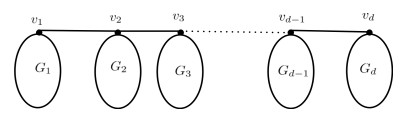
The use of synthetic data could facilitate data-driven innovation across industries and applications. Synthetic data can be generated using a range of methods, from statistical modeling to machine learning and generative AI, resulting in datasets of different formats and utility. In the health sector, the use of synthetic data is often motivated by privacy concerns. As generative AI is becoming an everyday tool, there is a need for practice-oriented insights into the prospects and limitations of synthetic data, especially in the privacy sensitive domains. We present an interdisciplinary outlook on the topic, focusing on, but not limited to, the Finnish regulatory context. First, we emphasize the need for working definitions to avoid misplaced assumptions. Second, we consider use cases for synthetic data, viewing it as a helpful tool for experimentation, decision-making, and building data literacy. Yet the complementary uses of synthetic datasets should not diminish the continued efforts to collect and share high-quality real-world data. Third, we discuss how privacy-preserving synthetic datasets fall into the existing data protection frameworks. Neither the process of synthetic data generation nor synthetic datasets are automatically exempt from the regulatory obligations concerning personal data. Finally, we explore the future research directions for generating synthetic data and conclude by discussing potential future developments at the societal level.
Citation: Tinja Pitkämäki, Tapio Pahikkala, Ileana Montoya Perez, Parisa Movahedi, Valtteri Nieminen, Tom Southerington, Juho Vaiste, Mojtaba Jafaritadi, Muhammad Irfan Khan, Elina Kontio, Pertti Ranttila, Juha Pajula, Harri Pölönen, Aysen Degerli, Johan Plomp, Antti Airola. Finnish perspective on using synthetic health data to protect privacy: the PRIVASA project[J]. Applied Computing and Intelligence, 2024, 4(2): 138-163. doi: 10.3934/aci.2024009
[1] | Ali Al Khabyah . Mathematical aspects and topological properties of two chemical networks. AIMS Mathematics, 2023, 8(2): 4666-4681. doi: 10.3934/math.2023230 |
[2] | Zhibin Du, Ayu Ameliatul Shahilah Ahmad Jamri, Roslan Hasni, Doost Ali Mojdeh . Maximal first Zagreb index of trees with given Roman domination number. AIMS Mathematics, 2022, 7(7): 11801-11812. doi: 10.3934/math.2022658 |
[3] | Zeeshan Saleem Mufti, Ali Tabraiz, Qin Xin, Bander Almutairi, Rukhshanda Anjum . Fuzzy topological analysis of pizza graph. AIMS Mathematics, 2023, 8(6): 12841-12856. doi: 10.3934/math.2023647 |
[4] | Ali N. A. Koam, Ali Ahmad, Azeem Haider, Moin A. Ansari . Computation of eccentric topological indices of zero-divisor graphs based on their edges. AIMS Mathematics, 2022, 7(7): 11509-11518. doi: 10.3934/math.2022641 |
[5] | Muhammad Kamran Jamil, Muhammad Imran, Aisha Javed, Roslan Hasni . On the first general Zagreb eccentricity index. AIMS Mathematics, 2021, 6(1): 532-542. doi: 10.3934/math.2021032 |
[6] | Muhammad Umar Mirza, Rukhshanda Anjum, Maged Z. Youssef, Turki Alsuraiheed . A comprehensive study on fuzzy and crisp graph indices: generalized formulae, proximity and accuracy analysis. AIMS Mathematics, 2023, 8(12): 30922-30939. doi: 10.3934/math.20231582 |
[7] | Edil D. Molina, José M. Rodríguez-García, José M. Sigarreta, Sergio J. Torralbas Fitz . On the Gutman-Milovanović index and chemical applications. AIMS Mathematics, 2025, 10(2): 1998-2020. doi: 10.3934/math.2025094 |
[8] | Zhen Lin . The biharmonic index of connected graphs. AIMS Mathematics, 2022, 7(4): 6050-6065. doi: 10.3934/math.2022337 |
[9] | Chenxu Yang, Meng Ji, Kinkar Chandra Das, Yaping Mao . Extreme graphs on the Sombor indices. AIMS Mathematics, 2022, 7(10): 19126-19146. doi: 10.3934/math.20221050 |
[10] | Milica Anđelić, Tamara Koledin, Zoran Stanić . Notes on Hamiltonian threshold and chain graphs. AIMS Mathematics, 2021, 6(5): 5078-5087. doi: 10.3934/math.2021300 |
The use of synthetic data could facilitate data-driven innovation across industries and applications. Synthetic data can be generated using a range of methods, from statistical modeling to machine learning and generative AI, resulting in datasets of different formats and utility. In the health sector, the use of synthetic data is often motivated by privacy concerns. As generative AI is becoming an everyday tool, there is a need for practice-oriented insights into the prospects and limitations of synthetic data, especially in the privacy sensitive domains. We present an interdisciplinary outlook on the topic, focusing on, but not limited to, the Finnish regulatory context. First, we emphasize the need for working definitions to avoid misplaced assumptions. Second, we consider use cases for synthetic data, viewing it as a helpful tool for experimentation, decision-making, and building data literacy. Yet the complementary uses of synthetic datasets should not diminish the continued efforts to collect and share high-quality real-world data. Third, we discuss how privacy-preserving synthetic datasets fall into the existing data protection frameworks. Neither the process of synthetic data generation nor synthetic datasets are automatically exempt from the regulatory obligations concerning personal data. Finally, we explore the future research directions for generating synthetic data and conclude by discussing potential future developments at the societal level.
A molecular graph in chemical graph theory is the graphical representation of the structural formula of a chemical compound in which the vertices represent atoms and edges represent chemical bond between those atoms. A topological index of a molecular graph G is a real number which characterizes the topology of G. Also it is invariant under graph automorphism. Topological indices have been widely used in Quantitative Structure-Activity Relationship (QSAR) and Quantitative Structure-Property Relationship (QSPR) studies. It has application in many folds, to name a few areas, biochemistry, nanotechnology, pharmacology. Bond energy is a measure of bond strength of a chemical compound. The distance between two atoms is considered as the bond length between them. The higher the bond energy, the smaller is the bond length between those atoms. The recently introduced 2-degree based topological invariants, analogous to novel graph invariants (Zagreb indices), namely leap Zagreb indices, may be applied in studying such bond energy between atoms in a molecular graph of a chemical compound.
Throughout this paper, G=(V,E) represents a connected molecular graph with the vertex set V(G) and the edge set E(G). Let the number of vertices and edges of G be n and m respectively. The degree of a vertex v in G is the number of vertices adjacent to v in G and denoted by deg(v:G). The 2-degree (or the second-degree) of a vertex v in G is the number of vertices which are at distance two from v in G and denoted by d2(v:G). The Zagreb indices, namely, the first and second Zagreb indices, are the most important and oldest molecular structure descriptors. These indices have been studied extensively in the field of Mathematical Chemistry [3,4,5]. Recently, the concept of Forgotten topological index also known as F-index have attracted many researchers which results in over 100 research articles related to F-index. A.M.Naji et al. [13] have recently introduced and studied some properties of a new topological invariant called Leap Zagreb indices. They are defined as follows:
Definition 1. (ⅰ) The first leap Zagreb index LM1(G) of a graph G is equal to the sum of squares of the second degrees of the vertices, LM1(G)=∑u∈V(G)d2(u)2.
(ⅱ) The second leap Zagreb index LM2(G) of a graph G is equal to the sum of the products of the second degrees of pairs of adjacent vertices, LM2(G)=∑uv∈E(G)d2(u)d2(v).
(ⅲ) The third leap Zagreb index LM3(G) of a graph G is equal to the sum of the products of the degree with the second degree of every vertex in G, LM3(G)=∑u∈V(G)deg(u)d2(u)
Subsequently, Z. Shao et al. [18] generalized the results of Naji et al.[13] for trees and unicyclic graphs and determined upper and lower bounds on leap Zagreb indices and characterized extremal graphs. Basavanagoud et al.[2] computed exact values for first and second leap hyper Zagreb indices of some nano structures. V. R. Kulli [7,8,9] introduced and studied various leap indices. Shiladhar et al.[17] computed leap Zagreb indices of wind mill graphs. Most recently, Naji et al.[14] have studied some properties of leap graphs.
