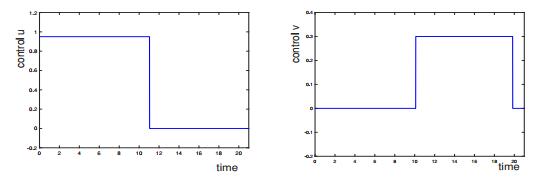
Example of locally optimal controls for a
We review results about the influence tumor heterogeneity has on optimal chemotherapy protocols (relative to timing, dosing and sequencing of the agents) that can be inferred from mathematical models. If a tumor consists of a homogeneous population of chemotherapeutically sensitive cells, then optimal protocols consist of upfront dosing of cytotoxic agents at maximum tolerated doses (MTD) followed by rest periods. This structure agrees with the MTD paradigm in medical practice where drug holidays limit the overall toxicity. As tumor heterogeneity becomes prevalent and sub-populations with resistant traits emerge, this structure no longer needs to be optimal. Depending on conditions relating to the growth rates of the sub-populations and whether drug resistance is intrinsic or acquired, various mathematical models point to administrations at lower than maximum dose rates as being superior. Such results are mirrored in the medical literature in the emergence of adaptive chemotherapy strategies. If conditions are unfavorable, however, it becomes difficult, if not impossible, to limit a resistant population from eventually becoming dominant. On the other hand, increased heterogeneity of tumor cell populations increases a tumor's immunogenicity and immunotherapies may provide a viable and novel alternative for such cases.
Citation: Urszula Ledzewicz, Heinz Schättler, Shuo Wang. On the role of tumor heterogeneity for optimal cancer chemotherapy[J]. Networks and Heterogeneous Media, 2019, 14(1): 131-147. doi: 10.3934/nhm.2019007
[1] | Urszula Ledzewicz, Heinz Schättler, Shuo Wang . On the role of tumor heterogeneity for optimal cancer chemotherapy. Networks and Heterogeneous Media, 2019, 14(1): 131-147. doi: 10.3934/nhm.2019007 |
[2] | Russell Betteridge, Markus R. Owen, H.M. Byrne, Tomás Alarcón, Philip K. Maini . The impact of cell crowding and active cell movement on vascular tumour growth. Networks and Heterogeneous Media, 2006, 1(4): 515-535. doi: 10.3934/nhm.2006.1.515 |
[3] | Benjamin Contri . Fisher-KPP equations and applications to a model in medical sciences. Networks and Heterogeneous Media, 2018, 13(1): 119-153. doi: 10.3934/nhm.2018006 |
[4] | Laura Cattaneo, Paolo Zunino . Computational models for fluid exchange between microcirculation and tissue interstitium. Networks and Heterogeneous Media, 2014, 9(1): 135-159. doi: 10.3934/nhm.2014.9.135 |
[5] | M.A.J Chaplain, G. Lolas . Mathematical modelling of cancer invasion of tissue: dynamic heterogeneity. Networks and Heterogeneous Media, 2006, 1(3): 399-439. doi: 10.3934/nhm.2006.1.399 |
[6] | Eman S. Attia, Ashraf A. M. Khalaf, Fathi E. Abd El-Samie, Saied M. Abd El-atty, Konstantinos A. Lizos, Osama Alfarraj . Performance analysis of nanosystem based on cooperative relay for nanonetworks. Networks and Heterogeneous Media, 2023, 18(4): 1657-1677. doi: 10.3934/nhm.2023072 |
[7] | Fabian Rüffler, Volker Mehrmann, Falk M. Hante . Optimal model switching for gas flow in pipe networks. Networks and Heterogeneous Media, 2018, 13(4): 641-661. doi: 10.3934/nhm.2018029 |
[8] | Paola Goatin, Chiara Daini, Maria Laura Delle Monache, Antonella Ferrara . Interacting moving bottlenecks in traffic flow. Networks and Heterogeneous Media, 2023, 18(2): 930-945. doi: 10.3934/nhm.2023040 |
[9] | Ryan Weightman, Temitope Akinode, Benedetto Piccoli . Optimal control of pandemics via a sociodemographic model of non-pharmaceutical interventions. Networks and Heterogeneous Media, 2024, 19(2): 500-525. doi: 10.3934/nhm.2024022 |
[10] | Alberto Bressan, Yunho Hong . Optimal control problems on stratified domains. Networks and Heterogeneous Media, 2007, 2(2): 313-331. doi: 10.3934/nhm.2007.2.313 |
We review results about the influence tumor heterogeneity has on optimal chemotherapy protocols (relative to timing, dosing and sequencing of the agents) that can be inferred from mathematical models. If a tumor consists of a homogeneous population of chemotherapeutically sensitive cells, then optimal protocols consist of upfront dosing of cytotoxic agents at maximum tolerated doses (MTD) followed by rest periods. This structure agrees with the MTD paradigm in medical practice where drug holidays limit the overall toxicity. As tumor heterogeneity becomes prevalent and sub-populations with resistant traits emerge, this structure no longer needs to be optimal. Depending on conditions relating to the growth rates of the sub-populations and whether drug resistance is intrinsic or acquired, various mathematical models point to administrations at lower than maximum dose rates as being superior. Such results are mirrored in the medical literature in the emergence of adaptive chemotherapy strategies. If conditions are unfavorable, however, it becomes difficult, if not impossible, to limit a resistant population from eventually becoming dominant. On the other hand, increased heterogeneity of tumor cell populations increases a tumor's immunogenicity and immunotherapies may provide a viable and novel alternative for such cases.
