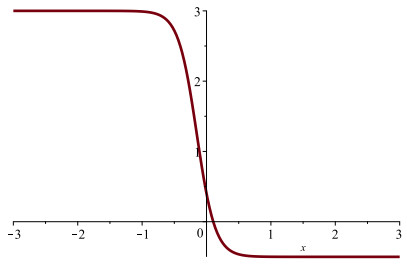
Autoimmune thyroiditis (AIT) is a chronic inflammatory that involves hyperactivation of the immune system against the thyroid gland, recognizing it as "nonself”. The aim of this research was to identify the relationships between genetic polymorphism in CTLA4, TNF-α and PTPN22 genes and the manifestation of AIT and levels of antithyroglobulin antibody (anti-TG Ab) and thyroid peroxidase antibody (anti-TPO Ab). The study was conducted during 2014–2020 and included 64 men and 106 women aged between 18 and 64 years with AIT. The control group consisted of 65 people (26 men, 39 women, aged between 20 and 65 years) without any thyroid pathologies or other autoimmune diseases. For molecular genetic analysis, real-time quantitative RT-PCR was used with fluorescently labeled FAM probes on a detection system CFX96 (BioRad). The results demonstrated that patients with the GG genotype and the G allele of the +49A/G polymorphism in the CTLA4 gene have significantly higher titers of anti-TG Ab. High titers of anti-TG Ab were detected in 22.4% of patients with the GG genotype (p = 0.005, χ2 = 7.86, OR = 0.237, 95% CI = 0.088–0.635), and in 55.6% of patients with the G allele (p = 0.0012, χ2 = 10.43, OR = 0.360, 95%, СI = 0.192–0.674). At the same time, the A allele of the +49A/G polymorphism is significantly more common in patients with normal anti-TG Ab values—in 68.1% of individuals (p = 0.0012, χ2 = 10.43, OR= 2.78, 95%, CI = 1.484–5.207). The results of the study indicate the prognostic significance of the G allele and the GG genotype of the +49A/G polymorphism of the CTLA4 gene predicting the probability of occurrence of anti-TG and anti-TPO antibodies.
Citation: Rena Rahimova. Relationship between CTLA4, TNF-α and PTPN22 gene polymorphism and the serum levels of antithyroglobulin and antiperoxidase antibodies in autoimmune thyroiditis[J]. AIMS Medical Science, 2023, 10(1): 14-23. doi: 10.3934/medsci.2023002
[1] | Ana Klobučar Barišić, Antoaneta Klobučar . Double total domination number in certain chemical graphs. AIMS Mathematics, 2022, 7(11): 19629-19640. doi: 10.3934/math.20221076 |
[2] | Bo Zhu, Shumin Zhang, Huifen Ge, Chengfu Ye . The $ g $-extra $ H $-structure connectivity and $ g $-extra $ H $-substructure connectivity of hypercubes. AIMS Mathematics, 2023, 8(10): 24848-24861. doi: 10.3934/math.20231267 |
[3] | Huifen Ge, Shumin Zhang, Chengfu Ye, Rongxia Hao . The generalized 4-connectivity of folded Petersen cube networks. AIMS Mathematics, 2022, 7(8): 14718-14737. doi: 10.3934/math.2022809 |
[4] | Abel Cabrera-Martínez, Andrea Conchado Peiró . On the $ \{2\} $-domination number of graphs. AIMS Mathematics, 2022, 7(6): 10731-10743. doi: 10.3934/math.2022599 |
[5] | Ahlam Almulhim . Signed double Italian domination. AIMS Mathematics, 2023, 8(12): 30895-30909. doi: 10.3934/math.20231580 |
[6] | Betül ATAY ATAKUL . Stability and domination exponentially in some graphs. AIMS Mathematics, 2020, 5(5): 5063-5075. doi: 10.3934/math.2020325 |
[7] | Shahid Zaman, Fouad A. Abolaban, Ali Ahmad, Muhammad Ahsan Asim . Maximum $ H $-index of bipartite network with some given parameters. AIMS Mathematics, 2021, 6(5): 5165-5175. doi: 10.3934/math.2021306 |
[8] | Abel Cabrera-Martínez, Andrea Conchado Peiró, Juan Manuel Rueda-Vázquez . Further results on the total Italian domination number of trees. AIMS Mathematics, 2023, 8(5): 10654-10664. doi: 10.3934/math.2023540 |
[9] | Zepeng Li . A note on the bounds of Roman domination numbers. AIMS Mathematics, 2021, 6(4): 3940-3946. doi: 10.3934/math.2021234 |
[10] | A. El-Mesady, Y. S. Hamed, M. S. Mohamed, H. Shabana . Partially balanced network designs and graph codes generation. AIMS Mathematics, 2022, 7(2): 2393-2412. doi: 10.3934/math.2022135 |
