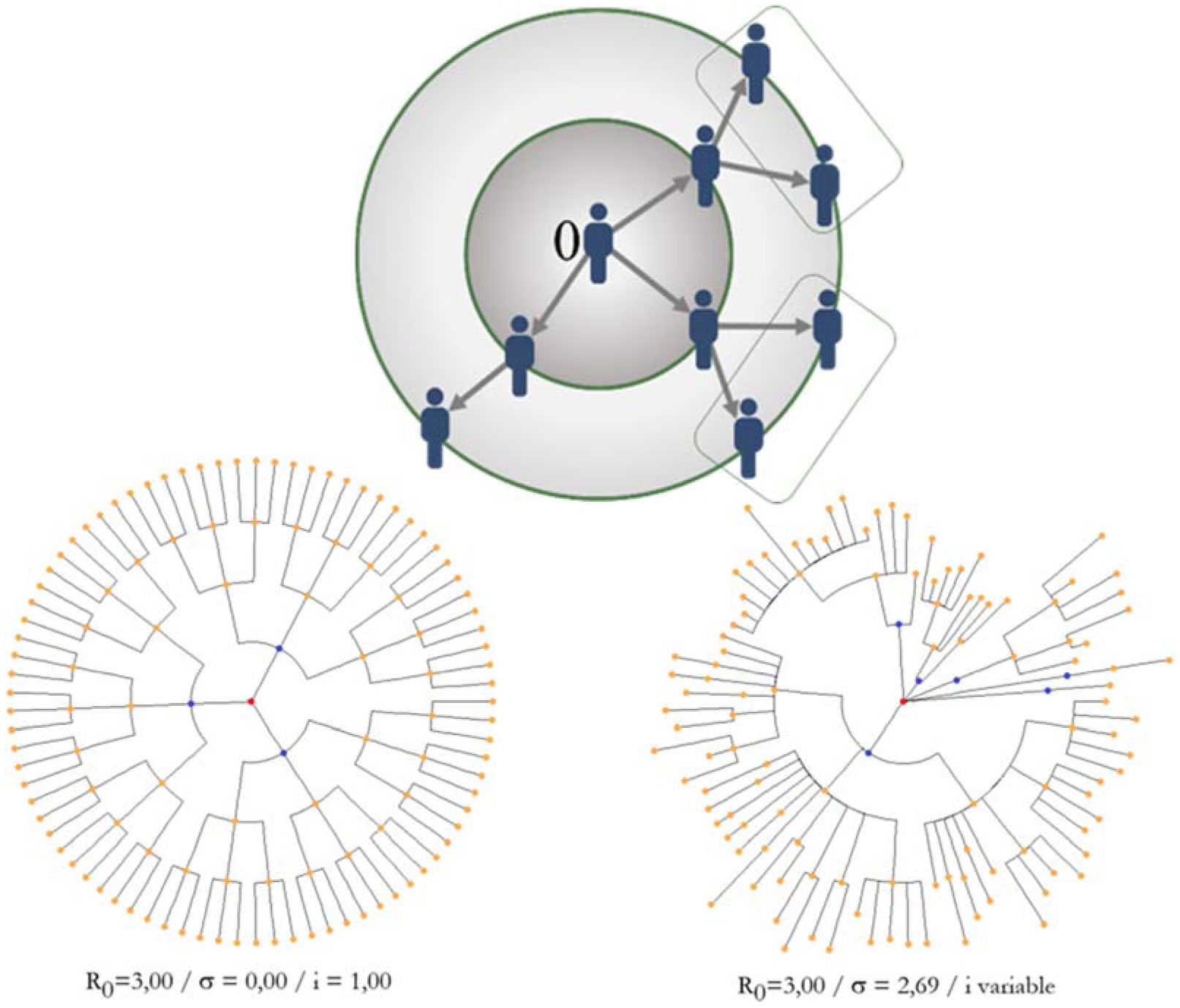
The present paper aims to apply the mathematical ideas of the contagion networks in a discrete dynamic context to the modeling of two current pandemics, i.e., COVID-19 and obesity, that are identified as major risks by the World Health Organization. After providing a reminder of the main tools necessary to model epidemic propagation in a Boolean framework (Hopfield-type propagation equation, notion of centrality, existence of stationary states), we present two applications derived from the observation of real data and involving mathematical models for their interpretation. After a discussion of the obtained results of model simulations, multidisciplinary work perspectives (both on mathematical and biomedical sides) are proposed in order to increase the efficiency of the models currently used and improve both the comprehension of the contagion mechanism and the prediction of the dynamic behaviors of the pandemics' present and future states.
