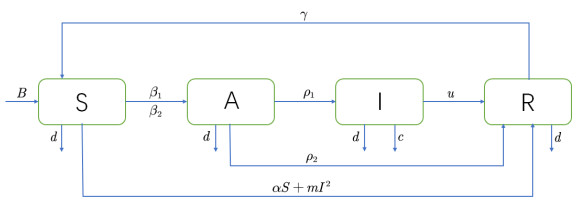
As the COVID-19 continues threatening public health worldwide, when to vaccinate the booster shots becomes the hot topic. In this paper, based on the characteristics of COVID-19 and its vaccine, an SAIR model associated with temporary immunity is proposed to study the effect on epidemic situation. Second, we theoretically analyze the existence and stability of equilibrium and the system undergoes Hopf bifurcation when delay passes through some critical values. Third, we study the dynamic properties of Hopf bifurcation and derive the normal form of Hopf bifurcation to determine the stability and direction of bifurcating periodic solutions. After that, numerical simulations are carried out to demonstrate the application of the theoretical results. Particularly, in order to ensure the validity, statistical analysis of data is conducted to determine the values for model parameters. Next, we study the impact of the infection rates on booster vaccination time to simulate the mutants, and the results are consistent with the facts. Finally, we predict the mean time of completing a round of vaccination worldwide with the help fitting and put forward some suggestions by comparing with the critical time of booster vaccination.
Citation: Zimeng Lv, Jiahong Zeng, Yuting Ding, Xinyu Liu. Stability analysis of time-delayed SAIR model for duration of vaccine in the context of temporary immunity for COVID-19 situation[J]. Electronic Research Archive, 2023, 31(2): 1004-1030. doi: 10.3934/era.2023050
[1] | Fengrong Zhang, Ruining Chen . Spatiotemporal patterns of a delayed diffusive prey-predator model with prey-taxis. Electronic Research Archive, 2024, 32(7): 4723-4740. doi: 10.3934/era.2024215 |
[2] | Xin Du, Quansheng Liu, Yuanhong Bi . Bifurcation analysis of a two–dimensional p53 gene regulatory network without and with time delay. Electronic Research Archive, 2024, 32(1): 293-316. doi: 10.3934/era.2024014 |
[3] | Miao Peng, Rui Lin, Zhengdi Zhang, Lei Huang . The dynamics of a delayed predator-prey model with square root functional response and stage structure. Electronic Research Archive, 2024, 32(5): 3275-3298. doi: 10.3934/era.2024150 |
[4] | Rina Su . Dynamic analysis for a class of hydrological model with time delay under fire disturbance. Electronic Research Archive, 2022, 30(9): 3290-3319. doi: 10.3934/era.2022167 |
[5] | Mengting Sui, Yanfei Du . Bifurcations, stability switches and chaos in a diffusive predator-prey model with fear response delay. Electronic Research Archive, 2023, 31(9): 5124-5150. doi: 10.3934/era.2023262 |
[6] | Yujia Xiang, Yuqi Jiao, Xin Wang, Ruizhi Yang . Dynamics of a delayed diffusive predator-prey model with Allee effect and nonlocal competition in prey and hunting cooperation in predator. Electronic Research Archive, 2023, 31(4): 2120-2138. doi: 10.3934/era.2023109 |
[7] | Yuan Xue, Jinli Xu, Yuting Ding . Dynamics analysis of a diffusional immunosuppressive infection model with Beddington-DeAngelis functional response. Electronic Research Archive, 2023, 31(10): 6071-6088. doi: 10.3934/era.2023309 |
[8] | Xiaowen Zhang, Wufei Huang, Jiaxin Ma, Ruizhi Yang . Hopf bifurcation analysis in a delayed diffusive predator-prey system with nonlocal competition and schooling behavior. Electronic Research Archive, 2022, 30(7): 2510-2523. doi: 10.3934/era.2022128 |
[9] | San-Xing Wu, Xin-You Meng . Hopf bifurcation analysis of a multiple delays stage-structure predator-prey model with refuge and cooperation. Electronic Research Archive, 2025, 33(2): 995-1036. doi: 10.3934/era.2025045 |
[10] | Ugo Avila-Ponce de León, Angel G. C. Pérez, Eric Avila-Vales . Modeling the SARS-CoV-2 sublineages XBB and BQ.1 in Mexico, considering multiple vaccinations, booster dose, waning immunity and cross-immunity. Electronic Research Archive, 2024, 32(2): 1082-1125. doi: 10.3934/era.2024053 |
As the COVID-19 continues threatening public health worldwide, when to vaccinate the booster shots becomes the hot topic. In this paper, based on the characteristics of COVID-19 and its vaccine, an SAIR model associated with temporary immunity is proposed to study the effect on epidemic situation. Second, we theoretically analyze the existence and stability of equilibrium and the system undergoes Hopf bifurcation when delay passes through some critical values. Third, we study the dynamic properties of Hopf bifurcation and derive the normal form of Hopf bifurcation to determine the stability and direction of bifurcating periodic solutions. After that, numerical simulations are carried out to demonstrate the application of the theoretical results. Particularly, in order to ensure the validity, statistical analysis of data is conducted to determine the values for model parameters. Next, we study the impact of the infection rates on booster vaccination time to simulate the mutants, and the results are consistent with the facts. Finally, we predict the mean time of completing a round of vaccination worldwide with the help fitting and put forward some suggestions by comparing with the critical time of booster vaccination.
In early 2020, COVID-19 (Corona Virus Disease 2019) outbroke among the population and became a worldwide pandemic. The COVID-19 is a kind of infectious disease caused by SARS-CoV-2 pathogen (severe acute respiratory syndrome coronavirus 2) that is highly contagious, universally susceptible and widely transmitted. In some serious areas, people must be quarantined at home or in concentrated isolation once exposed to the source of infection, which is detrimental to the development and progress of society. More importantly, the COVID-19 not only affects the social production and life, but also threatens our health. According to the statistics from World Health Organization, more than 200 million people have been diagnosed with COVID-2019 and more than 6 million people have died from this until November 1, 2022. Thereinto, about 20% of the patients require hospitalization and the death rate is nearly up to 2% [1]. In particular, in the early stage of the outbreak, the treatment process was difficult because people had never been exposed to the disease. Although we have better research on treatments with the continuous understanding of the virus, the test for human beings is far more than that. The COVID-19 continues mutating as the transmission progress among humans. In the first half of 2021, the first virus strain SARS-CoV-2 Delta variant of concern (B.1.617.2) originating from India, was discovered [2]. Compared to the previous strain, the Delta strain showed the higher transmissibility and drug resistance. In November of the same year, the new virus strain Omicron was found with more genetic mutations and easier transmission noted by The World Health Organization. As a result, vaccination is considered the most effective measure to control the epidemic situation.
For different infectious diseases, scholars have developed different models of infectious diseases to study their dynamical properties and behaviors [3,4,5,6]. Accordingly, there are always corresponding vaccines to enhance herd immunity, so as to control the epidemic at the root. In recent studies, many researchers have added vaccine effects into their infectious disease models [7,8,9,10,11]. In the study of Arino et al. [7], an SLIRA model, representing susceptible-latent-infected-recovered-asymptomatic, was established for the avian influenza (H5N1) with vaccination and antiviral therapy. They analyzed how to control the epidemic situation if it evolved into a strain with transmission among humans. In [8], Greenhalgh et al. established an SIRS model and analyzed recurrent epidemic cycles of infected diseases when the vaccine-induced immunity was only temporary. Ho and Chao [9] took vaccine capacity, strain mismatch and priority group into considerations and constructed an SEIR compartmental model aiming at studying the influenza vaccination policy. An SVIR model with distributed delay and imperfect vaccine was constructed, which included the susceptible, vaccinated, infected and recovered populations [10]. In [11], an SVIS cyclic epidemic vaccination model was established by Kabir et al.
Since the outbreak of COVID-19, the whole society has attached great importance to it. Many scholars have attempted to study the effect of COVID-19 vaccines on controlling the epidemic situation [12,13,14]. Vishaal et al. [12] presented a modified age-structured SIR model to analyze the effect of vaccination and age-targeted distributions. They found that population age-distribution with vaccination had a significant effect on transmission and mortality rates. In [13], Shen et al. developed a dynamic model with ten compartments of COVID-19 transmission in the four most severely affected states. They evaluated the vaccine effectiveness and coverage required to suppress the COVID-19 outbreaks under certain conditions. There is also a generalized epidemiological SEIR model for COVID-19 vaccine established by Rajaeia et al. [14]. It included the susceptible (S), exposed (E), infected (I), quarantined (Q), recovered (R), deceased (D) and insusceptible (P) populations to manage the COVID-19 by comparing the differences before and after its vaccine invention.
Previously, many models used ordinary differential equation (ODE) systems to describe the dynamic behavior of diseases. However, there were always some considerable differences between the realistic behavior and the response of their mathematical models. In 1979, Cooke first put forward "time-delay" to study the spread of epidemic diseases which made the models more realistic [15]. Since then, many researchers have attempted to consider time-delay in their models [16,17,18,19,20]. In [16], an SVEIR model with time-delay for imperfect vaccine was developed. They considered the time from the susceptible or the vaccinated to the exposed. Yang and Wang [17] evaluated the effect of the latency when they established the SEIRS epidemic model. And in [18], a delayed SEIS epidemic model was constructed regarding the time used to cure the infectious people. An SVEIR model was constructed [19] considering the time used to cure the infectious people as well. Din et al. [20] developed an infectious disease model for hepatitis B virus and introduced a time delay to represent the incubation period of the virus in the diffusion term.
Analysis of stability and Hopf bifurcation has always been the focus of epidemic models [21,22,23,24,25,26,27,28]. In [21], an age-structured SIR epidemic model was constructed by Kuniya et al. and was studied the sustained periodic solution through the Hopf bifurcation. Duan et al. [22] formulated and studied an age-structured epidemic model with age of recovery. They considered the existence and stability of equilibria and the bifurcation of periodic solutions. Cai and Wang [23] demonstrated that the stability of solutions only changed at every turning points of the bounded bifurcation. [24,25,26,27,28] discussed the dynamical properties of Hopf bifurcation in different systems.
The motivation of our paper is as follows. Firstly, it is reported that there are some cases of infection that have been vaccinated, suggesting that vaccine-induced immunity is not permanent, so it is necessary to strengthen the immunization. But how long our original vaccination will last and when to schedule a mass booster vaccination? That's where we're going to study. Secondly, as the virus continues to mutate, the infection rate gradually increases. What effect will this have on the time of booster vaccination? That's also what we're looking at here. Thirdly, we know that not all countries can accomplish booster vaccination in a short time under the constraints of human, material and financial resources. In order to ensure that the epidemic is within the controllable range, we want to figure out the suitable time of booster vaccination with above limitation.