Autoimmune thyroiditis (AIT) is a chronic inflammatory that involves hyperactivation of the immune system against the thyroid gland, recognizing it as "nonself”. The aim of this research was to identify the relationships between genetic polymorphism in CTLA4, TNF-α and PTPN22 genes and the manifestation of AIT and levels of antithyroglobulin antibody (anti-TG Ab) and thyroid peroxidase antibody (anti-TPO Ab). The study was conducted during 2014–2020 and included 64 men and 106 women aged between 18 and 64 years with AIT. The control group consisted of 65 people (26 men, 39 women, aged between 20 and 65 years) without any thyroid pathologies or other autoimmune diseases. For molecular genetic analysis, real-time quantitative RT-PCR was used with fluorescently labeled FAM probes on a detection system CFX96 (BioRad). The results demonstrated that patients with the GG genotype and the G allele of the +49A/G polymorphism in the CTLA4 gene have significantly higher titers of anti-TG Ab. High titers of anti-TG Ab were detected in 22.4% of patients with the GG genotype (p = 0.005, χ2 = 7.86, OR = 0.237, 95% CI = 0.088–0.635), and in 55.6% of patients with the G allele (p = 0.0012, χ2 = 10.43, OR = 0.360, 95%, СI = 0.192–0.674). At the same time, the A allele of the +49A/G polymorphism is significantly more common in patients with normal anti-TG Ab values—in 68.1% of individuals (p = 0.0012, χ2 = 10.43, OR= 2.78, 95%, CI = 1.484–5.207). The results of the study indicate the prognostic significance of the G allele and the GG genotype of the +49A/G polymorphism of the CTLA4 gene predicting the probability of occurrence of anti-TG and anti-TPO antibodies.
Even though the roots of fractional calculus are in the late 17th century [1], the subject has received significant attention from researchers only in recent decades. The importance of fractional calculus and fractional differential equations (FDEs) is evidenced by the emergence of fractional-order models for real-world phenomena, which has lead to a plethora of applications of FDEs [2]. A short review of the areas in which FDEs are applied is given below.
The advantage of fractional-order models is that they can be used to model systems with the memory effect, under which the current state of the system at time t=t0 is affected by an interval of states at time t<t0. Thus, such models lend themselves naturally to the description of viscoelastic materials [3,4]. It can be noted that most biological tissues possess viscoelastic properties [5] which has lead to the use of FDEs to describe the evolution of various biomedical systems [6,7,8]. In physics, fractional-order models are widely used to study dielectric materials [9,10,11] and Bose-Einstein condensates [12]. FDEs have also been used to describe the memory effect in economic models [13,14].
Due to the applicability of fractional-order models, a wide variety of methods have been developed to construct solutions to FDEs. The Q-homotopy analysis transform is applied in order to obtain analytical solutions to the fractional coupled Ramani equation in [15]. The solutions of integer-order differential equations are used to construct the solutions to the models of cooling and spread of epidemic diseases [16]. An Adams-type predictor-corrector method for the construction of numerical solutions to FDEs is discussed in [17]. A new family of predictor-corrector methods for FDEs is discussed in [18]. A Legendre operational matrix approach is used to numerically solve FDEs in [19,20].
The homotopy analysis method is adapted to FDEs for the computation of approximate solutions to various equations, including fractional Korteweg-de-Vries, Burgers and Boussinesq models in [21]. A class of rational Krylov methods for the construction of numerical solutions to partial fractional differential equations is considered in [22]. The traditional reproducing kernel method is adapted to be applicable to FDEs in [23]. A restricted transform technique is used to solve irrational order FDEs in [24]. Artificial neural networks are used to construct approximate solutions to FDEs in [25].