Citation: Mariem Jelassi, Kayode Oshinubi, Mustapha Rachdi, Jacques Demongeot. Epidemic dynamics on social interaction networks[J]. AIMS Bioengineering, 2022, 9(4): 348-361. doi: 10.3934/bioeng.2022025
[1] | Ayub Ahmed, Bashdar Salam, Mahmud Mohammad, Ali Akgül, Sarbaz H. A. Khoshnaw . Analysis coronavirus disease (COVID-19) model using numerical approaches and logistic model. AIMS Bioengineering, 2020, 7(3): 130-146. doi: 10.3934/bioeng.2020013 |
[2] | Sarbaz H. A. Khoshnaw, Azhi Sabir Mohammed . Computational simulations of the effects of social distancing interventions on the COVID-19 pandemic. AIMS Bioengineering, 2022, 9(3): 239-251. doi: 10.3934/bioeng.2022016 |
[3] | Kayode Oshinubi, Augustina Amakor, Olumuyiwa James Peter, Mustapha Rachdi, Jacques Demongeot . Approach to COVID-19 time series data using deep learning and spectral analysis methods. AIMS Bioengineering, 2022, 9(1): 1-21. doi: 10.3934/bioeng.2022001 |
[4] | Júlia Novaes Matias, Gyovanna Sorrentino dos Santos Campanari, Gabriela Achete de Souza, Vinícius Marinho Lima, Ricardo José Tofano, Claudia Rucco Penteado Detregiachi, Sandra M. Barbalho . Metabolic syndrome and COVID-19. AIMS Bioengineering, 2020, 7(4): 242-253. doi: 10.3934/bioeng.2020021 |
[5] | Prasantha Bharathi Dhandapani, Dumitru Baleanu, Jayakumar Thippan, Vinoth Sivakumar . On stiff, fuzzy IRD-14 day average transmission model of COVID-19 pandemic disease. AIMS Bioengineering, 2020, 7(4): 208-223. doi: 10.3934/bioeng.2020018 |
[6] | Sarbaz H. A. Khoshnaw, Kawther Y. Abdulrahman, Arkan N. Mustafa . Identifying key critical model parameters in spreading of COVID-19 pandemic. AIMS Bioengineering, 2022, 9(2): 163-177. doi: 10.3934/bioeng.2022012 |
[7] | Eduardo Federighi Baisi Chagas, Piero Biteli, Bruno Moreira Candeloro, Miguel Angelo Rodrigues, Pedro Henrique Rodrigues . Physical exercise and COVID-19: a summary of the recommendations. AIMS Bioengineering, 2020, 7(4): 236-241. doi: 10.3934/bioeng.2020020 |
[8] | Cong Toai Truong, Kim Hieu Huynh, Van Tu Duong, Huy Hung Nguyen, Le An Pham, Tan Tien Nguyen . Model-free volume and pressure cycled control of automatic bag valve mask ventilator. AIMS Bioengineering, 2021, 8(3): 192-207. doi: 10.3934/bioeng.2021017 |
[9] | Kranthi Kumar Lella, Alphonse PJA . A literature review on COVID-19 disease diagnosis from respiratory sound data. AIMS Bioengineering, 2021, 8(2): 140-153. doi: 10.3934/bioeng.2021013 |
[10] | Rogério Leone Buchaim . Bioengineering applied to Covid-19 pandemic: from bench to bedside. AIMS Bioengineering, 2021, 8(1): 14-15. doi: 10.3934/bioeng.2021002 |
The present paper aims to apply the mathematical ideas of the contagion networks in a discrete dynamic context to the modeling of two current pandemics, i.e., COVID-19 and obesity, that are identified as major risks by the World Health Organization. After providing a reminder of the main tools necessary to model epidemic propagation in a Boolean framework (Hopfield-type propagation equation, notion of centrality, existence of stationary states), we present two applications derived from the observation of real data and involving mathematical models for their interpretation. After a discussion of the obtained results of model simulations, multidisciplinary work perspectives (both on mathematical and biomedical sides) are proposed in order to increase the efficiency of the models currently used and improve both the comprehension of the contagion mechanism and the prediction of the dynamic behaviors of the pandemics' present and future states.