We review and discuss some results that can be obtained about the structure of optimal chemotherapy protocols from mathematical models. This question has been addressed at least since the 1970s and 1980s starting with some of the fundamental work by Eisen [13], Swierniak and Kimmel [23,51] and Swan [49,50]. In most of this early work, it is implicitly assumed that the tumor population is homogeneous and consists of chemotherapeutically sensitive cells. Under this assumption it is natural-and this is generally borne out by all these early models as well-to give chemotherapy as early as possible and with as much force as possible. Medically this corresponds to the so-called maximum tolerated dose (MTD) paradigm [47] which still is at the heart of many chemotherapy schedules for the initial induction phase of chemotherapy. MTD is simply "the highest dose of a drug or treatment that does not cause unacceptable side effects" [1]. Generally, the higher the dose, the more harm is caused to the tumor (although this relation, and especially for high doses, is not linear). Since what matters is the marginal benefit, this reasoning, however, critically relies on the assumption of a homogeneous chemosensitive tumor cell population. Heterogeneity blurs this simple understanding.
Genetic instability of cancer cells [16,17] coupled with faster mutation rates and epigenetic abnormalities foster the creation of diverse sub-populations of tumor cells which have widely varying phenotypes and chemotherapeutic sensitivities. In addition, growing tumors often exhibit considerable evolutionary ability to enhance cell survival in an environment that is becoming hostile. According to the Norton-Simon hypothesis [36,37], generally faster replicating cell lines are more susceptible to a chemotherapeutic attack while slower growing ones are more resistant. One widely accepted explanation for this feature is that resistance is achieved through pathways that use up more energy which thus cannot be used for proliferation [14]. Under traditional MTD type chemotherapy, the more sensitive populations will be eradicated allowing the resistant populations to become dominant possibly leading to full resistance and failure of the therapy. Naturally, this world view is a bit oversimplified as the specifics depend on such features as the relative growth rates of the sub-populations. For example, if the resistant population grows exceedingly slow, for all practical and also mathematical purposes, it can be ignored. But it also matters whether drug resistance is intrinsic (i.e., inherent in the sense that there simply is no treatment for it yet) or whether it is acquired. In the later case, interruptions of treatment, so-called drug holidays, may allow for resensitizations to take place which then enable repeated treatments with high doses (rechallenge therapy [57]). Such considerations, combined with ideas about evolution and competition between cell populations [15] have led to the consideration of various alternative chemotherapy dosing schemes in medical practice, most prominently metronomic [2,22], chemo-switch [39] and adaptive chemotherapy [14,15].
Metronomic chemotherapy is "the chronic administration of chemotherapy at relatively low, minimally toxic doses on a frequent schedule of administration, at close regular intervals, with no prolonged drug-free breaks" [2]. It is hoped that by avoiding the limiting toxic side effects of high dose therapy the overall effect of therapy can be improved because of the greatly extended time horizon over which drugs can be given when compared with repeated MTD doses: "concentration
Mathematical models, on the other hand, can be freely used to explore such ideas. There exist an abundance of mathematical models for chemotherapy under tumor heterogeneity ranging from rudimentary models that just distinguish a small number of sub-populations (e.g., Hahnfeldt et al. [21], Ledzewicz and Schättler [27,29,31]) to models with increasing degrees of resistance (e.g., Swierniak et al. [55,56] or Wang and Schättler [58]) and even a continuum of traits (e.g., Lorz et al. [34,35], Billy and Clairambault [3], Delitalia and Lorenzi [8,10], Lavi, Green et al. [19,24]). Recurrent conclusions that can be drawn from a variety of these models are that, indeed, if conditions are right (i.e., under assumptions on the relative growth rates of the sub-populations and about the effectiveness of the drugs) it is possible to limit cancer growth through a judicious choice of drug administration schedules. If, however, there exists an intrinsically resistant subpopulation which has a significant growth rate, then it is rather difficult, and plainly impossible in some cases to prevent the drug resistant strain from becoming dominant. In such a situation, the only mathematically feasible control problem becomes to delay the onset, respectively, the emerging dominance of the resistant populations.
