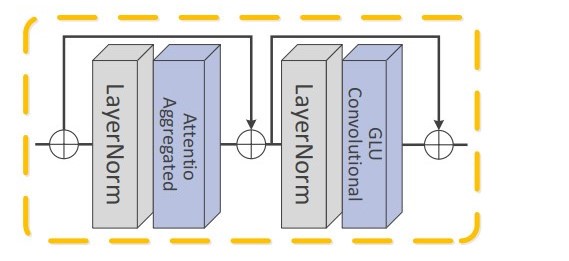
The fusion of infrared and visible images highlights the target while preserving detailed information, which helps to comprehensively capture the scene information. However, the existing methods continue to face challenges in managing the integration of global and local information, as well as enhancing the extraction of detailed image features, thus ultimately leading to constrained fusion outcomes. To enhance the fusion effect, this paper proposes a dual-branch residual attention-based infrared and visible image fusion network (TBRAFusion). The network utilizes two key modules, TransNext and the dual-branch residual attention (DBRA) module, which are used to process the input images in parallel to extract contrast and detail information. Additionally, an auxiliary function is incorporated into the loss function. Compared with mainstream fusion models, TBRAFusion achieves better fusion results and metrics through these improvements. The experimental results on the TNO dataset show that TBRAFusion improves the metrics in entropy (EN), spatial frequency (SF), sum ofcorrelation differences (SCD), and visual information fidelity (VIF) by 0.42%, 4%, 3.9%, and 1.2%, respectively. Tests on the MSRDS dataset show improvements of 1.7%, 5.4%, 9.6%, and 4.9% in EN, standard deviation (SD), SF, and SCD, respectively.
Citation: Wangwei Zhang, Hao Sun, Bin Zhou. TBRAFusion: Infrared and visible image fusion based on two-branch residual attention Transformer[J]. Electronic Research Archive, 2025, 33(1): 158-180. doi: 10.3934/era.2025009
[1] | Wangwei Zhang, Menghao Dai, Bin Zhou, Changhai Wang . MCADFusion: a novel multi-scale convolutional attention decomposition method for enhanced infrared and visible light image fusion. Electronic Research Archive, 2024, 32(8): 5067-5089. doi: 10.3934/era.2024233 |
[2] | Huimin Qu, Haiyan Xie, Qianying Wang . Multi-convolutional neural network brain image denoising study based on feature distillation learning and dense residual attention. Electronic Research Archive, 2025, 33(3): 1231-1266. doi: 10.3934/era.2025055 |
[3] | Zongsheng Zheng, Jia Du, Yuewei Zhang, Xulong Wang . CoReFuNet: A coarse-to-fine registration and fusion network for typhoon intensity classification using multimodal satellite imagery. Electronic Research Archive, 2025, 33(4): 1875-1901. doi: 10.3934/era.2025085 |
[4] | Xu Chen, Wenbing Chang, Yongxiang Li, Zhao He, Xiang Ma, Shenghan Zhou . Resnet-1DCNN-REA bearing fault diagnosis method based on multi-source and multi-modal information fusion. Electronic Research Archive, 2024, 32(11): 6276-6300. doi: 10.3934/era.2024292 |
[5] | Haijun Wang, Wenli Zheng, Yaowei Wang, Tengfei Yang, Kaibing Zhang, Youlin Shang . Single hyperspectral image super-resolution using a progressive upsampling deep prior network. Electronic Research Archive, 2024, 32(7): 4517-4542. doi: 10.3934/era.2024205 |
[6] | Meng Xu, Xiangyang Luo, Jinwei Wang, Hao Wang . Color image steganalysis based on quaternion discrete cosine transform. Electronic Research Archive, 2023, 31(7): 4102-4118. doi: 10.3934/era.2023209 |
[7] | Bingsheng Li, Na Li, Jianmin Ren, Xupeng Guo, Chao Liu, Hao Wang, Qingwu Li . Enhanced spectral attention and adaptive spatial learning guided network for hyperspectral and LiDAR classification. Electronic Research Archive, 2024, 32(7): 4218-4236. doi: 10.3934/era.2024190 |
[8] | Zengyu Cai, Liusen Xu, Jianwei Zhang, Yuan Feng, Liang Zhu, Fangmei Liu . ViT-DualAtt: An efficient pornographic image classification method based on Vision Transformer with dual attention. Electronic Research Archive, 2024, 32(12): 6698-6716. doi: 10.3934/era.2024313 |
[9] | Ling Li, Dan He, Cheng Zhang . An image filtering method for dataset production. Electronic Research Archive, 2024, 32(6): 4164-4180. doi: 10.3934/era.2024187 |
[10] | Jiange Liu, Yu Chen, Xin Dai, Li Cao, Qingwu Li . MFCEN: A lightweight multi-scale feature cooperative enhancement network for single-image super-resolution. Electronic Research Archive, 2024, 32(10): 5783-5803. doi: 10.3934/era.2024267 |
The fusion of infrared and visible images highlights the target while preserving detailed information, which helps to comprehensively capture the scene information. However, the existing methods continue to face challenges in managing the integration of global and local information, as well as enhancing the extraction of detailed image features, thus ultimately leading to constrained fusion outcomes. To enhance the fusion effect, this paper proposes a dual-branch residual attention-based infrared and visible image fusion network (TBRAFusion). The network utilizes two key modules, TransNext and the dual-branch residual attention (DBRA) module, which are used to process the input images in parallel to extract contrast and detail information. Additionally, an auxiliary function is incorporated into the loss function. Compared with mainstream fusion models, TBRAFusion achieves better fusion results and metrics through these improvements. The experimental results on the TNO dataset show that TBRAFusion improves the metrics in entropy (EN), spatial frequency (SF), sum ofcorrelation differences (SCD), and visual information fidelity (VIF) by 0.42%, 4%, 3.9%, and 1.2%, respectively. Tests on the MSRDS dataset show improvements of 1.7%, 5.4%, 9.6%, and 4.9% in EN, standard deviation (SD), SF, and SCD, respectively.
Image fusion can extract and integrate the image information from different sensors. Compared with the image from a single sensor, the image information generated after fusion is more abundant[1]. The fusion of an infrared image and a visible image is widely used[2]. Infrared sensing equipment can capture the thermal radiation information from the target and generate infrared images that highlight the target; however the details of infrared images are poor due to noise. A visible sensor can capture reflected light information and generate visible images containing details and color information; however, it can't highlight the target due to environmental influence. The fusion image of infrared and visible images can contain both contrast information and detail information, thus making such fusion images widely applicable in areas such as target tracking[3], target detection[4], semantic segmentation[5], and saliency detection[6].
Over the years, many fusion methods have been proposed to improve the fusion of infrared and visible images[7]. Traditional methods can be categorized into the following types: multi-scale transformation methods[8], sparse representation methods[9], subspace representation methods[10], and hybrid methods[11]. Multi-scale transformation methods enhance the richness of the fused images by combining the feature information extracted at different scales and orientations, thus resulting in fusion images with good visual effects[12,13,14,15]. Sparse matrix methods rely on two key factors: first, training overcomplete dictionaries on large datasets to obtain sparse representations; and second, reconstructing the fused image using sparse coefficients based on different fusion strategies[16,17,18]. Subspace clustering extracts the independent inherent structures from the original images by reducing the dimensionality of the image features and by projecting high-dimensional features into a low-dimensional space for the information fusion[19,20,21]. Hybrid methods combine the strengths of the above approaches [22].
