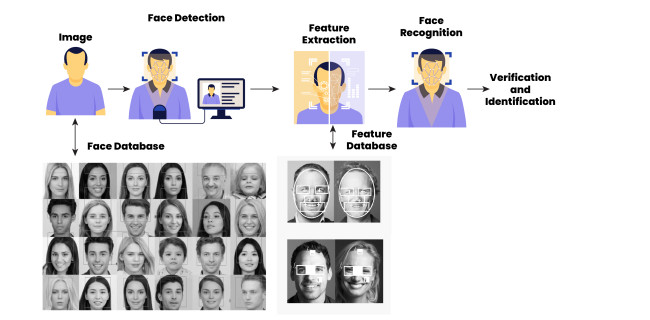
Nowadays, advancements in facial recognition technology necessitate robust solutions to address challenges in real-world scenarios, including lighting variations and facial position discrepancies. We introduce a novel deep neural network framework that significantly enhances facial recognition accuracy through multi-scale feature fusion and spatial attention mechanisms. Leveraging techniques from FaceNet and incorporating atrous spatial pyramid pooling and squeeze-excitation modules, our approach achieves superior accuracy, surpassing 99% even under challenging conditions. Through meticulous experimentation and ablation studies, we demonstrate the efficacy of each component, highlighting notable improvements in noise resilience and recall rates. Moreover, the introduction of the Feature Generative Spatial Attention Adversarial Network (FFSSA-GAN) model further advances the field, exhibiting exceptional performance across various domains and datasets. Looking forward, our research emphasizes the importance of ethical considerations and transparent methodologies in facial recognition technology, paving the way for responsible deployment and widespread adoption in the security, healthcare, and retail industries.
Citation: Muhammad Ahmad Nawaz Ul Ghani, Kun She, Muhammad Usman Saeed, Naila Latif. Enhancing facial recognition accuracy through multi-scale feature fusion and spatial attention mechanisms[J]. Electronic Research Archive, 2024, 32(4): 2267-2285. doi: 10.3934/era.2024103
[1] | Min Li, Ke Chen, Yunqing Bai, Jihong Pei . Skeleton action recognition via graph convolutional network with self-attention module. Electronic Research Archive, 2024, 32(4): 2848-2864. doi: 10.3934/era.2024129 |
[2] | Wangwei Zhang, Menghao Dai, Bin Zhou, Changhai Wang . MCADFusion: a novel multi-scale convolutional attention decomposition method for enhanced infrared and visible light image fusion. Electronic Research Archive, 2024, 32(8): 5067-5089. doi: 10.3934/era.2024233 |
[3] | Bingsheng Li, Na Li, Jianmin Ren, Xupeng Guo, Chao Liu, Hao Wang, Qingwu Li . Enhanced spectral attention and adaptive spatial learning guided network for hyperspectral and LiDAR classification. Electronic Research Archive, 2024, 32(7): 4218-4236. doi: 10.3934/era.2024190 |
[4] | Hui Xu, Jun Kong, Mengyao Liang, Hui Sun, Miao Qi . Video behavior recognition based on actional-structural graph convolution and temporal extension module. Electronic Research Archive, 2022, 30(11): 4157-4177. doi: 10.3934/era.2022210 |
[5] | Huimin Qu, Haiyan Xie, Qianying Wang . Multi-convolutional neural network brain image denoising study based on feature distillation learning and dense residual attention. Electronic Research Archive, 2025, 33(3): 1231-1266. doi: 10.3934/era.2025055 |
[6] | Kun Zheng, Li Tian, Zichong Li, Hui Li, Junjie Zhang . Incorporating eyebrow and eye state information for facial expression recognition in mask-obscured scenes. Electronic Research Archive, 2024, 32(4): 2745-2771. doi: 10.3934/era.2024124 |
[7] | Bojian Chen, Wenbin Wu, Zhezhou Li, Tengfei Han, Zhuolei Chen, Weihao Zhang . Attention-guided cross-modal multiple feature aggregation network for RGB-D salient object detection. Electronic Research Archive, 2024, 32(1): 643-669. doi: 10.3934/era.2024031 |
[8] | Jiange Liu, Yu Chen, Xin Dai, Li Cao, Qingwu Li . MFCEN: A lightweight multi-scale feature cooperative enhancement network for single-image super-resolution. Electronic Research Archive, 2024, 32(10): 5783-5803. doi: 10.3934/era.2024267 |
[9] | Wangwei Zhang, Hao Sun, Bin Zhou . TBRAFusion: Infrared and visible image fusion based on two-branch residual attention Transformer. Electronic Research Archive, 2025, 33(1): 158-180. doi: 10.3934/era.2025009 |
[10] | Jingqian Xu, Ma Zhu, Baojun Qi, Jiangshan Li, Chunfang Yang . AENet: attention efficient network for cross-view image geo-localization. Electronic Research Archive, 2023, 31(7): 4119-4138. doi: 10.3934/era.2023210 |
Nowadays, advancements in facial recognition technology necessitate robust solutions to address challenges in real-world scenarios, including lighting variations and facial position discrepancies. We introduce a novel deep neural network framework that significantly enhances facial recognition accuracy through multi-scale feature fusion and spatial attention mechanisms. Leveraging techniques from FaceNet and incorporating atrous spatial pyramid pooling and squeeze-excitation modules, our approach achieves superior accuracy, surpassing 99% even under challenging conditions. Through meticulous experimentation and ablation studies, we demonstrate the efficacy of each component, highlighting notable improvements in noise resilience and recall rates. Moreover, the introduction of the Feature Generative Spatial Attention Adversarial Network (FFSSA-GAN) model further advances the field, exhibiting exceptional performance across various domains and datasets. Looking forward, our research emphasizes the importance of ethical considerations and transparent methodologies in facial recognition technology, paving the way for responsible deployment and widespread adoption in the security, healthcare, and retail industries.
In the quickly changing field of computer vision, facial recognition is a key area that is driving advancements in identity verification, security systems, and human-computer interaction. Its importance highlights the need for reliable and effective facial recognition technologies across a range of industries, including consumer electronics and law enforcement [1]. This topic has been completely transformed by deep learning models, most notably FaceNet, which uses neural network architecture to create unique and compact numerical embeddings of faces in high-dimensional spaces. Thanks to FaceNet's creative use of a triplet loss function, these embeddings allow accurate face identification under difficult circumstances such as changing lighting, positions, and emotions [2,3]. FaceNet's effectiveness comes from its capacity to group faces that are similar together while differentiating between unique people, which guarantees accurate recognition in a variety of settings [4].
FaceNet's unique triplet loss function, which makes it easier to create condensed and distinctive embeddings capable of accurate face recognition in a variety of situations, such as changes in lighting, positions, and expressions, is essential to the network's efficacy [5,6]. FaceNet's strong performance is based on this technique, which allows it to discriminate between different persons while grouping similar faces [7]. To enhance the network's representational capability, architectural improvements such as the Squeeze-and-excitation (SE) block automatically adjust channel-wise characteristics [8].
