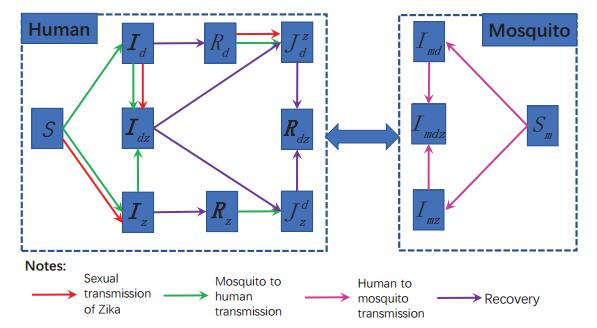
Low-light stress can inhibit plant growth and production. The selection of superior genotypes that are adaptive and tolerant to low light intensity needs to be performed. This study evaluated the agronomic responses of ten doubled haploid lines of eggplant and three varieties to various shade levels (0%, 25%, and 50%). This study used a split-plot design with three replicates. The results indicate that 25% shade is the optimal selection environment for shade tolerance. Genotypes grown under 25% shade exhibited varying responses, with two genotypes classified as shade-loving, seven as tolerant, three as moderately tolerant, and one as shade-sensitive. Shade levels of up to 50% significantly reduced yield, with production dropping to 58%–81% of the unshaded conditions. In general, growth characteristics such as plant height, leaf width, and leaf area were better under shaded conditions, whereas the yield was better under unshaded conditions. AM 23, AM 14, and AM 10 showed better tolerance responses and maintained better yields than the other lines under 25% shade. This study provides important insights into the development of shade-tolerant eggplant varieties. These findings can be used as a basis for recommendations for eggplant planting in shaded areas such as agroforestry systems.
Citation: Samsul Ma'arif, Bambang Sapta Purwoko, Arya Widura Ritonga, Iswari Saraswati Dewi. Effects of shade on agronomic traits of doubled haploid lines of eggplant (Solanum melongena L.) obtained from anther culture[J]. AIMS Agriculture and Food, 2025, 10(1): 199-217. doi: 10.3934/agrfood.2025011
[1] | Fabio Sanchez, Luis A. Barboza, Paola Vásquez . Parameter estimates of the 2016-2017 Zika outbreak in Costa Rica: An Approximate Bayesian Computation (ABC) approach. Mathematical Biosciences and Engineering, 2019, 16(4): 2738-2755. doi: 10.3934/mbe.2019136 |
[2] | Hai-Feng Huo, Tian Fu, Hong Xiang . Dynamics and optimal control of a Zika model with sexual and vertical transmissions. Mathematical Biosciences and Engineering, 2023, 20(5): 8279-8304. doi: 10.3934/mbe.2023361 |
[3] | Minna Shao, Hongyong Zhao . Dynamics and optimal control of a stochastic Zika virus model with spatial diffusion. Mathematical Biosciences and Engineering, 2023, 20(9): 17520-17553. doi: 10.3934/mbe.2023778 |
[4] | Yanfeng Liang, David Greenhalgh . Estimation of the expected number of cases of microcephaly in Brazil as a result of Zika. Mathematical Biosciences and Engineering, 2019, 16(6): 8217-8242. doi: 10.3934/mbe.2019416 |
[5] | Maghnia Hamou Maamar, Matthias Ehrhardt, Louiza Tabharit . A nonstandard finite difference scheme for a time-fractional model of Zika virus transmission. Mathematical Biosciences and Engineering, 2024, 21(1): 924-962. doi: 10.3934/mbe.2024039 |
[6] | Vanessa Steindorf, Sergio Oliva, Jianhong Wu . Cross immunity protection and antibody-dependent enhancement in a distributed delay dynamic model. Mathematical Biosciences and Engineering, 2022, 19(3): 2950-2984. doi: 10.3934/mbe.2022136 |
[7] | Eunha Shim . Optimal dengue vaccination strategies of seropositive individuals. Mathematical Biosciences and Engineering, 2019, 16(3): 1171-1189. doi: 10.3934/mbe.2019056 |
[8] | Bo Zheng, Wenliang Guo, Linchao Hu, Mugen Huang, Jianshe Yu . Complex wolbachia infection dynamics in mosquitoes with imperfect maternal transmission. Mathematical Biosciences and Engineering, 2018, 15(2): 523-541. doi: 10.3934/mbe.2018024 |
[9] | Haitao Song, Dan Tian, Chunhua Shan . Modeling the effect of temperature on dengue virus transmission with periodic delay differential equations. Mathematical Biosciences and Engineering, 2020, 17(4): 4147-4164. doi: 10.3934/mbe.2020230 |
[10] | Andrea Pugliese, Abba B. Gumel, Fabio A. Milner, Jorge X. Velasco-Hernandez . Sex-biased prevalence in infections with heterosexual, direct, and vector-mediated transmission: a theoretical analysis. Mathematical Biosciences and Engineering, 2018, 15(1): 125-140. doi: 10.3934/mbe.2018005 |
Low-light stress can inhibit plant growth and production. The selection of superior genotypes that are adaptive and tolerant to low light intensity needs to be performed. This study evaluated the agronomic responses of ten doubled haploid lines of eggplant and three varieties to various shade levels (0%, 25%, and 50%). This study used a split-plot design with three replicates. The results indicate that 25% shade is the optimal selection environment for shade tolerance. Genotypes grown under 25% shade exhibited varying responses, with two genotypes classified as shade-loving, seven as tolerant, three as moderately tolerant, and one as shade-sensitive. Shade levels of up to 50% significantly reduced yield, with production dropping to 58%–81% of the unshaded conditions. In general, growth characteristics such as plant height, leaf width, and leaf area were better under shaded conditions, whereas the yield was better under unshaded conditions. AM 23, AM 14, and AM 10 showed better tolerance responses and maintained better yields than the other lines under 25% shade. This study provides important insights into the development of shade-tolerant eggplant varieties. These findings can be used as a basis for recommendations for eggplant planting in shaded areas such as agroforestry systems.
Dengue is a vector-borne disease recognized as the major arbovirus in the world with approximately 500,000 dengue hemorrhagic fever (DHF) cases and 22,000 deaths [1,2,3]. Today, more than a third of the world's population lives in countries where dengue is endemic [4], with the dengue belt covering Central America, most of South America, sub-Saharan Africa, India, and South East Asia. Zika virus (ZIKV), a member of the Flavivirus family, was first isolated in Uganda in 1947 [5]. The first documented Zika outbreak occurred on Yap Island in the North Pacific in 2007 [6]. During 2013, a large-scale Zika outbreak was reported in French Polynesia [7]. After being spread to Brazil in 2015 [8], ZIKV was subsequently spread to other countries and territories, with more than 70 countries and territories being reported evidence of ZIKV transmission since 2007 by the end of January, 2017 [9].
Dengue can be transmitted to humans by one bite of the Aedes aegypti mosquitoes infected with one of four closely related dengue serotypes [2,10]. ZIKV is also primarily transmitted by the same mosquito species. However, ZIKV has been isolated in serum, saliva, urine, and semen [11,12,13,14], confirming the possibility of non-vector transmission (through sexual contact). The first case of ZIKV transmission via sexual contact of female to male was reported in July, 2016 [15]. Subsequently, six more cases of sexual transmission of ZIKV in the U.S. and the first case in France were diagnosed [16]. Many mathematical modelling studies [17,18,19,16,20,21,22,23,24,25,26] have investigated the impact of sexual transmission on the epidemiology of Zika, with a conclusion that Zika sexual transmission can influence the magnitude of Zika outbreaks. In 2016, Gao et al. pioneered the modelling based estimation of the impact of sexual transmission on Zika epidemiology [16]. Similarly, by assessing the basic reproduction number, the study [25] evaluated the relative role of sexual transmission. In [24], a mathematical model was conducted to quantify the Zika prevalence between a source region to an import region. Furthermore, the modelling study [27] pointed out that the risk of sustained sexual transmission of Zika is underestimated, which strongly support that Zika should be classified as sexually transmitted infection.
Since cocirculation and particularly coinfection of dengue and Zika have been documented [28,29,30], it is natural to ask how Zika sexual transmission impacts the Zika transmission dynamics, and further how it affects the dengue transmission dynamics. Our main objective of this study is to address this issue through a mathematical model. Previously, we developed a mathematical model of co-infection of dengue and Zika with a particular focus on the potential impact of vaccination against dengue for Zika outbreak [31]. In our current work, we extend the dengue-Zika coinfection dynamic model by incorporating the natural birth and death of humans. We further expand it by including the Zika sexual transmission routes among humans. To our best knowledge, our work here is the first attempt to develop a mathematical model to address how Zika sexual transmission affects the dynamics of both Zika and dengue.
The manuscript is organized as follows. In the next section, we first develop a dengue-Zika coinfection model involving the sexual transmission of Zika among humans. In section 3, we analyse the dynamics of the proposed model, including the global dynamics of the two submodels with only one disease being considered. In section 4, we discuss the impact of Zika sexual transmission on dengue endemic through cascade effect analysis. We then investigate how Zika sexual transmission affects the transmission dynamics of both dengue and Zika through numerical simulations in section 5. Finally, we make some concluding remarks and discussion in section 6.