With the rapid development of deep learning, neural networks are widely used in image fusion. Deep learning-based methods can be divided into three categories: convolutional neural networks[23] (CNN), autoencoders[24] (AE), and generative adversarial networks[25] (GAN). Liu et al. [26] were the first to apply CNNs to image fusion by establishing a mapping relationship between the source images and the focused images to learn the activity level measurements and fusion strategies. DenseFuse [27] combines encoding networks, convolutional layers, fusion, and dense blocks based on traditional CNNs, thus improving the deep feature extraction capabilities while retaining more multi-scale feature information. To further enhance the model's ability to extract deep features, Li et al. [28] proposed NestFuse, thereby incorporating both spatial and channel attention mechanisms to guide image fusion with different fusion weights through attention modules, thus enhancing the performance of the deep feature fusion. SEDRFuse [29] extracts intermediate and compensatory features through multiple residual blocks, thereby fusing the intermediate features with selected compensatory features using attention maps, which enables the model to make better use of the detail features and enhances the contrast of the fused image. To improve the accuracy of feature extraction, Park et al. [30] used the Transformer to enable the information exchange between the spatial domain and the channel domain, thus successfully removing redundant information from the image. Zhu et al. [31] proposed a method for multimodal spatial enhancement and edge shape correction, which further improved the processing accuracy of multimodal information.
GANs can estimate the probability distribution of detail and contrast information in images under unsupervised conditions. By applying different discriminative constraints to the generator, various fusion effects can be achieved. Ma et al. [32] applied the GAN model to image fusion tasks, thereby proposing FusionGAN. Based on FusionGAN, Ma et al. [33] improved it by proposing the Dual-Discriminator Conditional Generative Adersarial Netowork (DDCGAN), which addresses the issue of fusing images of different resolutions. In the study of the Generative Adversarial Network with Multiclassification Constraints (GANMcC)[34], a multi-classification task was used to optimize the discriminator, while primary and auxiliary functions were employed to supplement the gradient and contrast information, thus resulting in a more enriched and balanced gradient and contrast information in the fused image. Li et al. [35] combined multi-scale attention mechanisms with GANs to propose AttentionGAN, which, guided by two multi-scale attention mechanisms, allows the network to focus on more infrared target information and visible detail information, thereby enhancing the model's ability to extract multi-scal features. Rao et al. [36] added channel Transform and spatial Transform in the generator, thus enabling the generator to learn the correlation between spatial information and dimensional information.
Traditional methods achieve good visual results in image fusion by using different feature representations [37,38], though they have some drawbacks: traditional methods can fuse different source images using pixel-level fusion strategies, but they often rely on manually designed activity level measures and fusion strategies. This may lead to the loss of important feature information during the fusion process and the inability to adapt to complex scene requirements. Deep learning-based fusion methods also have some drawbacks: CNN methods lack the adequate extraction of cross-modal features, making it difficult to distinguish between the specific features of different modalities. Additionally, these methods also have weak global feature extraction capabilities, thus resulting in the loss of high-frequency information. GAN networks usually require constant adjustments to the discriminator's classification strategy to improve the fusion results, which often results in an imbalance between the features of visible and infrared images during fusion.
The keys to infrared and visible image fusion are as follows:
Fully extract the feature information from the images: For visible images, it is crucial to extract detailed information while not neglecting the contrast information contained within the visible image. In certain scenes, the contrast information from the visible image can serve as a supplement to enhance the contrast in the fused image. Similarly, for infrared images, it is important to extract the contrast information adequately. At the same time, the detail information contained in the infrared image should also be incorporated to enrich the fused image.
Balance contrast information and detail information during fusion: For infrared and visible images fusion, rich details help enhance the scene information, while the contrast emphasizes the target. It is essential to fully utilize the detailed information while maintaining the contrast between the target and the background during fusion.
Establish the relationship between the global and local features to fully utilize the feature information during fusion: It is critical to adequately extract features a from both the infrared and visible images in the same scene. However, if the network only focuses on the local information, then it may hinder the fusion effect. Therefore, establishing a connection between the global and local information is essential to improve the fusion quality.
To address these issues, we will focus on three aspects. First, visual Transformers have achieved significant results in image fusion. TransNext is a novel visual Transformer. In TransNext, the pixel aggregation attention block can establish feature correlations between global and local information, while the convolutional GLU can perform the preliminary detail feature extraction. In the fusion layer, all feature information can be fully utilized, which helps enhance the extraction of the detail features and establish global attention and long-range dependencies. Second, to maximize the extraction and preservation of the detail information, we combine a dual-branch attention mechanism with a residual module. This increases the weight of the detail information while ensuring that no information is lost. The attention mechanism in the dual-branch residual attention (DBRA) module enhances the extraction of detailed features, while the residual module better preserves the input feature information. Third, most fusion networks only focus on extracting the detail information from visible images, thus neglecting the fact that infrared images can also contain rich detail information in certain scenes. Therefore, in this paper, we introduce auxiliary gradient loss in the loss function to supplement the fused image with the infrared image detail information, thus improving the fusion effect. Our contributions are as follows:
● By combining the Vision Transformer and DBRA, we improve the extraction of deep features. TransNext can establish the relationship between the global and local feature information, while DBRA effectively extracts the detailed features. The integration of both can enhance the fusion of the detail information and improve the contrast of the fused image.
● To enrich and balance the fused image, we introduce a feature decomposition loss and an auxiliary gradient loss into the loss function. The feature decomposition loss computes the similarity during fusion and uses similarity constraints to balance the fusion of the detail and contrast information. The auxiliary gradient loss allows the infrared detail information to complement and enrich the fused image.
● TBRAFusion improves the quality of the fused image through a two-stage end-to-end training strategy. We compare our method with mainstream fusion methods using the TNO and MSRS datasets, both subjectively and objectively, and quantitatively analyze our method's performance using six metrics. The results show that our model effectively fuses infrared and visible images.
In this part, we review the development of Transform and its application in image fusion. At the same time, the structure and principle of DBRA and the latest visual Transform model TransNext are introduced.
Dosovitskiy [39] introduced the Vision Transformer (ViT), which achieved better results in image classification tasks compared to CNNs when trained on large-scale datasets. To enhance the performance of Vision Transformers, Liu et al. [40] proposed the Swing Transformer, which uses local convolution operations and computes self-attention within sliding windows, thus allowing the model to better extract multi-scale features and reduce the linear complexity of computing high-resolution images. Zamir et al.[41] combined gated-Dconv networks with multi-head attention using Restorm blocks to propose a lightweight and efficient mobile Natural Language Processing (NLP) architecture. To further reduce the high memory consumption and complexity of calculating self-attention for high-resolution images, Wang et al. [42] proposed a sparse attention mechanism, although this also reduced the ability to extract the detail features. Therefore, Shi [43] introduced TransNext, which replaces local attention mechanisms with aggregation attention, thus enabling the model to achieve a better global perception while extracting fine-grained features. Liu et al. [44] successfully enhanced the model's global feature extraction ability in multi-modal image processing by combining Transformers with CNNs. To further enhance the multi-modal information utilization, Zhu et al. [45] introduced the Swin Transformer with a shifted patch token strategy, thereby boosting the local perception ability of the Transformer and improving the feature fusion results.
In this paper, we use Restorm for the global feature extraction; then, we use TransNext to extract the detail information from the shared feature information and establish connections between the global and local information.
TransNext is a type of visual Transformer, where the dual-path design can focus on fine-grained attention for nearby features and coarse-grained attention for spatially downsampled features. Convolutional GLU is a convolutional algorithm that focuses on channel attention by fusing the nearest image features. The combination of convolutional GLU and aggregation attention significantly enhances the model's ability to extract local features and its robustness. The TransNext module can improve the extraction capability of shallow features and establish connections between the global and local information at a lower parameter cost. The structures of the aggregation attention module, TransNext, and convolutional GLU are shown in Figures 1–3, respectively.
