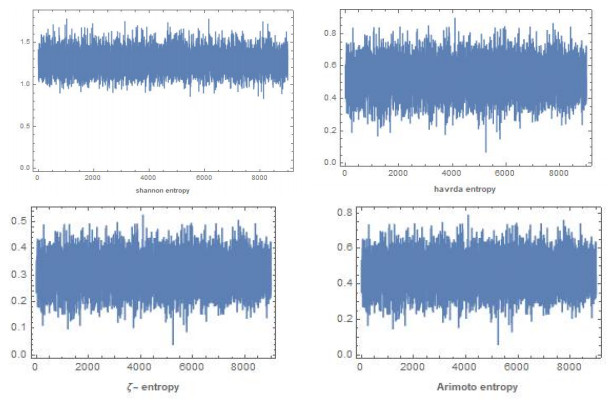
Given the application domains of soft set theory, such as decision-making processes, image processing, machine learning, and data mining, it is natural to consider that this theory could be utilized more effectively in encryption systems. A review of the literature reveals that soft set-based encryption systems have been explored in a limited number of studies. This study seeks to develop a new approach for soft sets in encryption systems by utilizing newly introduced algebraic and topological tools. In this system, parties will be able to generate encryption keys independently using soft sets they determine themselves rather than through prior mutual agreement. Additionally, the method of key generation and the size of the key space in the resulting encryption system provides a more secure and distinct alternative compared to existing soft set-based encryption systems.
Citation: Erdal Bayram, Gülşah Çelik, Mustafa Gezek. An advanced encryption system based on soft sets[J]. AIMS Mathematics, 2024, 9(11): 32232-32256. doi: 10.3934/math.20241547
[1] | Refah Alotaibi, Mazen Nassar, Zareen A. Khan, Wejdan Ali Alajlan, Ahmed Elshahhat . Entropy evaluation in inverse Weibull unified hybrid censored data with application to mechanical components and head-neck cancer patients. AIMS Mathematics, 2025, 10(1): 1085-1115. doi: 10.3934/math.2025052 |
[2] | Baria A. Helmy, Amal S. Hassan, Ahmed K. El-Kholy, Rashad A. R. Bantan, Mohammed Elgarhy . Analysis of information measures using generalized type-Ⅰ hybrid censored data. AIMS Mathematics, 2023, 8(9): 20283-20304. doi: 10.3934/math.20231034 |
[3] | Abdullah Ali H. Ahmadini, Amal S. Hassan, Ahmed N. Zaky, Shokrya S. Alshqaq . Bayesian inference of dynamic cumulative residual entropy from Pareto Ⅱ distribution with application to COVID-19. AIMS Mathematics, 2021, 6(3): 2196-2216. doi: 10.3934/math.2021133 |
[4] | Xue Hu, Haiping Ren . Statistical inference of the stress-strength reliability for inverse Weibull distribution under an adaptive progressive type-Ⅱ censored sample. AIMS Mathematics, 2023, 8(12): 28465-28487. doi: 10.3934/math.20231457 |
[5] | Abdulhakim A. Al-Babtain, Amal S. Hassan, Ahmed N. Zaky, Ibrahim Elbatal, Mohammed Elgarhy . Dynamic cumulative residual Rényi entropy for Lomax distribution: Bayesian and non-Bayesian methods. AIMS Mathematics, 2021, 6(4): 3889-3914. doi: 10.3934/math.2021231 |
[6] | M. R. Irshad, S. Aswathy, R. Maya, Amer I. Al-Omari, Ghadah Alomani . A flexible model for bounded data with bathtub shaped hazard rate function and applications. AIMS Mathematics, 2024, 9(9): 24810-24831. doi: 10.3934/math.20241208 |
[7] | Ayed. R. A. Alanzi, Muhammad Imran, M. H. Tahir, Christophe Chesneau, Farrukh Jamal, Saima Shakoor, Waqas Sami . Simulation analysis, properties and applications on a new Burr XII model based on the Bell-X functionalities. AIMS Mathematics, 2023, 8(3): 6970-7004. doi: 10.3934/math.2023352 |
[8] | Magdy Nagy, Khalaf S. Sultan, Mahmoud H. Abu-Moussa . Analysis of the generalized progressive hybrid censoring from Burr Type-Ⅻ lifetime model. AIMS Mathematics, 2021, 6(9): 9675-9704. doi: 10.3934/math.2021564 |
[9] | Qin Gong, Laijun Luo, Haiping Ren . Unit compound Rayleigh model: Statistical characteristics, estimation and application. AIMS Mathematics, 2024, 9(8): 22813-22841. doi: 10.3934/math.20241110 |