Mosquito population Nm is divided into four classes: susceptible (Sm), infected with dengue only (Imd), infected with Zika only (Imz), and infected with dengue and Zika (Imdz). We use a SI-type of structure for the coinfection of dengue and Zika in mosquito population. Thus the model for mosquitoes is given by
{dSmdt=Λm−c(ηdId+ηzIz+ηdzIdz+ηjzJzd+ηjdJdz)SmNh−μmSm,dImddt=c(ηdId+qηdzIdz+ηjdJdz)SmNh−c(η1zIz+η1dzIdz+η1jzJzd)ImdNh−μmImd,dImzdt=c(ηzIz+(1−q)ηdzIdz+ηjzJzd)SmNh−c(η1dId+η1zdIdz+η1jdJdz)ImzNh−μmImz,dImdzdt=c(η1zIz+η1dzIdz+η1jzJzd)ImdNh+c(η1dId+η1zdIdz+η1jdJdz)ImzNh−μmImdz. | (2.1) |
Human population is stratified into: susceptible to dengue and Zika (S), dengue infected but susceptible to Zika (Id), Zika infected but susceptible to dengue (Iz), dengue and Zika co-infected (Idz), recovered from dengue and susceptible to Zika (Rd), recovered from Zika and susceptible to dengue (Rz), Zika infected but immune to dengue (Jzd), dengue infected but immune to Zika (Jdz), recovered from both dengue and Zika (Rdz). Nh=S+Id+Iz+Idz+Rd+Rz+Jzd+Jdz+Rdz denotes the total number of humans.
We assume a SIR-type model for the co-infection of dengue and Zika among humans, which gives
{dSdt=Λh−c(βdImd+βzImz+βdzImdz)SNh−βs(Iz+as1Idz+as2Jzd)SNh−μhS,dIddt=c(βdImd+pβdzImdz)SNh−β1s(Iz+a1Idz+a2Jzd)IdNh−c(β1zImz+β1dzImdz)IdNh−(γd+μh)Id,dIzdt=c(βzImz+(1−p)βdzImdz)SNh+βs(Iz+as1Idz+as2Jzd)SNh−c(β1dImd+β1zdImdz)IzNh−(γz+μh)Iz,dIdzdt=β1s(Iz+a1Idz+a2Jzd)IdNh+c(β1dImd+β1zdImdz)IzNh+c(β1zImz+β1dzImdz)IdNh−γddzIdz−γzdzIdz−μhIdz,dRddt=γdId−βrs(Iz+ar1Idz+ar2Jzd)RdNh−c(βrzImz+βrdzImdz)RdNh−μhRd,dRzdt=γzIz−c(βrdImd+βrzdImdz)RzNh−μhRz,dJzddt=βrs(Iz+ar1Idz+ar2Jzd)RdNh+c(βrzImz+βrdzImdz)RdNh−γzdJzd+γddzIdz−μhJzd,dJdzdt=c(βrdImd+βrzdImdz)RzNh−γdzJdz+γzdzIdz−μhJdz,dRdzdt=γzdJzd+γdzJdz−μhRdz. | (2.2) |
Here, βs is the sexual transmission coefficient from humans infected with Zika only (Iz) to humans who are susceptible to both viruses (S), as1 and as2 denote the relative human-to-human sexual transmissibility of humans infected with both virus (Idz) and humans with Zika infection but dengue immune (Jzd), respectively, compared with the humans infected with Zika only (Iz). Similarly, β1s is the sexual transmission coefficient from humans with Zika only (Iz) to human with dengue only (Id), with a1 and a2 being the relative human-to-human transmissibility corresponding to Idz and Jzd, respectively. βrs represents the sexual transmission coefficient from humans with Zika only (Iz) to humans recovered from dengue but susceptible to Zika (Rd), with ar1 and ar2 being the relative human-to-human transmissibility of Idz and Jzd, respectively. Λh is the constant birth rate of humans, μh is the mortality rate of humans while 1/μh can be seen as the life span of sexual activity for humans [19]. The transmission diagram is shown in Figure 1. The definitions of the variables are listed in Table 1 while the definitions and values for the other parameters of model (2.1)–(2.2) can refer to Table 2.
Variables | Definitions |
Sm | Susceptible mosquitoes |
Imd | Mosquitoes infected with dengue only |
Imz | Mosquitoes infected with Zika only |
Imdz | Mosquitoes infected with dengue and Zika |
Nh | Total number of humans |
S | Humans susceptible to dengue and Zika |
Id | Humans infected with dengue but susceptible to Zika |
Iz | Humans infected with Zika but susceptible to dengue |
Idz | Humans infected with both dengue and Zika |
Rd | Humans recovered from dengue and susceptible to Zika |
Rz | Humans recovered from Zika and susceptible to dengue |
Jzd | Humans recovered from dengue but infected with Zika |
Jdz | Humans recovered from Zika but infected with dengue |
Rdz | Humans recovered from both dengue and Zika |
Definitions | Value(range) | Reference | |
μh | Human mortality rate | 0.00014[118×365,150×365] | [19] |
Λm | Mosquito recruitment rate | 600[400,5000] | [33] |
μm | Mosquito mortality rate | 0.1[0.028,0.25] | [33,16] |
c | Mosquito biting rate | 0.7[0.3,1] | [33,16] |
βd (β1d) | Mosquito-to-human transmission probability for dengue | 0.15[0.125,0.385] | [33,16] |
βz (β1z) | Mosquito-to-human transmission probability for Zika | 0.15[0.125,0.385] | [33,16] |
βdz | Mosquito-to-human transmission probability for both | 0.15[0.03,0.75] | [33,16] |
βrd(βrz) | Dengue transmission probability from Imd(Imz) to Iz(Id) | κβd(βz) | Assumed |
β1zd(β1dz) | Dengue(Zika) transmission probability from Imdz to Iz(Id) | pβdz((1−p)βdz) | Assumed |
βrzd(βrdz) | Dengue(Zika) transmission probability from Imdz to Rz(Rd) | κpβdz((1−p)βdz) | Assumed |
ηd(η1d,η1zd,ηjd,η1jd) | Human-to-mosquito transmission probability of dengue | 0.3[0.3,0.75] | [33,16] |
ηz(η1z,η1dz,ηjz,η1jz) | Human-to-mosquito transmission probability of Zika | 0.3[0.3,0.75] | [33,16] |
ηdz | Human-to-mosquito transmission probability of both | 0.3[0.3,0.75] | [33,16] |
γd(γddz,γdz) | Recovery rate of humans infected with dengue | 0.1[0.017,0.33] | [33] |
γz(γzdz,γzd) | Recovery rate of humans infected with Zika | 0.1[0.1,0.2] | [16] |
κ | Antibody dependent enhancement factor of the susceptibility of dengue | Varied [1,3] | [34,35,36] |
p | Probability of dengue infection when S is infected with Imdz | 0.5(0, 1) | Assumed |
q | Probability of dengue infection when Sm is infected with Idz | 0.5(0, 1) | Assumed |
βs(β1s/βrs) | Sexual transmission coefficient of Zika from Iz to S(Id/Rd) | Varied [0, 1] | Assumed |
as1(a1/ar1) | Relative sexual transmissibility of Idz to S(Id/Rd) compared with Iz | 1 | Assumed |
as2(a2/ar2) | Relative sexual transmissibility of Jzd to S(Id/Rd) compared with Iz | 1 | Assumed |
Λh | Human recruitment rate | 2.2 | Assumed |
In this section, we mainly discuss the existence and stability of the disease-free equilibria for system (2.1)–(2.2). Define
D={(S,Id,Iz,Idz,Rd,Rz,Jzd,Jdz,Rdz,Sm,Imd,Imz,Imdz)∈R13+| 0<S+Id+Iz+Idz+Rd+Rz+Jzd+Jdz+Rdz≤Λhμh, 0<Sm+Imd+Imz+Imdz≤Λmμm}. |
Obviously, D is a positively invariant and attracting region in R13+ for system (2.1)–(2.2).
It is easy to see that system (2.1)–(2.2) has a disease-free equilibrium, which is given
E0=(Λhμh,0,0,0,0,0,0,0,0,Λmμm,0,0,0). |
Using the next generation matrix method introduced in papers [37,38], the basic reproduction number for system (2.1)–(2.2) is calculated as R0=max{Rd,Rsz} (see Appendix A for more details), where
Rd=√cβdμmcηdμhΛmμm(γd+μh)Λh,Rsz=Rs+√Rs2+4Rz22=βs2(γz+μh)+√β2s4(γz+μh)2+cβzμmcηzμhΛmμm(γz+μh)Λh | (3.1) |
are the dengue basic reproduction number and the Zika basic reproduction number, respectively. Here
Rs=βsγz+μh, Rz=√cβzμmcηzμhΛmμm(γz+μh)Λh | (3.2) |
are the basic reproduction numbers of sexual transmission and vectorial transmission for Zika, respectively. In summary, we have established
Theorem 3.1. The disease-free equilibrium E0 is locally asymptotically stable if R0<1 and unstable if R0>1.
