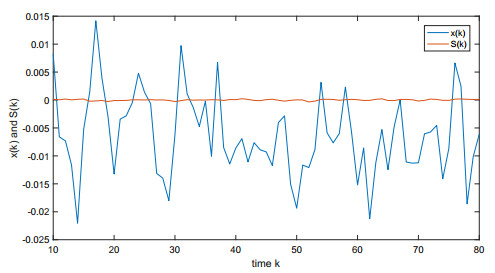
Citation: Tianwei Zhang, Zhouhong Li, Jianwen Zhou. 2p-th mean dynamic behaviors for semi-discrete stochastic competitive neural networks with time delays[J]. AIMS Mathematics, 2020, 5(6): 6419-6435. doi: 10.3934/math.2020413
[1] | M. Syed Ali, M. Hymavathi, Bandana Priya, Syeda Asma Kauser, Ganesh Kumar Thakur . Stability analysis of stochastic fractional-order competitive neural networks with leakage delay. AIMS Mathematics, 2021, 6(4): 3205-3241. doi: 10.3934/math.2021193 |
[2] | Zhengqi Zhang, Huaiqin Wu . Cluster synchronization in finite/fixed time for semi-Markovian switching T-S fuzzy complex dynamical networks with discontinuous dynamic nodes. AIMS Mathematics, 2022, 7(7): 11942-11971. doi: 10.3934/math.2022666 |
[3] | Tian Xu, Ailong Wu . Stabilization of nonlinear hybrid stochastic time-delay neural networks with Lévy noise using discrete-time feedback control. AIMS Mathematics, 2024, 9(10): 27080-27101. doi: 10.3934/math.20241317 |
[4] | Dong Pan, Huizhen Qu . Finite-time boundary synchronization of space-time discretized stochastic fuzzy genetic regulatory networks with time delays. AIMS Mathematics, 2025, 10(2): 2163-2190. doi: 10.3934/math.2025101 |
[5] | Nina Huo, Bing Li, Yongkun Li . Global exponential stability and existence of almost periodic solutions in distribution for Clifford-valued stochastic high-order Hopfield neural networks with time-varying delays. AIMS Mathematics, 2022, 7(3): 3653-3679. doi: 10.3934/math.2022202 |
[6] | Qingyi Cui, Changjin Xu, Wei Ou, Yicheng Pang, Zixin Liu, Jianwei Shen, Muhammad Farman, Shabir Ahmad . Further study on Hopf bifurcation and hybrid control strategy in BAM neural networks concerning time delay. AIMS Mathematics, 2024, 9(5): 13265-13290. doi: 10.3934/math.2024647 |
[7] | Qinghua Zhou, Li Wan, Hongbo Fu, Qunjiao Zhang . Exponential stability of stochastic Hopfield neural network with mixed multiple delays. AIMS Mathematics, 2021, 6(4): 4142-4155. doi: 10.3934/math.2021245 |
[8] | R. Sriraman, R. Samidurai, V. C. Amritha, G. Rachakit, Prasanalakshmi Balaji . System decomposition-based stability criteria for Takagi-Sugeno fuzzy uncertain stochastic delayed neural networks in quaternion field. AIMS Mathematics, 2023, 8(5): 11589-11616. doi: 10.3934/math.2023587 |
[9] | Jingyang Ran, Tiecheng Zhang . Fixed-time synchronization control of fuzzy inertial neural networks with mismatched parameters and structures. AIMS Mathematics, 2024, 9(11): 31721-31739. doi: 10.3934/math.20241525 |
[10] | Yijia Zhang, Tao Xie, Yunlong Ma . Robustness analysis of exponential stability of Cohen-Grossberg neural network with neutral terms. AIMS Mathematics, 2025, 10(3): 4938-4954. doi: 10.3934/math.2025226 |
Competitive neural networks (CNNs) simulate the cortical cognitive maps with unsupervised synaptic modifications. In the structure of CNNs, it has two kinds of states: one depicts the fast neural activity and the other one depicts the slow unsupervised synaptic modifications. Recently, CNNs had been extensively used in artificial intelligence, robotics, adaptive control and combinatorial optimization, etc. In the dynamical behaviours of CNNs, (almost) periodicity and exponential stability are extremely important. Consequently, many literatures [1,2,3,4,5,6,7,8] concerned synchronization, the existence and global exponential stability of equilibrium point, (almost) periodic solutions of CNNs. Such as, Wang and Huang [6] studied the CNNs below:
{˙xi(t)=−ai(t)xi(t)+n∑j=1bij(t)fj(xj(t))+n∑j=1cij(t)fj(xj(t−τij(t)))+Bi(t)p∑j=1mij(t)yj+Ii(t),˙mij(t)=−˜ai(t)mij(t)+˜bi(t)yj˜fi(xi(t)), | (1.1) |
where xi is the ith neuron, mij is the synaptic efficiency, fj and ˜fi are the activation functions, i,j=1,2,…,n. In [6], the authors studied the equilibrium point and exponential asymptotic stability for system (1.1).
In practical applications, stochastic disturbances frequently occur in NNs and the stochastic factors always happen in the uncertainties of the parameter process. Therefore, this paper concerns the SCNNs below:
{dxi(t)=[−ai(t)xi(t)+n∑j=1bij(t)fj(xj(t))+n∑j=1cij(t)fj(xj(t−τij(t)))+Bi(t)p∑j=1mij(t)yj+Ii(t)]dt+n∑j=1dij(t)σj(xj(t−ηij(t)))dwj(t),dmij(t)=[−˜ai(t)mij(t)+˜bi(t)yj˜fi(xi(t))]dt+˜di(t)mij(t)d˜wi(t), | (1.2) |
where wj and ˜wi are the standard Brownian motions, τij(t) and ηij(t) are bounded continuous functions with 0≤τij(t)≤ˉτij, 0≤ηij(t)≤ˉηij, ∀t∈R, ˉτij and ˉηij are positive constants, i,j=1,2,…,n.
