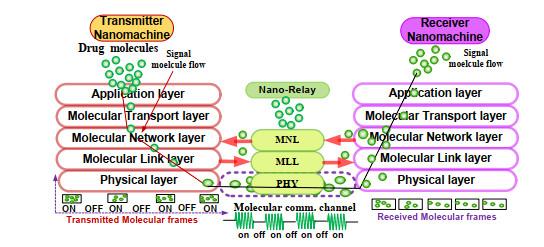
Recent nanomedical applications, particularly targeted drug delivery system (TDDS) scenarios, have made use of molecular communications via diffusion (MCvD) based on nanosystems. In order to improve the performance of such nanosystems, nanonetworks-based molecular communication is investigated. Employing a nanorelay approach and cooperative molecular communications, we provide a method for optimizing the performance of the nanosystem while taking blood flow effects into consideration in terms of drift velocity. Unlike the earlier studies, the position of the nanorelay and the allocated amount of molecular drugs will be optimized. We provide closed-form expressions for molecular channel capacity and the error probability of a molecular frame. According to the simulation results, it is possible to significantly reduce error probability of a molecular frame and thus increase channel capacity by optimizing the drift velocity, detection threshold and the location of the nanorelay within the proposed nanosystem.
Citation: Eman S. Attia, Ashraf A. M. Khalaf, Fathi E. Abd El-Samie, Saied M. Abd El-atty, Konstantinos A. Lizos, Osama Alfarraj. Performance analysis of nanosystem based on cooperative relay for nanonetworks[J]. Networks and Heterogeneous Media, 2023, 18(4): 1657-1677. doi: 10.3934/nhm.2023072
[1] | Yao-Li Chuang, Tom Chou, Maria R. D'Orsogna . A network model of immigration: Enclave formation vs. cultural integration. Networks and Heterogeneous Media, 2019, 14(1): 53-77. doi: 10.3934/nhm.2019004 |
[2] | Rudy R. Negenborn, Peter-Jules van Overloop, Tamás Keviczky, Bart De Schutter . Distributed model predictive control of irrigation canals. Networks and Heterogeneous Media, 2009, 4(2): 359-380. doi: 10.3934/nhm.2009.4.359 |
[3] | David Hales, Stefano Arteconi . Motifs in evolving cooperative networks look like protein structure networks. Networks and Heterogeneous Media, 2008, 3(2): 239-249. doi: 10.3934/nhm.2008.3.239 |
[4] | Hirotada Honda . On a model of target detection in molecular communication networks. Networks and Heterogeneous Media, 2019, 14(4): 633-657. doi: 10.3934/nhm.2019025 |
[5] | Philippe Souplet . Liouville-type theorems for elliptic Schrödinger systems associated with copositive matrices. Networks and Heterogeneous Media, 2012, 7(4): 967-988. doi: 10.3934/nhm.2012.7.967 |
[6] | Vinay Aggarwal, Anja Feldmann . Locality-aware P2P query search with ISP collaboration. Networks and Heterogeneous Media, 2008, 3(2): 251-265. doi: 10.3934/nhm.2008.3.251 |
[7] | Luca Schenato, Sandro Zampieri . On rendezvous control with randomly switching communication graphs. Networks and Heterogeneous Media, 2007, 2(4): 627-646. doi: 10.3934/nhm.2007.2.627 |
[8] | Raúl M. Falcón, Venkitachalam Aparna, Nagaraj Mohanapriya . Optimal secret share distribution in degree splitting communication networks. Networks and Heterogeneous Media, 2023, 18(4): 1713-1746. doi: 10.3934/nhm.2023075 |
[9] | A M Ishtiaque Mahbub, Behdad Chalaki, Andreas A. Malikopoulos . A constrained optimal control framework for vehicle platoons with delayed communication. Networks and Heterogeneous Media, 2023, 18(3): 982-1005. doi: 10.3934/nhm.2023043 |
[10] | Yuntian Zhang, Xiaoliang Chen, Zexia Huang, Xianyong Li, Yajun Du . Managing consensus based on community classification in opinion dynamics. Networks and Heterogeneous Media, 2023, 18(2): 813-841. doi: 10.3934/nhm.2023035 |
Recent nanomedical applications, particularly targeted drug delivery system (TDDS) scenarios, have made use of molecular communications via diffusion (MCvD) based on nanosystems. In order to improve the performance of such nanosystems, nanonetworks-based molecular communication is investigated. Employing a nanorelay approach and cooperative molecular communications, we provide a method for optimizing the performance of the nanosystem while taking blood flow effects into consideration in terms of drift velocity. Unlike the earlier studies, the position of the nanorelay and the allocated amount of molecular drugs will be optimized. We provide closed-form expressions for molecular channel capacity and the error probability of a molecular frame. According to the simulation results, it is possible to significantly reduce error probability of a molecular frame and thus increase channel capacity by optimizing the drift velocity, detection threshold and the location of the nanorelay within the proposed nanosystem.
In the wake of the COVID-19 crisis, the globe is searching for a new medical monitoring system. Researchers have developed a novel nanosystem that may be utilized for the diagnosis of infectious diseases with the use of cutting-edge nanotechnology and nanoscience [1,2,3]. Additionally, such a nanosystem can be utilized to transport therapeutic medications to the targeted diseased site. The nanosystem is composed of thousands of tiny devices, or nanomachines, including nanotransmitters, nanorelays and nanoreceivers that are designed to resemble biological cells, earning them the name "bionanomachines" [4]. Information can be transmitted using a variety of communication techniques, such as electromagnetic, wire, acoustic and thermal. However, none of those are appropriate for communications between bio-nanomachines. On the other hand, molecular communication systems are systems that are either employed by living cells or are inspired by biological cell communication (e.g., communication via diffusion, ion signaling, microtubule-molecular motors and pheromone signaling). Subsequently, bionanomachines can communicate with each other by utilizing molecular communication (MC) technology. The interchange of molecules is used by MC to transport information, making it the most promising strategy in the nanomedical applications [5]. Unfortunately, the bionanomachines can only perform simple tasks. A network of bionanomachines may perform complex tasks that a single bionanomachine cannot, and thus the concept of nanonetwork emerges. Bionanomachines that can recognize target sites or target signals and release medication molecules at certain locations have recently been developed using molecular communication to create smart targeted drug delivery systems (TDDS). The nanonetwork can be a portion of the human body. Furthermore, the nanosystem networks can be remotely controlled and monitored using the internet of bio nano things (IoBNTs) technology [6,7]. The IoBNT is a broad paradigm for remotely managing a biological network of nanosystems and thus interfacing the biological network with an external network such as the internet [8,9].
