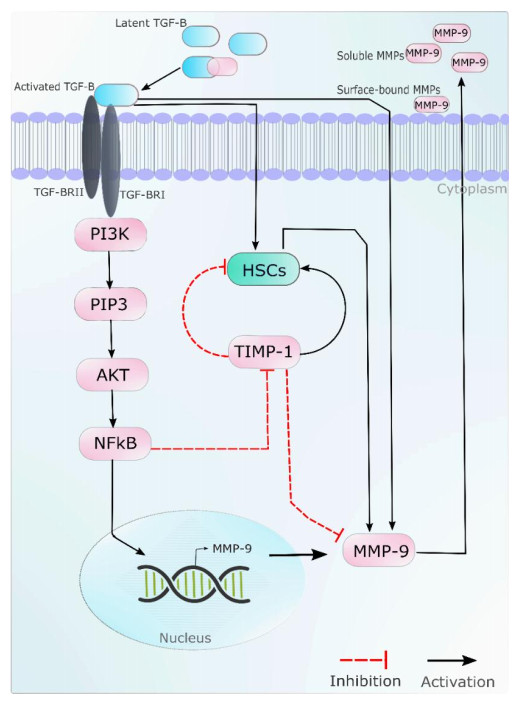
In the dynamic landscape of today's digitized markets, organizations harness the power of vast and swiftly accessible data to glean invaluable insights. A significant portion of this data emanates from user behavior on business websites. Unraveling the intricacies of this user behavior has become paramount for businesses, serving as the compass guiding the adaptation and evolution of their digital marketing strategies. Embarking on an exploration of this digital frontier, our study delves into the virtual domains of enterprises entrenched in the supply chain sector of the Greek economy. The spotlight falls upon four dominant transportation firms of the Greek supply chain sector, to unravel the relationship between their website activities and the prediction of their stock market prices. Our analytical tools, adorned with sophisticated statistical methodologies, embracing normality tests, correlations, ANOVA, linear regressions and the utilization of regression residual tests were deployed with precision. As the analytical methodology was deployed, a revelation emerged: The digital footprints left by customers on the virtual domains of supply chain firms provided the ability to predict and influence stock prices. Metrics such as bounce rates, the influx of new visitors and the average time on websites emerged as important factors, that could predict the fluctuations in the stock prices of these Greek supply chain firms. Web analytics have been discerned as a determining factor for predicting the course of transportation firms' stock prices. It serves as a clarion call for global scrutiny, inviting scholars and practitioners alike to scrutinize analogous firms on a global canvas. In this convergence of virtual footprints and financial trajectories lies not just a revelation for today but a harbinger of insights that resonate far beyond the digital borders of the Hellenic transportation sector.
Citation: Nikolaos T. Giannakopoulos, Damianos P. Sakas, Nikos Kanellos, Christos Christopoulos. Web analytics and supply chain transportation firms' financial performance[J]. National Accounting Review, 2023, 5(4): 405-420. doi: 10.3934/NAR.2023023
[1] | Yu Jiang, Lijuan Lin, Huiming Lv, He Zhang, Lili Jiang, Fenfen Ma, Qiuyue Wang, Xue Ma, Shengjin Yu . Immune cell infiltration and immunotherapy in hepatocellular carcinoma. Mathematical Biosciences and Engineering, 2022, 19(7): 7178-7200. doi: 10.3934/mbe.2022339 |
[2] | Kazuhisa Ichikawa . Synergistic effect of blocking cancer cell invasionrevealed by computer simulations. Mathematical Biosciences and Engineering, 2015, 12(6): 1189-1202. doi: 10.3934/mbe.2015.12.1189 |
[3] | Yonghui Wang, Jianhui Huang, Zhifeng Tian, Yuefen Zhou, Jie Yang . The role of CXC cytokines as biomarkers and potential targets in hepatocellular carcinoma. Mathematical Biosciences and Engineering, 2020, 17(2): 1381-1395. doi: 10.3934/mbe.2020070 |
[4] | Qi-Jia Zhang, Li Wan, Han-Feng Xu . High expression of agrin is associated with tumor progression and poor prognosis in hepatocellular carcinoma. Mathematical Biosciences and Engineering, 2019, 16(6): 7375-7383. doi: 10.3934/mbe.2019368 |
[5] | Caihao Qu, Tengda Ma, Xin YAN, Xiaomei Li, Yumin Li . Overexpressed PAQR4 predicts poor overall survival and construction of a prognostic nomogram based on PAQR family for hepatocellular carcinoma. Mathematical Biosciences and Engineering, 2022, 19(3): 3069-3090. doi: 10.3934/mbe.2022142 |
[6] | Yuanqian Yao, Jianlin Lv, Guangyao Wang, Xiaohua Hong . Multi-omics analysis and validation of the tumor microenvironment of hepatocellular carcinoma under RNA modification patterns. Mathematical Biosciences and Engineering, 2023, 20(10): 18318-18344. doi: 10.3934/mbe.2023814 |
[7] | Lingling Li, Congbo Li, Li Li, Ying Tang, Qingshan Yang . An integrated approach for remanufacturing job shop scheduling with routing alternatives. Mathematical Biosciences and Engineering, 2019, 16(4): 2063-2085. doi: 10.3934/mbe.2019101 |
[8] | Jing Liu, Wancheng Qiu, Xiaoying Shen, Guangchun Sun . Bioinformatics analysis revealed hub genes and pathways involved in sorafenib resistance in hepatocellular carcinoma. Mathematical Biosciences and Engineering, 2019, 16(6): 6319-6334. doi: 10.3934/mbe.2019315 |
[9] | Donggu Lee, Sunju Oh, Sean Lawler, Yangjin Kim . Bistable dynamics of TAN-NK cells in tumor growth and control of radiotherapy-induced neutropenia in lung cancer treatment. Mathematical Biosciences and Engineering, 2025, 22(4): 744-809. doi: 10.3934/mbe.2025028 |
[10] | Heinz Schättler, Urszula Ledzewicz, Benjamin Cardwell . Robustness of optimal controls for a class of mathematical models for tumor anti-angiogenesis. Mathematical Biosciences and Engineering, 2011, 8(2): 355-369. doi: 10.3934/mbe.2011.8.355 |
In the dynamic landscape of today's digitized markets, organizations harness the power of vast and swiftly accessible data to glean invaluable insights. A significant portion of this data emanates from user behavior on business websites. Unraveling the intricacies of this user behavior has become paramount for businesses, serving as the compass guiding the adaptation and evolution of their digital marketing strategies. Embarking on an exploration of this digital frontier, our study delves into the virtual domains of enterprises entrenched in the supply chain sector of the Greek economy. The spotlight falls upon four dominant transportation firms of the Greek supply chain sector, to unravel the relationship between their website activities and the prediction of their stock market prices. Our analytical tools, adorned with sophisticated statistical methodologies, embracing normality tests, correlations, ANOVA, linear regressions and the utilization of regression residual tests were deployed with precision. As the analytical methodology was deployed, a revelation emerged: The digital footprints left by customers on the virtual domains of supply chain firms provided the ability to predict and influence stock prices. Metrics such as bounce rates, the influx of new visitors and the average time on websites emerged as important factors, that could predict the fluctuations in the stock prices of these Greek supply chain firms. Web analytics have been discerned as a determining factor for predicting the course of transportation firms' stock prices. It serves as a clarion call for global scrutiny, inviting scholars and practitioners alike to scrutinize analogous firms on a global canvas. In this convergence of virtual footprints and financial trajectories lies not just a revelation for today but a harbinger of insights that resonate far beyond the digital borders of the Hellenic transportation sector.
Hepatocellular carcinoma (HCC), is the most frequently occurring form of primary liver cancer and the main reason for tumor-related deaths globally [1]. Despite advances in technology for screening, diagnosis, and treatment, there is still a significant rise in the rate of incidence and mortality worldwide. Rapidly growing HCC cells that provide a site for early vascular invasion, tend to be unaffected by currently available chemotherapeutic options [2]. This leads to an increased incidence of recurrence and metastasis post-surgery and results in poor prognoses [3]. Over the previous decade, several studies have significantly increased our understanding of the pathogenesis of HCC, but the exact molecular mechanism involved in the metastasis of HCC is still ambiguous.
There exist a plethora of signaling pathways which regulate the system to maintain a homeostatic condition. These regulations are highly significant in preventing the cells from metastasis. Once these regulations are disturbed, there is a chance that the system might move towards a pathogenic state. Thus, there is an imminent need to explore the critical molecular regulatory mechanisms in the key signaling pathways involved in HCC metastasis which will help in the identification of new therapeutic targets. TGF-β and PI3K/AKT pathways are the key signaling pathways that have been identified during the progression of HCC. The misregulation of the TGFβ signaling pathway may result in tumor development. According to the recent studies, matrix metalloproteinases (MMPs) like MMP-2, MMP-1, MMP-9, and MMP-8 along with transforming growth factor beta1 (TGF-β1) actively contribute to HCC progression. They play a significant role as the major key players in metastatic and invasive tumor cells [4]. MMPs or matrix metalloproteinases are a group of proteolytic enzymes that are responsible for the collective degradation of the components of proteins found in the basement membranes and extracellular matrix (ECM). Several studies indicate that the over-expression of MMP- 2, 1, 7, 9 and 14 lead to invasion as well as metastasis of HCC through the induction of epithelial to mesenchymal transition (EMT) [5,6,7]. It has been implied that enhanced expression of MMP-9 is highly anticipated in invasive HCC cells as compared to non-invasive cells. Moreover, during HCC, the upregulation of MMP-9 has been associated with metastasis, tumor recurrence and invasion [5]. Previous reports indicate that it may act as a tumor promoter or as an anti-cancer agent in highly rare situations [6]. This was observed from studies performed on MMP-9 knockdown mice models where the tumor incidence was significantly decreased [7]. In addition, overexpression of MMP-9 also correlates to tumor progression and reduced survival rate of HCC affected individuals [11,12]. Nevertheless, the importance of MMP-9 in HCC progression still needs to be clarified.