Through the study of this paper, we have a more accurate grasp of when to carry out booster vaccination. By simulating the current epidemic state, a reasonable time range for booster vaccination is proposed, which can ensure that the epidemic can reach a stable state instead of being out of control. That is meaningful to the formulation of epidemic prevention and control policies.
The structure of this paper is as follows. In Section 2, considering the characteristics of COVID-19, we construct a delayed Susceptible-Asymptomatic-Infected-Recovered (hereinafter referred to as SAIR) model associated with temporary immunity. In Section 3, we analyse the existence of equilibria and discuss the existence of Hopf bifurcation. In Section 4, we calculate the normal form of Hopf bifurcation by using multiple time scales method. And in Section 5, we show some numerical simulations and obtain the conclusion of booster vaccination time by analysis. Finally we show the conclusions and propose some suggestions in Section 6.
The susceptible people (S) can be infected by COVID-19 through contact with the source of infection. They first become the asymptomatic people (A: one group of people with the virus latent in their bodies but with negative results of nucleic acid detection, and another group of people who have positive results but do not show any symptoms). Some of them become the recovered people (R) through the immune response of their autoimmune system, while others become the infected people (I) with symptoms such as fever and weakness since they are less fit and cannot fully fight off the virus. Such people is either cured, also called the recovered people (R), or die from the failure of resuscitation. For those who are vaccinated, since the vaccine is the inactivated virus, similar to the mechanism of self-healing or cure after infection, it also causes the body to produce an immune response and thus produce antibodies. Therefore, we classify vaccinated people as the recovered people (R) as well, which can be understood as recovering from the risk of infection.
We consider the two effects of vaccination rates. In general, if there are no diagnosed cases of COVID-19 in a district, the vaccination can also be organized to enhance herd immunity and reduce the risk of infection. So we denote this part as the natural vaccination rate. On the other hand, when there are infection people in an area, it will be in a state of emergency. In addition to the necessary protection, isolation and treatment, the vaccination process will be accelerated as well. However, we believe that the rate of vaccination is not simply linear with respect to the number of infections, but grows in a much faster way. Since all nonlinear functions can be Taylor expanded, we approximate the expansion to its simplest second-order form, denoted as αS2, where α is the vaccine distribution rate which can be interpreted as vaccine distribution from other areas to speed up the vaccination process.
Taking all these considerations into account, we obtain the specific conversions between the four cabins that are given in Figure 1. The specific definitions of variables and paraments are given in the Table 1. In this table, all parameters and variables are positive.
Symbol | Descriptions |
S | Number of suspected people |
A | Number of asymptomatic people |
I | Number of infected people |
R | Number of recovered people |
B | Population migration rate |
d | Population natural mortality rate |
c | COVID-19 mortality rate of infected people |
β1 | Infection rate by contacting A |
β2 | Infection rate by contacting I |
ρ1 | Definitive diagnosis rate of A |
ρ2 | Self-healing rate of A |
u | Cure rate of I |
γ | Antibody failure rate of R |
α | Natural vaccination rate |
m | Vaccine distribution rate |
For the COVID-19 vaccine, antibodies will be produced in susceptible people after vaccination, but this immunity is not permanent. After a period of time, the antibody efficiency will gradually decrease until it disappears. So there is a time delay from the recovered people to the susceptible people. Meanwhile, although there is also a period of time when an asymptomatic person turn into infected one, it can be ignored comparing with the time delay from the recovered people to the susceptible people. Combining the above considerations, we use τ for the time from the recovered people (R) to the suspected people (S), which is the time for vaccine failure. Thus, we construct the following delayed differential equation:
{dSdt=B+γR(t−τ)−αS−mI2−β1SA−β2SI−dS,dAdt=β1SA+β2SI−ρ1A−ρ2A−dA,dIdt=ρ1A−uI−dI−cI,dRdt=uI+ρ2A+αS+mI2−γR(t−τ)−dR, | (2.1) |
where the meanings of variables and parameters are given in Table 1, and τ is the time of vaccine failed.
When the vaccine fails, the antibodies disappear and the person move from R to S. But under normal circumstances, in order to prevent the failure of the vaccine to lead to a large area of infection, which leads to the epidemic out of control, we often improve the population immunization rates through booster vaccination. Therefore, it is particularly important to select the right time for booster vaccination to control the epidemic.
Generally speaking, the booster vaccination time is often earlier than the expiration time of the vaccine. But when the booster shot is injected, the amount of antibody in the body is significantly more than that when not vaccinated, and the antiviral efficiency will be greatly improved. So we can believe that the original antibody has no immunity relative to the existing antibody. In addition, due to the constant mutation of the virus, even if the antibody did not disappear, the original antibody has been unable to resist the attack of the mutant strain, it will also have the possibility of infection. Considering the above two points, we can think that the vaccine failure time is consistent with the booster vaccination time.
Let C([−τ,0],R4+0) be the Banach space of continuous functions mapping interval [−τ,0] into R4+0. For any ϕ∈C([−τ,0],R4+0), we define the norm ‖ϕ‖ = supθ∈[−τ,0]|(θ)| and the initial condition of the system (2.1) is
{ϕS(θ)≥0,ϕA(θ)≥0,ϕI(θ)≥0,ϕR(θ)≥0,θ∈[−τ,0],S(0)≥0,A(0)≥0,I(0)≥0,R(0)≥0. |
The system (2.1) has a unique solution under the above initial conditions. Let (S(t),A(t),I(t),R(t)) be an arbitrary solution of the system under this initial condition, we give the discussion about the positivity and boundedness of solution of the system (2.1).
1) The positivity of solution
When t∈[0,τ], we solve each equation of system (2.1) and obtain:
S(t)=e−∫t0(β1A(ξ)+β2I(ξ)+α+d)dξ[S(0)+∫t0(B+γR(η−τ)−mI2(η))e∫η0(β1A(ξ)+β2I(ξ)+α+d)dξdη],A(t)=e−∫t0(−β1S(ξ)−ρ1−ρ2−d)dξ[A(0)+∫t0(β2S(η)I(η))e∫η0(−β1S(ξ)−ρ1−ρ2−d)dξdη],I(t)=e−(u+d+c)t[I(0)+∫t0(ρ1A(η))e−(u+d+c)ηdη],R(t)=e−dt[R(0)+∫t0(uI(η)+ρ2A(η)+αS(η)+mI2(η)−γR(η−τ))e−dηdη]. | (2.2) |
In this paper, since we are mainly considering the issues related to booster vaccination, we assume that most people have received their first vaccination. The state is mainly located in the middle and late stages of the epidemic with a small number of infected people relative to the beginning, that is, the number of the recovered people R (the vaccinated people and the cured people) is so far greater than the infected people I, so that B+γR(t−τ)−mI2(t)>0 is reasonable. By the expression of S(t), we can obtain that S(t)>0 holds when t∈[0,τ].
Now we prove I(t)>0. We assume that I(t) is not always positive for t∈[0,τ] and make t1 be the first time that I(t1)=0, I′(t1)<0. According to the third equation of the system (2.1), we can obtain I′(t1)=ρ1A(t1)>0. In addition,
A(t1)=e−∫t10(−β1S(ξ)−ρ1−ρ2−d)dξ[A(0)+∫t10(β2S(η)I(η))e∫η0(−β1S(ξ)−ρ1−ρ2−d)dξdη]. |
Thus, A(t1)>0 and I′(t1)>0. Since t1 is the first time that pass through t-axis and makes I(t)<0, so I′(t1)<0. The two conclusion we obtain are contradictory and I(t)>0. In the same way, we can also obtain A(t)≥0 when t∈[0,τ].
Finally, we prove R(t)≥0. Similarly, using the proof by contradiction, we assume that t2 be the first time that R(t2)=0, R′(t2)<0. That is to say, for any t∈[0,t2], we have R(t)>0, so R(t2−τ)>0 holds due to t2−τ∈[−τ,0]. By the forth equation of system (2.1), we have R′(t2)=uI(t2)+ρ2A(t2)+αS(t2)+mI(t2)2−γR(t2−τ). If τ=0, we can obtain R′(t2)=uI(t2)+ρ2A(t)+αS(t2)+mI(t2)2>0 under the condition of S(t)>0,A(t)>0,I(t)>0, which is contradictory with assumption. So R(t)≥0 when τ=0. If τ>0, we focus on the expression of R(t) in Eq (2.2). In Subsection 5.1, we find that the values of parameters u,ρ2,m are larger than γ through the analysis of the actual, so it is reasonable to assume that uI(t)+ρ2A(t)+αS(t)+mI(t)2−γR(t−τ)>0, which can ensures that R(t)>0 according to the expression of R(t) in Eq (2.2).
Overall, when t∈[0,τ], the all solutions of system (2.1) are positive after we consider the realistic situation and give some assumptions. By the similar approach, it can be shown that all solutions of the system (2.1) are also positive on [τ,2τ] under some restrictions. This conclusion can be extended to the interval [nτ,(n+1)τ](n∈N). Therefore, for any t>0, all solutions of system (2.1) are positive with the restrictions.
2) The boundedness of solution
Let N(t)=S(t)+A(t)+I(t)+R(t), and N(t) represents the total number of people at time t. Adding four equations of system (2.1), we obtain N′(t)=B−dN(t)−cI(t). According to the discussion of the positivity of solution, we can obtain that I(t)>0 under certain condition. Thus, N′(t)=B−dN(t)−cI(t)≤B−dN(t). Based on the comparison theorem, we can get:
0<N(t)≤e−dt[N(0)+Λ∫t0edηdη]=e−dt[N(0)−Bd]+Bd. |
Therefore, limt→∞supN(t)≤Bd and the solution S(t), A(t), I(t), R(t) of the system (2.1) is bounded when t>0.
Under the conditions and assumptions that the positivity of solution exists we discussed above, we mark the feasible region of the system (2.1) is,
Ω={S(t),A(t),I(t),R(t)|N(t)=S(t)+I(t)+Q(t)+R(t)≤Λd,S(t)≥0,A(t)≥0,I(t)≥0,R(t)≥0,uI(t)+ρ2A(t)+αS(t)+mI(t)2−γR(t−τ)>0,B+γR(t−τ)−mI2(t)>0}. | (2.3) |
Although the positivity and boundedness of our model are conditional, our analysis of the epidemic shows that the present state is satisfying the conditions for the existence of positivity and boundedness, and therefore our model is reasonable and meaningful.