[10] | Monthira Duangsaphon, Sukit Sokampang, Kannat Na Bangchang . Bayesian estimation for median discrete Weibull regression model. AIMS Mathematics, 2024, 9(1): 270-288. doi: 10.3934/math.2024016 |
Given the application domains of soft set theory, such as decision-making processes, image processing, machine learning, and data mining, it is natural to consider that this theory could be utilized more effectively in encryption systems. A review of the literature reveals that soft set-based encryption systems have been explored in a limited number of studies. This study seeks to develop a new approach for soft sets in encryption systems by utilizing newly introduced algebraic and topological tools. In this system, parties will be able to generate encryption keys independently using soft sets they determine themselves rather than through prior mutual agreement. Additionally, the method of key generation and the size of the key space in the resulting encryption system provides a more secure and distinct alternative compared to existing soft set-based encryption systems.
The Weibull distribution is of particular importance since it naturally follows the extreme value theorem [1] and has a useful physical interpretation in numerous practical applications. In various engineering applications, such as independent component analysis, image analysis, genetic analysis, and time delay estimation, it is useful to estimate the entropy of a system or process given some observations (see [2,3,4,5]). The cumulative distribution function (CDF) and probability density function (PDF) of this distribution, for x>0, are, respectively, as follows:
F(x)=1−e−λxβ, | (1.1) |
and
f(x)=βλxβ−1e−λxβ, | (1.2) |
where β>0 is the shape parameter and λ>0 is the scale parameter.
The entropy in the Weibull distribution for progressive censoring was analyzed by Cramer and Bag [6], and Cho et al. [7] using generalized progressive Type Ⅱ hybrid censored samples to develop estimators for the entropy function of a Weibull distribution. Entropy estimation for an inverse Weibull distribution using multiple censoring samples has been discussed by Hassan and Zaky [8]. The estimation of entropy for the Weibull distribution based on record values was considered by Chacko and Asha [9].
It is advisable to end the test before all of the items fail because most trials in life are time- and money-constrained. The observations arising from that situation are referred to as censored samples, and many censoring methods exist. Two of the most prevalent forms of censorship are Type Ⅱ (T-Ⅱ) and Type Ⅰ (T-Ⅰ). Childs et al. [10] combined T-Ⅰ and T-Ⅱ censoring to create a hybrid censoring scheme (HCS), which is divided into two categories: T-Ⅰ HCS and T-Ⅱ HCS. These two variants have been widely implemented in various research studies. Chandrasekar et al. [11] expanded these methods by introducing two new forms, termed generalized T-Ⅰ HCS (GT-Ⅰ HCS) and generalized T-Ⅱ HCS (GT-Ⅱ HCS). The GT-Ⅱ HCS allows for a flexible censoring scheme that combines T-Ⅰ and T-Ⅱ censoring, accommodating various censoring patterns observed in real-world data. In many practical scenarios, data may exhibit a combination of T-Ⅰ and T-Ⅱ censorship due to different reasons, such as administrative constraints, equipment failures, or study design considerations. For some recent studies, see [12,13,14].