TGF-β1 or transforming growth factor beta1 is a widely known cytokine that mediates cellular processes like apoptosis, cell differentiation, and homeostasis. It acts as a driver of tumor progression by inducing EMT and is involved in the activation of several MMPs, including MMP-9 [13,14]. During breast cancer, TGF-β1 promotes metastasis by upregulating the expression of MMP-9 [10]. Likewise, MMP-9, 2, and 14, can induce the biological activity of TGF-β1 via cleavage of latent TGF-β-binding protein-1 [11]. Furthermore, MMP-9 is involved in HCC cell invasion and EMT induced by autophagy through the stimulation of TGF-β/Smad3 signaling [12]. A constant relationship between TGF-β1 and MMP-9 has been established through numerous in vitro studies. Moreover, it has been implied that genetically targeted TGF-β1 could alter expression intensities of MMP-9 and MMP-2 in HCC infected cells [13]. Despite the evidence, the molecular mechanisms of positive feedback loop between TGF-β1 and MMP-9 on HCC still remain ambiguous.
The latent TGF-β1 ligand is activated by either a surface-bound or soluble MMP-9 (Figure 1). The activated TGF-β1 ligand adheres to the cell surface type Ⅱ receptor (TβRII) which is activated by autophosphorylation and recruits the type Ⅰ receptor (TβRI). TβRI is then activated by phosphorylation. This heterotetrameric complex then stimulates the association of the intracellular signal mediators. TGF-β1 stimulates phosphatidylinositol 3-kinase (PI3K) via phosphorylating Akt, a downstream effector. The PI3K/Akt pathway exists as a non-Smad pathway that is known to contribute to EMT prompted by TGF-β [14]. In case of head and neck cancer, stimulation of PI3K/Akt intensifies the expression of MMP-9, leading to degradation of E-cadherin, hence promoting invasion and passage of cells [15]. MMP-9 production is enhanced by stimulated transcriptional activity (mediated by Akt/PKB) of nuclear factor-kappa B.
It is well established that HCC progression is caused by the progression of liver fibrosis i.e., deposition of ECM proteins. Henceforth, hepatic stellate cells (HSCs) along with EMT reportedly play a part in HCC progression and metastasis [16]. Also, TGF-β1 stimulates fibrogenesis through induction of the HSCs, thus stimulating HCC progression [17]. Also, MMPs are responsible for regulating the remodeling of ECM, hence they are critical in HCC progression. It has been previously testified that TGF-β1, MMP-8, 2 and 9 can trigger EMT in HCC cells and lead to pathogenesis [16]. These studies reveal that MMP-9 and TGF-β1 can induce progression of HCC by triggering the activation of HSCs and/or EMT.
Modulation of gene expression determines the overall dynamics of each cell and this is a complex process [18]. Computational and mathematical approaches in systems biology expedite to observe dynamics of a biological system where entities like RNA, DNA, proteins, enzymes and other biological molecules are involved [19]. The qualitative modeling framework is amongst the most recognized methods to study the dynamics of gene expression [25,26] by constructing BRNs (biological regulatory networks). Systems in biology are usually modeled using partial or ordinary differential equations, which depend on time derivatives of expression levels, along with kinetic rates of entities. The system is represented in a detailed manner by using quantitative models, which requires data which is either specific or too difficult to find. Hence, qualitative models (BRN, petri net) are preferred to understand the complex dynamics of the biological systems. Construction of the BRN requires qualitative thresholds and logical parameters, which can be easily adjusted according to the system dynamics and biological observations.
In this study, we examined the prospective interplay between TGF-β1 and MMP-9 in HCC progression and homeostasis. A BRN was constructed comprising significant entities encompassing TGF-β1 signaling to determine the inhibition and activation relationships. Different parameters of the model are generated from prior experimental knowledge by using the model checking technique. The parameters are then rendered into a qualitative model to analyze the significant system behaviors, such as, cycles, stable states, and paths that lead to recovery and pathogenesis. Therefore, extensive analysis of the resulting model is performed to find these paths. This was followed by a prediction of cycles that symbolize the condition of homeostasis in the overall system, along with deadlock states that reveal the over-expression of the entities, from where the system can't move towards recovery. The results of our study highlight the reciprocal and persistent activation of TGF-β1 and MMP-9 as salient contributors in pathogenesis. Finally, we convert the model into a continuous petri net (PN) to study the evolution of proteins and their relative expression levels based on time. Together, our findings suggest that the TGF-β1 and the MMP-9 feedback loop is critical in tumor progression and targeting these mediators may represent a novel therapeutic strategy to make progress in the treatment of cancer.
A biological system is comprised of numerous elements that are tightly regulated through signaling networks in which the expression level of an entity may either trigger or inhibit the synthesis rate of additional entities or itself. Generally, conventional models (such as ODE and PDE models) are used to represent the entities in a biological system through differential equations that implicate time derivatives for the estimation of various quantities like levels of concentrations, the rate of reactions and temperatures. These models are continuous in nature and rely on specific kinetic values, which are typically not available for all the entities in the network and have been assumed. Hence, the modeling approaches based on graph theory make use of linear methods to observe the behaviors exhibited by biological systems. The methods employed in the current study are divided into consequent sub-sections presented in Figure 2.
In the 70s, a method of qualitative modeling framework was put forward by Rene` Thomas based on Boolean logic to model BRNs [26,27]. The framework comprises various qualitative parameters and thresholds with discrete variables, having only two levels of expression (0 or 1) that can be used to analyze the dynamics of a biological regulatory network. This approach makes use of interaction graphs to imply the basic topology of a BRN.
Definition 1 (directed graph): "A directed graph is an ordered pair D (V, E), where:
Ⅰ. V is the set of all nodes and
Ⅱ.E⊆V × V is the set of ordered pairs called edges or arcs"
An edge e.g. (a, b) is directed from an entity or node "a" to "b", where "a" is the tail and "b" is the head of that respective edge. In a directed graph, D-(x) and D+(x) denote the set of predecessors and successors of a specific node xϵV, respectively".
Definition 2 (biological regulatory network): "A BRN is a labeled directed graph D (V, E), where V is a set of nodes which represents biological entities and E ⊆ V × V is a set of all possible edges, which represent the interaction between entities".
Ⅰ. "Each edge can be labeled with a pair of variables (σ, ψ), where σ represents the qualitative threshold levels and is a positive integer and ψ is "+" or "–" representing the type of interaction, which can either be "activation" or "inhibition", respectively".
Ⅱ. "Each node e.g. "a" has a limit (la), in its threshold level, which is equal to its out-degree (the total number of outgoing edges from "a"). This relation can be presented by ∀bϵD+(a) and σabϵ{1, 2, 3, ….., ra} where ra ≤ la which means that the threshold levels of entity "a" can be set within a range "1" to "total number of outgoing edges" and because it has only one outgoing edge towards predecessor "b" so the threshold level which can be set for it can only be "1" ".
Ⅲ. "Each entity, e.g. "a", has its abstract expression in the set Za = {0, 1, 2, …., ra}".
Definition 3 (states): "The state of a BRN is a tuple sϵM, where M in terms of entity "a" is:
M=ΠaϵVZa |
The qualitative states are represented by vector (Mv)aϵV, where v denotes the level of expression of an entity like "a". According to this definition, M is the Cartesian product of the sets of abstract expressions of all entities. A qualitative state represents a configuration of all the elements of a BRN at any instant of time. The number of activators of a variable at a given level of expression is represented by its set of resources (see the definition of resources given below)".
Definition 4 (resources). "The set of resources Rva of a variable aϵV at a level v is defined as Rva={bϵD−(a)|vb≥σbaandψba=+)or(vb<σbaandψba=−)}. The dynamic behaviors of BRN depend on logical parameters. The set of these logical parameters is defined as K(D)={Ka(Rva)ϵZa∀aϵV}.
The parameter Ka(Rva) (at a level v of a) gives the information about the evolution of a. There are three cases: 1) if va > Ka(Rva) then va increases by one unit 2) if va > Ka(Rva) then va decreases by one unit and 3) if c = Ka(Rva) then va cannot evolve from its current level. It is convenient to describe the evolution from one level to another by an evolution operator "↱" [68], which is defined in terms of entity "a" as follows:
va↱Ka(Rva)={va+1ifva<Ka(Rva):va−1ifva>Ka(Rva):,vaifva=Ka(Rva).} |
Where va and Ka(Rva)∈Z≥0
Definition 5 (state graph): "Let D be the BRN and va represents the expression level of an entity e.g. "a" in a state sϵM. Then the state graph of the BRN will be the directed graph G = (S, T), where S is set of states and T⊆S×S represents a relation between states, called the transition relation, such that s→s'ϵT if and only if:
Ⅰ Ǝ a unique aϵV such that sva≠sva' and sva' = sva ↱ Ka(Rva) and
Ⅱ ∀bϵV\{a}svb' = svb"
Computation tree logic (CTL) formulae are formed using various state and path quantifiers. State quantifiers represent the state which holds a property, wherein the path quantifier defines a path from the present state, where a property holds [22].
Following are the descriptions of the path and state quantifiers:
∀: This quantifier is read as "For all paths". This state quantifier enforces that all paths initiating from the present state must be holding the given property.
∃: This is read as "There exists a path". It specifies that a minimum of one path starting from the present state must be holding the given characteristic.
◻: This state quantifier is read as "globally", which specifies that in a path initiating from a current state, all the states, must hold the given property, including the current state.
O: This quantifier is read as "Next". It enforces that the state which is the immediate successor of the present state must be holding the given property.
◊_: This is a state quantifier which specifies that in a path initiating from a current state, that state or at least one of the future states must hold the given property. This is known as the "Future Quantifier".
SMBioNet [23] is software for estimating parameters by employing the approach of Bernot et al. [24]. The BRN based on Rene Thomas formalism, where CTL formulae and the set of interactions in the form of activation and inhibition are provided to this software. Furthermore, it computes the probable sets of parameters which are further confirmed by a model checking software, NuSMV [25]. NuSMV employs the Snoussi and observability constraints to select the parameter combinations according to the CTL formula. The combinations are further validated to select the models which best suits the behaviors exhibited by our BRN.