The discreteness of NNs is a very effective tool to investigate the dynamics of the networks in a digital way. Therefore, lots of research findings were gained for the dynamics of discrete-time determinant or stochastic NNs by Euler scheme [9,10,11,12,13]. In recent years, the semi-discretization [14] of determinant differential equations received wide attentions and the authors [14,15,16,17,18] displayed that semi-discrete models provide more precise approximation of continuous-time models than those by Euler method. For example, the following semi-discrete NNs had been discussed in [16]:
xi(k+1)=e−ai(k)xi(k)+1−e−ai(k)ai(k)[n∑j=1bij(k)fj(xj(k))+Ii(k)],k∈Z,i=1,2,…,n. | (1.3) |
Assuming that all coefficients are almost periodic, the stable almost periodic sequence solution of system (1.3) was studied. Next, Huang et al. [18] studied the semi-discrete analogue of a general neural networks as follows:
xi(k+1)=e−ai(k)xi(k)+1−e−ai(k)ai(k)[m∑l=1n∑j=1bijl(k)fj(xj(k−τijl))+Ii(k)],k∈Z,i=1,2,…,n. |
In [18], some sufficient conditions were obtained for the existence of 2n locally stable almost periodic solutions of the above NNs.
In literatures [14,15,16,17,18], the disquisitive models are deterministic. To the best of authors' knowledge, few results study the semi-discrete stochastic NNs. The major contributions of this paper are as follows:
● Semi-discrete analogue of SCNNs (1.2) is achieved.
● A Volterra integral expression in difference form is got for semi-discrete model of SCNNs (1.2).
● Some new results are gained for 2p-th MAPS and MGES to semi-discrete stochastic model.
● The issues discussed in this article can motivate the researches of other discrete stochastic models.
Motivated by the above reasons, the aim of this literature is to achieve the semi-discrete SCNNs and the mean dynamic behaviours (MAPS and MGES) of the model should be discussed. The rest of this article is organized as follows. In Section 2, the semi-discrete model of system (1.2) is obtained and some preparatory works are given. In Section 3, the 2p-th MAPS of the semi-dicrete model is studied by fixed point theory. In Section 4, 2p-th MGES of the model is also discussed. An example and simulations are provided to expound the chief results of this paper in Section 5. Section 6 displays the conclusions and future perspectives of this article.
Denote by Rn the n-dimensional real vector space and (Ω,F,P) is a complete probability space. Let BC(Z,Lp(Ω,Rn)) be the space of all bounded continuous functions from Z to Lp(Ω,Rn), where Lp(Ω,Rn) is the space of all p-th integrable Rn-valued random variables. So BC(Z,Lp(Ω,Rn)) is a Banach space with ‖X‖p=supk∈Z|X|p, |X|p=max1≤i≤n(E|xi(k)|p)1p, ∀X={xi}:={x1,x2,…,xn}∈BC(Z,Lp(Ω,Rn)), where p>1 and E(⋅) stands for the expectation operator. [a,b]Z=[a,b]∩Z, ∀a,b∈R.
Letting Si(t)=p∑j=1mij(t)yj=yTmi(t), where y=(y1,y2,…,yp)T, mi=(mi1,mi2,…,mip)T, then system (1.2) is changed to
{dxi(t)=[−ai(t)xi(t)+n∑j=1bij(t)fj(xj(t))+n∑j=1cij(t)fj(xj(t−τij(t)))+Bi(t)Si(t)+Ii(t)]dt+n∑j=1dij(t)σj(xj(t−ηij(t)))dwj(t),dSi(t)=[−˜ai(t)Si(t)+˜bi(t)˜fi(xi(t))]dt+˜di(t)Si(t)d˜wi(t),i=1,2,…,n,k∈Z. | (2.1) |
Similar to the argument as that in [14], the semi-discrete analogue of system (2.1) is obtained as follows:
{xi(k+1)=e−ai(k)xi(k)+1−e−ai(k)ai(k)[n∑j=1bij(k)fj(xj(k))+n∑j=1cij(k)fj(xj(k−τij(k)))+Bi(k)Si(k)+Ii(k)+n∑j=1dij(k)σj(xj(k−ηij(k)))Δwj(k)],Si(k+1)=e−˜ai(k)Si(k)+1−e−˜ai(k)˜ai(k)[˜bi(k)˜fi(xi(k))+˜di(k)Si(k)Δ˜wi(k)],i=1,2,…,n,k∈Z. | (2.2) |
Definition 2.1. ([19]) For X∈BC(Z;Lp(Ω;Rn)) and each ϵ>0, there exists an integer l(ϵ)>0 such that each interval of length l(ϵ) contains an integer ω for which
supk∈ZE|X(k+ω)−X(k)|p<ϵ. |
Then stochastic process X is said to be p-th MAPS.