The entire module contains three aggregation attention blocks and one self-attention block. The self-attention block includes two layer normalization (LN) layers, an aggregation attention (AA) layer, a convolutional GLU layer, and an additive operation for the connections. The convolutional GLU is a channel mixer that outperforms multi-layer perceptrons (MLPs) in certain tasks. The structure of GLU includes two linear projections: one projection outputs the result without modification, while the other applies an activation function to the result. To reduce the computational complexity, a convolution operation is introduced before the activation function. This helps to improve the efficiency while maintaining the performance of the channel mixing operation. The calculation process of aggregation attention is as follows: for the input image pixel set Xi,j∈I,V, where I∈RH×W×1, V∈RH×W×3 represents the input image; the input image is processed through the LN layer to obtain the pooled pixel set σ(X); and then, the input image pixel set is then segmented using a sliding window to obtain the processed pixel set ρ(i,j), with the sliding window size is K×K. Throughout the entire computation process, there are two paths that perform attention calculations on the original pixel set and the pooled pixel set. These two paths can establish fine-grained attention and global feature perception. The mathematical expression for the entire computation process is as follows:
PFAx(i,j)=S(Q(i,j)KTconcat√d+Bi,j)Vconcat, | (2.1) |
where S(⋅) represents the sigmoid, Kconcat=Concat(Kρ(i,j),Kσ(X)), d is the dimension, and the B is offset.
In the mathematical expression, it can be seen that after performing the Sigmoid calculation on the two paths and placing them in the same competition, both the fine-grained features and the global similarity comparisons can be comprehensively perceived. This is beneficial to enhance the extraction effect of the global feature information and to increase the model's receptive field.
To fully extract the detailed information from either visible images or infrared images, two different attention modules are employed for the feature extraction on the feature map. The two attention modules are the channel attention module[46] (CAB) and the spatial attention module[47] (SAB). After extracting the feature information, a residual module is used to skip-connect all the feature information. The process of CAB processing is as follows: after the feature map is input, the average pool (GAP) layer is used for processing, the dimension is 1 × 1 × C, the fully connected layer (FC) is used for reducing the dimension, ReLU is the activation function, and the second FC layer will be upgraded to 1 × 1 × C; and finally, the Sigmoid function is used to obtain a weight map, and the weight is multiplied by the original input feature map. The SAB processing process is as follows: after inputting the feature map, the dimension of the feature map is changed to H × W through two convolutions and ReLU; and then, the spatial weight map is obtained by using Sigmoid function, and the weight is multiplied by the original input feature map. The specific process is as follows: the input image {I∈RH×W×1,V∈RH×W×3} passes through two attention blocks on separate branches to extract the important features, thus producing the output results {FSABout,FCABout}; the weight maps from both branches are multiplied with the input; and finally, the outputs of the two branches are concatenated along the dimension, with a residual connection between the input and the output. The structure of DBRA is shown in Figure 4, where the kernel size is 3×3.
The expression for DBRA is as follows:
Fout=FSABout+FCAMout+FIn, | (2.2) |
where FSABout and FCABout represent the output results of the spatial attention block and the channel attention block, respectively.
From the computational process, using two branches to perform attention calculations separately allows for better extraction of detailed features, while the residual connection ensures that all feature information is preserved.
In this section, we introduce the overall framework of TBRAFusion and the structure of each module. Additionally, the design of the loss function in this paper is expressed in detail.
The encoder extracts and decomposes the image, including three modules: the shared coding block based on Restormer, TransNet based on convergent attention, and the two-branch residual attention module.
For ease of presentation, we define the following notation: the infrared image V∈RH×H×3, visible image I∈RH×W, shared coding Restormer block, TransNet, and DBRA are denoted by S(⋅), T(⋅), D(⋅) denote.
Shared coding Restormer block: The shared coding block performs a shallow feature extraction on the input infrared and visible images, which can be expressed as follows:
ΦSV=S(V),ΦSI=S(I), | (3.1) |
where ΦSV and ΦSI represent the shallow shared features extracted from the input image.
The TransNext block: Through the structure in graph 1, the TransNext block allows aggregated attention to enable the shared features of the input and to establish the global correlations, while the convolutional GLU can further perform a fine-grained feature extraction on the shared features, which is expressed as follows:
ΦTI=D(ΦSI),ΦTV=D(ΦSV), | (3.2) |
where ΦTI and ΦTV represent the extraction of global feature information from the shared features and the establishment of global dependencies.
DBRA block: DBRA is a lossless feature extraction module, and one of the keys to the fusion of infrared and visible images is to extract and retain the detail information, Therefore, we will use the DBRA module to extract features from the shared feature map of Restormer more fully. Considering that the infrared image may also have detail information in a certain scene, after extracting the detail information of the infrared image, it will be complementary to the detail information of the visible layer in the fusion layer. DBRA will process the space and channel separately in the form of double branches, and finally connect with the residual, which is expressed as follows:
ΦDI=D(ΦSI),ΦDV=D(ΦSV), | (3.3) |
where ΦDI and ΦDV represent local detail information in the extracted shared features.
The main role of the fusion layer is to fuse the contrast information and the detail information after the feature extraction. The contrast and detail information of the fusion layer comes from the TransNext layer and DBRA layer, which is expressed as follows:
ΦT=FT(ΦTI,ΦTV),ΦD=FD(ΦDI,ΦDV), | (3.4) |
where ΦT and ΦD represent global information and local information, respectively.
In the decoder DC(.), the extracted feature maps of infrared and visible images are used as the input after dimension splicing. The original image and the fused image in the first and second stages of training are used as the output, and finally the fused image is output through the decoder, which is expressed as follows:
Stage I: ˆI=D(ΦTI,ΦDI),ˆV=D(ΦTV,ΦDV),Stage II: ˆF=D(ΦT,ΦD). | (3.5) |
Our network is trained in two stages. The first stage aims to train a network model capable of extracting both the global and local information by reconstructing the original image. In the second stage, the encoder network parameters trained in the first stage are used to extract the frequency-specific feature information from the infrared and visible images. These features are fused in the fusion layer, and the combined features are input into a Restormer decoder block based on multi-head attention to reconstruct the final fused image. The entire network can be seen as a progressive cross-modal differential perception network. Since our network needs to extract information from different source images, we adopt a two-stage end-to-end training strategy to better fuse the infrared and visible information. The flow of training is shown in Figures 5 and 6.
Training stage 1: Infrared and visible images {I∈RH×W×,V∈RH×H×3} will be input into Restormer, respectively, to extract shallow features {ΦSI,ΦSV}; at the same time, the extracted feature maps will be coded as shared features. Then, the fine-grained feature information will be extracted and the global feature {ΦTI,ΦTV} mapping is established by TransNext. Finally, the dual-branch residual attention module will extract sufficient detailed features {ΦDI,ΦDV} from the input. After the visible and infrared feature maps are obtained, they will be spliced in dimensions and input into the decoder to obtain the reconstructed visible and infrared images. Training stage 2: Infrared and visible images {I∈RH×W,V∈RH×W×3} will be the input to the encoder after the first stage of training for the feature extraction. The extracted detail features and basic features will be fused through the fusion layer to obtain a fused image.
The loss function for the first stage is expressed as follows:
LItotal=Lir+α1Lvis+α2Ldecomp+α3Lgrad, | (3.6) |
where Lir and Lvis are the structural esimilarity errors after the infrared and visible image reconstruction, respectively(i.e., reconstruction errors). Ldecomp is the feature decomposition loss. α1,α2,α3 are the weight coefficients used to adjust the contribution of information during the training process.
