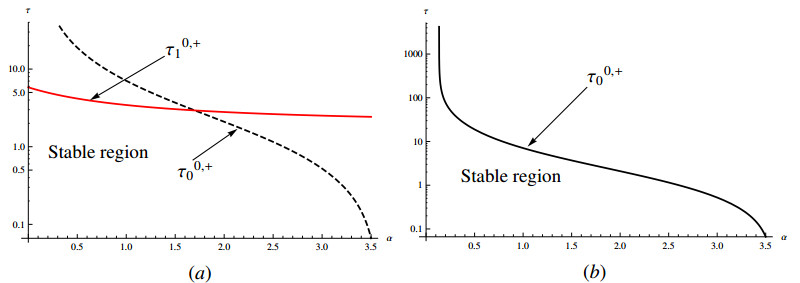
In this paper, a complete optimization design verification process is proposed and a novel structure of connecting brackets is presented, solving the fatigue failure of chassis connecting brackets operating on harsh roads. First, an endurance road test and fatigue life analysis were applied to the truck equipped with the original brackets, verifying the fatigue damage of the structure. Based on the solid isotropic material with penalization method, a novel lightweight connecting bracket layout was obtained by using the method of moving asymptotes (MMA) for topology optimization under multiple working conditions with multiple performance constraints. Moreover, the derivatives of objective and constraint functions concerning design variables were applied for the MMA. Considering manufacturability and functionality, the improved model based on the topology optimization results was further optimized by size optimization. Finally, fatigue life analysis and an endurance road test were conducted using the optimal design. Compared with the original structure, the novel brackets showed better stiffness, strength and fatigue performance while reducing the total mass by 15.2%. The whole optimization and validation process can provide practical ideas and value for developing multi-performance suspensions in the pre-product development stage.
Citation: Furong Xie, Yunkai Gao, Ting Pan, De Gao, Lei Wang, Yanan Xu, Chi Wu. Novel lightweight connecting bracket design with multiple performance constraints based on optimization and verification process[J]. Electronic Research Archive, 2023, 31(4): 2019-2047. doi: 10.3934/era.2023104
[1] | Yichao Shao, Hengguo Yu, Chenglei Jin, Jingzhe Fang, Min Zhao . Dynamics analysis of a predator-prey model with Allee effect and harvesting effort. Electronic Research Archive, 2024, 32(10): 5682-5716. doi: 10.3934/era.2024263 |
[2] | Xiaowen Zhang, Wufei Huang, Jiaxin Ma, Ruizhi Yang . Hopf bifurcation analysis in a delayed diffusive predator-prey system with nonlocal competition and schooling behavior. Electronic Research Archive, 2022, 30(7): 2510-2523. doi: 10.3934/era.2022128 |
[3] | Fengrong Zhang, Ruining Chen . Spatiotemporal patterns of a delayed diffusive prey-predator model with prey-taxis. Electronic Research Archive, 2024, 32(7): 4723-4740. doi: 10.3934/era.2024215 |
[4] | Yuan Tian, Yang Liu, Kaibiao Sun . Complex dynamics of a predator-prey fishery model: The impact of the Allee effect and bilateral intervention. Electronic Research Archive, 2024, 32(11): 6379-6404. doi: 10.3934/era.2024297 |
[5] | Mengting Sui, Yanfei Du . Bifurcations, stability switches and chaos in a diffusive predator-prey model with fear response delay. Electronic Research Archive, 2023, 31(9): 5124-5150. doi: 10.3934/era.2023262 |
[6] | Ruizhi Yang, Dan Jin . Dynamics in a predator-prey model with memory effect in predator and fear effect in prey. Electronic Research Archive, 2022, 30(4): 1322-1339. doi: 10.3934/era.2022069 |
[7] | Miao Peng, Rui Lin, Zhengdi Zhang, Lei Huang . The dynamics of a delayed predator-prey model with square root functional response and stage structure. Electronic Research Archive, 2024, 32(5): 3275-3298. doi: 10.3934/era.2024150 |
[8] | San-Xing Wu, Xin-You Meng . Hopf bifurcation analysis of a multiple delays stage-structure predator-prey model with refuge and cooperation. Electronic Research Archive, 2025, 33(2): 995-1036. doi: 10.3934/era.2025045 |
[9] | Jiani Jin, Haokun Qi, Bing Liu . Hopf bifurcation induced by fear: A Leslie-Gower reaction-diffusion predator-prey model. Electronic Research Archive, 2024, 32(12): 6503-6534. doi: 10.3934/era.2024304 |
[10] | Zhuo Ba, Xianyi Li . Period-doubling bifurcation and Neimark-Sacker bifurcation of a discrete predator-prey model with Allee effect and cannibalism. Electronic Research Archive, 2023, 31(3): 1405-1438. doi: 10.3934/era.2023072 |
In this paper, a complete optimization design verification process is proposed and a novel structure of connecting brackets is presented, solving the fatigue failure of chassis connecting brackets operating on harsh roads. First, an endurance road test and fatigue life analysis were applied to the truck equipped with the original brackets, verifying the fatigue damage of the structure. Based on the solid isotropic material with penalization method, a novel lightweight connecting bracket layout was obtained by using the method of moving asymptotes (MMA) for topology optimization under multiple working conditions with multiple performance constraints. Moreover, the derivatives of objective and constraint functions concerning design variables were applied for the MMA. Considering manufacturability and functionality, the improved model based on the topology optimization results was further optimized by size optimization. Finally, fatigue life analysis and an endurance road test were conducted using the optimal design. Compared with the original structure, the novel brackets showed better stiffness, strength and fatigue performance while reducing the total mass by 15.2%. The whole optimization and validation process can provide practical ideas and value for developing multi-performance suspensions in the pre-product development stage.
The predator-prey model has always been an important research content of biomathematics, because a predator-prey relationship is widespread in nature [1,2,3,4]. Among the population growth laws, the Allee effect is an important biological phenomenon. W. Allee proposed the famous Allee effect to describe the phenomenon that low-density populations are prone to extinction [5]. Since then, the predator-prey model with the Allee effect has received extensive attention from scholars. Cooperative hunting is also widespread in nature, such as gray wolves, chimpanzees, banded mongooses, lions, etc. [6,7]. They all hunt collectively.
