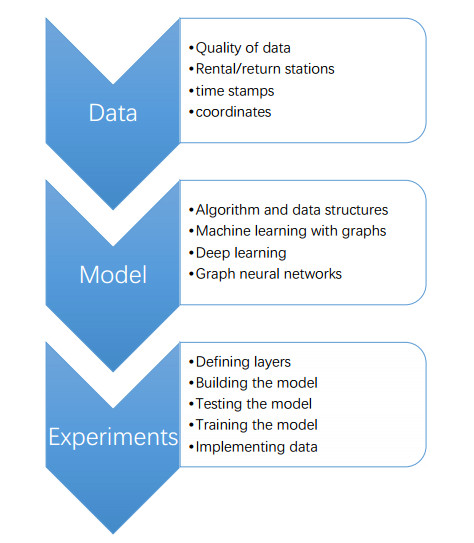
Bike-sharing systems are widely operated in many cities as green transportation means to solve the last mile problem and reduce traffic congestion. One of the critical challenges in operating high-quality bike-sharing systems is rebalancing bike stations from being full or empty. However, the complex characteristics of spatiotemporal dependency on usage demand may lead to difficulties for traditional statistical models in dealing with this complex relationship. To address this issue, we propose a graph-based neural network model to learn the representation of bike-sharing demand spatial-temporal graph. The model has the ability to use graph-structured data and takes both spatial- and temporal aspects into consideration. A case study about bike-sharing systems in Nanjing, a large city in China, is conducted based on the proposed method. The results show that the algorithm can predict short-term bike demand with relatively high accuracy and low computing time. The predicted errors for the hourly station level usage demand prediction are often within 20 bikes. The results provide helpful tools for short-term usage demand prediction of bike-sharing systems and other similar shared mobility systems.
Citation: Ruo Jia, Richard Chamoun, Alexander Wallenbring, Masoomeh Advand, Shanchuan Yu, Yang Liu, Kun Gao. A spatio-temporal deep learning model for short-term bike-sharing demand prediction[J]. Electronic Research Archive, 2023, 31(2): 1031-1047. doi: 10.3934/era.2023051
[1] | Gang Cheng, Yadong Liu . Hybrid short-term traffic flow prediction based on the effect of non-linear sequence noise. Electronic Research Archive, 2024, 32(2): 707-732. doi: 10.3934/era.2024034 |
[2] | Zhiyuan Feng, Kai Qi, Bin Shi, Hao Mei, Qinghua Zheng, Hua Wei . Deep evidential learning in diffusion convolutional recurrent neural network. Electronic Research Archive, 2023, 31(4): 2252-2264. doi: 10.3934/era.2023115 |
[3] | Ju Wang, Leifeng Zhang, Sanqiang Yang, Shaoning Lian, Peng Wang, Lei Yu, Zhenyu Yang . Optimized LSTM based on improved whale algorithm for surface subsidence deformation prediction. Electronic Research Archive, 2023, 31(6): 3435-3452. doi: 10.3934/era.2023174 |
[4] | Wenhui Feng, Yuan Li, Xingfa Zhang . A mixture deep neural network GARCH model for volatility forecasting. Electronic Research Archive, 2023, 31(7): 3814-3831. doi: 10.3934/era.2023194 |
[5] | Xite Yang, Ankang Zou, Jidi Cao, Yongzeng Lai, Jilin Zhang . Systemic risk prediction based on Savitzky-Golay smoothing and temporal convolutional networks. Electronic Research Archive, 2023, 31(5): 2667-2688. doi: 10.3934/era.2023135 |
[6] | Xianfei Hui, Baiqing Sun, Indranil SenGupta, Yan Zhou, Hui Jiang . Stochastic volatility modeling of high-frequency CSI 300 index and dynamic jump prediction driven by machine learning. Electronic Research Archive, 2023, 31(3): 1365-1386. doi: 10.3934/era.2023070 |
[7] | Xia Liu, Liwan Wu . FAGRec: Alleviating data sparsity in POI recommendations via the feature-aware graph learning. Electronic Research Archive, 2024, 32(4): 2728-2744. doi: 10.3934/era.2024123 |
[8] | Tej Bahadur Shahi, Cheng-Yuan Xu, Arjun Neupane, William Guo . Machine learning methods for precision agriculture with UAV imagery: a review. Electronic Research Archive, 2022, 30(12): 4277-4317. doi: 10.3934/era.2022218 |
[9] | Boshuo Geng, Jianxiao Ma, Shaohu Zhang . Ensemble deep learning-based lane-changing behavior prediction of manually driven vehicles in mixed traffic environments. Electronic Research Archive, 2023, 31(10): 6216-6235. doi: 10.3934/era.2023315 |
[10] | Nihar Patel, Nakul Vasani, Nilesh Kumar Jadav, Rajesh Gupta, Sudeep Tanwar, Zdzislaw Polkowski, Fayez Alqahtani, Amr Gafar . F-LSTM: Federated learning-based LSTM framework for cryptocurrency price prediction. Electronic Research Archive, 2023, 31(10): 6525-6551. doi: 10.3934/era.2023330 |
Bike-sharing systems are widely operated in many cities as green transportation means to solve the last mile problem and reduce traffic congestion. One of the critical challenges in operating high-quality bike-sharing systems is rebalancing bike stations from being full or empty. However, the complex characteristics of spatiotemporal dependency on usage demand may lead to difficulties for traditional statistical models in dealing with this complex relationship. To address this issue, we propose a graph-based neural network model to learn the representation of bike-sharing demand spatial-temporal graph. The model has the ability to use graph-structured data and takes both spatial- and temporal aspects into consideration. A case study about bike-sharing systems in Nanjing, a large city in China, is conducted based on the proposed method. The results show that the algorithm can predict short-term bike demand with relatively high accuracy and low computing time. The predicted errors for the hourly station level usage demand prediction are often within 20 bikes. The results provide helpful tools for short-term usage demand prediction of bike-sharing systems and other similar shared mobility systems.