Entropy is a measure of uncertainty in a random variable and is used in information theory to determine the expected value of the information contained in that random variable. In many fields, including statistics, physics, chemistry, economics, insurance, financial analysis, and biological phenomena, measuring entropy is important. Less information in a sample is referred to as having more entropy. One of the most popular ways to estimate entropy is Shannon's entropy. This measure has proven to be successful in the research of many applications. One of Shannon's measure's biggest drawbacks is that it could be negative for specific probability distributions, making it useless as a measure of uncertainty. Measures of uncertainty, including ζ-entropy, Arimoto entropy, and Havrda and Charvat entropy, which are the subject of our attention, are explained in Section 2.
Numerous academics have researched entropy estimates for various life distributions. Cui and Ding [15] studied the convergence of Rényi entropy of the normalized sums of independent, identically distributed random variables. Entropy estimators for a double exponential distribution were created by Kang et al. [16], and Cho et al. [17] addressed entropy estimates for the Rayleigh distribution using double G-Ⅱ HCS. Cho et al. [18] used generalized progressive T-Ⅱ HCS to derive estimators for the entropy measure of a Weibull distribution. Ahmadini et al. [19] examined a Bayesian estimator of dynamic cumulative residual entropy based on the Parto Ⅱ distribution. Entropy estimators for the Lomax distribution have been considered, respectively, by Al-Babtain et al. [20], and Hassan and Zaki [21]. Al-Omari et al. [22] used record data to investigate an entropy Bayesian estimator for an extended inverse exponential distribution. For more recent studies, see [23,24,25,26,27].
The goal of this work is to examine the challenges associated with estimating uncertainty measures of the Weibull distribution using the GT-Ⅱ HCS. We were motivated to investigate this issue due to the significance of the Weibull distribution in various fields, including survival analysis and reliability engineering. Uncertainty measures are essential as they reflect the degree of confidence in the estimates and help in making informed decisions in practical applications. Moreover, the GT-Ⅱ HCS provides flexibility, realism, efficiency, and a comprehensive analysis framework for studies in these areas. Notably, there are no existing studies that have utilized the GT-Ⅱ HCS in conjunction with various entropy measures in this context. The current work will now be summarized as follows:
● The maximum likelihood (ML) and Bayesian estimators of Shannon entropy, ζ-entropy, Arimoto entropy, and Havrda and Charvat entropy are addressed using the GT-Ⅱ HCS.
● The asymmetric loss function (ASLOF) and the symmetric loss function (SLOF) are both used in the formulation of the Bayesian estimator.
● Asymptotic confidence intervals (ACIs), based on the delta method, and the highest posterior density (HPD) intervals are established.
● The complicated forms of different entropy estimates and how to construct the HPD intervals need the use of the Metropolis-Hastings (M-H) algorithm in the Markov chain Monte Carlo (MCMC) approach.
● To evaluate the performance of different entropy metrics, Monte Carlo simulations were conducted using accuracy measures such as mean squared errors (MSEs), average lengths (ALs), and coverage probabilities (CPs). Additionally, the inferential approaches presented in this paper were applied to real-world data to demonstrate their effectiveness.
This paper is structured as follows: Section 2 provides the derivation of the formulae for the entropy measures. Section 3 examines the four various kinds of entropy measurements under the GT-Ⅱ HCS using both classical and Bayesian techniques. Also, in Section 3, the MCMC procedure is used to get the Bayesian estimates based on the Metropolis-Hastings algorithm. The discussion of the simulation issue and its application using actual data sets is covered in Section 4. The paper concludes with a series of final remarks and observations in Section 5.
In this section, we derive analytical formulas for Shannon, Arimoto, Havrda and Charvat, and ζ-entropy measures for the Weibull distribution.
Shannon entropy is defined as follows:
E1=−∫∞−∞f(x)lnf(x)dx. | (2.1) |
Let X be a random variable following the Weibull distribution. Then from Eqs (1.2) and (2.1), and according to Cho et al. [7], Shannon entropy takes the following form:
E1=−∫∞0(βλxβ−1e−λxβ)ln(βλxβ−1e−λxβ)dx=−ln(βλ)+(β−1)β[γ+ln(λ)]+1 | (2.2) |
where γ is the Euler constant.