For more than 24 years [1]–[15], specialists of discrete modeling have investigated the mechanism of epidemic spread whereby an infected patient can be infectious during a period of contagiousness and can recover in certain models [3] if they are connected through a path of healthy nodes to a central “node”, which could represent a public health or medical authority able to prescribe mitigation measures, treatments and/or vaccines. The authors applied, in general, an SIRS (Susceptible-Infected-Recovering-Susceptible) approach to an underlying network of individuals susceptible to enter into contact or to have interactions implying a physical proximity (Figure 1). An example of a contagion network, called the “school network”, authorizes long-distance links breaking the regular links of the geographic topology, and it seems to be realistic for modeling the remote interactions through which, for example, a social epidemic can diffuse. We will study in this paper two examples of epidemics: i) the COVID-19 outbreak and ii) a contagious social epidemic, obesity, which is not caused by infectious agents, but by alimentation habits, ways of life, propagation of rumors, etc., and is considered by the World Heath Organization (WHO) as a pandemic killing more individuals than many contagious infectious diseases.
We present in Section 2 the mathematical framework needed to model the infectious spread; in Section 3, we present the simulation results in the cases of COVID-19 and obesity, and eventually a discussion and conclusions with some perspectives concerning the application of the proposed methodology for the simulation of infectious diseases like the COVID-19 pandemic.
The methodology chosen in this study comes from an attempt to reconstruct an epidemic dynamic only from the observation of the number Rikj of people infected at day j by a given infectious individual i on the kth day of their period of contagiousness of length r days. By summing the number of infectious individuals Xj-k at day j–k, the number of new infected people on day j equals
We will assume now that Rikj is the same, i.e., equal to Rk, for all individuals i and days j, and depends only on day k. Then, we have
The convolution Eq (2) is the basis of our modeling of the epidemic dynamics and the sum of the daily reproduction numbers Rk's over the contagiousness period of length r, ∑k= 1,r Rk, plays the same role than the classical basic reproduction number R0 [17].
Let us suppose now that the secondarily infected individuals are recruited from the center of the sphere of influence of an infectious patient zero, and that the subsequently infected individuals remain on a sphere centered about this patient zero, by just widening its radius on Day 2. Therefore, the susceptible individuals Xj, whom the infectious individuals at day j-1 can recruit, are inside their zone of influence on day j-1 (rectangles on Figure 1, top). Let us now choose the simple deterministic rule of infection of Eq 2 and denote by Xj the number of new infected cases at day j (j ≥ 1), and by Rk (k = 1,..., r) the daily reproduction number at day k of the contagiousness period of length r for all infectious individuals. Then, we have obtained Eq 2 by supposing that the contagiousness behavior is the same for all infectious individuals: for example, if r = 3, we get, for the number X5 of new cases at Day 5, the equation X5 = R1X4 + R2X3 + R3X2; this means that, for example, new cases at Day 4 have contributed to new cases at Day 5 with the term R1X4, R1 being the reproduction number at the first day of contagiousness of new infectious patients at Day 4. In matrix form, we get:
where X = (Xj,..., Xj-r-1) and R = (R1,..., Rr) are r-dimensional vectors and M is the r-r matrix:
It is easy to show that, if X0 = 1 and r =5, we obtain
If r = 2 and R1 = R2 = R/2, we have
If r = R = 2, {Xj}i=1,∞ is exactly the Fibonacci sequence, and for R > 0, the generalized Fibonacci sequence.
This approach has been generalized to the continuous differential case where there is an unknown set of patient zeros [18],[19], and to the fractional differential framework [20]–[22].
Let us consider a stochastic Hopfield-like transition rule for the calculation of the Boolean weight state Oi(t + 1) of an individual i (equal to 0 in the case of normal weight and to 1 in the case of being overweight or obesity) as a function of the Ok(t)s by using a potential function Pi:
where N(i) is the set of nodes linked to i in the oriented interaction graph of the contagion network between the individuals of the studied population (Figure 1), Ti is a tolerance parameter (varying between 0 and +∞) quantifying the level of indifference shown by i to the weight state of the nodes belonging to the neighborhood N(i) of i and wik is the interaction coefficient measuring the influence (positive or negative) an individual k has on individual i (its value can be equal to 1, 0 or -1 in the absence of precise estimation of this influence). Then, the stochastic transition rule is as follows:
In Eq 8, the function Pi is the analogue of the Hamiltonian function of the Hopfield model [9], and the indifference parameter Ti is an analogue of the temperature: higher the indifference, nearer the value ½ is the probability to become obese, which corresponds to a quasi-absence of influence of the individual i on their neighbors regardless of their weight status.