The urban transportation systems are under more pressure than ever, with more people living in urban areas, higher travel demand, congestion, and increased emissions. Developing sustainable transportation to reduce traffic-related issues is an urgent and indispensable trend. One of the emerging sustainable transportation systems is shared mobility, such as ride-hailing, shared e-scooter, and bike/e-bikes. Shared mobility enables the sharing of different types of vehicles and increases the usage efficiency of vehicles. Meanwhile, active mobility sharing such as bike, e-bike, and e-scooter sharing are environmental alternatives compared to private cars and are very prevalent in current European countries. One of the fundamental components of operating shared mobility is accurate demand prediction in spatial and temporal dimensions, which are critical inputs for vehicle dispatching and rebalancing. Thanks to the digitalization of transportation systems, big data regarding the usage demand in different periods and areas in a city are collected. Meanwhile, advancements in artificial intelligence and machine learning have paved the way for new techniques to leverage the great power of data-driven methods and big data for precise travel demand prediction of shared mobility systems [1].
In recent years, as regarded as an environmentally friendly travel mode, shared bikes have been popular in urban public transit. The bike-sharing systems (BSS) bring great convenience in resolving the "last mile" problem for the public transport system. As a shared mobility mode, the users have the option to pick up a bike from a bike-sharing station and return it to another station near where they're going. However, due to the unbalanced usage of bike sharing, the spatio-temporal disparity has occurred, and resulted in a shortage of shared-bike for some areas and excess in others, thus reducing user satisfaction. To solve this unbalanced bike-sharing distribution problem, it is vital to propose an accurate demand prediction model. Most existing studies focus on predicting passenger demand originating from each station [2]. However, there are only a few attempts toward spatial and temporal differences predictions. To overcome the challenge, this paper proposes a deep-learning framework to simultaneously predict bike-sharing demand in each station. First, we construct multiple station graphs in which each station is viewed as a node. The adjacent matrices of nodes are established to represent different aspects of relationships among the bike-sharing station, such as neighborhood, distance, functional similarity, historical demand correlations, and so on. Second, to capture both spatial and temporal correlations, we use a graph convolutional network to capture the spatial correlations among stations in different time intervals and a regular long-short term memory (LSTM) network to characterize the temporal correlations of each station itself.
Therefore, the contribution of this paper includes three parts: 1) multiple station graphs of bike sharing system are established to represent different aspects of relationships among bike sharing stations, which fully explores the exclusive information of bike sharing usage; 2) a time series based temporal structure LSTM that considering the cycle and trend is designed to capture the periodic pattern for bike sharing usage; 3) unlike other methods based on smaller bike sharing usage samples, sufficient samples make it easy to explore shared bike systems' characteristics. In this study, we discuss and validate the parameter learning experiment in large-scale bike usage systems to enhance the predictive capability of the model and reduce estimation errors.
The rest of the paper is organized as follows: Section 2 summarizes the recent studies related to bike-sharing demand forecasting; Section 3 gives a clear definition of the research problem and spatiotemporal features used as inputs and describes the proposed spatio-temporal deep learning framework from overall architecture to its detailed components; Section 4 presents the dataset and the experimental results, and Section 5 concludes the paper and outlooks future studies.
Predicting the number of available bikes in the bike-sharing system is considered a crucial step toward managing the system under different operating scenarios and various factors. Previous studies have investigated the effect of many factors, such as time, day, month, weather variables, environmental indicators, and transportation infrastructure. These studies were implemented for different goals (e.g., rebalance process [3]) to obtain new real-world insights on the interactions between bike trips and other features [4] and, ultimately, help policymakers and operators in optimizing their decisions. There are two main approaches to predicting the number of available bikes in dock-based bike-sharing systems: modeling at the individual station level or over the aggregated network level.