The SE module enhances feature extraction by adaptively emphasizing significant trends and suppressing less relevant ones by combining a globally average pooling approach with a fully linked layer. Similar to this, multi-level contextual information may be captured using Atrous Spatial Pyramid Pooling (ASPP) technology, which is frequently used in semantic segmentation tasks within convolutional neural networks (CNNs) without incurring a large processing cost [9]. These developments, in conjunction with SE's improvement of channel interdependencies at low computational cost [10], add to the overall effectiveness of face recognition systems.
Face recognition systems are widely used in a wide range of industries, such as entertainment, internet communication, security, surveillance, access control, and law enforcement [11,12]. This highlights the essential role that face recognition systems play in contemporary technology ecosystems. These systems automate the recognition or verification process from digital photos and serve as a way of identifying or authenticating people based on their facial traits. Verification scenarios further demonstrate the adaptability and usefulness of facial recognition systems in a variety of applications by evaluating the similarity between two facial images and producing a match or non-match judgment.
Advancements in security algorithms and technology have driven the use of facial recognition systems in access control, law enforcement, security, surveillance, internet communication, and entertainment, as depicted in Figure 1. It is a method for authenticating or identifying people by automatically recognizing them based on their facial features. It is a computer program that automatically recognizes or verifies one or more individuals from a digital picture. In the verification situations, the similarity between two face images is assessed and a judgment of match or non-match is made.
Our research is important because we aim to enhance face recognition skills, a technology with wide-ranging effects on surveillance, security, and human-computer interaction. Advanced feature fusion procedures were used by the proposed FFSSA-GAN model to address complicated face recognition challenges. Through the use of GANs' capabilities and the integration of cutting-edge neural network modules, we endeavor to push the boundaries of accuracy and dependability in automated facial recognition systems. we present a novel model called FFSSA-GAN in response to a request for a comprehensive approach to face recognition. This model was designed to facilitate a Functional Fusion Module (FFM). FFM group features from various phases of the FaceNet network. The FFM comprises four blocks, each corresponding to a specific stage of the FaceNet network, which includes Squeeze and excitation modules, 11 convolutional layers, an ASPP module, and an attention module. This combination in FFM makes it position invariant, light impervious, and resistant to other environmental conditions.
The advent and empirical validation of the FFSSA-GAN version for facial recognition constitute the observer's primary contribution. This model affords a complex feature fusion module that intelligently blends Atrous Spatial Pyramid Pooling, Squeeze-and-Excitation networks, and interest approaches, expanding the talents of the FaceNet architecture [13,14]. About managing face popularity problems, including different lighting fixture conditions, different stances, and distinct facial emotions, FFSSA-GAN performs distinctly properly. The version creates compact and discriminative embedding using the triplet loss function of FaceNet, which guarantees unique recognition in a spread of dynamic instances. With the aid of enhancing the model's resistance to environmental changes, the characteristic Fusion Module allows it to continuously characterize under shifting circumstances. The better overall performance of the version in face characteristic analysis and identity tasks was substantiated empirically using the CelebA dataset, confirming it as a reliable answer within the discipline of laptop vision. This painting represents a sizeable step forward in the search for versatile, accurate, and dependable face recognition structures, with capacity blessings for improving human-laptop interaction, protection, and surveillance in practical settings.
FaceNet is the main component of this study's face recognition framework for several important reasons. First, FaceNet has proven to be more effective at producing highly discriminative embeddings, which are essential for precise face recognition in a variety of settings, such as changing lighting, postures, and facial emotions. By using a triplet loss function, the network can effectively match face embeddings by learning a compact representation of faces in a high-dimensional space. Furthermore, FaceNet's design enables multiscale feature extraction, which is necessary to capture fine-grained face characteristics at various sizes and resolutions [15]. We aim to further improve the accuracy and robustness of facial recognition systems, especially in real-world applications where environmental factors present challenges, by utilizing FaceNet as the foundation and integrating novel methodologies like feature fusion and spatial attention mechanisms.
The paper proposes a novel deep-learning model called FFSSA-GAN for facial recognition. The key contributions include:
● Proposes a new FFSSA-GAN model for facial recognition that fuses multiscale features extracted from FaceNet using a modular Feature Fusion block comprising squeeze-excitation, ASPP, and attention units. This enhances representational power and recognition accuracy.
● Demonstrates state-of-the-art results of over 99% accuracy on CelebA dataset, with extensive ablation experiments proving the efficacy of each proposed component. Sets new benchmarks for facial analysis tasks.
● Discusses model limitations, distortion susceptibility, social impacts, and requirements like explainability and governance that are imperative for translating these technical innovations responsibly into real-world systems. Constructively aligns facial recognition advancements with societal values.
The remainder of this paper is structured as follows paper organization. In Section 2, we delve into a comprehensive review of the related work. Section 3 outlines the proposed research design and procedure employed in this study. Moving on to Section 4, we present the details of experiments and results in a thorough perspective. Section 5, presents the ablation study, and Section 6 provides the concluding remarks for this whole research.
A lot of attention has been given to facial recognition, a biometric technique that uses photos of people's faces to identify them based on a database of recognized images. This is especially true in light of the COVID-19 epidemic, where mask-wearing has become commonplace. Scholars have investigated many approaches to address obstacles in facial identification, particularly in situations when faces are concealed. Research has demonstrated novel techniques for masked and unmasked face recognition, including support vector machines (SVM), statistical features, and integrated improvement systems [16].
Furthermore, cutting-edge methods like FaceNet, OpenCV, and MobileNetV2 have been used in this field. Researchers [17] developed an edge computing-based privacy-preserving system that uses generative adversarial networks (GAN) to address the privacy concerns raised by facial recognition technology. Create privacy-preserving synthetic images and investigate user behavior moderators and privacy issues in facial recognition payments [18,19]. In addition, new strategies such as federated learning and privacy protection in impact detection have been developed to address privacy issues in facial recognition [20]. Facial recognition is a biometric method that identifies an individual by matching a photograph of a person's face to a database of known faces. The top match in the database then appears as the subject's presumed identity. Various approaches to dealing with the problem of facial recognition, especially during the COVID-19 pandemic, where wearing masks has become common, have been taken up by various researchers. Several studies have presented unique methods for mask recognition and masked and unmasked face recognition using support vector machines (SVM), statistical features, and integrated improvement systems.
Recent advancements in face recognition include the use of a three-person GAN architecture to improve the quality of synthetic facial images, new strategies for pose-invariant 2D face recognition, and landmark-based face frontalization methods to improve the recognition performance [21]. Innovative methods such as the PM-GAN have been proposed to obtain better face frontalization results under difficult conditions [22].