Definition 6 (betweenness centrality): "For a state graph R = (S, T) of an interaction graph G = (V; E), let x, y and z be the distinct qualitative states in R, and let σx, y be the total number of trajectories from state x to state y, and let σy, x be the total number of trajectories from qualitative state y to x, passing through a state z. Let Ox represents the set of all ordered pairs, (y, x) such that x, y, and z are all distinct. Then, the betweenness centrality of the qualitative state z can be computed from the following equation:"
Vy(c)=∑(a,b)∈Oσa,b(c)σa,b |
A BRN is constructed based on the Boolean logic formalism established by Rene Thomas to model the interplay between TGF-β1 and MMP-9 in hepatocellular carcinoma using the GINSIM version 2.4 [26] (available at http://ginsim.org/downloads) and GENOTECH tools [27] (https://github.com/DrJamilAhmad/GENOTECH/blob/master/GenoTechE.jar). The BRN comprises a set of nodes that represent the biological entities, connected by edges that are directed from one node/vertex to another [24]. The edges represent positive and negative interactions amongst the entities. Positive interactions (illustrated by + 1) depict activation, while negative interactions (illustrated by -1) depict inhibition. Experimental knowledge is encrypted in a model checker (SMbioNET) to assign logical parameters to each entity. Furthermore, the BRN is simulated as a state graph to identify the important trajectories, cycles (homeostasis) and steady states (disease progression). The network analysis is performed in Cytoscape, to identify various paths leading to a pathogenic state or the state of homeostasis.
A PN is a directed bipartite graph where places (illustrated by circles) and transitions (illustrated by squares) denote entities of a pathway and the processes amongst them, respectively. Moreover, the transition and places are connected by directed arcs to allow the flow of tokens in the pathway. By firing transitions, the source can affect the number of tokens consigned to the target, called the token-count. Therefore, this enables the signals to circulate through the directed protein interactions in a cellular pathway.
For the analysis of signaling networks, PN has emerged as a reliable tool. This approach allows the user to vary inputs, to create a flow of signal through the network based on connectivity of the network, eliminating the need for kinetic parameters. So we employed this approach by converting our BRN to a continuous PN to study the system behavior in a continuous and timed manner.
Formally, continuous PNs formalism is defined as follows:
Definition 7 (continuous petri nets): "A continuous petri net is a quintuple CPN = (P, T, f, v, m0), where:
P, T is finite, non-empty, disjoint sets. P is the set of continuous places. T is the set of continuous transitions."
"f: ((P × T) ∪ (T × P)) → R0 + defines the set of directed arcs, weighted by non-negative integer values"
"v: T→H is a function, which assigns a firing rate function ht to each transition t, whereby H: = ∪t ∈T {ht | ht : R|•t|→R +} is the set of all firing rate functions, v(t) = ht for all transitions t ∈ T, and |•t| represents the cardinality of the preplaces of transition t (reaction's precursor)"
"m0: P→R0 + gives the initial marking."
We use the tool GINsim [26] to export a standard PN file which can be imported in SNOOPY [28] for further analysis.
A detailed literature review was carried out to identify pathways and entities that are critical in inducing the feedback loop between TGF-β1 and MMP-9. The extended pathway shown in Figure 1 was further reduced to a BRN (Figure 3) such that the impact of interactions amongst all the entities was significantly highlighted. This particular method of abstraction is explained in detail by Naldi et al 2009 and Saadatpour et al [35,36]. The reduced BRN comprises many well-characterized regulatory motifs that give rise to a particular functionality of the system. The BRN is constructed with five nodes representing the entities: TGF-β1, MMP-9, NFκB, TIMP-1 and HSC. The integers, + 1 and -1 are labeled with directed edges to represent activation (illustrated with a straight line) and inhibition (illustrated with a red dashed line) induced by the cellular and non-cellular components. The BRN highlights a positive feedback loop between TGF-β1 and MMP-9 through reciprocal activation. This loop exerts certain changes that direct the system to move away from its state of equilibrium, causing instability and eventually leading towards the state of disease i.e., HCC. A positive feedback loop consists of an even set of positive elements [31]. Whereas, a negative feedback loop is composed of an odd set of negative interactions, for instance, the interaction found between timp-1 and HSC tend to move towards either homeostasis or an interrupted behavior. Analysis of these motifs may provide significant insights into the probable dynamics of a system. The presence of TIMP-1 negatively regulates the expression of MMP-9, while its production is positively regulated by HSCs. Nonetheless, the dynamic behavior of a complex system, that comprises both negative and positive feedback loops, can be rendered with an appropriate set of parameters.
The constructed BRN has 5 entities: TGF-β1, MMP-9, NFκB, TIMP-1 and HSC (Figure 3). Each of these five entities comprises several discrete parameters that show the level of each attribute implicated in the model.
SMBioNet (selection of models of biological networks) [23] has been applied to compute parameters according to known qualitative observations in the form of computational tree logic (CTL) formulae (Table 1).
experimental observations | references | CTL formula |
Expression of TGF-β is often increased in HCC | [32] | (TGF=0 ∧NFKB=0 ∧HSC=0 ∧TIMP =0 ∧MMP=0) →(∀◊_∀ ◻TGF=1 ∧NFKB =1 ∧HSC=1 ∧TIMP =1 ∧MMP=1))) |
Overexpression of MMP-9 has been correlated to the invasiveness and metastasis of HCC | [33] | |
TGF-β1 and MMP-9 can promote the progression of HCC via stimulating HSCs | [39] | |
NF-κB is a key transcription factor in the regulation of MMP9 expression | [34] | |
Overexpression of TIMP-1 have been associated with invasion and metastasis in hepatocellular carcinoma | [35] |
It takes BRN as inputs and selects the models which verify these formulae. The formula was thoughtfully derived from biological observations indicating an increase in expression from an initial state with the help of state and path quantifiers. The CTL formula is based on the fact that the enhanced expression of TGF-β1, MMP-9, and TIMP-1 is often associated with invasiveness and metastasis of [13,32,35]. TGF-β1 and MMP-9 are known to promote the progression of HCC via stimulating HSCs and NF-κB is a key transcription factor in the regulation of MMP-9 expression [35]. Hence, the CTL formula encodes these observations that during HCC pathogenesis all the entities are upregulated due to dysregulation of the tightly regulated elements in the network (i.e., TGF-β1 = 1, MMP-9 = 1, TIMP-1 = 1). The analysis from SMBioNet resulted in four sets of parameters in the form of models which satisfied the CTL formula's properties (Table 2).
model 1 | model 2 | model 3 | model 4 |
K_TGF = 0 | K_TGF = 0 | K_TGF = 0 | # K_TGF = 0 |
# K_TGF + MMP = 1 | # K_TGF + MMP = 1 | # K_TGF + MMP = 1 | # K_TGF + MMP = 1 |
# K_NFKB = 0 | # K_NFKB = 0 | # K_NFKB = 0 | # K_NFKB = 0 |
# K_NFKB + TGF = 1 | # K_NFKB + TGF = 1 | # K_NFKB + TGF = 1 | # K_NFKB + TGF = 1 |
# K_HSC = 0 | # K_HSC = 0 | # K_HSC = 0 | # K_HSC = 0 |
# K_HSC + TGF = 1 | # K_HSC + TGF = 1 | # K_HSC + TGF = 1 | # K_HSC + TGF = 1 |
# K_HSC + TIMP = 0 | # K_HSC + TIMP = 0 | # K_HSC + TIMP = 0 | # K_HSC + TIMP = 0 |
# K_HSC + TGF + TIMP = 1 | # K_HSC + TGF + TIMP = 1 | # K_HSC + TGF + TIMP = 1 | # K_HSC + TGF +TIMP = 1 |
# K_TIMP = 0 | # K_TIMP = 0 | # K_TIMP = 0 | # K_TIMP = 0 |
# K_TIMP + HSC = 1 | # K_TIMP + HSC = 1 | # K_TIMP + HSC = 1 | # K_TIMP + HSC = 1 |
# K_TIMP + NFKB = 0 | # K_TIMP + NFKB = 0 | # K_TIMP + NFKB = 0 | # K_TIMP + NFKB = 0 |
# K_TIMP + HSC + NFKB = 1 | # K_TIMP + HSC + NFKB = 1 | # K_TIMP + HSC + NFKB = 1 | # K_TIMP+HSC +NFKB = 1 |
# K_MMP = 0 | # K_MMP = 0 | # K_MMP = 0 | # K_MMP = 0 |
# K_MMP + HSC = 0 | # K_MMP + HSC = 0 | # K_MMP + HSC = 0 | # K_MMP + HSC = 0 |
# K_MMP + NFKB = 0 | # K_MMP + NFKB = 0 | # K_MMP + NFKB = 0 | # K_MMP + NFKB = 0 |
# K_MMP + TGF = 0 | # K_MMP + TGF = 0 | # K_MMP + TGF = 0 | # K_MMP + TGF = 0 |
# K_MMP + TIMP = 1 | # K_MMP + TIMP = 1 | # K_MMP + TIMP = 1 | # K_MMP + TIMP = 1 |
# K_MMP + HSC + NFKB = 0 | # K_MMP + HSC + NFKB = 1 | # K_MMP + HSC + NFKB = 0 | # K_MMP + HSC +NFKB = 1 |
# K_MMP + NFKB + TGF = 0 | # K_MMP + NFKB + TGF = 0 | # K_MMP + NFKB + TGF = 1 | # K_MMP + NFKB +TGF = 1 |
# K_MMP + NFKB +TIMP = 1 | # K_MMP + NFKB + TIMP = 1 | # K_MMP + NFKB + TIMP = 1 | # K_MMP + NFKB +TIMP = 1 |
# K_MMP + HSC + TGF = 1 | # K_MMP + HSC + TGF = 1 | # K_MMP + HSC + TGF = 1 | # K_MMP + HSC +TGF = 1 |
# K_MMP + TGF + TIMP = 1 | # K_MMP + TGF + TIMP = 1 | # K_MMP + TGF + TIMP = 1 | # K_MMP + TGF +TIMP = 1 |
# K_MMP + HSC + TIMP = 1 | # K_MMP + HSC + TIMP = 1 | # K_MMP + HSC + TIMP = 1 | # K_MMP + HSC +TIMP = 1 |
#K_MMP + HSC + NFKB +TIMP = 1 | #K_MMP + HSC + NFKB +TIMP = 1 | #K_MMP + HSC + NFKB +TIMP = 1 | #K_MMP + HSC +NFKB + TIMP = 1 |
#K_MMP + HSC + NFKB +TGF = 1 | #K_MMP + HSC + NFKB +TGF = 1 | #K_MMP + HSC + NFKB +TGF = 1 | #K_MMP + HSC +NFKB + TGF = 1 |
#K_MMP + HSC + TGF +TIMP = 1 | #K_MMP + HSC + TGF +TIMP = 1 | #K_MMP + HSC + TGF +TIMP = 1 | #K_MMP + HSC +TGF + TIMP = 1 |
#K_MMP + NFKB + TGF+ TIMP = 1 | #K_MMP + NFKB + TGF +TIMP = 1 | #K_MMP + NFKB + TGF +TIMP = 1 | #K_MMP + NFKB +TGF + TIMP = 1 |
#K_MMP + HSC + NFKB +TGF + TIMP = 1 | #K_MMP + HSC + NFKB +TGF + TIMP = 1 | #K_MMP + HSC + NFKB +TGF + TIMP = 1 | #K_MMP + HSC +NFKB + TGF +TIMP = 1 |
Amongst these, the fourth set was selected. The fourth model was selected based on prior knowledge from the literature that in the presence of its activators, MMP-9 is upregulated as the HSCs frequently secrete the protein along with TGF-β and NFκB that too are responsible for the activation of MMP-9. In qualitative modeling, these selected parameters aid in observing the system dynamics in the form of cycles, stable states (SS) and paths. The selected parameters are presented in Table 3.