Recently, the IEEE P1906.1 nanonetworking standard for nanoscale and molecular communication evolved to define a nanonetwork as a human-designed communication system [10]. Molecular communication via diffusion (MCvD) has gained a lot of attention for its biocompatibility and energy efficiency. Molecular information in MCvD is encoded via a variety of methods, including molecular concentration, timing and type [11]. The study of MC systems considering characteristics of classical diffusion phenomena has received a lot of attention in the literature. Classical diffusion phenomena, however, are not nearly applicable in several contemporary cases. The distance between bionanomachines is an important factor to take into account when using diffusion for establishing reliable molecular communication. The relay concept was used by the researchers to improve the reliability of molecular communication and make it more practical for long-distance transmissions. Therefore, it is necessary to take into account how the medium's drift velocity affects the reliability of molecular communication. In the present investigation, we introduce the medium's drift velocity, where the signalling molecules are subjected to advection in addition to their random walk. In terms of modulation schemes [12], an isomer-based ratio shift keying (IRSK) system was proposed by the authors in [13]. The transmitter in the system releases the information based on the ratios of different types of messenger molecules to achieve binary or quaternary communication. A concentration shift keying (CSK) is the most easy and popular type of modulation, which is analogous to amplitude shift keying (ASK) in digital modulation. The information is represented by varying the concentration level of molecules, which means a fixed number of molecules are released with the information bit represented by "1" and nothing is released for information bit "0", which uses a single type of information molecules. If a frame represents one-bit of information, this technique is called binary CSK (BCSK), and if a frame represents two-bits of information it is called quadrature CSK (QCSK). In [14], a molecule shift keying (MoSK) scheme is represented where two different types of molecules with the same number are regarded as separate signals. Signal detection schemes can be classified as energy-based signal detection and sampling-based signal detection. In energy-based signal detection techniques, the receiver gathers the number of molecules absorbed over a time period and then compares the estimated number to a predetermined threshold of molecules. On the other hand, in the sampling-based signal detection scheme the receiver senses the concentration of information molecules and counts the number of available molecules at either one or more time instants [15].
The rest of the paper is structured as follows: Section 2 explains the related work and the motivation. Section 3 presents the nanonetwork-based molecular communications and its layered architecture. Section 4 describes the proposed nanosystem based on MCvD and the proposed nanorelay approach based on flow velocity of the medium. Section 5 presents the performance analysis of the proposed approach in terms of probability of error and the molecular channel capacity. Section 6 presents the optimization procedure. The numerical and simulation results are presented in Section 7. Finally, Section 8 concludes this work.
As nanorelay will be crucial in MCvD-based nanonetworks, numerous research efforts on flow-based diffusive molecular communication, relay molecular communication and optimization criteria have been published in the literature. In [16], a molecular reaction process involving two distinct molecule types from the two transmitters (i.e., transmitter and relay nodes) is employed as an XOR logic operation to vanish or strengthen the transmitted signal on the physical layer network coding level, which decreases the complexity of the relay node. Relaying was implemented in [17] at microfluidic channels, allowing communication between a large number of lab-on-a-chip components with the goal of sharing information. Geometric modification was employed to enhance performance. The authors of [18] introduced a multi-hop relay method based on attractants nodes that control the direction of the sent signal across the relay network based on a larger concentration due to use of the relative relevance of the attractants to achieve higher performance. The authors made a significant effort to mimic molecular communication using wireless systems, and they used an adaptive relay selection methodology to reduce transmission times [19]. This methodology used buffered data traffic from active users with the link capacity and categorised the available active relays. Additionally, exploiting the phenomena of fluorescence resonance energy transfer (FRET), the authors of [20] have proposed an embedded nanorelay to replicate the relay transport protein (RTP) that synthesizes the plasma membrane in living cells. The authors of [21] investigated the effects of velocity under various mobility conditions in the blood stream using a flow-induced diffusive channel model. The on-off keying modulation (OOK) for MC system performance was assessed in terms of bit error rate (BER). The likelihood ratio has been used to develop a decision rule and an adaptive decision threshold. A nanorelay between the source and destination nodes has been proposed in [22]. However, some drawbacks may be generated when using the relay node such as more nodes implanted in human body, more complicated networks and delayed transmission. It gathers the molecules that have been released from the transmitter, temporarily stores them and then releases them to the receiver. As a result, the signal strength is increased and the delayed and non-delayed received molecules arrive at the same time. In contrast to earlier literature, the current work presents how well the cooperative relay system under drift flow velocity performs in terms of the placement of the decoding nanorelay and the amount of molecular drugs that are allocated based on optimization criterion for the rate transmission of these drug molecules. Additionally, it offers closed-form formulas for the probability of bit error and molecular channel capacity. Moreover, unlike [23,24,25] and [26], which concern the optimization criterion, the novelty of the current study is to introduce a novel multi-parameter optimization criterion, namely the modified central force optimization (MCFO) algorithm, based on molecular signal processing. The drift velocity is the more crucial parameter that we optimize in addition to the preceding parameters in the previous literature. To deliver predetermined therapeutic drug molecules with carefully calculated drift velocity (optimized drift velocity) to the targeted cell, a cooperative nanorelay node based on flow velocity is created. Therefore, the main contributions of this work are as follows to achieve our motivation:
1. Proposing a cooperative molecular communication system based on nanorelay approach,
2. Introducing a more realistic molecular medium taking into account flow drift velocity,
3. Evaluating the probability of error and molecular channel capacity and
4. Providing an optimization procedure to optimize the nanosystem parameters.
The proposed cooperative nanonetwork-based molecular communications architecture consists of four layers as illustrated in Figure 1. These layers are described as follows:
1. Physical layer (PHY): This layer describes how the communication medium is physically constructed, including the molecular signaling techniques used. Molecules are employed in this layer to carry data and relay information between nodes.