Azari et al.[1] found formulae for first and second Zagreb indices of bridge and chain graphs. Nilanjan De [15,16] computed F-index and hyper Zagreb index of bridge and chain graphs. Jerline et al. [6] obtained exact values for harmonic index of bridge and chain graphs. E. Litta et al. [10] worked on modified Zagreb indices of bridge graphs. Mohanad Ali et al. [11] computed F-leap index of some special classes of bridge and chain graphs. Zhang et al.[12] worked on Edge-Version Atom-Bond Connectivity and Geometric Arithmetic Indices of generalized bridge molecular graphs. Motivated by their results, we compute exact values for the first and third leap Zagreb indices of bridges and chain graphs. Also we discuss some applications related to these indices in the last section of this paper. First, we recall the definitions of bridge and chain graphs from [1] as follows:
Definition 2. Let {Gi}di=1 be a set of finite pairwise disjoint graphs with distinct vertices vi∈V(Gi). The bridge graph B1=B1(G1,G2,…,Gd;v1,v2,v3,…,vd) of {Gi}di=1 with respect to the vertices {vi}di=1 as shown in Figure 1, is the graph obtained from the graphs G1,G2,…,Gd by connecting the vertices vi and vi+1 by an edge for all i=1,2,…,d−1.
Definition 3. The bridge graph B2=B2(G1,G2,…,Gd;v1,w1,v2,w2,…,vd,wd) of {Gi}di=1 with respect to the vertices {vi,wi}di=1 as shown in Figure 2, is the graph obtained from the graphs G1,G2,G3,…,Gd by connecting the vertices wi and vi+1 by an edge for all i=1,2,…,d−1.
Definition 4. The chain graph C=C(G1,G2,…,Gd;v1,w1,v2,w2,…,vd,wd) of {Gi}di=1 with respect to the vertices {vi,wi}di=1 as shown in Figure 3, is the graph obtained from the graphs G1,G2,…,Gd by identifying the vertices wi and vi+1 for all i=1,2,…,d−1.
The following lemma gives the 2-degree of any arbitrary vertex in the bridge graph B1.
Lemma 5. Let G1,G2,⋯,Gd be d≥5 connected graphs. Then the 2-degree of any arbitrary vertex u in the bridge graph B1 formed by these graphs is as follows:
d2(u:B1)={ν1+μ2+1,ifu=v1νd+μd−1+1,ifu=vdν2+μ1+μ3+1,ifu=v2νd−1+μd+μd−2+1,ifu=vd−1νi+μi−1+μi+1+2,,ifu=vi,3≤i≤d−2d2(u:G1)+1,ifu∈NG1(v1)d2(u:Gd)+1,ifu∈NGd(vd)d2(u:Gi)+2,ifu∈NGi(vi),2≤i≤d−1d2(u:Gi),ifu∈V(Gi)∖NGi[vi],1≤i≤d, | (2.1) |
where νi=d2(vi:Gi) and μi=deg(vi:Gi),1≤i≤d.
Next, we compute the first leap Zagreb index of the type-Ⅰ bridge graph B1.
Let Si=∑u∈NGi(vi)d2(u:Gi), 1≤i≤d.
Theorem 6. LM1(B1)=d∑i=1LM1(Gi)+d−1∑i=2[(μi−1+μi+1+1)2+2νi(μi−1+μi+1+1)+4Si+8μi]+2d−2∑i=3νi+2(S1+Sd)+(μ1+μd−2μ3−2μd−2)+(μ2+1)(μ2+2ν1+1)+(μd−1+1)(μd−1+2νd+1)+3d−12.
Proof. By virtue of Lemma 5
LM1(B1)=∑u∈V(B1)d2(u:B1)2=(ν1+μ2+1)2+(νd+μd−1+1)2+(ν2+μ1+μ3+1)2+(νd−1+μd+μd−2+1)2+d−2∑i=3(νi+μi−1+μi+1+2)2+∑u∈NG1(v1)(d2(u:G1)+1)2+∑u∈NGd(vd)(d2(u:Gd)+1)2+d−1∑i=2∑u∈NGi(vi)(d2(u:Gi)+2)2+d∑i=1∑u∈V(Gi)∖NGi[vi]d2(u:Gi)2 |
=ν21+(μ2+1)2+2ν1(μ2+1)+ν2d+(μd−1+1)2+2νd(μd−1+1)+ν22+(μ1+μ3+1)2+2ν2(μ1+μ3+1)+ν2d−1+(μd+μd−2+1)2+2νd−1(μd+μd−2+1)+d−2∑i=3[(νi+1)2+2(νi+1)(μi−1+μi+1+1)+(μi−1+μi+1+1)2]+∑u∈NG1(v1)[d2(u:G1)2+2d2(u:G1)]+μ1+∑u∈NGd(vd)[d2(u:Gd)2+2d2(u:Gd)]+μd+d−1∑i=2∑u∈NGi(vi)[d2(u:Gi)2+4d2(u:Gi)]+4d−1∑i=2μi+d∑i=1∑u∈V(Gi)∖NGi[vi]d2(u:Gi)2 |
=d∑i=1LM1(Gi)+d−1∑i=2[(μi−1+μi+1+1)2+2νi(μi−1+μi+1+1)+4Si+8μi]+2d−2∑i=3νi+2(S1+Sd)+(μ1+μd−2μ2−2μ3−2μd−2−2μd−1)+(μ2+1)(μ2+2ν1+1)+(μd−1+1)(μd−1+2νd+1)+3d−12. |
Thus the result follows.
Corollary 7. If G1=G2=⋯=Gd=G in a bridge graph B1, then LM1(B1)=dLM1(G)+(4d−6)μ2+(4d−8)ν+(12d−26)μ+(4d−4)(νμ+S)+4d−12, where S=∑u∈NG(v)d2(u:G).
Lemma 8. [1] The degree of an arbitrary vertex u of the bridge graph B1,d≥5 is given by:
deg(u:B1)={μ1+1,ifu=v1μd+1,ifu=vdμi+2,ifu=vi,2≤i≤d−1deg(u:Gi),ifu∈V(Gi)∖{vi},1≤i≤d, | (2.2) |
where μi=deg(vi:Gi),1≤i≤d.
Next, we compute the third leap Zagreb index of the type-Ⅰ bridge graph B1 Let us denote si=∑u∈NGi(vi)deg(u:Gi), 1≤i≤d.
Theorem 9. LM3(B1)=d∑i=1LM3(Gi)+(s1+sd)+2d−1∑i=2si+d∑i=1(2νi+6μi)+2d∑i=2(μi−1μi)−2(μ2+μd−1)−(ν1+νd)−3(μ1+μd)+4d−10.
Proof. By virtue of Lemma 5 and 8
LM3(B1)=∑u∈v(B1)d2(u)deg(u)=(ν1+μ2+1)(μ1+1)+(ν2+μ1+μ3+1)(μ2+2)+(νd+μd−1+1)(μd+1)+(νd−1+μd+μd−2+1)(μd−1+2)+d−2∑i=3(νi+μi−1+μi+1+2)(μi+2)+∑u∈NG1(v1)(d2(u:G1)+1)(deg(u:G1))+∑u∈NGd(vd)(d2(u:Gd)+1)(deg(u:Gd))+d−1∑i=2∑u∈NGi(vi)(d2(u:Gi)+2)(deg(u:Gi))+d∑i=1∑u∈V(Gi)∖NGi[vi](d2(u:Gi))(deg(u:Gi)) |
=(ν1μ1+ν1+μ2μ1+μ2+μ1+1)+(ν2μ2+2ν2+μ1μ2+2μ1+μ3μ2+2μ3+μ2+2)+(νdμd+νd+μd−1μd+μd−1+μd+1)+(νd−1μd−1+2νd−1+μdμd−1+2μd+μd−2μd−1+2μd−2+μd−1+2)+d−2∑i=3(νiμi+2νi+μi−1μi+2μi−1+μi+1μi+2μi+1+2μi+4)+∑u∈NG1(v1)(d2(u:G1)deg(u:G1)+deg(u:G1))+∑u∈NGd(vd)(d2(u:Gd)deg(u:Gd)+deg(u:Gd))+d−1∑i=2∑u∈NGi(vi)(d2(u:Gi)deg(u:Gi)+2deg(u:Gi))+d∑i=1∑u∈V(Gi)∖NGi[vi]d2(u:Gi)deg(u:Gi) |
Thus the result follows.
Corollary 10. If G1=G2=⋯=Gd=G in a bridge graph B1, then LM3(B1)=dLM3(G)+2(d−1)(s+ν+μ2)+2μ(3d−5)+4d−10, where s=∑u∈NG(v)deg(u:G).