In [8], R. Yadav et al. studied a predator-prey model with the Allee effect and hunting cooperation, that is
{dudt=ru(1−uK)(u−u0)−(λ+av)u2v1+A(λ+av)u2,dvdt=e(λ+av)u2v1+A(λ+av)u2−mv. | (1.1) |
u(t) and v(t) are densities of prey and predator, respectively. r, K and u0 represent intrinsic growth rate, carrying capacity and Allee effect parameter of prey, respectively. The term (λ+av)u21+A(λ+av)u2 is the functional response function including the hunting cooperation in predator, with capturing rate λ, handling time A and hunting cooperation parameter a. e and m are conversion efficiency and death rate of a predator. Make the changes u=K˜u, v=rλ˜v, t=1rK˜t, α=arλ2, β=N0K, σ=mK2eλ, h=AK2λ, η=Keλr, and drop "~", the model (1.1) is changed into
{dudt=u(1−u)(u−β)−(1+αv)u2v1+h(1+αv)u2,dvdt=η((1+αv)u2v1+h(1+αv)u2−σv). | (1.2) |
The authors mainly studied the Turing pattern of the model (1.2) by applying the amplitude equation through weakly nonlinear analysis [8]. The model (1.2) shows the spiral and target patterns.
In the inter-population interaction, time delay often occurs, such as gestation delay, maturation time, capturing time, and so on. Some scholars have discussed the dynamic properties of predator-prey models with time delay, mainly focusing on Hopf bifurcation [9,10,11]. They obtained that time delay may affect the stability of equilibria, and induce Hopf bifurcation [12,13,14]. In particular, in the reaction-diffusion predator-prey model with time delay, there may be spatially homogeneous and inhomogeneous periodic solutions, but the stable periodic solutions are often spatially homogeneous in the numerical simulation. This is not consistent with the actual situation, because in the real world, the spatial distribution of the population is difficult to reach a completely uniform state, that is, a stable spatial homogeneous periodic solution. This is one of our motivations, that is, will there be stably spatially inhomogeneous periodic solutions for the delayed reaction-diffusion predator-prey model.
In addition, due to the limited resources and the competition within the population, many scholars have chosen the Logistic growth law to describe the growth law of the prey population. Logistic growth law is mainly applicable to the predator-prey model in the form of an ordinary differential equation, and it is assumed that the spatial distribution of resources is uniform. However, in fact, the spatial distribution of resources is often nonuniform, and the population competition among prey is often spatially nonlocal competition [15,16]. To describe this phenomenon, the authors [17,18] modified the uK as 1K∫ΩG(x,y)u(y,t)dy with some kernel function G(x,y). In [19], D. Geng and H. Wang studied the normal form of double-Hopf bifurcation for a predator-prey model with nonlocal competition with nonlocal effect. In [21], Liu et al. studied a delayed diffusive predator-prey model with group defense effect and nonlocal competition and observed stably spatially inhomogeneous oscillations. In [20] the authors analyzed a diffusive predator-prey model with nonlocal competition from the perspective of bifurcation. In this paper, we want to study what new dynamic phenomena will appear when adding spatial nonlocal competition in the model (1.2), and what impact it will have on the distribution of prey and predator densities. This is another motivation for our work.
Motivated by above, we studied the following model
{∂u(x,t)∂t=d1Δu+u(1−∫ΩG(x,y)u(y,t)dy)(u−β)−(1+αv)u2v1+h(1+αv)u2,∂v(x,t)∂t=d2Δv+η((1+αv(t−τ))u2(t−τ)v(t−τ)1+h(1+αv(t−τ))u2(t−τ)−σv),x∈Ω,t>0∂u(x,t)∂ˉν=∂v(x,t)∂ˉν=0,x∈∂Ω,t>0u(x,θ)=u0(x,θ)≥0,v(x,θ)=v0(x,θ)≥0,x∈ˉΩ,θ∈[−τ,0]. | (1.3) |
where d1 and d2 are diffusive coefficients. τ is the gestation delay in predator. ∫ΩG(x,y)u(y,t)dy represents the nonlocal competition effect. The kernel function is
G(x,y)=1|Ω|=1lπ,x,y∈Ω, |
which is widely used [20,21]. This is based on the assumption that the competition strength among prey individuals in the habitat is the same. The region Ω=(0,lπ) with l>0 just for the convenience of calculation.
The article is structured as follows. In Section 2, the stability and existence of Hopf bifurcation for the models with and without nonlocal competition are studied. In Section 3, the parameters that determine the properties of Hopf bifurcation are given. In Section 4, some numerical simulations are shown. In Section 5, a short conclusion is given.
The authors obtain that the system (1.3) has at least one coexisting equilibrium (u∗,v∗) when β2β2h+1<σ<1h+1 and β<1 in [8], where u∗ is the root of the following equation falling in the interval (β,1),
u2(α(1−u)u(u−β)σ+1)−σ1−hσ=0, |
and v∗=u∗(1−u∗)(u∗−β)σ. In the following, we just denote the coexisting equilibrium as (u∗,v∗).
Linearize system (1.3) at E∗(u∗,v∗)
∂u∂t(u(x,t)u(x,t))=D(Δu(t)Δv(t))+L1(u(x,t)v(x,t))+L2(u(x,t−τ)v(x,t−τ))+L3(ˆu(x,t)ˆv(x,t)), | (2.1) |
where
D=(d100d2),L1=(a1a20−ησ),L2=(00b1b2),L3=(ˆa000), |
a1=u∗(v∗(αv∗+1)(hu2∗(αv∗+1)−1)(hu2∗(αv∗+1)+1)2+1−u∗),a2=−u2∗(h(αu∗v∗+u∗)2+2αv∗+1)(hu2∗(αv∗+1)+1)2<0,b1=2ηu∗v∗(αv∗+1)(hu2∗(αv∗+1)+1)2>0,b2=ηu2∗(h(αu∗v∗+u∗)2+2αv∗+1)(hu2∗(αv∗+1)+1)2>0,ˆa=−u∗(u∗−β)<0, | (2.2) |
and ˆu=1lπ∫lπ0u(y,t)dy. The characteristic equations are
λ2+Enλ+Mn+(Gn−b2λ)e−λτ=0,n∈N0, | (2.3) |
where
E0=ησ−(ˆa+a1),M0=−ησ(ˆa+a1),G0=b2(ˆa+a1)−a2b1,En=+(d1+d2)n2l2+ησ−a1,Mn=d1d2n4l4+(d1ησ−a1d2)n2l2−a1ησ,Gn=−b2d1n2l2+a1b2−a2b1,n∈N. | (2.4) |
N and N0 represent the positive integer set and the non-negative integer set.
When τ=0, the characteristic equations are as follow
λ2+(En−b2)λ+Mn+Gn=0,n∈N0. | (2.5) |
Make the following hypothesis
(H1)En−b2>0,Mn+Gn>0,forn∈N0. |
Under the hypothesis (H1), E∗(u∗,v∗) is locally asymptotically stable when τ=0.
Next, we will discuss the case of τ>0.
Lemma 2.1. Assume (H1) holds, the following results hold.