There are four classical types of centrality in the oriented interaction graph G of a contagion network (Figure 1, top). The first is the betweenness centrality, defined for a node k as follows [23]:
where ßij(k) is the total number of shortest paths from the node i to node j that pass through the node k, and ßk = ∑i ≠ j∈G ßij(k). The second type of centrality is the degree centrality, defined from the notions of out-, in- or total-degree of a node i, corresponding to the number of arrows of the interaction graph respectively exiting or entering in node i, or both, e.g., the total-degree centrality is defined by
where aij = sign(wij) denotes the general coefficient of the signed incidence matrix A of the graph G.
The third type of centrality is the closeness centrality. The closeness is the inverse of the average farness, which is defined by averaging a distance between i and j ≠ i:
where the distance can be L(i,j), i.e., the length of the closest path between i and j.
The last type of classical centrality is the spectral centrality or eigen-centrality, which takes into account the fact that the neighbors of a node i in the interaction graph G can also be highly connected to the other nodes of G, considering that connections to highly connected nodes contribute to the centrality of i more than connections to weakly connected nodes. Hence, the eigenvector centrality of the node i better represents the global influence of i on the whole contagion network and verifies [11]:
where λ is the dominant eigenvalue of the incidence matrix of the interaction graph G and V is its eigenvector. The four centralities above can be very different (Figure 3), but they each have intrinsic interests: i) betweenness centrality relates to the global connectivity of a node with all nodes of the network, ii) degree centralities correspond to the local connectivity with only the nearest nodes, iii) closeness centrality measures the relative proximity with other nodes for a given distance on the interaction graph and iv) spectral centrality corresponds to the ability to be connected to, possibly, a few number of nodes, but having a high connectivity, e.g., important hub-relays controlling wide sub-networks [24]. Despite the already complementary properties of the classical centralities, we introduce a new notion of centrality, called entropy centrality, taking into account the heterogeneity of the distribution of states of the neighbors of a node i, i.e., not only its connectivity in the graph, and highlighting the symmetric sub-graphs:
where νk denotes the kth frequency among si frequencies of the histogram of the state values observed in the neighborhood N(i) of the node i, and N(i) is the set of the nodes out- or in-linked to the node i.
Let us now consider the COVID-19 outbreak in the USA, for which the estimation of the length of the contagiousness period equal 7 days [25]. The numbers of new cases at the start of the disease from February 21 to March 5 2020 were extracted from [26]. Then, we have, by deconvoluting Eq 2:
The evolution of the Rj values shows in Figure 2 a U-shape with a minimum on Day 4 and a sum of the Rj values that is equal to 2.72, which is less than the value of 3 of the effective reproduction number calculated in [27]. This U-shape is similar to the classical shape observed for the influenza disease [28].
We are interested in modeling the social contagion mechanism by which obesity can propagate from individual to individual in the same or close classes from a high school via the pupil population, where pupils change their weight under the influence of their school friends connected to them through a declared friendship link (possibly not reciprocal).
In Figure 3, we have represented on the left the observed friendship network in two classes of a Tunisian high school with 274 pupils having 524 friendship links between them [29]–[33]. The Tunisian sample contains 18 individuals who are overweight or obese.
The links are directed, and they can be unique between two nodes, when a friendship without reciprocity has been detected (for the sake of simplicity, arrows have not been represented, but in a couple of potential friends, the final node has in general an area more important than the first one). To visually represent the connectivity of the network, we have chosen to give the nodes a surface proportional to their in-degree centrality in Figures 3a,b, total-degree centrality in Figure 3c, and spectral centrality in Figure 3d. In the three last representations, we have leaved the friendship network evolve to its asymptotic state following the Eq 8, we have kept the proportionality of the node area to its centrality and put together the nodes of the network having their centrality in the same value interval. As we can see on Figures 3b and 3c, the total and in-degree centralities give about the same feature, but for the spectral centrality in Figure 3d, the neighbors of a node i play contribute more to its area if they are highly connected to the other nodes, because these second level highly connected nodes contribute to the spectral centrality of i more than the weakly connected ones.
We see in Figure 3 that the spectral centrality (b) gives priority to the nodes of the contagion network (a) connected to hubs on the left bottom part of the contagion network. On the contrary, the total-degree centrality (c) favors all hubs, even when they are connected to a relatively small number of nodes, and the in-degree centrality (d) gives an importance to all nodes depending on the number of other nodes expressing their friendship for them. If the aim of such a study is to diminish the number of obese individuals, the identification of hubs in the obese or overweight state is important: applying nutritional reeducation to a part of these obese or overweight hubs can drastically reduce the number of obese or overweight individuals in their neighborhood.