Next, we will have an insight into the dynamics of the disease-free equilibrium if only one disease circulates among humans and mosquitoes. Firstly, we assume that only dengue occurs, then system (2.1)–(2.2) can be simplified to the following subsystem
{dSdt=Λh−cβdImdSNh−μhS,dIddt=cβdImdSNh−(γd+μh)Id,dSmdt=Λm−cηdIdSmNh−μmSm,dImddt=cηdIdSmNh−μmImd. | (3.3) |
For system (3.3), D1={(S,Id,Sm,Imd)∈R4+|0<S+Id≤Λhμh,0<Sm+Imd≤Λmμm} is a positively invariant and attracting region in R4+. Correspondingly, the disease-free equilibrium reduces to Ed0=(Λhμh,0,Λmμm,0), which is locally asymptotically stable when Rd<1 with Rd being given in equation (3.1). Note that Rd is also the basic reproduction number of model (3.3) [32,39]. In fact, Rd<1 can ensure that Ed0 is globally stable, that is,
Theorem 3.2. If Rd<1, the disease-free equilibrium Ed0 of system (3.3) is globally asymptotically stable in D1.
Note that, the similar models of dengue have been studied in [40,32]. We can use the same methods in these two studies to prove Theorem 3.2 (see Appendix B for details).
Performing the similar process by assuming that only Zika circulates among the humans and mosquitoes, system (2.1)–(2.2) becomes
{dSdt=Λh−cβzImzSNh−βsIzSNh−μhS,dIzdt=cβzImzSNh+βsIzSNh−(γz+μh)Iz,dSmdt=Λm−cηzIzSmNh−μmSm,dImzdt=cηzIzSmNh−μmImz. | (3.4) |
The positively invariant and attracting region should now be defined as D2={(S,Iz,Sm,Imz)∈R4+|0<S+Iz≤Λhμh,0<Sm+Imz≤Λmμm} in R4+. Also, system (3.4) has a disease-free equilibrium Ez0=(Λhμh,0,Λmμm,0). It is locally asymptotically stable if Rsz<1 and unstable if Rsz>1, here, Rsz is the basic reproduction number of system (3.4) with the formula being given in equation (3.1). The global dynamics of Ez0 can be concluded as in the following.
Theorem 3.3. If Rsz<1, the disease-free equilibrium Ez0 of system (3.4) is globally asymptotically stable in D2.
Proof. Similar to Theorem 3.2, if Rsz<1, there must exist an ε2>0 such that cηzμhΛmμm(γz+μh)Λh11−βsγz+μh<ε2<μmcβz. We introduce a function L2=ε2Iz+Imz, which satisfies L2≥0 along the solution of system (3.4) with L2=0 if and only if both Iz and Imz are zero. The derivative of L2 along the solution of system (3.4) satisfies
L′2=ε2cβzImzSNh+ε2βsIzSNh−ε2(γz+μh)Iz+cηzIzSmNh−μmImz ≤cβz(ε2−μmcβz)Imz+(γz+μh)(1−Rs)(cηzμhΛmμm(γz+μh)Λh11−βsγz+μh−ε2)Iz ≤0, |
since SNh≤1 and Sm≤Λmμm hold true in D2 and the assumptions Rs<1, cηzμhΛmμm(γz+μh)Λh11−βsγz+μh<ε2<μmcβz are satisfied. Further, L′2=0 if and only if Iz=0 and Imz=0. Therefore, according to the LaSalle's Invariance Principle, we have that Ez0 is globally asymptotically stable in D2.
In this section, we will illustrate how the Zika sexual transmission can first lead to Zika endemic, and then lead to dengue endemic through cascade effect analysis. The endemic equilibrium of Zika only model Ez(ˆS,ˆIz,ˆRz,ˆSm,ˆImz) satisfies the following conditions:
{Λh−cβzˆImzˆSNh−βsˆIzˆSNh−μhˆS=0,cβzˆImzˆSNh+βsˆIzˆSNh−(γz+μh)ˆIz=0,γzˆIz−μhˆRz=0,Λm−cηzˆSmNhˆIz−μmˆSm=0,cηzˆSmNhˆIz−μmˆImz=0. |
Solving the above equations, there are
ˆSm=ΛmNhcηzˆIz+μmNh, ˆImz=cηzΛmˆIzμm(cηzˆIz+μmNh),ˆRz=γzμzˆIz, ˆS=μmNh(γz+μh)(cηzˆIz+μmNh)cβzcηzΛm+μmβs(cηzˆIz+μmNh), |
where ˆIz is the positive root of the following equation
f(Iz)≐RsIz2+[A(Rs+Rz2)+B(1−Rs)]Iz+AB(1−Rs−Rz2)=0 |
with A=μmΛhcηzμh>0, B=Λhγz+μh>0 and Rs, Rz being given in (3.2).
Denote Δ=[A(Rs+Rz2)+B(1−Rs)]2−4ABRs(1−Rs−Rz2), then
Δ=[A(Rs+Rz2)−B(1−Rs)]2+4ABRsRz2>0. |
Through some straightforward analysis, we find that f(Iz)=0 has only one positive root given by
ˆIz=−A(Rs+Rz2)−B(1−Rs)+√Δ2Rs |
if and only if 1−Rs−Rz2<0 which is equivalent to Rsz>1. That is, the endemic equilibrium of Zika only model exits if and only if Rsz>1. Therefore, Zika can become endemic by including the sexual transmission because Rsz is increasing as βs increases, for the area that the vectorial transmission only can not (i.e. Rz<1).
We then consider the transmission dynamics of dengue after Zika reaching its endemic state. That is, we assume that only dengue circulates among humans and mosquitoes and ignore all the Zika transmission, but we set the initial conditions as the Zika endemic state. Then system (2.1)–(2.2) can be reduced to the following model:
{dSdt=Λh−cβdImdSNh−μhS,dIddt=cβdImdSNh+cβ1dImdIzNh−(γd+μh)Id,dIzdt=−cβ1dImdIzNh−(γz+μh)Iz,dRddt=γdId−μhRd,dRzdt=−cβrdImdRzNh−μhRz,dJdzdt=cβrdImdRzNh−γdzJdz−μhJdz,dRdzdt=γdzJdz−μhRdz.dSmdt=Λm−c(ηdId+ηjdJdz)SmNh−μmSm,dImddt=c(ηdId+ηjdJdz)SmNh+c(η1dId+η1jdJdz)ImzNh−μmImd,dImzdt=−c(η1dId+η1jdJdz)ImzNh−μmImz. | (4.1) |
Note that, now the disease-free equilibrium of dengue should be E∗d(ˆS,0,ˆIz,0,ˆRz,0,0,ˆSm,0,ˆImz).
In order to investigate the stability of E∗d, we also use the next generation operator. Thus, we just need to consider the compartments Id, Jdz and Imd with
F=(cβdImdSNh+cβ1dImdIzNhcβrdImdRzNhc(ηdId+ηjdJdz)SmNh+c(η1dId+η1jdJdz)ImzNh),V=((γd+μh)Id(γdz+μh)JdzμmImd). |
Therefore, we have
F=(00cβdˆSNh+cβ1dˆIzNh00cβrdˆRzNhcηdˆSmNh+cη1dˆImzNhcηjdˆSmNh+cη1jdˆImzNh0),V=(γd+μh000γdz+μh000μm). |
Through easy calculation, we then get
FV−1=(00cβdˆSμmNh+cβ1dˆIzμmNh00cβrdˆRzμmNhK1K20), |
with
K1=cηdˆSm(γd+μh)Nh+cη1dˆImz(γd+μh)Nh,K2=cηjdˆSm(γdz+μh)Nh+cη1jdˆImz(γdz+μh)Nh. |
Then by solving |λI−FV−1|=0, there is
λ(λ2−c2βrdˆRz(ηjdˆSm+η1jdˆImz)μm(γdz+μh)N2h−c2μm(γd+μh)N2h(βdˆS+β1dˆIz)(ηdˆSm+η1dˆImz))=0. |
Therefore, we can define a reproduction number of dengue with Zika being endemic which is called invasion reproduction number [41] as
R∗d=cNh√βrdˆRz(ηjdˆSm+η1jdˆImz)μm(γdz+μh)+1μm(γd+μh)(βdˆS+β1dˆIz)(ηdˆSm+η1dˆImz). |
Thus, the disease-free equilibrium of dengue E∗d is stable if R∗d<1 and unstable if R∗d>1. In other words, dengue can further become endemic after Zika being endemic if R∗d>1.
Then we discuss how the Zika sexual transmission will affect dengue transmission dynamics when Rd<1. It is easy to see that if Rsz<1, there can be ˆS=Λh/μh, ˆSm=Λm/μm, and ˆIz=ˆImz=ˆRz=0, hence R∗d=Rd. This means, Zika sexual transmission can not lead to dengue to be endemic before invoking a Zika endemic. Further, if we set βrd=β1d=βd, η1d=ηjd=η1jd=ηd and γd=γdz, there is also R∗d=Rd<1 with ˆS+ˆIz+ˆRz=Nh=Λh/μh and ˆSm+ˆImz=Λm/μm. Therefore, under this situation, the sexual transmission of Zika can not lead dengue to be endemic as well.
It is worth mentioning that the phenomenon of antibody dependent enhancement (ADE) usually occurs among the different serotypes of dengue, and between dengue and Zika. There are studies[42,43,44,45] showing that previous exposure to one dengue serotype can increase the risk of the infection by a second serotype. The similar results that plasma to dengue can enhance the infection of Zika have been reported in the clinical studies [46,47,48]. Furthermore, ADE impact between dengue and Zika is actually bidirectional [49]. Therefore, we can assume that there is the ADE of Zika for dengue infection, that is we assume that βrd>βd. Also, we let β1d=βd, η1d=ηjd=η1jd=ηd and γd=γdz. Then, we have that R∗d is increasing as ˆRz increases. Furthermore, ˆRz is increasing as βs increases (see Appendix C for more details). That is, R∗d is increasing as βs increases. This meas that Zika sexual transmission can first induce a Zika endemic, and then can a dengue endemic if there is ADE of Zika for dengue infection.