● Equation (2.3) has a pair of purely imaginary roots ±iω+n at τj,+n for j∈N0 and n∈W1.
● Equation (2.3) has two pairs of purely imaginary roots ±iω±n at τj,±n for j∈N0 and n∈W2.
● Equation (2.3) has no purely imaginary root for n∈W3.
Where ±iω±n, τj,±n, W1, W2 and W3 are defined in (2.8) and (2.9).
Proof. Let iω (ω>0) be a solution of Eq (2.3), then
−ω2+iωEn+Mn+(Gn−b2iω)(cosωτ−isinωτ)=0. |
Obviously. cosωτ=ω2(b2En+Gn)−MnGnG2n+b22ω2, sinωτ=ω(EnGn+Mnb2−b2ω2)G2n+b22ω2. It leads to
ω4+ω2(E2n−2Mn−b22)+M2n−G2n=0. | (2.6) |
Let z=ω2, then (2.6) becomes
z2+z(E2n−2Mn−b22)+M2n−G2n=0, | (2.7) |
and the roots of (2.7) are z±=12[−Hn±√H2n−4JnKn], where Hn=E2n−2Mn−b22, Jn=Mn+Gn, and Kn=Mn−Gn. If (H1) holds, Jn>0(n∈N0). By direct calculation, we have
H0=(ˆa+a1)2+η2σ2−b22,Hk=(a1−d1k2l2)2+(d2k2l2+ησ)2−b22,fork∈NK0=a2b1−(ˆa+a1)(b2+ησ),Kk=d1d2k4l4+[d1(b2+ησ)−a1d2]k2l2+a2b1−a1b2+ησ,fork∈N. |
Define
S1={n|Kn<0,n∈N0},S2={n|Kn>0,Hn<0,H2n−4JnKn>0,n∈N0},S3={n|Kn>0,H2n−4JnKn<0,n∈N0}, | (2.8) |
and
ω±n=√z±n,τj,±n={1ω±narccos(V(n,±)cos)+2jπ,V(n,±)sin≥0,1ω±n[2π−arccos(V(n,±)cos)]+2jπ,V(n,±)sin<0.V(n,±)cos=(ω±n)2(b2En+Gn)−MnGnG2n+b22(ω±n)2,V(n,±)sin=ω±n(EnGn+Mnb2−b2(ω±n)2)G2n+b22(ω±n)2. | (2.9) |
It is easy to verify the conclusion in the Lemma 2.1.
Next, we verify the transversal condition for the existence of Hopf bifurcation.
Lemma 2.2. Assume (H1) holds. Then Re(dλdτ)|τ=τj,+n>0, Re(dλdτ)|τ=τj,−n<0 for n∈S1∪S2 and j∈N0.
Proof. By (2.3), we have
(dλdτ)−1=2λ+En−b2e−λτ(Gn−b2λ)λe−λτ−τλ. |
Then
[Re(dλdτ)−1]τ=τj,±n=Re[2λ+En−b2e−λτ(Gn−b2λ)λe−λτ−τλ]τ=τj,±n=[1G2n+b22ω2(2ω2+E2n−2Mn−b22)]τ=τj,±n=±[1G2n+b22ω2√(E2n−2Mn−b22)2−4(M2n−G2n)]τ=τj,±n. |
Therefore, Re(dλdτ)|τ=τj,+n>0, Re(dλdτ)|τ=τj,−n<0.
Denote τ∗=min{τ0n|n∈S1∪S2}. We have the following theorem.
Theorem 2.1. For system (1.3), assume (H1) holds.
● E∗(u∗,v∗) is locally asymptotically stable for τ>0 when S1∪S2=∅.
● E∗(u∗,v∗) is locally asymptotically stable for τ∈[0,τ∗) when S1∪S2≠∅.
● E∗(u∗,v∗) is unstable for τ∈(τ∗,τ∗+ε) for some ε>0 when S1∪S2≠∅.
● Hopf bifurcation occurs at (u∗,v∗) when τ=τj,+n (τ=τj,−n), j∈N0, n∈S1∪S2. In addition, the spatially homogeneous (inhomogeneous) periodic solutions occur when τ=τj,±0 (τ=τj,±n, n>0).
The model (1.3) without nonlocal competition is as follow
{∂u(x,t)∂t=d1Δu+u(1−u)(u−β)−(1+αv)u2v1+h(1+αv)u2,∂v(x,t)∂t=d2Δv+η((1+αv(t−τ))u2(t−τ)v(t−τ)1+h(1+αv(t−τ))u2(t−τ)−σv). | (2.10) |
Linearize system (2.10) at E∗(u∗,v∗)
∂u∂t(u(x,t)u(x,t))=D(Δu(t)Δv(t))+(L1+L3)(u(x,t)v(x,t))+L2(u(x,t−τ)v(x,t−τ)). | (2.11) |
The characteristic equations are
λ2+˜Anλ+˜Mn+(˜Gn−b2λ)e−λτ=0,n∈N0, | (2.12) |
where
˜En=+(d1+d2)n2l2+ησ−(a1+ˆa),˜Mn=d1d2n4l4+(d1ησ−(a1+ˆa)d2)n2l2−(a1+ˆa)ησ,˜Gn=−b2d1n2l2+(a1+ˆa)b2−a2b1,n∈N0. | (2.13) |
When τ=0, the characteristic equations are as follow
λ2+(˜En−b2)λ+˜Mn+˜Gn=0,n∈N0. | (2.14) |
Make the following hypothesis
(H2)˜En−b2>0,˜Mn+˜Gn>0,forn∈N0. |
Under the hypothesis (H2), E∗(u∗,v∗) is locally asymptotically stable when τ=0.
Remark 2.1. It is easy to obtain that ˜A0−b2=E0−b2, ˜B0+˜C0=M0+G0, ˜En−b2−(En−b2)=−ˆa>0 and ˜Mn+˜Gn−(Mn+Gn)=−ˆa(d2n2l2+ησ−b2) for n∈N. Hence, under condition d2l2+ησ−b2≥0, hypothesis (H1) can deduce (H2).