For any two nonempty sets A and B, AΔB denotes the symmetric difference of A and B and defined as AΔB=(A∖B)∪(B∖A)=(A∪B)∖(A∩B). First, we obtain the 2-degree of any arbitrary vertex in the type-Ⅱ bridge graph B2 as follows:
Lemma 11. Let G1,G2,⋯,Gd be d≥5 triangle free connected graphs. Then 2-degree of any arbitrary vertex u in the bridge graph B2 formed by these graphs is as follows:
d2(u:B2)={d2(u:G1),ifu∈V(G1)∖NG1[w1]d2(u:G1)+1,ifu∈NG1(w1)d2(u:Gi),ifu∈V(Gi)∖{NGi[vi]∪NGi[wi]},2≤i≤d−1d2(u:Gd),ifu∈V(Gd)∖NGd[vd]d2(u:Gd)+1,ifu∈NGd(vd)d2(u:Gi)+1,ifu∈(NGi(vi)ΔNGi(wi)),2≤i≤d−1d2(u:Gi)+2,ifu∈NGi(vi)∩NGi(wi),2≤i≤d−1δi+μi+1,ifu=wi,1≤i≤d−1νi+λi−1,ifu=vi,2≤i≤d. | (2.3) |
where νi=d2(vi:Gi),μi=deg(vi:Gi);2≤i≤d,δi=d2(wi:Gi),λi=deg(wi:Gi);1≤i≤d−1.
Next, we compute the first leap Zagreb index of type-Ⅱ bridge graph B2.
Let us denote S′1=∑u∈NG1(w1)d2(u:G1) and Sd=∑u∈NGd(vd)d2(u:Gd)
Theorem 12. LM1(B2)=d∑i=1LM1(Gi)+2(S′1+Sd)+(λ1+μd)+d−1∑i=2∑u∈NGi(vi)ΔNGi(wi)[2d2(u:Gi)+1]+4d−1∑i=2∑u∈NGi(vi)∩NGi(wi)[d2(u:Gi)+1]+d−1∑i=1(μ2i+1+2δiμi+1)+d∑i=2(λ2i−1+2νiλi−1).
Proof.
LM1(B2)=∑u∈V(B2)d2(u:B2)2=∑u∈V(G1)∖NG1[w1]d2(u:G1)2+d−1∑i=2 ∑u∈V(Gi)∖{NGi[vi]∪NGi[wi]}d2(u:Gi)2+∑u∈V(Gd)∖NGd[vd]d2(u:Gd)2+∑u∈NG1(w1)(d2(u:G1)+1)2+d−1∑i=2∑u∈NGi(vi)ΔNGi(wi)(d2(u:Gi)+1)2+∑u∈NGd(vd)(d2(u:Gd)+1)2+d−1∑i=2∑u∈NGi(vi)∩NGi(wi)(d2(u:Gi)+2)2+d−1∑i=1(δi+μi+1)2+d∑i=2(νi+λi−1)2 |
=LM1(G1)−δ21−∑u∈NG1(w1)d2(u:G1)2+d−1∑i=2[∑u∈V(Gi)d2(u:Gi)2−∑u∈N(vi)∪N(wi)d2(u:Gi)2−ν2i−δ2i]+LM1(Gd)−ν2d−∑u∈NGd(vd)d2(u:Gd)2+∑u∈NG1(w1)d2(u:G1)2+2∑u∈NG1(w1)d2(u:G1)+λ1+d−1∑i=2[∑u∈NGi(vi)ΔNGi(wi)[d2(u:Gi)2+2d2(u:Gi)+1]]+∑u∈NGd(vd)[d2(u:Gd)2+2d2(u:Gd)+1]+d−1∑i=2[∑u∈NGi(vi)∩NGi(wi)[d2(u:Gi)2+4d2(u:Gi)+4]]+d−1∑i=1[δ2i+2δiμi+1+μ2i+1]+d∑i=2[ν2i+2νiλi−1+λ2i−1] |
Thus,
LM1(B2)=d∑i=1LM1(Gi)+2(S′1+Sd)+(λ1+μd)+d−1∑i=2∑u∈NGi(vi)ΔNGi(wi)[2d2(u:Gi)+1]+4d−1∑i=2∑u∈NGi(vi)∩NGi(wi)[d2(u:Gi)+1]+d−1∑i=1(μ2i+1+2δiμi+1)+d∑i=2(λ2i−1+2νiλi−1). |
Corollary 13. If G1=G2=⋯,Gd=G, in a bridge graph B2, then LM1(B2)=dLM1(G)+λ+μ+2(S+S′)+(d−2)∑u∈NG(v)ΔNG(w)(2d2(u:G)+1)+4(d−2)∑u∈NG(v)∩NG(w)(d2(u:G)+1)+(d−1)[μ2+λ2]+2(d−1)[δμ+νλ], where S=∑u∈NG(w)d2(u:G) and S′=∑u∈NG(v)d2(u:G).
In what follows next, we compute the third leap Zagreb index of B2.
Lemma 14. The degree of an arbitrary vertex u of the bridge graph B2, d≥5 is given by:
deg(u:B2)={deg(u:G1),ifu∈V(G1)∖{w1}deg(u:Gd),ifu∈V(Gd)∖{vd}deg(u:Gi),ifu∈V(Gi)∖{vi,wi},2≤i≤d−1λi+1,ifu=wi,1≤i≤d−1μi+1,ifu=vi,2≤i≤d. | (2.4) |
where μi=deg(vi:Gi);2≤i≤d,λi=deg(wi:Gi);1≤i≤d−1.
Theorem 15. LM3(B2)=d∑i=1LM3(Gi)+∑u∈NG1(w1)deg(u:G1)+∑u∈NGd(vd)deg(u:Gd)+d−1∑i=2∑u∈NGi(wi)∖NGi(vi)deg(u:Gi)+d−1∑i=2∑u∈NGi(vi)∖NGi(wi)deg(u:Gi)+d−1∑i=2∑u∈NGi(vi)∩NGi(wi)2deg(u:Gi)+d−1∑i=12μi+1λi+d−1∑i=1μi+1+d∑i=2λi−1+d∑i=1(δi+νi)−ν1−δd.
Proof. By virtue of Lemma 2.7 and 2.10
LM3(B2)=∑u∈V(B2)d2(u)deg(u)=∑u∈V(G1)∖NG1[w1]d2(u:G1)deg(u:G1)+d−1∑i=2∑u∈V(Gi)∖{NGi[vi]∪NGi[wi]}d2(u:Gi)deg(u:Gi)+∑u∈V(Gd)∖NGd[vd]d2(u:Gd)deg(u:Gd)+∑u∈NG1(w1)(d2(u:G1)+1)(deg(u:G1))+d−1∑i=2∑u∈NGi(wi)∖NGi(vi)(d2(u:Gi)+1)(deg(u:Gi))+d−1∑i=2∑u∈NGi(vi)∖NGi(wi)(d2(u:Gi)+1)(deg(u:Gi))+∑u∈NGd(vd)(d2(u:Gd)+1)(deg(u:Gd))+d−1∑i=2∑u∈NGi(vi)∩NGi(wi)(d2(u:Gi)+2)(deg(u:Gi))+d−1∑i=1(δi+μi+1)(λi+1)+d∑i=2(νi+λi−1)(μi+1) |
Thus the result follows.
Corollary 16. If G1=G2=⋯=Gd=G in a bridge graph B2, then LM3(B2)=dLM3(G)+∑u∈NG(w)deg(u:G)+∑u∈NG(v)deg(u:G)+(d−2)(∑u∈NG(w)∖NG(v)deg(u:G)+∑u∈NG(v)∖NG(w)deg(u:G)+∑u∈NG(v)∩NG(w)2deg(u:G))+(d−1)(2μλ+μ+λ)+d(δ+ν)−(ν+δ).
In the following lemma, we obtain the 2-degree of any vertex in the chain graph C.
Lemma 17. Let G1,G2,⋯,Gd, d≥5 be C3-free connected graphs and let C=C(G1,G2,⋯,Gd;w1,v2,w2,v3,⋯,wd−1,vd) be the chain graph formed using these graphs. Then the 2-degree of any vertex u in C is given as follows:
d2(u:C)={d2(u:G1),ifu∈V(G1)∖NG1[w1]d2(u:G1)+μ2,ifu∈NG1(w1)d2(u:Gd),ifu∈V(Gd)∖NGd[vd]d2(u:Gd)+λd−1,ifu∈NGd(vd)d2(u:Gi),ifu∈V(Gi)∖{NGi[wi]∪NGi[vi]},2≤i≤d−1d2(u:Gi)+μi+1,ifu∈NGi(wi)∖NGi(vi),2≤i≤d−1d2(u:Gi)+λi−1,ifu∈NGi(vi)∖NGi(wi),2≤i≤d−1d2(u:Gi)+λi−1+μi+1,ifu∈NGi(vi)∩NGi(wi),2≤i≤d−1δi+νi+1,ifu=wi=vi+1,1≤i≤d−1, | (2.5) |
where νi=d2(vi:Gi),μi=deg(vi:Gi),λi=deg(wi:Gi) and δi=d2(wi:Gi) for all 1≤i≤d.