ζ-entropy is a parametric extension of Shannon entropy. It was introduced by the physicist Tsallis [28], and this type has several applications in physics, statistical mechanics econophysics, and finance. For a random variable X with a PDF f(x), for ζ>0, ζ≠1, the ζ-entropy (E2) measure is defined as follows:
E2=1ζ−1(1−∫∞−∞f(x)ζdx). |
The expression of ζ-entropy of the Weibull distribution can be calculated from Eq (2) as follows:
E2=1ζ−1(1−∫∞0(βλxβ−1e−λxβ)ζdx), |
where the value of the integral is given by:
I=∫∞0(βλxβ−1e−λxβ)ζdx=(βλ)ζ∫∞0(xβ−1e−λxβ)ζdx. |
Let u=xβ, x=u1β, and dx=1βu1β−1du, and then
I=(βλ)ζ∫∞0(uβ−1βe−λu)ζ(1βu1β−1). |
After simplification, I is as follows:
I=(βλ−1β)ζ−1Γ(ζ(1−1β)+1β)ζζ(1−1β)+1β, |
where Γ(.) is the gamma function. Then the value of ζ-entropy is given by:
E2=1ζ−1(1−(βλ−1β)ζ−1Γ(ζ(1−1β)+1β)ζζ(1−1β)+1β). | (2.3) |
Arimoto entropy is a generalized form of the well-known Shannon entropy and has several applications in clustering, image processing, and data analysis. The characteristics of Arimoto's (E3) entropy [29] measure is given by:
E3=ζ1−ζ[(∫∞−∞f(x)ζdx)1ζ−1]. |
The value of Arimoto's entropy is
E3=ζ1−ζ[((βλ−1β)ζ−1Γ(ζ(1−1β)+1β)ζζ(1−1β)+1β)1ζ−1]. | (2.4) |
Havrda and Charvat (HC) entropy [30] represents an extension of Shannon entropy. This particular extension is denoted as E4 entropy of degree ζ, ζ≠1, and is characterized by the following properties:
E4=121−ζ−1[∫∞−∞f(x)ζdx−1]. |
In the same way as Arimoto entropy, the value of E4 entropy is calculated as follows:
E4=121−ζ−1[((βλ−1β)ζ−1Γ(ζ(1−1β)+1β)ζζ(1−1β)+1β)ζ−1]. | (2.5) |
In this section, we examine the entropies measures of the Weibull distribution using the ML and Bayesian methods. When using the Bayesian method, we acquire the entropy measure estimators for SLOF and ASLOF, and compute these estimators using the Metropolis-Hastings (M-H) algorithm.
The ML estimators for the Weibull distribution are obtained based on the GT-Ⅱ HCS. The GT-Ⅱ HCS is explained as follows:
In the GT-Ⅱ HCS, one sets r∈(1,2,...,n) and time T1,T2∈(0,∞), where T1<T2. If the rth failure occurs before T1, then the termination time is T∗=T1, if the rth failure occurs between T1 and T2, then the termination time is T∗=xr:n, and if the rth failure occurs after T2, the termination time is T∗=T2. Therefore under the GT-Ⅱ HCS, there are three forms of data:
Case1:x1:n<...<xd1:nifxr:n<T1;Case2:x1:n<...<xd1:n,...<xr:nifT1<xr:n<T2;Case3:x1:n<...<xd2:n,..<T2ifxr:n≥T2. |
Suppose in a life-testing study, there are n identical items, and let x1:n,x2:n,...,xn:n represent the ordered failure times of these items, T1,T2∈(0,∞). Then the likelihood function of β and λ is as follows:
L(x_|β;λ)=n!(n−D)![D∏i=1f(xi:n)][1−F(C)]n−D, | (3.1) |
where D is the number of total failures in the experiment up to time C and its value is given by:
(D;C)={(d1,T1)for Case 1(r,xr:n)for Case 2(d2,T2)for Case 3, |
where di denotes the number of failures that occurred until time Ti. Then inserting (1.1) and (1.2) in (3.1) gives:
L(x_|β;λ)=n!(n−D)![D∏i=1βλxβ−1ie−λxβi][e−λCβ]n−D. | (3.2) |
For a simplified form, replace xi:n with xi in Equation (3.2). By taking the logarithm of each side, indicated by l, we have
l∝Dln(β)+Dln(λ)+(β−1)D∑i=1ln(xi)−λD∑i=1xβi−(n−D)λCβ. | (3.3) |
The derivatives of (3.3), owing to β and λ, allow us to obtain
∂l∂β=Dβ+D∑i=1ln(xi)−λD∑i=1xβiln(xi)−(n−D)λCβln(C), | (3.4) |
and
∂l∂λ=Dλ−D∑i=1xβi−(n−D)Cβ. | (3.5) |
To obtain the ML estimators of the two parameters, set (3.4) and (3.5) to zero and solve the resulting system of equations. Equating (3.5) with zero, we have
Dˆλ−D∑i=1xˆβi−(n−D)Cˆβ=0, |
and this can be written as
ˆλ=D∑Di=1xˆβi+(n−D)Cˆβ=A(ˆβ). | (3.6) |
Substituting from (3.6) into (3.4) and setting it to zero, we have
Dˆβ+D∑i=1ln(xi)−A(ˆβ)D∑i=1xˆβiln(xi)−A(ˆβ)(n−D)Cˆβln(C)=0. | (3.7) |
The ML estimator of β may be obtained iteratively by calculating the ML estimator from (3.7) and then substituting it into (3.6) to compute the ML estimator of λ. Hence, based on the invariance property, the ML estimator of E1,E2,E3, and E4 are produced by inserting ˆβ and ˆλ in Eqs (2.2)–(2.5), respectively, as follows:
^E1=−ln(ˆβˆλ)+(ˆβ−1)ˆβ[γ+ln(ˆλ)]+1, | (3.8) |
^E2=1ζ−1(1−(ˆβˆλ−1ˆβ)ζ−1Γ(ζ(1−1ˆβ)+1ˆβ)ζζ(1−1ˆβ)+1ˆβ), | (3.9) |
^E3=ζ1−ζ[((ˆβˆλ−1ˆβ)ζ−1Γ(ζ(1−1ˆβ)+1ˆβ)ζζ(1−1ˆβ)+1ˆβ)1ζ−1], | (3.10) |
and
^E4=121−ζ−1[((ˆβˆλ−1ˆβ)ζ−1Γ(ζ(1−1ˆβ)+1ˆβ)ζζ(1−1ˆβ)+1ˆβ)ζ−1]. | (3.11) |
To compute the ACIs, the asymptotic variance-covariance matrix (AV-CM) of ˆβ and ˆλ can be obtained by inverting the Fisher information matrix (FM) defined as the negative expected value of the second derivative of the log-likelihood function.
ˆI(ˆβ,ˆλ)=−E[∂2l∂β2∂2l∂β∂λ∂2l∂λ∂β∂2l∂λ2](ˆβ,ˆλ). |
It is difficult to find exact closed-form solutions for the given requirements. Therefore, the observed Fisher information matrix ˆI(ˆβ,ˆλ), obtained by removing the expectation operator E, will be used to construct ACIs for the parameters, see [31]. The second partial derivative of the log-likelihood function from the entries of the observed matrix is represented by
ˆI(ˆβ,ˆλ)=−[∂2l∂β2∂2l∂β∂λ∂2l∂λ∂β∂2l∂λ2](ˆβ,ˆλ). |
The elements of the FM are obtained as follows:
∂2l∂β2=−Dβ2−(n−D)λCβln(C)2−λD∑i=1xβi(ln(xi))2, |
∂2l∂λ2=−Dλ2, |
∂2l∂λ∂β=−D∑i=1xβiln(xi)−(n−D)λCβln(C). |
To construct the AV-CM for the ML estimators, the observed FM is inverted as follows:
[ˆV]=ˆI−1(ˆβ,ˆλ)=[−∂2l∂β2−∂2l∂β∂λ−∂2l∂λ∂β−∂2l∂λ2]−1(ˆβ,ˆλ)=[−var(ˆβ)−cov(ˆβ,ˆλ)−cov(ˆβ,ˆλ)−var(ˆλ)]. |
The two-sided 100(1−ω)% ACI for β and λ can be constructed based on the asymptotic normality conditions of the ML estimators as:
(ˆβ±Zω2√var(ˆβ))(ˆλ±Zω2√var(ˆλ)), |
where Zω2 is an upper ω2 % of the standard normal distribution.