Demand forecasting of bike-sharing is commonly defined as a time series forecasting problem using heterogeneous data from multiple sources. The traditional approach to time series prediction is to establish an appropriate predictive model for a set of points that have been indexed in time to take advantage of the intricate sequence dependencies. The auto-regressive moving average model (ARMA) and the auto-regressive integrated moving average (ARIMA) model [5] are both well-known models for time series prediction methods with statistical regression techniques. As machine learning methods gain popularity, more researchers are focusing on studies to develop nonlinear prediction models based on large-scale historical data. Typical models such as the support vector regression (SVR) [6] based on kernel methods and the artificial neural networks (ANN) [7] with nonlinear solid function approximation ability and the k-Nearest Neighbor (K-NN) regression [8] based on distance metric in feature space and some tree-based ensemble learning methods, for instance, the random forests (RF) regression [9] and the gradient boosting regression tree (GBRT) [10].
With the rise of deep learning methods, the recurrent neural network (RNN) [11] gradually becomes the state-of-the-art method for temporal modeling. However, with a longer driving sequence, some problems, such as vanishing gradient limit the prediction accuracy of this model. To address these issues, the long short-term memory units (LSTM) and its variants, the gated recurrent unit (GRU) [12], were proposed based on the original RNN, which balances memorizing and forgetting by adding multiple threshold gates. Inspired by some successful applications in natural language processing, some researchers [13] introduce attention mechanisms to the encoding-decoding framework based on LSTMs to better establish the nonlinear relationship [14]. As a station-level prediction problem, it is important to utilize the complex heterogeneous spatiotemporal graph which describes bicycle riding relationships [15].
With the wide application of bike-sharing in urban transportation, progress has been made in related research accordingly. Studies, including data analysis and visualization [16], have employed data on bike-sharing trajectories to solve specific problems in urban management [14] and other areas. Demand prediction, as the most classic problem, has received the widest attention in this field. Based on predictive granularity, there are three groups of prediction models in existing research: city-level, cluster-level, and station-level. For the city-level and cluster-level groups, traditional methods [17] usually predict the bike demand for a whole city or design a clustering algorithm to cluster bike stations into groups as prediction units. Although city-level or cluster-level does simplify the problem, it's not as good as the station-level prediction for bike-sharing managers to help when scheduling [18].
For station-level prediction problems, some researchers furtherly explore the external context data such as time factors and weather information, using feature engineering. Also, some studies develop the recurrent neural network with temporal modeling techniques. Meanwhile, other researchers are interested in utilizing the underlying correlations between stations to predict the hourly demand at the station level with deep learning techniques such as graph convolutional neural network (GCN) with the classic support vector regression model [19]. Although the above studies have improved the accuracy and efficiency of station-level demand prediction models in different ways, there is no way to align temporal modeling with complex nonlinear spatial-temporal relations mining.
In bike-sharing demand prediction, any stations are not isolated. The station establishes complex connections through riding relationships to each other, which can be expressed by graph structures. In order to capture complex nonlinear spatial-temporal relations among stations, it is necessary to establish a length-fixed representation from graph structures. The study on dynamic graph embedding proposed a deep learning model based on deep autoencoders [20], which inspires a new idea for dynamic graph representation learning. However, it still has room to improve when capturing dependencies between each snapshot of dynamic graphs.
This paper proposes a deep learning framework to simultaneously predict bike-sharing demand in each station to overcome the above-mentioned challenge. To explain the spatial correlations, we construct multiple station graphs in which each station is viewed as a node. The adjacent matrices of nodes are established to represent different aspects of relationships among the bike-sharing station, such as neighborhood, distance, functional similarity, and historical demand correlations. Second, for both spatial and temporal correlations, we use a graph convolutional network to capture the spatial correlations among stations in different time intervals and a regular long short-term memory (LSTM) network to characterize the temporal correlations of each station itself. Second, the unique feature of the proposed method is the hyperparameter setting in our deep learning structure. The hyperparameter is responsible for updating the weights in the model in the backpropagation during the training procedure. The convolutional blocks and learning rate are discussed and validated in the experiment to reduce error estimates.
The architectural layout of the neural network is quite complex and will be elaborated in this section step-by-step and shown in Figure 1. The spatial-temporal graph convolutional neural network comprises spatial-temporal convolutional blocks, where every block has two successive gated convolutional layers with a spatial graph convolutional layer between every sequence of gated layers [21].