Lemma 2.1. ([20]) If X={xi} is a solution of system (2.2), then
{xi(k)=k−1∏s=k0e−ai(s)xi(k0)+k−1∑v=k0k−1∏s=v+1e−ai(s)[1−e−ai(v)]ai(v)[n∑j=1bij(v)fj(xj(v))+n∑j=1cij(v)fj(xj(v−τij(v)))+Bi(v)Si(v)+Ii(v)+n∑j=1dij(v)σj(xj(v−ηij(v)))Δwj(v)],Si(k)=k−1∏s=k0e−˜ai(s)Si(k0)+k−1∑v=k0k−1∏s=v+1e−˜ai(s)[1−e−˜ai(v)]˜ai(v)[˜bi(v)˜fi(xi(v))+˜di(v)Si(v)Δ˜wi(v)], | (2.3) |
where k0∈Z, k∈(k0,+∞)Z, i=1,2,…,n.
Lemma 2.2. ([21]) If g∈L2([a,b],R), then
E[supt∈[a,b]|∫tag(s)dω(s)|p]≤CpE[∫ba|g(t)|2dt]p2,Cp={(32/p)p/2,0<p<2,4,p=2,[pp+12(p−1)(p−1)]p2,p>2. |
Lemma 2.3. ([20]) If {x(k):k∈Z} is real-valued stochastic process and w(k) is the standard Brownian motion, then
E|x(k)Δw(k)|p≤CpE|x(k)|p,k∈Z, |
where Cp is defined as that in Lemma 2.2, p>0.
Let fu=supk∈Z|f(k)| and fl=infk∈Z|f(k)| for bounded function f defined on Z. In this paper, assume that the assumptions below hold:
(H1)ali>0 and ˜ali>0, i=1,2,…,n.
(H2) There are some positive constants Lfj, Lσj and L˜fi such that
|fj(u)−fj(v)|≤Lfj|u−v|,|σj(u)−σj(v)|≤Lσj|u−v|,|˜fi(u)−˜fi(v)|≤L˜fi|u−v|, |
for all u,v∈R, i,j=1,2,…,n.
Define
au:=max1≤i≤naui,al:=min1≤i≤nali,˜au:=max1≤i≤n˜aui,˜al:=max1≤i≤n˜ali, |
D∗:=max1≤i≤n[Bui+n∑j=1(buij+cuij)Lfj],˜D∗:=max1≤i≤n˜buiL˜fi,K∗:=max1≤i≤nn∑j=1duijLσj,˜K∗:=max1≤i≤n˜dui, |
r2p:=max{(1−e−au)al(1−e−al)(D∗+K∗C12p2p),(1−e−˜au)˜al(1−e−˜al)(˜D∗+˜K∗C12p2p)},β2p:=α2p1−r2p, |
where
α2p:=max{e2p,˜e2p},e2p:=(1−e−au)al(1−e−al)max1≤i≤n[n∑j=1(buij+cuij)|fj(0)|+Iui+n∑j=1duijσj(0)C12p2p], |
˜e2p:=(1−e−˜au)˜al(1−e−˜al)max1≤i≤n˜bui|˜fi(0)|. |
Lemma 3.1. ([22]) If X is a Banach space, Λ⊆X is closed and convex. Suppose further that B, C:Λ→X such that
(1)x,y∈Λ⇒Bx+Cy∈Λ;
(2)B is continuous and BΛ is contained in a compact set;
(3)C is contractive.
Then it has z∈Λ with z=Bz+Cz.
Theorem 3.1. Assume that (H1)–(H2) and the following conditions hold.
(AP) All of the coefficients in system (2.2) are almost periodic sequences.
(H3)r2p<1.
Then it has a 2p-th MAPS X of system (2.2) with ‖X‖2p≤β2p, p≥1.
Proof. Let Λ⊆BC(Z;L2p(Ω;Rm+n)) denote the set of all 2p-th MAPS X satisfying ‖X‖2p≤β2p.
On the basis of (2.3), define ΦX(k)=BX(k)+CX(k), where
ΦX(k)={(ΦX)i(k);(ΦX)n+i(k)}=((ΦX)1(k),…,(ΦX)n(k),(ΦX)n+1(k),…,(ΦX)2n(k))T, |
(ΦX)i(k)=(BX)i(k)+(CX)i(k),(ΦX)n+i(k)=(BX)n+i(k)+(CX)n+i(k), | (3.1) |
{(BX)i(k)=k−1∑v=−∞k−1∏s=v+1e−ai(s)[1−e−ai(v)]ai(v)[n∑j=1bij(v)fj(xj(v))+n∑j=1cij(v)fj(xj(v−τij(v)))+Bi(v)Si(v)+Ii(v)],(BX)n+i(k)=k−1∑v=−∞k−1∏s=v+1e−˜ai(s)[1−e−˜ai(v)]˜ai(v)˜bi(v)˜fi(xi(v)), | (3.2) |
