Yinan County experiences considerable interannual fluctuations in precipitation, and Junan County differs greatly from other areas in terms of evaporation. Therefore, further attention should be given to the small-scale climate changes in these areas to ensure stable crop yields and increased income in practical agricultural production.
The annual precipitation in Linyi exhibited a dominant cycle of 16 years. This finding is similar to the conclusion of Ding et al. [35], that is, the annual average precipitation in Linyi City oscillates violently around a 15-year period. The 27-year variation in the annual average temperature is consistent with the conclusions of Xu et al. [13]. Under the dominant short-term variation of the three meteorological elements, precipitation and temperature are expected to decline in the next five years, with their values being above their long-term averages, whereas evaporation is expected to increase to a value that is higher than the long-term average of Linyi City. Therefore, water-saving irrigation strategies need to be developed, and digital agriculture must be actively promoted for the short-term development of regional agriculture [36].
Our findings can serve as a foundation for guiding local agricultural practices in Linyi and similar areas. Additionally, the results indicate that Linyi will experience a relatively dry situation in near future. In order to mitigate the effects of drought, agriculturalists should select more drought-tolerant varieties during the early stages of planting. Relevant organizations can also investigate more suitable varieties for the current climate change situation. The government can implement effective water resource management measures, including the construction of reservoirs, waterways, and irrigation systems, to ensure the supply of water for agricultural use and mitigate the adverse effects of drought on agricultural production. Additionally, proactive efforts can be made to promote digital agriculture, facilitating precise monitoring and regulation, thereby enhancing agricultural efficiency and quality, and improving resilience to climate change and drought.
We investigated the spatiotemporal variations of three meteorological variables (precipitation, temperature, and evaporation) in Linyi City, one of the largest agricultural cities in China, over the past 50 years. The trends, spatiotemporal modes, and multitime scale characteristics of the three elements were determined using the methods of linear regression analysis, EOF, and Morlet wavelet transformation. Our results of this study can provide a theoretical reference for the efficient utilization of climate resources in the future development of regional agricultural production. The major conclusions are as follows:
1) The annual averages of precipitation and temperature in Linyi City increased at the rates of 2.09 mm/a and 0.04 ℃/a, respectively. Conversely, evaporation decreased (−6.47 mm/a) because of a considerable reduction in the energy available for evaporation, which is known as the evaporation paradox. Given the irreversible trends of global warming and the development of water-consuming agriculture, improving the efficient use of climate and water resources is essential for future agricultural production of this region.
2) Precipitation exhibited two main modes. The first mode was dominated by a consistent increasing trend in the early period and a consistent decreasing trend in the late period. The second mode was characterized by a southeast–northwest phase difference. The interannual variability of precipitation was highly significant in Yinan County. Temperature showed a consistent warming trend across the region. Evaporation also exhibited two major modes. The first one was dominated by a spatial distribution pattern that was different from the spatial distribution patterns of the other regions, especially in Junan County, and exhibited a trend of increasing evaporation in Junan County in the late period and decreasing evaporation in the other regions. The second mode showed an east–west difference. Therefore, attention should be given to local climate changes in Yinan and Junan Counties to ensure stable and increased agricultural production in the two areas.
3) The three meteorological variables exhibited interannual and interdecadal variations over short-term (5 years), medium-term (16 years), and long-term (25 years) periods. Precipitation showed a dominant period of 16 years, temperature of 24 years, and evaporation of 31 years. In the short term, precipitation and temperature in Linyi City are expected to fall below the multiyear average, while evaporation is expected to rise above the multiyear average. The projections showed a reversal of the historical trend, with a decrease in sources and an increase in sinks of atmospheric water. The continuous growth of water-intensive crops in Linyi City, a large agricultural city where agriculture consumes more than 70% of water, poses serious challenges to its agriculture development. The demand for water resources is increasing. Therefore, promoting efficient water-saving irrigation and advancing digital agriculture are essential for the future development of Linyi City.
Many questions related to this study require further in-depth research. For example, how can the conclusions of our study guide local agricultural practices, and how can the evaporation paradox phenomenon be used to manage local water resources for agricultural development? Further research will be conducted in the future.
The authors declare they have not used Artificial Intelligence (AI) tools in the creation of this article.
This study was supported by the Key Projects of Shandong Meteorological Bureau (2020sdqxz09) and Guiding Projects of Key Laboratory of Meteorological Disaster Prevention and Mitigation in Shandong Province (2021SDYD29).
The authors declare there are no conflicts of interest.