In this section, through numerical analyses, we investigate how sexual transmission of Zika affects the dynamics of both dengue and Zika. First, in order to determine the most important parameters for impacting the Zika basic reproduction number, we explored the parameter space by performing an uncertainty analysis using a Latin hypercube sampling method. We used a partial rank correlation coefficients (PRCCs) [50,51,52] to examine the sensitivity analysis for the Zika basic reproduction number with respect to all the parameters involved in Rsz. In the absence of data to inform distribution functions, we chose a uniform distribution for all input parameters. The PRCC values for Rsz is shown in Figure 2. Figure 2 shows that the first three parameters with most impact on Rsz are the mosquito mortality rate μm, the biting rate c and the mosquito recruitment rate Λm, and the sexual transmission coefficient is positively correlated to Zika basic reproduction number with a relatively large PRCCs.
As mentioned in the last section, we can assume that there is the ADE of Zika for dengue infection, and we use a parameter κ to describe the multiplication factor of the susceptibility to dengue induced by ADE. Thus, we set βrd=κβd, βrzd=κpβdz. And we assume no ADE of dengue for Zika infection with βrz=βz, βrdz=(1−p)βdz. In order to perform the simulations, we mainly let the parameters βs and κ change and fixed the other parameter values. Table 1 has given an overview of the setup of the basic parameters. The other mosquito-to-humans transmission probabilities are assumed as: β1d=βd, β1zd=pβdz, β1z=βz and β1dz=(1−p)βdz, and the rest parameter values are fixed as:
a1=a2=as1=as2=ar1=ar2=1,γd=γz=γddz=γzdz=γzd=γdz=0.1,c=0.7,μm=0.1,p=0.5,q=0.5,Λm=600,Λh=2.2,μh=0.00014,ηdz=ηjz=ηjd=η1z=η1d=η1dz=η1zd=ηjz=ηjd=ηd=ηz=0.3. |
By fixing κ=1, we plotted the solutions of system (2.1)–(2.2) with various values of βs, as shown in Figure 3. From Figure 3(A) and (C), we find that if there is no sexual transmission of Zika (i.e. βs=0), the basic reproduction number values are Rd=Rsz=0.916<1 and the disease-free equilibrium E0 is stable. When the sexual transmission of Zika is included, it can make the Zika only endemic equilibrium Ez become stable by increasing the Zika basic reproduction number above the threshold 1, as shown in Figure 3(B) and (D). The similar results can also be obtained from the simulation of Zika only model (i.e. model (3.4)), as shown in Figure 4. Furthermore, it follows from Figure 4 that the number of humans infected with Zika can stabilize at a higher level as βs increases. This means that the endemic level of Zika is increasing as Zika sexual transmission coefficient increases. In Figure 3 we fixed κ=1, that is, we assumed that there is no ADE of Zika for dengue infection. Under this scenario, sexual transmission of Zika does not have a significant effect on the dynamics of dengue while it always dies out finally.
Further, by letting κ=3, we plotted the solutions of system (2.1)–(2.2) with various values of Zika sexual transmission coefficient βs in Figure 5. It follows from Figure 5 that the disease-free equilibrium E0 is globally stable when βs=0. However, if we include the Zika sexual transmission by letting βs=0.8, then system (2.1)–(2.2) has a stable endemic equilibrium. Note that, when βs=0.8, Rd is also equal to 0.916 which is less than the threshold 1. However, as we can see from Theorem 3.2, dengue always dies out whenever Rd<1 if no Zika circulates within this area. This means that, due to the cocirculation of dengue and Zika and ADE of Zika for dengue infection, for an area where vector transmission only is not enough to make dengue and Zika be endemic, Zika sexual transmission can not only lead Zika to be endemic, but also make dengue become endemic even though the dengue basic reproduction number is less than unit.
Recently, there is increasing evidence confirming that sexual transmission of Zika occurs among humans. Several studies have investigated how Zika sexual transmission affects the dynamics of the spread of Zika via mathematical modelling. In this work, we developed a dengue-Zika coinfection model to study the impact of Zika sexual transmission on the transmission dynamics of both dengue and Zika when the two diseases cocirculate within a same area.
The basic reproduction number for both dengue and Zika, Rd and Rsz, is determined, and R0=max{Rd,Rsz}<1 is the necessary and sufficient condition for the local stability of the disease-free equilibrium E0. Theoretically, we have proved that the disease-free equilibrium for the dengue only model (Ed0) or Zika only model (Ez0) is globally stable if and only if their basic reproduction number is less than 1. Correspondingly, if the Zika (or dengue) basic reproduction number exceeds the threshold 1, then a Zika (or dengue) only endemic equilibrium for system (2.1)–(2.2) appears.
Through cascade effect analysis and numerical simulations, we try to have a further insight into the impact of Zika sexual transmission on the dynamics of dengue and Zika. The cascade effect analysis shows that Zika sexual transmission can not lead dengue to be endemic before making Zika be endemic, but it can first make Zika be endemic, and then lead dengue to be endemic if there is ADE of Zika for dengue infection. Furthermore, our sensitivity analysis shows that the first three parameters with significant impact on the Zika basic reproduction number are the Zika sexual transmission coefficient, the mortality rate of mosquitoes, and the Zika recovery rate of humans. Rsz increases if the sexual transmission route is included. As a result, the sexual transmission of Zika can lead Zika to be endemic in an area where Zika will die out if there is only the vector-borne transmission. We further found that the epidemic level of Zika increases as Zika transmission coefficient increases.
As mentioned above, when Rd<1, the disease-free equilibrium for the dengue only model is globally stable. That is, if the dengue basic reproduction number is less than 1, dengue always goes to extinction if no Zika circulates in this area. In Figure 3, we showed that Zika sexual transmission may not affect the dynamics of dengue significantly when there isn't ADE of Zika for dengue infection. However, if we assume that there is ADE of Zika for dengue infection, increasing Zika sexual transmission coefficient can make dengue and Zika be endemic simultaneously, as shown in Figure 5. Note that, here the dengue basic reproduction number is equal to 0.916 which is less than 1. In the current study, we take 1/μh as the lifespan of sexual activity for humans as we focus on the effect of sexual transmission on the dynamics of both dengue and Zika in sexual active group. The ignorance of sexual inaction group will disregard the contribution of sexual inactive people to the vector-borne transmission and hence may underestimate disease infections. To estimate the contribution of sexual inactive group on the vector-borne transmission, we plotted the solutions of model (3.4) in Figure 6 by changing the death rate and the recruitment rate of humans and fixing all the other parameters. In Figure 6, we set the lifespan of human as 35 years (taking 1/μh=35 years as the life span of sexual activity for humans) and 70 years (taking 1/μh=70 years as the natural death rate), respectively. Correspondingly, we assume that the recruitment rate of the total population (including the sexual active and inactive groups) is 1.3 or 1.6 times to the recruitment rate of the sexual active group. We obtain that the values of Iz at the endemic equilibrium are 20.16 for μh=1/(35×365),Λh=2.2, 22.43 for μh=1/(70×365),Λh=2.2×1.3, and 25.85 for μh=1/(70×365),Λh=2.2×1.3. Thus, we can estimate the contribution of the sexual inactive group to the vector-borne transmission is about 10 percent with 1.3 times recruitment rate and 22 percent for 1.6 times recruitment rate. There need further study to address this issue in more details.
Our model captures some important results that Zika sexual transmission can not only lead Zika to be endemic, but also further make dengue endemic with an assumption that there is ADE of Zika for dengue infection for the area both the diseases will die out with vectorial transmission only. This means that, due to the coinfection and ADE, increasing the transmission probability (or the endemic level) of one disease can lead to the persistent of the other disease and increase its endemic level. Note that, we set βdz=βd=βz in Figure 5. However, due to the competition on two pathogens within a host, the transmission probability of the co-infection class may be less than the probability of the classes infected of one disease. Thus, we have done further numerical experiments by setting βdz=0.01<βd=βz=0.15 and fixed all the other parameter values as those in Figure 5, and we get the similar conclusion to those shown in Figure 5. This implies that the choice of parameter βdz may not be the unique reason for inducing the interesting result. Hence a deep study will be needed to identify the key factors that promote the transmission of both pathogens when they are co-circulating within the same area, and we leave this for future study. For another aspect, ADE between dengue and Zika is usually bidirectional, and our model is easy to be modified considering this bidirectional effect. Another non-vector borne transmission, vertical transmission, has also been reported [53]. How it will affect the dynamics of both dengue and Zika is still unclear. Addressing this issue would require a further indepth research, and will be the goal of future work. In our model, we did not include the exposed classes for both humans and mosquitos, which can have a significant effect on the transmission dynamics of vector-borne diseases [16,54,55]. It was known that ignoring the extrinsic incubation period in mosquitoes may lead to overestimation of the infection risk. Hence our conclusion obtained here may overestimate the disease infection, but we hope the approaches we used for investigation of coinfection are able to be applied more generally. The higher dimensional model or delay differential equations will be formulated to model the coinfection of dengue and Zika in future study.