While it appears rather difficult to develop drugs that will be effective for tumor populations with ever increasing degrees of heterogeneity, for such a case hope may lie with different therapies. One of the hallmarks of cancer therapies is that the body's immune system does not recognize tumor cells as 'foreign' and thus only elicits no or at best a weak and ineffective immune response. With increasing mutations and a greater variety of genetic changes present in tumor cells, however, it becomes more likely that such a response will be provoked in the first place, i.e., that the tumor becomes immunogenic, or that such a response is stronger. Simply put, the chances that cells will be recognized as hostile increases (also, see [9]). The hope then is that, even if the innate immune system response is initiated by specific mutations, its reaction may be a broader attack on the full tumor. In fact, immunotherapies have proven themselves to be quite effective for cancers like melanoma whose cells exhibit a great variety of mutations.
In this paper, we discuss various conclusions that can be drawn about the structure of optimal chemotherapy protocols from an analysis of mathematical models done in our work and we relate the results to some current efforts in medical practice. We review existing models and summarize their findings, but also include some new results in the context of mutations.
We briefly describe a mathematical model for chemotherapy based on Swierniak's models [52] that can be used as a common framework for both homogeneous and heterogeneous cell populations. In this section, however, we only discuss it as a framework for cell-cycle specific models for cancer chemotherapy of homogeneous chemosensitive tumors. The mathematical model divides the state into compartments which cluster cells in phases of the cell cycle (and, more generally, could represent cells of certain phenotypes in specific cell-cycle compartments). The state space is the first orthant
$ ˙N(t)=(A+m∑j=1ujBj)N(t),N(0)=N0, $
|
(1) |
with
The problem of cancer chemotherapy can then be formulated as an optimal control problem once an appropriate criterion to be minimized is imposed on this dynamics. While there exist many alternatives to do this, we would like to stress that for a meaningful interpretation of side effects and total drug dose, it seems to be imperative to include the controls as linear terms in this formulation. Thus, with
$ J=J(u)=rN(T)+∫T0{qN(t)+su(t)}dt=n∑i=1riNi(T)+∫T0{n∑i=1qiNi(t)+m∑j=1sjuj(t)}dt→min $
|
(2) |
The term
The formulation of the objective (2) attempts to make a reasonable compromise between minimizing the tumor kill and limiting the side effects of treatment. These are measured indirectly through a weighted average of the total amount of drugs administered. Obviously, these weights are important for the optimal solutions and a biological calibration of these weights always needs to be undertaken to obtain meaningful results. If the weights on the drugs are too small, optimal solutions will simply give drugs all the time at full dose; if the weights are too high, no drugs will be given. Generally, the weight for a strong cytotoxic (killing) agent should be significantly higher than the weight for a cytostatic (blocking) or recruiting agent. Also, if the model differentiates between compartments of drug sensitive and resistant agents (as they will be considered in Section 3), it may be advisable to use higher weights for the resistant compartments. Similarly, if one takes
Keeping such restrictions in mind, it can be stated that for cell-cycle specific models of homogeneous tumor cell populations, optimal controls apply cytotoxic agents in one full dose segment at the beginning of the therapy interval. Mathematically more precisely, numerous models for cancer chemotherapy with cytotoxic agents have been considered and analyzed in the work of Swierniak et al. [52,53,54] and Ledzewicz and Schättler [25,26,44] and for each of them it has been verified theoretically that optimal controls are bang-bang, i.e., alternate between full dose treatments and rest periods. Generally such statements depend on properties of the matrices
Figure 1 shows a typical example of optimal controls for a
Example of locally optimal controls for a
A bilinear dynamics of the type (1) can also be used to investigate the structure of optimal chemotherapy protocols for a heterogeneous tumor population. In the paper [21], Hahnfeldt, Folkman and Hlatky compare the effects of MTD and metronomic chemotherapy dosings (for bolus type injections) for a 2-compartment model consisting of sensitive and resistant populations. They analyze the maximum asymptotic factor reduction in tumor size in an infinite cycle of periodic therapy periods and come to the conclusion that a metronomic, regular scheduling of the drugs has better long term effects when compared with MTD bolus injections. Alternatively, minimizing an objective of the type (2), one can explore the structure of optimal protocols that minimize the tumor burden as measured by the average over one large therapy interval (e.g., see [27,28]).