The main objective of this paper is to present a novel framework for the extension of the solutions to fractional differential equation (FDEs) to negative argument values. The main idea of this procedure is to construct the ordinary differential equation (ODE) that has a solution which can be transformed to the solution of the considered FDE. Since the solution of the ODE also exists for negative argument values, it can be used to extend the solution of the FDE for such argument values as well. This results in complex-valued solutions to FDEs for negative argument values. Such extension is a novel viewpoint into the solutions to FDEs, as they can be analyzed not only in the set x∈R+, but in the entire real line x∈R. This goes beyond the current state-of-the-art, as with current algorithms the solutions to FDEs cannot be considered in the negative x-axis.
In this paper we consider functions defined via power series:
f(x)=+∞∑j=0vjw(n)j, | (2.1) |
where vj∈R are coefficients of the series and the base functions w(n)j;n∈N,j=0,1,… are defined as:
w(n)j(x)=xjnΓ(jn+1);n∈N,j=0,1,…. | (2.2) |
The usual notation Γ(x) is used to denote the gamma function [26].
The number n which denotes the order of the basis of fractional power series is selected with respect to the fractional derivative order which is analyzed. If the fractional derivative is of order α=km, and gcd(k,m)=1, it is taken that n=m [27].
Consider x>0 and let n√x∈R. Using the substitution t:=n√x, (2.1) can be rewritten as:
f(x)=ˆf(t)=+∞∑j=0γj(n√x)j=+∞∑j=0γjtj,γj=vjΓ(jn+1). | (2.3) |
For subsequent computations it is required that ˆf(t) be analytic (except for some finite number of singularity points) and non-singular at t=0. Thus, there exists a convergence radius |t|<T0;T0>0. The function ˆf will be referred to as the characteristic function of f.
For any given n∈N, the set of all functions defined by (2.1) and meeting the requirements stated above is denoted as CFn. Conventional addition and multiplication operations on functions belonging to CFn are defined. For example, given two series f=∑+∞j=0vjw(n)j and g=∑+∞j=0bjw(n)j, the product is defined as:
f⋅g=+∞∑j=0(j∑k=0(jk)vkbj−k)w(n)j. | (2.4) |
Note that the sum above obeys the finite summation principle: all coefficients of the above series are given by finite sums, not series [28].
The set CFn with addition and multiplication operations forms an algebra over C. The properties of algebra CFn are discussed in detail in [27] and [29].
Let f=∑+∞j=0vjw(n)j∈CFn. Caputo differentiation operator of order 1n is then defined as [30]:
CD(1/n)f=+∞∑j=0vj+1w(n)j. | (2.5) |
Note the Caputo derivatives of basis functions [27]:
CD(1/n)w(n)j={0,j=0w(n)j−1,j=1,2,… | (2.6) |
Derivatives of rational order kn;k∈N are defined by higher powers of operator CD(1/n) [29]:
(CD(1/n))kf=+∞∑j=0vj+kw(n)j=+∞∑j=0Γ(j+kn+1)γj+kΓ(jn+1)(n√x)j. | (2.7) |
In the special case of k=m⋅n;m∈N, (2.7) reads:
(CD(1/n))m⋅nf=+∞∑j=0Γ(jn+m+1)Γ(jn+1)γj+mn(n√x)j=+∞∑j=0(m∏k=1(jn+k))γj+mn(n√x)j. | (2.8) |
It is well-known that analytic functions can be extended beyond their convergence radius [31]. The same idea is adapted for series of the type (2.1). Let f=∑+∞j=0γj(n√x)j and choose n√x0 such that x0 is nonzero and in the characteristic function's convergence radius, 0<x0<Tn0. Then (2.1) can be rearranged:
f=+∞∑j=0γj((n√x−n√x0)+n√x0)j=+∞∑j=0j∑k=0(jk)γj(n√x0)j−k(n√x−n√x0)k=+∞∑k=0(+∞∑j=k(jk)γj(n√x0)j−k)(n√x−n√x0)k=+∞∑j=0ˆγj(n√x−n√x0)j. | (2.9) |
Coefficients ˆγj are defined as:
ˆγj=+∞∑k=j(kj)γk(n√x0)k−j. | (2.10) |
Note that coefficients (2.10) are finite, because the non-extended series f converges in its convergence radius 0<x0<Tn0. The convergence radius for (2.9) is |n√x−n√x0|<T1,T1>0. Furthermore, if the same procedure is repeated for x1≠x0 that also satisfies 0<x1<Tn0, then:
+∞∑j=0ˆγj(n√x−n√x0)j=+∞∑j=0˜γj(n√x−n√x1)j, | (2.11) |
as long as x is such that series on both sides of the equation converge.