Through a similar process, we have the following results. Define
˜Hk=(a1+ˆa−d1k2l2)2+(d2k2l2+ησ)2−b22,˜Jk=d1d2k4l4+[d1(b2−ησ)+(a1+ˆa)d2]k2l2−a2b1+(ˆa+a1)(b2−ησ),˜Kk=d1d2k4l4+[d1(b2+ησ)−(a1+ˆa)d2]k2l2+a2b1−(ˆa+a1)(b2+ησ),fork∈N0. | (2.15) |
˜S1={n|˜Kn<0,n∈N0},˜S2={n|˜Kn>0,˜Hn<0,˜H2n−4˜Jn˜Kn>0,n∈N0},˜S3={n|˜Kn>0,˜H2n−4˜Jn˜Kn<0,n∈N0}, | (2.16) |
ω±n=√12[−˜Hn±√˜H2n−4˜Jn˜Kn],τj,±n={1ω±narccos(V(n,±)cos)+2jπ,V(n,±)sin≥0,1ω±n[2π−arccos(V(n,±)cos)]+2jπ,V(n,±)sin<0.V(n,±)cos=(ω±n)2(b2˜En+˜Gn)−˜Mn˜Gn˜G2n+b22(ω±n)2,V(n,±)sin=ω±n(˜En˜Gn+˜Mnb2−b2(ω±n)2)˜G2n+b22(ω±n)2. | (2.17) |
Corollary 2.1. Assume (H2) holds, the following results hold.
● Equation (2.12) has a pair of purely imaginary roots ±iω+n at τj,+n for j∈N0 and n∈S1.
● Equation (2.12) has two pairs of purely imaginary roots ±iω±n at τj,±n for j∈N0 and n∈S2.
● Equation (2.12) has no purely imaginary root for n∈S3.
The transversal condition is also valid.
Corollary 2.2. Assume (H2) holds. Then Re(dλdτ)|τ=τj,+n>0, Re(dλdτ)|τ=τj,−n<0 for n∈˜S1∪˜S2 and j∈N0.
Denote ˜τ∗=min{τ0n|n∈˜S1∪˜S2}. We have the following theorem.
Corollary 2.3. For the model (2.10), assume (H2) holds.
● E∗(u∗,v∗) is locally asymptotically stable for τ>0 when ˜S1∪˜S2=∅.
● E∗(u∗,v∗) is locally asymptotically stable for τ∈[0,˜τ∗) when ˜S1∪˜S2≠∅.
● E∗(u∗,v∗) is unstable for τ∈(˜τ∗,˜τ∗+ε) for some ε>0 when ˜S1∪˜S2≠∅.
● Hopf bifurcation occurs at (u∗,v∗) when τ=τj,+n (τ=τj,−n), j∈N0, n∈˜S1∪˜S2. In addition, the spatially homogeneous (inhomogeneous) periodic solutions occur when τ=τj,±0 (τ=τj,±n, n>0).
By the work [22,23], we study the property of Hopf bifurcation. For fixed j∈N0 and n∈S1∪S2, we denote ˜τ=τj,±n. Let ˉu(x,t)=u(x,τt)−u∗ and ˉv(x,t)=v(x,τt)−v∗. Drop the bar, (1.3) can be written as
{∂u∂t=τ[d1Δu+(u+u∗)(1−1lπ∫lπ0(u(y,t)+u∗)dy)(u+u∗−β)−(1+α(v+v∗))(u+u∗)2(v+v∗)1+h(1+α(v+v∗))(u+u∗)2],∂v∂t=τ[d2Δvη((1+α(v(t−1)+v∗))(u(t−1)+u∗)2v(t−τ)1+h(1+α(v(t−1)+v∗))(u(t−1)+u∗)2−σv)]. | (3.1) |
We rewrite system (3.1) as following system
{∂u∂t=τ[d1Δu+a1u+a2v−ˆaˆu+α1u2−(2u∗−β)uˆu+α2uv+α3v2+α4u3+α5u2v+α6uv2+α7v3]+h.o.t.,∂v∂t=τ[d2Δv−ησv+b1u(t−1)+b2v(t−1)+β1u2(t−1)+β2u(t−1)v(t−1)+β3u2(t−1)+β4u3(t−1)+β5u2(t−1)v(t−1)]+β6u(t−1)v2(t−1)+β7v3(t−1)]+h.o.t., | (3.2) |
where α1=2v∗(αv∗+1)(3hu2∗(αv∗+1)−1)(hu2∗(αv∗+1)+1)3−2u∗+2, α2=−2(u3∗(αhv∗+h)+2αu∗v∗+u∗)(hu2∗(αv∗+1)+1)3, α3=−2u2∗(α+αhu2∗)(hu2∗(αv∗+1)+1)3, α4=−24hu∗v∗(αv∗+1)2(hu2∗(αv∗+1)−1)(hu2∗(αv∗+1)+1)4, α5=6u4∗(αhv∗+h)2+4hu2∗(5α2v2∗+6αv∗+1)−4αv∗−2(hu2∗(αv∗+1)+1)4, α6=4αu∗(h2u4∗(αv∗+1)+2αhu2∗v∗−1)(hu2∗(αv∗+1)+1)4, α7=6α2hu4∗(hu2∗+1)(hu2∗(αv∗+1)+1)4, β1=−2ηv∗(αv∗+1)(3hu2∗(αv∗+1)−1)(hu2∗(αv∗+1)+1)3, β2=2η(u3∗(αhv∗+h)+2αu∗v∗+u∗)(hu2∗(αv∗+1)+1)3;β3=2αηu2∗(hu2∗+1)(hu2∗(αv∗+1)+1)3, β4=24ηhu∗v∗(αv∗+1)2(hu2∗(αv∗+1)−1)(hu2∗(αv∗+1)+1)4, β5=−2η(3u4∗(αhv∗+h)2+2hu2∗(5α2v2∗+6αv∗+1)−2αv∗−1)(hu2∗(αv∗+1)+1)4, β6=−4αηu∗(h2u4∗(αv∗+1)+2αhu2∗v∗−1)(hu2∗(αv∗+1)+1)4, β7=−6α2ηhu4∗(hu2∗+1)(hu2∗(αv∗+1)+1)4.