Now, we compute the first leap Zagreb index of the chain graph C by applying Lemma 17.
Theorem 18. For the chain graph C,
LM1(C)=d∑i=1LM1(Gi)+∑u∈NG1(w1)[2μ2d2(u:G1)+μ22]+∑u∈NGd(vd)[2λd−1d2(u:Gd)+λ2d−1]+d−1∑i=2 ∑u∈NGi(wi)∖NGi(vi)[2μi+1d2(u:Gi)+μ2i+1]+d−1∑i=2 ∑u∈NGi(vi)∖NGi(wi)[2λi−1d2(u:Gi)+λ2i−1]+2d−1∑i=2 ∑u∈NGi(vi)∩NGi(wi)[λi−1d2(u:Gi)+μi+1d2(u:Gi)+λi−1μi+1]+d−1∑i=2 ∑u∈NGi(vi)∩NGi(wi)(λ2i−1+μ2i+1)+2d−1∑i=1δiνi+1. |
Proof. By Lemma 17, we have
LM1(C)=∑u∈V(C)d2(u:C)2=∑u∈V(G1)∖NG1[w1]d2(u:G1)2+∑u∈NG1(w1)[d2(u:G1)+μ2]2+∑u∈V(Gd)∖NGd[vd]d2(u:Gd)2+∑u∈NGd(vd)[d2(u:Gd)+λd−1]2+d−1∑i=2 ∑u∈V(Gi)∖{NGi[vi]∪NGi[wi]}d2(u:Gi)2+d−1∑i=2 ∑u∈NGi(wi)∖NGi(vi)[d2(u:Gi)+μi+1]2+d−1∑i=2 ∑u∈NGi(vi)∖NGi(wi)[d2(u:Gi)+λi−1]2+d−1∑i=2 ∑u∈NGi(vi)∩NGi(wi)[d2(u:Gi)+λi−1+μi+1]2+d−1∑i=1[δi+νi+1]2 |
=LM1(G1)−∑u∈NG1(w1)[d2(u:G1)2]−δ21+∑u∈NG1(w1)[d2(u:G1)2+2d2(u:G1)μ2+μ22]+LM1(Gd)−∑u∈NGd(vd)d2(u:Gd)2−ν2d+∑u∈NGd(vd)[d2(u:Gd)2+2λd−1d2(u:Gd)+λ2d−1]+d−1∑i=2∑u∈V(Gi)d2(u:Gi)2−d−1∑i=2 ∑u∈NGi[vi]∪NGi[wi]d2(u:Gi)2+d−1∑i=2 ∑u∈NGi(wi)∖NGi(vi)[d2(u:Gi)2+2μi+1d2(u:Gi)+μ2i+1]+d−1∑i=2 ∑u∈NGi(vi)∖NGi(wi)[d2(u:Gi)2+2λi−1d2(u:Gi)+λ2i−1]+d−1∑i=2∑u∈NGi(vi)∩NGi(wi)[d2(u:Gi)2+2λi−1d2(u:Gi)+2μi+1d2(u:Gi)+2λi−1μi+1+λ2i−1+μ2i+1]+d−1∑i=1[δ2i+ν2i+1]+2d−1∑i=1δiνi+1 |
=d∑i=1LM1(Gi)+∑u∈NG1(w1)[2μ2d2(u:G1)+μ22]+∑u∈NGd(vd)[2λd−1d2(u:Gd)+λ2d−1]+d−1∑i=2 ∑u∈NGi(wi)∖NGi(vi)[2μi+1d2(u:Gi)+μ2i+1]+d−1∑i=2 ∑u∈NGi(vi)∖NGi(wi)[2λi−1d2(u:Gi)+λ2i−1]+2d−1∑i=2 ∑u∈NGi(vi)∩NGi(wi)[λi−1d2(u:Gi)+μi+1d2(u:Gi)+λi−1μi+1]+d−1∑i=2 ∑u∈NGi(vi)∩NGi(wi)(λ2i−1+μ2i+1)+2d−1∑i=1δiνi+1. |
Corollary 19. In a chain graph C, if G1=G2=⋯=Gd=G, then LM1(C)=dLM1(G)+∑u∈NG(w)[2μd2(u:G)+μ2]+∑u∈NG(v)[2λd2(u:G)+λ2]+(d−2)∑u∈NG(w)∖NG(v)[2μd2(u:G)+μ2]+(d−2)∑u∈NG(v)∖NG(w)[2λd2(u:G)+λ2]+2(d−2)∑u∈NG(v)∩NG(w)[λd2(u:G)+μd2(u:G)+λμ]+(d−2)∑u∈NG(v)∩NG(w)(λ2+μ2)+2(d−1)δν.
Lemma 20. Let G1,G2,⋯,Gd, d≥5 be C3-free connected graphs and let C=C(G1,G2,⋯,Gd;w1,v2,w2,v3,⋯,wd−1,vd) be the chain graph formed using these graphs. Then the degree of any vertex u in C is given as follows:
deg(u:C)={deg(u:G1),ifu∈V(G1)∖{w1}deg(u:Gd),ifu∈V(Gd)∖{vd}deg(u:Gi),ifu∈V(Gi)∖{vi,wi},2≤i≤d−1λi+μi+1,ifu=wi=vi+1,1≤i≤d−1, | (2.6) |
where μi=deg(vi:Gi),λi=deg(wi:Gi) for all 1≤i≤d
Finally, we compute the third leap Zagreb index of the chain graph C by applying Lemma 17 and 2.16.
Theorem 21. LM3(C)=d∑i=1LM3(Gi)+∑u∈NG1(w1)μ2deg(u:G1)+∑u∈NGd(vd)λd−1deg(u:Gd)+d−1∑i=2∑u∈NGi(wi)∖NGi(vi)μi+1deg(u:Gi)+d−1∑i=2∑u∈NGi(vi)∖NGi(wi)λi−1deg(u:Gi)+d−1∑i=2∑u∈NGi(vi)∩NGi(wi)(λi−1deg(u:Gi)+μi+1deg(u:Gi))+d−1∑i=1(δiμi+1+νi+1λi).
Proof. By virtue of Lemma 17 and 20
LM3(C)=∑u∈V(C)d2(u)deg(u)=∑u∈V(G1)∖NG1[w1]d2(u:G1)deg(u:G1)+∑u∈NG1(w1)(d2(u:G1)+μ2)deg(u:G1)+∑u∈V(Gd)∖NGd[vd]d2(u:Gd)deg(u:Gd)+∑u∈NGd(vd)(d2(u:Gd)+λd−1)deg(u:Gd)+d−1∑i=2∑u∈V(Gi)∖{NGi[wi]∪NGi[vi]}d2(u:Gi)deg(u:Gi)+d−1∑i=2∑u∈NGi(wi)∖NGi(vi)(d2(u:Gi)+μi+1)deg(u:Gi)+d−1∑i=2∑u∈NGi(vi)∖NGi(wi)(d2(u:Gi)+λi−1)deg(u:Gi)+d−1∑i=2∑u∈NGi(vi)∩NGi(wi)(d2(u:Gi)+λi−1+μi+1)deg(u:Gi)+d−1∑i=1(δi+νi+1)(λi+μi+1). |
Thus the result follows.
Corollary 22. In a chain graph C, if G1=G2=⋯=Gd=G, then LM3(C)=dLM3(G)+∑u∈NG(w)μdeg(u:G)+∑u∈NG(v)λdeg(u:G)+(d−2)(∑u∈NG(w)∖NG(v)μdeg(u:G)+∑u∈NG(v)∖NG(w)λdeg(u:G)+∑u∈NG(v)∩NG(w)(λ+μ)deg(u:G))+(d−1)(δμ+νλ).
In this section, we determine the first and third leap Zagreb indices of some molecular graph structures. Two vertices v and w of a hexagon H (C6) (please refer Figure 4) are said to be in
(ⅰ) ortho-position, if they are adjacent in H
(ⅱ) meta-position, if they are distance two in H
(ⅲ) para-position, if they are distance three in H.