The Lir is expressed as follows:
Lir=β1LIint(I,ˆI)+β2LSSIM(I,ˆI), | (3.7) |
where LSSIM is the structural similarity, which measures the quality loss between the reconstructed image and the original image through contrast and structural similarity. β1 and β2 are weight coefficients used to ensure the structural similarity between the original image and the reconstructed image. The detail information contained in the infrared image is extracted and supplemented into the fused image during the training phase of fusion.
The LSSIm formula is expressed as follows:
LSSIM(I,ˆI)=1−SSIM(I,ˆI). | (3.8) |
The Ldecomp formula is expressed as follows:
Ldecomp=(LCCC)2LSTCC=(CC(ΦSTI,ΦSTV))2CC(ΦCI,ΦCV)+ε, | (3.9) |
where CC(⋅) is the correlation coefficient operator, and the ε value is 1.01 to prevent the denominator from being 0.
The training loss function of the second stage is expressed as follows:
LIItotal=β1+β2Lgradaux+β4Ldecomp, | (3.10) |
Lint=1HW||If−max(Iir,Ivis)||1, | (3.11) |
Lgradmain=||∇If−∇Ivis||1, | (3.12) |
Lgradaux=||∇If−∇Iir||1. | (3.13) |
The symbol ∇ represents the gradient operation, and max(⋅) represents the maximum selection. Lgradmain is the gradient information of the visible image, Lgradaux is the gradient information of the infrared image, and α4,α5,α6,α7 are the weight coefficients used to balance the gradient information and the contrast information during the fusion process.
The design logic of the entire loss function is as follows. In the first stage of training, we need to extract the gradient information and contrast information to ensure the completeness of the information, while using the feature decomposition to establish the correlation between the infrared and visible image features. The contrast information is often the background of the image, while the gradient information represents the edge details of the target. The contrast information between the infrared and visible images usually has a high degree of correlation, whereas the gradient detail information often has a lower correlation. Therefore, Ldecomp is used to ensure the effectiveness of the feature decomposition. In the second stage, the focus is mainly on fusing the gradient and contrast information. By considering the detail information in the infrared images in certain scenes, we introduce an auxiliary function in the fusion of the detail information to enhance the fusion effect.
In this section, we introduce the experimental environment, training steps, and public datasets used, while also comparing our approach with other mainstream image fusion models through both subjective and objective assessments. Finally, we validate the model's performance and rationality through ablation experiments.
Datasets: We used the public datasets MSRS and TNO as the training datasets. In testing the experimental results, we conducted comparison experiments with mainstream fusion models, including DIDFuse[48], SDNet[49], U2Fusion[50], TarDAL[51], DeFusion[52], DATFuse[53], and CDDFuse[54].
{Implementing rules:} The training and testing were conducted using a GPU configured with NVIDIA RTX 4090, with the framework being PyTorch. During the preprocessing stage of the experiment, the images used for training were randomly cropped into 128 × 128 patches. The total number of training iterations was 120, which as divided into two rounds: the first round consisted of 80 iterations, and the second round consisted of 40 iterations. The batch size was set to 16. The optimization method used was Adam, with an initial learning rate set to 10−4, thus reducing the learning rate by half every 20 epochs. The correlation coefficients in the loss function were set as follows: α1 is 1, α2 is 2.5, α3 is 6, β1 is 1, β2 is 10, β3 is 6.5, and β4 is 2.5.
Metrics: We employed six metrics, namely entropy (EN[55]), standard deviation (SD[56]), spatial frequency (SF), sum of correlation differences (SCD[57]), structural similarity (MI[58]), and visual information fidelity (VIF[59]), to quantitatively analyze the results of the image fusion. EN measures the information richness of the fused image: a higher value indicates richer information and better fusion effects. SD reflects the pixel distribution of the fused image: a larger value signifies a greater contrast and improved fusion results. SF indicates the rate of gray-level change in the fused image: a higher value suggests a clearer image and better fusion. MI represents the information retention between the fused image and the source image: a larger value means that the fused image retains more source information, thus resulting in a better fusion. SCD assesses the quality of the fused image through correlation differences: a lower value signifies better fusion results. A larger VIF value indicates a higher visual fidelity of the fused image, thus leading to better fusion effects.
Data set comparison results: In Figure 7, we present some typical fused contrast images, and in Figure 8, we present some fusion results for the dataset MSRS. Tables 1 and 2 quantitatively compare the different fusion methods. Our approach demonstrates advantages in both the visual and quantitative analyses. To visually demonstrate the comparison between our fusion method and others, we will present the original images alongside locally enlarged sections of the fused images. From a subjective perspective, we aim for the fused images to contain as much detail and contrast information as possible. The more details the fused image includes, the more effectively we have extracted the features of the visible image. If the targets in the fused image are more prominent, then it indicates that we have successfully captured the contrast information from the infrared image. Moreover, if the fused image contains clear targets and distinct environmental information, then it suggests a good fusion of contrast and detail information. Based on the comparison results, while the methods discussed above can achieve good fusion results, they all have certain shortcomings. DID, SDN, and DEF are not effective in extracting details (such as the close-up of the second column), while DAT and U2F are good in extracting details, though the contrast between the background and the target in the picture is insufficient (such as the close-up of the third column). The details obtained by CDD are rich, and the contrast between the scene and the target is large, though it will produce artifacts (such as the enlarged close-up of the third column). On the whole, our TBRAT can retain the details of visible images and some infrared details well, the contrast between the target and the background is obvious, and the fused image has rich scene information.
EN | SD | SF | MI | SCD | VIF | |
DID | 6.97 | 45.12 | 12.59 | 1.70 | 1.71 | 0.68 |
SDN | 6.64 | 32.66 | 12.05 | 1.37 | 1.49 | 0.56 |
U2F | 6.83 | 34.55 | 11.52 | 1.52 | 1.71 | 0.58 |
TarD | 6.84 | 45.63 | 8.68 | 1.86 | 1.52 | 0.53 |
DeFusion | 6.95 | 38.41 | 8.21 | 1.78 | 1.64 | 0.60 |
DATF | 6.58 | 29.65 | 10.09 | 2.36 | 1.45 | 0.70 |
CDDFuse | 7.12 | 46.00 | 13.15 | 2.19 | 1.76 | 0.77 |
TBRAF | 7.15 | 45.76 | 13.68 | 2.23 | 1.83 | 0.78 |
Note: Black fonts and underscores represent the best and second best values, respectively. |
EN | SD | SF | MI | SCD | VIF | |
DID | 4.27 | 31.49 | 10.15 | 1.61 | 1.11 | 0.31 |
SDN | 5.25 | 17.35 | 8.67 | 1, 19 | 0.99 | 0.50 |
U2F | 5.37 | 25.52 | 9.07 | 1.40 | 1.24 | 0.54 |
TarD | 5.28 | 25.22 | 5.98 | 1.49 | 0.71 | 0.42 |
DeF | 6.46 | 37.63 | 6.60 | 2.16 | 1.35 | 0.77 |
DAT | 6.58 | 40.45 | 11.63 | 2.73 | 1.44 | 0.88 |
CDDFuse | 6.70 | 43.38 | 11.56 | 2.47 | 1.62 | 1.05 |
TBRAF | 6.82 | 45.75 | 12.68 | 2.53 | 1.70 | 0.80 |
Note: Black fonts and underscores represent the best and second best values, respectively. |
For the EN and MI metrics, DID, SDN, U2F, and TraD achieve poor results, while DeF, DAT, CDDFuse, and DBRAT achieve better results.DID, SDN, and U2F use the stacking of multiple convolutional layers during the encoding process to improve the feature extraction effect by deepening the depth of the network. Additionally, TraD employs convolutional stacking in the generator. DAT, CDDFuse, and DBRAT use different feature extraction modules in the process of feature extraction to achieve deep feature extraction, (i.e., the previous methods are not sufficient for deep feature extraction, and thus achieve poorer results in EN and MI). For the SD metric, SDN, U2F, and TraD achieve poor results, in the loss function of SDN; moreover, the focus is on the detail information of the fused image, and there is no balance between the contrast information and the detail information during fusion.The feature extraction in the U2F coding network is insufficient, and the same generator network used in TraD does not adequately extract the contrast information from the IR image; therefore, the contrast of the fused image is lower than that of the U2F coding network, and the contrast information from the IR image is not adequately extracted by the generator network. Hence the contrast of the fused image is poor. For the SCD metric, SDN and TraD achieve the worst results: SDN considers more detail information when fusing, and TraD wants the fused image to be closer to either the infrared or visible images when fusing the images, and does not balance the fusion information well. Therefore the results in the SCD metric are poor. For the VIF metric, DID, SDN, U2F, TraD, and DeF achieve poorer results, while DAT, CDDFuse, and DBRAT achieve better results. The previous methods do not establish the relationship between the global and local information, while the latter three methods use Transform to carry out the remote dependency between the global and local information, thus achieving better results in VIF.