Parameter | Resources | Range of Values | Selected Parameters |
KTGF | {} | 0 | 0 |
KTGF | {MMP} | 1 | 1 |
KNFΚB | {} | 0 | 0 |
KNFΚB | {TGF} | 1 | 1 |
KHSC | {} | 0 | 0 |
KHSC | {TGF} | 0, 1 | 1 |
KHSC | {TIMP} | 0, 1 | 0 |
KHSC | {TGF, TIMP} | 0, 1 | 1 |
KTIMP | {} | 0 | 0 |
KTIMP | {HSC} | 0, 1 | 1 |
KTIMP | {NFκB} | 0, 1 | 0 |
KTIMP | {HSC, NFκB} | 0, 1 | 1 |
KMMP | {} | 0 | 0 |
KMMP | {HSC} | 0, 1 | 0 |
KMMP | {NFκB} | 0, 1 | 0 |
KMMP | {TGF} | 0, 1 | 0 |
KMMP | {TIMP} | 0, 1 | 1 |
KMMP | {HSC, NFκB} | 0, 1 | 1 |
KMMP | {NFκB, TGF} | 0, 1 | 1 |
KMMP | {NFκB, TIMP} | 0, 1 | 1 |
KMMP | {HSC, TGF} | 0, 1 | 1 |
KMMP | {TGF, TIMP} | 0, 1 | 1 |
KMMP | {HSC, TIMP} | 0, 1 | 1 |
KMMP | {HSC, NFκB, TIMP} | 0, 1 | 1 |
KMMP | {HSC, NFκB, TGF} | 0, 1 | 1 |
KMMP | {HSC, TGF, TIMP} | 0, 1 | 1 |
KMMP | {NFκB, TGF, TIMP} | 0, 1 | 1 |
KMMP | {HSC, NFκB, TGF, TIMP} | 0, 1 | 1 |
A state graph of the BRN was simulated from the selected parameters (Table 3) using the GINsim software [26]. The logical set of parameters of the four models, which satisfied the CTL properties, were analyzed using the state graph (as shown in Figure 4, starting from M1 to M4). In this representation, a logical variable associated with each pertinent element of the system where "0" represents the normal to down-regulation of an entity, whereas "1" represents up-regulation of an entity. Following model selection, the simulated state graph consists of 32 nodes and 80 edges which were rendered using Cytoscape [36] (available at http://www.cytoscape.org/) (Figure 4) and the states were sorted on the basis of betweenness centrality. The state graph represents all possible transitions in the form of states that denote relative expressions of all entities at a specific time. The order of the qualitative states is TGF-β1, NFκB, MMP-9, HSC, and TIMP-1. The deadlock state (1, 1, 1, 1, 1) shows up-regulation of the all the entities. The model also generates several cycles, computed by GENOTECH software, which reveals normal system behavior categorized by down-regulation of TGF-β1 and MMP-9, with a normal expression of other entities. The state of the system, in a state graph, is denoted by a vector that includes the level of expression of all the entities. The analysis revealed that the system can either lead to a disease state or a recovery state. The recovery state is denoted as (TGF-β1 = 0, NFκB = 0, MMP9 = 0, HSC = 0, TIMP1 = 0), where all the entities exhibit low expression. On the contrary, the pathogenic state is indicated by high expressions of TGF-β1 and MMP-9 that induce the overexpression of the other entities leading to a deadlock. Under normal circumstances, biological systems maintain homeostasis where the system remains in a cycle. Hence, a desired qualitative model should demonstrate a closed path showing homeostasis along with trajectories that lead to pathogenesis. It is also revealed through our analysis that there is one significant state which results in either a deadlock state or can lead to recovery. This state (TGF-β1 = 1, NFκB = 0, MMP9 = 1, HSC = 0, TIMP1 = 1) as shown in Figure 4 highlights that TGF-β1 and MMP-9 exhibit high expression levels, but they seem to be regulated by the expression TIMP-1, which is an inhibitor of MMP-9. As soon as TIMP-1 is down-regulated, both the entities overexpress each other resulting in the overexpression of NF-kappaB. The expression of MMP-9 is further upregulated by the high expression level of NF-kappaB. Also, NF-κB is known to augment the invasive ability of tumor cells [37]. This indicates that the system moves toward HCC pathogenesis. On the other hand, if TGF-β1 or MMP-9's expression is down-regulated the system may move towards the state of recovery, indicating the fact that inactivation of MMP-9 is crucial to recovering from the disease. Since MMPs play a key role in stimulating tumor metastasis and angiogenesis [38], it is confirmed by our results that agents required for down-regulating the expression levels may prove useful in handling HCC treatment outcome.
It is significant to identify the most apparent biological cycle since homeostasis is represented by cycles. Our model generated 30 cycles of varying lengths and one of them was selected on the basis of betweenness centrality rendered in the Cytoscape tool [36]. Betweenness centrality is defined as "the number of shortest paths that go through a node among all shortest paths between all possible pairs of nodes" [39]. All states were sorted based on their measure of betweenness centrality as presented in Figure 5, where nodes with a larger diameter and darker color signify states with high betweenness centrality. Amongst all the cycles, the cycle with highest betweenness centrality was as follows: (0, 0, 1, 0, 1) → (1, 0, 1, 0, 1) → (1, 0, 0, 0, 1) → (1, 0, 0, 0, 0) → (1, 0, 0, 1, 0) → (1, 1, 0, 1, 0) → (1, 1, 0, 1, 1) → (0, 1, 0, 1, 1) → (0, 1, 1, 1, 1) → (0, 0, 1, 1, 1) → (0, 0, 1, 0, 1).
The cycle initiates at the point where the expression of MMP-9 is tightly regulated by its inhibitor (TIMP-1). In the following state, the expression profile of TGF-β1 shifts from down-regulation to up-regulation. As soon as this state is met, MMP-9 is downregulated in the next state of the cycle, preventing activation of the loop and signaling the system to remain in a regulated cycle. The cycle also reveals that down-regulation of either TGF-β1 or MMP-9 is essential for homeostasis. Any deviation from this cycle would head towards a pathogenic stable state i.e., (1, 1, 1, 1, 1).
It is very challenging to find defined kinetic parameters for each reaction; hence we depend upon the PN model so that we are not restricted to certain values and expressions. The signaling pathways in biology have evolved to an extent where the network connectivity has an alleviating effect on the systems' entities and their associated interactions. Hence, the PN analysis results in an easy interpretation of the simulations run to predict the biological systems behavior under variable stimuli, be it internal or external.
The BRN modeled in GINSIM was exported into a standard petri nets (PN) format where the places and transitions were discrete in nature. Since the biological entities tend to be continuous in nature, the standard petri net was then converted into a continuous petri net using SNOOPY tool (version 2) (Figure 6). Modelling with PN involves the use of simulations to study the changes in relative expression levels of biological entities i.e., genes/proteins over time. There are two places for each network entity in the converted PN, i.e., active and inactive state. The activated states are represented by a prime place, and the inactivated state is shown by a complementary state. Furthermore, the related transitions are labeled as "p" and "n" to represent the activating and inactivating signals, respectively. The transitions are created by GINsim to model the parameters generated in SMBioNet. This aids in analyzing the BRN dynamics over time. Over the course of disease or homeostasis, the biological entities are activated in a variable manner. Hence, the need to include inactivated places for proteins is significant so to understand the inhibition signals that are received intracellularly. The resulting PN in our study is simulated on the basis of mass action kinetics for continuous transitions, where the presence of tokens is represented by the markings in the places.
Simulation makes obvious the time-based changes of the concentration of molecules and thus enables to graphically represent their paths and check the refined properties of the system [40]. Figure 7 shows the simulation results in the case of recovery where MMP-9 and TGF-β decline towards the state where the system maintains homeostasis leading to the downregulation of all the other entities (i.e., HSCs, NFκB, and TIMP-1). It reveals that the downregulation of MMP-9 results in lowering the expression of TGF-β1. Furthermore, NFκB also declines towards homeostasis mediating the downregulation of TIMP-1 to recover from the pathogenic state.