Additionally, we must ascertain the variations of the entropy measures to derive the ACI. We employ the delta method described in [32] to obtain a rough estimate of the entropy measures. This method is a statistical technique used to approximate the distribution of a nonlinear function of random variables using derivatives. This method is based on the principle that a nonlinear function can be approximated using its first derivative, allowing for the estimation of the variance of complex statistics.
This methodology allows us to approximate the variance of E1,E2,E3, and E4 as follows:
var(^E1)=[∇1^E1]T[ˆV][∇1^E1],var(^E2)=[∇2^E2]T[ˆV][∇2^E2], |
var(^E3)=[∇3^E3]T[ˆV][∇3^E3],var(^E4)=[∇4^E4]T[ˆV][∇4^E4], |
where ∇i^Ei=(∂Ei∂β,∂Ei∂λ).
∂E1∂β=−1β+1β2[γ+ln(λ)],∂E1∂λ=−1βλ, |
∂E2∂λ=λ1β−1(βλ−1β)ζ−2Γ(ζ(1−1β)+1β)ζζ(1−1β)+1β, |
∂E2∂β=ζζ(1−β)−1βΓ(1+(−1+β)ζβ)(β−log(ζ)−log(λ)+ψ(1+(−1+β)ζβ))β3−ζλ1−ζβ, |
∂E3dλ=λ1β−1ζ(ζ−11−ζ(βλ−1β)ζ−2Γ(ζ(1−1β)+1β)1ζζζ(1−1β)+1β, |
∂E3dβ=ζ−1+ζ−βζβζΓ(1+(−1+β)ζβ)1ζ(−βζ+ζlog(λ)+log(ζ)−ψ(1+(−1+β)ζβ))β3−ζλ1−ζβ, |
∂E4dλ=λ1β−1ζ−121−ζ−1(βλ−1β)ζ−2Γ(ζ(1−1β)+1β)ζζζ(1−1β)+1β, |
∂E4∂β=2ζ(−1+ζ)ζ−ζ+ζ2−βζ2βΓ(1+(−1+β)ζβ)ζ(−β+log(λ)+ζlog(ζ)−ζψ(1+(−1+β)ζβ))(−2+2ζ)β3−ζλ1−ζβ, |
where ψ(z)=Γ′(z)Γ(z) is the digamma function.
The delta method is employed because it effectively approximates the distribution, simplifying the variance computation for the entropy measures. It also facilitates estimating uncertainty and constructing confidence intervals, especially when using ML estimates. Thus, the two-sided 100(1−ω) ACI for E1,E2,E3, and E4 can be constructed as follows:
(^Ei±Zω2√var(^Ei)),i=1,2,3,4. |
Since both β and λ are unknown and lack a natural conjugate bivariate prior distribution, independent gamma distributions are assumed for each. Specifically, β is assigned a gamma distribution with parameters (a1,b1) and λ with parameters (a2,b2). The means of these distributions are given by a1b1 for β and a2b2 for λ.
The joint prior distribution is as follows:
π(β,λ)=1Γ(β)Γ(λ)βa1−1λa2−1e−(b1β+b2λ), | (3.12) |
where a1,b1,a2, and b2 are positive hyperparameters that represent prior knowledge.