In order to verify the effectiveness of the DBRA block and TransNext in the model, we conducted ablation experiments to prove the complementarity of the two modules in the detail feature extraction and the global and local features. Table 3 shows the quantitative results of removing the DBRA and TransNext blocks. Bold numbers in the table indicates the optimal value. From the results in the table, we can see that the complete network structure achieves good results. As can be seen from the comparison diagram in Figure 9, the ability to extract detailed features decreases after removing the DBRA module, and the network can't accurately pay attention to the detailed features. After removing TransNext, the network can't pay attention to global and local features well, and the fused image becomes blurred. The complete network obtains the best effect.
Metrics | Remove TransNext | Remove DBRA | Complete |
EN | 7.05 | 7.09 | 7.15 |
SD | 41.73 | 41.48 | 47.86 |
SF | 12.14 | 12.11 | 13.68 |
MI | 1.63 | 1.61 | 2.07 |
SCD | 1.76 | 1.80 | 1.83 |
VIF | 0.61 | 0.63 | 0.76 |
Note: The best performance is shown in bold. |
The loss function of the network consists of four parts: pixel loss, auxiliary gradient loss, structure loss, and feature decomposition loss. The structure loss, auxiliary gradient loss, and feature decomposition loss are the key loss functions; therefore, we will verify the significance of these three loss functions. We performed ablation experiments on the verification set; from the design motivation, the loss function of each part will achieve different results. Gradient loss makes the fused image obtain the detailed information in the visible and infrared images. At the same time, we introduce auxiliary gradient loss; therefore, we hope that the model can make full use of the visible details and make the infrared details as a supplement. Structural loss allows the model to better measure the visual image effect between the fused image and the original image. The loss function of feature decomposition is used to balance the infrared contrast information and the visible detail information during fusion. Therefore, we will compare the loss function in the first stage and the second stage of training. In the first stage of training, the structural loss function and the characteristic decomposition loss function are removed. In the second stage, the auxiliary loss function is removed. Specifically, this is expressed as follows:
LI1=α1Lirint+α2Lviint+α3Ldecomp+α4Lgrad, | (4.1) |
LII1=β1Lint+β2Lgradmain+β3Lgradaux+β4Ldecomp, | (4.2) |
LI2=Lir+α1Lvis+α3Lgrad, | (4.3) |
LII2=β1Lint+β2Lgradmain+β3Lgradaux, | (4.4) |
LI3=Lir+α1Lvis+α2Ldecomp+α3Lgrad, | (4.5) |
LII3=β1Lint+β2Lgradmain+β2Ldecomp. | (4.6) |
From the result diagram of Figure 10, we can see the influence of different loss functions. The complete loss function can make full use of the characteristic information of infrared and visible images, and the obtained fused image has complete scene information, which not only highlights the target, but also enriches the details. After removing the structural loss, it can be clearly seen that the details of the infrared and visible images are not utilized, the details of the fused image are greatly affected, and the edges become blurred. It can be seen that the fused image will lose some details by removing the auxiliary gradient loss. Without the loss of feature decomposition, the model will produce artifacts and lose some details. Table 4 shows the results of the quantitative analysis after removing each loss function. Objectively speaking, the results of each index are consistent with subjective feelings.
Metrics | L1 | L2 | L3 | Complete |
EN | 7.09 | 7.11 | 7.09 | 7.09 |
SD | 43.65 | 45.04 | 41.48 | 47.86 |
SF | 11.33 | 12.71 | 12.11 | 13.68 |
MI | 1.61 | 1.62 | 1.61 | 2.07 |
SCD | 1.78 | 1.80 | 1.80 | 1.83 |
VIF | 0.63 | 0.62 | 0.63 | 0.76 |
Note: The best performance is shown in bold. |
In this paper, we proposed a fusion network of infrared and visible images based on two-branch attention mechanism. The whole network is an end-to-end model. The fusion of infrared and visible images should not only fully extract important information, but also balance the contrast information and detail information. Therefore, we combined visual Transform with an attention mechanism. In the training process, we adopted a phased training to reduce the training complexity; at the same time, we used auxiliary loss to supplement the detail information in the loss function. The effectiveness of each module was verified by the TNO and MSRS data sets. The ablation experiments showed that the combination of different modules achieved the best fusion effect. For the ablation experiment of the loss function, it can be seen that the feature decomposition loss and the auxiliary gradient loss were helpful to balance and enrich the feature information of the fused image. Compared with the mainstream 8 fusion methods, our method achieved the best performance in a qualitative analysis and subjective vision. At present, our model achieved good results in the fusion of infrared and visible images; however, it was not effective in the fusion of multi-exposure images with different brightnesses in the same scene. In the future, we will focus on the fusion of multi-exposure images. We will consider using the illumination intensity as an indicator, thereby introducing the illumination perception network into the existing model, and calculating the illumination intensity of each image through the illumination perception network to adaptively adjust the feature weight of image fusion with different brightnesses.
The authors declare they have not used Artificial Intelligence (AI) tools in the creation of this article.
This study was financially supported by the following projects: Science and Technology Research Project of Henan Province (Nos. 242102211110 and 242102210217); the Key Research Project of Higher Education Institutions of Henan Province (No. 24A510013); and the Open Project of Key Laboratory of Microsystems Technology (No. 6142804231002).
WangWei Zhang and Hao Sun proposed and conceptualized the innovations of this paper, Bin Zhou prepared the experimental data and verified the experimental results in the paper. Hao Sun was responsible for the overall framework, implementation, etc., and evaluated the performance of the proposed methodology in this paper. All authors reviewed the manuscript. The data in this study are available on request to the second author.
The authors declare there are no conflicts of interest.