This procedure can be applied to extend a function from the set CFn between two singularity points (or singularity and infinity, if no other singularities are present) by rewriting the function in different basis (n√x−n√xk)j,k=0,1,…. Note that these extended series coincide for x that lies in all of their convergence radii, thus the extensions are unique.
Note that computational operations, such as the Caputo differentiation operator are only defined for basis (n√x)j (in the neighbourhood of x=0). For this reason, all computations are first performed in this neighbourhood and then extended throughout the entire function domain. Thus, the zero neighbourhood of x is called the algebraic operation neighbourhood. The concept of this neighbourhood is introduced in [28].
In the subsequent sections of this paper, constructing series solutions to ordinary differential equations (ODEs) is necessary. The generalized differential operator technique is used for this task. A short description of this method is given in this section. A detailed overview can be found in [32,33].
Let us consider an ordinary differential equation in the explicit form:
dz dt=P(t,z);z(c)=s;c,s∈R, | (2.12) |
where P is an arbitrary bivariate analytic function. The following generalized differential operator is associated with the ODE (2.12) [34]:
Dcs=Dc+P(c,s)Ds, | (2.13) |
where Dλ denotes the partial differentiation operator with respect to variable λ.
The series solution to (2.12) can then be written in the form [32]:
z(t,c,s)=+∞∑j=0(t−c)jj!pj(c,s), | (2.14) |
where pj(c,s)=Djcss.
The following fractional differential equation is considered in this section:
(CD(1/n))nyn=Qm(yn);yn=yn(x), | (3.1) |
where yn∈CFn and Qm is an arbitrary mth order polynomial:
Qm(y)=m∑k=0akyk;m∈N,am≠0. | (3.2) |
In the remainder of this section, solutions in the operation neighbourhood of x=0 will be constructed directly and later extended into different neighbourhoods using the procedure described in previous sections.
Consider a series yn=∑+∞j=0vjw(n)j=∑+∞j=0γj(n√x)j∈CFn. Inserting the function into (3.1) yields:
+∞∑j=0(jn+1)γj+n(n√x)j=m∑k=1(ak(+∞∑j=0(∑k1+⋯+km=jk∏l=1γkl)(n√x)j))+a0. | (3.3) |
Let n√x=t and ˆyn=∑+∞j=1γjtj. Then, (3.3) yields:
+∞∑j=0(j+n)γj+ntj=nQm(ˆyn). | (3.4) |
Rearranging the sums in (3.4) yields:
+∞∑j=njγjtj−1=ntn−1Qm(ˆyn). | (3.5) |
It can be noted that adding ∑n−1j=1jγjtj−1 to both sides of (3.5) transforms the right side to an ordinary derivative of ˆyn:
dˆyndt=ntn−1Qm+n−1∑j=1jγjtj−1. | (3.6) |
Noting that γj=vjΓ(jn+1) yields a different form of (3.6):
dˆyndt=n(tn−1Qm(ˆyn)+n−1∑j=1vjΓ(jn+1)tj−1). | (3.7) |
Adding the initial condition yn(c)=s, Equation (3.7) can be solved using the method described in 2.4. The generalized differential operator for (3.7) reads:
Dcs=Dc+n(cn−1Qm(s)+n−1∑j=1vjΓ(jn+1)cj−1)Ds. | (3.8) |
The solution to (3.7) (taking values of t for which the series converges) has the form:
ˆyn=ˆyn(t,c,s)=+∞∑j=0(t−c)jj!pj(c,s), | (3.9) |
where pj(c,s)=Djcss. Noting that yn(x)=ˆyn(n√x) yields Theorem 3.1.