As previously mentioned, traffic networks can naturally be represented with graph structures. Therefore, it is appropriate to design networks mathematically as graphs. Many models have ignored the spatial attributes in road networks where connectivity is often disregarded. This can mainly be because of the separation of traffic networks into several different grids. This model will implement graph convolution directly on the graph-organized data in order to accurately analyze important patterns and features in the space realm. First, the concept of a graph convolution operator needs to be instituted. This vector is denoted in G and is established on the idea of spectral graph convolution.
ΘGx=Θ(L)x=Θ(UΛUT)x=UΘ(Λ)UTx | (1) |
where:
- x∈Rn is a signal with the kernel Θ
- U∈Rn denotes the matrix of eigenvectors of the normalized graph Laplacian, L
L=In−D−12WD−12=UΛUT∈Rnxn | (2) |
- In is the identity matrix
- D∈Rnxn is the diagonal degree matrix and Dii=∑jWij, where Wij is the weighted adjacency matrix with for the ith row and jth column
- Λ∈Rnxn is the diagonal matrix of eigenvalues of L
- Θ(Λ) is the filter with a diagonal matrix.
There are two different approaches that can be selected in terms of approximation procedure, the Chebyshev Polynomials Approximation and the 1st order approximation. This approach will utilize the former. Here, the kernel, denoted Θ, will be confined to a polynomial of Λ according to the following expression,
Θ(Λ)=∑K−1k=0θkΛk | (3) |
where θk∈Rk is a vector comprised of coefficients that are polynomial in nature. K denotes the size of the kernel of the graph convolution, which subsequently decides the max radius of the convolution measured from the nodes located centrally. The Chebyshev Polynomial, denoted Tk(x), is usually utilized in order to estimate kernels and can be described accordingly:
Θ(Λ)≈∑K−1k=0θkTk(˜Λ) | (4) |
where Eq (4) is the rescaled diagonal matrix.
˜Λ=2Λλmax−In | (5) |
Chebyshev polynomials provide two benefits: they form an orthogonal basis of L2 and one avoids the spectral decomposition of in the filtering. However, the functional form of the spectral filter is not learnable, and cannot adapt to the data. In this paper, instead of using the modified graph Laplacian, we use the aforementioned Chebyshev polynomial [21] and λmax is simply the maximum eigenvalue of L. Ultimately, the graph convolution can be simplified according to the following equation:
ΘGx=Θ(L)x≈∑K−1k=0θkTk(˜L)x | (6) |
Here, Tk(˜L) denotes the Chebyshev polynomial of order k and is computed through
˜L=2Lλmax−In | (7) |
By repeatedly calculating the K-confined convolutions through the polynomial estimation, the computing cost of Eq (6) can be lowered. The previously mentioned vector, G, for graph convolution for x∈Rn can be expanded to tensors of multiple dimensions. For a signal x with Ci channels where x∈RnxCi, we can generalize the graph convolution with Eq (7).
yj=∑Cii=1Θi,j(L)xi∈Rn,1≤j≤Co | (8) |
where,
- Ci is the size of the input feature maps
- Co is the size of the output feature maps
For two-dimensional variables, the graph convolution can be expressed as ΘGx for Θ∈RKxCixCo. When it comes to traffic prediction, the input is comprised of frames denoted vt, where each frame is a road graph. These frames can be viewed as matrices where column i outlines the Ci-dimensional value of vt in the graph Gt at the ith node. The graph Gt for time step t can be denoted as Gt=(Vt,ε,W), where Vt stands for a fixed number of vertices and are related to the number of observations coming from n monitor stations and W∈Rn symbolizes the weighted adjacency matrix of Gt. Visualizes how this process works, where H is the time steps, M is the number of traffic observations and vt∈Rn is the vector for n road elements at time.
While graphs are employed in order to analyze spatial features, gates will be used in order to analyze temporal features. By taking inspiration from, this model will apply convolutional formations on time elements in order to analyze dynamic temporal patterns in traffic demand. This architectural layout has a training procedure that can be controlled with several layers of convolutional structures that are hierarchically organized. The temporal convolutional layer includes a one-dimensional convolution where its width is denoted Kt (temporal kernel) and is succeeded by gated linear units.
The temporal convolution analyzes each input for neighbors Kt for every node in graph G. Therefore, the input of temporal convolution for every node in the graphs can be described as the length M with Ci channels with input Y where Y∈RMxCi. After designing a convolution kernel, Γ∈RKtxCix2C0, the input Y can be transformed to a unique output [P,Q]∈R(M−Kt+1)∗(2C0) and the temporal gated convolution can be expressed mathematically with the following equation:
ΓGτY=P⊙σ(Q)∈R(M−Kt+1)xC0 | (9) |
where both P and Q are the input for the gates, ⊙ is the Hadamard product performed element-wise. σ(Q) denotes the sigmoid gate, and the purpose of this gate is to manage which input P is of importance in order to discover dynamic variances in the time series.