Define the real-valued Sobolev space X:={(u,v)T:u,v∈H2(0,lπ),(ux,vx)|x=0,lπ=0}, the complexification of X is XC:=X⊕iX={x1+ix2|x1,x2∈X}. The inner product <˜u,˜v>:=∫lπ0¯u1v1dx+∫lπ0¯u2v2dx is for ˜u=(u1,u2)T, ˜v=(v1,v2)T, ˜u,˜v∈XC. The phase space C:=C([−1,0],X) is with the sup norm, then we can write ϕt∈C, ϕt(θ)=ϕ(t+θ) or −1≤θ≤0. Denote β(1)n(x)=(γn(x),0)T, β(2)n(x)=(0,γn(x))T, and βn={β(1)n(x),β(2)n(x)}, where {β(i)n(x)} is an orthonormal basis of X. We define the subspace of C as Bn:=span{<ϕ(⋅),β(j)n>β(j)n|ϕ∈C,j=1,2}, n∈N0. There exists a 2×2 matrix function ηn(σ,˜τ) −1≤σ≤0, such that −˜τDn2l2ϕ(0)+˜τL(ϕ)=∫0−1dηn(σ,τ)ϕ(σ) for ϕ∈C. The bilinear form on C∗×C is defined by
(ψ,ϕ)=ψ(0)ϕ(0)−∫0−1∫σξ=0ψ(ξ−σ)dηn(σ,˜τ)ϕ(ξ)dξ, | (3.3) |
for ϕ∈C, ψ∈C∗. Define τ=˜τ+μ, then the system undergoes a Hopf bifurcation at (0,0) when μ=0, with a pair of purely imaginary roots ±iωn0. Let A denote the infinitesimal generators of semigroup, and A∗ be the formal adjoint of A under the bilinear form (3.3). Define the following function
δ(n0)={1n0=0,0n0∈N. | (3.4) |
Choose ηn0(0,˜τ)=˜τ[(−n20/l2)D+L1+L3δ(nn0)], ηn0(−1,˜τ)=−˜τL2, ηn0(σ,˜τ)=0 for −1<σ<0. Let p(θ)=p(0)eiωn0˜τθ(θ∈[−1,0]), q(ϑ)=q(0)e−iωn0˜τϑ(ϑ∈[0,1]) be the eigenfunctions of A(˜τ) and A∗ corresponds to iωn0˜τ respectively. We can choose p(0)=(1,p1)T, q(0)=M(1,q2), where p1=1a2(iωn0+d1n20/l2−a1−ˆaδ(n0)), q2=e−iτωn0b1(−ˆaδ(n0)−a1+d1n2l2+iωn0), and M=(1+p1q2+˜τq2(b1+b2p1)e−iωn0˜τ)−1. Then (3.1) can be rewritten in an abstract form
dU(t)dt=(˜τ+μ)DΔU(t)+(˜τ+μ)[L1(Ut)+L2U(t−1)+L3ˆU(t)]+F(Ut,ˆUt,μ), | (3.5) |
where
F(ϕ,μ)=(˜τ+μ)(α1ϕ1(0)2−(2u∗−β)ϕ1(0)ˆϕ1(0)+α2ϕ1(0)ϕ2(0)+α3ϕ2(0)2+α4ϕ31(0)+α5ϕ21(0)ϕ2(0)+α6ϕ1(0)ϕ22(0)+α7ϕ32(0)β1ϕ21(−1)+β2ϕ1(−1)ϕ2(−1)+β3ϕ22(−1)+β4ϕ31(−1)+β4ϕ21(−1)ϕ2(−1)+β6ϕ1(−1)ϕ22(−1)+β7ϕ32(−1)) | (3.6) |
respectively, for ϕ=(ϕ1,ϕ2)T∈C and ˆϕ1=1lπ∫lπ0ϕdx. Then the space C can be decomposed as C=P⊕Q, where P={zpγn0(x)+ˉzˉpγn0(x)|z∈C}, Q={ϕ∈C|(qγn0(x),ϕ)=0and(ˉqγn0(x),ϕ)=0}. Then, system (3.6) can be rewritten as Ut=z(t)p(⋅)γn0(x)+ˉz(t)ˉp(⋅)γn0(x)+ω(t,⋅) and ^Ut=1lπ∫lπ0Utdx, where
z(t)=(qγn0(x),Ut),ω(t,θ)=Ut(θ)−2Re{z(t)p(θ)γn0(x)}. | (3.7) |
then, we have ˙z(t)=iω)n0˜τz(t)+ˉq(0)<F(0,Ut),βn0>. There exists a center manifold C0 and ω can be written as follow near (0,0).
ω(t,θ)=ω(z(t),ˉz(t),θ)=ω20(θ)z22+ω11(θ)zˉz+ω02(θ)ˉz22+⋯. | (3.8) |
Restrict the system to the center manifold is ˙z(t)=iωn0˜τz(t)+g(z,ˉz). Denote g(z,ˉz)=g20z22+g11zˉz+g02ˉz22+g21z2ˉz2+⋯. By direct computation, we have
g20=2˜τM(ς1+q2ς2)I3,g11=˜τM(ϱ1+q2ϱ2)I3,g02=ˉg20, |
g21=2˜τM[(κ11+q2κ21)I2+(κ12+q2κ22)I4], |
where I2=∫lπ0γ2n0(x)dx, I3=∫lπ0γ3n0(x)dx, I4=∫lπ0γ4n0(x)dx, ς1=(α1+ξ(α2+α3ξ))+δn0(β−2u∗), ς2=e−2iτωn(β1+ξ(β2+β3ξ)), ϱ1=14((2α1+α2(¯ξ+ξ)+2α3¯ξξ)+2δn0(β−2u∗)), ϱ2=14(2β1+β2(¯ξ+ξ)+2β3¯ξξ), κ11=2W(1)11(0)(2α1+α2ξ+βδn0+β−2(δn0+1)u∗)+W(1)20(0)(2α1+α2¯ξ+βδn0+β−2(δn0+1)u∗)+2W(2)11(0)(α2+2α3ξ)+W(2)20(0)(α2+2α3¯ξ), κ12=12(3α4+α5(¯ξ+2ξ)+ξ(2α6¯ξ+α6ξ+3α7¯ξξ)), κ21=2W(1)11(−1)(2β1+β2ξ)e−iτωn+2W(2)11(−1)(β2+2β3ξ)e−iτωn+W(1)20(−1)(2β1+β2¯ξ)eiτωn+W(2)20(−1)(β2+2β3¯ξ)eiτωn, κ22=12e−iτωn(3β4+β5(¯ξ+2ξ)+ξ(2β6¯ξ+β6ξ+3β7¯ξξ)).
Now, we compute W20(θ) and W11(θ) for θ∈[−1,0] to give g21. By (3.7), we have
˙ω=˙Ut−˙zpγn0(x)−˙ˉzˉpγn0(x)=Aω+H(z,ˉz,θ), | (3.9) |
where
H(z,¯z,θ)=H20(θ)z22+H11(θ)z¯z+H02(θ)¯z22+⋯. | (3.10) |
Compare the coeffcients of (3.8) with (3.9), we have
(A−2iωn0˜τI)ω20=−H20(θ),Aω11(θ)=−H11(θ). | (3.11) |
Then, we have
ω20(θ)=−g20iωn0˜τp(0)eiωn0˜τθ−ˉg023iωn0˜τˉp(0)e−iωn0˜τθ+E1e2iωn0˜τθ,ω11(θ)=g11iωn0˜τp(0)eiωn0˜τθ−ˉg11iωn0˜τˉp(0)e−iωn0˜τθ+E2, | (3.12) |
where E1=∑∞n=0E(n)1, E2=∑∞n=0E(n)2,
E(n)1=(2iωn0˜τI−∫0−1e2iωn0˜τθdηn0(θ,ˉτ))−1<˜F20,βn>,E(n)2=−(∫0−1dηn0(θ,ˉτ))−1<˜F11,βn>,n∈N0, |
<˜F20,βn>={1lπˆF20,n0≠0,n=0,12lπˆF20,n0≠0,n=2n0,1lπˆF20,n0=0,n=0,0,other,<˜F11,βn>={1lπˆF11,n0≠0,n=0,12lπˆF11,n0≠0,n=2n0,1lπˆF11,n0=0,n=0,0,other, |
and ˆF20=2(ς1,ς2)T, ˆF11=2(ϱ1,ϱ2)T.