{(CX)i(k)=k−1∑v=∞k−1∏s=v+1e−ai(s)[1−e−ai(v)]ai(v)n∑j=1dij(v)σj(xj(v−ηij(v)))Δwj(v),(CX)n+i(k)=k−1∑v=−∞k−1∏s=v+1e−˜ai(s)[1−e−˜ai(v)]˜ai(v)˜di(v)Si(v)Δ˜wi(v),i=1,2,…,n,k∈Z. | (3.3) |
Let X0={x0i;S0i} be given by
{x0i(k)=k−1∑v=−∞k−1∏s=v+1e−ai(s)[1−e−ai(v)]ai(v)[n∑j=1bij(v)fj(0)+n∑j=1cij(v)fj(0)+n∑j=1dij(v)σj(0)Δwj(v)+Ii(v)],S0i(k)=k−1∑v=−∞k−1∏s=v+1e−˜ai(s)[1−e−˜ai(v)]˜ai(v)˜bi(v)˜fi(0),i=1,2,…,n,k∈Z. |
By the above equations, it has
‖{x0i}‖2p≤max1≤i≤nsupk∈Z{[E|k−1∑v=−∞k−1∏s=v+1e−ai(s)[1−e−ai(v)]ai(v)n∑j=1(bij(v)fj(0)+cij(v)fj(0))|2p]12p+[E|k−1∑v=−∞k−1∏s=v+1e−ai(s)[1−e−ai(v)]ai(v)n∑j=1dij(v)σj(0)Δwj(v)|2p]12p+[E|k−1∑v=−∞k−1∏s=v+1e−ai(s)[1−e−ai(v)]ai(v)Ii(v)|2p]12p}. |
Then
‖{x0i}‖2p≤max1≤i≤nsupk∈Z{(1−e−au)al(1−e−al)[n∑j=1(buij|fj(0)|+cuij|fj(0)|)+Iui]+n∑j=1duijσj(0)[k−1∑v=−∞k−1∏s=v+1e−ai(s)[1−e−ai(v)]ai(v)]1−12p×[k−1∑v=−∞k−1∏s=v+1e−ai(s)[1−e−ai(v)]ai(v)E|Δwj(v)|2p]12p}≤(1−e−au)al(1−e−al)max1≤i≤n[n∑j=1(buij+cuij)|fj(0)|+Iui+n∑j=1duijσj(0)C12p2p]:=e2p. |
Homoplastically,
‖{S0i}‖2p≤(1−e−˜au)˜al(1−e−˜al)max1≤i≤n˜bui|˜fi(0)|:=˜e2p. |
Thus
‖X0‖2p≤max{e2p,˜e2p}:=α2p. |
From (3.1–3.3), one has
‖{(ΦX)i(k)−x0i(k)}ni=1‖2p≤max1≤i≤nsupk∈Zn∑j=1buijLfj{E[k−1∑v=−∞k−1∏s=v+1e−ai(s)[1−e−ai(v)]ai(v)|xj(v)|]2p}12p+max1≤i≤nsupk∈ZBui{E[k−1∑v=−∞k−1∏s=v+1e−ai(s)[1−e−ai(v)]ai(v)|Si(v)|]2p}12p+max1≤i,j≤nsupk∈ZD∗∗i{E[k−1∑v=−∞k−1∏s=v+1e−ai(s)[1−e−ai(v)]ai(v)|xj(v−τij(v))|]2p}12p+max1≤i,j≤nsupk∈ZK∗{E[k−1∑v=−∞k−1∏s=v+1e−ai(s)[1−e−ai(v)]ai(v)|xj(v−ηij(v))Δwj(v)|]2p}12p, |
which implies
‖{(ΦX)i(k)−x0i(k)}ni=1‖2p≤max1≤i≤nsupk∈Zn∑j=1buijLfj{[k−1∑v=−∞k−1∏s=v+1e−ai(s)[1−e−ai(v)]ai(v)]p−1×k−1∑v=−∞k−1∏s=v+1e−ai(s)[1−e−ai(v)]ai(v)E|xj(v)|2p}12p+max1≤i≤nsupk∈ZBui{[k−1∑v=−∞k−1∏s=v+1e−ai(s)[1−e−ai(v)]ai(v)]p−1×k−1∑v=−∞k−1∏s=v+1e−ai(s)[1−e−ai(v)]ai(v)E|Si(v)|2p}12p+max1≤i,j≤nsupk∈ZD∗∗i{[k−1∑v=−∞k−1∏s=v+1e−ai(s)[1−e−ai(v)]ai(v)]p−1×k−1∑v=−∞k−1∏s=v+1e−ai(s)[1−e−ai(v)]ai(v)E|xj(v−τij(v))|2p}12p+max1≤i,j≤nsupk∈ZK∗{[k−1∑v=−∞k−1∏s=v+1e−ai(s)[1−e−ai(v)]ai(v)]p−1×k−1∑v=−∞k−1∏s=v+1e−ai(s)[1−e−ai(v)]ai(v)E|xj(v−ηij(v))Δwj(v)|2p}12p, |
where D∗∗i=D∗−Bui−∑nj=1buijLfj, i=1,2,…,n. It gains from Lemma 2.3 that
‖{(ΦX)i(k)−x0i(k)}ni=1‖2p≤(1−e−au)al(1−e−al){D∗+K∗C12p2p}‖X‖2p≤r2p‖X‖2p≤r2pα2p1−r2p. |
Homoplastically,
‖{(ΦX)n+i(k)−S0i(k)}ni=1‖2p≤(1−e−˜au)˜al(1−e−˜al){˜D∗+˜K∗C12p2p}‖X‖2p≤r2p‖X‖2p≤r2pα2p1−r2p. |
Consequently,
‖ΦX−X0‖2p≤r2pα2p1−r2p. |
So
‖ΦX‖2p≤‖X0‖2p+‖ΦX−X0‖2p≤α2p+r2pα2p1−r2p=α2p1−r2p:=β2p. | (3.4) |
By (3.4) and similar to the argument as that in [20], (1)–(2) of Lemma 3.1 hold.