[1] |
Y. Wang, A. Wang, J. Zhai, H. Tao, T. Jiang, B. Su, et al., Tens of thousands additional deaths annually in cities of China between 1.5 ℃ and 2.0 ℃ warming, Nat. Commun., 10 (2019), 3376. https://doi.org/10.1038/s41467-019-11283-w doi: 10.1038/s41467-019-11283-w
![]() |
[2] |
Z. Zhou, Y. Ding, H. Shi, H. Cai, Q. Fu, S. Liu, et al., Analysis and prediction of vegetation dynamic changes in China: Past, present and future, Ecol. Indic., 117 (2020), 106642. https://doi.org/10.1016/j.ecolind.2020.106642 doi: 10.1016/j.ecolind.2020.106642
![]() |
[3] |
W. Yang, L. Zhang, Z. Yang, Spatiotemporal characteristics of droughts and floods in Shandong Province, China and their relationship with food loss, Chin. Geogr. Sci., 33 (2023), 304–319. https://doi.org/10.1007/s11769-023-1338-0 doi: 10.1007/s11769-023-1338-0
![]() |
[4] | Y. Tang, W. Cai, J. Zhai, S. Wang, Y. Liu, Y. Chen, et al., Climatic anomalous features and major meteorological disasters in China in summer of 2021 (in Chinese), Arid Meteorol., 40 (2022), 179–186. |
[5] |
Z. Shu, W. Li, J. Zhang, J. Jin, Q. Xue, Y. Wang, et al., Historical changes and future trends of extreme precipitation and high temperature in China, Chin. J. Eng. Sci., 24 (2022), 116–125. https://doi.org/10.15302/j-sscae-2022.05.014 doi: 10.15302/j-sscae-2022.05.014
![]() |
[6] | B. Li, X. Shi, L. Lian, Y. Chen, Z. Chen, X. Sun, Quantifying the effects of climate variability, direct and indirect land use change, and human activities on runoff, J. Hydrol., 584 (2020), 124684. https://doi.org/10.1016/j.jhydrol.2020.124684 |
[7] | Y. Liu, M. You, J. Zhu, F. Wang, R. Ran, Integrated risk assessment for agricultural drought and flood disasters based on entropy information diffusion theory in the middle and lower reaches of the Yangtze River, China, Int. J. Disaster Risk Reduct., 38 (2019), 101194. https://doi.org/10.1016/j.ijdrr.2019.101194 |
[8] |
Y. Li, H. Li, D. Li, Z. Zhang, Y. Feng, Construction of rural water ecological civilization index system in China, Water Pract. Technol., 15 (2020), 797–806. https://doi.org/10.2166/wpt.2020.064 doi: 10.2166/wpt.2020.064
![]() |
[9] |
J. Ren, S. Gu, C. Feng, X. Lu, Analysis of spatial and temporal variability of temperature and precipitation in Shandong Province in the context of climate change (in Chinese), Water Resour. Hydropower Eng., 54 (2023), 27–37. https://doi.org/10.13928/j.cnki.wrahe.2023.05.003 doi: 10.13928/j.cnki.wrahe.2023.05.003
![]() |
[10] | X. Dong, W. Gu, X. Meng, H. Liu, Characteristics of changes in precipitation events in Shandong Province in the past 50 years (in Chinese), Acta Geogr. Sin., 69 (2014), 661–671. |
[11] | Z. Cheng, B. Lu, H. Wang, S. Xiong, Y. Hu, Analysis and prediction of climate elements change characteristics in Shandong region (in Chinese), Water Resour. Power, 35 (2017), 10–14. |
[12] |
W. Wu, X. Tang, N. Guo, C. Yang, H. Liu, Y. Shang, Spatiotemporal modeling of monthly soil temperature using artificial neural networks, Theor. Appl. Climatol., 113 (2013), 481–494. https://doi.org/10.1007/s00704-012-0807-7 doi: 10.1007/s00704-012-0807-7
![]() |
[13] | L. Xu, P. Zhang, C. Wang, K. Wang, Wavelet analysis and trend prediction of temperature change in Linyi City (in Chinese), Agric. Technol., 38 (2018), 120–123. |
[14] |
R. Zhang, Y. Qu, X. Zhang, X. Wu, X. Zhou, B. Ren, et al., Spatiotemporal variability in annual drought severity, duration, and frequency from 1901 to 2020, Clim. Res., 87 (2022), 81–97. https://doi.org/10.3354/cr01680 doi: 10.3354/cr01680