2. Molecular data link layer (MLL): This layer manages the synchronization and error-control procedures as well as the transmission and receiving of data between adjacent nodes. It enables dependable data packet delivery between the sender and receiver nodes.
3. Molecular network layer (MNL): This layer decides how data packets are routed between sources and destinations, as well as how congestion management systems and other pertinent transport protocols work.
4. Molecular application layer: This layer establishes the higher-level controls that support the many applications that are built on top of the network layer. It consists of ways to access transmitted data and an interface that lets users communicate with the network using protocols.
There are certain acceptable assumptions that can be made about the nanonetwork, or nano scale dimension, such as the assumption that all nanomachines are uniform, located nearby and at close range to the cancerous location. However, the architecture levels in the proposed molecular communications system based on cooperative nanonetworks are comparable to those in a traditional computer network.
This stack architecture layer allows for the investigation of issues such as transmission medium, routing and mobility-aware protocols and transmission control protocol/internet protocol (TCP/IP), synchronization and security. However, the current study is focused on the transmission medium, i.e., physical layer, wherein the molecular communication environment consists of nanomachines as carrier information in an aqueous medium, e.g., a blood vessel, inside the human body, and it provides an energy source to such nanomachines from adenosine triphosphate (ATP) [27]. Furthermore, inter-symbol interference (ISI), which results from releasing various numbers of molecules to represent various molecular frames, and an undesirable random disturbance of signal molecules in a molecular communication channel can both contribute to noise. Therefore, the main objective of this work is to concentrate on the transmission and reception processes in the molecular communication system employing stochastic diffusion in the environment and fluid flow drift velocity in (for example, a blood vessel). The performance of molecular communications based on nanonetworks is then evaluated by determining the molecular channel capacity and calculating the probability of error for delivering molecular frames.
As illustrated in Figure 2, the suggested nanosystem model consists of a transmitter nanomachine, a decoding relay nanomachine and a receiver nanomachine, which are denoted by A, B and C nodes, respectively. The terms {transmitter, receiver and relay} nanomachine and nanotransmitter, nanoreceiver and nanorelay node are used interchangeably. To keep things as simple as possible, we suppose that B and C are spheres and A is a point source while B node "nanorelay" is a nanotransceiver because it either transmits or receives molecules, when acting as a destination. The lengths of AB, AC, and BC links are represented as dAB, dAC, and dBC, respectively. For a more realistic model, we examined the angles between the three nodes, which yields more improvement in system performance, as shown in Figure 2. Moreover, node B is assumed to emit a different type of molecule (type B) than node A (type A) in order to prevent self-interference, which decreases the efficacy of the proposed nanosystem. The operation of transmission and reception of therapeutic drug molecules in the proposed nanosystem model can be summarized as follows:
● Nanotransmitter A releases the molecular frames "therapeutic drug molecules" as molecules of type A to both B and C nanomachine nodes via the diffusive drift channel, at the beginning of nth molecular frame.
● The molecular frames are received and decoded by nanorelay node B, then forwarded to the nanoreceiver C, at the (n+1) th molecular frame via molecules of type B.
● The performance of the proposed nano model is improved by using diversity technique on the two different combined signals at C node. The two signals come from ABC and the AC paths.
The cooperative molecular communication system is depicted with the nanorelay node represented as a triangle, and its lengths denote the distance between each node relative to the others by the following angles:
sinαidBC=sinβidAB=sinγidAC | (4.1) |
Assume
dABdAC=sinβisinγi=m |
and
dBCdAC=sinαisinγi |
Hence, dAB=m.dAC, where
sinγi=sinβim | (4.2) |
Therefore,
dBC=m.dACsinαisinβi | (4.3) |
by assuming that dAC is the largest side in the triangle, i.e., γi=π/2.
The proposed nanosystem-based nanonetwork is inserted into the human body; as a result, the diffusion of the therapeutic drug molecule in blood flow is based on positive drift velocity. Fick's second law states that after the drifting distance, d, and following the probability density function (PDF), f (t) of any therapeutic drug molecules of type A released by the nanotransmitter A node will take the amount of time, t to reach the B or C nodes [29]:
f(t)=d(4πDAt)3/2e(−(vt−d)2/4DAt) | (4.4) |
where d (i.e., dAB, dAC, and dBC) is distance depending on the analysis of the link, DA is the diffusion coefficient of type A molecules and v is the drift velocity. The following formula can be used to determine the likelihood that a type A molecule will hit the nanoreceiver within time t:
FCDF(t)=12[1+erf[√d4DAt(vtd−1)]]+12exp(vdDA)[1+erf[−√d4DAt(vtd+1)]] | (4.5) |
where erf(.) is the error function. The drift velocity, diffusion coefficient, time and distance all affect thecumulative distribution function (CDF) in Eq (4.5). The block diagram of the molecular communication via diffusion (MCvD) is depicted in Figure 3. Analogous to a conventional wireless communication system, the transmission process operates on a frame-by-frame basis [28]. The length of the frame is specified by Ts. To convey the information bit "1" at the start of each Ts, OOK modulation scheme is used by node A and B, which is applied in discrete network conditions.
Additionally, the diffusion model of a molecule is mathematically represented as a channel impulse response (CIR) in the proposed nanosystem based on MCvD. The PDF that the released molecule A exists at position d at time t is then shown in Figure 4. The amount of molecules that reach the nanoreceiver when blood flow velocity is taken into account is obviously more than when flow velocity is not taken into account. In the next subsections, we provide the proposed cooperative molecular communication relaying method based on drift velocity as well as the performance analysis in terms of BER and molecular channel capacity.