For example, the dynamics for a 3-compartmental model that distinguishes between "sensitive" cells,
$ ˙S=(α1−σP−σR−φ1u)S+πSP+ρSR, $
|
(3) |
$ ˙P=σPS+(α2−πS−πR−φ2u)P+ρPR, $
|
(4) |
$ ˙R=σRS+πRP+(α3−ρS−ρP−φ3u)R. $
|
(5) |
The labels
If we denote the proportions of the respective populations by
$ x = \frac{S}{S+P+R},\qquad y = \frac{P}{S+P+R}, \qquad {\rm{and}} \qquad z = \frac{R}{S+P+R}, $ |
a direct computation verifies that
Contrary to the models for homogeneous populations, here optimal controls that take values in the interior of the control set are viable alternatives. It follows from the necessary conditions for optimality of the Pontryagin maximum principle [40] (for some more recent texts, see [6,7,43]) that optimal controls
$ H=qN+su+λ(A+Bu)N, $
|
(6) |
pointwise over the control set along the optimal trajectory
$ ˙λ=−q−λ(A+Bu), $
|
(7) |
with terminal condition
$ Φ(t)=s+λ(t)BN∗(t), $
|
(8) |
then optimal controls satisfy
$ u∗(t)={0ifΦ(t)>0,umaxifΦ(t)<0, $
|
(9) |
while the minimization condition a priori gives no information about the optimal control
It follows from general Lie algebraic identities that the control can appear for the first time only in an even derivative when the switching function is differentiated. If this happens in the second derivative, a singular control is said to be of order one. In this case, it is a second-order necessary condition for optimality, the Legendre Clebsch condition that
$ ∂∂ud2dt2∂H∂u=∂∂u¨Φ(t)≥0. $
|
(10) |
If this quantity is positive, we say the strengthened Legendre-Clebsch condition holds.
Proposition 1. [29] For the compartmental model defined by equations (3)-(5), singular controls exist, are of order
$ using(t)={λ(t)[A,[A,B]]−q[A,B]−qBA}N∗(t){−λ(t)[B,[A,B]]+qB2}N∗(t). $
|
(11) |
where the commutator of the matrices
Dividing both numerator and denominator by the total number of cancer cells, we note that the singular control actually does not depend on the values
Figure 2 shows an example of an extremal controlled trajectory starting from proportions given by the steady-state of the uncontrolled dynamics. Initially the control is given by the maximum dose rate, but then, as a certain equilibrium between the proportions of the three populations is reached, administration of the agent switches to a singular control with reduced dose rates adapting to the changed composition of the tumor. In the medical literature, such protocols have been called chemo-switch protocols [39]. But note that this lower dose rate no longer is able to shrink the total tumor size: all three subpopulations grow during the period when the singular control is administered, but at reduced rates. This example illustrates that, as differing chemotherapeutic sensitivities come into play, with time lower dose rates become the better alternative to MTD protocols.
We note that the analysis of the multi-input scenario corresponding to combination treatments with different drugs would be an interesting and relevant extension of this work as in such cases drug holidays indeed have shown to be effective in resensitizing sub-populations that have acquired drug resistance [57]. However, incorporating such effects would also require to make the transition rates in the dynamics to be drug dependent. From the mathematical perspective, additional difficulties will arise as now controls might be simultaneously singular. Necessary conditions for optimality such as the Goh condition also exist for such cases.
Some cancers, like melanoma, are characterized by a large number of mutations that can easily go into the hundreds, even thousands. Aside from progressive mutations, a second key reason for the emergence of cellular heterogeneity and drug resistance in cancers of all types are epigenetic abnormalities, i.e., mistakes in the control mechanisms that govern gene expression patterns [12]. This is important from a therapy perspective since reversing such abnormalities appears to be more promising than correcting for mutations. Given such a diversity, it therefore makes sense to also consider distributed mathematical models for phenotypic heterogeneity and drug resistance in tumors that allow for a continuum of possible traits. Such models have been formulated by Lorz, Lorenzi, Clairambault et al. [34,35] and are also considered in the work of Billy and Clairambault [3] and Delitalia and Lorenzi [8,10]. Lavi, Greene et al. [19,24] consider such models as a means to explain the roles played by increasing cell densities and mutations in the emergence of specific traits that become dominant. We briefly recall their formulation.