At last, ∀X={xi;Si},X∗={x∗i;S∗i}∈Λ, one has
‖{(CX)i(k)−(CX∗)i(k)}ni=1‖2p≤[1−e−au]almax1≤i≤nsupk∈Z{E[k−1∑v=−∞k−1∏s=v+1e−al×n∑j=1dij(v)(σj(xj(v−ηij(v)))−σj(yj(v−ηij(v))))Δwj(v)]2p}12p≤[1−e−au]almax1≤i,j≤nsupk∈ZK∗{[k−1∑v=−∞k−1∏s=v+1e−al]2p−1×k−1∑v=−∞k−1∏s=v+1e−alE|[xj(v−ηij(v))−yj(v−ηij(v))]Δwj(v)|2p}12p≤K∗C12p2p(1−e−au)al(1−e−al)‖X−Y‖2p≤r2p‖X−Y‖2p, |
homoplastically,
‖{(CX)n+i(k)−(CX∗)n+i(k)}ni=1‖2p≤˜K∗C12p2p(1−e−˜au)˜al(1−e−˜al)‖X−Y‖2p≤r2p‖X−Y‖2p. |
Then
‖CX−CY‖2p≤r2p‖X−Y‖2p. |
By (H3), C is contractive. So (3) of Lemma 3.1 holds. By Lemma 3.1, system (2.2) has a 2p-th MAPS. The proof is finished.
Remark 3.1. In view of Theorem 3.1, (H3) is hard to be verified. For the sake of enabling (H3) to be met, the following items should be followed in applications:
(1) The coefficients ai and ˜ai in NNs (2.2), i=1,2,…,n, should be selected to be large enough.
(2) The coefficients bij, cij, Bi, dij, ˜bi and ˜di of NNs (2.2) should be selected to meet (H3) with large enough numbers, i=1,2,…,n.
(3) If the activation functions fj, σj and ˜fi in NNs (2.2) are selected by some small enough constants Lfj, Lσj and L˜fi, then (H2) is easier to be valid, i,j=1,2,…,n.
Theorem 4.1. If (H1)–(H3) hold, then system (2.2) has the property of 2p-th MGES.
Proof. Assume that X={xi;Si} with initial condition φ={φi;ψi} and X∗={x∗i;S∗i} with initial condition φ∗={φ∗i;ψ∗i} are any two solutions of system (2.2). So
|xi(k)−x∗i(k)|≤k−1∏s=0e−ai(s)|φi(0)−φ∗i(0)|+(1−e−au)alk−1∑v=0k−1∏s=v+1e−ai(s){n∑j=1[buijLfj|xj(v)−x∗j(v)|+cuijLfj|xj(v−τij(v))−x∗j(v−τij(v))|+duijLσj|xj(v−ηij(v))−x∗j(v−ηij(v))||Δwj(v)|]+Bui|Si(v)−S∗i(v)|}≤e−alk|φi(0)−φ∗i(0)|+(1−e−au)alk−1∑v=0e−al(k−v−1)n∑j=1{n∑j=1[buijLfj|xj(v)−x∗j(v)|+cuijLfj|xj(v−τij(v))−x∗j(v−τij(v))|+duijLσj|xj(v−ηij(v))−x∗j(v−ηij(v))||Δwj(v)|]+Bui|Si(v)−S∗i(v)|}, | (4.1) |
|Si(k)−S∗i(k)|≤k−1∏s=0e−˜ai(s)|ψi(0)−ψ∗i(0)|+(1−e−˜au)˜alk−1∑v=0k−1∏s=v+1e−˜ai(s){˜buiL˜fi|xi(v)−x∗i(v)|˜dui|Si(v)−S∗i(v)||Δ˜wi(v)|}≤e−˜alk|ψi(0)−ψ∗i(0)|+(1−e−˜au)˜alk−1∑v=0e−˜al(k−v−1){˜buiL˜fi|xi(v)−x∗i(v)|+˜dui|Si(v)−S∗i(v)||Δ˜wi(v)|}, | (4.2) |
where i=1,2,…,n, k∈[−μ0,+∞)Z, μ0=max(i,j){τuij,ηuij}.
To facilitate, set
a0=1−e−aual,˜a0=1−e−˜au˜al, |
γ2p=max1≤i≤nsups∈[−μ0,0]Z{(E|φi(s)−φ∗i(s)|2p)12p,(E|ψi(s)−ψ∗i(s)|2p)12p}, |
Z={zi}, W={wi}, zi(k)=xi(k)−x∗i(k), wi(k)=Si(k)−S∗i(k), i=1,2,…,n, k∈Z.