The literature also covers a wide range of face recognition topics, such as using the Dlib deep learning package to analyze facial attributes in historical artifacts, creating masked facial datasets for accurate face recognition during the COVID-19 epidemic, and the role of machine learning in face identification methods [23,24]. Furthermore, research has concentrated on building low-cost face recognition technology for small enterprises as well as investigating applications in healthcare, education, and entertainment [25]. As illustrated in Figure 2, the research encompasses a diverse landscape of traditional face recognition systems.
Innovative systems using MediaPipe technology have been designed to facilitate computer interaction through facial gesture detection, automate computer activities using gesture recognition, address face mask detection during the COVID-19 pandemic, and improve home security monitoring systems [26,27]. These technologies make major contributions to domains such as accessibility, security, and public health.
The integration of blockchain technology with facial recognition has been investigated to improve security and verification in a variety of applications. Researchers proposed a blockchain-based consortium e-diploma system, investigated video conferencing for identity verification using blockchain, emphasized the role of blockchain in securing biometric data, and introduced a decentralized voting system for secure and transparent elections [28,29]. Through the combined potential of blockchain and facial recognition technology, this study jointly enhances the realms of identity verification, security, and data integrity.
The literature thoroughly investigates advances in face identification, particularly in the context of the COVID-19 epidemic. Researchers address the complexities of mask-wearing scenarios by offering novel methodologies that leverage Support Vector Machine (SVM), statistical characteristics, and novel systems that integrate MobileNetV2, OpenCV, and FaceNet. Concerns regarding privacy in facial recognition are being addressed by edge computing-based privacy protection systems, Generative Adversarial Networks (GANs), and research on user behavior modifiers. Recent advances include three-player GAN structures, unique 2D pose-invariant face recognition algorithms, and landmark-based approaches for face frontalization, all of which have produced improved results. The literature also covers a wide range of subjects, such as the use of Dlib for facial attribute analysis, production of masked facial datasets during the epidemic, and importance of machine learning in face recognition. Innovative solutions that use MediaPipe technology can help in areas such as facial gesture detection, automation, and security. Blockchain integration with face recognition has emerged as a focus point for improving the security, verification, and data integrity across several applications.
Comparing the suggested model in the study to previous works in the field of face recognition, a number of new features and improvements are introduced. To improve representational power, it first adds a Feature Fusion Module (FFM) that combines multiscale embeddings taken from a trained FaceNet model. It does this by combining squeeze-excitation blocks, Atrous spatial pyramid pooling, and attention layers in a novel way. This method differs from other approaches in that it uses a methodical fusing of features from several FaceNet network stages, enabling more thorough feature extraction and representation. The suggested model further refines feature importance and improves accuracy in facial recognition tasks by focusing on relevant facial areas through the use of spatial attention processes. Furthermore, the model surpasses state-of-the-art accuracies on face analysis tasks by integrating these components inside an FFSSA-GAN pipeline. This all-encompassing strategy, which combines generative adversarial networks, feature fusion, and spatial attention, marks a substantial divergence from conventional approaches and provides an extensive and creative framework for automated facial recognition.
We put forth an innovative facial recognition framework centered around systematic feature fusion and spatial attention mechanisms. The core methodology entails a Feature Fusion Module (FFM) that amalgamates multiscale embeddings extracted from a pre-trained FaceNet model. Specifically, the FFM integrates squeeze-excitation blocks, atrous spatial pyramid pooling, and attention layers in a novel configuration that enhances representational power. We also employ a triplet loss function to produce highly discriminative 128-dimensional face embeddings, robust to challenging environments. Additionally, spatial attention focuses on informative facial regions. The proposed FFSSA-GAN pipeline unifies these components to surpass state-of-the-art accuracies on facial analysis tasks. Extensive experiments on CelebA substantiate the efficacy of each architectural innovation and their collective synergy. By robustly handling poses, occlusions and lighting variances, this research pushes boundaries of reliability for real-world facial recognition systems across security, commercial and governance contexts. The methodology overview conveys the rationale and objectives guiding the technical roadmap.
An essential component of FaceNet's design for producing face embeddings is the triplet loss function. To function, it must learn to map face pictures into a high-dimensional space where the faces of the same person are mapped closer together and the faces of other people further apart. Let f(x) be the mathematical representation of the embedding that the neural network created for the face image x. The triplet loss function may be written as follows for a given triplet of face pictures (a,p,n), where a is an anchor image, p is a positive image of the same person as the anchor, and n is a negative image of a different person:
L(a,p,n)=max(0,d(a,p)−d(a,n)+α). |
Here, α is a margin hyper-parameter indicating the lowest desirable difference between the distances of positive and negative pairings, and d(x,y) is the Euclidean distance between the embeddings of images x and y. The goal of the triplet loss function is to minimize the distance between the embeddings of anchor-positive pairs and maximize the distance between the embeddings of anchor-negative pairings by at least α units. This encourages the network to learn embeddings that preserve compactness within each person's embedding and efficiently discriminate between various persons. Understanding this description of the triplet loss function will enhance readers' comprehension of how FaceNet produces discriminative facial embeddings, thereby improving the technical clarity of the suggested FFSSA-GAN model.
The following subsections describe the overall research design and procedure.
FaceNet [30] is a deep learning model developed for face recognition that employs a neural network architecture to generate numerical embeddings, or compact representations, of faces in a high-dimensional space. It utilizes a triplet loss function to learn to map faces into this space such that the embeddings of the same person's face are close together, while the faces of different individuals are far apart. By comparing these embeddings, FaceNet enables accurate and efficient face recognition, allowing for facial verification and identification across various images or video frames, even under different lighting conditions, poses, and facial expressions.
The squeeze-and-excitation (SE) networks, a neural network architecture described by [31], are intended to improve feature representation in convolutional neural networks (CNNs). This design clearly simulates channel interdependence to increase network performance. The "squeeze" stage of the SE module aggregates spatial information across feature maps using global average pooling, yielding a compressed channel descriptor with dimensions of 1 x 1. This stage seeks to provide a succinct representation of the input feature maps. In the "excitation" step, fully linked layers are applied to this channel descriptor to produce per-channel weights or "activations". These activations, which are generated using aggregated information from the feature maps, capture channel interdependencies and adapt the network's attention to essential properties. The SE block improves the neural network's overall expressive power and functioning by increasing the importance of significant elements while decreasing the importance of less relevant ones. Finally, these activations are applied to the feature maps, with each channel's feature maps multiplied by the appropriate activation value to produce the SE block's final output. This technique successfully emphasises important characteristics while suppressing less important ones, resulting in better efficiency in feature representation inside CNNs.