In contrast, Figure 8 depicts a clear picture of how all the entities are increasing towards the disease state and then becomes constant as it reaches a pathogenic stable state. It is observed that the overexpression of NFκB results in an increase in MMP-9 that may enhance invasion and progression. Moreover, high expression of MMP-9 and TGF-β1 can induce progression of HCC via induction of HSCs. Also, the relative expression level of MMP-9 is higher than that of TIMP-1 in the simulation result. It can be clearly seen in Figure 8 how the overexpression of MMP-9 leads to the activation and upregulation of TGF-β confirming our hypothesis where the reciprocal activation of both entities is considered as the potential molecular mechanism in accelerating the progression of HCC.
The proposed premise has been suggested in a recent study where the idea has been put forward for further analysis [13]. The role of TGF-β1 has been highlighted in HCC by means of two mechanisms: First, as a paracrine or autocrine growth factor through an intrinsic activity and, second, by stimulating microenvironment changes by an extrinsic activity [41]. In various experimental models, inhibitors of TGF-β1 have revealed to hinder the progression and growth of HCC by modulating EMT. TGF-β inhibitors arise as a novel therapeutic approach, which targets the cancer growth and its microenvironment. As TGF-β is present at almost every stage of tumorigenesis, blocking it may have a more powerful effect than agents that block specific processes during the pathogenesis of HCC, such as neo-angiogenesis [42]. TGF-β inhibition is directed against the most central pathological processes which lead to chronic liver disease and consequently to HCC. Over-expression of MMP-9 in tumor progression via cell invasion has been correlated with invasion and metastasis. Inhibitors for suppressing the expression of MMP-9 have been revealed through recent studies [43,44,45]. Taken together, therapies targeting both mediators may lead to medical breakthroughs in the treatment of cancer.
A consistent relationship between TGF-β1 and MMP-9 has been well-established in studies carried out on various fibroblasts and cancer cells [13]. The high expression of MMP-9 has been associated to invasion and metastasis in multiple tumors because of its capacity to remodel the tissues via the ECM, along with its ability to cause angiogenesis and degradation of the basement membrane [46,47]. In HCC, increased mRNA and protein levels of MMP-9 have been associated with highly invasive cancer cells [5]. Moreover, TGF-β1 has proven to be an independent biomarker to indicate survival in HCC [48]. By directly activating various signaling pathways and transcription factors, TGF-β1 can upregulate the expression of MMP-9, leading to an increased proliferation in various tumor cells [16]. Similarly, the link between the two key players was demonstrated in some studies, that MMP-9 increases the TGF-β1 bioactivity by proteolytically cleaving the latent peptide of TGF-β1. Finally, this relationship between MMP-9 and TGF-β1 forms a potential regulatory feedback loop correlated with fibrogenesis and HCC via the activation of hepatic stellate cells (HSCs) [16]. Therefore, it is significant to identify the mechanism of the positive interplay in the TGFβ1-associated BRN that plays a key role in triggering the signaling pathway that leads to pathogenesis.
Formal modeling approaches are extensively applicable because of their computational capacity of testing exhaustively. These approaches are being used successfully over a few years for the purpose of modeling and validation of intricate systems in biology [49]. Boolean logic formalism is a well-established method for modeling a qualitative BRN that decrypts the dynamics of the qualitative model in the form of a directed graph. A qualitative state is represented by a node, whereas the edges demonstrate the interactions between the nodes [21,50,51]. The qualitative model of the TGFβ1-associated BRN was simulated as a state graph, which resulted in cycles and a deadlock stable state. The most apparent biological cycle was selected on the basis of betweenness centrality that shows the expression profile TGF-β1 and MMP-9 is tightly regulated by TIMP-1, which controls the up-regulation and down-regulation of both the entities throughout the cycle, so only one of the key players exhibits expression in a given state. On the other hand, the down-regulation of TIMP-1 results in the overexpression of the TGF-β1 and MMP-9 via reciprocal activation. This tends to lead the system towards a deadlock stable state (1, 1, 1, 1, 1).
To maintain homeostasis, the expression profiles of genes go through numerous levels (such as low or high) during the regulatory mechanisms. The regulation and activation of the TGF-β1 and MMPs is found to be perturbed in tumor tissues and has been associated with increased progression of the tumor [52,53]. It has been suggested that when the MMPs activate latent TGF-β1, they can mediate metastasis and invasion in the stroma by exhibiting intense tumor-promoting effects [54]. The deadlock state generated by our model exhibits this behavior, where MMP-9 and TGF-β1 are found to be overexpressed. In recent studies, activated TGF-β1 has been demonstrated to mediate the critical balance of the remodeling of extracellular matrix by modulating the expression level of TIMPs and MMPs which is demonstrated in the qualitative cycle [54]. The simulation from the continuous petri net (PN) model was also consistent with the finding that the reciprocal activation of both the major entities (i.e., TGF-β1 and MMP-9) is considered as the potential molecular mechanism in accelerating the progression of HCC. It also suggests that TGF-β inhibitors may be used as a novel therapeutic approach, which targets the cancer growth and its microenvironment. As TGF-β1 is present at almost every stage of tumorigenesis, blocking it may have a more powerful effect than agents that block specific processes during the pathogenesis of HCC, such as neo-angiogenesis [42]. Inhibitors for suppressing the expression of MMP-9 have been revealed through recent studies [43,44,45]. Taken together, further wet-lab based experiments are required to explore the roles of TGF-β1, MMP-9, and TIMP-1 to target the key players in the tumor microenvironment.
The impact of reciprocal activation of TGF-β1 and MMP-9 in HCC has been elucidated in prior experiments. However, the precise molecular mechanism behind this interplay has not been established yet. In this study, we employed a robust computational technique to analyze the loop between TGF-β and MMP-9, which triggers invasion and metastasis in HCC. We found that both entities must be expressed in a regulated manner and that the inactivation of MMP-9 may aid in restoring the system in a recovery state and preserve homeostasis. Moreover, when MMP-9 forms a positive regulatory loop with TGF-β1, the system approaches a point from where recovery is beyond the bounds of possibility i.e., the deadlock stable state. These findings propose that this loop serves as a significant mediator of EMT resulting in HCC growth and development. Taken together, these findings may aid in the development of treatment strategies that are designed to target both TGF-β and MMP-9. Our constructed model could aid in further understanding of the dynamics of the disease by adding and exploring other entities (genes/proteins/cells) involved in HCC. Furthermore, the model can be extended with necessary perturbations and interventions to observe the effect of the entities on expression patterns.
We thank our colleagues from Atta-ur-Rahman School of Applied Biosciences (ASAB) and Research Center for Modeling and Simulation (RCMS) who provided thoughtful insight and great expertise that assisted the research.
All authors declare no conflicts of interest in this paper.