In the model, a continuum of possible traits/resistance levels
$ ∂n∂τ(τ,x)={r(x)(1−θ)−G(ˉN(τ))μ(x)}n(τ,x)+θ∫10p(x|y)r(y)n(τ,y)dy $
|
(12) |
with trait specific replication rates
$ dˉNdτ(τ)=∫10{r(x)−G(ˉN(τ))μ(x)}n(τ,x)dx. $
|
(13) |
The incorporation of cell densities, i.e., the addition of the
Proposition 2. Let
$ G∗=max0≤x≤1{r(x)μ(x)} $
|
and suppose the function
Drug treatment can be incorporated into equations (12) and (13) by subtracting trait dependent killing terms. If one considers constant controls, one may model the effects of such terms by still subtracting
Here, rather than using a linear log-kill term, we consider a Michealis-Menten type pharmacodynamic model of the form
$ c(x)u(τ)UC50+u(τ)n(τ,x) $
|
(14) |
where
Optimal control problems of this type are inherently difficult as the aim is to control a large number of distinct cell populations by means of a what in reality are a very small number of controls (the concentrations of just a few drugs, maybe 1 to 4) giving rise to the notion of multi-targeted optimal control problems: a large number of variables needs to be controlled with a small number of inputs. Mathematically, allowing for a continuum of traits in the model gives the problem a distributed aspect and the resulting optimization problem becomes non-standard and of great complexity. This is also attested to by the theoretical analysis given by Pouchol et al. [41] who considered optimal control problems for such systems determined by phenotype-structured equations.
In our work [58], we have taken the approach to approximate this structure by a finite-dimensional model, but with a potentially large dimension. If we discretize the traits and again denote the state by the vector
$ ˙N(t)=(R−Cu(t)UC50+u(t)−G(ˉN(t))M)N(t). $
|
(15) |
with
$ C = {\rm{diag}} ( \varphi_1, \ldots, \varphi_n ), \quad M = {\rm{diag}} ( \mu_1, \ldots, \mu_n ), $ |
and
$ R = [r1(1−θ)θr2p(r1|r2)⋅θrnp(r1|rn)θr1p(r2|r1)r2(1−θ)⋅θrnp(r2|rn)⋮⋮⋱⋮θr1p(rn|r1)θr2p(rn|r2)⋅rn(1−θ)] . $
|
Using the same type of objective as before, for a fixed terminal time
$ J(u)=αN(T)+∫T0(βN(t)+γu(t))dt $
|
(16) |
over all Lebesgue measurable functions
Figure 3 below gives, for various mutation rates
$ r(x)=21.1+2x5andφ(x)=21+x2 $
|
(17) |
evaluated at these nodes; we kept the death rate constant,
$ p(x|y)=k(y)exp(−12(x−yσ)2) $
|
(18) |
with variance
The top row of Fig. 3 shows the optimal controls coded with different colors for different mutation rates
This particular simulation provides only a snapshot of one specific scenario to illustrate some of the comments made above. The bottom figure shows the emergence of a dominant trait. There is only a small group of traits located around
Once heterogeneity in tumor cells increases and cells become resistant, the attempt to develop drugs that could deal with each and every possibility becomes futile (e.g., because of exorbitant costs connected with drug development) and it appears that the answer to treating strongly heterogeneous tumors must lie elsewhere. This may be immunotherapies. As abnormalities accumulate, it simply becomes more likely that either the innate immune system recognizes the tumor cells as foreign or that it becomes easier to teach the immune system through outside intervention that these cells are foreign and thus elicit a strong reaction of the immune system. In principle, as with chemotherapies, it may happen that the immune system only reacts to specific mutations which will selectively be killed (immuno-editing [11,45]), but the hope is that the immune reaction will be more systemic and attack the full tumor.
There have been a number of mathematical models that analyze tumor immune interactions starting with the largely influential model by Stepanova [48]. In that model, the main features of tumor immune system interactions are aggregated into just two variables, the tumor volume,
$ ˙x=μCxF(x)−γxy, $
|
(19) |
$ ˙y=μI(1−βx)xy−δy+α, $
|
(20) |
with
Precisely because of its simplicity-a few parameters incorporate many medically important features-the underlying equations have been widely accepted as a basic model which, despite its simplicity, accurately describes essential aspects of tumor immune system interactions. Depending on the parameter values, there exist three possibilities. The dynamics can have a unique globally asymptotically equilibrium point with small tumor volumes, the benign situation, in which the immune system simply is able to control the disease. On the other side of the spectrum lies the scenario when there still exists a unique globally asymptotically equilibrium point, but now with large tumor volumes, the malignant case, when even outside intervention can only move this equilibrium point to lower values that may still be lethal. The third scenario is the medically relevant one and it lies in between these extremes: the dynamics is multi-stable and the system has both a locally asymptotically stable microscopic and macroscopic equilibrium point as well as an unstable saddle point. The tumor volumes are small and the immuno-competent cell densities are upregulated for the microscopic equilibrium point while they are large, almost at their carrying capacity, with depressed immuno-competent cell densities at the macroscopic equilibrium point. Both equilibria are locally asymptotically stable and their regions of attraction are separated by the stable manifold of the saddle which forms the common boundary of these regions. Figure 4 gives a characteristic illustrative example of this structure.