In order to combine the features of both domains (spatial and temporal) we design spatio-temporal convolutional blocks that have the ability to operate graph-structured time series. There are two temporal layers with a spatial layer in between where layer normalization will be implemented as a precaution for overfitting the neural network. Regarding the data preprocessing, the adjacency matrix is calculated by taking the distance between the bike stations into consideration. The matrix, denoted as w, can then be formulated as follows:
wij={e(−d2ijσ2),i≠jande(−d2ijσ2)≥ϵ0 | (10) |
wij represents the weight of every edge, and this is computed by dij, which is the distance between station i and station j. ϵ and σ2 are simply values that limit the sparsity and distribution of the matrix w.
We collect the trip data of the public bike system in Nanjing, China, from 2016/3/15–2016/8/31 as our dataset. The data contains the station-level bike usage, which includes the origin station (station ID, station name, station latitude, and longitude), destination station (station ID, station name, station latitude, and longitude), start time (when a bike is checked out), stop time (when a bike is checked in) as shown in Table 1 and Figure 3.
Zone | Color | District |
1 | Blue | Gulou District |
2 | Red | Jianye District |
3 | Green | Xuanwu District |
4 | Yellow | Yuhuatai District |
5 | Black | Qixia District |
Nanjing has over 800 bike-sharing stations in 2016. However, this study will cover 50,100, and 200 stations in order to see how the results vary with the number of stations and also due to time constraints. The neural network will use the data for October and November 2016 to train the model and then test the model in order to compute the travel demand for the month of December. More importantly, a comparison between the actual demand and the predicted demand by the proposed graph neural network is conducted to highlight the difference. To improve the accuracy of the neural network, two different hyperparameters will be modified. The first parameter is the spatial-temporal convolutional blocks, and the base case for both blocks will be the following:
[1,32,64]and[64,32,128] |
This model is relatively small, and having too many channels in the base layout would cost too much in terms of computation and time. Therefore, it is appropriate to have the initial layout with 32 channels and increase the number of channels with experimental layouts in order to investigate how the efficiency fluctuates. Figure 4 highlights the structure of a spatial-temporal convolutional block. As previously mentioned, every spatial-temporal convolutional block contains two gated temporal convolutions with a spatial graph convolution layer in between. The first block has one channel as input, 32 channels in the middle, and 64 channels as output. The second block will subsequently have 64 channels as input, 32 channels in the middle, and 128 channels as output.
The first experimental block layout will be:
[1,64,128]and[128,64,256] |
and the second experimental block layout will be:
[1,128,256]and[256,128,512] |
The second hyperparameter is the learning rate during training. This hyperparameter is responsible for updating the weights in the model in the backpropagation during the training procedure and is typically defined in the range of 0-1. Instead of constantly changing weights, the learning rate is multiplied by the weights in order to reduce error estimates. The initial learning rate that is used for the base cases is 0.001. The experimental learning rates that will be tested are 0.005 and 0.01. The number of epochs will be set to 30, and the number of batches will be set to 10, both remaining constant throughout the analysis. The algorithm will use 12 hours of station-level usage to compute the next 3 hours station level usage, according to the following schematic shown in Figure 5:
To evaluate the performance of the prediction algorithm in this study, Mean Absolute Error (MAE) and Root Mean Squared Error (RMSE) was used as the evaluation index. The smaller the MAE, RMSE value is, the higher the prediction accuracy and the stronger the feature expression ability of the model.
MAE=∑Nn=1|rˆn−rn|N | (11) |
RMSE=√∑Nn=1(rˆn−rn)2N | (12) |
where rˆn is the prediction rating, rn is the true rating in test data and N is the sample size.
To present the prediction result, we first examine the bike-sharing demand prediction for particular hours. We select the demand of the examined stations that are distributed over 25 districts and build a 10 by 50 heat map matrix to illustrate the prediction results, as shown in Figures 6 and 7. The demand for the selected 50 stations shows that the majority of stations from 14:00-16:00 have a demand between 0–50 bikes. When it comes to 17:00, it can be seen that many stations have reached peak demand, where the highest is for station 8, which is approximately 200 bikes. Most stations fall below 50 bike demand after 19:00-20:00 and reach below 25 at 23:00.
For the comparison of the predicted demand by the neural network and the actual demand, Figure 7. shows that the majority of the predictions have a difference of fewer than 20 bikes compared to the real demand, with some exceptions. In Figure 8, it can be seen that almost 60 predictions had a difference ranging between -20 to 20 compared to the real hourly demand, which is a significant increase compared to the previous station analysis. Comparing the real demand with the predicted demand, Figure 9 shows that most predictions have a relatively low difference.