[1] |
H. Zhang, H. Xu, X. Tian, J. Jiang, J. Ma, Image fusion meets deep learning: A survey and perspective, Inf. Fusion, 76 (2021), 323–336. https://doi.org/10.1016/j.inffus.2021.06.008 doi: 10.1016/j.inffus.2021.06.008
![]() |
[2] |
J. Ma, L. Tang, M. Xu, H. Zhang, G. Xiao, Stdfusionnet: An infrared and visible image fusion network based on salient target detection, IEEE Trans. Instrum. Meas., 70 (2021), 1–13. https://doi.org/10.1109/TIM.2021.3075747 doi: 10.1109/TIM.2021.3075747
![]() |
[3] | C. Li, C. Zhu, Y. Huang, J. Tang, L. Wang, Cross-modal ranking with soft consistency and noisy labels for robust rgb-t tracking, in Proceedings of the European Conference on Computer Vision (ECCV), (2018), 808–823. |
[4] |
L. Tang, J. Yuan, J. Ma, Image fusion in the loop of high-level vision tasks: A semantic-aware real-time infrared and visible image fusion network, Inf. Fusion, 82 (2022), 28–42. https://doi.org/10.1016/j.inffus.2021.12.004 doi: 10.1016/j.inffus.2021.12.004
![]() |
[5] | Q. Ha, K. Watanabe, T. Karasawa, Y. Ushiku, T. Harada, Mfnet: Towards real-time semantic segmentation for autonomous vehicles with multi-spectral scenes, in 2017 IEEE/RSJ International Conference on Intelligent Robots and Systems (IROS), IEEE, (2017), 5108–5115. https://doi.org/10.1109/IROS.2017.8206396 |
[6] | J. Wang, A. Liu, Z. Yin, S. Liu, S. Tang, X. Liu, Dual attention suppression attack: Generate adversarial camouflage in physical world, in Proceedings of the IEEE/CVF Conference on Computer Vision and Pattern Recognition, (2021), 8565–8574. |
[7] |
J. Ma, Y. Ma, C. Li, Infrared and visible image fusion methods and applications: A survey, Inf. Fusion, 45 (2019), 153–178. https://doi.org/10.1016/j.inffus.2018.02.004 doi: 10.1016/j.inffus.2018.02.004
![]() |
[8] |
A. B. Hamida, A. Benoit, P. Lambert, C. B. Amar, 3-D deep learning approach for remote sensing image classification, IEEE Trans. Geosci. Remote Sens., 56 (2018), 4420–4434. https://doi.org/10.1109/TGRS.2018.2818945 doi: 10.1109/TGRS.2018.2818945
![]() |
[9] |
S. Li, H. Yin, L. Fang, Remote sensing image fusion via sparse representations over learned dictionaries, IEEE Trans. Geosci. Remote Sens., 51 (2013), 4779–4789. https://doi.org/10.1109/TGRS.2012.2230332 doi: 10.1109/TGRS.2012.2230332
![]() |
[10] |
Z. Fu, X. Wang, J. Xu, N. Zhou, Y. Zhao, Infrared and visible images fusion based on RCPA and NSCT, Infrared Phys. Technol., 77 (2016), 114–123. https://doi.org/10.1016/j.infrared.2016.05.012 doi: 10.1016/j.infrared.2016.05.012
![]() |
[11] |
J. Zhao, Q. Zhou, Y. Chen, H. Feng, Z. Xu, Q. Li, Fusion of visible and infrared images using saliency analysis and detail preserving based image decomposition, Infrared Phys. Technol., 56 (2013), 93–99. https://doi.org/10.1016/j.infrared.2012.11.003 doi: 10.1016/j.infrared.2012.11.003
![]() |
[12] |
Y. Liu, J. Jin, Q. Wang, Y. Shen, X. Dong, Region level based multi-focus image fusion using quaternion wavelet and normalized cut, Signal Process., 97 (2014), 9–30. https://doi.org/10.1016/j.sigpro.2013.10.010 doi: 10.1016/j.sigpro.2013.10.010
![]() |
[13] | P. R. Hill, C. N. Canagarajah, D. R. Bull, Image fusion using complex wavelets, in BMVC, (2002), 1–10. |
[14] |
X. Liu, W. Mei, H. Du, Structure tensor and nonsubsampled shearlet transform based algorithm for CT and MRI image fusion, Neurocomputing, 235 (2017), 131–139. https://doi.org/10.1016/j.neucom.2017.01.006 doi: 10.1016/j.neucom.2017.01.006
![]() |
[15] |
Q. Zhang, X. Maldague, An adaptive fusion approach for infrared and visible images based on NSCT and compressed sensing, Infrared Phys. Technol., 74 (2016), 11–20. https://doi.org/10.1016/j.infrared.2015.11.003 doi: 10.1016/j.infrared.2015.11.003
![]() |
[16] |
M. Wu, Y. Ma, F. Fan, X. Mei, J. Huang, Infrared and visible image fusion via joint convolutional sparse representation, J. Opt. Soc. Am. A, 37 (2020), 1105–1115. https://doi.org/10.1364/JOSAA.388447 doi: 10.1364/JOSAA.388447
![]() |
[17] |
Y. Liu, X. Chen, R. K. Ward, Z. J. Wang, Image fusion with convolutional sparse representation, IEEE Signal Process Lett., 23 (2016), 1882–1886. https://doi.org/10.1109/LSP.2016.2618776 doi: 10.1109/LSP.2016.2618776
![]() |
[18] |
H. Li, X. J. Wu, J. Kittler, MDLatLRR: A novel decomposition method for infrared and visible image fusion, IEEE Trans. Image Process., 29 (2020), 4733–4746. https://doi.org/10.1109/TIP.2020.2975984 doi: 10.1109/TIP.2020.2975984
![]() |
[19] | D. P. Bavirisetti, G. Xiao, G. Liu, Multi-sensor image fusion based on fourth order partial differential equations, in 2017 20th International Conference on Information Fusion (Fusion), IEEE, (2017), 1–9. https://doi.org/10.23919/ICIF.2017.8009719 |
[20] |
N. Cvejic, D. Bull, N. Canagarajah, Region-based multimodal image fusion using ica bases, IEEE Sens. J., 7 (2007), 743–751. https://doi.org/10.1109/JSEN.2007.894926 doi: 10.1109/JSEN.2007.894926
![]() |
[21] | J. Mou, W. Gao, Z. Song, Image fusion based on non-negative matrix factorization and infrared feature extraction, in 2013 6th International Congress on Image and Signal Processing (CISP), IEEE, (2013), 1046–1050. https://doi.org/10.1109/CISP.2013.6745210 |
[22] |
C. H. Liu, Y. Qi, W. R. Ding, Infrared and visible image fusion method based on saliency detection in sparse domain, Infrared Phys. Technol., 83 (2017), 94–102. https://doi.org/10.1016/j.infrared.2017.04.018 doi: 10.1016/j.infrared.2017.04.018
![]() |
[23] |
Y. LeCun, L. Bottou, Y. Bengio, P. Haffner, Gradient-based learning applied to document recognition, Proc. IEEE, 86 (1998), 2278–2324. https://doi.org/10.1109/5.726791 doi: 10.1109/5.726791
![]() |
[24] | D. P. Kingma, Auto-encoding variational bayes, preprint, arXiv: 1312.6114. |