Example of the phase portraits for the system (19)-(20) with a Gompertzian growth function
Given the bi-stable character of the underlying dynamics, now the control problem becomes to move the system-through therapy-back into the region of attraction of the microscopic equilibrium point. Note that there is no need to steer the system into the benign equilibrium point itself as this point is locally asymptotically stable. Once the state of the system lies within its region of attraction, possibly with some safe distance from its stability boundary, the system will self-regulate to this point, i.e., the immune system takes over the rest of the treatment. Such a geometric situation naturally leaves a tremendous freedom in the formulation of the objective. We found it especially useful to take relative weights for the variables
Progressive mutations and epigenetic abnormalities can lead to great heterogeneity in tumor cell populations which may prevent successful treatment. In this paper, at the hand of some minimally parameterised mathematical models, we discussed effects that tumor heterogeneity has on the structure of optimal chemotherapy protocols. From a practical perspective, these solutions suggest that lower, properly calibrated doses may be more advantageous as drug resistance builds up as they may also be able to control the growth of a more malignant, but slower growing drug resistant strain of cells.
The authors would like to express their thanks to two anonymous reviewers of this manuscript whose comments helped us greatly in improving the presentation of the material and who directed us to some relevant literature cited in the paper.
[1] |
MTD, NCI Dictionary of Cancer Terms, https://www.cancer.gov/publications/dictionaries/cancer-terms/def/mtd, accessed 08/18/18. |
[2] | Metronomic scheduling of anticancer treatment: The next generation of multitarget therapy?. Future Oncology (2011) 7: 385-394. |
[3] |
Designing proliferating cell population models with functional targets for control by anti-cancer drugs. Discr. and Cont. Dyn. Syst., Series B (2013) 18: 865-889. ![]() |
[4] |
F. Billy, J. Clairambault and O. Fercoq, Optimisation of Cancer Drug Treatments Using Cell Population Dynamics, in: Mathematical Methods and Models in Biomedicine, (U. Ledzewicz, H. Schättler, A. Friedman and E. Kashdan, Eds.), Springer, New York, 2013, 265–309. |
[5] |
Concentration phenomena in some non-local equation. J. Discrete and Continuous Dynamical Systems, Series B (2017) 22: 763-781. ![]() |
[6] |
B. Bonnard and M. Chyba, Singular Trajectories and their Role in Control Theory, Mathématiques & Applications, vol. 40, Springer Verlag, Paris, 2003. |
[7] |
A. Bressan and B. Piccoli, Introduction to the Mathematical Theory of Control, American Institute of Mathematical Sciences, 2007. |
[8] |
M. Delitalia and T. Lorenzi, Formations of evolutionary patterns in cancer dynamics, in: Pattern Formation in Morphogenesis: Problems and Mathematical Issues, (V. Capasso et al., Eds.), Springer Proceedings in Mathematics, 15 (2013), 179–190. |
[9] |
Recognition and learning in a mathematical model for immune response against cancer. Discr. and Cont. Dyn. Syst., Series B (2013) 18: 891-914. ![]() |
[10] |
M. Delitalia and T. Lorenzi, Mathematical modelling of cancer under target therapeutic actions: Selection, mutation and drug resistance, in: Managing Complexity, Reducing Perplexity in Biological Systems, (M. Delitala and G. Ajmone Marsan Eds.), Springer Proceedings in Mathematics & Statistics, 2014, 81–99. |
[11] |
Cancer immunoediting: From immunosurveillance to tumor escape. Nat. Immunol. (2002) 3: 991-998. ![]() |
[12] |
Cancer epigenetics: Tumor Heterogeneity, Plasticity of Stem-like States, and Drug Resistance. Molecular Cell (2014) 54: 716-727. ![]() |
[13] |
M. Eisen, Mathematical Models in Cell Biology and Cancer Chemotherapy, Lecture Notes in Biomathematics, Vol. 30, Springer Verlag, Berlin, 1979. |
[14] |
A change of strategy in the war on cancer. Nature (2009) 459: 508-509. ![]() |
[15] |
Adaptive therapy. Cancer Research (2009) 69: 4894-4903. ![]() |