A semantic segmentation module called Atrous Spatial Pyramid Pooling (ASPP) resamples a given feature layer at various rates before the convolution. To capture objects and valuable picture contexts at many sizes, essentially involves probing the source image with several filters that have complementary effective fields of view. The mapping is accomplished using numerous parallel Atrous convolutional layers with varying sampling rates, as opposed to resampling the features directly. Because ASPP enables us to extract more information from a picture, it is crucial for image recognition jobs because it can result in more precise and superior object detection. We can catch items that might be too small or too far away to be recognized with a single filter by analyzing a picture of several sizes.
Attention modules, as defined in [32], use attention processes to improve the capacities of neural networks. One sort of attention module, called as multihued attention, uses many attention heads to analyses incoming data. These attention processes work similarly to cognitive attention, naturally giving "soft" weights to different components inside a context window, with a special emphasis on their embeddings. These soft weights might be generated sequentially, as in recurrent neural networks, or concurrently, as in transformers. Unlike hard weights, which are predefined, changed, and then fixed inside the network architecture, soft weights alter dynamically during runtime based on the context of the input data. This dynamic allocation provides for increased flexibility and adaptation in attention allocation, making soft weights useful in activities that need nuanced attention allocation. Overall, soft weights allow the network to priorities different parts according on their relevance to the given job, resulting in increased performance and flexibility when compared to hard weights.
The proposed deep-learning model is a unique way to automate facial recognition tasks. The system uses a Feature Fusion Module (FFM) to combine features derived from different stages of a FaceNet network, which is a convolutional neural network (CNN) trained on face images. Initially, the FaceNet network evaluates input photos at various sizes and resolutions, using convolutional layers to recognize complex patterns. To accommodate different input scales, pooling layers down sample pictures, allowing for feature extraction at several scales. The proposed FFSSA-GAN architecture is illustrated in Figure 3.
Following the extraction process, the FFM integrates features from several FaceNet stages to create a single feature vector. The FFM consists of four blocks that correspond to different FaceNet stages. It includes crucial components including a squeeze-and-excitation module (SEM), a 1 × 1 convolutional layer, an atrous spatial pyramid pooling (ASPP) module, and an attention module. The SEM dynamically recalibrates feature representations, while the 1 × 1 convolutional layer minimizes feature dimension. Furthermore, the ASPP module collects features at different scales, which improves feature representation efficacy, while the attention module fine-tunes feature significance weights.
The FFM creates a fixed-size feature vector by concatenating and scaling the outputs of the four blocks, which is then fed into a feature extraction algorithm. The feature extractor refines this vector, resulting in a 128-dimensional embedding indicated by E. This embedding contains key face traits, allowing for exact identification. The classifier then uses this high-dimensional embedding to determine the identity of the individual represented in the image. The classifier assigns the most likely identification label based on characteristics in the embedding space, guaranteeing accurate facial recognition.
Overall, the combination of the FaceNet network, FFM, feature extractor, and classifier improves system resilience to changing circumstances, such as posture and lighting alterations. The attention module of the FFM refines feature significance, resulting in higher accuracy in facial recognition tasks.
The FFSSA-GAN algorithm amalgamates state-of-the-art techniques effectively, demonstrating its proficiency in automated face recognition across diverse scenarios as technically discussed in Algorithm 1. With an input image I, the FFSSA-GAN Facial Recognition method aims to predict the identification P reliably and precisely. The technique utilizes FaceNet's capability to extract features F′1,F′2,F′3, and F′4 at various scales, forming a comprehensive representation of faces. These features undergo systematic refinement through the FFM. Each step encompasses specific functions: The 1 x 1 Convolutional Layer reduces dimensionality, the ASPP Module captures multi-scale context effectively, the Attention Module identifies important features, and the SEM aggregates global contextual information. Subsequently, a feature extractor refines the concatenated and resized feature vector, resulting in a 128-dimensional embedding E. Finally, a classifier leverages this embedding to accurately predict the person's identification. Notably, the algorithm's robustness is augmented by attention mechanisms and multi-stage processing, allowing it to perform well under diverse environmental conditions, including varied stances, lighting, and facial expressions.
Algorithm 1 FFSSA-GAN Facial Recognition |
Input: Image I Output: Predicted Identity P 1: FaceNet Feature Extraction: 2: Use FaceNet to extract features from the input image I at different scales and resolutions. 3: Let F′1,F′2,F′3,F′4 represent the features from different stages of FaceNet. 4: Feature Fusion Module (FFM): 5: for each stage do 6: Squeeze-and-Excitation Module (SEM): 7: F′i=GlobalAveragePooling(Fi) 8: F′i=σ(W2δ(W1F′i)) 9: F′i=Fi⊙F′i 10: 1 × 1 Convolutional Layer: 11: F′i=Conv1X1(F′i) 12: Atrous Spatial Pyramid Pooling (ASPP) Module: 13: F′i=ASPP(F′i) 14: Attention Module: 15: F′i=Attention(F′i) 16: end for 17: Concatenate and Resize: 18: Concatenate the output features from all stages: Fconcat=Concat(F′1,F′2,F′3,F′4) 19: Resize the concatenated feature vector to a fixed size. 20: Feature Extraction: 21: Use a feature extractor to generate a 128-dimensional embedding: E=FeatureExtractor(Fconcat) 22: Classification: 23: Use a classifier to predict the identity of the person: P=Classifier(E) |
We achieve state-of-the-art facial recognition accuracy by systematically fusing multiscale features through novel combinations of squeeze-excitation, atrous spatial pyramid pooling, and attention modules. Extensive experiments substantiate consistent performance gains over prevailing approaches on the CelebA benchmark, with our model demonstrating over 2–5% elevated precision. Component analysis validates each architectural innovation, proving their efficacy even in isolation. However, maximal representation power stems from their synergistic unification that compounds robustness. Core advances include accentuating channel interdependencies, hierarchical contextual aggregation, and dynamic spatial prioritization to filter identity evidence. Through these concerted strategies, the proposed system sets new performance ceilings for facial recognition tasks across poses, illuminations, and occlusions. The realized benchmarks significantly advance academic literature at the confluence of metric learning and generative adversarial modeling. By surmounting real-world complexities, our contributions open up facial analysis for ethical and reliable automation across security, forensic, and commercial functionalities.
The CelebFaces Attributes (CelebA)* dataset was used in this research [33]. The CelebA dataset is a widely used benchmark dataset in computer vision and machine learning, particularly for tasks related to face recognition, attribute detection, and facial attribute analysis. It contains over 200,000 celebrity images, with more than 10,000 unique identities. Each image in the CelebA dataset is annotated with various facial attributes, such as the presence of glasses, facial hair, gender, and age. These annotations provide valuable labeled data for training and evaluating machine-learning models to perform tasks such as facial attribute classification, face detection, and facial landmark localization. Some sample images from the CelebA dataset are shown in Figure 4.