In this section, we present the performance analysis of the proposed approach in terms of the probability of the molecular channel capacity and the error probability of a molecular frame.
According to the end-to-end MCvD system model described in Figure 3, in order to compute the error probability of a molecular frame for the entire cooperative molecular communication with nanorelay, we consider a linear combination of the two received signals from the two links, ABC link and AC link, as illustrated in Figure 2. We exploit the diversity combining principle in a wireless communication system, which combines received signals from earlier links linearly at the nanoreceiver C node. As a result of the flow drift velocity, these two signals are detected in distinct molecular frames n and n+1. Because each signal employs a different type of chemical, the nanoreceiver C can distinguish between them.
For the ABC transmission path: Let the total amount of A molecules absorbed at nanorelay B be yAAB(n), the number of molecules sent and received at the present frame Ts, is denoted by NANc,AB(n), the ISI term is denoted by NAP,AB(n), NANo,AB(n) is the number of molecules acting as noise from other sources and the counting noise, denoted by the number of molecules absorbed by the nanorelay B node, is NANc,AB(n). As a result, the equation of yAAB(n) can be written as follows:
yAAB[n]=NAC,AB[n]+NAP,AB[n]+NANo,AB[n]+NANc,AB[n] | (5.1) |
where, NANc,AB[n], NANo,AB[n] and NAP,AB[n] have a binomial distribution. As a result, it is possible to estimate that the B node absorbed the following number of molecules:
yAAB[n]∼N(QAxs[n]PA1AB,QAxs[n]PA1AB(1−PA1AB))+∑Ii=1(QAxs[n−i]qAiAB,QAxs[n−i]qAiAB(1−qAiAB))+N(μno,AB,σ2no,AB)+N(0,σ2nc,AB) | (5.2) |
where, I is the length of ISI, xs[n−i] signifies the transmitted bit by A node at (n−i)th molecular frame interval and PA(i)AB=F(v,DA,dAB,its), which is likewise subject to the normal distribution σ2nc,AB is proportional to the number of molecules gathered by the nanorelay B node, qAiAB={PA(i+1)AB−PA(i)AB} and the fraction of molecules released by the transmitter at node A is denoted by QA.
As a result, in order to determine the error probability of a molecular frame that has gathered at nanorelay node B, yAAB(n) equally follows the Gaussian distribution, which is denoted by [25]:
Pr{yAAB[n]|xs[n]=0}∼N(μ0,AB,σ20,AB)andPr{yAAB(n)|xs[n]=1}∼N(μ1,AB,σ21,AB) | (5.3) |
The mean and variance in Eq (5.3) is provided in Appendix A. Subsequently, the received molecular frame can be detected by nanorelay B node using the likelihood detection method [30]:
ˆxr[n]={1,ifyAAB[n]≥τR0,ifyAAB[n]<τR | (5.4) |
The bit information detected by the nanorelay node B in the nth frame interval is denoted by ˆxr[n] and the detection threshold at the B node at the nanorelay is denoted by τR. The likelihood-ratio test π1π0=Pr{yAAB[n]|xs[n]=0}Pr{yAAB[n]|xs[n]=1} can be used to determine τR.
For the BC transmission path: By repeating the equations of the absorbed molecules for type A by nanorelay, which are given in Eqs (4.3–4.5) and (5.1–5.3), we can similarly derive the equations for (type B) molecules absorbed by the C nanoreceiver at [n+1]th frame, which is represented by yBBC[n+1]. As a consequence, the distribution of yBBC[n+1] is obtained as follows:
Pr(yBBC[n+1]|xr[n+1]=0)∼N(μ0,BC,σ20,BC)andPr(yBBC[n+1]|xr[n+1]=1)∼N(μ1,BC,σ21,BC) | (5.5) |
where, the mean and variance values are provided in Appendix A by applying all parameter abbreviations of BC path where Pr(xr[n+1]=1) can be represented as [25,28]:
Pr(xr[n+1]=1)=π1Pr(ˆxr(n+1)=1|xs[n]=1)+π0Pr(ˆxr[n+1]=1|xs[n]=0)=14[(1−erf(τR−μ1,AB√2σ21,AB))+(1−erf(τR−μ0,AB√2σ20,AB))] | (5.6) |
For the AC transmission path: Again, the transmission through path AC is similar to the transmission of drug molecules in AB path but with its affected parameters. Subsequently, the number of type A molecules collected by nano receiver C node along AC path in the kth frame interval is defined by yAAC(n) like the AB path. The distribution of yAAC(n) also obeys the Gaussian distribution as follows:
Pr(yAAC[n]|xs[n]=0)∼N(μ0,AC,σ20,AC)andPr(yAAC[n]|xs[n]=1)∼N(μ1,AC,σ21,AC) | (5.7) |
As a method of combining diversity in wireless communications, energy detection is used in the detection process. The effectiveness of detection at the C nanoreceiver can be improved by applying the diversity combining equal gain combining technique in the proposed MC cooperative networks based on drift velocity. The linear combination technique collects the energy signals that are received from the AC and BC paths with different molecule types A and B in different frames. Therefore, we can detect the signal by the following decision rule:
ˆxd(n+1)={1,ifqDyAAC[n]+qRyBBC[n+1]≥τD0,ifqDyAAC[n]+qRyBBC[n+1]<τD | (5.8) |
where ˆxd[n+1] is the bit detected at C nanoreceiver node in the (n+1)th frame, and qD and qR are the weights of the AC and BC paths, respectively.