[1] | Akhil NSB, Kumar V, Raj R, et al. (2023) Adoption of human resource sourcing strategies for managing supply chain performance during COVID-19 crisis: evidence from manufacturing companies. Int J Product Perform Manag. https://doi.org/10.1108/IJPPM-06-2023-0292 |
[2] |
Amado A, Cortez P, Rita P, et al. (2018) Research trends on Big Data in Marketing: A text mining and topic modeling based literature analysis. Eur Res Manag Bus Econ 24: 1–7. https://doi.org/10.1016/j.iedeen.2017.06.002 doi: 10.1016/j.iedeen.2017.06.002
![]() |
[3] |
Barbosa MW, Vicente ADLC, Ladeira MB, et al. (2018) Managing supply chain resources with big data analytics: a systematic review. Int J Logist Res Appl 21: 177–200. https://doi/10.1080/13675567.2017.1369501 doi: 10.1080/13675567.2017.1369501
![]() |
[4] |
Barrales-Molina V, Martínez-López FJ, Gázquez-Abad JC (2014) Dynamic marketing capabilities: Toward an integrative framework. Int J Manag Rev 16: 397–416. https://doi.org/10.1111/ijmr.12026 doi: 10.1111/ijmr.12026
![]() |
[5] |
Cao G, Duan Y, Banna ΑΕ (2019) A dynamic capability view of marketing analytics: Evidence from UK firms. Ind Mark Manag 76: 72–83. https://doi.org/10.1016/j.indmarman.2018.08.002 doi: 10.1016/j.indmarman.2018.08.002
![]() |
[6] | Chase CW (2013) Demand-driven forecasting: A structured approach to forecasting. New Jersey: John Wiley & Sons. https://doi.org/10.1002/9781118691861 |
[7] |
Chen D, Preston DS, Swink M (2015) How the use of big data analytics affects value creation in supply chain management. J Manag Inf Syst 32: 4–39. 10.1080/07421222.2015.1138364 doi: 10.1080/07421222.2015.1138364
![]() |
[8] |
Chowdhury LAM, Rana T, Akter M, et al. (2018) Impact of intellectual capital on financial performance: evidence from the Bangladeshi textile sector. J Account Organ Change 14: 429–454. https://doi.org/10.1108/JAOC-11-2017-0109 doi: 10.1108/JAOC-11-2017-0109
![]() |
[9] |
DuHadway S, Carnovale S, Hazen B (2019) Understanding risk management for intentional supply chain disruptions: risk detection, risk mitigation, and risk recovery. Ann Oper Res 283: 179–198. 10.1007/s10479-017-2452-0 doi: 10.1007/s10479-017-2452-0
![]() |
[10] |
Erevelles S, Fukawa N, Swayne L (2016) Big Data consumer analytics and the transformation of marketing. J Bus Res 69: 897–904. 10.1016/j.jbusres.2015.07.001 doi: 10.1016/j.jbusres.2015.07.001
![]() |
[11] |
Fagan JC (2014) The Suitability of Web Analytics Key Performance Indicators in the Academic Library Environment. J Acad Librariansh 40: 25–34. http://dx.doi.org/10.1016/j.acalib.2013.06.005 doi: 10.1016/j.acalib.2013.06.005
![]() |
[12] |
Fan SK, Lau RYK, Zhao JL (2015) Demystifying Big Data Analytics for Business Intelligence Through the Lens of Marketing Mix. Big Data Res 2: 28–32. https://doi.org/10.1016/j.bdr.2015.02.006 doi: 10.1016/j.bdr.2015.02.006
![]() |
[13] | Gangaraju PK, Raj R, Kumar V, et al. (2023) Financial performance in Industry 4.0 agile supply chains: evidence from manufacturing companies. TQM J. https://doi.org/10.1108/TQM-07-2023-0214 |
[14] |
Germann F, Lilien GL, Rangaswamy A (2013) Performance implications of deploying marketing analytics. Int J Res Mark 30: 114–128. 10.1016/j.ijresmar.2012.10.001 doi: 10.1016/j.ijresmar.2012.10.001
![]() |
[15] |
Ghadge A, Kaklamanou M, Choudhary S, et al. (2017) Implementing environmental practices within the Greek dairy supply chain: Drivers and barriers for SMEs. Ind Manag Data Syst 117: 1995–2014. https://doi.org/10.1108/IMDS-07-2016-0270 doi: 10.1108/IMDS-07-2016-0270
![]() |
[16] | Hassani H, Huang X, Silva E (2018) Digitalisation and big data mining in banking. Big Data Cogn Comput 2: 18. https://doi.org/10.3390/bdcc2030018 |
[17] |
Hajli N, Tajvidi M, Gbadamosi A, et al. (2020) Understanding market agility for new product success with big data analytics. Ind Mark Manag 86: 135–143. https://doi.org/10.1016/j.indmarman.2019.09.010 doi: 10.1016/j.indmarman.2019.09.010
![]() |
[18] | Hedin H, Hirvensalo I, Vaarnas M (2014) The Handbook of Market Intelligence: Understand, Compete and Grow in Global Markets. New Jersey: John Wiley & Sons. |
[19] |
Hung JL, He W, Shen J (2020) Big data analytics for supply chain relationship in banking. Ind Mark Manag 86: 144–153. https://doi.org/10.1016/j.indmarman.2019.11.001 doi: 10.1016/j.indmarman.2019.11.001
![]() |
[20] |
Jabbar A, Akhtar P, Dani S (2020) Real-time big data processing for instantaneous marketing decisions: A problematization approach. Ind Mark Manag 90: 558–569. https://doi.org/10.1016/j.indmarman.2019.09.001 doi: 10.1016/j.indmarman.2019.09.001
![]() |
[21] |
Jacobs A (2009) The pathologies of big data. Commun ACM 52: 36–44. https://doi.org/10.1145/1536616.1536632 doi: 10.1145/1536616.1536632
![]() |
[22] |
Jeon S, Hong B, Chang V (2018) Pattern graph tracking-based stock price prediction using big data. Future Gener Comput Syst 80: 171–187. http://dx.doi.org/10.1016/j.future.2017.02.010 doi: 10.1016/j.future.2017.02.010
![]() |
[23] |
Jha A, Sharma RRK, Kumar V, et al. (2022) Designing Supply Chain Performance System: A Strategic Study on Indian Manufacturing Sector. Supply Chain Manag 27: 66–88. https://doi.org/10.1108/SCM-05-2020-0198 doi: 10.1108/SCM-05-2020-0198
![]() |
[24] |
Kaufman BE (2015) The RBV theory foundation of strategic HRM: critical flaws, problems for research and practice, and an alternative economics paradigm. Hum Resour Manag J 25: 516–540. https://doi.org/10.1111/1748-8583.12085 doi: 10.1111/1748-8583.12085
![]() |
[25] |
Kaur P, Arora S (2015) Regression and Endogeneity Bias in Big Marketing Data. 4th International Conference on Eco-friendly Computing and Communication Systems, Procedia Computer Science 70: 41–47. 10.1016/j.procs.2015.10.025 doi: 10.1016/j.procs.2015.10.025
![]() |
[26] |
Kumar A, Shankar R, Aljohani NR (2020a) A big data driven framework for demand-driven forecasting with effects of marketing-mix variables. Ind Mark Manag 90: 493–507. https://doi.org/10.1016/j.indmarman.2019.05.003 doi: 10.1016/j.indmarman.2019.05.003
![]() |
[27] |
Kumar V, Verma P, Jha A, et al. (2020) Dynamics of a medium value consumer apparel supply chain key parameters. Int J Product Perform Manag 71: 445–476. https://doi.org/10.1108/IJPPM-10-2019-0501 doi: 10.1108/IJPPM-10-2019-0501
![]() |
[28] |
Kumar V, Verma P, So TC, et al. (2022) Managing supply chains during COVID-19 outbreak: a case of Hong Kong toy manufacturing company. J Humanit Logist Supply Chain Manag 12: 502–531. https://doi.org/10.1108/JHLSCM-10-2021-0109 doi: 10.1108/JHLSCM-10-2021-0109
![]() |
[29] |
Kumar V, Raj R, Verma P, et al. (2023) Assessing Risk and Sustainability Factors in Spice Supply Chain Management. Oper Manag Res, 1–20. https://doi.org/10.1007/s12063-023-00424-6 doi: 10.1007/s12063-023-00424-6
![]() |
[30] |
Lambert DM, Cooper MC, Pagh JD (1998) Supply chain management: implementation issues and research opportunities. Int J Logist Manag 9: 1–20. https://doi.org/10.1108/09574099810805807 doi: 10.1108/09574099810805807
![]() |
[31] |
Leeflang PS, Verhoef PC, Dahlstrφm P, et al. (2014) Challenges and solutions for marketing in a digital era. Eur Manag J 32: 1–12. https://doi.org/10.1016/j.emj.2013.12.001 doi: 10.1016/j.emj.2013.12.001
![]() |
[32] |
Lilien GL (2016) The B2B knowledge gap. Int J Res Mark 33: 543–556. https://doi.org/10.1016/j.ijresmar.2016.01.003 doi: 10.1016/j.ijresmar.2016.01.003
![]() |
[33] | Mikalef P, Krogstie J, Pappas IO, et al. (2019) Exploring the relationship between big data analytics capability and competitive performance: the mediating roles of dynamic and operational capabilities. Inf Manag 57: 103169. https://doi.org/10.1016/j.im.2019.05.004 |
[34] |
Morgan NA (2012) Marketing and business performance. J Acad Mark Sci 40: 102–119. https://doi.org/10.1007/s11747-011-0279-9 doi: 10.1007/s11747-011-0279-9
![]() |
[35] |