Figure 10 shows the mean absolute error for the base analysis plotted against the number of epochs. There are two different categories for the metric. The first line shows how the MAE for the validation set (blue), and another one shows how the MAE is on the testing set (orange). Initially, both sets have high values but significantly decrease after four completed epochs. From epochs 4–7, the MAE for both sets practically remains unchanged, whereas a slight decrease occurs from epochs 7–9. From epoch 10 to epoch 14, the MAE remains constant, where the MAE for the testing set slightly increases while the MAE for the validation set goes the opposite way and decreases. The final value for the validation set was 6.985 and 7.241 for the testing set.
Figure 11 shows the root mean square error for the validation and testing set. The RMSE exhibits similar patterns compared to the MAE for the base analysis. Initially, both sets have high values but decrease to epoch 4 and are constant from epoch 4–7. The RMSE for both sets slightly decreases towards epoch 10 and again stays constant for 4 epochs, where the same pattern is repeated for epochs 15–28. The RMSE reaches its lowest value during the final two epochs, where RMSE for the validation set is approximately 9.2 and 10.6 for the testing set.
Figure 12 shows the percentage improvements for the error metric compared to the original block layout. The biggest improvement was the MAE for the validation set, which showed a 7% decrease (improvement).
The purpose of introducing these graphs was to highlight the difference between actual demand and predicted demand and how close the predictions are to the neural network. While the actual hourly demand was shown for daily demand, the actual vs. predicted demand comparison will be for the monthly hourly demand. This will give a better overview of the difference between the computed bike demand by the neural network and the real demand. For the examined analysis, between 74–84% of the predictions had a difference of fewer than 100 bikes. As previously mentioned, this is just a simple metric that allows for a simplified comparison between actual- and predicted demand and showcases how close the predictions computed by the algorithm are.
When it comes to patterns in the given data and the predicted demand by the algorithm, some flaws can be seen. Some of the data presented in the graphs for the actual demand show that for some days, the bike demand for some stations suddenly dropped to zero, which was a reoccurring pattern for some stations and most likely influenced the accuracy of the predictions. One more pattern that could be seen when looking at the comparison between the actual- and predicted demand is that the neural network was very inaccurate for the final hour of the day for many stations where the model very often predicted significantly higher demand than what was.
As previously mentioned, the purpose of using the error metrics MAE and RMSE is to measure the accuracy of the neural network by analyzing the magnitude of the difference between actual and predicted demand. The mean absolute error highlights the absolute value of the difference between the actual demand and the predicted demand by the neural network on average. For example, for the examined station analysis, the predictions computed by the neural network are, on average, 4.77–4.90 bikes from the actual demand, which can be seen as very accurate depending on the demand for every specific hour.
When it comes to the RMSE values, similar conclusions can be drawn. As previously stated, the RMSE value is simply an error metric that quantifies the prediction errors by using standard deviation. Similar to the reasoning behind finding a "good" MAE value, establishing a good RMSE value is problematic and is relative to the dataset and dependent on the variables.
Finally, determining if the algorithm is accurate can be done by analyzing if the model is overfitting or underfitting. Discovering whether the model is indeed overfitting or underfitting can be established when analyzing the error metrics, which are in this case, MAE and RMSE. If the model is performing well on the validation set but has a large error on the testing set, then the model is overfitting. If the model has poor performance on both validation and testing set, then the model is instead underfitting. So, finding a balance and subsequently, finding a good fit for the neural network is vital. Looking at the Figures showcasing the MAE and RMSE values for all the station analysis shows that the error metric for both the validation and testing set converge and are very close relative to each other, meaning that the model has a robust fit and is well-fitted.
The aim of this study was to design a spatio-temporal framework that has the ability to use graph-structured data and take both spatial and temporal aspects into consideration when predicting the high-resolution demand of shared mobility systems. A case study about bike-sharing systems in Nanjing, a large city in China, is conducted based on the proposed method. The results show that the algorithm is able to predict short-term bike demand with relatively high accuracy and low computing time. The results show that the graph neural network is able to predict traffic demand in an accurate way with MAE/RMSE values that are quite good. The predicted errors for the hourly usage demand prediction of a station are often within 20 bikes. By utilizing station-to-station data combined with time data, the algorithm could accurately predict short-term bike demand. The hyperparameters are modified in order to see how the accuracy fluctuates and the efficiency of the model is affected. This included changing the learning rate and layout for the blocks. The results vary with hyperparameters, the learning rate that showed the best results was 0.005 compared to the original 0.01.
This study focuses on the short-term prediction for bike-sharing, which is a base layer for future studies. This base layer can be used to evaluate the transportation accessible in terms of congestion and roads. Based on this study, the major flow patterns can be evaluated, and further development of the transportation routes to make it easier to use a bike in the city of Nanjing. Due to the highly populated urban area, it is very hard to build new infrastructure on a bigger scale. Therefore, the bike-sharing transportation system is a good choice in these terms. The study can also be used for a comparison of different transportation systems in Nanjing to evaluate what transportation system is the most beneficial and effective to use.