This project was supported by the National Natural Science Foundation of China (NSFC, 11631012, 11571273(YX)), and by the Canada Research Chair Program (230720 (JW)), the Natural Sciences and Engineering Research Council of Canada (105588-2011 (JW)).
The authors declare no conflict of interest.
Appendix A. Calculation of R0 for system (2.1)–(2.2)
The basic reproduction number is determined using the next generation operator. Considering the equations of Id,Iz,Idz,Jzd,Jdz,Imd,Imz,Imdz, we have
F=(c(βdImd+pβdzImdz)SNhc(βzImz+(1−p)βdzImdz)SNh+βs(Iz+as1Idz+as2Jzd)SNhβ1s(Iz+a1Idz+a2Jzd)IdNh+c(β1dImd+β1zdImdz)IzNh+c(β1zImz+β1dzImdz)IdNhβrs(Iz+ar1Idz+ar2Jzd)RdNh+c(βrzImz+βrdzImdz)RdNhc(βrdImd+βrzdImdz)RzNhc(ηdId+qηdzIdz+ηjdJdz)SmNhc(ηzIz+(1−q)ηdzIdz+ηjzJzd)SmNhc(η1zIz+η1dzIdz+η1jzJzd)ImdNh+c(η1dId+η1zdIdz+η1jdJdz)ImzNh) |
and
V=(β1s(Iz+a1Idz+a2Jzd)IdNh+c(β1zImz+β1dzImdz)IdNh−(γd+μh)Idc(β1dImd+β1zdImdz)IzNh+(γz+μh)Iz(γddz+γzdz+μh)Idz(γzd+μh)Jzd−γddzIdz(γdz+μh)Jdz−γzdzIdzc(η1zIz+η1dzIdz+η1jzJzd)ImdNh+μmImdc(η1dId+η1zdIdz+η1jdJdz)ImzNh+μmImzμmImdz). |
Then, we obtain
F=(00000cβd0pcβdz0βsas1βsas2βs00cβzC2βdz000000000000000000000000cηdΘ0cqηdzΘ0cηjdΘ0000cηzΘC1ηdzΘcηjzΘ000000000000) |
with Θ=μhΛmμmΛh,C1=c(1−q),C2=c(1−p), and
V=(γd+μh00000000γz+μh00000000v330000000−γddzγzd+μh000000−γzdz0γdz+μh00000000μm00000000μm00000000μm). |
with v33=γddz+γzdz+μh. Thus we have
V−1=(1γd+μh000000001γz+μh00000000v−1330000000v−1431γzd+μh000000v−15301γdz+μh000000001μm000000001μm000000001μm) |
with
v−133=1γddz+γzdz+μh,v−143=γddz(γzd+μh)(γddz+γzdz+μh),v−153=γzdz(γdz+μh)(γddz+γzdz+μh). |
Therefore,
FV−1=(00000b160b180b22b23b2400b27b28000000000000000000000000b610b630b650000b72b73b74000000000000), |
where
b16=cβdμm,b18=pcβdzμm,b22=βsγz+μh,b23=βs[as1(γzd+μh)+as2γddz](γzd+μh)(γddz+γzdz+μh), b24=as2βsγzd+μh, b27=cβzμm,b28=(1−p)cβdzμm, b61=cηdμhΛmμm(γd+μh)Λh,b63=c[qηdz(γdz+μh)+ηjdγzdz]μhΛmμm(γdz+μh)(γddz+γzdz+μh)Λh,b65=cηjdμhΛmμm(γddz+μh)Λh, b72=cηzμhΛmμm(γz+μh)Λh,b73=c[(1−q)ηdz(γzd+μh)+ηjzγddz]μhΛmμm(γzd+μh)(γddz+γzdz+μh)Λh, b74=cηjzμhΛmμm(γzd+μh)Λh. |
By simple calculating, the corresponding characteristic equation is
λ4(λ2−b16b61)(λ2−b22λ−b27b72)=0, |
from which we have that the dominant eigenvalues of FV−1 are
λ1=√b16b61=√cβdμmcηdμhΛmμm(γd+μh)Λh,λ2=b22+√b222+4b27b722=βsγz+μh+√(βsγz+μh)2+4cβzμmcηzμhΛmμm(γz+μh)Λh2. |
Therefore, the basic reproduction number for system (2.1)–(2.2) is the spectral radius of FV−1, that is R0=ρ(FV−1)=max{Rd,Rsz}, where
Rd=√cβdμmcηdμhΛmμm(γd+μh)Λh |
is the basic reproduction number of dengue, and
Rsz=Rs+√Rs2+4Rz22 |
is the basic reproduction number of Zika with
Rs=βsγz+μh and Rz=√cβzμmcηzμhΛmμm(γz+μh)Λh |
being the basic reproduction numbers of sexual transmission and vectorial transmission for Zika, respectively.
Appendix B. Proof of Theorem 3.2.
Proof. If Rd<1, which means cβdμmcηdμhΛmμm(γd+μh)Λh<1, there must exist an ε1>0 satisfying cηdμhΛmμm(γd+μh)Λh<ε1<μmcβd. Define a function L1=ε1Id+Imd. It satisfies that L1≥0 along the solution of system (3.3) with L1=0 if and only if Id and Imd are both equal to zero. The derivative of L1 along the solution of system (3.3) satisfies
L′1=ε1cβdImdSNh−ε1(γd+μh)Id+cηdIdSmNh−μmImd ≤cβd(ε1−μmcβd)Imd+(γd+μh)(cηdμhΛmμm(γd+μh)Λh−ε1)Id ≤0, |
due to the fact that SNh≤1, Sm≤Λmμm in D1 and the assumption cηdμhΛmμm(γd+μh)Λh<ε1<μmcβd. Further, we have L′1=0 if and only if Id=0 and Imd=0. Thus according to the LaSalle's Invariance Principle, we obtain that Ed0 is globally asymptotically stable in D1.
Appendix C. ˆS and ˆSm are decreasing, and ˆIz, ˆRz and ˆImz are increasing as βs increases if Rsz>1.
Regarding ˆIz as a function of βs, we can prove that it is increasing as βs increases whenever it exists. It follows from the formula of ˆIz that only Rs includes the parameter βs, and it is a monotonous increasing function of βs. Thus we just need to verify the monotonicity of ˆIz with respect to Rs instead. Calculating the derivative of ˆIz with respect to Rs, there is
dˆIzdRs=12Rs2{ARz2+B+1√Δ[(B−ARz2)(ARs+BRs+ARz2−B)−4ABRsRz2]}. |
Definitely there are ARz2+B>0 and 1√Δ>0, further if (B−ARz2)(ARs+BRs+ARz2−B)−4ABRsRz2≥0, there must be dˆIzdRs>0, which means that ˆIz is an increasing function of Rs. Otherwise, when (B−ARz2)(ARs+BRs+ARz2−B)−4ABRsRz2<0, we can verify that ARz2+B+1√Δ[(B−ARz2)(ARs+BRs+ARz2−B)−4ABRsRz2]>0 due to (ARz2+B)2Δ−[(B−ARz2)(ARs+BRs+ARz2−B)−4ABRsRz2]2=(A+B)2Rs2>0. As a conclusion, there is always that ˆIz is increasing as Rs increases, hence increasing as βs increases. Then, it follows from the relationships of ˆS, ˆRz, ˆImz and ˆSm that ˆS and ˆSm are decreasing as βs increases, and ˆRz and ˆImz are increasing as βs increases.