We connect h≥5 ortho-hexagons to form a polyphenyl chain denoted by Oh as follows:
One can observe that the Polyphenyl chain Oh shown in Figure 5 is a B1 type bridge graph. Therefore, from Corollary 7, we get
LM1(Oh)=hLM1(G)+(4h−6)μ2+(4h−8)ν+(12h−26)μ+(4h−4)[νμ+∑u∈NG(v)d2(u:G)]+4h−12=24h+(4h−6)(4)+(4h−8)(2)+(12h−26)(2)+(4h−4)(4)+(4h−4)(4)+4h−12=108h−136. |
Similarly,
From Corollary 10, we get
LM3(Oh)=24h+(2h−2)(2)+(2h−2)(2)+2(2)(3h−5)+2(h−1)(2+4)+4h−10=60h−50 |
The polyphenyl chain Mh is formed by connecting h≥5 meta-hexagons as shown in Figure 6.
The polyphenyl chain Ph is formed by connecting h≥5 para-hexagons as shown in the following Figure 7.
It is clear that the Polyphenyl chains Mh and Ph are type-Ⅱ bridge graphs B2.
Using Corollary 2.9, we get
LM1(Mh)=hLM1(G)+λ+μ+2∑u∈NG(w)d2(u:G)+(h−2)[∑u∈NG(w)∖NG(v)(2d2(u:G)+1)]+(h−2)∑u∈NG(v)∖NG(w)(2d2(u:G)+1)+4(h−2)∑u∈NG(v)∩NG(w)(d2(u:G)+1)+2∑u∈NG(v)d2(u:G)+(h−1)μ2+2(h−1)δμ+2(h−1)νλ+(h−1)λ2=24h+4+2(4)+(h−2)[2(2)+1]+(h−2)[2(2)+1]+4(h−2)(2+1)+2(4)+(h−1)(4)+4(h−1)(4)+(h−1)(4) |
Thus LM1(Mh)=70h−48.
Similarly, by Corollary 13, we have
LM1(Ph)=24h+4+2(4)+(h−2)[2(4)+2]+(h−2)(8+2)+4(h−2)(0)+2(4)+(h−1)(4)+8(h−1)+8(h−1)+(h−1)(4) |
Therefore, LM1(Ph)=68h−44.
Using Corollary 2.12, we get
LM3(Mh)=24h+8+(h−2)8+(h−1)12+h(4)−4=48h−24 |
LM3(Ph)=24h+8+(h−2)8+(h−1)12+4h−4=48h−24. |
Next, we shall see an application related to the chain graph C. The spiro-chain SPC4(d,3) is a chain graph formed using d≥5 copies of the cycle C4.
Here the number 3 in the construction denotes the position of the vertices v and w in the spiro-chain (refer Figure 8).
The spiro-chain SPC6(d,4) is a chain graph formed using d≥5 copies of the cycle C6 or hexagon where the vertices v and w are connected in the 4th position (refer Figure 9).
By applying Corollary 19, we get
LM1(SPC4(d,3))=dLM1(G)+∑u∈NG(w)[2μd2(u:G)+μ2]+∑u∈NG(v)[2λd2(u:G)+λ2]+(d−2)∑u∈NG(w)∖NG(v)[2μd2(u:G)+μ2]+(d−2)∑u∈NG(v)∖NG(w)[2λd2(u:G)+λ2]+2(d−2)∑u∈NG(v)∩NG(w)[λd2(u:G)+μd2(u:G)+λμ]+(d−2)∑u∈NG(v)∩NG(w)(λ2+μ2)+2(d−1)δν=54d−66. |
Similarly, from Corollary 19, we have LM1(SPC6(d,4))=80d−56.
By applying Corollary 22, we get
LM3(SPC4(d,3))=8d+2(2+2)+2(2+2)+(d−2)(16)+(d−1)(4)=28d−20 |
Similarly, from Corollary 22, we have LM3(SPC6(d,4))=48d−24.
We have computed exact values of one of the recent topological invariants namely first and third leap Zagreb indices for bridge and chain graphs. It is worth mentioning that computing second leap Zagreb index of bridges and chain graphs has not yet addressed and interested researchers may work on it. Also these indices need to be explored for several other interesting graph structures arising from mathematical chemistry and other branches of science.
The authors wish to thank the referees for their careful reading of the manuscript and valuable suggestions. This work was supported in part by the National Key Technologies R & D Program of China under Grant 2017YFB0802300, 2018YFB0904205, in part by the Key Laboratory of Pattern Recognition and Intelligent Information Processing, Institutions of Higher Education of Sichuan Province under Grant MSSB-2020-12.
The authors declare that no competing interests exist.
[1] |
V. Aula, Institutions, infrastructures, and data friction—reforming secondary use of health data in Finland, Big Data Soc., 6 (2019), 1–13. http://dx.doi.org/10.1177/2053951719875980 doi: 10.1177/2053951719875980
![]() |
[2] | European commission, Proposal for a regulation of the European parliament and of the council on the European health data space, European parliament, 2022. Available from: https://www.europarl.europa.eu/legislative-train/theme-promoting-our-european-way-of-life/file-european-health-data-space. |
[3] |
R. Lun, D. Siegal, T. Ramsay, G. Stotts, D. Dowlatshahi, Synthetic data in cancer and cerebrovascular disease research: a novel approach to big data, PLoS ONE, 19 (2024), e0295921. http://dx.doi.org/10.1371/journal.pone.0295921 doi: 10.1371/journal.pone.0295921
![]() |
[4] |
E. Sizikova, A. Badal, J. G. Delfino, M. Lago, B. Nelson, N. Saharkhiz, et al., Synthetic data in radiological imaging: current state and future outlook, Artif. Intell., 1 (2024), ubae007. http://dx.doi.org/10.1093/bjrai/ubae007 doi: 10.1093/bjrai/ubae007
![]() |
[5] |
J. A. Thomas, R. E. Foraker, N. Zamstein, J. D. Morrow, P. R. Payne, A. B. Wilcox, Demonstrating an approach for evaluating synthetic geospatial and temporal epidemiologic data utility: results from analyzing > 1.8 million SARS-CoV-2 tests in the United States national COVID cohort collaborative (N3C), J. Am. Med. Inform. Asso., 29 (2022), 1350–1365. http://dx.doi.org/10.1093/jamia/ocac045 doi: 10.1093/jamia/ocac045
![]() |
[6] |
H. Murtaza, M. Ahmed, N. F. Khan, G. Murtaza, S. Zafar, A. Bano, Synthetic data generation: state of the art in health care domain, Comput. Sci. Rev., 48 (2023), 100546. http://dx.doi.org/10.1016/j.cosrev.2023.100546 doi: 10.1016/j.cosrev.2023.100546
![]() |
[7] |
A. Gonzales, G. Guruswamy, S. R. Smith, Synthetic data in health care: a narrative review, PLOS Digit Health, 2 (2023), e0000082. http://dx.doi.org/10.1371/journal.pdig.0000082 doi: 10.1371/journal.pdig.0000082
![]() |
[8] |
S. James, C. Harbron, J. Branson, M. Sundler, Synthetic data use: exploring use cases to optimise data utility, Discov. Artif. Intell., 1 (2021), 15. http://dx.doi.org/10.1007/s44163-021-00016-y doi: 10.1007/s44163-021-00016-y
![]() |
[9] |
V. C. Pezoulas, D. I. Zaridis, E. Mylona, C. Androutsos, K. Apostolidis, N. S. Tachos, et al., Synthetic data generation methods in healthcare: a review on open-source tools and methods, Comput. Struct. Biotec., 23 (2024), 2892–2910. http://dx.doi.org/10.1016/j.csbj.2024.07.005 doi: 10.1016/j.csbj.2024.07.005
![]() |
[10] | C. A. F. López, A. Elbi, On the legal nature of synthetic data, NeurIPS 2022 Workshop on Synthetic Data for Empowering ML Research, 2022. |
[11] |
M. S. Gal, O. Lynskey, Synthetic data: legal implications of the data-generation revolution, Iowa L. Rev., 109 (2023), 1087. http://dx.doi.org/10.2139/ssrn.4414385 doi: 10.2139/ssrn.4414385
![]() |
[12] | J. Drechsler, A. C. Haensch, 30 years of synthetic data, Statist. Sci., 39 (2024), 221–242. http://dx.doi.org/10.1214/24-STS927 |
[13] | J. Jordon, L. Szpruch, F. Houssiau, M. Bottarelli, G. Cherubin, C. Maple, et al., Synthetic data—what, why and how? arXiv: 2205.03257. http://dx.doi.org/10.48550/arXiv.2205.03257 |
[14] | T. E. Raghunathan, J. P. Reiter, D. B. Rubin, Multiple imputation for statistical disclosure limitation, J. Off. Stat., 19 (2003), 1. |
[15] |
K. El Emam, L. Mosquera, J. Bass, Evaluating identity disclosure risk in fully synthetic health data: model development and validation, J. Med. Internet Res., 22 (2020), 23139. http://dx.doi.org/10.2196/23139 doi: 10.2196/23139