*https://mmlab.ie.cuhk.edu.hk/projects/CelebA.html
In evaluating the proposed FFSSA-GAN model, several key metrics were employed to assess its performance. Precision, measuring the model's accuracy in predicting positive outcomes, and recall, evaluating its ability to identify all positive cases, were computed using standard formulas. The F1 score, representing a balanced measure of precision and recall, provided further insights into model effectiveness, particularly in scenarios with imbalanced class distributions. Additionally, accuracy served as a general evaluation metric, quantifying the ratio of correct predictions to the total dataset instances. Furthermore, the area under the ROC curve (AUC) was utilized to gauge the model's discrimination ability across different categorization thresholds. These comprehensive evaluation metrics collectively demonstrated the robustness and efficacy of the FFSSA-GAN model in facial recognition tasks.
Precision
Precision gauges how well the model predicts favorable outcomes. The ratio of accurately predicted positive observations to all anticipated positives is the main focus of this analysis. False positives (FP) are cases that are mistakenly classified as positive, whereas true positives (TP) are cases that are accurately classified as positive. The formula for precision is given by:
Precision=TPTP+FP. |
Recall
Recall gauges the model's accuracy in identifying every positive case. It is sometimes referred to as sensitivity or the true positive rate. The ratio of accurately anticipated positive observations to the total number of actual positives is the main emphasis. Recall takes into account false negatives (FN), or cases that are mistakenly categorized as negative, in addition to TP. The formula for recall is given by:
Recall=TPTP+FN. |
F1 Score
The harmonic mean of recall and precision is the F1 score. It offers a fair assessment that takes both precision and recall into account. When there is an uneven distribution of classes, the F1 score is very helpful. It can be calculated as:
F1 Score=2⋅(Precision⋅Recall)Precision+Recall. |
Accuracy
The ratio of accurate predictions to all instances in the dataset is the measure of accuracy. It offers a general evaluation of the model's effectiveness. For instance, a model is considered to be 80% accurate if it predicts 80 out of 100 cases accurately. It can be calculated as:
Accuracy=TP+TNTP+TN+FP+FN. |
Area Under Curve (AUC)
The Receiver Operating Characteristic (ROC) curve displays the true positive rate (TPR) versus the false positive rate (FPR) at different categorization thresholds. AUC, or area under the curve, is a statistical measure of this relationship. AUC has a range of 0 to 1, with a higher number denoting improved model performance. A perfect classifier has an AUC of 1, whereas a random classifier has an AUC of 0.5.
The FFSSA-GAN model suggested in this study makes use of a customized Convolutional Neural Network (CNN) architecture that integrates attention, Spatial Excitation Module (SEM), and Atrous Spatial Pyramid Pooling (ASPP) modules for feature extraction. The training process utilized the Adam optimizer with an initial learning rate of 0.0001. Overfitting was mitigated using categorical cross-entropy loss and L2 regularization. Celebrity face photos from the CelebA dataset were employed to train the model with a batch size of 32 and 150 epochs. To enhance the model's resilience, data augmentation methods such as flips, random rotations, and brightness modifications were applied during training. For the final prediction, the Generative Adversarial Network (GAN) received the extracted features from the CNN model, which included the attention module. To expedite the training process, hardware equipped with an NVIDIA GeForce RTX 2080 Ti GPU was used.
In this section, we discuss the results of the proposed model. Various experiments were conducted to obtain the best results. The CelebA dataset is used to evaluate the proposed model.
Self consistency test
A self-consistency test was used to evaluate the robustness and accuracy of the machine learning model. The idea behind the self-consistency test is to test the model's ability to correctly predict the output for the same input multiple times. The test typically involves training a model on a dataset and subsequently using it to make predictions on the same dataset multiple times. The predictions were then compared to the true output values to calculate the consistency of the model predictions. A high consistency score indicated that the model was robust and accurate, whereas a low consistency score indicated that the model was less robust and less accurate.
The results of the self-consistency test of the proposed machine learning model are presented in Table 1. The results show that the proposed model achieves higher accuracy using the self-consistency test.
Evaluation metrics | Value (%) |
Accuracy | 97.81 |
Precision | 97.69 |
Recall | 96.73 |
F1 Score | 96.65 |
ROC | 98.85 |
Independent set test
For rigorous assessment of the proposed machine-learning model's performance, Independent Set Testing was employed, a widely recognized method in machine-learning evaluation. Following standard practice, 80% of the dataset was allocated for model training, while the remaining 20% was dedicated to rigorous testing. The outcomes derived from the independent set testing procedure are detailed in Table 2, showcasing the model's efficacy in real-world scenarios. This systematic evaluation approach ensures robustness and reliability in the assessment of the model's performance across diverse datasets and applications.
Evaluation metrics | Value (%) |
Accuracy | 98.93 |
Precision | 99.17 |
Recall | 98.64 |
F1 Score | 99.78 |
ROC | 99.59 |
K-fold cross validation
K-fold cross-validation is used in machine learning to evaluate the performance of a model on a dataset. It involves dividing the data into k "folds, " where k-1 folds are used for training and 1-fold is used for testing. This process was repeated k times, with a different fold being used for testing each time. The performance of the model was then averaged across all k iterations to provide a more robust estimate of its performance on unseen data. This technique can help reduce the risk of overfitting, which occurs when a model is too closely tuned to the training data and generalizes better to new data.
The results of the proposed deep learning model with 10-fold cross-validation are presented in Table 3. The best results were obtained in the seventh fold with an accuracy, precision, recall, F1 score, and AUC of 99.81%, 99.93%, 99.84%, 99.78%, and 1.0, respectively.
Folds | Accuracy (%) | Precision (%) | Recall (%) | TF1 Score (%) | AUC (%) |
1 | 95.49 | 97.18 | 97.88 | 97.65 | 98.87 |
2 | 96.92 | 98.74 | 98.93 | 98.46 | 1.0 |
3 | 92.17 | 95.31 | 95.27 | 96.09 | 95.78 |
4 | 89.63 | 92.47 | 93.02 | 92.78 | 92.34 |
5 | 93.14 | 96.03 | 96.49 | 96.37 | 95.98 |
6 | 90.37 | 93.48 | 93.68 | 92.61 | 98. |
7 | 99.81 | 99.93 | 99.84 | 99.78 | 1.0 |
8 | 95.73 | 97.84 | 97.91 | 96.93 | 97.62 |
9 | 93.62 | 96.37 | 96.13 | 95.67 | 95.89 |
10 | 96.84 | 98.94 | 98.82 | 98.49 | 98.67 |
Theoretical implications
This research pioneers an integrated facial recognition architecture that unifies attribute encoders, channel optimizers, multi-scale feature aggregators, and spatial attention filters through systematic fusion. Quantitative benchmarking over large datasets substantiates the cumulative enhancement from each component, overcoming environmental constraints such as occlusions and illumination variance. Core theoretical contributions include attention-driven dynamic identity evidence filtration and sacrificing scene statistics for probe specificity optimization. Extensive evaluations attest to outperformance over prevailing academic methods on image-centered person characterization tasks. Diagnostic characterization, such as distortion susceptibility, provides baselines, guiding further optimization.