Thus, we can obtain
c1(0)=i2ωn˜τ(g20g11−2|g11|2−|g02|23)+12g21,μ2=−Re(c1(0))Re(λ′(˜τ)),T2=−1ωn0˜τ[Im(c1(0))+μ2Im(λ′(τjn))],β2=2Re(c1(0)). | (3.13) |
By the work [22], we can obtain the following theorem.
Theorem 3.1. For any critical value τjn (n∈S,j∈N0), we have the following results.
● When μ2>0 (resp. <0), the Hopf bifurcation is forward (resp. backward).
● When β2<0 (resp. >0), the bifurcating periodic solutions on the center manifold are orbitally asymptotically stable (resp. unstable).
● When T2>0 (resp. T2<0), the period increases (resp. decreases).
To analyze the effect of the Allee effect, hunting cooperation, nonlocal competition and time delay on the model (1.3), we carry out numerical simulations in this section which is done with Matlab. The numerical simulation of the systems is implemented by finite-difference methods. In the later numerical simulation, we select the initial value as (u0(x)=u∗+0.001cosx,v0(x)=v∗−0.001cosx.), and have similar conclusions when we randomly select other initial values in the convergence domain. Fix the following parameters.
h=0.5,σ=0.3,η=0.2,d1=0.1,d2=0.1,l=1. |
The bifurcation diagrams of models (1.3) and (2.10) are given in Figures 1 and 2. It can be seen that the coexistence equilibrium will change from stable to unstable with the appearance of periodic solutions. In the model (1.3), the inhomogeneous Hopf bifurcation curve τ0,+1 exists, which implies that the stably spatially inhomogeneous periodic solutions may exist. But in the model (2.10), only the homogeneous Hopf bifurcation curve τ0,+0 exists, which implies that only the spatially homogeneous periodic solutions may exist. This implies that the model (1.3) with nonlocal competition is more realistic than the model (2.10), since the existence of periodic solutions in the model (1.3) is spatially inhomogeneous. Because the prey and predator will continue to spread in space and move from the place with high survival pressure to the place with low survival pressure, thus forming a non-uniform periodic oscillation. Therefore, we should consider the nonlocal competition within the population when establishing the delayed reaction-diffusion predator-prey model. We can obtain that increasing the Allee effect parameter β and hunting cooperation parameter α is not conducive to the stability of coexistence equilibrium points.
If we choose β=0.1 and α=1, then (u∗,v∗)=(0.5125,0.3436) is the unique coexisting equilibrium and the hypothesis (H1) holds. By direct computation, we have τ∗=τ01≈3.4439<τ00≈7.0688. By Theorem 2.1, we know that E∗(u∗,v∗) is locally asymptotically stable when τ∈[0,τ∗) (Figure 3). It can be seen that the coexisting equilibrium (u∗,v∗) is stable for models (1.3) and (2.10). For model (1.3), the Hopf bifurcation occurs when τ=τ∗. By Theorem 2.3, we have
μ2≈637.4179>0,β2≈−9.1705<0,T2≈−4.0035<0. |
Hence, the stably spatially inhomogeneous bifurcating periodic solutions exist for τ>τ∗ (Figure 4). This means that increasing the time delay τ can affect the stability of the coexisting equilibrium (u∗,v∗). In addition, the coexisting equilibrium (u∗,v∗) changes from stable to unstable and the stably spatially inhomogeneous bifurcating periodic solutions appear for the model (1.3). But with the same parameters, the coexisting equilibrium (u∗,v∗) is still stable for the model (2.10). Comparing Figure 4 and Figure 5, we can see that the nonlocal competition in prey can affect the dynamic properties of the predator-prey model and induce new dynamic phenomena (stably spatially inhomogeneous bifurcating periodic solutions).
Continue to increase the time delay τ until it is larger than the critical value τ0,+0, we can observe stable periodic solutions for both models (1.3) and (2.10). However, the stably spatially inhomogeneous bifurcating periodic solutions appear in model (1.3), and stably spatially homogeneous bifurcating periodic solutions appear in model (2.10). This also shows that nonlocal competition can affect the dynamic properties of the predator-prey model.
In this paper, considering the self-diffusion of prey and predator, nonlocal competition in prey, and gestation delay in predators, we propose a delayed diffusive predator-prey model with the Allee effect and nonlocal competition in prey and hunting cooperation in predators. We study the local stability of coexisting equilibrium and existence of Hopf bifurcation by analyzing the distribution of eigenvalues. We also study the property of Hopf bifurcation: bifurcation direction, stability of the periodic solution, period of the periodic solution by center manifold theorem and normal form method.
Our analysis results are verified by numerical simulation, and the influence of the Allee effect, hunting cooperation, nonlocal competition and time delay on the model is analyzed. By numerical simulation, we obtain that increasing the Allee effect parameter β and hunting cooperation parameter α will affect the stability of the coexistence equilibrium point, and there will be periodic solutions. The time delay can also affect the stability of coexisting equilibrium. When the time delay is less than the critical value, the coexistence equilibrium point is stable, and the densities of prey and predator will tend to the coexistence equilibrium. However, when the time delay is larger than the critical value, the coexistence equilibrium is unstable and the stable periodic solution appears. At this time, the density of prey and predator will produce periodic oscillation. The nonlocal competition in prey can affect the dynamic properties of the predator-prey model and induce new dynamic phenomena (stably spatially inhomogeneous bifurcating periodic solutions). Sometimes, the stability interval of a predator-prey model with nonlocal competition is smaller than that of a predator-prey model without nonlocal competition. This is also the reason why the predator-prey model with the nonlocal competition will have stably spatial inhomogeneous periodic solutions.
The main findings show that the Allee effect parameter β, hunting cooperation parameter α, and time delay τ can significantly affect the stability of the coexistence equilibrium point, and can be used control the development of the population.
This research is supported by the Fundamental Research Funds for the Central Universities (Grant No.2572022DJ05), Postdoctoral program of Heilongjiang Province (No.LBHQ21060) and College Students Innovations Special Project funded by Northeast Forestry University. Data sharing is not applicable to this article as no datasets were generated or analyzed during the current study.