In order to study the existence of equilibrium, Driessche introduced the next generation matrix method in [29]. By this way, they defined the basic reproduction number R0, that is, the number of secondary infections resulting from a single primary infection into an otherwise susceptible population [30]. Since then, many models have discussed the existence conditions of equilibria by the basic reproduction number R0. According to this method, we can obtain the basic reproduction number R0 of our model:
R0=B(γ+d)[β1(u+d+c)+β2ρ1]d(α+γ+d)(ρ1+ρ2+d)(u+d+c). | (3.1) |
The basic reproduction value R0 is a very important tool when we discuss the spread of a disease, and the threshold condition R0=1 completely determines whether an infectious disease can invade the population or not. When the value is less than 1, it predicts that the disease is relaxed, that is, there is no epidemic prevalence in the population. On the contrary, when the value is greater than 1, the disease will be transformed into an epidemic [31,32].
System (2.1) has two equilibria
E1=(S∗1,A∗1,I∗1,R∗1),E2=(S∗2,A∗2,I∗2,R∗2), | (3.2) |
where
S∗1=(γ+d)Bd(γ+d+α),A∗1=0,I∗1=0,R∗1=αBd(γ+d+α),S∗2=(ρ1+ρ2+d)(u+d+c)β1(u+d+c)+β2ρ1,I∗2=−p+√p2−4dmq2dm,A∗2=u+d+cρ1I∗2,R∗2=uI∗2+ρ2A∗2+αS∗2+mI∗22γ+d, |
with
p=d(ρ1+ρ2+d+γ)(u+d+c)ρ1,q=(d2+γd+αd)S∗2−(γ+d)B. |
The equilibrium E1=(S∗1,A∗1,I∗1,R∗1) always exists. For the equilibrium E2=(S∗2,A∗2,I∗2,R∗2), if R0>1 holds, q is negative, so S∗2, A∗2, I∗2, R∗2 are all positive, which means that equilibrium E2 is meaningful under this condition. On the contrary, if R0<1, we can deduce that I∗2<0, so it is nonsignificant in reality. Therefore, we have the following conclusion.
Lemma 3.1. If R0<1, the system (2.1) has unique equilibrium: the disease-free equilibrium E1=(S∗1,A∗1,I∗1,R∗1). If R0>1, the system not only has the equilibrium E1, but also has an endemic equilibrium E2=(S∗2,A∗2,I∗2,R∗2).
Then we make linear transformation: ˜S=S−S∗k, ˜A=A−A∗k, ˜I=I−I∗k, ˜R=R−R∗k, k=1,2. Substitute ˜S, ˜A, ˜I, ˜R into system (2.1) and drop the tilde for convenience, the system (2.1) becomes:
{dSdt=γR(t−τ)−αS−mI2−2mI∗kI−β1SA−β1S∗kA−β1SA∗k−β2SI−β2S∗kI−β2SI∗k−dS,dAdt=β1SA+β1S∗kA+β1SA∗k+β2SI+β2S∗kI+β2SI∗k−ρ1A−ρ2A−dA,dIdt=ρ1A−uI−dI−cI,dRdt=uI+ρ2A+αS+2mI∗kI−γR(t−τ)−dR+mI2,k=1,2. | (3.3) |
Since we are more concerned about the impact of antibody failure time on the epidemic, we take time delay τ as bifurcation parameter when discussing the Hopf bifurcation.
Firstly, we consider R0<1. The characteristic equation of the linearized system (3.3) about E1 is as follows:
(λ+α)(λ+α+d+γe−λτ)(λ2+Π1λ+Π2)=0, | (3.4) |
where Π1=−β1S∗1+ρ1+ρ2+u+2d+c,Π2=(−β1S∗1+ρ1+ρ2+d)(u+d+c)−ρ1β2S∗1.
When τ=0, Eq (3.4) becomes:
(λ+α)(λ+α+d+γ)(λ2+Π1λ+Π2)=0, | (3.5) |
where Π1 and Π2 are given by Eq (3.4). So we can obtain the eigenvalues: λ11=−α<0, λ12=−α−d−γ<0 and λ13, λ14 satisfying equation λ2+Π1λ+Π2=0. Due to R0<1, we have:
λ13+λ14=−β1(u+d+c)2β1(u+d+c)2+β2ρ1−β2ρ1(ρ1+ρ2+u+2d+c)β1(u+d+c)2+β2ρ1<0,λ13λ14=(−β1S∗1+ρ1+ρ2+d)(u+d+c)−ρ1β2S∗1=(ρ1+ρ2+d)(u+d+c)−[β1(u+d+c)+β2ρ1](γ+d)Bd(γ+d+α)>(ρ1+ρ2+d)(u+d+c)−[β1(u+d+c)2+β2ρ1](ρ1+ρ2+d)(u+d+c)β1(u+d+c)2+β2ρ1=0. |
Thus, all the roots of Eq (3.5) have negative real parts. We can conclude the disease-free equilibrium E1 is locally asymptotically stable when τ=0.
When τ>0, λ11<0, λ13<0 and λ14<0 is available with R0<1. So we just need to consider the following equation.
λ+α+d+γe−λτ=0. | (3.6) |
Substitute λ=iω(ω>0) into Eq (3.6) and separate the real and imaginary parts, we can obtain:
{ω=γsin(ωτ),−α−d=γcos(ωτ). | (3.7) |
Thus,
{sin(ωτ)=ωγ,cos(ωτ)=−α+dγ. | (3.8) |
Adding the square of the two equations in Eq (3.8), we obtain:
ω2+(α+d)2−γ2=0. | (3.9) |
Therefore, we show the following assumption:
(H1)γ>α+d. |
Under (H1), Eq (3.9) has unique positive root: ω0=√γ2−(α+d)2. Then we substitute ω0 into Eq (3.8). Since ω0>0, r>0 we can get sin(ωτ)=ωr>0 and
τ(j)0=1ω0[arccos(−α+dγ)+2jπ]. | (3.10) |
Let λ(τ)=a(τ)+iω(τ) be the root of Eq (3.6). If τ=τ(j)0, Eq (3.6) has a pair of imaginary roots: ±iω0 with a(τ(j)0)=0, ω(τ(j)0)=ω0=√γ2−(α+d)2 (j=0,1,2,⋯), where τ(j)0 is given by Eq (3.10).
Lemma 3.2. If (H1) holds, zk=ω2k and h′(zk)≠0, we have the transversality condition:
Re(dλdτ)−1|τ=τ(j)0=Re(dτdλ)|τ=τ(j)0=1γ2>0(j=0,1,2,⋯). |
When R0>1, we can get λ13λ14=Π2<0 according to Eq (3.4), the characteristic equation has roots whose real parts are greater than zero. Thus, the disease-free equilibrium E1 is unstable.
Theorem 3.1. For system (2.1), there always exists equilibrium E1. And we have the following conclusions.
1) If R0<1, the equilibrium E1 is locally asymptotically stable when τ=0. Further, if (H1) holds, the system undergoes Hopf bifurcation when τ=τ(j)0(j=0,1,2,⋯). E1 is locally asymptotically stable for 0<τ<τ(0)0 and is unstable for τ>τ(0)0, where τ(j)0 is given by Eq (3.10).
2) If R0>1, the disease-free equilibrium E1 is unstable.
For the endemic equilibrium E2, the characteristic equation of system (3.3) becomes:
λ4+q1λ3+q2λ2+q3λ+q4+γe−λτ(λ3+p1λ2+p2λ+p3)=0, | (3.11) |
where
q1=D1+d,q2=dD1+D2,q3=D3+dD2,q4=dD3,p1=D1+C1,p2=D2+C2,p3=D3+C3,D1=u+d+c+α+β1A∗2+β2I∗2−β1S∗2+ρ1+ρ2+2d,D2=(α+β1A∗2+β2I∗2+d)(−β1S∗2+ρ1+ρ2+d)+β2S∗2(β1A∗2+β2I∗2)+(α+β1A∗2+β2I∗2−β1S∗2+ρ1+ρ2+2d)(u+d+c)−ρ1β2S∗2,D3=(u+d+c)[(α+β1A∗2+β2I∗2+d)(−β1S∗2+ρ1+ρ2+d)+β1S∗2(β1A∗2+β2I∗2)]−ρ1β2S∗2(α+β1A∗2+β2I∗2+d)+ρ1(2mI∗2+β2S∗2)(β1A∗2+β2I∗2),C1=−α,C2=−α(u+2d+c−β1S∗2+ρ1+ρ2)+ρ2(β1A∗2+β2I∗2),C3=−[α(u+d+c)(−β1S∗2+ρ1+ρ2)+ρ1(β1A∗2+β2I∗2)(u+2mI∗2)−αρ1β2S∗2+ρ2(u+d+c)(β1A∗2+β2I∗2)]. |
When τ=0, the Eq (3.11) can be transformed into the following form:
λ4+(q1+γ)λ3+(q2+γp1)λ2+(q3+γp2)λ+(q4+γp3)=λ4+a1λ3+a2λ2+a3λ+a4=0, | (3.12) |
where q1, q2, q3, q4, p1, p2, p3 are shown in Eq (3.11), a1=q1+γ, a2=q2+γp1, a3=q3+γp2, a4=q4+γp3. According to the Routh-Hurwitz criterion, we show the following hypothesis:
(H2)a1a2−a3>0,a3(a1a2−a3)>a21a4,a4>0. |
If (H2) is satisfied, all eigenvalues of Eq (3.12) have negative real parts, the endemic equilibrium E2 of system (2.1) is locally asymptotically stable when τ=0.
When τ>0, we try to discuss the existence of Hopf bifurcation. We assume that λ=iω(ω>0) is a pure imaginary root of Eq (3.11). Substitute it into Eq (3.11) and separate the real and imaginary parts, we can obtain:
{ω4−q2ω2+q4=−γ(−p1ω2+p3)cos(ωτ)−γ(−ω3+p2ω)sin(ωτ),−q1ω3+q3ω=γ(−p1ω2+p3)sin(ωτ)−γ(−ω3+p2ω)cos(ωτ). | (3.13) |
Equation (3.13) derives to:
{sin(ωτ)=(−q1ω3+q3ω)(−p1ω2+p3)γ(−p1ω2+p3)2+γ(−ω3+p2ω)2−(ω4−q2ω2+q4)(−ω3+p2ω)γ(−p1ω2+p3)2+γ(−ω3+p2ω)2,cos(ωτ)=−(ω4−q2ω2+q4)(−p1ω2+p3)γ(−p1ω2+p3)2+γ(−ω3+p2ω)2−(−q1ω3+q3ω)(−ω3+p2ω)γ(−p1ω2+p3)2+γ(−ω3+p2ω)2. | (3.14) |
Adding the square of the two equations in Eq (3.13) and letting z=ω2, we get:
h(z)=z4+c1z3+c2z2+c3z+c4=0, | (3.15) |
where
c1=−2q2+q21−γ2,c2=q22+2q4−2q1q3−γ2(p21−2p2),c3=−2q2q4+q23−γ2(−2p1p3+p22),c4=q24−γ2p23. |
Discussion about the roots of Eq (3.15) is similar to that in [33]. According to this, we give the following lemma.