[16] | Drug resistance in cancer: A perspective. Cancer and Metastasis Review (2001) 20: 63-68. |
[17] | A model for resistance of tumor cells to cancer chemotherapeutic agents. Mathematical Biosciences (1983) 65: 291-307. |
[18] |
The penetration of anticancer drugs through tumor tissue as a function of cellular adhesion and packing density of tumor cells. Cancer Research (2006) 66: 1033-1039. ![]() |
[19] |
The impact of cell density and mutations in a model of multidrug resistance in solid tumors. Bull. Math. Biol. (2014) 74: 627-653. ![]() |
[20] |
Cell resensitization during protracted dosing of heterogeneous cell populations. Radiation Research (1998) 150: 681-687. ![]() |
[21] |
Minimizing long-term burden: the logic for metronomic chemotherapeutic dosing and its angiogenic basis. J. of Theoretical Biology (2003) 220: 545-554. ![]() |
[22] |
Less is more, regularly: Metronomic dosing of cytotoxic drugs can target tumor angiogenesis in mice. J. Clinical Investigations (2000) 105: 1045-1047. ![]() |
[23] | An optimal control problem related to leukemia chemotherapy. Scientific Bulletins of the Silesian Technical University (1983) 65: 120-130. |
[24] |
The role of cell density and intratumoral heterogeneity in multidrug resistance. Cancer Research (2013) 73: 7168-7175. ![]() |
[25] |
Optimal bang-bang controls for a 2-compartment model in cancer chemotherapy. J. of Optimization Theory and Applications - JOTA (2002) 114: 609-637. ![]() |
[26] |
Analysis of a cell-cycle specific model for cancer chemotherapy. J. of Biological Systems (2002) 10: 183-206. ![]() |
[27] |
Drug resistance in cancer chemotherapy as an optimal control problem. Discrete and Continuous Dynamical Systems, Series B (2006) 6: 129-150. ![]() |
[28] |
U. Ledzewicz and H. Schättler, Tumor microenvironment and anticancer therapies: An optimal control approach, in: Mathematical Oncolgy 2013, (A. d'Onofrio and A. Gandolfi, Eds.), Birkhäuser, New York, 2013, 295–334. |
[29] |
A $3$-compartment model for chemotherapy of heterogeneous tumor populations. Acta Applicandae Matematicae (2015) 135: 191-207. ![]() |
[30] |
Optimal controls for a mathematical model of tumor-immune interactions under targeted chemotherapy with immune boost. Discrete and Continuous Dynamical Systems, Series B (2013) 18: 1031-1051. ![]() |
[31] |
On optimal chemotherapy for heterogeneous tumors. J. of Biological Systems (2014) 22: 177-197. ![]() |
[32] |
A review of optimal chemotherapy protocols: From MTD towards metronomic therapy. Math. Model. Nat. Phenom. (2014) 9: 131-152. ![]() |
[33] |
U. Ledzewicz and H. Schättler, Optimizing chemotherapeutic anti-cancer treatment and the tumor microenvironment: an analysis of mathematical models, in: Systems Biology of Tumor Microenvironment, Advances in Experimental Medicine and Biology Vol. 936, (K.A. Rejniak (ed.)), 2016, 209–223. |
[34] |
Population adaptive evolution, chemotherapeutic resistance and multiple anti-cancer therapies. ESAIM: Mathematical Modelling and Numerical Analysis (2013) 47: 377-399. ![]() |
[35] |
Effects of space structure and combination therapies on phenotypic heterogeneity and drug resistance in solid tumors. Bull. Math. Biol. (2015) 77: 1-22. ![]() |
[36] | Tumor size, sensitivity to therapy, and design of treatment schedules. Cancer Treatment Reports (1977) 61: 1307-1317. |
[37] | The Norton-Simon hypothesis revisited. Cancer Treatment Reports (1986) 70: 41-61. |
[38] |
B. Perthame, Transport Equations in Biology, Birkhäuser, Basel, Switzerland, 2007. |
[39] | A multi-targeted, metronomic and maximum tolerated dose "chemo-switch" regimen is antiangiogenic, producing objective responses and survival benefit in a mouse model of cancer. J. of Clinical Oncology (2005) 23: 939-952. |
[40] |
L. S. Pontryagin, V. G. Boltyanskii, R. V. Gamkrelidze and E. F. Mishchenko, The Mathematical Theory of Optimal Processes, Macmillan, New York, 1964. |
[41] |
C. Pouchol, J. Clairambault, A. Lorz and E. Trélat, Asymptotic analysis and optimal control of integro-differential system modelling healtyh and ccells exposed to chemotherapy, J. de Mathématiques Pures et Appliquées, 2017; arXiv: 1612.04698 [math.OC] |