The posterior distribution is given by
π∗(β,λ|x_)=M1βD+a1−1λD+a2−1e−(b1β+b2λ)[D∏i=1xβ−1ie−λxβi][e−(n−D)λCβ], | (3.13) |
which can be written as:
π∗(β,λ|x_)=M1βD+a1−1λD+a2−1e−b1βe−b2λ×[e(β−1)∑Di=1ln(xi)−λ∑Di=1xβi)][e−(n−D)λCβ], | (3.14) |
and further simplified as:
π∗(β,λ|x_)=M1βD+a1−1λD+a2−1e−∑Di=1ln(xi)e−β(b1−∑Di=1ln(xi))e−λ[b2+∑Di=1xβi+(n−D)Cβ], | (3.15) |
where
M−11=∫∞0∫∞0L(x_|β,λ)π(β,λ)dβdλ |
is the normalizing constant.
The marginal posterior distributions of β and λ are given by:
(1) Marginal posterior distribution of β:
π∗1(β|x_)∝βD+a1−1e−β(b1−∑Di=1ln(xi))×∫∞0λD+a2−1e−λ[b2+∑Di=1xβi+(n−D)Cβ]dλ, | (3.16) |
(2) Marginal posterior distribution of λ:
π∗2(λ|x_)∝λD+a2−1e−λb2×∫∞0βD+a1−1e−λ[∑Di=1xβi+(n−D)Cβ]e−β(b1−∑Di=1ln(xi))dβ. | (3.17) |
From the expressions in (3.15)–(3.17), the conditional posterior distribution of λ given β is:
π∗1(λ|β,x_)∝λD+a2−1e−λ[b2+∑Di=1xβi+(n−D)Cβ]. | (3.18) |
As a result, the gamma distribution with shape parameter (D+a2−1) and scale parameter (b2+∑Di=1xβi+(n−D)Cβ) is the posterior density function of π∗1(λ|β,x_). So, any gamma-producing technique can be used to generate λ samples with ease. One cannot sample directly from π∗2(β|λ,x_) as it cannot be analytically reduced to well-known distributions. The MCMC method-based M-H algorithm is employed to get an estimate.
One of the accuracy metrics used in the Bayesian estimating process is the loss function, which is defined as the amount of loss incurred while making a Bayesian judgment for an unknown parameter. It is a measurement of the discrepancy between this parameter's estimated and actual values. Generally speaking, loss functions may be divided into two primary categories based on symmetry criteria: First, there are SLOFs, which assume that the loss incurred in a positive direction is equal to the loss incurred in a negative direction. The second class of loss functions is known as ASLOFs; in this class, it is assumed that the amount of loss under the Bayes decision in both the positive and negative directions need not be equal.
This sub-section examines the Bayesian estimators of different entropy measures for both SLOFs and ASLOFs. The squared error (SE) LOF is one of the most extensively utilized SLOFs. This kind is appropriate for reducing the mean squared error since it penalizes greater mistakes more severely than smaller ones. The SE LOF is provided as below:
L1(ϕ,δ)=(δ−ϕ)2, |
where δ is an estimator of ϕ. In this situation, the Bayesian estimator is calculated as follows:
ˆϕSE=E(ϕ|data). | (3.19) |
In the context of ASLOFs, the linear-exponential (LINEX) LOF exhibits less sensitivity to outliers than the SE LOF, striking a compromise between bias and variance. The LINEX LOF is defined as follows:
L2(ϕ,δ)=e−q(δ−ϕ)−q(δ−ϕ)−1, |
where q represents the sign that indicates the direction of asymmetry. Under the LINEX LOF, the Bayesian estimator is provided by
ˆϕLINEX=−1qln[E(e−qϕ|data)]. | (3.20) |
Another ASLOF is the general entropy (GE) LOF which provides a measure of dissimilarity between probability distributions and is used to focus on maximizing the similarity between predicted and actual distributions rather than minimizing prediction errors. The GE LOF has the following formula:
L3(ϕ,δ)=(δϕ)q−qlog(δϕ)−1. |
The Bayesian estimator via GE LOF is:
ˆϕGE=[E(ϕ−q|data)]−1q. | (3.21) |
Now the entropy Bayesian estimators via SE, LINEX, and GE LOFs, are as follows:
ˆJSE=M1∫∞0∫∞0JβD+a1−1λD+a2−1e−b1β×e−b2λ+Ki(β,λ,xi)[e−(n−D)λcβ]dβdλ, | (3.22) |
ˆJLINEX=−1qln[M1∫∞0∫∞0e−qJβD+a1−1λD+a2−1e−b1β×e−b2λ+Ki(β,λ,xi)(e−(n−D)λcβ)dβdλ], | (3.23) |
ˆJGE=[M1∫∞0∫∞0(J)−qβD+a1−1λD+a2−1e−b1β×e−b2λ+Ki(β,λ,xi)(e−(n−D)λcβ)dβdλ]−1q, | (3.24) |
where
Ki(β,λ,xi)=((β−1)D∑i=1ln(xi)−λD∑i=1xβi), |
M1 is the normalizing constant, and to calculate the different entropy measures, we put J=E1,E2,E3, and E4. It is important to note that all Bayesian entropy estimators are formulated as a ratio of two integrals. These integrals cannot be simplified or calculated directly. Therefore, to compute these estimators and to construct their HPD intervals, the MCMC method is employed.