By (4.1), it has
|Z(k)|2p≤e−alkγ2p+max1≤i≤nn∑j=1a0buijLfj{[k−1∑s=0e−al(k−s−1)]2p−1k−1∑s=0e−al(k−s−1)E|xj(s)−x∗j(s)|2p}12p+max1≤i≤nn∑j=1a0cuijLfj{[k−1∑s=0e−al(k−s−1)]2p−1×k−1∑s=0e−al(k−s−1)E|xj(s−τij(s))−x∗j(s−τij(s))|2p}12p+max1≤i≤nn∑j=1a0duijLσj{[k−1∑s=0e−al(k−s−1)]2p−1×k−1∑s=0e−al(k−s−1)E|[xj(s−ηij(s))−x∗j(s−ηij(s))]Δwj(s)|2p}12p+max1≤i≤nBui{[k−1∑s=0e−al(k−s−1)]2p−1k−1∑s=0e−al(k−s−1)E|Si(s)−S∗i(s)|2p}12p≤e−alkγ2p+max1≤i≤nn∑j=1a0buijLfj{[k−1∑s=0e−al(k−s−1)]2p−1k−1∑s=0e−al(k−s−1)|Z(s)|2p2p}12p+max1≤i≤nn∑j=1a0cuijLfj{[k−1∑s=0e−al(k−s−1)]2p−1k−1∑s=0e−al(k−s−1)|Z(s−τij(s))|2p2p}12p+max1≤i≤nn∑j=1a0C12p2pduijLσj{[k−1∑s=0e−al(k−s−1)]2p−1k−1∑s=0e−al(k−s−1)|Z(s−ηij(s))|2p2p}12p+max1≤i≤nBui{[k−1∑s=0e−al(k−s−1)]2p−1k−1∑s=0e−al(k−s−1)|W(s)|2p2p}12p. | (4.3) |
From (4.2), it induces
|W(k)|2p≤e−˜alkγ2p+max1≤i≤n˜a0˜buiL˜fi{[k−1∑s=0e−˜al(k−s−1)]2p−1k−1∑s=0e−˜al(k−s−1)|Z(s)|2p2p}12p+max1≤i≤n˜a0C12p2p˜dui{[k−1∑s=0e−˜al(k−s−1)]2p−1k−1∑s=0e−˜al(k−s−1)|W(s)|2p2p}12p. | (4.4) |
By (H3), it has λ>0 small enough ensuring that
max1≤i≤neλa01−e−(al−2pλ)[Bui+n∑j=1(buijLfj+eμ0λcuijLfj+eμ0λC12p2pduijLσj)]def=ρ≤1, |
max1≤i≤neλ˜a01−e−(˜al−2pλ)[˜buiL˜fi+eμ0λC12p2p˜dui]def=˜ρ≤1. |
Define V(k)=max{|Z(k)|2p,|W(k)|2p}, k∈Z.
Next, assume that it has M0>1 ensuring that
V(k)≤M0γ2pe−λk,∀k∈[−μ0,+∞)Z. | (4.5) |
If (4.5) fails, then it has k0∈(0,+∞)Z such that one of the following two cases is valid:
(C1)|Z(k0)|2p>M0γ2pe−λk0 and V(k)≤M0γ2pe−λk,∀k∈[−μ0,k0)Z.
(C2)|W(k0)|2p>M0γ2pe−λk0 and V(k)≤M0γ2pe−λk,∀k∈[−μ0,k0)Z.
If (C1) holds, then
|Z(k0)|2p≤e−alk0γ2p+max1≤i≤nn∑j=1a0buijLfjM0γ2p{[k−1∑s=0e−al(k−s−1)]2p−1k−1∑s=0e−al(k−s−1)e−2pλs}12p+max1≤i≤na0BuiM0γ2p{[k−1∑s=0e−al(k−s−1)]2p−1k−1∑s=0e−al(k−s−1)e−2pλs}12p+max1≤i≤nn∑j=1a0M0γ2p[cuijLfj+C12p2pduijLσj]×{[k−1∑s=0e−al(k−s−1)]2p−1k−1∑s=0e−al(k−s−1)e−2pλ(s−μ0)}12p≤e−alk0γ2p+max1≤i≤nn∑j=1a0buijLfjM0γ2pe−λk0eλ[1−e−alk01−e−al]1−12p[k0−1∑s=0e−(al−pλ)(k0−s−1)]12p+max1≤i≤na0BuiM0γ2pe−λk0eλ[1−e−alk01−e−al]1−12p[k0−1∑s=0e−(al−pλ)(k0−s−1)]12p+max1≤i≤nn∑j=1a0M0γ2p[cuijLfj+C12p2pduijLσj]×e−λk0e(μ0+1)λ[1−e−alk01−e−al]1−12p[k0−1∑s=0e−(al−pλ)(k0−s−1)]12p≤e−alk0γ2p+max1≤i≤na0M0γ2pe−λk0[Bui+n∑j=1(buijLfj+eμ0λcuijLfj+eμ0λC12p2pduijLσj)]×eλ[1−e−alk01−e−al]1−12p[1−e−(al−2pλ)k01−e−(al−2pλ)]12p≤M0γ2pe−λk0{1M0e−(al−λ)k0+max1≤i≤na0[Bui+n∑j=1(buijLfj+eμ0λcuijLfj+eμ0λC12p2pduijLσj)]eλ[1−e−(al−λ)k0]1−e−(al−2pλ)≤M0γ2pe−λk0{e−(al−λ)k0+ρ[1−e−(al−λ)k0]}≤M0γ2pe−λk0. | (4.6) |
Thus (C1) fails. Homoplastically, (C2) is not satisfied. So (4.5) hold. Then system (2.2) has the property of 2p-th MGES. The proof is finished.
By Theorem 3.1, it obtains
Theorem 4.2. Assume that all conditions in Theorem 3.1 hold, then system (2.2) admits a 2p-th MAPS, which has the property of 2p-th MGES.
Remark 4.1. In literature [17], Ji discussed a delayed semi-discrete neural networks and the existence and global attractivity of a unique almost periodic sequence solution were investigated. In literatures [14,15,16,18], the authors discussed the almost periodic dynamics of various NNs. But neither of them considered the stochastic disturbances. Therefore, the work of this paper complements the works in [14,15,16,17,18].