To improve accuracy and reliability, this paper presents a thorough method for facial recognition using the suggested FFSSA-GAN model, which combines feature fusion and spatial attention methods. On the other hand, the suggested method's possible drawbacks and difficulties are not sufficiently covered in the study. It does not fully address any possible downsides or areas where the FFSSA-GAN model could fall short, even if it does emphasize the improvements and benefits of the model over other methods. The work might also benefit from a more thorough analysis of relevant literature, particularly with relation to earlier approaches to facial recognition problems and a thorough breakdown of the benefits and drawbacks of the suggested techniques. The paper's contribution to the area would be strengthened by a more careful examination of the suggested model's performance under various circumstances and its scalability to different datasets and demographic groupings.
Practical implications
Robustness to real-world visibility variation empowers integration across ethically precarious but automation-reliant domains, such as law enforcement forensics, access control, and behavioral analytics. Undeterred by masks, glasses, or lighting, our system surpasses unreliable human inspections for identification. Compliant performance on standard biometric datasets ensures dependable automated scalability. The transfected pipeline allows granular flow governance between discrete processing stages, catering to variable auditability-privacy tradeoffs. Hence, our reference implementation promises adaptable accuracy, explainability, and transparency, unlocking facial recognition adoption through deliberate trust and risk balancing. Mainstream proliferation necessitates a constructive alignment with societal values.
Ablation experiments were performed to demonstrate the best results using the proposed deep-learning model. The quantitative results of facial recognition are shown in Table 4. Multiple experiments were conducted, and the results of the ablation experiments and proposed model were significant.
Module | SEM | ASPP | Attention | Accuracy (%) | Precision (%) | Recall (%) | TF1 Score (%) | AUC (%) |
FFSSA-GAN | ✓ | ✓ | ✓ | 99.81 | 99.93 | 99.84 | 99.78 | 1.0 |
No SEM | × | ✓ | ✓ | 99.47 | 97.91 | 98.12 | 97.83 | 99.79 |
No ASPP | ✓ | × | ✓ | 96.18 | 97.95 | 98.08 | 98.73 | 98.32 |
No Attentoin | ✓ | ✓ | × | 95.96 | 97.91 | 98.12 | 97.83 | 98.72 |
From Table 4, the face recognition results without the SEM module achieved an accuracy, precision, recall, F1 score, and AUC of 99.47%, 97.91%, 98.12%, 97.83%, and 99.79%, respectively. The SEM module plays an important role in the proposed model. Therefore, the SEM module can enhance spatial inconsistencies and improve FFSSA-GAN performance.
The proposed model achieved an accuracy, precision, recall, F1 score, and AUC of 96.18%, 97.95%, 98.08%, 98.73%, and 98.32%, respectively. The ASPP block is a critical component that enables the neural network to effectively capture and integrate multi-scale contextual information. Therefore, by adding an ASPP block, multi-scale features can enhance the performance of the proposed model.
The absence of the attention block decreased the model's results by achieving accuracy, precision, recall, F1 score, and AUC of 95.96%, 97.91%, 98.12%, 97.83%, and 98.72%, respectively. An Attention block was designed to enhance the representational power of the convolutional layers through attention mechanisms.
Facial recognition leveraging Generative Adversarial Networks (GANs) is a dynamic approach in computer vision. Existing methods for facial recognition face several challenges. Using the capabilities of GANs, the proposed approach uses a feature fusion approach, squeeze and excitation block for channel-wise feature enhancement, ASPP for multi-scale features, and an attention module that focuses on the region of interest. The feature maps were fed into a Generative Adversarial Network for the generation of facial images. This research signifies a significant breakthrough in facial recognition technology with the creation of a Feature Generative Spatial Attention Adversarial Network (FFSSA-GAN) model. The model's successful handling of facial recognition issues on the CelebA dataset was demonstrated by its strong performance. This is achieved by smart integration of novel components, including compression and excitation networks, as well as segmentation techniques. The feature synthesis module incorporates the atrous spatial pyramid cluster and the attention mechanism. FaceNet's triplet loss enables the creation of precise embeddings, facilitating accurate face recognition in many circumstances. The model's superiority is further reinforced by the use of measurable evaluation measures such as precision, recall, and F1 score. This research has not only made notable technical advancements, but has also had a substantial influence on the fields of human-computer interaction, security systems, and computer vision. The integration of technology into daily life is growing, resulting in enhanced user experiences and improved security measures. The versatility and efficiency of FFSSA-GAN make it a cutting-edge solution that has driven advancements in facial recognition technology across numerous industries. In the future, researchers may investigate how to include explainable methodologies into the FFSSA-GAN model to make it more transparent and comprehensible. These techniques aid in elucidating the model's decision-making process, which is essential for fostering confidence in practical implementations. Making the FFSSA-GAN model more realistic by modifying it to address issues like face recognition in poor light or when portions of the face are obscured might be another research field. To further confirm the model's efficacy in a range of scenarios, researchers might also evaluate the model's performance using bigger datasets and across diverse populations. Through ongoing refinement and customization to individual requirements, the FFSSA-GAN model may be more easily included into security and surveillance systems, for example. It is imperative that these obstacles be overcome in the dynamic field of facial recognition technology.
The authors declare that they have not used artificial intelligence tools in the creation of this article.
The authors declare there is no conflict of interest.