Ngai EW, Xiu L, Chau DC (2009) Application of data mining techniques in customer relationship management: a literature review and classification. Expert Syst Appl 36: 2592–2602. https://doi.org/10.1016/j.eswa.2008.02.021 doi: 10.1016/j.eswa.2008.02.021
![]() |
[36] | Raj R, Kumar V, Shah B (2023) Big data analytics adaptive prospects in sustainable manufacturing supply chain. Benchmarking: An International Journal. https://doi.org/10.1108/BIJ-11-2022-0690 |
[37] | Raguseo E, Vitari C, Pigni F (2020) Profiting from big data analytics: The moderating roles of industry concentration and firm size. Int J Prod Econ 229: 107758. https://doi.org/10.1016/j.ijpe.2020.107758 |
[38] |
Sachan S, Kumar V, Vardhan S, et al. (2023) Key supply chain strategies for post-COVID-19 recovery: evidence from an Indian smart furniture industry. Int J Emerg Mark 18: 1378–1396. https://doi.org/10.1108/IJOEM-12-2021-1926 doi: 10.1108/IJOEM-12-2021-1926
![]() |
[39] | Sakas DP, Giannakopoulos NT, Trivellas P (2023a) Exploring affiliate marketing's impact on customers' brand engagement and vulnerability in the online banking service sector. Int J Bank Mark. https://doi.org/10.1108/IJBM-01-2023-0009 |
[40] | Sakas DP, Giannakopoulos NT, Nasiopoulos DK, et al. (2023b) Assessing the Efficacy of Cryptocurrency Applications' Affiliate Marketing Process on Supply Chain Firms' Website Visibility. Sustainability 15: 7326. https://doi.org/10.3390/su15097326 |
[41] | Sakas DP, Giannakopoulos NT, Kanellos N, et al. (2023) Supply Chain Firms' Financial Performance Connection with Digital Marketing Website Data. Economic and Social Development: Book of Proceedings, 116–124. |
[42] | Sakas DP, Giannakopoulos NT, Margaritis M, et al. (2023d) Modeling Supply Chain Firms' Stock Prices in the Fertilizer Industry through Innovative Cryptocurrency Market Big Data. Int J Financial Stud 11: 88. https://doi.org/10.3390/ijfs11030088 |
[43] | Sathi M (2014) Engaging customers using big data: how Marketing analytics are transforming business. New York: Palgrave Macmillan. |
[44] | Sena V, Ozdemir S (2020) Spillover effects of investment in big data analytics in B2B relationships: what is the role of human capital? Ind Mark Manag 86: 77–89. https://doi.org/10.1016/j.indmarman.2019.05.016 |
[45] | Sharda R, Delen D, Turban E (2015) Business intelligence and analytics: Systems for decision support 10. London: Pearson. |
[46] |
Sharma R, Mithas S, Kankanhalli A (2014) Transforming decision-making processes: a research agenda for understanding the impact of business analytics on organizations. Eur J Inf Syst 23: 433–441. https://doi.org/10.1057/ejis.2014.17 doi: 10.1057/ejis.2014.17
![]() |
[47] |
Sivarajah U, Irani Z, Gupta S, et al. (2020) Role of big data and social media analytics for business to business sustainability: a participatory web context. Ind Mark Manag 86: 163–179. https://doi.org/10.1016/j.indmarman.2019.04.005 doi: 10.1016/j.indmarman.2019.04.005
![]() |
[48] |
Story V, O'Malley L, Hart S (2011) Roles, role performance, and radical innovation competencies. Ind Mark Manag 40: 952–966. https://doi.org/10.1016/j.indmarman.2011.06.025 doi: 10.1016/j.indmarman.2011.06.025
![]() |
[49] |
Tan W, Blake MB, Saleh I, et al. (2013) Social-network-sourced big data analytics. IEEE Internet Comput 17: 62–69. 10.1109/MIC.2013.100 doi: 10.1109/MIC.2013.100
![]() |
[50] |
Tang O, Musa SN (2011) Identifying risk issues and research advancements in supply chain risk management. Int J Prod Econ 133: 25–34. https://doi.org/10.1016/j.ijpe.2010.06.013 doi: 10.1016/j.ijpe.2010.06.013
![]() |
[51] | Trivellas P, Malindretos G, Reklitis P (2020) Implications of Green Logistics Management on Sustainable Business and Supply Chain Performance: Evidence from a Survey in the Greek Agri-Food Sector. Sustainability 12: 10515. https://doi.org/10.3390/su122410515 |
[52] |
Troisi Ο, Maione G, Grimaldi M, et al. (2020) Growth hacking: Insights on data-driven decision-making from three firms. Ind Mark Manag 90: 538–557. https://doi.org/10.1016/j.indmarman.2019.08.005 doi: 10.1016/j.indmarman.2019.08.005
![]() |
[53] |
Tseng PH, Liao CH (2015) Supply Chain Integration, Information Technology, Market Orientation and Firm Performance in Container Shipping Firms. Int J Logist Manag 26: 82–106. https://doi.org/10.1108/IJLM-09-2012-0088 doi: 10.1108/IJLM-09-2012-0088
![]() |
[54] | Verma P, Kumar V (2017) Conquering the Emerging Markets: Enhance Supply Chain and Diversified Firm Performance. LAP LAMBERT Academic Publishing. |
[55] |
Vorhies DW, Morgan NA (2005) Benchmarking marketing capabilities for sustainable competitive advantage. J Mark 69: 80–94. https://doi.org/10.1509/jmkg.69.1.80.55505 doi: 10.1509/jmkg.69.1.80.55505
![]() |
[56] |
Vorhies DW, Orr LM, Bush VD (2011) Improving customer-focused marketing capabilities and firm financial performance via marketing exploration and exploitation. J Acad Mark Sci 39: 736–756. https://doi.org/10.1007/s11747-010-0228-z doi: 10.1007/s11747-010-0228-z
![]() |
[57] | Waller MA, Fawcett SE (2013). Data science, predictive analytics, and big data: a revolution that will transform supply chain design and management. J Bus Logist 34: 77–84. https://doi.org/10.1111/jbl.12010 |
[58] |
Wamba SF, Gunasekaran A, Akter S, et al. (2017) Big data analytics and firm performance: Effects of dynamic capabilities. J Bus Res 70: 356–365. http://dx.doi.org/10.1016/j.jbusres.2016.08.009 doi: 10.1016/j.jbusres.2016.08.009
![]() |
[59] |
Wedel M, Kannan PK (2016) Marketing analytics for data-rich environments. J Mark 80: 97–121. https://doi.org/10.1509/jm.15.0413 doi: 10.1509/jm.15.0413
![]() |
[60] |
Wu X, Zhu X, Wu G, et al. (2014) Data mining with big data. IEEE Trans Knowl Data Eng 26: 97–107. https://doi.org/10.1109/TKDE.2013.109 doi: 10.1109/TKDE.2013.109
![]() |
[61] |
Zaridis A, Vlachos I, Bourlakis M (2021) SMEs strategy and scale constraints impact on agri-food supply chain collaboration and firm performance. Prod Plan Control 32: 1165–1178. https://doi.org/10.1080/09537287.2020.1796136 doi: 10.1080/09537287.2020.1796136
![]() |
[62] | Zheng K, Zhang Z, Song B (2020) E-commerce logistics distribution mode in big-data context: a case analysis of JD. COM. Ind Mark Manag 86: 154–162. https://doi.org/10.1016/j.indmarman.2019.10.009 |
[63] |
Zokaei K, Hines P (2007) Achieving consumer focus in supply chains. Int J Phys Distrib Logist Manag 37: 223–247. https://doi.org/10.1108/09600030710742434 doi: 10.1108/09600030710742434
![]() |
1. | Xingwei Xu, Jianweng Wang, Weiwei Ming, Ming Chen, Qinglong An, In-process tap tool wear monitoring and prediction using a novel model based on deep learning, 2021, 112, 0268-3768, 453, 10.1007/s00170-020-06354-y | |
2. | Yanli Wan, Xifu Wang, Quan Chen, Xingyun Lei, Yan Wang, Chongde Chen, Hongpu Hu, Tao Song, A disease category feature database construction method of brain image based on deep convolutional neural network, 2020, 15, 1932-6203, e0232791, 10.1371/journal.pone.0232791 | |
3. | Xingwei Xu, Xiang Li, Weiwei Ming, Ming Chen, A novel multi-scale CNN and attention mechanism method with multi-sensor signal for remaining useful life prediction, 2022, 169, 03608352, 108204, 10.1016/j.cie.2022.108204 | |
4. | Chao Che, Chengjie Zhou, Hanyu Zhao, Bo Jin, Zhan Gao, Fast and effective biomedical named entity recognition using temporal convolutional network with conditional random field, 2020, 17, 1551-0018, 3553, 10.3934/mbe.2020200 | |
5. | Bancha Yingngam, 2024, 9781394234165, 407, 10.1002/9781394234196.ch13 | |
6. | Santhi Palanisamy, K. Deepa, M. Sathya Sundaram, 2023, chapter 17, 9798369317181, 289, 10.4018/979-8-3693-1718-1.ch017 |
experimental observations | references | CTL formula |
Expression of TGF-β is often increased in HCC | [32] | (TGF=0 ∧NFKB=0 ∧HSC=0 ∧TIMP =0 ∧MMP=0) →(∀◊_∀ ◻TGF=1 ∧NFKB =1 ∧HSC=1 ∧TIMP =1 ∧MMP=1))) |
Overexpression of MMP-9 has been correlated to the invasiveness and metastasis of HCC | [33] | |