![]() |
[16] | J. P. Reiter, Inference for partially synthetic, public use microdata sets, Surv. Methodol., 29 (2003), 181–188. |
[17] | H. Surendra, H. Mohan, A review of synthetic data generation methods for privacy preserving data publishing, International Journal of Scientific and Technology Research, 6 (2017), 95–101. |
[18] |
S. Mohiuddin, R. Gardiner, M. Crofts, P. Muir, J. Steer, J. Turner, et al., Modelling patient flows and resource use within a sexual health clinic through discrete event simulation to inform service redesign, BMJ Open, 10 (2020), e037084. http://dx.doi.org/10.1136/bmjopen-2020-037084 doi: 10.1136/bmjopen-2020-037084
![]() |
[19] |
A. A. Tako, K. Kotiadis, C. Vasilakis, A. Miras, C. W. le Roux, Improving patient waiting times: a simulation study of an obesity care service, BMJ Qual. Saf., 23 (2014), 373–381. http://dx.doi.org/10.1136/bmjqs-2013-002107 doi: 10.1136/bmjqs-2013-002107
![]() |
[20] |
J. Yoon, M. Mizrahi, N. F. Ghalaty, T. Jarvinen, A. S. Ravi, P. Brune, et al., EHR-Safe: generating high-fidelity and privacy-preserving synthetic electronic health records, NPJ Digit. Med., 6 (2023), 141. http://dx.doi.org/10.1038/s41746-023-00888-7 doi: 10.1038/s41746-023-00888-7
![]() |
[21] |
L. Juwara, A. El-Hussuna, K. El Emam, An evaluation of synthetic data augmentation for mitigating covariate bias in health data, Patterns, 5 (2024), 100946. http://dx.doi.org/10.1016/j.patter.2024.100946 doi: 10.1016/j.patter.2024.100946
![]() |
[22] |
S. Kaji, S. Kida, Overview of image-to-image translation by use of deep neural networks: denoising, super-resolution, modality conversion, and reconstruction in medical imaging, Radiol. Phys. Technol., 12 (2019), 235–248. http://dx.doi.org/10.1007/s12194-019-00520-y doi: 10.1007/s12194-019-00520-y
![]() |
[23] |
S. Dayarathna, K. T. Islam, S. Uribe, G. Yang, M. Hayat, Z. Chen, Deep learning based synthesis of MRI, CT and PET: review and analysis, Med. Image Anal., 92 (2024), 103046. http://dx.doi.org/10.1016/j.media.2023.103046 doi: 10.1016/j.media.2023.103046
![]() |
[24] |
K. Armanious, C. Jiang, M. Fischer, T. Küstner, T. Hepp, K. Nikolaou, et al., MedGAN: medical image translation using GANs, Comput. Med. Imag. Grap., 79 (2020), 101684. http://dx.doi.org/10.1016/j.compmedimag.2019.101684 doi: 10.1016/j.compmedimag.2019.101684
![]() |
[25] |
J. Zhang, X. He, L. Qing, F. Gao, B. Wang, BPGAN: brain PET synthesis from MRI using generative adversarial network for multi-modal Alzheimer's disease diagnosis, Comput. Meth. Prog. Bio., 217 (2022), 106676. http://dx.doi.org/10.1016/j.cmpb.2022.106676 doi: 10.1016/j.cmpb.2022.106676
![]() |
[26] | M. J. Tadi, J. Teuho, R. Klén, E. Lehtonen, A. Saraste, C. S. Levin, Synthetic full dose cardiac PET images from low dose scans using conditional GANs, Proceedings of IEEE Nuclear Science Symposium and Medical Imaging Conference (NSS/MIC), 2022, 1–2. http://dx.doi.org/10.1109/NSS/MIC44845.2022.10399148 |
[27] | D. Doncenco, Exploring medical image data augmentation and synthesis using conditional generative adversarial networks, B.S. Thesis, Turku University of Applied Sciences, 2022. |
[28] |
J. T. Huhtanen, M. Nyman, D. Doncenco, M. Hamedian, D. Kawalya, L. Salminen, et al., Deep learning accurately classifies elbow joint effusion in adult and pediatric radiographs, Sci. Rep., 12 (2022), 11803. http://dx.doi.org/10.1038/s41598-022-16154-x doi: 10.1038/s41598-022-16154-x
![]() |
[29] |
P. Movahedi, V. Nieminen, I. M. Perez, H. Daafane, D. Sukhwal, T. Pahikkala et al., Benchmarking evaluation protocols for classifiers trained on differentially private synthetic data, IEEE Access, 12 (2024), 118637–118648. http://dx.doi.org/10.1109/ACCESS.2024.3446913 doi: 10.1109/ACCESS.2024.3446913
![]() |
[30] |
A. R. Benaim, R. Almog, Y. Gorelik, I. Hochberg, L. Nassar, T. Mashiach, et al., Analyzing medical research results based on synthetic data and their relation to real data results: systematic comparison from five observational studies, JMIR Med. Inform., 8 (2020), e16492. http://dx.doi.org/10.2196/16492 doi: 10.2196/16492
![]() |
[31] | P. Movahedi, V. Nieminen, I. M. Perez, T. Pahikkala, A. Airola, Evaluating classifiers trained on differentially private synthetic health data, Proceedings of IEEE 36th International Symposium on Computer-Based Medical Systems (CBMS), 2023,748–753. http://dx.doi.org/10.1109/CBMS58004.2023.00313 |
[32] |
B. Nowok, G. M. Raab, C. Dibben, Synthpop: bespoke creation of synthetic data in R, J. Stat. Softw., 74 (2016), 1–26. http://dx.doi.org/10.18637/jss.v074.i11 doi: 10.18637/jss.v074.i11
![]() |
[33] | A. Montanez, SDV: an open source library for synthetic data generation, Ph.D Thesis, Massachusetts Institute of Technology, 2018. |
[34] | T. Li, N. Li, On the tradeoff between privacy and utility in data publishing, Proceedings of the 15th ACM SIGKDD International Conference on Knowledge Discovery and Data Mining, 2009,517–526. http://dx.doi.org/10.1145/1557019.1557079 |
[35] |
A. Slavković, J. Seeman, Statistical data privacy: a song of privacy and utility, Annu. Rev. Stat. Appl., 10 (2023), 189–218. http://dx.doi.org/10.1146/annurev-statistics-033121-112921 doi: 10.1146/annurev-statistics-033121-112921
![]() |
[36] | B. Zhao, M. A. Kaafar, N. Kourtellis, Not one but many tradeoffs: privacy vs. utility in differentially private machine learning, Proceedings of the 2020 ACM SIGSAC Conference on Cloud Computing Security Workshop, 2020, 15–26. http://dx.doi.org/10.1145/3411495.3421352 |
[37] | M. Hittmeir, R. Mayer, A. Ekelhart, A baseline for attribute disclosure risk in synthetic data, Proceedings of the Tenth ACM Conference on Data and Application Security and Privacy, 2020,133–143. http://dx.doi.org/10.1145/3374664.3375722 |
[38] |
K. El Emam, L. Mosquera, X. Fang, Validating a membership disclosure metric for synthetic health data, JAMIA Open, 5 (2022), ooac083. http://dx.doi.org/10.1093/jamiaopen/ooac083 doi: 10.1093/jamiaopen/ooac083
![]() |
[39] | L. Sweeney, Simple demographics often identify people uniquely, Data Privacy Working Paper, 2000. |
[40] |
L. Sweeney, k-anonymity: a model for protecting privacy, Int. J. Uncertain. Fuzz., 10 (2002), 557–570. http://dx.doi.org/10.1142/S0218488502001648 doi: 10.1142/S0218488502001648
![]() |
[41] | N. Li, T. Li, S. Venkatasubramanian, t-closeness: privacy beyond k-anonymity and l-diversity, Proceedings of the 23rd international conference on data engineering, 2007,106–115. http://dx.doi.org/10.1109/ICDE.2007.367856 |
[42] |
C. Dwork, A. Roth, The algorithmic foundations of differential privacy, Found. Trends Theor. C., 9 (2014), 211–407. http://dx.doi.org/10.1561/0400000042 doi: 10.1561/0400000042
![]() |
[43] |