[1] |
Saini DK, Kaushik P (2019) Visiting eggplant from a biotechnological perspective: A review. Sci Hortic 253: 327–340. https://doi.org/10.1016/j.scienta.2019.04.042 doi: 10.1016/j.scienta.2019.04.042
![]() |
[2] |
Taher D, Solberg S, Prohens J, et al. (2017) World vegetable center eggplant collection: origin, composition, seed dissemination and utilization in breeding. Front Plant Sci 8: 1484. https://doi.org/10.3389/fpls.2017.01484 doi: 10.3389/fpls.2017.01484
![]() |
[3] | Mahanta CL, Kalita D (2020) Eggplant. In: Nutritional Composition and Antioxidant Properties of Fruits and Vegetables, 16 Eds., New York: Academic Press, 273–287. https://doi.org/10.1016/B978-0-12-812780-3.00016-7 |
[4] | Food and Agriculture Organization (2024) Food and Agriculture Data. |
[5] | Food and Agriculture Organization (2023) Land statistics and indicators 2000–2021, Italy: FAO. |
[6] | Badan Pusat Statistik (2022) Statistik Hortikultura 2022, Jakarta: Badan Pusat Statistik. |
[7] | Badan Pusat Statistik (2018) The First Indonesian Agriculture Integrated Survey (AGRIS), Badan Pusat Statistik Kabupaten Penajam Paser Utara. |
[8] |
Wang L, Liang W, Xing J, et al. (2013) Dynamics of chloroplast proteome in salt-stressed mangrove Kandelia candel (L.) Druce. J Proteome Res 12: 5124–5136. https://doi.org/10.1021/pr4006469 doi: 10.1021/pr4006469
![]() |
[9] |
Niinemets Ü, Valladares F (2004) Photosynthetic acclimation to simultaneous and interacting environmental stresses along natural light gradients: optimality and constraints. Plant Biol 6: 254–268. https://doi.org/10.1055/s-2004-817881 doi: 10.1055/s-2004-817881
![]() |
[10] |
Niinemets Ü (2007) Photosynthesis and resource distribution through plant canopies. Plant Cell Environ 30: 1052–1071. https://doi.org/10.1111/j.1365-3040.2007.01683.x doi: 10.1111/j.1365-3040.2007.01683.x
![]() |
[11] |
Laanisto L, Niinemets Ü (2015) Polytolerance to abiotic stresses: how universal is the shade–drought tolerance trade‐off in woody species? Glob Ecol Biogeogr 24: 571–580. https://doi.org/10.1111/geb.12288 doi: 10.1111/geb.12288
![]() |
[12] | Hillis DM, Sadava D, Heller HC, et al. (2012) Principles of life. USA: Sinauer Associates Inc. |
[13] |
Nguyen GN, Lantzke N, Van Burgel A (2022) Effects of shade nets on microclimatic conditions, growth, fruit yield, and quality of eggplant (Solanum melongena L.): a case study in Carnarvon, Western Australia. Horticulturae 8: 696. https://doi.org/10.3390/horticulturae8080696 doi: 10.3390/horticulturae8080696
![]() |
[14] |
Kesumawati E, Apriyatna D, Rahmawati M (2020) The effect of shading levels and varieties on the growth and yield of chili plants (Capsicum annuum L.). IOP Conf Ser Earth Environ Sci 425: 012080. https://doi.org/10.1088/1755-1315/425/1/012080 doi: 10.1088/1755-1315/425/1/012080
![]() |
[15] |
Baharuddin R, Chozin M, Syukur M (2014) Shade tolerance of 20 genotypes of tomato (Lycopersicon esculentum Mill). Indones J Agron 42: 130–135. https://doi.org/10.24831/jai.v42i2.8431 doi: 10.24831/jai.v42i2.8431
![]() |
[16] |
Putri DRM, Syukur M, Ritonga AW (2025) Variability of yield and yield components of 23 hybrid cayenne pepper (Capsicum frutescens) genotypes under shaded and unshaded conditions. Biodiversitas J Biol Divers 26: 396–406. https://doi.org/10.13057/biodiv/d260139 doi: 10.13057/biodiv/d260139
![]() |
[17] | Sopandie D (2014) Fisiologi Adaptasi Tanaman terhadap Cekaman Abiotik pada Agroekosistem Tropika, Bogor: IPB Press. |
[18] |
Kumar A, Sharma V, Jain BT, et al. (2020) Heterosis breeding in eggplant (Solanum melongena L.): gains and provocations. Plants 9: 403. https://doi.org/10.3390/plants9030403 doi: 10.3390/plants9030403
![]() |
[19] | Acquaah G (2012) Principles of Plant Genetics and Breeding, 2 Eds., New York (NY): Wiley. |
[20] |
Mir R, Calabuig-Serna A, Seguí-Simarro JM (2021) Doubled haploids in eggplant. Biology 10: 685. https://doi.org/10.3390/biology10070685 doi: 10.3390/biology10070685
![]() |
[21] |
Prigge V, Xu X, Li L, et al. (2012) New insights into the genetics of in vivo induction of maternal haploids, the backbone of doubled haploid technology in maize. Genetics 190: 781–793. https://doi.org/10.1534/genetics.111.133066 doi: 10.1534/genetics.111.133066
![]() |
[22] | Tamilzharasi M, Umamaheswari D, Umabalan J, et al. (2024) Doubled haploid rice breeding. In: Singh A, Singh SK, Shrestha J (Eds.), Climate-Smart Rice Breeding, Singapore, Springer Nature Singapore, 63–87. https://doi.org/10.1007/978-981-97-7098-4_4 |
[23] |
Boughazi K, Wuyts N, Muller O, et al. (2024) Doubled haploid lines derived from a european maize flint landrace contrast in recovery from cold stress. Agronomy 14: 408. https://doi.org/10.3390/agronomy14030408 doi: 10.3390/agronomy14030408
![]() |
[24] |
Rivas-Sendra A, Campos-Vega M, Calabuig-Serna A, et al. (2017) Development and characterization of an eggplant (Solanum melongena) doubled haploid population and a doubled haploid line with high androgenic response. Euphytica 213: 89. https://doi.org/10.1007/s10681-017-1879-325 doi: 10.1007/s10681-017-1879-325
![]() |
[25] |
Mulyana A, Purwoko BS, Dewi IS, et al. (2023) Comparison of six anther culture methods for the production of doubled haploids in eggplant (Solanum melongena L.). Euphytica 219: 44. https://doi.org/10.1007/s10681-023-03171-8 doi: 10.1007/s10681-023-03171-8
![]() |
[26] | Rotino GL, Acciarri N, Sunseri F Development of eggplant varietal resistance to insects and diseases via plant breeding. Adv Hortic Sci 11: 193–201. |
[27] | Caguiat X, Hautea D (2014) Genetic diversity analysis of eggplant (Solanum melongena L.) and related wild species in the Philippines using morphological and SSR markers. SABRAO J Breed Genet 46: 183–201. |
[28] | Kumar S, Arumugam T, Ulaganathan V (2016) Genetic diversity in eggplant germplasm by principal component analysis. SABRAO J Breed Genet 48: 167–171. |
[29] |
Niazian M, Shariatpanahi ME (2020) In vitro-based doubled haploid production: recent improvements. Euphytica 216: 69. https://doi.org/10.1007/s10681-020-02609-7 doi: 10.1007/s10681-020-02609-7
![]() |
[30] | Sabra D, Younis A, Nassar S, et al. Performance of 30 maize double haploid lines under drought stress and soil conditioners. J Chem Health Risks 14: 1199–1211. |
[31] |
Fereidooni L, Tahmasebi Z, Kahrizi D, et al. (2023) Evaluation of drought resistance of camelina (Camelina sativa L.) doubled haploid lines in the climate conditions of kermanshah province. Agrotech Ind Crops 4: 134–146. https://10.22126/atic.2023.9570.1111 doi: 10.22126/atic.2023.9570.1111
![]() |
[32] |
Al-Ashkar I, Romdhane WB, El-Said RA, et al. (2021) Agro-physiologic responses and stress-related gene expression of four doubled haploid wheat lines under salinity stress conditions. Biology 10: 56. https://doi.org/10.3390/biology10010056 doi: 10.3390/biology10010056
![]() |
[33] | Ma'arif S, Purwoko BS, Ritonga AW, et al. (2024) Study of genetic variability and shade tolerance of doubled haploid lines of eggplant (Solanum melongena L.) obtained from anther culture. Bogor Agricultural University, Faculty of Agriculture, Bogor, Indonesia, 99p. |
[34] |
Wang X, Shen L, Liu T, et al. (2022) Microclimate, yield, and income of a jujube–cotton agroforestry system in Xinjiang, China. Ind Crops Prod 182: 114941. http://doi.org/10.1016/j.indcrop.2022.114941 doi: 10.1016/j.indcrop.2022.114941
![]() |
[35] |
Yan Z, He D, Niu G, et al. (2019) Evaluation of growth and quality of hydroponic lettuce at harvest as affected by the light intensity, photoperiod and light quality at seedling stage. Sci Hortic 248: 138–144. https://doi.org/10.1016/j.scienta.2019.01.002 doi: 10.1016/j.scienta.2019.01.002
![]() |
[36] |
Fan X-X, Xu Z-G, Liu X-Y, et al. (2013) Effects of light intensity on the growth and leaf development of young tomato plants grown under a combination of red and blue light. Sci Hortic 153: 50–55. https://doi.org/10.1016/j.scienta.2013.01.017 doi: 10.1016/j.scienta.2013.01.017
![]() |