Lemma 3.3. Assume that δ1=c22−316c21, δ2=c3132−c1c28+c3, Φ=(δ22)2+(δ13)3, we can get the following results.
1) If c4<0, Eq (3.15) has at least one positive root.
2) If c4≥0, Eq (3.15) has positive roots if and only if z1>0 and h(z1)<0 when Φ≥0. On the contrary, when Φ<0, Eq (3.15) has positive root if and only if there exists at least one z∗∈{z1,z2,z3}, such that z∗>0 and h(z∗)≤0, where z1, z2 and z3 are the roots of h′(z).
If h(z) satisfies the existence condition for positive roots in Lemma 3.3, we hypothesize the Eq (3.15) has n(n=1,2,3,4) positive roots marked as z1<z2<⋯<zn (n=1,2,3,4). Substituting ωn=√zn into Eq (3.14), we can get the expression of τ:
τ(j)n={1ωn[arccos(Pn)+2jπ], Qn≥0,1ωn[2π−arccos(Pn)+2jπ], Qn<0, | (3.16) |
where Qn=sin(ωτ)|ω=ωn,τ=τ(j)n,Pn=cos(ωτ)|ω=ωn,τ=τ(j)n, sin(ωτ), cos(ωτ) are given in Eq (3.14).
Let λ(τ)=a(τ)+iω(τ) be the root of Eq (3.11). If τ=τ(j)n, characteristic Eq (3.11) has a pair of imaginary roots: ±iωn with a(τjn)=0,ω(τjn)=ωn(j=0,1,2,⋯,n=1,2,3,4).
Lemma 3.4. If (H2) holds and zn=ω2n, h′(zn)≠0, we have the transversality condition:
Re(dλdτ)−1|τ=τ(j)n=Re(dτdλ)|τ=τ(j)n=h′(ω2n)γ2[(−p1ω2n+p3)2+(−ω3n+p2ωn)2]≠0(j=0,1,2,⋯). |
Theorem 3.2. When R0>1 and (H2) hold, the equilibrium E2 is locally asymptotically stable for τ=0. When τ>0, we have the following conclusions.
1) If h(z) does not satisfy the condition for the existence of positive root in Lemma 3.3, the equilibrium E2 is locally asymptotically stable when τ>0.
2) If h(z) satisfies the condition of the existence of positive root in Lemma 3.3 and only has one positive root z1, the system (2.1) undergoes Hopf bifurcation at E2 when τ=τ(j)1 and h′(z1)>0. The equilibrium E2 is locally asymptotically stable for ∀0<τ<τ(0)1 and is unstable for ∀ τ>τ(0)1.
3) If h(z) satisfies the condition of the existence of positive root in Lemma 3.3 and has two positive roots z1, z2, the system (2.1) undergoes Hopf bifurcation at E2 when τ=τ(j)1 and τ=τ(j)2(j=0,1,2,⋯).Assuming that z2<z1, we can get h′(z1)>0,h′(z2)<0. Thus, if τ(0)1<τ(0)2, there exists k that makes: 0<τ(0)1<τ(0)2<τ(1)1<τ(1)2<⋯<τ(k)1<τ(k+1)1. When τ∈[0,τ(0)1)∪⋃ki=1(τ(i−1)2,τ(i)1), the equilibrium E2 is locally asymptotically stable and when τ∈⋃k−1i=0(τ(i)1,τ(i)2)∪(τ(k)1,+∞), the equilibrium E2 is unstable.
4) If h(z) satisfies the condition of the existence of positive root in Lemma 3.3 and has three positive roots z1, z2, z3, the system (2.1) undergoes Hopf bifurcation at E2 when τ=τ(j)1(n=1,2,3). Assuming that z3<z2<z1, we have h′(z1)>0,h′(z2)<0,h′(z3)>0. Similar to the analysis of (3), the stability of equilibrium E2 switches with the increase of τ. And finally, the equilibrium E2 is unstable.
5) If h(z) satisfies the condition of the existence of positive root in Lemma 3.3 and has four positive roots z1, z2, z3 and z4, the system (2.1) undergoes Hopf bifurcation at E2 when τ=τ(j)1(n=1,2,3,4). Assuming that z4<z3<z2<z1, we can obtain h′(z1)>0,h′(z2)<0,h′(z3)>0,h′(z4)<0. Similar to the analysis of (3), the stability of equilibrium E2 switches with the increase of τ. Finally, the equilibrium E2 is unstable.
In this section, we will deduce the normal form of Hopf bifurcation for system (3.3) with the help of multiple time scales method. Assuming that Eq (3.4) or (3.11) has a pair of pure imaginary roots λ=±iωk at equilibrium Ek=(S∗k,A∗k,I∗k,R∗k)(k=1,2), we can obtain the normal form as follows. The calculating details can be referred to Appendix.
˙G=χkMkτεG+χkHkτεG2ˉG, | (4.1) |
where χk,Mk,Hk are given in Eqs (A11) and (A16) in Appendix.
Then, we let G=γeiθk and substitute it into Eq (A17). The normal form of Hopf bifurcation in polar coordinate is as follows:
{˙r=Re(Mk)τεr+Re(Hkχk)r3,˙θk=Im(Mk)τε+Im(Hkχk)r2. | (4.2) |
The nontrivial equilibrium of Eq (4.2) corresponds to the periodic solution of system (2.1). So we can discuss the stability of periodic solution in system (2.1) by studying the stability of nontrivial equilibrium in Eq (4.2).
Theorem 4.1. If Re(χkMk)τεRe(χkHk)τc<0(k=1,2) holds, the Eq (4.2) has nontrivial fixed point r∗=√−Re(χkMk)τεRe(χkHk)τc, so the system (2.1) has periodic solution around the equilibrium Ek.
1) If Re(χkMk)τε<0, the periodic solution of system (2.1) is unstable. When τε>0(τε<0), the unstable periodic solution of Hopf bifucation is forward (backward).
2) If Re(χkMk)τε>0, the periodic solution of system (2.1) is stable. When τε>0(τε<0), the stable periodic solution of Hopf bifurcation is forward (backward).
Remark 1:
In this paper, in order to determine the time for booster vaccination, we propose an SAIR epidemic model with time delay for exploring the impact of booster vaccination time on the epidemic. We obtain the basic reproduction number R0 and the following conclusions.
1) R0<1: when τ=0, the disease-free equilibrium E1 is locally asymptotically stable, which means the epidemic can be effectively controlled; when τ=τ(j)0(j=1,2,⋯), equilibrium E1 undergoes Hopf bifurcation, where τ(j)0 is critical value of Hopf bifurcation near E1 given by Eq (3.10). In order to effectively control the epidemic, we need to control τ within τ(0)0. That is to say, we should vaccinate the booster shot within the critical time while the original antibody is invalid in a sense. The booster vaccination can generate antibodies, which can improving herd immunity and further control the outbreak of the epidemic. E2 is unstable in this condition.
2) R0>1: the disease-free equilibrium E1 is unstable and when τ=0, E2 is locally asymptotically stable under the preconditions of Theorem 3.2, the epidemic can be controlled effectively; when τ=τ(j)n(j=1,2,⋯,n=1,2,3,4), the equilibrium E2 undergoes Hopf bifurcation if Eq (3.15) has positive root, where τ(j)n is critical value of Hopf bifurcation near E2 given by Eq (3.16). In order to control the epidemic, we also need to control the booster vaccination time within the time delay that can make epidemic stability.
With analysis of equilibria, we can also get the direction of Hopf bifurcation by calculating the normal form according to Appendix. We can also obtain the stability of periodic solutions and the direction of Hopf bifurcation in Theorem 4.1.
In this section, we will carry out numerical simulations to verify our theoretical analysis. Then, we will simulate the effect of virus mutation on the critical time of the booster vaccination by controlling the infection rates β1 and β2. Finally, we will estimate the time required to complete a round of vaccination worldwide through fitting and prediction, and will propose reasonable suggestions about the booster vaccination time by analysis of the results.
Based on official statistics (https://ourworldindata.org/covid-vaccinations; http://2019ncov.chinacdc.cn/2019-nCoV/global.html), we can obtain the data of cure rates and vaccination rates for 211 countries. In order to ensure that the data can reflect the average, we take countries with more than 400,000 infections and eliminate outliers and missing values. Then, we screen cure rates for 52 countries and vaccination rates for 116 countries. Since most COVID-19 vaccines are inactivated vaccines with two doses, we considered the vaccination rates of all two doses. As a result, we make bar chart and line chart respectively, as shown in Figure 2.
For Figure 2(a), we can clearly see that the cure rates of 52 countries are almost at the same level, so we choose the mean value: 0.9383 as the value of u. As for vaccination rate, it's not difficult to find that the vaccination rates of these countries are mostly in the range of 0.2 to 0.6 see in Figure 2(b), so we choose c=0.3089,c=0.4126∈(0.3,0.6) respectively.
Based on the above consideration and the actual situation, we take the following two groups of parameters:
1) B=10, d=0.00714, c=0.0484, α=0.3098, γ=0.50, u=0.9383, β1=0.00046, β2=0.00092, ρ1=0.0025, ρ2=0.74, m=0.6;
2) B=100, d=0.00714, c=0.0484, α=0.4126, γ=0.35, u=0.9383, β1=0.00011, β2=0.00089, ρ1=0.1528, ρ2=0.84, m=0.6.
For the group of parameters (1): B=10, d=0.00714, c=0.0484, α=0.3098, γ=0.50, u=0.9383, β1=0.00046, β2=0.00092,ρ1=0.0025, ρ2=0.74, m=0.6, we calculate the non-negative equilibrium E1=[S∗1,A∗1,I∗1,R∗1]=[869,0,0,531] according to Eq (3.2). This group of parameters meets (H1), so R0<1, the equilibrium E1 is locally asymptotically stable when τ=0. Substitute parameters (1) into Eqs (3.8)–(3.10), we get ω0=0.3867,τ(0)0=5.8372,sin(ω0τ)=0.7734,cos(ω0τ)=−0.6339. According to Theorem 3.1, we know that E1 is locally asymptotically stable for any 0<τ<τ(0)0=5.8372 and it is unstable for any τ>τ(0)0=5.8372. Then we calculate the normal form of Hopf bifurcation and obtain Re(χkMk)>0,Re(χkHk)>0 from Eqs (A11) and (A16). According to Theorem 4.1, we can get when τε<0, Re(χkMk)τε<0, the periodic solution is unstable and the direction of Hopf bifurcation is backward.