![]() |
[15] |
A. K. Yetik, B. Arslan, B. Şen, Trends and variability in precipitation across Turkey: A multimethod statistical analysis, Theor. Appl. Climatol., 155 (2024), 473–488. https://doi.org/10.1007/s00704-023-04645-4 doi: 10.1007/s00704-023-04645-4
![]() |
[16] |
Y. Zhao, Y. Luo, Wavelet analysis on temperature and precipitation changes in Dabie Mountain of West Anhui, J. Phys. Conf. Ser., 1732 (2021), 012105. https://doi.org/10.1088/1742-6596/1732/1/012105 doi: 10.1088/1742-6596/1732/1/012105
![]() |
[17] |
B. Song, K. Park, Temperature trend analysis associated with land-cover changes using time-series data (1980–2019) from 38 weather stations in South Korea, Sustainable Cities Soc., 65 (2021), 102615. https://doi.org/10.1016/j.scs.2020.102615 doi: 10.1016/j.scs.2020.102615
![]() |
[18] |
G. Bürger, Intraseasonal oscillation indices from complex EOFs, J. Clim., 34 (2021), 107–122. https://doi.org/10.1175/jcli-d-20-0427.1 doi: 10.1175/jcli-d-20-0427.1
![]() |
[19] |
X. Chen, J. M. Wallace, K. K. Tung, Pairwise-rotated EOFs of global SST, J. Clim., 30 (2017), 5473–5489. https://doi.org/10.1175/jcli-d-16-0786.1 doi: 10.1175/jcli-d-16-0786.1
![]() |
[20] |
G. R. North, T. L. Bell, R. F. Cahalan, F. J. Moeng, Sampling errors in the estimation of empirical orthogonal functions, Mon. Weather Rev., 110 (1982), 699–706. https://doi.org/10.1175/1520-0493(1982)110<0699:seiteo>2.0.co;2 doi: 10.1175/1520-0493(1982)110<0699:seiteo>2.0.co;2
![]() |
[21] |
Q. Li, P. He, Y. He, X. Han, T. Zeng, G. Lu, et al., Investigation to the relation between meteorological drought and hydrological drought in the upper Shaying River Basin using wavelet analysis, Atmos. Res., 234 (2020), 104743. https://doi.org/10.1016/j.atmosres.2019.104743 doi: 10.1016/j.atmosres.2019.104743
![]() |
[22] | V. Cheng, A. Saber, C. A. Arnillas, A. Javed, A. Richards, G. B. Arhonditsis, Effects of hydrological forcing on short- and long-term water level fluctuations in Lake Huron-Michigan: A continuous wavelet analysis. J. Hydrol., 603 (2021), 127164. https://doi.org/10.1016/j.jhydrol.2021.127164 |
[23] |
E. Lee, S. Kim, Wavelet analysis of soil moisture measurements for hillslope hydrological processes, J. Hydrol., 575 (2019), 82–93. https://doi.org/10.1016/j.jhydrol.2019.05.023 doi: 10.1016/j.jhydrol.2019.05.023
![]() |
[24] |
H. Xu, B. Lei, Z. Li, A reconstruction of total solar irradiance based on wavelet analysis, Earth Space Sci., 8 (2021), e2021EA001819. https://doi.org/10.1029/2021ea001819 doi: 10.1029/2021ea001819
![]() |
[25] |
T. Guo, T. Zhang, E. Lim, M. Lopez-Benitez, F. Ma, L. Yu, A review of wavelet analysis and its applications: Challenges and opportunities, IEEE Access, 10 (2022), 58869–58903. https://doi.org/10.1109/ACCESS.2022.3179517 doi: 10.1109/ACCESS.2022.3179517
![]() |
[26] | Z. Yan, S. Wang, D. Ma, B. Liu, H. Lin, S. Li, Meteorological factors affecting pan evaporation in the Haihe River Basin, China, Water, 11 (2019), 317. https://doi.org/10.3390/w11020317 |
[27] | T. Gao, X. Shi, Spatio-temporal characteristics of extreme precipitation events during 1951–2011 in Shandong, China and possible connection to the large scale atmospheric circulation, Stochastic Environ. Res. Risk Assess., 30 (2016), 1421–1440. https://doi.org/10.1007/s00477-015-1149-7 |
[28] |
H. Li, S. Liu, M. Yin, L. Zhu, E. Shen, B. Sun, et al., Spatial and temporal variability and risk assessment of regional climate change in northern China: A case study in Shandong Province, Nat. Hazard., 111 (2022), 2749–2786. https://doi.org/10.1007/s11069-021-05156-z doi: 10.1007/s11069-021-05156-z