The received signals from two paths as cooperative values with equal gain combining in different frames, yBBC[n+1] and yAAC[n], are gaussian random variables given by Eqs (5.5) and (5.7), yABCO[n+1], yAAC[n]+yBBC[n+1] is also a gaussian random variable as follows [25]:
Pr(yABCO[n+1]|xs[n]=0,xr[n+1]=0)∼N(μ00,CO,σ200,CO)andPr(yABCO[n+1]|xs[n]=0,xr[n+1]=1)∼N(μ01,CO,σ201,CO) | (5.9) |
Pr(yABCO[n+1]|xs[n]=1,xr[n+1]=0)∼N(μ10,CO,σ210,CO)andPr(yABCO[n+1]|xs[n]=1,xr[n+1]=1)∼N(μ11,CO,σ211,CO) | (5.10) |
where the mean values can be obtained as follows:
μlj,CO=μl,AC+μj,BC,∀l,j∈{0,1} | (5.11) |
σ2lj,CO=σ2l,AC+σ2j,BC,∀l,j∈{0,1} | (5.12) |
Finally, the overall error probability of a molecular frame for all paths in the proposed nanosystem can be given by:
Pe[n]=π1Pr(ˆxd[n+1]=0|xs[n]=1)+π0Pr(ˆxd[n+1]=1|xs[n]=0)=12+18[(erf(τD−μ10,CO√2σ210,CO))(1+erf(τR−μ1,AB√2σ21,AB))]+18[(erf(τD−μ11,CO√2σ211,CO))(1−erf(τR−μ1,AB√2σ21,AB))]−18[(erf(τD−μ00,CO√2σ200,CO))(1+erf(τR−μ0,AB√2σ20,AB))]−18[(erf(τD−μ01,CO√2σ201,CO))(1−erf(τR−μ0,AB√2σ20,AB))] | (5.13) |
We formulate the molecular channel capacity of the proposed approach to compute the amount of molecular budget per frame. We will adopt the same strategy as [7]. According to the proposed approach depicted in Figure 2, we compute the total channel capacity by determining the capacity of each path. In fact, the mutual information between each path is randomly determined by the binary entropy if the transmitted bit is X and the received bit is Y as follows:
I(X;Y)=H(Y)−H(Y|X) | (5.14) |
where H(Y) is considered as the amount of minimum information that should be received at the nanoreceiver or nanorelay and H(Y|X) represents the actual amount of information received by nanoreceiver or nanorelay. The two amounts can be expressed as follows:
H(Y)=−∑1i=0Py(i)×log2Py(i) | (5.15) |
H(Y|X)=−∑1j=0∑1i=0P(x=i,y=j)×log2P(y=j|x=i) | (5.16) |
Hence, we can compute the total capacity of the merged paths as:
I(X;Y)=IAC(X;Y)+IABC(X;Y) | (5.17) |
In fact, the conditional probabilities in each path are based on the probability of a false alarm (receiving 1 when 0 was transmitted) and the probability of a miss detection (receiving 0 while 1 was transmitted). Subsequently, the conditional probabilities for ABC path are calculated as follows:
PABC(0|0)=π0[PFABPMBC+(1−PFAB)(1−PFBC)] | (5.18) |
PABC(1|0)=π0[PFAB(1−PMBC)+(1−PFAB)PFBC) | (5.19) |
PABC(0|1)=π1[(1−PMAB)PMBC+PMAB(1−PFBC)] | (5.20) |
PABC(1|1)=π1[(1−PMAB)(1−PMBC)+PFBCPMAB] | (5.21) |
where PFAB is the probability of false alarm between A, B nodes, PFBC is the probability of false alarm between B, C nodes, PMAB is the probability of miss detection between A, B nodes and PMBC is the probability of miss detection between B, C nodes for ABC path.
The conditional probabilities for AC path are calculated as follows:
PAC(0|0)=π0(1−PFAC) | (5.22) |
PAC(1|0)=π0(PFAC) | (5.23) |
PAC(0|1)=π1(PMAC) | (5.24) |
PAC(1|1)=π1(1−PMAC) | (5.25) |
where PFAC is considered as the probability of false alarm between A, C nodes and PMAC is the probability of miss detection between A, C nodes for AC path.
Also, the probabilities of receiving 0 and 1 for ABC path are calculated as follows:
Py_ABC(0)=π0[(1−PFAB)(1−PFBC)+PFABPMBC]+π1[PMAB(1−PFBC)+(1−PMAB)PMBC | (5.26) |
Py_ABC(1)=π0[(1−PFAB)PFBC+PFAB(1−PMBC)+π1(1−PMAB)(1−PMBC)+PMABpFBC] | (5.27) |
The probabilities of receiving 0 and 1 for AC path are calculated as follows:
Py_AC(0)=π0(1−PFAC)+π1(PMAC) | (5.28) |
Py_AC(1)=π0(PFAC)+π1(1−PMAC) | (5.29) |
Thereafter, the molecular channel capacity can be expressed as:
C=MaxI(X;Y)bits/sec | (5.30) |
Equation (5.13) computes the overall error probability of a molecular frame in the proposed cooperative relaying nanonetwork. Obviously, it indicates that the error probability of a molecular frame is mainly based on two parameters: flow drift velocity and the detection threshold. As a consequence, these parameters are strongly affected by the performance of the proposed nanosystem. Therefore, we apply an optimization procedure to solve this issue in order to achieve the best system performance; we require effective and trustworthy computational techniques to address optimization problems. The optimal solution for the most critical parameters such as drift velocity (v) and detection threshold (τD) at the destination node can be optimized to achieve the minimum probability of error, Pe as follows:
minv,τDPe | (6.1) |
Then we optimize the nanorelay position and resources allocated to the nanotransmitter and nanorelay with fixed optimal v and τD by:
minm,nPe | (6.2) |
where m = dAB/dAc and nQ = QA/(QA + QB).
In other words, to reduce the Pe in the proposed approach, we must compute the ideal drift velocity v and τD. We minimize our objective function Pe by using MCFO as a productive optimization procedure. In fact, the particle swarm optimization (PSO), which is based on swarm movement, and MCFO, which is subject to the law of gravity, are the two well-known global optimization algorithms that have just recently been introduced in [30] and [31]. The PSO has few parameters and is simple to use. On the other hand, despite its complexity, the MCFO performs well when optimizing many parameters compared with block coordinate descent algorithm (BCDA) [32,33]. The goal of this improvement or modification algorithm (MCFO) is to promote the probes' convergence to the global optimum at the end of the search and to improve the global search during the early stages of the optimization by time varying acceleration coefficients. Figure 5 depicts the procedure steps of the MCFO algorithm. The algorithm starts with the use of a succession of probes in three-dimensional space. Each probe has two main category position vectors which represent a solution to the optimization problem (the optimal values for decision variables) and acceleration distributions. With aid from a gravitational constant, MCFO exponents and a repositioning factor that has a significant influence on the algorithm's convergence, the probes are drawn to the best positions.