When τ=0, it means people consistently vaccinate to avoid infection. In this case, the virus constantly mutates, making the original antibody unable to defend against the mutated strains, so the immune system continues to fail. We choose the initial values [1000,100, 50,500], the equilibrium is locally asymptotically stable shown in Figure 3. Although there are fluctuations in 0–4 years, it tends to be stable after the 4th year. There are no infected people and the disease is eliminated. This means that in this case, it is efficient for people to vaccinate against disease consistently, but this situation is under the perfect condition. Considering that it takes a certain time to complete a round of universal vaccination and the antibody are not instantaneous failure, this situation is basically impossible.
When τ=1.5∈(0,τ(0)0) with τ(0)0=5.8372, which means that we will get the booster vaccination 1.5 years later, we still choose the initial values [1000,100, 50,500] and the figure of numerical simulation is shown in Figure 4.
From Figure 4, we find that the disease-free equilibrium E1 is also locally asymptotically stable. The fluctuation in the first eight years is obvious, but it gradually stabilizes after 6th years, and the disease can also be eliminated. Although its regional stability speed is less than that τ=0, it can still ensure stability in the short time. That is to say, when we do the booster vaccination 1.5 years later, the immune effectiveness of the original antibody is lost in a sense, and people can vaccinate again to deal with the constantly changeable virus. This will enhance the immunity of the population and control the epidemic effectively.
Through the analysis of Hopf bifurcation direction, we obtain: when τ=τ(0)0=5.8372, Re(χkMk)>0,Re(χkHk)>0,τε<0, so there is backward Hopf bifurcation near τ(0)0, and the bifurcating periodic solution is unstable. In order to control the epidemic effectively, we hope to avoid this situation.
Remark 2: According to the numerical simulations of parameters (1), we can get: when τ<τ(0)0, the shorter time of booster vaccination is, the better the epidemic can be controlled. However, if τ>τ(0)0, the equilibrium E2 is unstable. In other words, if people are vaccinated after more than τ(0)0 years, the epidemic will be difficult to control.
For the group of parameters (2): B=100, d=0.00714, c=0.0484, α=0.4126, γ=0.35, u=0.9383, β1=0.00011, β2=0.00089, ρ1=0.1528, ρ2=0.84, m=0.6, we can also calculate the equilibria E1=[S∗1,A∗1,I∗1,R∗1]=[6498,0,0,7507], E2=[S∗2,A∗2,I∗2,R∗2] =[4051,307,47,9279] by Eq (3.2). We find that (H1) is not satisfied and (H2) is available. So the equilibrium E1 is unstable and E2 is locally asymptotically stable when τ=0. Substitute this group of parameters into Eqs (3.11)–(3.15), we obtain that h(z) has two different positive real roots z1≈0.5658>z2≈0.2869. According to Eq (3.16), we have τ(0)1=3.1114<τ(0)2=7.2011<τ(1)1=11.4645<τ(2)1=18.9323<τ(1)2=19.8176.
In accordance with Theorem 3.2, the system (2.1) has the conclusion: if τ∈(0,τ(0)1)⋃(τ(0)2,τ(1)1), the equilibrium E2 is locally asymptotically stable, and if τ∈(τ(0)1,τ(0)2)⋃(τ(1)1, +∞), E2 is unstable. Besides, the system undergoes Hopf bifurcation near E2. Through Eqs (A10)–(A17) and Theorem 3.2, we get: when τ=τ(0)1, Re(χ2M2)>0, Re(χ2H2)<0, τε>0, the periodic solution is stable; when τ=τ(0)2, Re(χ2M2)<0, Re(χ2H2)<0, τε<0, the periodic solution is stable; and when τ=τ(1)1, Re(χ2M2)>0, Re(χ2H2)<0, τε>0, the periodic solution is also stable.
When τ=0 which means people consistently vaccinate to avoid infection with instantaneous failure of immunity, we can see the equilibrium E2 is asymptotically stable with the initial value [5000,300,100, 10000] shown in Figure 5. In this figure, there are fluctuations in 0–6 years, but it tends to be stable after the 6th year. Similar to the situation of parameters (1), although the epidemic can be effectively controlled in a short time, this situation does not exist.
When τ=1∈(0,τ(0)1) where τ(0)1=3.1114, which means that people get booster vaccination after one year along with failure of original immunity, we can get the equilibrium E2 is also asymptotically stable shown in Figure 6. Although there are fluctuations in the epidemic in the previous 8 years, it tends to be stable after 10th year.
When τ∈(τ(0)1,τ(0)2)=(3.1114,7.2011), which means that people get booster vaccination between 3.1114 and 7.2011 years, the equilibrium E2 is unstable. Since Re(χ2M2)>0,Re(χ2H2)<0,τε>0 at τ=τ(0)1, system (2.1) has forward Hopf bifurcation near τ(0)1 and the periodic solution is stable. This is not ideal for controlling the outbreak.
When τ=8∈(τ(0)2,τ(1)1), which means that people get booster vaccination after 8 years and the original immunity fails at this time, the results of the numerical simulation are presented in Figure 7 with the initial value [5000,300,100, 10000]. Although the equilibrium is asymptotically stable in the long term, it continues to fluctuate in the next two decades and the epidemic can not be effectively controlled in a short time. Therefore, it will not be adopted.
Remark 3: Through numerical simulations, it can be found that as time delay τ changes, the stability of the equilibrium E2 constantly switches. However, in the short term, the epidemic can be controlled effectively only when τ<τ(0)1. And when τ>τ(0)1, the outbreak of epidemic will continue. Because the small variation of time delay will lead to great changes near τ(0)1, therefore, much emphasis should be put on controlling the booster vaccination time.
Next, we will simulate the virus mutation based on the second group of parameters. To keep the epidemic under control, the booster vaccination time must be kept within τ(0)1. Otherwise, antibody will fail, the epidemic will be difficult to control.
As the virus mutates and selective expression of genes, the infection rate and the drug resistance increases, so we consider that the infection rates β1 and β2 change within a certain range to simulate the virus mutation. And we use the critical time delay τ(0)1 to reflect the efficiency of vaccine. We find that when β1∈(0.00009,0.00014), β2∈(0.00053,0.00185) and other parameters remain unchanged, the stability and the direction of Hopf bifurcation is the same as situation of parameter (2). Therefore, we choose β1=0.00009,0.00010,0.00011,0.00012 and β2 is changed within (0.00053, 0.00185), and we obtain a graph about τ(0)1 with respect to the infection rates.
According to Figure 8, we can clearly see that τ(0)1 decreases as β2 increases when β1 is fixed, and the delay τ(0)1 decreases for the four decreasing values of β1 when β2 is fixed. In fact, as the virus continues to mutate and the rate of infection increases, the greater the probability that the original antibody will fail, requiring us to take action for booster vaccination in a shorter period of time. This is consistent with the results of our simulations. Therefore, in order to keep the outbreak under control and rather than the sustained outbreak, we need to reduce the booster vaccination time when the virus mutates.
It takes a certain amount of time for a region to complete a round of vaccination which cannot be ignored in the study of the booster vaccination time. So we should consider this in our model.
Now, we use the available data to predict the time to complete a round of vaccination. We obtain the time-varying data of the number of people vaccinated per 100 people in each country from the official data (https://ourworldindata.org/grapher/covid-vaccination-doses-per-capita?time=latest). And then we select the average as the level of the world through the data of 2020/12/1–2021/10/12. We choose the initial time as t=0 with day as a unit, perform fitting with logistic function on the data and get the equation as:
V(t)=94.846461091+157.68865977e−0.02193805x. | (5.1) |
The fitting and prediction result is shown in Figure 9. Through calculation, it is concluded the correlation coefficient between predicted value and actual value R2=0.9803, with strong correlation and good fitting effect.
In fact, due to individual variability, not all people can be vaccinated, especially for elderly people with some underlying diseases. So it is not possible to achieve the vaccination rate of 100%, which is consistent with the results of our fitting. In the Figure 9, we find that the growth of vaccination is already slow when t is at 450 days, so we consider that vaccination is complete at t=450 days, which means that a round of vaccination can be completed in 1.23 years with the world average.
From the simulation results in Subsection 5.2, we can obtain that under the second group of parameters, the booster vaccination can eventually reach stability within 3.11 years. Based on the fitting result in this subsection, the time to complete a round of vaccination is predicted to 1.23 year. Therefore, when the vaccination process starts within 3.11-1.23 = 1.88 years, it can ensure that the round of vaccination can be completed and the final stabilization can be guaranteed.
Next we illustrate the more general relationship between fitting results and system (2.1). Firstly, we give the definitions of some function and region in Table 2.
Labels | Explanations |
t1=τ(0)1(β2) | Function of critical booster vaccination time |
t0 | Time required to complete a round of vaccines |
t2=τ(0)1(β2)−t0 | Function of critical booster vaccination time with restriction |
D1={(β2,t)|t>τ(0)1(β2)} | Unstable region |
D2={(β2,t)|τ(0)1(β2)−t0<t<τ(0)1(β2)} | Buffered region |
D3={(β2,t)|0<t<τ(0)1(β2)−t0} | Stable region |
The virus mutation affects the infection rate β2 most predominantly, so we define the booster vaccination time function as t1=τ(0)1(β2) expressed as the relationship between the critical booster vaccination time and the infection rate β2. However, since vaccination is a large-scale behavior, the time to complete a round of vaccination is not negligible, assuming t0. Taking this factor into account in the system, we define the restricted booster vaccination time function as t2=τ(0)1(β2)−t0, expressed as the relationship between the critical booster vaccination time that allows vaccination to proceed smoothly and the infection rate β2. With the above two functions, we can divide the β2−t plane into three parts: D1={(β2,t)|t>τ(0)1(β2)}, D2={(β2,t)|τ(0)1(β2)−t0<t<τ(0)1(β2)} and D3={(β2,t)|0<t<τ(0)1(β2)−t0}, as shown in Figure 10(a). D1 describes the region where the critical booster vaccination time beyond the critical booster vaccination time, which is unstable according to the previous simulations. D3 describes the region where the booster vaccination time is less than the critical booster vaccination time after removing the time to complete a round of vaccination, which is clearly the stable state. The region between the two functions D1, D2 is not able to complete the vaccination before reaching instability with the current vaccination process, but if we intervene appropriately to speed up the vaccination process, we can complete the vaccination on time and eventually reach the stable state. So we call this region as buffer region.