[37] | Kalmatskaya OA, Karavaev VA, Tikhonov AN (2019) Induction of chlorophyll fluorescence in Tradescantia leaves acclimated to growth light of different intensities. In: Tuchin VV, Genina EA (Eds.), Saratov Fall Meeting 2018: Optical and Nano-Technologies for Biology and Medicine, Saratov, Russian Federation, SPIE, 72. https://doi.org/10.1117/12.2523333 |
[38] |
Ding J, Zhao J, Pan T, et al. (2019) Comparative transcriptome analysis of gene expression patterns in tomato under dynamic light conditions. Genes 10: 662. https://doi.org.10.3390/genes10090662 doi: 10.3390/genes10090662
![]() |
[39] | Vialet-Chabrand S, Matthews JSA, Simkin AJ, et al. (2017) Importance of fluctuations in light on plant photosynthetic acclimation. Plant Physiol 173: 2163–2179. |
[40] | Prabhu M, Natarajan S, Veeraragavathatham D (2008) Correlation and path coefficient analysis in eggplant (Solanum melongena L.). Indian J Agric Res 42: 232–234. |
[41] |
Khalid MHB, Raza MA, Yu HQ, et al. (2019) Effect of shade treatments on morphology, photosynthetic and chlorophyll fluorescence characteristics of soybeans (Glycine max L. Merr.). Appl Ecol Environ Res 17: 2551–2569. https://doi.org/10.15666/aeer/1702_25512569 doi: 10.15666/aeer/1702_25512569
![]() |
[42] |
Ma L, Li G (2019) Auxin-dependent cell elongation during the shade avoidance response. Front Plant Sci 10: 914. https://doi.org/10.3389/fpls.2019.00914 doi: 10.3389/fpls.2019.00914
![]() |
[43] |
Jiang Y, Ding X, Wang J, et al. (2021) Decreased low-light regulates plant morphogenesis through the manipulation of hormone biosynthesis in Solanum lycopersicum. Environ Exp Bot 185: 104409. https://doi.org/10.1016/j.envexpbot.2021.104409 doi: 10.1016/j.envexpbot.2021.104409
![]() |
[44] |
Frébortová J, Frébort I (2021) Biochemical and structural aspects of cytokinin biosynthesis and degradation in bacteria. Microorganisms 9: 1314. https://doi.org/10.3390/microorganisms9061314 doi: 10.3390/microorganisms9061314
![]() |
[45] |
Hussain HA, Men S, Hussain S, et al. (2019) Interactive effects of drought and heat stresses on morpho-physiological attributes, yield, nutrient uptake and oxidative status in maize hybrids. Sci Rep 9: 3890. https://doi.org/10.1038/s41598-019-40362-7 doi: 10.1038/s41598-019-40362-7
![]() |
[46] |
Wu Y, Gong W, Yang W (2017) Shade inhibits leaf size by controlling cell proliferation and enlargement in soybean. Sci Rep 7: 9259. https://doi.org/10.1038/s41598-017-10026-5 doi: 10.1038/s41598-017-10026-5
![]() |
[47] |
Zhang H, Cao Y, Wang Z, et al. (2023) Functional mapping of genes modulating plant shade avoidance using leaf traits. Plants 12: 608. https://doi.org/10.3390/plants12030608 doi: 10.3390/plants12030608
![]() |
[48] |
Ren B, Zhang J, Dong S, et al. (2017) Regulations of 6-Benzyladenine (6-BA) on leaf ultrastructure and photosynthetic characteristics of waterlogged summer maize. J Plant Growth Regul 36: 743–754. https://doi.org/10.1007/s00344-017-9677-7 doi: 10.1007/s00344-017-9677-7
![]() |
[49] |
Ilić ZS, Fallik E (2017) Light quality manipulation improves vegetable quality at harvest and postharvest: A review. Environ Exp Bot 139: 79–90. https://doi.org/10.1016/j.envexpbot.2017.04.006 doi: 10.1016/j.envexpbot.2017.04.006
![]() |
[50] | Sunaryanti D, Chozin M, Aziz S (2018) Growth analysis and physiological characteristics of several tomato genotipes under the low light intensity. J ISSAAS 24: 129–140. |
[51] |
Panigrahy M, Ranga A, Das J, et al. (2019) Shade tolerance in Swarnaprabha rice is associated with higher rate of panicle emergence and positively regulated by genes of ethylene and cytokinin pathway. Sci Rep 9: 6817. https://doi.org/10.1038/s41598-019-43096-8 doi: 10.1038/s41598-019-43096-8
![]() |
[52] |
Munir M, Jamil M, Baloch J, et al. (2004) Impact of light intensity on flowering time and plant quality of Antirrhinum majus L. cultivar Chimes White. J Zhejiang Univ-Sci A 5: 400–405. https://doi.org/10.1631/jzus.2004.0400 doi: 10.1631/jzus.2004.0400
![]() |
[53] |
Faust JE, Holcombe V, Rajapakse NC, et al. (2005) The effect of daily light integral on bedding plant growth and flowering. Hort Science 40: 645–649. https://doi.org/10.21273/hortsci.40.3.645 doi: 10.21273/hortsci.40.3.645
![]() |
[54] |
Cober ER, Voldeng HD (2001) Low R:FR light quality delays flowering of E7E7 soybean lines. Crop Sci 41: 1823–1826. https://doi.org/10.2135/cropsci2001.1823 doi: 10.2135/cropsci2001.1823
![]() |
[55] |
Qin F, Shen Y, Li Z, et al. (2022) Shade delayed flowering phenology and decreased reproductive growth of Medicago sativa L. Front Plant Sci 13: 835380. https://doi.org/10.3389/fpls.2022.835380 doi: 10.3389/fpls.2022.835380
![]() |
[56] |
Stagnari F, Ficcadenti N, Manetta AC, et al. (2022) Modulation of light and nitrogen for quality-traits improvement: a case study of altino sweet pepper. Horticulturae 8: 499. https://doi.org/10.3390/horticulturae8060499 doi: 10.3390/horticulturae8060499
![]() |
[57] |
Hope E, Carins‐Murphy MR, Hudson C, et al. (2020) Opium poppy capsule growth and alkaloid production is constrained by shade during early floral development. Ann Appl Biol 176: 296–307. https://doi.org/10.1111/aab.12581 doi: 10.1111/aab.12581
![]() |
[58] |
Choi HG (2021) Correlation among phenotypic parameters related to the growth and photosynthesis of strawberry (Fragaria×ananassa Duch.) grown under various light intensity conditions. Front Plant Sci 12: 647585. https://doi.org/10.3389/fpls.2021.647585 doi: 10.3389/fpls.2021.647585
![]() |
[59] | Solankey SS, Kumari M, Akhtar S, et al. (2021) Challenges and Opportunities in Vegetable Production in Changing Climate: Mitigation and Adaptation Strategies, In: Solankey SS, Kumari M, Kumar M (Eds.), Advances in Research on Vegetable Production Under a Changing Climate, Cham: Springer International Publishing, 13–59. https://doi.org/10.1007/978-3-030-63497-1_2 |
[60] |
Leonardi C, Guichard S, Bertin N (2000) High vapour pressure deficit influences growth, transpiration and quality of tomato fruits. Sci Hortic 84: 285–296. https://doi.org/10.1016/s0304-4238(99)00127-2 doi: 10.1016/s0304-4238(99)00127-2
![]() |
[61] |
Yang F, Liu Q, Cheng Y, et al. (2020) Low red/far-red ratio as a signal promotes carbon assimilation of soybean seedlings by increasing the photosynthetic capacity. BMC Plant Biol 20: 148. https://doi.org/10.1186/s12870-020-02352-0 doi: 10.1186/s12870-020-02352-0
![]() |
[62] |
Touil S, Richa A, Fizir M, et al. (2021) Shading effect of photovoltaic panels on horticulture crops production: A mini review. Rev Environ Sci Biotechnol 20: 281–296. https://doi.org/10.1007/s11157-021-09572-2 doi: 10.1007/s11157-021-09572-2
![]() |
[63] |
Laub M, Pataczek L, Feuerbacher A, et al. (2022) Contrasting yield responses at varying levels of shade suggest different suitability of crops for dual land-use systems: a meta-analysis. Agron Sustain Dev 42: 51. https://doi.org/10.1007/s13593-022-00783-7 doi: 10.1007/s13593-022-00783-7
![]() |
[64] |
Susanti ED, Chozin MA, Ritonga AW, et al. (2023) Identification of morpho-physiological and yield traits of sweet corn hybrids at various shade levels. Caraka Tani J Sustain Agric 38: 327. http://doi.org/10.20961/carakatani.v38i2.73567 doi: 10.20961/carakatani.v38i2.73567
![]() |
[65] |
Xie X, Cheng H, Hou C, et al. (2022) Integration of light and auxin signaling in shade plants: from mechanisms to opportunities in urban agriculture. Int J Mol Sci 23: 3422. http://doi.org/10.3390/ijms23073422 doi: 10.3390/ijms23073422
![]() |
[66] |
Liu W, Tollenaar M (2009) Physiological mechanisms underlying heterosis for shade tolerance in maize. Crop Sci 49: 1817–1826. http://doi.org/10.2135/cropsci2008.07.0423 doi: 10.2135/cropsci2008.07.0423
![]() |
[67] |
Shifriss C, Pilowsky M, Aloni B (1994) Variation in flower abscission of peppers under stress shading conditions. Euphytica 78: 133–136. http://doi.org/10.1007/bf00021408 doi: 10.1007/bf00021408
![]() |
[68] | Shehata S, Elsagheer A, El-Helaly M, et al. (2013) Shading effect on vegetative and fruit characters of tomato plant. J Appl Sci Res 9: 1434–1437. |
[69] |