For example, if we diminish sufficiently their influence by increasing their tolerance parameter Ti, we can suppress their influence and then reduce the risk to become obese at their contact. Let us suppose that we start with an interaction network of 100 individuals including 100 obese or overweight individuals and zero normal weight individuals, with interaction weights wij chosen at random to be either 0 or 1. Figure 4 shows that, by increasing the value of the tolerance parameter Ti for the eight most connected nodes, the mean percentage of normal weight individuals increases until the value of ½, corresponding to a random choice equilibrated between normality on one hand, and overweight or obesity on the second hand. That shows that the states of the nodes of the network can be regularized to the normality after a campaign targeted at the most influential obese or overweight individuals of the contagion network.
The top image of Figure 5 shows the main connected component (89 nodes) of a friendship interaction graph for two classes of a French high school with 104 pupils having 348 friendship links between them [29]–[33]. The French sample contains 17 overweight or obese individuals.
We see in Figure 5 that the spectral centrality (niddle-left) of the French sample friendship interaction graph gives priority to the big hubs and nodes connected to these hubs. On the contrary, the total-degree centrality (middle-right) favors all hubs, even those connected to a relatively small number of nodes.
The entropy centrality identifies nodes having different states in their neighborhood and gives them an importance higher than that for the nodes having only one type of state in their neighborhood. Once stabilized after changing the state of the 20 most influential nodes (for example, after a dedicated nutritional education) in the left image and of the 21 most influential in the right image of the bottom of Figure 5, we can observe that the stationary state of the network is fully overweight or obese in the first case of 20 state changes, and fully normal in the second case of 21 state changes, showing a transition of the invariant measure of the contagion network.
In the two applications of contagion networks presented in Section 3, we can discuss some simplifications made in the models:
- The small number of states simplifies the dynamics but complicates the examination of realistic solutions to stop the epidemic. We should, for example, distinguish overweight and obese states in the first case, because the return to a normal weight is much easier for an overweight individual than for an obese one. In the second case, the two-state variable "vaccinated or not" should be added in order to properly take into account the effect of a vaccination policy.
- The diffusion through nearest neighbors is often unrealistic; hence. it is necessary to build contagion networks with variable links over time to take into account, for example, the movement of individuals. This non-constancy of the links can be the subject of a periodic dynamic (linked to school or professional constraints), but it adds a high level of complexity to the classical modeling with fixed links over time.
Another limitation is the comparison of the results of the simulations with the real behavior of the epidemic, of which we see, in the case of the COVID-19 outbreak, that most of the parameters of transmissibility and contagiousness can change over time [8],[13],[25],[34]–[40]. These changes are linked to the following:
i) the source host of the virus (in whom the intensity of the symptoms varies during the period of contagiousness),
ii) the infectious agent (whose virulence is variable, due, for example, to mutations),
iii) the medium of atmospheric, cutaneous or intestinal transmission (because it depends on numerous environmental conditions, such as temperature and humidity),
iv) the future virus host (in whom the immunological state depends on multiple factors, including age, comorbidities, cross immunity, etc.).
The long reaction time to mitigation or vaccination measures decided on the basis of the models makes it very difficult to adapt them to the new propagation conditions and considerably limits the operational character of the models, even if their short-term predictions are often of very good quality.
Among other possible approaches is the use of the Caputo fractional derivative [20]–[22], which is particularly interesting. The Caputo fractional derivative of a function f equals
where the integers n and p verify n − 1 < q < n.
The use of the fractional derivative in the models makes it possible to obtain simulations of the increase in the cumulative cases of infected individuals, which fits the data better than the classical derivative, particularly when the phenomenon of growth of the infected is damped (at the end of an epidemic wave), i.e., after the very early exponential phase where the classical approach presents a slightly lower error than the fractional one [22].