Taking the second set of parameters and the results of the fit and prediction, we get β2=0.00089, t0=1.23, and we draw the function t1, t2 and the region D1–D3, as shown in Figure 10(b). Nowadays, most countries in the world require booster vaccination at 6 months, so we mark the point (0.00089, 0.5) in the figure as the current state. It is obvious that this point is in region D3, so the current state is the stable state. However, as the virus mutates, the transmission rate β2 will gradually increase, which we represent by the red line with arrow in Figure 10(b). We can clearly see that the state point reaches the region D2 when β2>0.0073, at which time we think we cannot complete a round of vaccination. However, we can change the size of the region D1 and D2 by shortening the vaccination process, so that the state point reaches again within the new stable region D3.
Remark 4: For the numerical simulation results, we have the following conclusions.
1) Through the simulation of the two groups, we find that the epidemic would be effectively controlled when the booster vaccination time is controlled within the critical delay τ(0)0 or τ(0)1, while the epidemic would be difficult to be controlled if the booster vaccination time was larger than the critical delay.
2) For the mutant strain, the infection rate will increase with the continuous mutation of the virus, which will lead to the continuous reduction of the critical booster vaccination time. That is detrimental to the control of the epidemic.
3) Not all countries are able to complete a round of mass vaccination within a short period of time, taking human, material and financial factors into account. For the current world average, we should keep the vaccination time within 1.88 years. Therefore, it is reasonable to carry out booster vaccination at 6 months, because it can keep the epidemic at a stable state. But as the virus mutates and the infection rate increases, we may reach a state within the buffer zone, at which point the vaccination process needs to be accelerated so that the state is in the new stable zone.
In this paper, considering of the characteristics of COVID-19 vaccine, we have constructed an SAIR model with time delay to study the booster vaccination time. We have studied the stability of the equilibria and the existence of Hopf bifurcation with the help of the basic reproduction number R0. Then we have analysed the stability and direction of Hopf bifurcation periodic solution by calculating the normal form with the multiple time scales method.
In Section 5, we carried out numerical simulations. Firstly, the changes of epidemic situation are simulated through two groups of parameters to verify the theoretical analysis results. Secondly, we considered the impact of mutant strains on the critical time of booster vaccination. With the continuous variation of virus and the continuous increase of infection rate, the critical time of booster vaccination should gradually be decreased to ensure the safety and effectiveness of epidemic prevention and control. Then, using the official vaccination data, we fitted and predicted the time required to complete a round of universal vaccination. Through the analysis, we define three regions: stable region, unstable region, and buffer region. Based on this, we judge that the current 6-month vaccination is reasonable, but with the mutation of the virus, it is necessary to accelerate the booster vaccination process or advance the booster vaccination time appropriately.
In the future, as the virus continues to mutate, it is possible that the virus will have a high rate of transmission but will gradually become less aggressive to humans, and COVID-19 may become a disease like influenza. What kind of dynamic phenomenon will be generated at that time? This is an issue for future research to explore.
This study was funded by Fundamental Research Funds for the Central Universities of China (Grant No. 2572022DJ06).
The authors declare that they have no competing interests.
System (3.3) can be written as:
X′(t)=AX(t)+BX(t−τ)+F[X(t),X(t−τ)], | (A1) |
where
X(t)=(Sk,Ak,Ik,Rk)T,X(t−τ)=(Sk(t−τ),Ak(t−τ),Ik(t−τ),Rk(t−τ))T,A=[ϵ1−β1S∗k−2mI∗k−β2S∗k0β1A∗k+β2I∗kϵ2β2S∗k00ρ1−u−d−c0αρ22mI∗k+u−d],B=[000γ00000000000−γ],F=[FSFAFIFR]=[ϵ3β1SkAk+β2SkIk0mI2k],ϵ1=−α−β1A∗−β2I∗k−d,ϵ2=β1S∗k−ρ1−ρ2−d,ϵ3=−mI2k−β1SkAk−β2SkIk. |
Then, make transformation: t↦t/τ for convenience, we have:
X′(t)=τAX(t)+τBX(t−1)+τF[X(t),X(t−1)]. | (A2) |
where A, B, F are given in Eq (A1).
We linearize system (A2): ˜X′(t)=τAX(t)+τBX(t−1). Let hk(k=1,2) be the eigenvector of the linear operator corresponding to the eigenvalue iωkτ and let h∗k(k=1,2) be the normalized eigenvector of the adjoint operator of the liner operator corresponding to the eigenvalues −iωkτ satisfying the inner product ⟨hk,h∗k⟩=¯h∗kThk=1. By a simple calculation, we get:
hk=(hk1,hk2,hk3,hk4)T,h∗k=(h∗k1,h∗k2,h∗k3,h∗k4)T, | (A3) |
where
hk1=[iωk−(β1S∗k−ρ1−ρ2−d)][iωk+(u+d+c)]ρ1β2S∗kρ1(β1A∗k+βI∗k),hk2=iωk+(u+d+c)ρ1,hk3=1,hk4=α[iωk−(β1S∗k−ρ1−ρ2−d)][iωk+(u+d+c)]−αρ1β2S∗kρ1(β1A∗k+βI∗k)(iωk+d+γe−iωkτ)+ρ2(iωk+u+d+c)+ρ1(2mI∗k+u)ρ1(iωk+d+γe−iωkτ), |
~h∗k1=−iωk+d+γeiωkτγeiωkτ,~h∗k2=[−iωk+α+β1A∗k+β2I∗k+d](−iωk+d+γeiωkτ)−αγeiωkτγeiωkτ(β1A∗k+β2I∗k),~h∗k3=[2mI∗k+u−iωk+u+d+c+β2S∗k(−iωk+α+β1A∗k+β2I∗k+d)(−iωk+d+γeiωkτ)γeiωkτ(β1A∗k+β2I∗k)(−iω+u+d+c)−αβ2S∗kγeiωkτγeiωkτ(β1A∗k+β2I∗k)(−iω+u+d+c)−(2mI∗k+β2S∗k)(−iωk+d+γeiωkτ)γeiωkτ(−iωk+u+d+c)],~h∗k4=1,dk=hk1¯~h∗k1+hk2¯~h∗k2+h3¯~h∗k3+hk4¯~h∗k4,h∗k1=~h∗k1dk,h∗k2=~h∗k2dk,h∗k3=~h∗k3dk,h∗k4=~h∗k4dk(k=1,2). |
We consider τ as a bifurcation parameter and take a time scale τ=τc+ετε, where τc=τ(j)n(n=0,1,2,3,4) in Eq (3.11) or (3.16). ε is the scale parameter without dimension and τε is the disturbance parameter. Thus, X(t) can be written as:
X(t)=X(T0,T1,T2,⋯)=∞∑k=1εkXk(T0,T1,T2,⋯), | (A4) |
where
X(T0,T1,T2,⋯)=[S(T0,T1,T2,⋯),A(T0,T1,T2,⋯),I(T0,T1,T2,⋯),R(T0,T1,T2,⋯)]T,Xk(T0,T1,T2,⋯)=[Sk(T0,T1,T2,⋯),Ak(T0,T1,T2,⋯),Ik(T0,T1,T2,⋯),Rk(T0,T1,T2,⋯)]T,k=1,2,Tj=εjt,j=1,2,⋯. |
X′(t) can be written as:
X′(t)=dX(t)dt=εdX1dt+ε2dX2dt+ε3dX3dt+⋯=ε(∂X1∂T0+ε∂X1∂T1+ε2∂X1∂T2)+ε2(∂X2∂T0+ε∂X2∂T1)+ε3∂X3∂T0+⋯=εD0X1+ε2D1X1+ε3D2X1+ε2D0X2+ε3D1X2+ε3D0X3+⋯, | (A5) |
where Di=∂∂Ti(i=1,2,3,⋯) is differential operator.
Using the Taylor expansion, we can obtain the formula of X(t−1):
X(t−1)=εX1[t−1,ε(t−1),ε2(t−1),⋯]+ε2X2[t−1,ε(t−1),ε2(t−1),⋯]+ε3X1[t−1,ε(t−1),ε2(t−1),⋯]+⋯=ε[X11−D1X11ε−D2X11ε2]+ε2[X21−D1X21ε]+ε3X31+⋯=εX11+ε2(X21−D1X11)+ε3(X31−D1X21−D2X11)+⋯, | (A6) |
where Xi1=Xi(t−1,εt,ε2t,⋯)(i=1,2,⋯).