Otoni BDS, Mota WFD, Belfort GR, et al. (2012) Produção de híbridos de tomateiro cultivados sob diferentes porcentagens de sombreamento. Rev Ceres 59: 816–825. https://doi.org/10.1590/s0034-737x2012000600012 doi: 10.1590/s0034-737x2012000600012
![]() |
[70] |
Masabni J, Sun Y, Niu G, et al. (2016) Shade effect on growth and productivity of tomato and chili pepper. HortTechnology 26: 344–350. https://doi.org/10.21273/HORTTECH.26.3.344 doi: 10.21273/HORTTECH.26.3.344
![]() |
[71] |
Siahaan GF, Muhamad Achmad Chozin, Muhamad Syukur, et al. (2022) Perbedaan respon pertumbuhan, fisiologi dan produksi 20 genotipe cabai rawit terhadap berbagai tingkat naungan. J Agron Indones Indones J Agron 50: 73–79. https://doi.org/10.24831/jai.v50i1.38832 doi: 10.24831/jai.v50i1.38832
![]() |
1. | Ibrahim M. Hezam, Abdelaziz Foul, Adel Alrasheedi, A dynamic optimal control model for COVID-19 and cholera co-infection in Yemen, 2021, 2021, 1687-1847, 10.1186/s13662-021-03271-6 | |
2. | Laura Atzori, Caterina Ferreli, Valeria Mateeva, Snejina Vassileva, Franco Rongioletti, Clinico-pathologic features between different viral epidemic outbreaks involving the skin, 2021, 0738081X, 10.1016/j.clindermatol.2021.04.002 | |
3. | Xiaoyan Yuan, Yijun Lou, Daihai He, Jinliang Wang, Daozhou Gao, A Zika Endemic Model for the Contribution of Multiple Transmission Routes, 2021, 83, 0092-8240, 10.1007/s11538-021-00945-w | |
4. | James W. Mungin, Xin Chen, Bindong Liu, Interferon Epsilon Signaling Confers Attenuated Zika Replication in Human Vaginal Epithelial Cells, 2022, 11, 2076-0817, 853, 10.3390/pathogens11080853 | |
5. | Laura Atzori, Caterina Ferreli, Valeria Mateeva, Snejina Vassileva, Franco Rongioletti, Clinicopathologic features among different viral epidemic outbreaks involving the skin, 2022, 40, 0738081X, 573, 10.1016/j.clindermatol.2021.06.003 | |
6. | Weike Zhou, Biao Tang, Yao Bai, Yiming Shao, Yanni Xiao, Sanyi Tang, The resurgence risk of COVID-19 in China in the presence of immunity waning and ADE: A mathematical modelling study, 2022, 40, 0264410X, 7141, 10.1016/j.vaccine.2022.10.043 | |
7. | Wafa F. Alfwzan, Ali Raza, Jesus Martin-Vaquero, Dumitru Baleanu, Muhammad Rafiq, Nauman Ahmed, Zafar Iqbal, Modeling and transmission dynamics of Zika virus through efficient numerical method, 2023, 13, 2158-3226, 10.1063/5.0168945 | |
8. | Jiwei Jia, Jian Ding, Siyu Liu, Guidong Liao, Jingzhi Li, Ben Duan, Guoqing Wang, Ran Zhang, Modeling the control of COVID-19: impact of policy interventions and meteorological factors, 2020, 2020, 1072-6691, 23, 10.58997/ejde.2020.23 | |
9. | Jhoana P. Romero-Leiton, Elda K.E. Laison, Rowin Alfaro, E. Jane Parmley, Julien Arino, Kamal R. Acharya, Bouchra Nasri, Exploring Zika's Dynamics: A Scoping Review Journey from Epidemic to Equations Through Mathematical Modelling, 2024, 24680427, 10.1016/j.idm.2024.12.016 |
Variables | Definitions |
Sm | Susceptible mosquitoes |
Imd | Mosquitoes infected with dengue only |
Imz | Mosquitoes infected with Zika only |
Imdz | Mosquitoes infected with dengue and Zika |
Nh | Total number of humans |
S | Humans susceptible to dengue and Zika |
Id | Humans infected with dengue but susceptible to Zika |
Iz | Humans infected with Zika but susceptible to dengue |
Idz | Humans infected with both dengue and Zika |
Rd | Humans recovered from dengue and susceptible to Zika |
Rz | Humans recovered from Zika and susceptible to dengue |
Jzd | Humans recovered from dengue but infected with Zika |
Jdz | Humans recovered from Zika but infected with dengue |
Rdz | Humans recovered from both dengue and Zika |
Definitions | Value(range) | Reference | |
μh | Human mortality rate | 0.00014[118×365,150×365] | [19] |
Λm | Mosquito recruitment rate | 600[400,5000] | [33] |
μm | Mosquito mortality rate | 0.1[0.028,0.25] | [33,16] |
c | Mosquito biting rate | 0.7[0.3,1] | [33,16] |
βd (β1d) | Mosquito-to-human transmission probability for dengue | 0.15[0.125,0.385] | [33,16] |
βz (β1z) | Mosquito-to-human transmission probability for Zika | 0.15[0.125,0.385] | [33,16] |
βdz | Mosquito-to-human transmission probability for both | 0.15[0.03,0.75] | [33,16] |
βrd(βrz) | Dengue transmission probability from Imd(Imz) to Iz(Id) | κβd(βz) | Assumed |
β1zd(β1dz) | Dengue(Zika) transmission probability from Imdz to Iz(Id) | pβdz((1−p)βdz) | Assumed |
βrzd(βrdz) | Dengue(Zika) transmission probability from Imdz to Rz(Rd) | κpβdz((1−p)βdz) | Assumed |
ηd(η1d,η1zd,ηjd,η1jd) | Human-to-mosquito transmission probability of dengue | 0.3[0.3,0.75] | [33,16] |
ηz(η1z,η1dz,ηjz,η1jz) | Human-to-mosquito transmission probability of Zika | 0.3[0.3,0.75] | [33,16] |
ηdz | Human-to-mosquito transmission probability of both | 0.3[0.3,0.75] | [33,16] |
γd(γddz,γdz) | Recovery rate of humans infected with dengue | 0.1[0.017,0.33] | [33] |
γz(γzdz,γzd) | Recovery rate of humans infected with Zika | 0.1[0.1,0.2] | [16] |
κ | Antibody dependent enhancement factor of the susceptibility of dengue | Varied [1,3] | [34,35,36] |
p | Probability of dengue infection when S is infected with Imdz | 0.5(0, 1) | Assumed |
q | Probability of dengue infection when Sm is infected with Idz | 0.5(0, 1) | Assumed |
βs(β1s/βrs) | Sexual transmission coefficient of Zika from Iz to S(Id/Rd) | Varied [0, 1] | Assumed |
as1(a1/ar1) | Relative sexual transmissibility of Idz to S(Id/Rd) compared with Iz | 1 | Assumed |
as2(a2/ar2) | Relative sexual transmissibility of Jzd to S(Id/Rd) compared with Iz | 1 | Assumed |
Λh | Human recruitment rate | 2.2 | Assumed |
Variables | Definitions |
Sm | Susceptible mosquitoes |
Imd | Mosquitoes infected with dengue only |
Imz | Mosquitoes infected with Zika only |
Imdz | Mosquitoes infected with dengue and Zika |
Nh | Total number of humans |
S | Humans susceptible to dengue and Zika |
Id | Humans infected with dengue but susceptible to Zika |
Iz | Humans infected with Zika but susceptible to dengue |
Idz | Humans infected with both dengue and Zika |
Rd | Humans recovered from dengue and susceptible to Zika |
Rz | Humans recovered from Zika and susceptible to dengue |
Jzd | Humans recovered from dengue but infected with Zika |
Jdz | Humans recovered from Zika but infected with dengue |
Rdz | Humans recovered from both dengue and Zika |
Definitions | Value(range) | Reference | |
μh | Human mortality rate | 0.00014[118×365,150×365] | [19] |
Λm | Mosquito recruitment rate | 600[400,5000] | [33] |
μm | Mosquito mortality rate | 0.1[0.028,0.25] | [33,16] |
c | Mosquito biting rate | 0.7[0.3,1] | [33,16] |
βd (β1d) | Mosquito-to-human transmission probability for dengue | 0.15[0.125,0.385] | [33,16] |
βz (β1z) | Mosquito-to-human transmission probability for Zika | 0.15[0.125,0.385] | [33,16] |
βdz | Mosquito-to-human transmission probability for both | 0.15[0.03,0.75] | [33,16] |
βrd(βrz) | Dengue transmission probability from Imd(Imz) to Iz(Id) | κβd(βz) | Assumed |
β1zd(β1dz) | Dengue(Zika) transmission probability from Imdz to Iz(Id) | pβdz((1−p)βdz) | Assumed |
βrzd(βrdz) | Dengue(Zika) transmission probability from Imdz to Rz(Rd) | κpβdz((1−p)βdz) | Assumed |
ηd(η1d,η1zd,ηjd,η1jd) | Human-to-mosquito transmission probability of dengue | 0.3[0.3,0.75] | [33,16] |
ηz(η1z,η1dz,ηjz,η1jz) | Human-to-mosquito transmission probability of Zika | 0.3[0.3,0.75] | [33,16] |
ηdz | Human-to-mosquito transmission probability of both | 0.3[0.3,0.75] | [33,16] |
γd(γddz,γdz) | Recovery rate of humans infected with dengue | 0.1[0.017,0.33] | [33] |
γz(γzdz,γzd) | Recovery rate of humans infected with Zika | 0.1[0.1,0.2] | [16] |
κ | Antibody dependent enhancement factor of the susceptibility of dengue | Varied [1,3] | [34,35,36] |
p | Probability of dengue infection when S is infected with Imdz | 0.5(0, 1) | Assumed |
q | Probability of dengue infection when Sm is infected with Idz | 0.5(0, 1) | Assumed |
βs(β1s/βrs) | Sexual transmission coefficient of Zika from Iz to S(Id/Rd) | Varied [0, 1] | Assumed |
as1(a1/ar1) | Relative sexual transmissibility of Idz to S(Id/Rd) compared with Iz | 1 | Assumed |
as2(a2/ar2) | Relative sexual transmissibility of Jzd to S(Id/Rd) compared with Iz | 1 | Assumed |
Λh | Human recruitment rate | 2.2 | Assumed |