The authors declare there is no conflicts of interest.
[1] |
A. C. R. Teixeira, P. G. Machado, F. M. de Almeida Collaço, D. Mouette, Alternative fuel technologies emissions for road heavy-duty trucks: a review, Environ. Sci. Pollut. Res., 28 (2021), 20954−20969. https://doi.org/10.1007/s11356-021-13219-8 doi: 10.1007/s11356-021-13219-8
![]() |
[2] |
T. Kuczek, Application of manufacturing constraints to structural optimization of thin-walled structures, Eng. Optim., 48 (2016), 351−360. https://doi.org/10.1080/0305215X.2015.1017350 doi: 10.1080/0305215X.2015.1017350
![]() |
[3] |
K. T. Cheng, N. Olhoff, An investigation concerning optimal design of solid elastic plates, Int. J. Solids Struct., 17 (1981), 305−323. https://doi.org/10.1016/0020-7683(81)90065-2 doi: 10.1016/0020-7683(81)90065-2
![]() |
[4] |
M. P. Bendsoe, N. Kikuchi, Generating optimal topologies in structural design using a homogenization method, Comput. Methods Appl. Mech. Eng., 71 (1988), 197−224. https://doi.org/10.1016/0045-7825(88)90086-2 doi: 10.1016/0045-7825(88)90086-2
![]() |
[5] |
M. P. Bendsoe, O. Sigmund, Material interpolation schemes in topology optimization, Arch. Appl. Mech., 69 (1999), 635−654. https://doi.org/10.1007/s004190050248 doi: 10.1007/s004190050248
![]() |
[6] |
J. M. Martínez, A note on the theoretical convergence properties of the SIMP method, Struct. Multidiscip. Optim., 29 (2005), 319−323. https://doi.org/10.1007/s00158-004-0479-8 doi: 10.1007/s00158-004-0479-8
![]() |
[7] |
M. Stolpe, K. Svanberg, An alternative interpolation scheme for minimum compliance topology optimization, Struct. Multidiscip. Optim., 22 (2001), 116−124. https://doi.org/10.1007/s001580100129 doi: 10.1007/s001580100129
![]() |
[8] | H. Zhang, X. H. Ren, Topology optimization of continuum structures based on SIMP, Adv. Mater. Res., Trans Tech Publications, Ltd., 255–260 (2011), 14–19. https://doi.org/10.4028/www.scientific.net/AMR.255-260.14 |
[9] |
W. J. Zuo, J. T. Bai, J. F. Yu, Sensitivity reanalysis of static displacement using Taylor series expansion and combined approximate method, Struct. Multidiscip. Optim., 53 (2016), 953−959. https://doi.org/10.1007/s00158-015-1368-z doi: 10.1007/s00158-015-1368-z
![]() |
[10] |
K. T. Zuo, L. P. Chen, Y. Q. Zhang, J. Yang, Manufacturing- and machining-based topology optimization, Int. J. Adv. Manuf. Technol., 27 (2006), 531−536. https://doi.org/10.1007/s00170-004-2210-8 doi: 10.1007/s00170-004-2210-8
![]() |
[11] |
X. J. Gao, L. J. Li, H. T. Ma, An adaptive continuation method for topology optimization of continuum structures considering buckling constraints, Int. J. Appl. Mech., 9 (2017). https://doi.org/10.1142/S1758825117500922 doi: 10.1142/S1758825117500922
![]() |
[12] |
S. Z. Xu, J. K. Liu, B. Zou, Q. H. Li, Y. S. Ma, Stress constrained multi-material topology optimization with the ordered SIMP method, Comput. Methods Appl. Mech. Eng., 373 (2021). https://doi.org/10.1016/j.cma.2020.113453 doi: 10.1016/j.cma.2020.113453
![]() |
[13] | Y. F. Bai, M. Cong, Y. Y. Li, Structural topology optimization for a robot upper arm based on SIMP method, in 3rd IEEE/IFToMM/ASME International Conference on Reconfigurable Mechanisms and Robots (ReMAR), 2015. https://doi.org/10.1007/978-3-319-23327-7_62 |
[14] | B. Mohamodhosen, F. Gillon, A. Tounzi, L. Chevallier, J. Korecki, Topology optimisation of a 3D electromagnetic device using the SIMP density-based method, in 2016 IEEE Conference on Electromagnetic Field Computation (CEFC), IEEE, Miami, FL, 2016. https://doi.org/10.1109/CEFC.2016.7816001 |
[15] | G. L. Srinivas, S. P. Singh, A. Javed, Experimental evaluation of topologically optimized manipulator-link using PLC and HMI based control system, in 3rd International E-Conference on Frontiers in Mechanical Engineering and NanoTechnology (ICFMET), 2020. https://doi.org/10.1016/j.matpr.2020.08.023 |
[16] | Q. Liu, X. K. Ma, Y. Z. Lin, Z. J. Zong, Topology and sizing optimization of light-weight frame for energy-saving vehicle, in International Conference on Advanced Design and Manufacturing Engineering (ADME 2011), Trans Tech Publications Ltd., 2011. https://doi.org/10.4028/www.scientific.net/AMR.308-310.1220 |
[17] |
B. Torstenfelt, A. Klarbring, Conceptual optimal design of modular car product families using simultaneous size, shape and topology optimization, Finite Elem. Anal. Des., 43 (2007), 1050−1061. https://doi.org/10.1016/j.finel.2007.06.005 doi: 10.1016/j.finel.2007.06.005
![]() |
[18] | L. Wang, X. K. Chen, Q. H. Zhao, Muti-objective topology optimization of an electric vehicle's traction battery enclosure, in Applied Energy Symposium and Summit - Low Carbon Cities and Urban Energy Systems (CUE), 2015. https://doi.org/10.1016/j.egypro.2016.06.103 |
[19] |
J. G. Cho, J. S. Koo, H. S. Jung, A lightweight design approach for an EMU carbody using a material selection method and size optimization, J. Mech. Sci. Technol., 30 (2016), 673−681. https://doi.org/10.1007/s12206-016-0123-8 doi: 10.1007/s12206-016-0123-8
![]() |
[20] |
S. B. Lu, H. G. Ma, W. J. Zuo, Lightweight design of bus frames from multi-material topology optimization to cross-sectional size optimization, Eng. Optim., 51 (2019), 961−977. https://doi.org/10.1080/0305215X.2018.1506770 doi: 10.1080/0305215X.2018.1506770
![]() |
[21] |
J. Bai, Y. Zhao, G. Meng, W. Zuo, Bridging topological results and thin-walled frame structures considering manufacturability, J. Mech. Des., 143 (2021). https://doi.org/10.1115/1.4050300 doi: 10.1115/1.4050300