![]() |
[29] | P. Sun, W. Qu, X. Zhu, Y. Wu, J. Wang, B. Zhang, et al., Variation of hydrothermal pattern of Huai River Basin from 1959 to 2018, (in Chinese), Resour. Environ. Yangtze Basin, 30 (2021), 1366–1377. |
[30] | J. Hu, G. Zhao, P. Li, X. Mu, Variations of pan evaporation and its attribution from 1961 to 2015 on the Loess Plateau, China, Nat. Hazard., 111 (2022), 1199–1217. https://doi.org/10.1007/s11069-021-05091-z |
[31] |
Z. Liu, L. Zhangzhong, W. Zheng, X. Zhang, J. Yu, F. Zhang, et al., Analysis of the variation tendency and influencing factors of reference evapotranspiration in Shandong Province, China, Irrig. Drain., 72 (2023), 390–407. https://doi.org/10.1002/ird.2776 doi: 10.1002/ird.2776
![]() |
[32] |
M. Li, R. Chu, S. Shen, A. Islam, Quantifying climatic impact on reference evapotranspiration trends in the Huai River Basin of Eastern China, Water, 10 (2018), 144. https://doi.org/10.3390/w10020144 doi: 10.3390/w10020144
![]() |
[33] |
X. Huang, M. Liu, R. Chen, S. Gao, Q. Xie, Q. Feng, Evaluation and spatiotemporal characteristics of atmospheric environment efficiency in Shandong province based on super-SBM model, Environ. Res. Commun., 3 (2021), 115002. https://doi.org/10.1088/2515-7620/ac3409 doi: 10.1088/2515-7620/ac3409
![]() |
[34] |
X. Yan, A. Mohammadian, R. Ao, J. Liu, X. Chen, Spatiotemporal variations in reference evapotranspiration and its contributing climatic variables at various spatial scales across China for 1984–2019, Water, 14 (2022), 2502. https://doi.org/10.3390/w14162502 doi: 10.3390/w14162502
![]() |
[35] | W. Ding, X. Zhao, K. Wang, Z. Xu, Y. Zhang, D. Wu, et al., Analysis on precipitation change characteristics in Linyi City from 1951 to 2016 (in Chinese), Mod. Agric. Sci. Technol., 10 (2018), 205–206+211. |
[36] |
J. A. J. Mendes, N. G. P. Carvalho, M. N. Mourarias, C. B. Careta, V. G. Zuin, M. C. Gerolamo, Dimensions of digital transformation in the context of modern agriculture, Sustain. Prod. Consum., 34 (2022), 613–637. https://doi.org/10.1016/j.spc.2022.09.027 doi: 10.1016/j.spc.2022.09.027
![]() |
1. | Li Li, Xiaoning Lu, Wu Jun, Correction: Spatiotemporal variation of the major meteorological elements in an agricultural region: A case study of Linyi City, Northern China, 2024, 32, 2688-1594, 3313, 10.3934/era.2024152 |
Areas | Precipitation variation rate (mm/a) | Temperature variation rate (℃/a) | Evaporation variation rate (mm/a) |
Mengyin | 3.67 | 0.04 | −8.04 |
Pingyi | 1.14 | 0.04 | −11.89 |
Feixian | 1.16 | 0.04 | −8.41 |
Yishui | 2.26 | 0.04 | −7.80 |
Yinan | 2.22 | 0.03 | −8.48 |
Linyi | 2.83 | 0.04 | −3.14 |
Junan | 1.37 | 0.05 | −6.19 |
Lanling | 2.62 | 0.04 | −9.71 |
Linshu | 1.14 | 0.04 | −0.75 |
Tancheng | 2.53 | 0.04 | −0.32 |
Model | Precipitation | Temperature | Evaporation |
1 | 75% | 96% | 70% |
2 | 9% | 1% | 12% |
3 | 4% | 1% | 7% |
4 | 3% | 0.6% | 3% |
Areas | Precipitation variation rate (mm/a) | Temperature variation rate (℃/a) | Evaporation variation rate (mm/a) |
Mengyin | 3.67 | 0.04 | −8.04 |
Pingyi | 1.14 | 0.04 | −11.89 |
Feixian | 1.16 | 0.04 | −8.41 |
Yishui | 2.26 | 0.04 | −7.80 |
Yinan | 2.22 | 0.03 | −8.48 |
Linyi | 2.83 | 0.04 | −3.14 |
Junan | 1.37 | 0.05 | −6.19 |
Lanling | 2.62 | 0.04 | −9.71 |
Linshu | 1.14 | 0.04 | −0.75 |
Tancheng | 2.53 | 0.04 | −0.32 |
Model | Precipitation | Temperature | Evaporation |
1 | 75% | 96% | 70% |
2 | 9% | 1% | 12% |
3 | 4% | 1% | 7% |
4 | 3% | 0.6% | 3% |