[42] |
E. Ramos, C. Nespoli and P. Ramos, Feedback optimal control for mathematical models for cancer treatment, Preprint, 2018. |
[43] |
H. Schättler and U. Ledzewicz, Geometric Optimal Control, Interdisciplinary Applied Mathematics, vol. 38, Springer, 2012. |
[44] |
H. Schättler and U. Ledzewicz, Optimal Control for Mathematical Models of Cancer Therapies, Interdisciplinary Applied Mathematics, vol. 42, Springer, 2015. |
[45] |
Cancer immunoediting: Integrating immunity's roles in cancer suppression and promotion. Science (2011) 331: 1565-1570. ![]() |
[46] |
S. V. Sharma et al., A chromatin-mediated reversible drug-tolerant state in cancer cell subpopulations, Cell, 141 (2010), 69–80. |
[47] |
On mathematical modeling of critical variables in cancer treatment (goals: better understanding of the past and better planning in the future). Bulletin of Mathematical Biology (1986) 48: 253-278. ![]() |
[48] | Course of the immune reaction during the development of a malignant tumour. Biophysics (1980) 24: 917-923. |
[49] |
G. W. Swan, Applications of Optimal Control Theory in Medicine, Marcel Dekker, New York, 1984. |
[50] |
General applications of optimal control theory in cancer chemotherapy. IMA J. of Mathematical Applications in Medicine and Biology (1988) 5: 303-316. ![]() |
[51] |
A. Swierniak, Optimal treatment protocols in leukemia - modelling the proliferation cycle, Biomedical Systems Modelling and Simulation (Paris, 1988), 51–53, IMACS Ann. Comput. Appl. Math., 5, IMACS Trans. Sci. Comput. '88, Baltzer, Basel, 1989. |
[52] | Cell cycle as an object of control. Journal of Biological Systems (1995) 3: 41-54. |
[53] | Optimal control for a class of compartmental models in cancer chemotherapy. Int. J. Applied Mathematics and Computer Science (2003) 13: 357-368. |
[54] | Optimal control problems arising in cell-cycle-specific cancer chemotherapy. Cell proliferation (1996) 29: 117-139. |
[55] | Qualitative analysis of controlled drug resistance model - inverse Laplace and semigroup approach. Control and Cybernetics (1999) 28: 61-75. |
[56] |
Cancer chemotherapy optimization under evolving drug resistance. Nonlinear Analysis (2000) 47: 375-386. ![]() |
[57] |
G. Tonini, M. Imperatori, B. Vincenci, A. M. Frezza and D. Santini, Rechallenge therapy and treatment holiday: Different strategies in management of metastatic colorectal cancer, J. Experimental Clinical Cancer Research, 32 (2013), p92. |
[58] |
Optimal control of a mathematical model for cancer chemotherapy under tumor heterogeneity. Mathematical Biosciences and Engineering - MBE (2016) 13: 1223-1240. ![]() |
[59] |
S. Wang and H. Schättler, Optimal control for cancer chemotherapy under tumor heterogeneity, Discrete and Continuous Dynamical Systems, Series B, 48 (2018). |
[60] |
Back to the basics: the importance of concentration $\times$ time in oncology. J. of Clinical Oncology (1993) 11: 820-821. ![]() |
1. | Urszula Ledzewicz, Heinz Schättler, Combination of antiangiogenic treatment with chemotherapy as a multi‐input optimal control problem, 2022, 45, 0170-4214, 3058, 10.1002/mma.7977 | |
2. | Ibrahim M. Chamseddine, Katarzyna A. Rejniak, Hybrid modeling frameworks of tumor development and treatment, 2020, 12, 1939-5094, 10.1002/wsbm.1461 | |
3. | Jean Clairambault, 2023, Mathematical Modelling of Cancer Growth and Drug Treatments: Taking Into Account Cell Population Heterogeneity and Plasticity, 978-3-907144-08-4, 1, 10.23919/ECC57647.2023.10178266 | |
4. | Hannah G. Anderson, Gregory P. Takacs, Jeffrey K. Harrison, Libin Rong, Tracy L. Stepien, Optimal control of combination immunotherapy for a virtual murine cohort in a glioblastoma-immune dynamics model, 2024, 595, 00225193, 111951, 10.1016/j.jtbi.2024.111951 |
Example of locally optimal controls for a
Example of an extremal control and associated states for a bang-singular controlled trajectory
Extremal controls (top), evolution of the total tumor
Example of the phase portraits for the system (19)-(20) with a Gompertzian growth function
Example of numerically computed optimal controls for the system (19)-(20) with a Gompertzian growth function