Example 5.1. Regarding the semi-discrete stochastic CNNs below:
{x(k+1)=0.4x(k)−0.6[0.01sin(√5k)sin(x(k))+0.05sin(√7k)S(k)+0.01Δw1(k)+0.01cos2(√17k)],S(k+1)=0.4S(k)−0.6[0.03cos(√2k)sin(x(k−1))+0.02S(k)Δw2(k)],k∈Z. | (5.1) |
Referring to system (2.2), it has ˜au=˜al=au=al=1, Lf=L˜f=1, Lσ=0, bu=0.01, ˜bu=0.03, Bu=0.05, du=0.01, ˜du=0.02. Taking p=2, then
C1/44=4,D∗≈0.06,˜D∗≈0.03,K∗=0,˜K∗≈0.01,r4≈0.68<1. |
By Theorem 4.2, system (5.1) admits a 4-th MAPS, which has the property of 4-th MGES, see Figures 1–3.
Figure 1 plots the almost periodic trajectories of state variables x and S in system (5.1). Obviously, the amplitude of state variable x is larger than that of state variable S. Figures 2,3 plot the trajectories of state variables x and S in system (5.1) with different initial values, respectively. They demonstrate that the trajectories of state variables in system (5.1) with different initial values will tend to the same trajectory since it has the property of 4-th MGES.
Considering the corresponding determinant model of system (5.1) below:
{x(k+1)=0.4x(k)−0.6[0.01sin(√5k)sin(x(k))+0.05sin(√7k)S(k)+0.01+0.01cos2(√17k)],S(k+1)=0.4S(k)−0.6[0.03cos(√2k)sin(x(k−1))+0.02S(k)],k∈Z. | (5.2) |
Figures 4 and 5 depict the comparison result between model (5.1) and model (5.2). Figures 4 and 5 imply that the influences of stochastic disturbances on x and S are pretty obvious. Observing Figures 4 and 5, it easily discovers that the effect of stochastic disturbance on x is larger than that on S.
To date, few people consider the problems of MAPS and MGES of semi-discrete SFCNNs. Hence, this article concerns the issues of 2p-th MAPS and 2p-th MGES of semi-discrete SFCNNs. The techniques of this literatures give an effective approach to investigate 2p-th MAPS and 2p-th MGES of semi-discrete stochastic fuzzy models. By (H3), r2p<1 is important to the studies of 2p-th MAPS and 2p-th MGES. There is no influence of time delay on 2p-th MAPS and time delay has negative influence in 2p-th MGES.
In the future, the following aspects could be studied further:
(1) p∈(0,1] in NNs (2.2) could be further investigated.
(2) Other dynamics in NNs (2.2) should be further considered, e.g., hopf bifurcation, chaos, automatic control, etc.
(3) Using the idea and method of this paper, other types of dynamic systems could be studied, such as impulsive dynamic systems [23,24], biomathematical systems [25,26,27], etc.
(4) Applications of NNs (2.2) in intelligent control, machine learning, image processing, etc, should be further investigated.
This work is supported by the National Natural Sciences Foundation of People's Republic of China under Grants 11961078. The authors are grateful to anonymous referees for their excellent suggestions, which greatly improve the presentation of the paper.
All authors declare no conflict of interest.
[1] | X. B. Nie, J. L. Liang, J. D. Cao, Multistability analysis of competitive neural networks with Gaussian-wavelet-type activation functions and unbounded time-varying delays, Appl. Math. Comput., 356 (2019), 449-468. |
[2] |
Y. K. Li, J. L. Qin, Existence and global exponential stability of anti-periodic solutions for generalised inertial competitive neural networks with time-varying delays, J. Exp. Theor. Artif. In., 32 (2020), 291-307. doi: 10.1080/0952813X.2019.1647564
![]() |
[3] |
S. Q. Gong, S. F. Yang, Z. T. Guo, et al. Global exponential synchronization of memristive competitive neural networks with time-varying delay via nonlinear control, Neural Process. Lett., 49 (2019), 103-119. doi: 10.1007/s11063-017-9777-1
![]() |
[4] | B. Du, Anti-periodic solutions problem for inertial competitive neutral-type neural networks via Wirtinger inequality, J. Inequal. Appl., 2019 (2019), 187. |
[5] |
X. M. Liu, C. Y. Yang, L. N. Zhou, Global asymptotic stability analysis of two-time-scale competitive neural networks with time-varying delays, Neurocomputing, 273 (2018), 357-366. doi: 10.1016/j.neucom.2017.07.047
![]() |
[6] |
Y. Q. Wang, L. H. Huang, Global stability analysis of competitive neural networks with mixed time-varying delays and discontinuous neuron activations, Neurocomputing, 152 (2015), 85-96. doi: 10.1016/j.neucom.2014.11.016
![]() |
[7] |
X. S. Yang, J. D. Cao, Y. Long, et al. Adaptive lag synchronization for competitive neural networks with mixed delays and uncertain hybrid perturbations, IEEE Trans. Neural Networks, 21 (2010), 1656-1667. doi: 10.1109/TNN.2010.2068560
![]() |
[8] |
T. T. Su, X. S. Yang, Finite-time synchronization of competitive neural networks with mixed delays, Discrete Cont. Dyn. Syst.-B, 21 (2016), 3655-3667. doi: 10.3934/dcdsb.2016115
![]() |
[9] |
H. J. Liu, Z. D. Wang, B. Shen, et al. Delay-distribution-dependent h-infinity state estimation for discrete-time memristive neural networks with mixed time-delays and fading measurements, IEEE Trans. Cybern., 50 (2020), 440-451. doi: 10.1109/TCYB.2018.2862914