M. Finck, F. Pallas, They who must not be identified—distinguishing personal from non-personal data under the GDPR, Int. Data Priv. Law, 10 (2020), 11–36. http://dx.doi.org/10.1093/idpl/ipz026 doi: 10.1093/idpl/ipz026
![]() |
[44] |
A. Cohen, K. Nissim, Towards formalizing the GDPR's notion of singling out, PNAS, 117 (2020), 8344–8352. http://dx.doi.org/10.1073/pnas.1914598117 doi: 10.1073/pnas.1914598117
![]() |
[45] |
M. Veale, R. Binns, L. Edwards, Algorithms that remember: model inversion attacks and data protection law, Phil. Trans. R. Soc. A, 376 (2018), 20180083. http://dx.doi.org/10.1098/rsta.2018.0083 doi: 10.1098/rsta.2018.0083
![]() |
[46] |
C. Sun, J. van Soest, M. Dumontier, Generating synthetic personal health data using conditional generative adversarial networks combining with differential privacy, J. Biomed. Inform., 143 (2023), 104404. http://dx.doi.org/10.1016/j.jbi.2023.104404 doi: 10.1016/j.jbi.2023.104404
![]() |
[47] | J. Jordon, J. Yoon, M. van der Schaar, PATE-GAN: generating synthetic data with differential privacy guarantees, Proceedings of International Conference on Learning Representations, 2019, 1–29. |
[48] | N. C. Abay, Y. Zhou, M. Kantarcioglu, B. Thuraisingham, L. Sweeney, Privacy preserving synthetic data release using deep learning, In: Machine learning and knowledge discovery in databases, Cham: Springer, 2019,510–526. http://dx.doi.org/10.1007/978-3-030-10925-7_31 |
[49] | I. Montoya Perez, P. Movahedi, V. Nieminen, A. Airola, T. Pahikkala, Does differentially private synthetic data lead to synthetic discoveries? Methods Inf. Med., in press. http://dx.doi.org/10.1055/a-2385-1355 |
[50] | M. I. Khan, M. A. Azeem, E. Alhoniemi, E. Kontio, S. A. Khan, M. Jafaritadi, Regularized weight aggregation in networked federated learning for glioblastoma segmentation, In: Brainlesion: glioma, multiple sclerosis, stroke and traumatic brain injuries, Cham: Springer, 2022,121–132. http://dx.doi.org/10.1007/978-3-031-44153-0_12 |
[51] | M. I. Khan, M. Jafaritadi, E. Alhoniemi, E. Kontio, S. A. Khan, Adaptive weight aggregation in federated learning for brain tumor segmentation, In: Brainlesion: glioma, multiple sclerosis, stroke and traumatic brain injuries, Cham: Springer, 2022,455–469. http://dx.doi.org/10.1007/978-3-031-09002-8_40 |
[52] | M. I. Khan, E. Alhoniemi, E. Kontio, S. A. Khan, M. Jafaritadi, RegAgg: a scalable approach for efficient weight aggregation in federated lesion segmentation of brain MRIs, Proceedings of Eighth International Conference on Fog and Mobile Edge Computing (FMEC), 2023,101–106. http://dx.doi.org/10.1109/FMEC59375.2023.10306171 |
[53] |
J. Xu, B. S. Glicksberg, C. Su, P. Walker, J. Bian, F. Wang, Federated learning for healthcare informatics, J. Healthc. Inform. Res., 5 (2021), 1–19. http://dx.doi.org/10.1007/s41666-020-00082-4 doi: 10.1007/s41666-020-00082-4
![]() |
[54] |
M. Giuffrè, D. L. Shung, Harnessing the power of synthetic data in healthcare: innovation, application, and privacy, NPJ Digit. Med., 6 (2023), 186. http://dx.doi.org/10.1038/s41746-023-00927-3 doi: 10.1038/s41746-023-00927-3
![]() |
[55] | D. Shanley, J. Hogenboom, F. Lysen, L. Wee, A. Lobo Gomes, A. Dekker, et al., Getting real about synthetic data ethics: are AI ethics principles a good starting point for synthetic data ethics? EMBO Rep., 25 (2024), 2152–2155. http://dx.doi.org/10.1038/s44319-024-00101-0 |
[56] |
B. N. Jacobsen, Machine learning and the politics of synthetic data, Big Data Soc., 10 (2023), 1–12. http://dx.doi.org/10.1177/20539517221145372 doi: 10.1177/20539517221145372
![]() |
[57] | C. D. Whitney, J. Norman, Real risks of fake data: synthetic data, diversity-washing and consent circumvention, Proceedings of the 2024 ACM Conference on Fairness, Accountability, and Transparency, 2024, 1733–1744. http://dx.doi.org/10.1145/3630106.3659002 |
[58] | G. Ganev, B. Oprisanu, E. De Cristofaro, Robin Hood and Matthew effects: differential privacy has disparate impact on synthetic data, Proceedings of the 39th International Conference on Machine Learning, 2022, 6944–6959. |
[59] |
T. Hayashi, D. Cimr, H. Fujita, R. Cimler, Interpretable synthetic signals for explainable one-class time-series classification, Eng. Appl. Artif. Intell., 131 (2024), 107716. http://dx.doi.org/10.1016/j.engappai.2023.107716 doi: 10.1016/j.engappai.2023.107716
![]() |
[60] | J. Vaiste, Ethical implications of AI-generated synthetic health data, HAL Id: hal-04216538. |
[61] | J. S. Franklin, K. Bhanot, M. Ghalwash, K. P. Bennett, J. McCusker, D. L. McGuinness, An ontology for fairness metrics, Proceedings of the 2022 AAAI/ACM Conference on AI, Ethics, and Society, 2022,265–275. http://dx.doi.org/10.1145/3514094.3534137 |
[62] |
K. Bhanot, M. Qi, J. S. Erickson, I. Guyon, K. P. Bennett, The problem of fairness in synthetic healthcare data, Entropy, 23 (2021), 1165. http://dx.doi.org/10.3390/e23091165 doi: 10.3390/e23091165
![]() |
[63] | T. Farrand, F. Mireshghallah, S. Singh, A. Trask, Neither private nor fair: impact of data imbalance on utility and fairness in differential privacy, Proceedings of the 2020 workshop on privacy-preserving machine learning in practice, 2020, 15–19. http://dx.doi.org/10.1145/3411501.3419419 |
[64] |
V. Volovici, N. L. Syn, A. Ercole, J. J. Zhao, N. Liu, Steps to avoid overuse and misuse of machine learning in clinical research, Nat. Med., 28 (2022), 1996–1999. http://dx.doi.org/10.1038/s41591-022-01961-6 doi: 10.1038/s41591-022-01961-6
![]() |
[65] |
A. S. Hashemi, A. Soliman, J. Lundström, K. Etminani, Domain knowledge-driven generation of synthetic healthcare data, Stud. Health Technol. Inform., 302 (2023), 352–353. http://dx.doi.org/10.3233/SHTI230136 doi: 10.3233/SHTI230136
![]() |
[66] | J. Latner, M. Neunhoeffer, J. Drechsler, Generating synthetic data is complicated: know your data and know your generator, In: Privacy in statistical databases, Cham: Springer, 2024,115–128. http://dx.doi.org/10.1007/978-3-031-69651-0_8 |
[67] |
F. K. Dankar, M. K. Ibrahim, L. Ismail, A multi-dimensional evaluation of synthetic data generators, IEEE Access, 10 (2022), 11147–11158. http://dx.doi.org/10.1109/ACCESS.2022.3144765 doi: 10.1109/ACCESS.2022.3144765
![]() |
[68] | M. Miletic, M. Sariyar, Assessing the potentials of LLMs and GANs as state-of-the-art tabular synthetic data generation methods, In: Privacy in statistical databases, Cham: Springer, 2024,374–389. http://dx.doi.org/10.1007/978-3-031-69651-0_25 |
[69] | R. Hamon, H. Junklewitz, I. Sanchez, Robustness and explainability of artificial intelligence, Luxembourg: Publications Office of the European Union, 2020. http://dx.doi.org/10.2760/57493 |
[70] |
M. Hernandez, G. Epelde, A. Alberdi, R. Cilla, D. Rankin, Synthetic data generation for tabular health records: a systematic review, Neurocomputing, 493 (2022), 28–45. http://dx.doi.org/10.1016/j.neucom.2022.04.053 doi: 10.1016/j.neucom.2022.04.053