[25] | I. Goodfellow, J. Pouget-Abadie, M. Mirza, B. Xu, D. Warde-Farley, S. Ozair, et al., Generative adversarial nets, in Advances in Neural Information Processing Systems, Curran Associates, Inc., 27 (2014), 1–9. |
[26] | Y. Liu, X. Chen, H. Peng, Z. Wang, Multi-focus image fusion with a deep convolutional neural network, Inf. Fusion, 36 (2017) 191–207. https://doi.org/10.1016/j.inffus.2016.12.001 |
[27] |
H. Li, X. J. Wu, Densefuse: A fusion approach to infrared and visible images, IEEE Trans. Image Process., 28 (2018), 2614–2623. https://doi.org/10.1109/TIP.2018.2887342 doi: 10.1109/TIP.2018.2887342
![]() |
[28] |
H. Li, X. J. Wu, T. Durrani, Nestfuse: An infrared and visible image fusion architecture based on nest connection and spatial/channel attention models, IEEE Trans. Instrum. Meas., 69 (2020), 9645–9656. https://doi.org/10.1109/TIM.2020.3005230 doi: 10.1109/TIM.2020.3005230
![]() |
[29] |
L. Jian, X. Yang, Z. Liu, G. Jeon, M. Gao, D. Chisholm, Sedrfuse: A symmetric encoder–decoder with residual block network for infrared and visible image fusion, IEEE Trans. Instrum. Meas., 70 (2020), 1–15. https://doi.org/10.1109/TIM.2020.3022438 doi: 10.1109/TIM.2020.3022438
![]() |
[30] |
S. Park, A. G. Vien, C. Lee, Cross-modal transformers for infrared and visible image fusion, IEEE Trans. Circuits Syst. Video Technol., 34 (2023), 770–785. https://doi.org/10.1109/TCSVT.2023.3289170 doi: 10.1109/TCSVT.2023.3289170
![]() |
[31] |
Z. Zhu, Z. Wang, G. Qi, N. Mazur, P. Yang, Y. Liu, Brain tumor segmentation in MRI with multi-modality spatial information enhancement and boundary shape correction, Pattern Recognit., 153 (2024), 110553. https://doi.org/10.1016/j.patcog.2024.110553 doi: 10.1016/j.patcog.2024.110553
![]() |
[32] |
J. Ma, W. Yu, P. Liang, C. Li, J. Jiang, Fusiongan: A generative adversarial network for infrared and visible image fusion, Inf. Fusion, 48 (2019), 11–26. https://doi.org/10.1016/j.inffus.2018.09.004 doi: 10.1016/j.inffus.2018.09.004
![]() |
[33] |
J. Ma, H. Xu, J. Jiang, X. Mei, X. P. Zhang, Ddcgan: A dual-discriminator conditional generative adversarial network for multi-resolution image fusion, IEEE Trans. Image Process., 29 (2020), 4980–4995. https://doi.org/10.1109/TIP.2020.2977573 doi: 10.1109/TIP.2020.2977573
![]() |
[34] |
J. Ma, H. Zhang, Z. Shao, P. Liang, Han Xu, Ganmcc: A generative adversarial network with multiclassification constraints for infrared and visible image fusion, IEEE Trans. Instrum. Meas., 70 (2020), 1–14. https://doi.org/10.1109/TIM.2020.3038013 doi: 10.1109/TIM.2020.3038013
![]() |
[35] |
J. Li, H. Huo, C. Li, R. Wang, Q. Feng, Attentionfgan: Infrared and visible image fusion using attention-based generative adversarial networks, IEEE Trans. Multimedia, 23 (2020), 1383–1396. https://doi.org/10.1109/TMM.2020.2997127 doi: 10.1109/TMM.2020.2997127
![]() |
[36] | D. Rao, T. Xu, X. J. Wu, Tgfuse: An infrared and visible image fusion approach based on transformer and generative adversarial network, IEEE Trans. Image Process., 2023, (2023). https://doi.org/10.1109/TIP.2023.3273451 |
[37] |
J. Tang, A contrast based image fusion technique in the DCT domain, Digital Signal Process., 14 (2004), 218–226. https://doi.org/10.1016/j.dsp.2003.06.001 doi: 10.1016/j.dsp.2003.06.001
![]() |
[38] | J. Tang, Q. Sun, Z.Wang, Y. Cao, Perfect-reconstruction four-tap size-limited filter banks for image fusion application, in 2007 International Conference on Mechatronics and Automation, IEEE, (2007), 255–260. https://doi.org/10.1109/ICMA.2007.4303550 |
[39] | A. Dosovitskiy, An image is worth 16 × 16 words: Transformers for image recognition at scale, preprint, arXiv: 2010.11929. |
[40] | Z. Liu, Y. Lin, Y. Cao, H. Hu, Y. Wei, Z. Zhang, et al., Swin transformer: Hierarchical vision transformer using shifted windows, in Proceedings of the IEEE/CVF International Conference on Computer Vision, (2021), 10012–10022. |
[41] | S. W. Zamir, A. Arora, S. Khan, M. Hayat, F. S. Khan, M. H. Yang, Restormer: Efficient transformer for high-resolution image restoration, in Proceedings of the IEEE/CVF Conference on Computer Vision and Pattern Recognition (CVPR), (2022), 5728–5739. |
[42] | W. Wang, E. Xie, X. Li, D. P. Fan, K. Song, D. Liang, et al., Pyramid vision transformer: A versatile backbone for dense prediction without convolutions, in Proceedings of the IEEE/CVF International Conference on Computer Vision, (2021), 568–578. |
[43] | D. Shi, Transnext: Robust foveal visual perception for vision transformers, in Proceedings of the IEEE/CVF Conference on Computer Vision and Pattern Recognition, (2024), 17773–17783. |
[44] |
Y. Liu, Y. Ma, Z. Zhu, J. Cheng, X. Chen, Transsea: Hybrid cnn-transformer with semantic awareness for 3d brain tumor segmentation, IEEE Trans. Instrum. Meas., 73 (2024), 16–31. https://doi.org/10.1109/TIM.2024.3413130 doi: 10.1109/TIM.2024.3413130
![]() |
[45] |
Z. Zhu, X. He, G. Qi, Y. Li, B. Cong, Y. Liu, Brain tumor segmentation based on the fusion of deep semantics and edge information in multimodal MRI, Inf. Fusion, 91 (2023), 376–387. https://doi.org/10.1016/j.inffus.2022.10.022 doi: 10.1016/j.inffus.2022.10.022
![]() |
[46] | J. Hu, L. Shen, G. Sun, Squeeze-and-excitation networks, in Proceedings of the IEEE Conference on Computer Vision and Pattern Recognition, (2018), 7132–7141. |
[47] | X. Zhu, D. Cheng, Z. Zhang, S. Lin, J. Dai, An empirical study of spatial attention mechanisms in deep networks, in Proceedings of the IEEE/CVF International Conference on Computer Vision, (2019), 6688–6697. |
[48] | Z. Zhao, S. Xu, C. Zhang, J. Liu, P. Li, J. Zhang, Didfuse: Deep image decomposition for infrared and visible image fusion, preprint, arXiv: 2003.09210. |
[49] |