In this section, we present the numerical results obtained by using the developed cooperative nanorelay approach based on MCvD, which is validated by the MCFO optimization procedure under various settings such as flow drift velocity and sample frame time. In fact, we used the presented numerical analysis to evaluate the performance of the proposed approach in terms of the probability of molecular frame error rate (FER) and molecular channel capacity. We also compared the results to the direct link relay (DLR) system [26], which indicates that nanorelay B is linearly intermediate between nanotransmitter A and nanoreceiver C, i.e., no cooperative mechanism is applied. Through the use of MATLAB, we conducted the program simulation. The simulation parameters are used in the simulation campaign are set as follows: dAC changes from 1 to 2 µm, DA and DB are 242 and 78 µm2/s, while v changes from 0 to 1 mm/s. The number of released molecules of type A and B are 200 and 1000, respectively, and the frame duration is changed from 2 to 7 msec.
The error probability of molecular frame with varying drift velocity of molecules for different frame duration while the detection threshold τD is fixed is depicted in Figure 6. We consider QA = QB = 400 molecules when the distance dAC = 2 μm. As expected, when the frame duration is large, the probability of molecular frame error rate is greatly lowered since a lower ISI may be possible. On the other hand, we see that every probability of error curve in the image behaves in a quasi-convex manner, indicating that the optimization problem of figuring out the best velocity is a quasi-convex problem. As a result, the purpose of the optimization technique in the cooperative nanosystem is to identify the best velocity. Additionally, it has been observed that the performance of error probability of the molecular frame grows with increasing velocity until a particular point indicated by the optimization technique, at which point the performance declines. As a result, the optimization technique can solve the challenge of selecting the best velocity.
Figures 7 and 8 show the joint optimization of nanorelay position and molecular budget to transmitter A node and relay B node nanomachines with fixed destination threshold, τD, and velocity, v, parameters. When the velocity (v = 0.3 mm/s) and Ts = 5 ms are fixed, QA + QB = 800. The error probability of a molecular frame is given as a function of nanorelay position m in Figure 7. Obviously, it is a quasi-convex function, and as a result, we can use the BCDA to optimize the nanorelay position with the MCFO algorithm, which is based on the idea of setting all parameters except one and determining the optimal value that minimizes the objective function Pe and then fixing m and finding the optimal molecular budget allocated to the transmitter A node and B node nanomachines until convergence is achieved.
Unlike the previous literature, the proposed nanosystem is considered as non-linear due to the force of velocity that affects the diffusion of molecules and different types of molecules (type A) and (type B) with different diffusion coefficients. We use more accurate calculations for this scenario by relation of the triangles and its angles. Also, we appreciate the performance by results of small and large distances between A and C nodes (dAC), as shown in Figures 7 and 8. Unlike most of the previous works that give the best performance for the scenario of intermediate relay between transmitter and receiver nodes, the optimal nanorelay position is obtained when m = 0.3 for small distance (dAC ≤ 1 µm). This indicates that the nanorelay B node is close to the nanotransmitter A node, which means there is a large angle (alpha α ≥ 50o), as shown in Figure 8. On the other hand, for large distance (dAC > 1 µm) to maintain suitable performance, the optimal positioning of nanorelay B node when it will be close to the nanoreceiver C node is m ≥ 0.6 and a small angle alpha (α < 45o). Moreover, that optimal molecular distribution is found when the nanorelay QA = 1/3 QB for small distance dAC, which means that more molecules are allocated to the nanorelay B node than nanotransmitter A node. On the other hand, for large distance the results give more improvement when QA = QB, as observed in Figures 7 and 8.
Here, we compare the performance of the proposed approach to the DLR system described in [26], as shown in Figure 9. We find that the proposed approach outperforms the DLR system, particularly at high threshold values (τD), whereas at low threshold values (τD), the two approaches perform similarly. This finding suggested that when a high number of molecules are utilized in the detection procedure, the proposed approach is more practical. The performance of the nanosystem is impacted by the MCFO optimization procedure by choosing an acceptable detection threshold, τD.
The molecular channel capacity is another important metric for analyzing the efficiency of the proposed approach. Despite conventional communication, the capacity for molecular communication is restricted and relies on the channel model's circumstances, as evidenced by the number of molecules received and captured by the nanoreceiver nodes.
Figures 10 and 11 depict the channel capacity for the proposed approach in comparison to DLR. It is clear in Figure 10 that using the MCFO optimization algorithm gives the optimal velocity of drug molecule injection at v = 0.3 mm/s, also giving the optimal capacity at the same drift velocity. Also, the channel capacity for the cooperative nanosystem is higher than the DLR.
Figure 11 represents the channel capacity as a function of frame duration. Frame duration has a significant effect on the channel capacity due to the nature of molecules that diffuse giving along tail causing ISI effect as shown in Figure 4. This indicates that as frame duration increases, ISI decreases. We can infer that the channel capacity, in addition to the performance of the proposed cooperative nanosystem, improve considerably with increasing frame duration when compared to the DLR.
We introduced a new nanosystem-based nanonetwork with a nanorelay cooperative MCvD system for targeted nanomedical applications. Taking into account molecular noise and ISI, the error probability of a molecular frame is given in a closed form in this study and therefore employed as an objective function within the MCFO optimization procedure to calculate the optimal velocity of the drug molecules and the detection threshold at the nanoreceiver. Based on the foregoing research, we find that the proposed cooperative nanosystem with nanorelay outperforms the DLR system. Furthermore, when compared to the nanosystem-based DLR system, the nanosystem-based proposed approach has a lower molecular budget and a reduced error probability of a molecular frame.