![]() |
[71] | K. Perkonoja, K. Auranen, J. Virta, Methods for generating and evaluating synthetic longitudinal patient data: a systematic review, arXiv: 2309.12380. http://dx.doi.org/10.48550/arXiv.2309.12380 |
[72] |
J. Zhang, G. Cormode, C. M. Procopiuc, D. Srivastava, X. Xiao, PrivBayes: private data release via Bayesian networks, ACM T. Database Syst., 42 (2017), 25. http://dx.doi.org/10.1145/3134428 doi: 10.1145/3134428
![]() |
[73] | J. de Benedetti, N. Oues, Z. Wang, P. Myles, A. Tucker, Practical lessons from generating synthetic healthcare data with Bayesian networks, In: ECML PKDD 2020 workshops, Cham: Springer, 2020, 38–47. http://dx.doi.org/10.1007/978-3-030-65965-3_3 |
[74] | I. Goodfellow, J. Pouget-Abadie, M. Mirza, B. Xu, D. Warde-Farley, S. Ozair, et al., Generative adversarial nets, Proceedings of the 27th International Conference on Neural Information Processing Systems, 2014, 2672–2680. |
[75] | D. P. Kingma, M. Welling, Auto-encoding variational Bayes, arXiv: 1312.6114. http://dx.doi.org/10.48550/arXiv.1312.6114 |
[76] |
C. Yan, Y. Yan, Z. Wan, Z. Zhang, L. Omberg, J. Guinney, et al., A multifaceted benchmarking of synthetic electronic health record generation models, Nat. Commun., 13 (2022), 7609. http://dx.doi.org/10.1038/s41467-022-35295-1 doi: 10.1038/s41467-022-35295-1
![]() |
[77] | S. Biswal, S. Ghosh, J. Duke, B. Malin, W. Stewart, C. Xiao, J. Sun, EVA: generating longitudinal electronic health records using conditional variational autoencoders, Proceedings of the 6th Machine Learning for Healthcare Conference, 2021,260–282. |
[78] |
F. K. Dankar, M. Ibrahim, Fake it till you make it: guidelines for effective synthetic data generation, Appl. Sci., 11 (2021), 2158. http://dx.doi.org/10.3390/app11052158 doi: 10.3390/app11052158
![]() |
[79] |
C. Yan, Z. Zhang, S. Nyemba, Z. Li, Generating synthetic electronic health record data using generative adversarial networks: tutorial, JMIR AI, 3 (2024), e52615. http://dx.doi.org/10.2196/52615 doi: 10.2196/52615
![]() |
[80] | V. Nieminen, T. Pahikkala, A. Airola, Empirical evaluation of amplifying privacy by subsampling for GANs to create differentially private synthetic tabular data, Proceedings of TKTP 2023: Annual Symposium for Computer Science, 2023, 72–81. |
[81] | A. Alaa, B. van Breugel, E. S. Saveliev, M. van der Schaar, How faithful is your synthetic data? Sample-level metrics for evaluating and auditing generative models, Proceedings of the 39th International Conference on Machine Learning, 2022,290–306. |
[82] |
A. Yale, S. Dash, R. Dutta, I. Guyon, A. Pavao, K. P. Bennett, Generation and evaluation of privacy preserving synthetic health data, Neurocomputing, 416 (2020), 244–255. http://dx.doi.org/10.1016/j.neucom.2019.12.136 doi: 10.1016/j.neucom.2019.12.136
![]() |
[83] |
J. Yoon, L. N. Drumright, M. van der Schaar, Anonymization through data synthesis using generative adversarial networks (ADS-GAN), IEEE J. Biomed. Health, 24 (2020), 2378–2388. http://dx.doi.org/10.1109/JBHI.2020.2980262 doi: 10.1109/JBHI.2020.2980262
![]() |
[84] |
V. B. Vallevik, A. Babic, S. E. Marshall, E. Severin, H. M. Brøgger, S. Alagaratnam, et al., Can I trust my fake data—a comprehensive quality assessment framework for synthetic tabular data in healthcare, Int. J. Med. Inform., 185 (2024), 105413. http://dx.doi.org/10.1016/j.ijmedinf.2024.105413 doi: 10.1016/j.ijmedinf.2024.105413
![]() |
[85] |
Z. Azizi, S. Lindner, Y. Shiba, V. Raparelli, C. M. Norris, K. Kublickiene, et al., A comparison of synthetic data generation and federated analysis for enabling international evaluations of cardiovascular health, Sci. Rep., 13 (2023), 11540. http://dx.doi.org/10.1038/s41598-023-38457-3 doi: 10.1038/s41598-023-38457-3
![]() |
[86] |
M. Hernandez, G. Epelde, A. Beristain, R. Álvarez, C. Molina, X. Larrea, et al., Incorporation of synthetic data generation techniques within a controlled data processing workflow in the health and wellbeing domain, Electronics, 11 (2022), 812. http://dx.doi.org/10.3390/electronics11050812 doi: 10.3390/electronics11050812
![]() |
[87] |
C. Little, M. Elliot, R. Allmendinger, Federated learning for generating synthetic data: a scoping review, Int. J. Popul. Data Sci., 8 (2023), 2158. http://dx.doi.org/10.23889/ijpds.v8i1.2158 doi: 10.23889/ijpds.v8i1.2158
![]() |
[88] |
J. W. Kim, B. Jang, Privacy-preserving generation and publication of synthetic trajectory microdata: a comprehensive survey, J. Netw. Comput. Appl., 230 (2024), 103951. http://dx.doi.org/10.1016/j.jnca.2024.103951 doi: 10.1016/j.jnca.2024.103951
![]() |
[89] |
C. Alloza, B. Knox, H. Raad, M. Aguilà, C. Coakley, Z. Mohrova, et al., A case for synthetic data in regulatory decision-making in Europe, Clin. Pharmacol. Ther., 114 (2023), 795–801. http://dx.doi.org/10.1002/cpt.3001 doi: 10.1002/cpt.3001
![]() |
[90] | A. Beduschi, Synthetic data protection: towards a paradigm change in data regulation? Big Data Soc., 11 (2024), 1–5. http://dx.doi.org/10.1177/20539517241231277 |
[91] | P. Lehto, S. Malkamäki, The Finnish health sector growth and competitiveness vision 2030, Helsinki: Sitra, 2023. |
[92] | Finnish association of private care providers, Sotedigin työkalupakista eväitä tiedon hyödyntämiseen sote-palveluissa, Hyvinvointiala Hali ry, 2023. Available from: https://www.hyvinvointiala.fi/sotedigin-tyokalupakista-evaita-tiedon-hyodyntamiseen-sote-palveluissa/. |
[93] |
S. Moazemi, T. Adams, H. G. NG, L. Kühnel, J. Schneider, A. F. Näher, et al., NFDI4Health workflow and service for synthetic data generation, assessment and risk management, Stud. Health Technol. Inform., 317 (2024), 21–29. http://dx.doi.org/10.3233/SHTI240834 doi: 10.3233/SHTI240834
![]() |
1. | Xiujun Zhang, Zhiqiang Zhang, Natarajan Chidambaram, Subhasri Jaganathan, Narasimhan Devadoss, Vignesh Ravi, On degree and distance-based topological indices of certain interconnection networks, 2022, 137, 2190-5444, 10.1140/epjp/s13360-022-03010-0 | |
2. | Kinkar Chandra Das, Sourav Mondal, Zahid Raza, On Zagreb connection indices, 2022, 137, 2190-5444, 10.1140/epjp/s13360-022-03437-5 | |
3. | Sourav Mondal, Kinkar Chandra Das, Zagreb connection indices in structure property modelling, 2023, 69, 1598-5865, 3005, 10.1007/s12190-023-01869-5 | |
4. | Deepa Balasubramaniyan, Natarajan Chidambaram, Vignesh Ravi, Muhammad Kamran Siddiqui, QSPR analysis of anti‐asthmatic drugs using some new distance‐based topological indices: A comparative study, 2024, 124, 0020-7608, 10.1002/qua.27372 | |
5. | Yaşar NACAROĞLU, ON LEAP ZAGREB INDICES OF A SPECIAL GRAPH OBTAINED BY SEMIGROUPS, 2023, 6, 2618-5660, 16, 10.33773/jum.1333260 |