![]() |
[10] |
F. Z. de Castro, M. E. Valle, A broad class of discrete-time hypercomplex-valued Hopfield neural networks, Neural Networks, 122 (2020), 54-67. doi: 10.1016/j.neunet.2019.09.040
![]() |
[11] |
E. Y. Cong, X. Han, X. Zhang, Global exponential stability analysis of discrete-time BAM neural networks with delays: A mathematical induction approach, Neurocomputing, 379 (2020), 227-235. doi: 10.1016/j.neucom.2019.10.089
![]() |
[12] | Q. Xiao, T. W. Huang, Quasisynchronization of discrete-time inertial neural networks with parameter mismatches and delays, IEEE Trans. Cybern., 2019. In press: doi:10.1109/tcyb.2019.2937526. |
[13] | R. X. Li, X. B. Gao, J. D. Cao, Exponential state estimation for stochastically disturbed discretetime memristive neural networks: Multiobjective approach, IEEE trans. Neural Networks learn. Syst., 2019. In press: doi:10.1109/tnnls.2019.2938774. |
[14] |
S. Mohamad, K. Gopalsamy, Dynamics of a class of discrete-time neural networks and their continuous-time counterparts, Math. Comput. Simul., 53 (2000), 1-39. doi: 10.1016/S0378-4754(00)00168-3
![]() |
[15] |
Z. K. Huang, S. Mohamad, F. Gao, Multi-almost periodicity in semi-discretizations of a general class of neural networks, Math. Comput. Simul., 101 (2014), 43-60. doi: 10.1016/j.matcom.2013.05.017
![]() |
[16] |
Z. K. Huang, X. H. Wang, F. Gao, The existence and global attractivity of almost periodic sequence solution of discrete-time neural networks, Phys. Lett. A, 350 (2006), 182-191. doi: 10.1016/j.physleta.2005.10.022
![]() |
[17] |
Y. Ji, Global attractivity of almost periodic sequence solutions of delayed discrete-time neural networks, Arabian J. Sci. Eng., 36 (2011), 1447-1459. doi: 10.1007/s13369-011-0109-x
![]() |
[18] |
Z. K. Huang, S. Mohamad, F. Gao, Multi-almost periodicity in semi-discretizations of a general class of neural networks, Math. Comput. Simul., 101 (2014), 43-60. doi: 10.1016/j.matcom.2013.05.017
![]() |
[19] | P. H. Bezandry, T. Diagana, Almost periodic stochastic processes, Springer, New York, 2011. |
[20] | T. W. Zhang, L. J. Xu, Mean almost periodicity and moment exponential stability of discrete-time stochastic shunting inhibitory cellular neural networks with time delays, Kybernetika, 55 (2019), 690-713. |
[21] | S. G. Hu, C. M. Huang, F. K. Wu, Stochastic differential equations, Science Press, Beijing, 2008. |
[22] | D. R. Smart, Fixed point theorems, Cambridge University Press, Cambridge, 1980. |
[23] | T. W. Zhang, L. L. Xiong, Periodic motion for impulsive fractional functional differential equations with piecewise Caputo derivative, Appl. Math. Lett., 101 (2020), 106072. |
[24] | R. Q. Tang, X. S. Yang, X. X. Wan, et al. Finite-time synchronization of nonidentical BAM discontinuous fuzzy neural networks with delays and impulsive effects via non-chattering quantized control, Commun. Nonlinear Sci. Numer. Simul., 78 (2019), 104893. |
[25] |
T. W. Zhang, L. Yang, L. J. Xu, Stage-structured control on a class of predator-prey system in almost periodic environment, Int. J. Control, 93 (2020), 1442-1460. doi: 10.1080/00207179.2018.1513165
![]() |
[26] |
T. W. Zhang, X. R. Gan, Almost periodic solutions for a discrete fishing model with feedback control and time delays, Commun. Nonlinear Sci. Numer. Simul., 19 (2014), 150-163. doi: 10.1016/j.cnsns.2013.06.019
![]() |
[27] | T. W. Zhang, Almost periodic oscillations in a generalized Mackey-Glass model of respiratory dynamics with several delays, Int. J. Biomath., 7 (2014), 1450029. |
1. | Yali Yang, Tianwei Zhang, Anti-periodic motion and mean-square exponential convergence of nonlocal discrete-time stochastic competitive lattice neural networks with fuzzy logic, 2023, 10641246, 1, 10.3233/JIFS-223495 | |
2. | Bin Wang, Random periodic sequence of globally mean-square exponentially stable discrete-time stochastic genetic regulatory networks with discrete spatial diffusions, 2023, 31, 2688-1594, 3097, 10.3934/era.2023157 | |
3. | Yali Yang, Yuntao Liu, Global exponential convergence and synchronization for exponential numerical competitive neural networks with different time scales and fuzzy logic, 2024, 238, 0954-4062, 6795, 10.1177/09544062231225513 | |
4. | Ting Yuan, Huizhen Qu, Dong Pan, Random periodic oscillations and global mean-square exponential stability of discrete-space and discrete-time stochastic competitive neural networks with Dirichlet boundary condition, 2023, 45, 10641246, 3729, 10.3233/JIFS-230821 | |
5. | Tianwei Zhang, Huizhen Qu, Jianwen Zhou, Asymptotically almost periodic synchronization in fuzzy competitive neural networks with Caputo-Fabrizio operator, 2023, 471, 01650114, 108676, 10.1016/j.fss.2023.108676 | |
6. | Yali Yang, Tianwei Zhang, Projective exponential synchronization of space-time differencing fuzzy competitive neural networks with uncertainties, 2025, 158, 10512004, 104924, 10.1016/j.dsp.2024.104924 |