The authors declare they have not used Artificial Intelligence (AI) tools in the creation of this article.
This work was funded by the Researchers Supporting Project number. (RSP2023R102) King Saud University, Riyadh, Saudi Arabia.
The authors declare that they have no conflicts of interest to report regarding the present study.
The mean and variance of Eqs (5.5) and (5.7) are given by
μ0,AB=π1QA∑Ii=1qAiAB+μno,AB | (A.1) |
μ1,AB=μ0,AB+QAPA1AB | (A.2) |
σ20,AB=π1QA∑Ii=1qAiAB(1−qAiAB)+π0π1Q2A∑Ii=1(qAi,AB)2+σ2no,AB+μ0,AB | (A.3) |
σ21,AB=QAPA1AB(1−PA1AB)+σ20,AB+σ2no,AB+μ1,AB | (A.4) |
where π1=Pr(xs[n]=1) and π0=Pr(xs[n] = 0).
[1] |
S. M. Abd El‐atty, Health monitoring scheme‐based Forster resonance energy transfer nano communications in the Internet of Biological Nanothings, Int. J. Commun. Syst., 33 (2020), e4398. https://doi.org/10.1002/dac.4398 doi: 10.1002/dac.4398
![]() |
[2] |
S. Mohamed, J. Dong, S. M. A. El-Atty, M. A. Eissa, Bio-Cyber Interface Parameter Estimation with Neural Network for the Internet of Bio-Nano Things, Wirel. Pers. Commun., 123 (2022), 1245–1263. https://doi.org/10.1007/s11277-021-09177-6 doi: 10.1007/s11277-021-09177-6
![]() |
[3] |
A. El-Fatyany, H. Z. Wang, S. M. Abd El-atty, Efficient framework analysis for targeted drug delivery based on internet of bio-nanothings, Arab. J. Sci. Eng., 46 (2021), 9965–9980. https://doi.org/10.1007/s13369-021-05651-2 doi: 10.1007/s13369-021-05651-2
![]() |
[4] |
S. M. Abd El-atty, N. A. Arafa, A. Abouelazm, O. Alfarraj, K. A. Lizos, F. Shawki, Performance analysis of an artificial intelligence nanosystem with biological internet of nano things, CMES-Comp. Model. Eng. Sci., 133 (2022), 111–131. https://doi.org/10.32604/cmes.2022.020793 doi: 10.32604/cmes.2022.020793
![]() |
[5] |
M. Germain, F. Caputo, S. Metcalfe, G. Tosi, K. Spring, A. K. Åslund, et al., Delivering the power of nanomedicine to patients today, J. Controlled Release, 326 (2020), 164–171. https://doi.org/10.1016/j.jconrel.2020.07.007 doi: 10.1016/j.jconrel.2020.07.007
![]() |
[6] |
E. S. Attia, A. A. M. Khalaf, F. E. A. El-Samie, S. M. A. El-Atty, K. A. Lizos, O. Alfarraj, et al., Embedded coded relay system for molecular communications, Comput. Mater. Contin., 72 (2022), 2729–2748. https://doi.org/10.32604/cmc.2022.026197 doi: 10.32604/cmc.2022.026197
![]() |
[7] | U. Chude-Okonkwo, R. Malekian, B. T. Maharaj, Advanced Targeted Nanomedicine A Communication Engineering Solution, Switzerland: Springer Nature, 2009. |
[8] |
S. Zafar, M. Nazir, T. Bakhshi, H. A. Khattak, S. Khan, M. Bilal, et al., A systematic review of bio-cyber interface technologies and security issues for internet of bio-nano things, IEEE Access, 9 (2021), 93529–93566. https://doi.org/10.1109/ACCESS.2021.3093442 doi: 10.1109/ACCESS.2021.3093442
![]() |
[9] |
W. X. Pan, X. K. Chen, X. D. Yang, N. Zhao, L. G. Meng, F. H. Shah, A molecular communication platform based on body area nanonetwork, Nanomaterials, 12 (2022), 722. https://doi.org/10.3390/nano12040722 doi: 10.3390/nano12040722
![]() |
[10] | S. Canovas-Carrasco, A. J. Garcia-Sanchez, J. Garcia-Haro, The IEEE 1906.1 Standard: Nanocommunications as a new source of data, in 2017 ITU Kaleidoscope: Challenges for a Data-Driven Society (ITU K), IEEE, Nanjing, (2017), 1–7. https://doi.org/10.23919/ITU-WT.2017.8247001 |
[11] |
N. Farsad, H. B. Yilmaz, A. Eckford, C. B. Chae, W. S. Guo, A comprehensive survey of recent advancements in molecular communication, IEEE Commun. Surv. Tutorials, 18 (2016), 1887–1919. https://doi.org/10.1109/COMST.2016.2527741 doi: 10.1109/COMST.2016.2527741
![]() |
[12] |
M. Ş. Kuran, H. B. Yilmaz, I. Demirkol, N. Farsad, A. Goldsmith, A survey on modulation techniques in molecular communication via diffusion, IEEE Commun. Surv. Tutorials, 23 (2020), 7–28. https://doi.org/10.1109/COMST.2020.3048099 doi: 10.1109/COMST.2020.3048099
![]() |
[13] |
N. R. Kim, C. B. Chae, Novel modulation techniques using isomers as messenger molecules for nano communication networks via diffusion, IEEE J. Sel. Areas Commun., 31 (2013), 847–856. https://doi.org/10.1109/JSAC.2013.SUP2.12130017 doi: 10.1109/JSAC.2013.SUP2.12130017
![]() |
[14] | H. B. Yilmaz, N. R. Kim, C. B. Chae, Modulation techniques for molecular communication via diffusion, in Modeling, Methodologies and Tools for Molecular and Nano-scale Communications: Modeling, Methodologies and Tools, Switzerland: Springer, 9 (2017), 99–118. https://doi.org/10.1007/978-3-319-50688-3-5 |
[15] |
M. U. Mahfuz, D. Makrakis, H. T. Mouftah, A comprehensive study of sampling-based optimum signal detection in concentration-encoded molecular communication, IEEE Trans. Nanobiosci., 13 (2014), 208–222. https://doi.org/10.1109/TNB.2014.2341693 doi: 10.1109/TNB.2014.2341693