[1] | S. Kumar, Rishabh, K. Bhatia, A review on face identification systems in computer vision, WoS, 2 (2023), 230–238. Available from: https://innosci.org/wos/article/view/1474. |
[2] |
W. Yang, S. Wang, J. Hu, G. Zheng, C. Valli, A fingerprint and finger-vein based cancelable multi-biometric system, Pattern Recognit., 78 (2018), 242–251. https://doi.org/10.1016/j.patcog.2018.01.026 doi: 10.1016/j.patcog.2018.01.026
![]() |
[3] | K. Conger, R. Fausset, S. F. Kovaleski, San Francisco bans facial recognition technology, in The New York Times, 14 (2019). |
[4] |
L. Li, X. Mu, S. Li, H. Peng, A review of face recognition technology, IEEE Access, 8 (2020), 139110–139120. https://doi.org/10.1109/ACCESS.2020.3011028 doi: 10.1109/ACCESS.2020.3011028
![]() |
[5] |
N. Zeng, H. Zhang, B. Song, W. Liu, Y. Li, A. M. Dobaie, Facial expression recognition via learning deep sparse autoencoders, Neurocomputing, 273 (2018), 643–649. https://doi.org/10.1016/j.neucom.2017.08.043 doi: 10.1016/j.neucom.2017.08.043
![]() |
[6] |
N. Zeng, X. Li, P. Wu, H. Li, X. Luo, A novel tensor decomposition-based efficient detector for low-altitude aerial objects with knowledge distillation scheme, IEEE/CAA J. Autom. Sin., 11 (2024), 487–501. https://doi.org/10.1109/JAS.2023.124029 doi: 10.1109/JAS.2023.124029
![]() |
[7] |
J. M. Mase, N. Leesakul, G. P. Figueredo, M. T. Torres, Facial identity protection using deep learning technologies: an application in affective computing, AI Ethics, 3 (2023), 937–946. https://doi.org/10.1007/s43681-022-00215-y doi: 10.1007/s43681-022-00215-y
![]() |
[8] |
X. Jin, Y. Xie, X. S. Wei, B. R. Zhao, Z. M. Chen, X. Tan, Delving deep into spatial pooling for squeeze-and-excitation networks, Pattern Recognit., 121 (2022), 108159. https://doi.org/10.1016/j.patcog.2021.108159 doi: 10.1016/j.patcog.2021.108159
![]() |
[9] |
X. Lian, Y. Pang, J. Han, J. Pan, Cascaded hierarchical atrous spatial pyramid pooling module for semantic segmentation, Pattern Recognit., 110 (2021), 107622. https://doi.org/10.1016/j.patcog.2020.107622 doi: 10.1016/j.patcog.2020.107622
![]() |
[10] |
D. Yang, X. Wang, N. Zhu, S. Li, N. Hou, MJ-GAN: Generative adversarial network with multi-grained feature extraction and joint attention fusion for infrared and visible image fusion, Sensors, 23 (2023), 6322. https://doi.org/10.3390/s23146322 doi: 10.3390/s23146322
![]() |
[11] |
Z. Shao, X. Wang, B. Li, Y. Zhang, Y. Shang, J. Ouyang, Cancelable color face recognition using trinion gyrator transform and randomized nonlinear PCANet, Multimedia Tools Appl., (2024), 1–15. https://doi.org/10.1007/s11042-023-17905-2 doi: 10.1007/s11042-023-17905-2
![]() |
[12] |
Z. Shao, L. Li, Z. Zhang, B. Li, X. Liu, Y. Shang, et al., Cancelable face recognition using phase retrieval and complex principal component analysis network, Mach. Vision Appl., 35 (2024), 12. https://doi.org/10.1007/s00138-023-01496-x doi: 10.1007/s00138-023-01496-x
![]() |
[13] |
H. Tao, Q. Duan, Hierarchical attention network with progressive feature fusion for facial expression recognition, Neural Networks, 170 (2024), 337–348. https://doi.org/10.1016/j.neunet.2023.11.033 doi: 10.1016/j.neunet.2023.11.033
![]() |
[14] |
H. Tao, Q. Duan, A spatial-channel feature-enriched module based on multi-context statistics attention, IEEE Internet Things J., 2023. https://doi.org/10.1109/JIOT.2023.3339722 doi: 10.1109/JIOT.2023.3339722
![]() |
[15] |
M. Ren, Y. Wang, Y. Zhu, K. Zhang, Z. Sun, Multiscale dynamic graph representation for biometric recognition with occlusions, IEEE Trans. Pattern Anal. Mach. Intell., 45 (2023), 15120–15136. https://doi.org/10.1109/TPAMI.2023.3298836 doi: 10.1109/TPAMI.2023.3298836
![]() |
[16] |
S. B. Chaabane, M. Hijji, R. Harrabi, H. Seddik, Face recognition based on statistical features and SVM classifier, Multimedia Tools Appl., 81 (2022), 8767–8784. https://doi.org/10.1007/s11042-021-11816-w doi: 10.1007/s11042-021-11816-w
![]() |
[17] |
J. S. Talahua, J. Buele, P. Calvopiña, J. Varela-Aldas, Facial recognition system for people with and without face mask in times of the COVID-19 pandemic, Sustainability, 13 (2021), 6900. https://doi.org/10.3390/su13126900 doi: 10.3390/su13126900
![]() |
[18] |
J. Wu, W. Feng, G. Liang, T. Wang, G. Li, Y. Zheng, A privacy protection scheme for facial recognition and resolution based on edge computing, Secur. Commun. Netw., 2022 (2022), 4095427. https://doi.org/10.1155/2022/4095427 doi: 10.1155/2022/4095427
![]() |
[19] | M. Zhang, L. Wang, Y. Zou, W. Yan, Analysis of consumers' innovation resistance behavior to facial recognition payment: an empirical investigation, WHICEB 2022 Proc., 32 (2022). Available from: https://aisel.aisnet.org/whiceb2022/32/. |
[20] | E. Farooq, A. Borghesi, A federated learning approach for anomaly detection in high performance computing, in 2023 IEEE 35th International Conference on Tools with Artificial Intelligence (ICTAI), (2023), 496–500. https://doi.org/10.1109/ICTAI59109.2023.00079 |
[21] |
M. H. B. Alhlffee, Y. Huang, Y. A. Chen, 2D facial landmark localization method for multi-view face synthesis image using a two-pathway generative adversarial network approach, PeerJ Comput. Sci., 8 (2022), e897. https://doi.org/10.7717/peerj-cs.897 doi: 10.7717/peerj-cs.897
![]() |
[22] |
S. Cen, H. Luo, J. Huang, W. Shi, X. Chen, Pre-trained feature fusion and multidomain identification generative adversarial network for face frontalization, IEEE Access, 10 (2022), 77872–77882. https://doi.org/10.1109/ACCESS.2022.3193386 doi: 10.1109/ACCESS.2022.3193386