Theorem 3.1. The fractional differential equation (3.1) admits the following general solution for any parameter values u0;v1,v2,…,vn−1;x0≥0:
yn(x)=+∞∑j=0(n√x−n√x0)jj!pj(n√x0,u0), | (3.10) |
where
yn(n√x0)=u0, | (3.11) |
and
(CD(1/n))kyn|x=0=vk;k=1,…,n−1, | (3.12) |
if x satisfies |n√x−n√x0|<Tx0. Here Tx0>0 denotes the convergence radius of (3.10).
Note that the results of this section can be extended to analytic functions. Taking Q(x)=∑+∞k=0akxk instead of Qm(x) and following the steps outlined above would yield a theorem that is analogous to Theorem 3.1. However, the analysis of such equations is not the focus of this paper, thus we will consider only polynomial Qm(x) in the remainder of the text.
Based on Theorem 3.1, the Cauchy initial value problem for (3.1) can be formulated at point x=0:
(CD(1/n))nyn=Qm(yn),yn=yn(x,u(0)0); | (3.13) |
(CD(1/n))kyn|x=0=vk;k=1,…,n−1; | (3.14) |
yn|x=0=u(0)0. | (3.15) |
Note that since n√x in general possesses n branches, in these computations we select the branch with a minimum value of argx.
By the results obtained in the previous section, in the neighbourhood of x=0, the problem (3.13)–(3.15) has the solution:
yn=+∞∑j=0(n√x)jj!pj(0,u(0)0). | (3.16) |
This solution can be extended by applying the algorithm discussed in section 2.3. First, choose a sequence x0=0<x1<x2<… and freely choose u(0)0∈R. Then, compute parameters u(1)0,u(2)0,… as:
u(k+1)0=ˆyn(n√xk+1,n√xk,u(k)0),k=0,1,… | (3.17) |
The general solution to (3.13)–(3.15) can then be written for any k:
yn=yn(x,u(0)0)=ˆyn(n√x,n√xk,u(k)0), | (3.18) |
for |n√x−n√xk|<Txk, Txk>0,xk≥0.
The rearrangements described in the previous section can be applied to construct a more general Cauchy initial value problem, where the first initial condition can be formulated at nonzero x:
(CD(1/n))nyn=Qm(yn),yn=yn(x,u(0)0); | (3.19) |
(CD(1/n))kyn|x=0=vk;k=1,…,n−1; | (3.20) |
yn|x=x0=u(0)0;x0≠0.. | (3.21) |
Analogously to problem (3.13)–(3.15), the solution reads:
yn=yn(x,x0,u(0)0)=ˆyn(n√x,n√x0,u(0)0). | (3.22) |
Note that initial conditions that correspond to fractional derivative values still must be formulated at x=0 for solution (3.22) to hold true.
As stated earlier, the main objective of this paper is to extend the solutions to FDEs so that they would exist for negative values of argument x. This extension results in complex-valued fractional power series, which are defined in the following subsection. Note that this extension is not possible when considering fractional derivatives in the traditional Caputo sense, as they are defined via integrals only for non-negative values of x.
Let us consider an extension to the power series basis presented in section 2.1:
(w(n)j)k:=(n√|x|)jΓ(jn+1)exp(ijargx+2πkn), | (4.1) |
where x∈R, k=0,…,n−1;j=0,1,… and n√|x|∈R denotes the root with the lowest value of argx. The basis with k=0 corresponds to w(n)j presented in section 2.1, while k=1,2,…,n−1 result in complex-valued functions (w(n)j)k.
Using (4.1), the standard fractional power series (2.1) can be extended into n complex-valued series:
fk(x)=+∞∑j=0vj(w(n)j)k;k=0,…,n−1. | (4.2) |
By Theorem 3.1, the solution to
(CD(1/n))nyn=Qm(yn);yn=yn(x), | (4.3) |
reads:
yn(x)=+∞∑j=0(n√x−n√x0)jj!pj(n√x0,u0), | (4.4) |
where coefficients pj are generated via the generalized differential operator technique as described in previous sections. Using the basis defined in the previous section, the solution (4.4) can extended into the complex plane:
(yn(x))k=+∞∑j=0(n√|x|−n√|x0|)jj!exp(ijargx0+2πkn)pj(n√|x0|exp(iargx0+2πkn),u0). | (4.5) |
Denoting α:=argx0 and β(k)n(α):=exp(iα+2πkn), series (4.5) can be rewritten as:
(yn(x))k=+∞∑j=0(n√|x|−n√|x0|)jj!(λ(k)j(|x0|,α,u0)+iμ(k)j(|x0|,α,u0)), | (4.6) |
where
λ(k)j(|x0|,α,u0):=Re((β(k)n(α))jpj(n√|x0|β(k)n(α),u0)); | (4.7) |
μ(k)j(|x0|,α,u0):=Im((β(k)n(α))jpj(n√|x0|β(k)n(α),u0)). | (4.8) |
Expression (4.6) allows the consideration of solutions for x<0, however, these solutions are multi-valued (a total of n branches corresponding to the number of distinct roots n√x) and take complex values.