Then we substitute Eqs (A4)–(A6) into Eq (A1). Corresponding to the coefficient of ε on both sides of the equation, we get the following expression:
{D0S1,k+τc(α+β1A∗k+β2I∗k+d)S1,k+τcβ1S∗kA1,k+τc(2mI∗k+β2S∗k)I1,k−τcγR11,k=0,D0A1,k−τc(β1A∗k+β2I∗k)S1,k−τc(β1S∗k−ρ1−ρ2−d)A1,k−τcβ2S∗kI1,k=0,D0I1,k−τcρ1A1,k+τc(u+d+c)I1,k=0,D0R1,k−τcαS1,k−τcρ2A1,k−τc(2mI∗k+u)I1,k+τcdR1,k+τcγR11,k=0. | (A7) |
Corresponding to the coefficient of ε2 on both sides of the equation, we obtain:
{D0S2,k+τc(α+β1A∗k+β2I∗k+d)S2,k+τcβ1S∗kA2,k−γτcD1R11,k+τc(2mI∗k+β2S∗k)I2,k−τcγR21,k=−τc(mI21,k+β1S1,kA1,k+β2S1,kI1,k)−D1S1,k+τε[−(α+β1A∗k+β2I∗k+d)S1,k−β1S∗kA1,k−(2mI∗k+β2S∗k)I1,k+γR11,k],D0A2,k−τc(β1A∗k+β2I∗k)S2,k−τc(β1S∗k−ρ1−ρ2−d)A2,k−τcβ2S∗kI2,k=−D1A1,k+τc(β1S1,kA1,k+β2S1,kI1,k)+τε[(β1A∗k+β2I∗k)S1,k+(β1S∗k−ρ1−ρ2−d)A1,k+β2S∗kI1,k],D0I2,k−τcρ1A2,k+τc(u+d+c)I2,k=−D1I1,k+τε[ρ1A1,k−(u+d+c)I1,k],D0R2,k−τcαS2,k−τcρ2A2,k−τc(2mI∗k+u)I2,k+τcdR2,k+τcγR21,k=−D1R1,k+γτcD1R11,k+τcmI21,k+τε[αS1,k+ρ2A1,k+(2mI∗k+u)I1,k−dR1,k−γR11,k]. | (A8) |
For ε3−order terms, we have:
{D0S3,k+τc(α+β1A∗k+β2I∗k+d)S3,k+τcβ1S∗kA3,k+τc(2mI∗k+β2S∗k)I3,k−τcγR31,k=−D1S2,k−D2S1,k−τcγ(D1R21,k−D2R11,k)−τc[2mI1,kI2,k+β1(S1,kA2,k+S2,kA1,k)+β2(S1,kI2,k+S2,kI1,k)]+τε[−(α+β1A∗k+β2I∗k+d)S2,k−β1S∗kA2,k−(2mI∗k+β2S∗k)I2,k]+τεγ(R21,k−D1R11,k)+τε(−mI21,k−β1S1,kA1,k−β2S1,kI1,k),D0A3,k−τc(β1A∗k+β2I∗k)S3,k−τc(β1S∗k−ρ1−ρ2−d)A3,k−τcβ2S∗kI3,k=−D1A2,k−D2A1,k+τc[β1(S1,kA2,k+S2,kA1,k)+β2(S1,kI2,k+S2,kI1,k)]+τε[(β1A∗k+β2I∗k)S2,k+(β1S∗k−ρ1−ρ2−d)A2,k+β2S∗kI2,k]+τε(β1S1,kA1,k+β2S1,kI1,k),D0I3,k−τcρ1A3,k+τc(u+d+c)I3,k=−D1I2,k−D2I1,k+τε[ρ1A2,k−(u+d+c)I2,k],D0R3,k−τcαS3,k−τcρ2A3,k−τc(2mI∗k+u)I3,k+τcdR3,k+τcγR31,k=−D1R2,k−D2R1,k+τcγ(D1R21,k+D2R11,k)+τc2mI1,kI2,k+τε[αS2,k+ρ2E2,k+(2mI∗k+u)I2,k−dR2,k]−τεγ(R21,k−D1R11,k)+τεmI21,k. | (A9) |
The solution of Eq (A7) can be expressed as the following form:
X1(T0,T1,T2,⋯)=G(T1,T2,T3,⋯)eiωτcT0hk+ˉG(T1,T2,T3,⋯)e−iωτcT0ˉhk,k=1,2, | (A10) |
where hk is given in (A3). Substitute Eq (A10) into the right hand side of Eq (A8), and mark the coefficient before eiωτcT0 as vector m1. Through solvability condition <h∗k,m1>=0, we can get the expression of ∂G∂T1:
∂G∂T1=χkMkτεG, | (A11) |
where χk=11+γτc(h4ˉh∗1−h4ˉh∗4)e−iωkτc,Mk=iωk.
Assume the solutions of (A8) are as follows:
S2,k=fk1eiωkτcT0G+ˉfk1e−iωkτcT0ˉG+gk1e2iωkτcT0G2+ˉgk1e−2iωkτcT0ˉG2+lk1GˉG,A2,k=fk2eiωkτcT0G+ˉfk2e−iωkτcT0ˉG+gk2e2iωkτcT0G2+ˉgk2e−2iωkτcT0ˉG2+lk2GˉG,I2,k=fk3eiωkτcT0G+ˉfk3e−iωkτcT0ˉG+gk3e2iωkτcT0G2+ˉgk3e−2iωkτcT0ˉG2+lk3GˉG,R2,k=fk4eiωkτcT0G+ˉfk4e−iωkτcT0ˉG+gk4e2iωkτcT0G2+ˉgk4e−2iωkτcT0ˉG2+lk4GˉG. | (A12) |
Then substitute Eq (A12) into Eq (A8), we have:
[κ1β1S∗k2mI∗k+β2S∗k−γe−iωkτc−β1A∗k−β2I∗kκ2−β2S∗k00−ρ1iωk+u+d+c0−α−ρ2−(2mI∗k+u)κ4][fk1fk2fk3fk4]=[bk1bk2bk3bk4], | (A13) |
[ν1β1S∗k2mI∗k+β2S∗k−γe−2iωkτc−(β1A∗k+β2I∗k)ν2−β2S∗k00−ρ12iωk+u+d+c0−α−ρ2−(2mI∗k+u)ν4][gk1gk2gk3gk4]=[dk1dk2dk3dk4], | (A14) |
[υ1β1S∗k2mI∗k+β2S∗k−γ−(β1A∗k+β2I∗k)υ2−β2S∗k00−ρ1u+d+c0−α−ρ2−(2mI∗k+u)d+γ][lk1lk2lk3lk4]=[ek1ek2ek3ek4], | (A15) |
where
κ1=iωk+α+β1A∗k+β2I∗k+d,κ2=iωk−(β1S∗k−ρ1−ρ2−d),κ4=iωk+d+γe−iωkτc,bk1=τετc[−γτcMke−iωkτchk4−(α+β1A∗k+β2I∗k+d+Mk)hk1−β1S∗khk2−(2mI∗k+β2S∗k)hk3+γe−iωkτchk4],bk2=τετc[−Mkhk2+(β1A∗k+β2I∗k)hk1+(β1S∗k−ρ1−ρ2−d)hk2+β2S∗khk3],bk3=τετc[−Mkhk3+ρ1hk2−(u+d+c)hk3],bk4=τετc[−Mkhk4+γτcMkeiωkτchk4+αhk1+ρ2hk2+(2mI∗k+u)hk3−dhk4−γe−iωkτchk4],ν1=2iω+α+β1A∗k+β2I∗k+d,ν2=2iωk−(β1S∗k−ρ1−ρ2−d),ν4=2iωk+d+γe−2iωkτc,dk1=−(mh2k3+β1hk1hk2+β2hk1hk3),dk2=β1hk1hk2+β2hk1hk3,dk3=0,dk4=mh2k3,υ1=α+β1A∗k+β2I∗k+d,υ2=−(β1S∗k−ρ1−ρ2−d),ek1=−(2mhk3ˉhk3+β1hk1ˉhk2+β1hk2ˉhk1+β2hk1ˉhk3+β2hk3ˉhk1),ek2=β1hk2ˉhk1+β1hk1ˉhk2+β2hk1ˉhk3+β2hk3ˉhk1,ek3=0,ek4=2mhk3ˉhk3. |
We can solve f1,f2,f3,f4,g1,g2,g3,g4,l1,l2,l3,l4 from Eqs (A13)–(A15). Thus, we can get the expressions of S2,k,A2,k,I2,k,R2,k according to Eq (A12). Then we substitute Eqs (A10)–(A12) into the right expression of Eq (A9), and note the coefficient of eiωkτcT0 as vector m2. On the basis of solvability condition <h∗k,m2>=0, we obtain the expression of ∂G∂T2. Because τ2ε has less impact on normal form, so we can ignore τ2εG term. Therefore, we have:
∂G∂T2=χkHkτεG2ˉG, | (A16) |
where
Hk=[−2m(lk3hk3+gk3ˉhk3)−β1(lk2hk1+gk2ˉhk1+lk1hk2+gk1ˉhk2)−β2(lk3hk1+gk3ˉhk1+lk1hk3+gk1ˉhk3)]ˉh∗k1+[β1(lk2hk1+gk2ˉhk1+lk1hk2+gk1ˉhk2)+β2(lk3hk1+gk3ˉhk1+lk1hk3+gk1ˉhk3)]ˉh∗k2+2m(lk3hk3+gk3ˉhk3)ˉh∗k4,χk=11+γτc(h4ˉh∗1−h4ˉh∗4)e−iωkτc. |
Then, we let G→G/ε. Therefore, we get the normal form of Hopf bifurcation for system (2.1):
˙G=χkMkτεG+χkHkτεG2ˉG, | (A17) |
where χk,Mk,Hk are given in Eqs (A11) and (A16).