The current context of the two pandemics closely monitored by the WHO, i.e., a contagious social pandemic, obesity, and a contagious infectious pandemic, COVID-19, shows that the problem of contagion control is still relevant. To be able to be both retro-predictive on past pandemics (like the great plague of 1348 in Europe) and anticipatory on present and future pandemics, it is necessary to be able, from the observed data (which are daily in the case of the COVID-19 [26],[27] and annual in the case of obesity [41]), to estimate the parameters of the model and then simulate it and interpret the scenarios of the simulations within the framework of concrete public health measures (change in eating behaviors, preventive improvement of the immune system defenses, implementation of mitigation measures, vaccination, etc.). This last interpretative part is the most difficult and is only effective within the framework of multidisciplinary teams, including mathematicians of dynamical systems, nutritionists and infectious disease specialists. Solid knowledge of the genetics of infectious agents and on the monitoring of environmental conditions is necessary because of the following reasons:
- the dynamical phenomena of propagation present isolated or periodic waves of new cases, with bifurcations (in the deterministic case) or invariant measure transitions (in the stochastic case) that are often difficult to study theoretically or simulate numerically [42];
- the changes in the virulence of the agents at the origin of pandemics require new knowledge about contagion mechanisms that are often partly unknown; this is linked to uneasily predictable and often hypothetical modifications in their genome (point mutations, deletion and insertion of more or less long sequences, recombination with other genomes, passage in the host nuclear genome, etc.). These changes require us to generally consider the stability of the model dynamics vis-à-vis the variations of their variables, as well as its robustness vis-à-vis the variations of their parameters [43],[44]. The study of the dependence of dynamical behaviors on changes due to the evolution of pandemics, particularly those under a vaccination policy [45]–[48], constitutes a difficult theoretical and numerical challenge for the future.
[1] |
Albert R, Thakar J (2014) Boolean modeling: a logic-based dynamic approach for understanding signaling and regulatory networks and for making useful predictions. Wires Syst Biol Med 6: 353-369. https://doi.org/10.1002/wsbm.1273 ![]() |
[2] |
Barrat A, Barthélémy M, Vespignani A (2008) Dynamical Processes on Complex Networks. Cambridge: Cambridge University Press. https://doi.org/10.1017/CBO9780511791383 ![]() |
[3] |
Böttcher L, Woolley-Meza O, Goles E, et al. (2016) Connectivity disruption sparks explosive epidemic spreading. Phys Rev E 93: 042315S. https://doi.org/10.1103/PhysRevE.93.042315 ![]() |
[4] |
Buscarino A, Fortuna L, Frasca M, et al. (2008) Disease spreading in populations of moving agents. Europhys Lett 82: 38002. https://doi.org/10.1209/0295-5075/82/38002 ![]() |
[5] |
Cheng HY, Jian SW, Liu DP, et al. (2020) Contact tracing assessment of COVID-19 transmission dynamics in Taiwan and risk at different exposure periods before and after symptom onset. JAMA Intern Med 180: 1156-1163. https://doi.org/10.1371/journal.pcbi.1000656 ![]() |
[6] |
Ferretti L, Wymant C, Kendall M, et al. (2020) Quantifying SARS-CoV-2 transmission suggests epidemic control with digital contact tracing. Science 368: eabb6936. https://doi.org/10.1126/science.abb6936 ![]() |
[7] |
Funk S, Gilad E, Watkins C, et al. (2009) The spread of awareness and its impact on epidemic outbreaks. Proc Natl Acad Sci USA 106: 6872-6877. https://doi.org/10.1073/pnas.0810762106 ![]() |
[8] |
Gaudart J, Landier J, Huiart L, et al. (2021) Factors associated with spatial heterogeneity of Covid-19 in France: a nationwide ecological study. The Lancet Public Health 6: e222-e231. https://doi.org/10.1016/S2468-2667(21)00006-2 ![]() |
[9] |
Hopfield JJ (1982) Neural networks and physical systems with emergent collective computational abilities. Proc Natl Acad Sci USA 79: 2554-2558. https://doi.org/10.1073/pnas.79.8.2554 ![]() |