A refinement of the procedure described in section 3.2 is needed to construct particular solutions to FDEs (4.3). Two sequences of numbers are chosen: ⋯<x−r<⋯<x−1<0; 0<x1<x2<… and x0=0. For any k=0,…,n−1, the initial condition s(k)0∈R can be taken freely.
Sequences s(k)−r and s(k)r are computed using relations:
s(k)r+1:=ˆy((n√xr+1)k,(n√xr)k,s(k)r);r=0,1,…; | (4.9) |
s(k)−r−1:=ˆy((n√x−r−1)k,(n√x−r)k,s(k)−r);r=0,1,…. | (4.10) |
Here ˆy denotes the solution of the ODE that the FDE (4.3) is transformed to, as given by Theorem 3.1.
Then the particular solution to (4.3) corresponding to the kth branch of the root n√x reads:
(yn(x))k=ˆy((n√x)k;(n√xr)k;s(k)r), | (4.11) |
where r=0,±1,±2,….
Note that the sequence ⋯<x−1<x0<x1<… must be chosen in such a way that the series generated by ˆy are convergent in both R and C. Also, the point x=0 is a branching point for the solution to the FDE, thus the functions (yn(x))k are non-differentiable at this point.
Let us consider the non-fractional Riccati equation:
dy dx=2y2−5y−3; | (5.1) |
y(x0)=u0. | (5.2) |
It is well-known that (5.1) admits the kink solitary solution:
y(x,x0,u0)=α2(u0−α1)exp(2α1(x−x0))−α1(u0−α2)exp(2α2(x−x0))(u0−α1)exp(2α1(x−x0))−(u0−α2)exp(2α2(x−x0)), | (5.3) |
where α1=3,α2=−12 are the roots of polynomial 2y2−5y−3. Solution (5.3) is depicted in Figure 1.
Suppose that n=2, x0=0 and let us consider the fractional Riccati equation:
(CD(1/2))2y=2y2−5y−3. | (5.4) |
As stated earlier, the initial conditions read:
CD(1/2)y|x=0=v1;y(0)=u(0)0. | (5.5) |
The solution to (5.4), (5.5) is constructed using the algorithm described in section 4.3. Note that since √x possesses two branches, two solutions exist. The real and imaginary parts of these solutions are shown in Figure 2. Note that solution is purely real for x≥0 – the imaginary parts appears only for negative values of x.
It can be seen in Figure 3 that as v1 approaches zero, the solutions to (5.4) approach the kink solitary solution (5.3).
Taking n=3 results in the fractional Riccati equation:
(CD(1/3))3y=2y2−5y−3. | (5.6) |
The number of initial conditions for (5.6) increases to three:
CD(1/3)y|x=0=v1;(CD(1/3))2y|x=0=v2;y(0)=u(0)0. | (5.7) |
Riccati equation (5.6) has three solutions: two of them are complex-valued and one is a real-valued solution. The real and imaginary parts of these solutions are depicted in Figure 4. A spatial plot for two different sets of initial conditions is shown in Figure 5.
Note that the as x→±∞, the fractional solitary solutions approach the non-fractional kink solitary solution. This is due to the properties of the characteristic equation (3.6). Thus, the limits of the fractional and non-fractional coincide as x→±∞.
An analytical framework for the extension of solutions to FDEs into the negative half-line is presented in this paper. The main idea of this extension is the construction of a characteristic differential equation for a given FDE. The solution to the characteristic equation is used to construct a fractional power series solution to the FDE. This solution is then extended using classical Riemann extension techniques to enable the consideration of the solution at a neighbourhood different than the origin x=0. Furthermore, this extension is valid for both positive and negative values of x, which extends the solution to the entire real line.