![]() |
[16] | M. F. Ghazani, G. Aminian, M. Mirmohseni, A. Gohari, M. N. Kenari, Physical layer network coding in molecular two-way relay networks, In 2016 Iran Workshop on Communication and Information Theory (IWCIT), IEEE, Tehran, Iran, (2016), 1–6. https://doi.org/10.1109/IWCIT.2016.7491619 |
[17] |
P. Manocha, G. Chandwani, Design methodology of passive in-line relays for molecular communication in flow-induced microfluidic channel, Biosensors, 11 (2021), 65. https://doi.org/10.3390/bios11030065 doi: 10.3390/bios11030065
![]() |
[18] | H. Du, Q. Liu, K. Yang, Multi-hop relay directional communication based on attractants in molecular communication, In Proceedings of the 9th ACM International Conference on Nanoscale Computing and Communication, (2022), 1–3. https://doi.org/10.1145/3558583.3558866 |
[19] |
Y. J. Na, J. S. Jung, Y. H. You, H. K. Song, Adaptive relay selection scheme for minimization of the transmission time, Comput. Mater. Contin., 69 (2021), 1361–1373. https://doi.org/10.32604/cmc.2021.018481 doi: 10.32604/cmc.2021.018481
![]() |
[20] |
S. M. Abd El-atty, R. Bidar, ES. M. El-Rabaie, Embedded nano relay for intra-body network-based molecular communications. Wireless Pers. Commun., 125 (2022), 3049–3066. https://doi.org/10.1007/s11277-022-09697-9 doi: 10.1007/s11277-022-09697-9
![]() |
[21] |
N. Varshney, W. Haselmayr, W. Guo, On flow-induced diffusive mobile molecular communication: First hitting time and performance analysis, IEEE Trans. Mol. Biol. Multi-Scale Commun., 4 (2018), 195–207. https://doi.org/10.1109/TMBMC.2019.2928543 doi: 10.1109/TMBMC.2019.2928543
![]() |
[22] |
J. Angjo, A. E. Pusane, H. B. Yilmaz, E. Basar, T. Tugcu, Optimal relaying in molecular communications, Nano Commun. Netw., 32 (2022), 100404. https://doi.org/10.1016/j.nancom.2022.100404 doi: 10.1016/j.nancom.2022.100404
![]() |
[23] |
Z. Cheng, J. Sun, J. Yan, Y. Tu, Optimizations for mobile MIMO relay molecular communication via diffusion with network coding, KSII Trans. Internet Inf. Syst., 16 (2022), 1373–1391. https://doi.org/10.3837/tiis.2022.04.015 doi: 10.3837/tiis.2022.04.015
![]() |
[24] |
S. K. Tiwari, T. R. T. Reddy, P. K. Upadhyay, D. B. Da Costa, Joint optimization of molecular resource allocation and relay positioning in diffusive nanonetworks, IEEE Access, 6 (2018), 67681–67687. https://doi.org/10.1109/ACCESS.2018.2879159 doi: 10.1109/ACCESS.2018.2879159
![]() |
[25] |
N. Tavakkoli, P. Azmi, N. Mokari, Optimal positioning of relay node in cooperative molecular communication networks. IEEE Trans. Commun., 65 (2017), 5293–5304. https://doi.org/10.1109/TCOMM.2017.2737623 doi: 10.1109/TCOMM.2017.2737623
![]() |
[26] |
N. Tavakkoli, P. Azmi, N. Mokari, Performance evaluation and optimal detection of relay-assisted diffusion-based molecular communication with drift, IEEE Trans. Nanobioscience, 16 (2016), 34–42. https://doi.org/10.1109/TNB.2016.2626313 doi: 10.1109/TNB.2016.2626313
![]() |
[27] |
P. P. Ray, K. Skala, Internet of things aware secure dew computing architecture for distributed hotspot network: A conceptual study. Appl. Sci., 12 (2022), 8963. https://doi.org/10.3390/app12188963 doi: 10.3390/app12188963
![]() |
[28] |
M. C. Gursoy, A. E. Pusane, T. Tugcu, Molecule-as-a-frame: A frame based communication approach for nanonetworks. Nano Commun. Netw., 16 (2018), 45–59. https://doi.org/10.1016/j.nancom.2018.02.005 doi: 10.1016/j.nancom.2018.02.005
![]() |
[29] |
X. L. Wang, Z. Jia, Reliability analysis of molecular communication based on drift diffusion in different topologies, J. Comput. Commun., 08 (2020), 71. https://doi.org/10.4236/jcc.2020.81005 doi: 10.4236/jcc.2020.81005
![]() |
[30] |
M. F. O. Hameed, K. R. Mahmoud, S. S. A. Obayya, Metaheuristic algorithms for dispersion optimization of photonic crystal fibers, Opt. Quantum Electron., 48 (2016), 1–11. https://doi.org/10.1007/s11082-016-0418-0 doi: 10.1007/s11082-016-0418-0
![]() |
[31] |
K. R. Mahmoud, Synthesis of unequally‐spaced linear array using modified central force optimisation algorithm, IET Microw. Antennas Propag., 10 (2016), 1011–1021. https://doi.org/10.1049/iet-map.2015.0801 doi: 10.1049/iet-map.2015.0801
![]() |
[32] | N. Siddique, H. Adeli, Central force metaheuristic optimization, Sci. Iran., 6 (2015), 1941–1953. |
[33] |
Y. B. Chen, J. Q. Yu, Y. S. Mei, Y. F. Wang, X. L. Su, Modified central force optimization (MCFO) algorithm for 3D UAV path planning, Neurocomputing, 171 (2016), 878–888. https://doi.org/10.1016/j.neucom.2015.07.044 doi: 10.1016/j.neucom.2015.07.044
![]() |