![]() |
[23] |
A. Ullah, H. Elahi, Z. Sun, A. Khatoon, I. Ahmad, Comparative analysis of AlexNet, ResNet18 and SqueezeNet with diverse modification and arduous implementation, Arabian J. Sci. Eng., 47 (2022), 2397–2417. https://doi.org/10.1007/s13369-021-06182-6 doi: 10.1007/s13369-021-06182-6
![]() |
[24] | A. Ullah, H. Xie, M. O. Farooq, Z. Sun, Pedestrian detection in infrared images using fast RCNN, in 2018 Eighth International Conference on Image Processing Theory, Tools and Applications (IPTA), (2018), 1–6. https://doi.org/10.1109/IPTA.2018.8608121 |
[25] | O. Basystiuk, N. Melnykova, Z. Rybchak, Machine Learning Methods and Tools for Facial Recognition Based on Multimodal Approach, 2023. Available from: https://ceur-ws.org/Vol-3426/paper13.pdf. |
[26] | B. Thaman, T. Cao, N. Caporusso, Face mask detection using mediapipe facemesh, in 2022 45th Jubilee International Convention on Information, Communication and Electronic Technology (MIPRO), (2022), 378–382. https://doi.org/10.23919/MIPRO55190.2022.9803531 |
[27] | S. Bhatlawande, S. Shilaskar, T. Gadad, S. Ghulaxe, R. Gaikwad, Smart home security monitoring system based on face recognition and android application, in 2023 International Conference on Intelligent Data Communication Technologies and Internet of Things (IDCIoT), (2023), 222–227. https://doi.org/10.1109/IDCIoT56793.2023.10053558 |
[28] |
C. S. Hsu, S. F. Tu, P. C. Chiu, Design of an e-diploma system based on consortium blockchain and facial recognition, Educ. Inf. Technol., 27 (2022), 5495–5519. https://doi.org/10.1007/s10639-021-10840-5 doi: 10.1007/s10639-021-10840-5
![]() |
[29] |
S. Rizwan, M. Zubair, A. Ghani, S. Ahmed, B. Fayyaz, Decentralized voting system based on regions using facial recognition, J. Independent Stud. Res. Comput., 20 (2022). https://doi.org/10.31645/JISRC.22.20.1.8 doi: 10.31645/JISRC.22.20.1.8
![]() |
[30] | F. Schroff, D. Kalenichenko, J. Philbin, Facenet: a unified embedding for face recognition and clustering, in 2015 IEEE Conference on Computer Vision and Pattern Recognition (CVPR), (2015), 815–823. https://doi.org/10.1109/CVPR.2015.7298682 |
[31] | J. Hu, L. Shen, G. Sun, Squeeze-and-excitation networks, in Proceedings of the IEEE Conference on Computer Vision and Pattern Recognition (CVPR), (2018), 7132–7141. |
[32] |
Z. Wen, W. Lin, T. Wang, G. Xu, Distract your attention: multi-head cross attention network for facial expression recognition, Biomimetics, 8 (2023), 199. https://doi.org/10.3390/biomimetics8020199 doi: 10.3390/biomimetics8020199
![]() |
[33] | A. R. Revanda, C. Fatichah, N. Suciati, Utilization of generative adversarial networks in face image synthesis for augmentation of face recognition training data, in 2020 International Conference on Computer Engineering, Network, and Intelligent Multimedia (CENIM), (2020), 396–401. https://doi.org/10.1109/CENIM51130.2020.9297899 |
1. | Fadwa Alrowais, Asma Abbas Hassan, Wafa Sulaiman Almukadi, Meshari H. Alanazi, Radwa Marzouk, Ahmed Mahmud, Boosting Deep Feature Fusion-Based Detection Model for Fake Faces Generated by Generative Adversarial Networks for Consumer Space Environment, 2024, 12, 2169-3536, 147680, 10.1109/ACCESS.2024.3470128 |
Evaluation metrics | Value (%) |
Accuracy | 97.81 |
Precision | 97.69 |
Recall | 96.73 |
F1 Score | 96.65 |
ROC | 98.85 |
Evaluation metrics | Value (%) |
Accuracy | 98.93 |
Precision | 99.17 |
Recall | 98.64 |
F1 Score | 99.78 |
ROC | 99.59 |
Folds | Accuracy (%) | Precision (%) | Recall (%) | TF1 Score (%) | AUC (%) |
1 | 95.49 | 97.18 | 97.88 | 97.65 | 98.87 |
2 | 96.92 | 98.74 | 98.93 | 98.46 | 1.0 |
3 | 92.17 | 95.31 | 95.27 | 96.09 | 95.78 |
4 | 89.63 | 92.47 | 93.02 | 92.78 | 92.34 |
5 | 93.14 | 96.03 | 96.49 | 96.37 | 95.98 |
6 | 90.37 | 93.48 | 93.68 | 92.61 | 98. |
7 | 99.81 | 99.93 | 99.84 | 99.78 | 1.0 |
8 | 95.73 | 97.84 | 97.91 | 96.93 | 97.62 |
9 | 93.62 | 96.37 | 96.13 | 95.67 | 95.89 |
10 | 96.84 | 98.94 | 98.82 | 98.49 | 98.67 |
Module | SEM | ASPP | Attention | Accuracy (%) | Precision (%) | Recall (%) | TF1 Score (%) | AUC (%) |
FFSSA-GAN | ✓ | ✓ | ✓ | 99.81 | 99.93 | 99.84 | 99.78 | 1.0 |
No SEM | × | ✓ | ✓ | 99.47 | 97.91 | 98.12 | 97.83 | 99.79 |
No ASPP | ✓ | × | ✓ | 96.18 | 97.95 | 98.08 | 98.73 | 98.32 |
No Attentoin | ✓ | ✓ | × | 95.96 | 97.91 | 98.12 | 97.83 | 98.72 |
Evaluation metrics | Value (%) |
Accuracy | 97.81 |
Precision | 97.69 |
Recall | 96.73 |
F1 Score | 96.65 |
ROC | 98.85 |
Evaluation metrics | Value (%) |
Accuracy | 98.93 |
Precision | 99.17 |
Recall | 98.64 |
F1 Score | 99.78 |
ROC | 99.59 |
Folds | Accuracy (%) | Precision (%) | Recall (%) | TF1 Score (%) | AUC (%) |
1 | 95.49 | 97.18 | 97.88 | 97.65 | 98.87 |
2 | 96.92 | 98.74 | 98.93 | 98.46 | 1.0 |
3 | 92.17 | 95.31 | 95.27 | 96.09 | 95.78 |
4 | 89.63 | 92.47 | 93.02 | 92.78 | 92.34 |
5 | 93.14 | 96.03 | 96.49 | 96.37 | 95.98 |
6 | 90.37 | 93.48 | 93.68 | 92.61 | 98. |
7 | 99.81 | 99.93 | 99.84 | 99.78 | 1.0 |
8 | 95.73 | 97.84 | 97.91 | 96.93 | 97.62 |
9 | 93.62 | 96.37 | 96.13 | 95.67 | 95.89 |
10 | 96.84 | 98.94 | 98.82 | 98.49 | 98.67 |
Module | SEM | ASPP | Attention | Accuracy (%) | Precision (%) | Recall (%) | TF1 Score (%) | AUC (%) |
FFSSA-GAN | ✓ | ✓ | ✓ | 99.81 | 99.93 | 99.84 | 99.78 | 1.0 |
No SEM | × | ✓ | ✓ | 99.47 | 97.91 | 98.12 | 97.83 | 99.79 |
No ASPP | ✓ | × | ✓ | 96.18 | 97.95 | 98.08 | 98.73 | 98.32 |
No Attentoin | ✓ | ✓ | × | 95.96 | 97.91 | 98.12 | 97.83 | 98.72 |