The study and method can also be applied to other transportation sectors, such as road transportation in Nanjing and other cities. The method is the same for all cases, and only the data is needed to be changed. In result, a demand prediction map for all the stations in Nanjing can be created. This will result in a good understanding of the bike usage patterns, which will be very useful for the city in terms of finding an acceptable and good balance in the stations. Unfortunately, due to time constraints, the analysis was limited to 200 stations, where it would have been interesting to see how the accuracy of the algorithm would be affected with more stations analyzed [22]. Something that would also be interesting to see would be to implement meteorological conditions into the algorithm, which is a large impacting factor when it comes to biking. This would make the algorithm become one level higher in terms of complexity but would significantly increase the accuracy of the neural network. Human behavior is not considered in this study in terms of weather, temperature, location, and year [23]. The study does not consider how this could have affected the result and changed the outcome. The travel path for the bikes is not considered, and only the station-to-station data are used in this study. And there is much room for improvement where meteorological conditions could have also been implemented in the model, which would have a high probability of increasing the accuracy of the predictions.
The authors are grateful to the Area of Advance Transport and AI Center (CHAIR) at the Chalmers University of Technology, and the European Union's Horizon 2020 research and innovation programme under the Marie Skłodowska-Curie grant agreement No. 101025896 for funding this research.
The authors declare there is no conflict of interest.
[1] |
K. Gao, Y. Yang, X. Qu, Diverging effects of subjective prospect values of uncertain time and money, Commun. Transp. Res., 1 (2021), 100007. https://doi.org/10.1016/j.commtr.2021.100007 doi: 10.1016/j.commtr.2021.100007
![]() |
[2] |
J. Ke, X. Qin, H. Yang, Z. Zheng, Z. Zhu, J. Ye, Predicting origin-destination ride-sourcing demand with a spatio-temporal encoder-decoder residual multi-graph convolutional network, Transp. Res. Part C Emerging Technol., 122 (2021), 102858. https://doi.org/10.1016/j.trc.2020.102858 doi: 10.1016/j.trc.2020.102858
![]() |
[3] |
J. G. Jin, H. Nieto, L. Lu, Robust bike-sharing stations allocation and path network design: a two-stage stochastic programming model, Transp. Lett., 12 (2020), 682–691. https://doi.org/10.1080/19427867.2019.1691299 doi: 10.1080/19427867.2019.1691299
![]() |
[4] |
H. I. Ashqar, M. Elhenawy, H. A. Rakha, M. Almannaa, L. House, Network and station-level bike-sharing system prediction: a San Francisco bay area case study, J. Intell. Transp. Syst., 26 (2022), 602–612. https://doi.org/10.1080/15472450.2021.1948412 doi: 10.1080/15472450.2021.1948412
![]() |
[5] |
G. E. Box, D. A. Pierce, Distribution of residual autocorrelations in autoregressive-integrated moving average time series models, J. Am. Stat. Assoc., 65 (1970), 1509–1526. https://doi.org/10.1080/01621459.1970.10481180 doi: 10.1080/01621459.1970.10481180
![]() |
[6] | H. Drucker, C. J. Burges, L. Kaufman, A. Smola, V. Vapnik, Support vector regression machines, Adv. Neural Inf. Process. Syst., 9 (1996), 155–161. |
[7] | K. Davoian, W. M. Lippe, Including phenotype information in mutation to evolve artificial neural networks, in International Joint Conference on Neural Networks, 2007, 2782–2787. https://doi.org/10.1109/IJCNN.2007.4371400 |
[8] | Y. Wang, B. Chaib-Draa, A KNN based Kalman filter Gaussian process regression, in Twenty-Third International Joint Conference on Artificial Intelligence, 2013, 1771–1777. https://dl.acm.org/doi/10.5555/2540128.2540382 |
[9] |
U. Johansson, H. Boström, T. Löfström, H. Linusson, Regression conformal prediction with random forests, Mach. Learn., 97 (2014), 155–176. https://doi.org/10.1007/s10994-014-5453-0 doi: 10.1007/s10994-014-5453-0
![]() |
[10] | X. Li, R. Bai, Freight vehicle travel time prediction using gradient boosting regression tree, in 2016 15th IEEE International Conference on Machine Learning and Applications (ICMLA), 2016, 1010–1015. https://doi.org/10.1109/ICMLA.2016.0182 |