[10] |
Morone F, Makse HA (2015) Influence maximization in complex networks through optimal percolation. Nature 524: 65-68. https://doi.org/10.1038/nature14604 ![]() |
[11] | Negre CFA, Morzan UN, Hendrickson HP, et al. (2021) Eigenvector centrality for characterization of protein allosteric pathways. Proc Natl Acad Sci USA 115: 12201-12208. https://doi.org/10.1073/pnas.1810452115 |
[12] | Oshinubi K, Rachdi M, Demongeot J (2022) Approach to COVID-19 time series data using deep learning and spectral analysis methods. AIMS Bioeng 8: 9-21. https://doi.org/10.3934/bioeng.2022001 |
[13] |
Rachdi M, Waku J, Hazgui H, et al. (2021) Entropy as robustness marker in genetic regulatory networks. Entropy 22: 260. https://doi.org/10.3390/e22030260 ![]() |
[14] |
Szell M, Lambiotte R, Thurner S (2010) Multirelational organization of large-scale social networks in an online world. Proc Natl Acad Sci 107: 13636-13641. https://doi.org/10.1073/pnas.1004008107 ![]() |
[15] | Zhu P, Wang X, Li S, et al. (2019) Investigation of epidemic spreading process on multiplex networks by incorporating fatal properties. Appl Math Comput 359: 512-524. https://doi.org/10.1016/j.amc.2019.02.049 |
[16] | Lacoude P (2020). Available from: https://www.contrepoints.org/2020/07/22/376624-covid-19-lx10-debut-dx10-la-fin-1. |
[17] |
Demongeot J, Oshinubi K, Rachdi M, et al. (2021) Estimation of Daily Reproduction Numbers in COVID-19 Outbreak. Computation 9: 109. https://doi.org/10.3390/computation9100109 ![]() |
[18] | Demongeot J, Griette Q, Maday Y, et al. (2022) A Kermack-McKendrick model with age of infection starting from a single or multiple cohorts of infected patients. ArXiv 2022: 2205.15634. |
[19] | Demongeot J, Magal P Spectral method in epidemic time series (2022). |
[20] |
Rezapour S, Etemad S, Mohammadi H (2020) A mathematical analysis of a system of Caputo–Fabrizio fractional differential equations for the anthrax disease model in animals. Adv Differ Equ 2020: 481. https://doi.org/10.1186/s13662-020-02937-x ![]() |
[21] |
Khan H, Alzabut J, Shah A, et al. (2022) A study on the fractal-fractional tobacco smoking model. AIMS Math 7: 13887-13909. https://doi.org/10.3934/math.2022767 ![]() |
[22] |
Tuan NH, Mohammadi H, Rezapour S (2020) Rezapour, A mathematical model for COVID-19 transmission by using the Caputo fractional derivative. Chaos Solitons Fractals 140: 110107. https://doi.org/10.1016/j.chaos.2020.110107 ![]() |
[23] |
Barthélémy M (2004) Betweenness centrality in large complex networks. Eur Phys J B 38: 163-168. https://doi.org/10.1140/epjb/e2004-00111-4 ![]() |
[24] |
Parmer T, Rocha LM, Radicchi F (2022) Influence maximization in Boolean networks. Nat Commun 13: 3457. https://doi.org/10.1038/s41467-022-31066-0 ![]() |
[25] | Demongeot J, Oshinubi K, Rachdi M, et al. (2021) The application of ARIMA model to analyze COVID-19 incidence pattern in several countries. J Math Comput Sci 12: 10. https://doi.org/10.28919/jmcs/6541 |
[26] | Worldometer database (2022). Available from: https://www.worldometers.info/coronavirus/. |
[27] | Renkulab database (2022). Available from: https://renkulab.shinyapps.io/COVID-19-Epidemic-Forecasting/_w_e213563a/?tab=ecdc_pred&country=France. |
[28] |
Chao DL, Halloran ME, Obenchain VJ, et al. (2010) FluTE, a publicly available stochastic influenza epidemic simulation model. PLoS Comput 6: e1000656. https://doi.org/10.1371/journal.pcbi.1000656 ![]() |
[29] |
Demongeot J, Taramasco C (2014) Evolution of social networks: the example of obesity. Biogerontology 15: 611-626. https://doi.org/10.1007/s10522-014-9542-z ![]() |
[30] |
Demongeot J, Hansen O, Taramasco C (2015) Complex systems and contagious social diseases: example of obesity. Virulence 7: 129-140. https://doi.org/10.1080/21505594.2015.1082708 ![]() |
[31] |
Demongeot J, Elena A, Jelassi M, et al. (2016) Smart homes and sensors for surveillance and preventive education at home: example of obesity. Information 7: 50. https://doi.org/10.3390/info7030050 ![]() |
[32] |