As demonstrated by computational experiments, the solution to the FDE is complex-valued for negative values of x. Furthermore, there exist n branches of solutions, where n is the denominator of the fractional differentiation order. If the initial conditions (3.20) that correspond to fractional derivatives tend to zero, the solution to the FDE tends to the non-fractional solution of the same equation.
The presented technique provides a solid foundation for the construction of solutions to FDEs on the negative half-line. In particular, this allows to travel backwards in time from the initial conditions of a FDE – such a possibility has not been reported previously. It is well-known that fractional order derivatives help to model memory effects in dynamical systems. Therefore it would be tempting to extend the available coronavirus pandemic models [35,36] by introducing fractional derivatives. However, the results of this paper show that such an extension would eliminate the possibility of the unique backwards extrapolation. These questions as well as the physical interpretation of the extensions to the negative half-line remain a definite objective of future research.
This research was funded by a grant (No. S-COV-20-8) from the Research Council of Lithuania.
All authors declare no conflicts of interest in this paper.
[1] |
Ragusa F, Fallahi P, Elia G, et al. (2019) Hashimotos' thyroiditis: epidemiology, pathogenesis, clinic and therapy. Best Pract Res Clin Endocrinol Metab 33: 101367. https://doi.org/10.1016/j.beem.2019.101367 ![]() |
[2] |
Weetman A (2020) Autoimmune thyroid disease. Endocrine 68: 258-260. https://doi.org/10.1007/s12020-020-02188-6 ![]() |
[3] |
Rayman MP (2019) Multiple nutritional factors and thyroid disease, with particular reference to autoimmune thyroid disease. Proc Nutr Soc 78: 34-44. https://doi.org/10.1017/S0029665118001192 ![]() |
[4] |
Antonelli A, Ferrari SM, Corrado A, et al. (2015) Autoimmune thyroid disorders. Autoimmun rev 14: 174-180. https://doi.org/10.1016/j.autrev.2014.10.016 ![]() |
[5] |
Caturegli P, De Remigis A, Rose NR (2014) Hashimoto thyroiditis: clinical and diagnostic criteria. Autoimmun rev 13: 391-397. https://doi.org/10.1016/j.autrev.2014.01.007 ![]() |
[6] | Rozhko VA (2019) The current state of the problem of autoimmune thyroiditis. Probl Health Ecol 60: 4-13. https://doi.org/10.51523/2708-6011.2019-16-2-1 (in Russian) |
[7] |
Mattozzi S, Sabater L, Escudero D, et al. (2020) Hashimoto encephalopathy in the 21st century. Neurology 94: e217-e224. https://doi.org/10.1212/WNL.0000000000008785 ![]() |
[8] |
Rahimova RR (2022) Autoimmune thyroiditis (review of literature). Russ Clin Lab Diagn 67: 286-291. https://doi.org/10.51620/0869-2084-2022-67-286-291 (in Russian) ![]() |
[9] |
Ralli M, Angeletti D, Fiore M, et al. (2020) Hashimoto's thyroiditis: An update on pathogenic mechanisms, diagnostic protocols, therapeutic strategies, and potential malignant transformation. Autoimmun Rev 19: 102649. https://doi.org/10.1016/j.autrev.2020.102649 ![]() |
[10] |
Steiner J, Schiltz K, Stoecker W, et al. (2020) Association of thyroid peroxidase antibodies with anti-neuronal surface antibodies in health, depression and schizophrenia—complementary linkage with somatic symptoms of major depression. Brain Behav Immun 90: 47-54. https://doi.org/10.1016/j.bbi.2020.07.039 ![]() |
[11] |
Calcaterra V, Nappi RE, Regalbuto C, et al. (2020) Gender differences at the onset of autoimmune thyroid diseases in children and adolescents. Front Endocrinol 11: 229. https://doi.org/10.3389/fendo.2020.00229 ![]() |