[11] |
D. E. Rumelhart, G. E. Hinton, R. J. Williams, Learning representations by back-propagating errors, Nature, 323 (1986), 533–536. https://doi.org/10.1038/323533a0 doi: 10.1038/323533a0
![]() |
[12] | K. Cho, B. Van Merriënboer, D. Bahdanau, Y. Bengio, On the properties of neural machine translation: Encoder-decoder approaches, arXiv preprint, 2014, arXiv: 1409.1259. https://doi.org/10.48550/arXiv.1409.1259 |
[13] | Y. Liang, S. Ke, J. Zhang, X. Yi, Y. Zheng, Geoman: Multi-level attention networks for geo-sensory time series prediction, in Proceedings of the Twenty-Seventh International Joint Conference on Artificial Intelligence (IJCAI-18), 1 (2018), 3428–3434. https://www.ijcai.org/proceedings/2018/0476.pdf. |
[14] | Y. Li, Z. Zhu, D. Kong, M. Xu, Y. Zhao, Learning heterogeneous spatial-temporal representation for bike-sharing demand prediction, in Proceedings of the AAAI Conference on Artificial Intelligence, 33 (2019), 1004–1011. https://doi.org/10.1609/aaai.v33i01.33011004 |
[15] | C. Guo, F. Berkhahn, Entity embeddings of categorical variables, arXiv preprint, 2022, arXiv: 1604.06737. |
[16] |
Y. Yan, Y. Tao, J. Xu, S. Ren, H. Lin, Visual analytics of bike-sharing data based on tensor factorization, J. Visualization, 21 (2018), 495–509. https://doi.org/10.1007/s12650-017-0463-1 doi: 10.1007/s12650-017-0463-1
![]() |
[17] |
Y. Guo, J. A. Kelly, J. P. Clinch, Variability in total cost of vehicle ownership across vehicle and user profiles, Commun. Transp. Res., 2 (2022), 100071. https://doi.org/10.1016/j.commtr.2022.100071 doi: 10.1016/j.commtr.2022.100071
![]() |
[18] |
K. Gao, H. Wang, S. Wang, X. Qu, Data and code disclosure and sharing policy of communications in transportation research, Commun. Transp. Res., 2 (2022), 100055. https://doi.org/10.1016/j.commtr.2022.100055 doi: 10.1016/j.commtr.2022.100055
![]() |
[19] |
L. Lin, Z. He, S. Peeta, Predicting station-level hourly demand in a large-scale bike-sharing network: A graph convolutional neural network approach, Transp. Res. Part C Emerging Technol., 97 (2018), 258–276. https://doi.org/10.1016/j.trc.2018.10.011 doi: 10.1016/j.trc.2018.10.011
![]() |
[20] | P. Goyal, N. Kamra, X. He, Y. Liu, Dyngem: Deep embedding method for dynamic graphs, arXiv preprint, 2018, arXiv: 1805.11273. https://doi.org/10.48550/arXiv.1805.11273 |
[21] | B. Yu, H. Yin, Z. Zhu, Spatio-temporal graph convolutional networks: A deep learning framework for traffic forecasting, arXiv preprint, 2018, arXiv: 1709.04875. https://doi.org/10.48550/arXiv.1709.04875 |
[22] |
K. Gao, Y. Yang, X. Qu, Examining nonlinear and interaction effects of multiple determinants on airline travel satisfaction, Transp. Res. Part D Transp. Environ., 97 (2021), 102957. https://doi.org/10.1016/j.trd.2021.102957 doi: 10.1016/j.trd.2021.102957
![]() |
[23] |
K. Gao, Y. Yang, A. Li, X. Qu, Spatial heterogeneity in distance decay of using bike sharing: An empirical large-scale analysis in Shanghai, Transp. Res. Part D Transp. Environ., 94 (2021), 102814. https://doi.org/10.1016/j.trd.2021.102814 doi: 10.1016/j.trd.2021.102814
![]() |
1. | Chen Qiu, Ruo Jia, 2023, Chapter 20, 978-981-99-3283-2, 221, 10.1007/978-981-99-3284-9_20 | |
2. | Chuanxiang Ren, Hui Xu, Chunxu Chai, Fangfang Fu, 2023, Shared Bike Demand Prediction Based on Combined Deep Learnings, 979-8-3503-1449-6, 200, 10.1109/ICECAI58670.2023.10176751 | |
3. | César Peláez-Rodríguez, Jorge Pérez-Aracil, Dušan Fister, Ricardo Torres-López, Sancho Salcedo-Sanz, Bike sharing and cable car demand forecasting using machine learning and deep learning multivariate time series approaches, 2024, 238, 09574174, 122264, 10.1016/j.eswa.2023.122264 | |
4. | Yan Shi, Da Wang, Xiaolong Wang, Bingrong Chen, Chen Ding, Shijuan Gao, Sensing Travel Source–Sink Spatiotemporal Ranges Using Dockless Bicycle Trajectory via Density-Based Adaptive Clustering, 2023, 15, 2072-4292, 3874, 10.3390/rs15153874 |
Zone | Color | District |
1 | Blue | Gulou District |
2 | Red | Jianye District |
3 | Green | Xuanwu District |
4 | Yellow | Yuhuatai District |
5 | Black | Qixia District |