[1] |
E. Petersen, M. Koopmans, U. Go, D. H. Hamer, N. Petrosillo, F. Castelli, et al., Comparing SARS-CoV-2 with SARS-CoV and influenza pandemics, Lancet Infect. Dis., 20 (2020), e238–e244. https://doi.org/10.1016/S1473-3099(20)30484-9 doi: 10.1016/S1473-3099(20)30484-9
![]() |
[2] |
B. A. Connor, M. Couto-Rodriguez, J. E. Barrows, M. Rodriguez, M. Rogova, N. B. O'Hara, et al., Monoclonal antibody therapy in a vaccine breakthrough SARS-CoV-2 hospitalized Delta (B.1.617.2) variant case, Int. J. Infect. Dis., 110 (2021), 232–234. https://doi.org/10.1016/j.ijid.2021.07.029 doi: 10.1016/j.ijid.2021.07.029
![]() |
[3] |
A. Din, Y. Li, A. Yusuf, A. I. Alt, Caputo type fractional operator applied to Hepatitis B system, Fractals, 30 (2022), 2240023. https://doi.org/10.1142/S0218348X22400230 doi: 10.1142/S0218348X22400230
![]() |
[4] |
A. Din, Y. Li, F. M. Khan, Z. U. Khan, P. Liu, On Analysis of fractional order mathematical model of Hepatitis B using Atangana Baleanu Caputo (ABC) derivative, Fractals, 30 (2022), 2240017. https://doi.org/10.1142/S0218348X22400175 doi: 10.1142/S0218348X22400175
![]() |
[5] |
P. Liu, A. Din, Zenab, Impact of information intervention on stochastic dengue epidemic model, Alexandria Eng. J., 60 (2021), 5725–5739. https://doi.org/10.1016/j.aej.2021.03.068 doi: 10.1016/j.aej.2021.03.068
![]() |
[6] |
A. Din, Y. Li, T. Khan, G. Zaman, Mathematical analysis of spread and control of the novel corona virus (COVID-19) in China, Chaos, Solitons Fractals, 141 (2020), 110286. https://doi.org/10.1016/j.chaos.2020.110286 doi: 10.1016/j.chaos.2020.110286
![]() |
[7] |
J. Arino, F. Brauer, P. van den Driessche, J. Watmough, J. Wu, A model for influenza with vaccination and antiviral treatment, J. Theor. Biol., 253 (2008), 118–130. https://doi.org/10.1016/j.jtbi.2008.02.026 doi: 10.1016/j.jtbi.2008.02.026
![]() |
[8] |
D. Greenhalgh, Q. J. A. Khan, F. I. Lewis, Recurrent epidemic cycles in an infectious disease model with a time delay in loss of vaccine immunity, Nonlinear Anal., 63 (2005), e779–e788. https://doi.org/10.1016/j.na.2004.12.018 doi: 10.1016/j.na.2004.12.018
![]() |
[9] |
B. Ho, K. Chao, On the influenza vaccination policy through mathematical modeling, Int. J. Infect. Dis., 98 (2020), 71–79. https://doi.org/10.1016/j.ijid.2020.06.043 doi: 10.1016/j.ijid.2020.06.043
![]() |
[10] |
S. Djilali, S. Bentout, Global dynamics of SVIR epidemic model with distributed delay and imperfect vaccine, Results Phys., 25 (2021), 104245. https://doi.org/10.1016/j.rinp.2021.104245 doi: 10.1016/j.rinp.2021.104245
![]() |
[11] |
K. M. A. Kabir, J. Tanimoto, A cyclic epidemic vaccination model: Embedding the attitude of individuals toward vaccination into SVIS dynamics through social interactions, Physica, 581 (2021), 126230. https://doi.org/10.1016/j.physa.2021.126230 doi: 10.1016/j.physa.2021.126230
![]() |
[12] |
V. Ram, L. P. Schaposnik, A modified age-structured SIR model for COVID-19 type viruses, Sci. Rep., 11 (2021), 15194. https://doi.org/10.1038/s41598-021-94609-3 doi: 10.1038/s41598-021-94609-3
![]() |
[13] |
M. Shen, J. Zu, C. K. Fairley, J. A. Pagïn, L. An, Z. Du, et al., Projected COVID-19 epidemic in the United States in the context of theeffectiveness of a potential vaccine and implications for social distancingand face mask use, Vaccine, 39 (2021), 2295–2302. https://doi.org/10.1016/j.vaccine.2021.02.056 doi: 10.1016/j.vaccine.2021.02.056
![]() |
[14] |
A. Rajaeia, M. Raeiszadeh, V. Azimi, M. Sharifi, State estimation-based control of COVID-19 epidemic before and after vaccine development, J. Process Control, 102 (2021), 1–14. https://doi.org/10.1016/j.jprocont.2021.03.008 doi: 10.1016/j.jprocont.2021.03.008
![]() |
[15] |
K. L. Cooke, Stability analysis for a vector disease model, Rocky Mt. J. Math., 9 (1979), 31–41. https://doi.org/10.1216/RMJ-1979-9-1-31 doi: 10.1216/RMJ-1979-9-1-31
![]() |
[16] |
I. AI-Darabsah, A time-delayed SVEIR model for imperfect vaccine with a generalized nonmonotone incidence and application to measles, Appl. Math. Modell., 91 (2021), 74–92. https://doi.org/10.1016/j.apm.2020.08.084 doi: 10.1016/j.apm.2020.08.084
![]() |
[17] |
P. Yang, K. Wang, Dynamics for an SEIRS epidemic model with time delay on a scale-free network, Physica A, 527 (2019), 121290. https://doi.org/10.1016/j.physa.2019.121290 doi: 10.1016/j.physa.2019.121290
![]() |
[18] |
J. Liu, Bifurcation of a delayed SEIS epidemic model with a changing delitesbcence and nonlinear incidence rate, Discrete Dyn. Nat. Soc., 2017 (2017), 2340549. https://doi.org/10.1155/2017/2340549 doi: 10.1155/2017/2340549
![]() |
[19] |
Z. Zhang, S. Kundu, J. P. Tripathi, S. Bugalia, Stability and Hopf bifurcation analysis of an SVEIR epidemic model with vaccination and multiple time delays, Chaos, Solitons Fractals, 131 (2020), 109483. https://doi.org/10.1016/j.chaos.2019.109483 doi: 10.1016/j.chaos.2019.109483
![]() |
[20] |
A. Din, Y. Li, A. Yusuf, Delayed hepatitis B epidemic model with stochastic analysis, Chaos, Solitons Fractals, 146 (2021), 110839. https://doi.org/10.1016/j.chaos.2021.110839 doi: 10.1016/j.chaos.2021.110839
![]() |
[21] |
T. Kuniya, Global stability analysis with a discretization approach for an age-structured multigroup SIR epidemic model, Nonlinear Anal. Real World Appl., 12 (2011), 2640–2655. https://doi.org/10.1016/j.nonrwa.2011.03.011 doi: 10.1016/j.nonrwa.2011.03.011
![]() |
[22] |
X. Duan, J. Yin, X. Li, Global Hopf bifurcation of an SIRS epidemic model with age-dependent recovery, Chaos, Solitons Fractals, 104 (2017), 613–624. https://doi.org/10.1016/j.chaos.2017.09.029 doi: 10.1016/j.chaos.2017.09.029
![]() |
[23] |
Y. Cai, W. Wang, Stability and Hopf bifurcation of the stationary solutions to an epidemic model with cross-diffusion, Comput. Math. Appl., 70 (2015), 1906–1920. https://doi.org/10.1016/j.camwa.2015.08.003 doi: 10.1016/j.camwa.2015.08.003
![]() |
[24] |
Y. Zhang, J. Jia, Hopf bifurcation of an epidemic model with a nonlinear birth in population and vertical transmission, Appl. Math. Comput., 230 (2014), 164–173. https://doi.org/10.1016/j.amc.2013.12.084 doi: 10.1016/j.amc.2013.12.084
![]() |
[25] |
Y. Song, Y. Peng, T. Zhang, The spatially inhomogeneous Hopf bifurcation induced by memory delay in a memory-based diffusion system, J. Differ. Equations, 300 (2021), 597–624. https://doi.org/10.1016/j.jde.2021.08.010 doi: 10.1016/j.jde.2021.08.010
![]() |
[26] |
S. Wang, Y. Ding, H. Lu, S. Gong, Stability and bifurcation analysis of SIQR for COVID-19 epidemic model with time-delay, Math. Biosci. Eng., 18 (2021), 5505–5524. https://doi.org/10.3934/mbe.2021278 doi: 10.3934/mbe.2021278
![]() |
[27] |
Y. Ding, L. Zheng, Mathematical modeling and dynamics analysis of delayed nonlinear VOC emission system, Nonlinear Dyn., 109 (2022), 3157–3167. https://doi.org/10.1007/s11071-022-07532-1 doi: 10.1007/s11071-022-07532-1
![]() |
[28] |
Y. Ding, L. Zheng, J. Guo, Stability analysis of nonlinear glue flow system with delay, Math. Methods Appl. Sci., 30 (2022), 6861–6877. https://doi.org/10.1002/mma.8211 doi: 10.1002/mma.8211
![]() |
[29] |
P. van den Driessche, J. Watmough, Reproduction numbers and sub-threshold endemic equilibria for compartmental models of disease transmission, Math. Biosci., 180 (2002), 29–48. https://doi.org/10.1016/S0025-5564(02)00108-6 doi: 10.1016/S0025-5564(02)00108-6
![]() |
[30] |
M. D'Arienzo, A. Coniglio, Assessment of the SARS-CoV-2 basic reproduction number, R0, based on the early phase of COVID-19 outbreak in Italy, Biosaf. Health, 2 (2020), 57–59. https://doi.org/10.1016/j.bsheal.2020.03.004 doi: 10.1016/j.bsheal.2020.03.004
![]() |
[31] |
M. Al-Marwan, The basic reproduction number of the new coronavirus pandemic with mortality for India, the Syrian Arab Republic, the United States, Yemen, China, France, Nigeria and Russia with different rate of cases, Clin. Epidemiol. Global Health, 9 (2021), 147–149. https://doi.org/10.1016/j.cegh.2020.08.005 doi: 10.1016/j.cegh.2020.08.005
![]() |
[32] |
Y. Wang, J. Ma, J. Cao, Basic reproduction number for the SIR epidemic in degree correlated networks, Physica D, 433 (2022), 133183. https://doi.org/10.1016/j.physd.2022.133183 doi: 10.1016/j.physd.2022.133183
![]() |
[33] |
X. Li, J. Wei, On the zeros of a fourth degree exponential polynomial with applications to a neural network model with delays, Chaos, Solitons Fractals, 26 (2005), 519–526. https://doi.org/10.1016/j.chaos.2005.01.019 doi: 10.1016/j.chaos.2005.01.019
![]() |
1. | Zimeng Lv, Xinyu Liu, Yuting Ding, Dynamic behavior analysis of an SVIR epidemic model with two time delays associated with the COVID-19 booster vaccination time, 2023, 20, 1551-0018, 6030, 10.3934/mbe.2023261 | |
2. | Zhiliang Li, Lijun Pei, Guangcai Duan, Shuaiyin Chen, A non-autonomous time-delayed SIR model for COVID-19 epidemics prediction in China during the transmission of Omicron variant, 2024, 32, 2688-1594, 2203, 10.3934/era.2024100 | |
3. | Liping Wang, Xinyu Wang, Dajun Liu, Xuekang Zhang, Peng Wu, Dynamical analysis of a heterogeneous spatial diffusion Zika model with vector-bias and environmental transmission, 2024, 32, 2688-1594, 1308, 10.3934/era.2024061 |
Symbol | Descriptions |
S | Number of suspected people |
A | Number of asymptomatic people |
I | Number of infected people |
R | Number of recovered people |
B | Population migration rate |
d | Population natural mortality rate |
c | COVID-19 mortality rate of infected people |
β1 | Infection rate by contacting A |
β2 | Infection rate by contacting I |
ρ1 | Definitive diagnosis rate of A |
ρ2 | Self-healing rate of A |
u | Cure rate of I |
γ | Antibody failure rate of R |
α | Natural vaccination rate |
m | Vaccine distribution rate |
Labels | Explanations |
t1=τ(0)1(β2) | Function of critical booster vaccination time |
t0 | Time required to complete a round of vaccines |
t2=τ(0)1(β2)−t0 | Function of critical booster vaccination time with restriction |
D1={(β2,t)|t>τ(0)1(β2)} | Unstable region |
D2={(β2,t)|τ(0)1(β2)−t0<t<τ(0)1(β2)} | Buffered region |
D3={(β2,t)|0<t<τ(0)1(β2)−t0} | Stable region |
Symbol | Descriptions |
S | Number of suspected people |
A | Number of asymptomatic people |
I | Number of infected people |
R | Number of recovered people |
B | Population migration rate |
d | Population natural mortality rate |
c | COVID-19 mortality rate of infected people |
β1 | Infection rate by contacting A |
β2 | Infection rate by contacting I |
ρ1 | Definitive diagnosis rate of A |
ρ2 | Self-healing rate of A |
u | Cure rate of I |
γ | Antibody failure rate of R |
α | Natural vaccination rate |
m | Vaccine distribution rate |
Labels | Explanations |
t1=τ(0)1(β2) | Function of critical booster vaccination time |
t0 | Time required to complete a round of vaccines |
t2=τ(0)1(β2)−t0 | Function of critical booster vaccination time with restriction |
D1={(β2,t)|t>τ(0)1(β2)} | Unstable region |
D2={(β2,t)|τ(0)1(β2)−t0<t<τ(0)1(β2)} | Buffered region |
D3={(β2,t)|0<t<τ(0)1(β2)−t0} | Stable region |