H. Zhang, J. Ma, Sdnet: A versatile squeeze-and-decomposition network for real-time image fusion, Int. J. Comput. Vis., 129 (2021), 2761–2785. https://doi.org/10.1007/s11263-021-01501-8 doi: 10.1007/s11263-021-01501-8
![]() |
[50] |
H. Xu, J. Ma, J. Jiang, X. Guo, H. Ling, U2fusion: A unified unsupervised image fusion network, IEEE Trans. Pattern Anal. Mach. Intell., 44 (2020), 502–518. https://doi.org/10.1109/TPAMI.2020.3012548 doi: 10.1109/TPAMI.2020.3012548
![]() |
[51] | J. Liu, X. Fan, Z. Huang, G. Wu, R. Liu, W. Zhong, et al., Target-aware dual adversarial learning and a multi-scenario multi-modality benchmark to fuse infrared and visible for object detection, in Proceedings of the IEEE/CVF Conference on Computer Vision and Pattern Recognition (CVPR), (2022), 5802–5811. |
[52] | P. Liang, J. Jiang, X. Liu, J. Ma, Fusion from decomposition: A self-supervised decomposition approach for image fusion, in European Conference on Computer Vision, Springer, (2022), 719–735. https://doi.org/10.1007/978-3-031-19797-0_41 |
[53] |
W. Tang, F. He, Y. Liu, Y. Duan, T. Si, Datfuse: Infrared and visible image fusion via dual attention transformer, IEEE Trans. Circuits Syst. Video Technol., 33 (2023), 3159–3172. https://doi.org/10.1109/TCSVT.2023.3234340 doi: 10.1109/TCSVT.2023.3234340
![]() |
[54] | Z. Zhao, H. Bai, J. Zhang, Y. Zhang, S. Xu, Z. Lin, et al., Cddfuse: Correlation-driven dual-branch feature decomposition for multi-modality image fusion, in Proceedings of the IEEE/CVF Conference on Computer Vision and Pattern Recognition, (2023), 5906–5916. |
[55] |
J. W. Roberts, J. A. Van Aardt, F. B. Ahmed, Assessment of image fusion procedures using entropy, image quality, and multispectral classification, J. Appl. Remote Sens., 2 (2008), 023522. https://doi.org/10.1117/1.2945910 doi: 10.1117/1.2945910
![]() |
[56] | Y. J. Rao, In-fibre bragg grating sensors, Meas. Sci. Technol., 8 (1997), 355. |
[57] |
V. Aslantas, E. Bendes, A new image quality metric for image fusion: The sum of the correlations of differences, AEU Int. J. Electron. Commun., 69 (2015), 1890–1896. https://doi.org/10.1016/j.aeue.2015.09.004 doi: 10.1016/j.aeue.2015.09.004
![]() |
[58] |
Z. Wang, A. C. Bovik, A universal image quality index, IEEE Signal Process Lett., 9 (2002), 81–84. https://doi.org/10.1109/97.995823 doi: 10.1109/97.995823
![]() |
[59] |
Y. Han, Y. Cai, Y. Cao, X. Xu, A new image fusion performance metric based on visual information fidelity, Inf. Fusion, 14 (2013), 127–135. https://doi.org/10.1016/j.inffus.2011.08.002 doi: 10.1016/j.inffus.2011.08.002
![]() |
EN | SD | SF | MI | SCD | VIF | |
DID | 6.97 | 45.12 | 12.59 | 1.70 | 1.71 | 0.68 |
SDN | 6.64 | 32.66 | 12.05 | 1.37 | 1.49 | 0.56 |
U2F | 6.83 | 34.55 | 11.52 | 1.52 | 1.71 | 0.58 |
TarD | 6.84 | 45.63 | 8.68 | 1.86 | 1.52 | 0.53 |
DeFusion | 6.95 | 38.41 | 8.21 | 1.78 | 1.64 | 0.60 |
DATF | 6.58 | 29.65 | 10.09 | 2.36 | 1.45 | 0.70 |
CDDFuse | 7.12 | 46.00 | 13.15 | 2.19 | 1.76 | 0.77 |
TBRAF | 7.15 | 45.76 | 13.68 | 2.23 | 1.83 | 0.78 |
Note: Black fonts and underscores represent the best and second best values, respectively. |
EN | SD | SF | MI | SCD | VIF | |
DID | 4.27 | 31.49 | 10.15 | 1.61 | 1.11 | 0.31 |
SDN | 5.25 | 17.35 | 8.67 | 1, 19 | 0.99 | 0.50 |
U2F | 5.37 | 25.52 | 9.07 | 1.40 | 1.24 | 0.54 |
TarD | 5.28 | 25.22 | 5.98 | 1.49 | 0.71 | 0.42 |
DeF | 6.46 | 37.63 | 6.60 | 2.16 | 1.35 | 0.77 |
DAT | 6.58 | 40.45 | 11.63 | 2.73 | 1.44 | 0.88 |
CDDFuse | 6.70 | 43.38 | 11.56 | 2.47 | 1.62 | 1.05 |
TBRAF | 6.82 | 45.75 | 12.68 | 2.53 | 1.70 | 0.80 |
Note: Black fonts and underscores represent the best and second best values, respectively. |
Metrics | Remove TransNext | Remove DBRA | Complete |
EN | 7.05 | 7.09 | 7.15 |
SD | 41.73 | 41.48 | 47.86 |
SF | 12.14 | 12.11 | 13.68 |
MI | 1.63 | 1.61 | 2.07 |
SCD | 1.76 | 1.80 | 1.83 |
VIF | 0.61 | 0.63 | 0.76 |
Note: The best performance is shown in bold. |
Metrics | L1 | L2 | L3 | Complete |
EN | 7.09 | 7.11 | 7.09 | 7.09 |
SD | 43.65 | 45.04 | 41.48 | 47.86 |
SF | 11.33 | 12.71 | 12.11 | 13.68 |
MI | 1.61 | 1.62 | 1.61 | 2.07 |
SCD | 1.78 | 1.80 | 1.80 | 1.83 |
VIF | 0.63 | 0.62 | 0.63 | 0.76 |
Note: The best performance is shown in bold. |
EN | SD | SF | MI | SCD | VIF | |
DID | 6.97 | 45.12 | 12.59 | 1.70 | 1.71 | 0.68 |
SDN | 6.64 | 32.66 | 12.05 | 1.37 | 1.49 | 0.56 |
U2F | 6.83 | 34.55 | 11.52 | 1.52 | 1.71 | 0.58 |
TarD | 6.84 | 45.63 | 8.68 | 1.86 | 1.52 | 0.53 |
DeFusion | 6.95 | 38.41 | 8.21 | 1.78 | 1.64 | 0.60 |
DATF | 6.58 | 29.65 | 10.09 | 2.36 | 1.45 | 0.70 |
CDDFuse | 7.12 | 46.00 | 13.15 | 2.19 | 1.76 | 0.77 |
TBRAF | 7.15 | 45.76 | 13.68 | 2.23 | 1.83 | 0.78 |
Note: Black fonts and underscores represent the best and second best values, respectively. |
EN | SD | SF | MI | SCD | VIF | |
DID | 4.27 | 31.49 | 10.15 | 1.61 | 1.11 | 0.31 |
SDN | 5.25 | 17.35 | 8.67 | 1, 19 | 0.99 | 0.50 |
U2F | 5.37 | 25.52 | 9.07 | 1.40 | 1.24 | 0.54 |
TarD | 5.28 | 25.22 | 5.98 | 1.49 | 0.71 | 0.42 |
DeF | 6.46 | 37.63 | 6.60 | 2.16 | 1.35 | 0.77 |
DAT | 6.58 | 40.45 | 11.63 | 2.73 | 1.44 | 0.88 |
CDDFuse | 6.70 | 43.38 | 11.56 | 2.47 | 1.62 | 1.05 |
TBRAF | 6.82 | 45.75 | 12.68 | 2.53 | 1.70 | 0.80 |
Note: Black fonts and underscores represent the best and second best values, respectively. |
Metrics | Remove TransNext | Remove DBRA | Complete |
EN | 7.05 | 7.09 | 7.15 |
SD | 41.73 | 41.48 | 47.86 |
SF | 12.14 | 12.11 | 13.68 |
MI | 1.63 | 1.61 | 2.07 |
SCD | 1.76 | 1.80 | 1.83 |
VIF | 0.61 | 0.63 | 0.76 |
Note: The best performance is shown in bold. |
Metrics | L1 | L2 | L3 | Complete |
EN | 7.09 | 7.11 | 7.09 | 7.09 |
SD | 43.65 | 45.04 | 41.48 | 47.86 |
SF | 11.33 | 12.71 | 12.11 | 13.68 |
MI | 1.61 | 1.62 | 1.61 | 2.07 |
SCD | 1.78 | 1.80 | 1.80 | 1.83 |
VIF | 0.63 | 0.62 | 0.63 | 0.76 |
Note: The best performance is shown in bold. |