Demongeot J, Jelassi M, Taramasco C (2017) From susceptibility to frailty in social networks: the case of obesity. Math Pop Studies 24: 219-245. https://doi.org/10.1080/08898480.2017.1348718 ![]() |
[33] |
Demongeot J, Jelassi M, Hazgui H, et al. (2018) Biological networks entropies: examples in neural memory networks, genetic regulation networks and social epidemic networks. Entropy 20: 36. https://doi.org/10.3390/e20010036 ![]() |
[34] |
Demongeot J, Griette Q, Magal P (2020) SI epidemic model applied to COVID-19 data in mainland China. Roy Soc Open Sci 7: 201878. https://doi.org/10.1098/rsos.201878 ![]() |
[35] | Demongeot J, Griette Q, Magal P, et al. (2022) Modelling vaccine efficacy for COVID-19 outbreak in New York City. Biology (Basel) 11: 345. https://doi.org/10.3390/biology11030345 |
[36] | Griette Q, Demongeot J, Magal P (2021) A robust phenomenological approach to investigate COVID-19 data for France. Math Appl Sci Eng 2: 149-160. https://doi.org/10.5206/mase/14031 |
[37] |
Griette Q, Demongeot J, Magal P (2021) What can we learn from COVID-19 data by using epidemic models with unidentified infectious cases?. Math Biosci Eng 19: 537-594. https://doi.org/10.3934/mbe.2022025 ![]() |
[38] |
Oshinubi K, Rachdi M, Demongeot J (2022) Modelling of COVID-19 pandemic vis-à-vis some socio-economic factors. Front Appl Math Stat 7: 786983. https://doi.org/10.3389/fams.2021.786983 ![]() |
[39] |
Oshinubi K, Ibrahim F, Rachdi M, et al. (2022) Functional data analysis: Application to daily observation of COVID-19 prevalence in France. AIMS Math 7: 5347-5385. https://doi.org/10.3934/math.2022298 ![]() |
[40] |
Waku J, Oshinubi K, Demongeot J (2022) Maximal reproduction number estimation and identification of transmission rate from the first inflection point of new infectious cases waves: COVID-19 outbreak example. Math Comput Simulat 198: 47-64. https://doi.org/10.1016/j.matcom.2022.02.023 ![]() |
[41] | Ourworldindata (2022). Available online: https://ourworldindata.org/obesity/. |
[42] |
Demongeot J, Goles E, Morvan M, et al. (2010) Attraction basins as gauges of environmental robustness in biological complex systems. PloS One 5: e11793. https://doi.org/10.1371/journal.pone.0011793 ![]() |
[43] |
Aracena J, Goles E, Moreira A, et al. (2009) On the robustness of update schedules in Boolean networks. Biosystems 97: 1-8. https://doi.org/10.1016/j.biosystems.2009.03.006 ![]() |
[44] |
Demongeot J, Ben Amor H, Elena A, et al. (2009) Robustness in regulatory interaction networks. A generic approach with applications at different levels: physiologic, metabolic and genetic. Int J Mol Sci 10: 4437-4473. https://doi.org/10.3390/ijms10104437 ![]() |
[45] |
Turkyilmazoglu M (2021) Explicit formulae for the peak time of an epidemic from the SIR model. Physica D 422: 132902. https://doi.org/10.1016/j.physd.2021.132902 ![]() |
[46] |
Turkyilmazoglu M (2022) An extended epidemic model with vaccination: weak-immune SIRVI. Physica A 598: 127429. https://doi.org/10.1016/j.physa.2022.127429 ![]() |
[47] |
Turkyilmazoglu M (2022) A restricted epidemic SIR model with elementary solutions. Physica A 600: 127570. https://doi.org/10.1016/j.physa.2022.127570 ![]() |
[48] |
Xu Z, Yang D, Wang L, et al. (2022) Statistical analysis supports UTR (untranslated region) deletion theory in SARS-CoV-2. Virulence 13: 1772-1789. https://doi.org/10.1080/21505594.2022.2132059 ![]() |
1. | Jules Waku, Kayode Oshinubi, Umar Muhammad Adam, Jacques Demongeot, Forecasting the Endemic/Epidemic Transition in COVID-19 in Some Countries: Influence of the Vaccination, 2023, 11, 2079-9721, 135, 10.3390/diseases11040135 | |
2. | Houssem Ben Khalfallah, Mariem Jelassi, Narjes Bellamine Ben Saoud, Jacques Demongeot, 2023, Chapter 19-2, 978-3-319-12125-3, 1, 10.1007/978-3-319-12125-3_19-2 | |
3. | Houssem Ben Khalfallah, Mariem Jelassi, Narjes Bellamine Ben Saoud, Jacques Demongeot, 2023, Chapter 19, 978-3-031-40115-2, 229, 10.1007/978-3-031-40116-9_19 | |
4. | Jacques Demongeot, Pierre Magal, Kayode Oshinubi, Forecasting the changes between endemic and epidemic phases of a contagious disease, with the example of COVID-19, 2024, 1477-8599, 10.1093/imammb/dqae012 |