[12] |
Stasiak M, Lewiński A (2021) New aspects in the pathogenesis and management of subacute thyroiditis. Rev Endocr Metab Disord 22: 1027-1039. https://doi.org/10.1007/s11154-021-09648-y ![]() |
[13] | Stojković M (2022) Thyroid function disorders. Arch Pharm 72: 429-443. https://doi.org/10.5937/arhfarm72-39952 |
[14] |
Komisarenko YI, Bobryk MI (2018) Vitamin D deficiency and immune disorders in combined endocrine pathology. Front Endocrinol 9: 600. https://doi.org/10.3389/fendo.2018.00600 ![]() |
[15] |
Abazova ZK, Borukaeva IK (2019) Hypoxic therapy in the correction of neuroimmunoendocrine disorders in autoimmune thyroiditis. Med Acad J 19: 49-51. ![]() |
[16] | Rahimova RR (2022) On the issue of prediction of autoimmune thyroiditis. Azerbaijan Med J 2: 64-71. https://doi.org/10.34921/amj.2022.2.010 (in Russian) |
[17] |
Kraus AU, Penna-Martinez M, Shoghi F, et al. (2019) HLA-DQB1 position 57 defines susceptibility to isolated and polyglandular autoimmunity in adults: interaction with gender. J Clin Endocrinol Metab 104: 1907-1916. https://doi.org/10.1210/jc.2018-01621 ![]() |
[18] | Bolotskaya LA, Tarlyun AA (2017) Pathophysiological significance of autoimmune markers for autoimmune thyroiditis onset in the middle OB residents. Vestnik SurGU Medicina 2: 31-35. Available from: https://surgumed.elpub.ru/jour/article/view/79?locale=en_US (in Russian) |
[19] |
Guliyeva SR, Guliyeva FE, Abiyev HA, et al. (2022) Autoimmune thyroid diseases and genetic factors. World J Adv Res Rev 16: 690-696. https://doi.org/10.30574/wjarr.2022.16.1.1038 ![]() |
[20] |
Gu LQ, Zhu W, Pan CM, et al. (2010) Tumor necrosis factor alpha (TNF-α) polymorphisms in Chinese patients with Graves' disease. Clin Biochem 43: 223-227. https://doi.org/10.1016/j.clinbiochem.2009.08.012 ![]() |
[21] |
Stasiak M, Tymoniuk B, Michalak R, et al. (2020) Subacute thyroiditis is associated with HLA-B*18:01, -DRB1*01 and -C*04:01—the significance of the new molecular background. J Clin Med 9: 534. https://doi.org/10.3390/jcm9020534 ![]() |
[22] |
Passali M, Josefsen K, Frederiksen JL, et al. (2020) Current evidence on the efficacy of gluten-free diets in multiple sclerosis, psoriasis, type 1 diabetes and autoimmune thyroid diseases. Nutrients 12: 2316. https://doi.org/10.3390/nu12082316 ![]() |
[23] |
Vieira IH, Rodrigues D, Paiva I (2020) Vitamin D and autoimmune thyroid disease—cause, consequence, or a vicious cycle?. Nutrients 12: 2791. https://doi.org/10.3390/nu12092791 ![]() |
[24] |
Wawrusiewicz-Kurylonek N, Koper-Lenkiewicz OM, Gościk J, et al. (2019) Association of PTPN22 polymorphism and its correlation with Graves' disease susceptibility in Polish adult population-A preliminary study. Mol Genet Genomic Med 7: e661. https://doi.org/10.1002/mgg3.661 ![]() |
[25] | Weetman A, DeGroot LJ (2016) Autoimmunity to the Thyroid Gland. [Updated 2016 Jan 14]. In: Feingold KR, Anawalt B, Boyce A, et al., editors. Endotext [Internet]. South Dartmouth (MA): MDText.com, Inc. 2000–. Available from: https://www.ncbi.nlm.nih.gov/books/NBK285552/ |
1. | Inga Timofejeva, Zenonas Navickas, Tadas Telksnys, Romas Marcinkevicius, Minvydas Ragulskis, An Operator-Based Scheme for the Numerical Integration of FDEs, 2021, 9, 2227-7390, 1372, 10.3390/math9121372 | |
2. | R. Marcinkevicius, I. Telksniene, T. Telksnys, Z. Navickas, M. Ragulskis, The construction of solutions to $ {}^{C} \mathit{\boldsymbol{{D}}}^{(1/n)} $ type FDEs via reduction to $ \left({}^{C} \mathit{\boldsymbol{{D}}}^{(1/n)}\right)^n $ type FDEs, 2022, 7, 2473-6988, 16536, 10.3934/math.2022905 |