TGF-β1 and MMP-9 can promote the progression of HCC via stimulating HSCs | [39] | |
NF-κB is a key transcription factor in the regulation of MMP9 expression | [34] | |
Overexpression of TIMP-1 have been associated with invasion and metastasis in hepatocellular carcinoma | [35] |
model 1 | model 2 | model 3 | model 4 |
K_TGF = 0 | K_TGF = 0 | K_TGF = 0 | # K_TGF = 0 |
# K_TGF + MMP = 1 | # K_TGF + MMP = 1 | # K_TGF + MMP = 1 | # K_TGF + MMP = 1 |
# K_NFKB = 0 | # K_NFKB = 0 | # K_NFKB = 0 | # K_NFKB = 0 |
# K_NFKB + TGF = 1 | # K_NFKB + TGF = 1 | # K_NFKB + TGF = 1 | # K_NFKB + TGF = 1 |
# K_HSC = 0 | # K_HSC = 0 | # K_HSC = 0 | # K_HSC = 0 |
# K_HSC + TGF = 1 | # K_HSC + TGF = 1 | # K_HSC + TGF = 1 | # K_HSC + TGF = 1 |
# K_HSC + TIMP = 0 | # K_HSC + TIMP = 0 | # K_HSC + TIMP = 0 | # K_HSC + TIMP = 0 |
# K_HSC + TGF + TIMP = 1 | # K_HSC + TGF + TIMP = 1 | # K_HSC + TGF + TIMP = 1 | # K_HSC + TGF +TIMP = 1 |
# K_TIMP = 0 | # K_TIMP = 0 | # K_TIMP = 0 | # K_TIMP = 0 |
# K_TIMP + HSC = 1 | # K_TIMP + HSC = 1 | # K_TIMP + HSC = 1 | # K_TIMP + HSC = 1 |
# K_TIMP + NFKB = 0 | # K_TIMP + NFKB = 0 | # K_TIMP + NFKB = 0 | # K_TIMP + NFKB = 0 |
# K_TIMP + HSC + NFKB = 1 | # K_TIMP + HSC + NFKB = 1 | # K_TIMP + HSC + NFKB = 1 | # K_TIMP+HSC +NFKB = 1 |
# K_MMP = 0 | # K_MMP = 0 | # K_MMP = 0 | # K_MMP = 0 |
# K_MMP + HSC = 0 | # K_MMP + HSC = 0 | # K_MMP + HSC = 0 | # K_MMP + HSC = 0 |
# K_MMP + NFKB = 0 | # K_MMP + NFKB = 0 | # K_MMP + NFKB = 0 | # K_MMP + NFKB = 0 |
# K_MMP + TGF = 0 | # K_MMP + TGF = 0 | # K_MMP + TGF = 0 | # K_MMP + TGF = 0 |
# K_MMP + TIMP = 1 | # K_MMP + TIMP = 1 | # K_MMP + TIMP = 1 | # K_MMP + TIMP = 1 |
# K_MMP + HSC + NFKB = 0 | # K_MMP + HSC + NFKB = 1 | # K_MMP + HSC + NFKB = 0 | # K_MMP + HSC +NFKB = 1 |
# K_MMP + NFKB + TGF = 0 | # K_MMP + NFKB + TGF = 0 | # K_MMP + NFKB + TGF = 1 | # K_MMP + NFKB +TGF = 1 |
# K_MMP + NFKB +TIMP = 1 | # K_MMP + NFKB + TIMP = 1 | # K_MMP + NFKB + TIMP = 1 | # K_MMP + NFKB +TIMP = 1 |
# K_MMP + HSC + TGF = 1 | # K_MMP + HSC + TGF = 1 | # K_MMP + HSC + TGF = 1 | # K_MMP + HSC +TGF = 1 |
# K_MMP + TGF + TIMP = 1 | # K_MMP + TGF + TIMP = 1 | # K_MMP + TGF + TIMP = 1 | # K_MMP + TGF +TIMP = 1 |
# K_MMP + HSC + TIMP = 1 | # K_MMP + HSC + TIMP = 1 | # K_MMP + HSC + TIMP = 1 | # K_MMP + HSC +TIMP = 1 |
#K_MMP + HSC + NFKB +TIMP = 1 | #K_MMP + HSC + NFKB +TIMP = 1 | #K_MMP + HSC + NFKB +TIMP = 1 | #K_MMP + HSC +NFKB + TIMP = 1 |
#K_MMP + HSC + NFKB +TGF = 1 | #K_MMP + HSC + NFKB +TGF = 1 | #K_MMP + HSC + NFKB +TGF = 1 | #K_MMP + HSC +NFKB + TGF = 1 |
#K_MMP + HSC + TGF +TIMP = 1 | #K_MMP + HSC + TGF +TIMP = 1 | #K_MMP + HSC + TGF +TIMP = 1 | #K_MMP + HSC +TGF + TIMP = 1 |
#K_MMP + NFKB + TGF+ TIMP = 1 | #K_MMP + NFKB + TGF +TIMP = 1 | #K_MMP + NFKB + TGF +TIMP = 1 | #K_MMP + NFKB +TGF + TIMP = 1 |
#K_MMP + HSC + NFKB +TGF + TIMP = 1 | #K_MMP + HSC + NFKB +TGF + TIMP = 1 | #K_MMP + HSC + NFKB +TGF + TIMP = 1 | #K_MMP + HSC +NFKB + TGF +TIMP = 1 |
Parameter | Resources | Range of Values | Selected Parameters |
KTGF | {} | 0 | 0 |
KTGF | {MMP} | 1 | 1 |
KNFΚB | {} | 0 | 0 |
KNFΚB | {TGF} | 1 | 1 |
KHSC | {} | 0 | 0 |
KHSC | {TGF} | 0, 1 | 1 |
KHSC | {TIMP} | 0, 1 | 0 |
KHSC | {TGF, TIMP} | 0, 1 | 1 |
KTIMP | {} | 0 | 0 |
KTIMP | {HSC} | 0, 1 | 1 |
KTIMP | {NFκB} | 0, 1 | 0 |
KTIMP | {HSC, NFκB} | 0, 1 | 1 |
KMMP | {} | 0 | 0 |
KMMP | {HSC} | 0, 1 | 0 |
KMMP | {NFκB} | 0, 1 | 0 |
KMMP | {TGF} | 0, 1 | 0 |
KMMP | {TIMP} | 0, 1 | 1 |
KMMP | {HSC, NFκB} | 0, 1 | 1 |
KMMP | {NFκB, TGF} | 0, 1 | 1 |
KMMP | {NFκB, TIMP} | 0, 1 | 1 |
KMMP | {HSC, TGF} | 0, 1 | 1 |
KMMP | {TGF, TIMP} | 0, 1 | 1 |
KMMP | {HSC, TIMP} | 0, 1 | 1 |
KMMP | {HSC, NFκB, TIMP} | 0, 1 | 1 |
KMMP | {HSC, NFκB, TGF} | 0, 1 | 1 |
KMMP | {HSC, TGF, TIMP} | 0, 1 | 1 |
KMMP | {NFκB, TGF, TIMP} | 0, 1 | 1 |
KMMP | {HSC, NFκB, TGF, TIMP} | 0, 1 | 1 |
experimental observations | references | CTL formula |
Expression of TGF-β is often increased in HCC | [32] | (TGF=0 ∧NFKB=0 ∧HSC=0 ∧TIMP =0 ∧MMP=0) →(∀◊_∀ ◻TGF=1 ∧NFKB =1 ∧HSC=1 ∧TIMP =1 ∧MMP=1))) |
Overexpression of MMP-9 has been correlated to the invasiveness and metastasis of HCC | [33] | |
TGF-β1 and MMP-9 can promote the progression of HCC via stimulating HSCs | [39] | |
NF-κB is a key transcription factor in the regulation of MMP9 expression | [34] | |
Overexpression of TIMP-1 have been associated with invasion and metastasis in hepatocellular carcinoma | [35] |
model 1 | model 2 | model 3 | model 4 |
K_TGF = 0 | K_TGF = 0 | K_TGF = 0 | # K_TGF = 0 |
# K_TGF + MMP = 1 | # K_TGF + MMP = 1 | # K_TGF + MMP = 1 | # K_TGF + MMP = 1 |
# K_NFKB = 0 | # K_NFKB = 0 | # K_NFKB = 0 | # K_NFKB = 0 |
# K_NFKB + TGF = 1 | # K_NFKB + TGF = 1 | # K_NFKB + TGF = 1 | # K_NFKB + TGF = 1 |
# K_HSC = 0 | # K_HSC = 0 | # K_HSC = 0 | # K_HSC = 0 |
# K_HSC + TGF = 1 | # K_HSC + TGF = 1 | # K_HSC + TGF = 1 | # K_HSC + TGF = 1 |
# K_HSC + TIMP = 0 | # K_HSC + TIMP = 0 | # K_HSC + TIMP = 0 | # K_HSC + TIMP = 0 |
# K_HSC + TGF + TIMP = 1 | # K_HSC + TGF + TIMP = 1 | # K_HSC + TGF + TIMP = 1 | # K_HSC + TGF +TIMP = 1 |
# K_TIMP = 0 | # K_TIMP = 0 | # K_TIMP = 0 | # K_TIMP = 0 |
# K_TIMP + HSC = 1 | # K_TIMP + HSC = 1 | # K_TIMP + HSC = 1 | # K_TIMP + HSC = 1 |
# K_TIMP + NFKB = 0 | # K_TIMP + NFKB = 0 | # K_TIMP + NFKB = 0 | # K_TIMP + NFKB = 0 |
# K_TIMP + HSC + NFKB = 1 | # K_TIMP + HSC + NFKB = 1 | # K_TIMP + HSC + NFKB = 1 | # K_TIMP+HSC +NFKB = 1 |
# K_MMP = 0 | # K_MMP = 0 | # K_MMP = 0 | # K_MMP = 0 |
# K_MMP + HSC = 0 | # K_MMP + HSC = 0 | # K_MMP + HSC = 0 | # K_MMP + HSC = 0 |
# K_MMP + NFKB = 0 | # K_MMP + NFKB = 0 | # K_MMP + NFKB = 0 | # K_MMP + NFKB = 0 |
# K_MMP + TGF = 0 | # K_MMP + TGF = 0 | # K_MMP + TGF = 0 | # K_MMP + TGF = 0 |
# K_MMP + TIMP = 1 | # K_MMP + TIMP = 1 | # K_MMP + TIMP = 1 | # K_MMP + TIMP = 1 |
# K_MMP + HSC + NFKB = 0 | # K_MMP + HSC + NFKB = 1 | # K_MMP + HSC + NFKB = 0 | # K_MMP + HSC +NFKB = 1 |
# K_MMP + NFKB + TGF = 0 | # K_MMP + NFKB + TGF = 0 | # K_MMP + NFKB + TGF = 1 | # K_MMP + NFKB +TGF = 1 |
# K_MMP + NFKB +TIMP = 1 | # K_MMP + NFKB + TIMP = 1 | # K_MMP + NFKB + TIMP = 1 | # K_MMP + NFKB +TIMP = 1 |
# K_MMP + HSC + TGF = 1 | # K_MMP + HSC + TGF = 1 | # K_MMP + HSC + TGF = 1 | # K_MMP + HSC +TGF = 1 |
# K_MMP + TGF + TIMP = 1 | # K_MMP + TGF + TIMP = 1 | # K_MMP + TGF + TIMP = 1 | # K_MMP + TGF +TIMP = 1 |
# K_MMP + HSC + TIMP = 1 | # K_MMP + HSC + TIMP = 1 | # K_MMP + HSC + TIMP = 1 | # K_MMP + HSC +TIMP = 1 |
#K_MMP + HSC + NFKB +TIMP = 1 | #K_MMP + HSC + NFKB +TIMP = 1 | #K_MMP + HSC + NFKB +TIMP = 1 | #K_MMP + HSC +NFKB + TIMP = 1 |
#K_MMP + HSC + NFKB +TGF = 1 | #K_MMP + HSC + NFKB +TGF = 1 | #K_MMP + HSC + NFKB +TGF = 1 | #K_MMP + HSC +NFKB + TGF = 1 |
#K_MMP + HSC + TGF +TIMP = 1 | #K_MMP + HSC + TGF +TIMP = 1 | #K_MMP + HSC + TGF +TIMP = 1 | #K_MMP + HSC +TGF + TIMP = 1 |
#K_MMP + NFKB + TGF+ TIMP = 1 | #K_MMP + NFKB + TGF +TIMP = 1 | #K_MMP + NFKB + TGF +TIMP = 1 | #K_MMP + NFKB +TGF + TIMP = 1 |
#K_MMP + HSC + NFKB +TGF + TIMP = 1 | #K_MMP + HSC + NFKB +TGF + TIMP = 1 | #K_MMP + HSC + NFKB +TGF + TIMP = 1 | #K_MMP + HSC +NFKB + TGF +TIMP = 1 |
Parameter | Resources | Range of Values | Selected Parameters |
KTGF | {} | 0 | 0 |
KTGF | {MMP} | 1 | 1 |
KNFΚB | {} | 0 | 0 |
KNFΚB | {TGF} | 1 | 1 |
KHSC | {} | 0 | 0 |
KHSC | {TGF} | 0, 1 | 1 |
KHSC | {TIMP} | 0, 1 | 0 |
KHSC | {TGF, TIMP} | 0, 1 | 1 |
KTIMP | {} | 0 | 0 |
KTIMP | {HSC} | 0, 1 | 1 |
KTIMP | {NFκB} | 0, 1 | 0 |
KTIMP | {HSC, NFκB} | 0, 1 | 1 |
KMMP | {} | 0 | 0 |
KMMP | {HSC} | 0, 1 | 0 |
KMMP | {NFκB} | 0, 1 | 0 |
KMMP | {TGF} | 0, 1 | 0 |
KMMP | {TIMP} | 0, 1 | 1 |
KMMP | {HSC, NFκB} | 0, 1 | 1 |
KMMP | {NFκB, TGF} | 0, 1 | 1 |
KMMP | {NFκB, TIMP} | 0, 1 | 1 |
KMMP | {HSC, TGF} | 0, 1 | 1 |
KMMP | {TGF, TIMP} | 0, 1 | 1 |
KMMP | {HSC, TIMP} | 0, 1 | 1 |
KMMP | {HSC, NFκB, TIMP} | 0, 1 | 1 |
KMMP | {HSC, NFκB, TGF} | 0, 1 | 1 |
KMMP | {HSC, TGF, TIMP} | 0, 1 | 1 |
KMMP | {NFκB, TGF, TIMP} | 0, 1 | 1 |
KMMP | {HSC, NFκB, TGF, TIMP} | 0, 1 | 1 |