![]() |
[22] |
H. Ma, J. Wang, Y. Lu, Y. Guo, Lightweight design of turnover frame of bridge detection vehicle using topology and thickness optimization, Struct. Multidiscip. Optim., 59 (2019), 1007−1019. https://doi.org/10.1007/s00158-018-2113-1 doi: 10.1007/s00158-018-2113-1
![]() |
[23] |
G. Y. Sun, D. D. Tan, X. J. Lv, X. L. Yan, Q. Li, X. D. Huang, Multi-objective topology optimization of a vehicle door using multiple material tailor-welded blank (TWB) technology, Adv. Eng. Software, 124 (2018), 1−9. https://doi.org/10.1016/j.advengsoft.2018.06.014 doi: 10.1016/j.advengsoft.2018.06.014
![]() |
[24] |
J. Zhang, L. Ning, Y. Hao, T. Sang, Topology optimization for crashworthiness and structural design of a battery electric vehicle, Int. J. Crashworthiness, 26 (2021), 651−660. https://doi.org/10.1080/13588265.2020.1766644 doi: 10.1080/13588265.2020.1766644
![]() |
[25] |
D. J. Munk, J. D. Miller, Topology optimization of aircraft components for increased sustainability, AIAAJ, 60 (2022), 445−460. https://doi.org/10.2514/1.J060259 doi: 10.2514/1.J060259
![]() |
[26] | Y. L. Lee, M. E. Barkey, H. T. Kang, Metal Fatigue Analysis Handbook: Practical Problem-Solving Techniques for Computer-Aided Engineering, Burlington: Elsevier Butterworth-Heinemann, (2012), 1−580. https://doi.org/10.1016/C2010-0-66376-0 |
[27] |
C. H. Wang, M. W. Brown, Life prediction techniques for variable amplitude multiaxial fatigue−Part 2: comparison with experimental results, J. Eng. Mater. Technol., 118 (1996), 371−374. https://doi.org/10.1115/1.2806822 doi: 10.1115/1.2806822
![]() |
[28] |
S. H. Jeong, J. W. Lee, G. H. Yoon, D. H. Choi, Topology optimization considering the fatigue constraint of variable amplitude load based on the equivalent static load approach, Appl. Math. Model., 56 (2018), 626−647. https://doi.org/10.1016/j.apm.2017.12.017 doi: 10.1016/j.apm.2017.12.017
![]() |
[29] | R. T. Haftka, Z. Gürdal, M. P. Kamat, Elements of structural optimization, Kluwer Academic Publishers, 1991. https://doi.org/10.1007/978-94-015-7862-2 |
[30] | D. L. Shu, Mechanical Property of Engineering Material, Beijing, China: China Machine Press, 2007. Available from: https://ss.zhizhen.com/detail_38502727e7500f26a296ae892b789bcc44b4f281d9485a991921b0a3ea25510134114c969f2eae5c13747adaf1f08d1c325955064941cd542e24c1535a7f8b4287df4e09375dba6c27789fd2eaaf69be. |
[31] |
A. Li, C. S. Liu, Lightweight design of a crane frame under stress and stiffness constraints using super-element technique, Adv. Mech. Eng., 9 (2017), 15. https://doi.org/10.1177/1687814017716621 doi: 10.1177/1687814017716621
![]() |
[32] |
O. Sigmund, J. Petersson, Numerical instabilities in topology optimization: a survey on procedures dealing with checkerboards, mesh-dependencies and local minima, Struct. Optim., 16 (1998), 68−75. https://doi.org/10.1007/BF01214002 doi: 10.1007/BF01214002
![]() |
[33] |
K. Svanberg, The method of moving asymptotes—a new method for structural optimization, Int. J. Numer. Methods Eng., 24 (1987), 359−373. https://doi.org/10.1002/nme.1620240207 doi: 10.1002/nme.1620240207
![]() |
[34] |
Z. Hu, S. Sun, O. Vambol, K. Tan, Topology optimization of laminated composite structures under harmonic force excitations, J. Compos. Mater., 56 (2022), 409−420. https://doi.org/10.1177/00219983211052605 doi: 10.1177/00219983211052605
![]() |
[35] |
Q. Q. Liang, Y. M. Xie, G. P. Steven, Optimal topology selection of continuum structures with displacement constraints, Comput. Struct., 77 (2000), 635−644. https://doi.org/10.1016/S0045-7949(00)00018-3 doi: 10.1016/S0045-7949(00)00018-3
![]() |
[36] |
X. J. Yang, J. Zheng, S. Y. Long, Topology optimization of continuum structures with displacement constraints based on meshless method, Int. J. Mech. Mater. Des., 13 (2017), 311−320. https://doi.org/10.1007/s10999-016-9337-2 doi: 10.1007/s10999-016-9337-2
![]() |
[37] |
M. Bruggi, On an alternative approach to stress constraints relaxation in topology optimization, Struct. Multidiscip. Optim., 36 (2008), 125−141. https://doi.org/10.1007/s00158-007-0203-6 doi: 10.1007/s00158-007-0203-6
![]() |
[38] |
C. Le, J. Norato, T. Bruns, C. Ha, D. Tortorelli, Stress-based topology optimization for continua, Struct. Multidiscip. Optim., 41 (2010), 605−620. https://doi.org/10.1007/s00158-009-0440-y doi: 10.1007/s00158-009-0440-y
![]() |
[39] |
S. H. Jeong, S. H. Park, D. H. Choi, G. H. Yoon, Topology optimization considering static failure theories for ductile and brittle materials, Comput. Struct., 110 (2012), 116−132. https://doi.org/10.1016/j.compstruc.2012.07.007 doi: 10.1016/j.compstruc.2012.07.007
![]() |
[40] | X. J. Peng, Fatigue Estimation based on Stress RMS under Stochastic Excitation, in 2nd International Conference on Advanced Electronic Materials, Computers and Materials Engineering (AEMCME), 2019. https://doi.org/10.1088/1757-899X/563/4/042058 |
1. | Miao Peng, Rui Lin, Zhengdi Zhang, Lei Huang, The dynamics of a delayed predator-prey model with square root functional response and stage structure, 2024, 32, 2688-1594, 3275, 10.3934/era.2024150 | |
2. | Xin Du, Quansheng Liu, Yuanhong Bi, Bifurcation analysis of a two–dimensional p53 gene regulatory network without and with time delay, 2023, 32, 2688-1594, 293, 10.3934/era.2024014 | |
3. | L. R. Ibrahim, D. K. Bahlool, Exploring the Role of Hunting Cooperation, and Fear in a Prey-Predator Model with Two Age Stages, 2024, 18, 1823-8343, 727, 10.47836/mjms.18.4.03 |