The behavior of the ML estimates (MLEs) and Bayesian estimates (BEs) for the different measures of entropy for the Weibull distribution was investigated numerically using various LOFs. Bayesian estimators were calculated using the M-H algorithm under the SE, LINEX, and GE LOFs. Samples were created from the posterior distributions using the MCMC method. The M-H algorithm proceeds as follows:
(1) Put β0=ˆβ.
(2) Let l=1.
(3) λ(l) is obtained from gamma π∗1(λ|βl−1,x_).
(4) Generate β(l) from π∗2(β|λl,x_) using the same procedure of Metropolis-Hastings [33] and use the normal distribution as a proposal distribution.
(5) Put l=l+1.
(6) Calculate β(t) and λ(t).
(7) Repeat Steps 3−6 N times.
(8) Acquire the BEs of β and λ and obtain the entropy measure concerning the LOFs.
To compute the BEs, we implemented the MCMC algorithm with a dataset of N=10000 observations. Initially, we used the MLEs for the unknown parameters λ and β as starting values for the MCMC algorithm. However, it is important to note that the initial values may differ from the final converged values. Therefore, we discarded the first M=1000 values to account for this discrepancy. To verify the convergence of the MCMC samples and determine the burn-in period, we conducted diagnostic tests, examined trace plots, and assessed posterior density plots for various parameters and censoring schemes. These analyses helped us identify the burn-in period and ensure the convergence of the MCMC algorithm before analyzing the data further.
The method proposed by Chen and Shao [34] is employed to construct the 100(1−ω)% HPD credible intervals for entropy measures.
From Figures 1 and 2, the estimation demonstrates that all of the generated posteriors closely match the theoretical posterior density functions, and it is evident that a big MCMC loop yields results that are comparable and more effective than smaller loops. These plots have no significant lengthy upward or downward trends, which are convergence markers.
This section is dedicated to evaluating the performance of all previously suggested estimators for entropy measures. To achieve this, a simulation study is conducted for estimation purposes. Additionally, an analysis of actual data is provided to further support the study.
The MCMC simulations were performed to compare the estimates using Mathematica 12. Using the following procedure, the simulation research is carried out.
(1) A random sample of sizes n=150 and 250, with true parameter values
λ=2.5,β=1.5, |
and entropy values
E1=0.267853,E2=0.183147,E3=0.27472,andE4=0.312651, |
was generated from the Weibull distribution using the quantile function. The MLE of β was obtained using an iterative technique based on Eq (3.7). Subsequently, the MLE of λ was derived by substituting the estimated ˆβ into Eq (3.6).
(2)Using the invariance property, the MLEs for the entropy values E1, E2, E3, and E4 were calculated by inserting ˆβ and ˆλ into Eqs (3.8)–(3.11), respectively. After obtaining these estimates, the 95% ACIs, ALs, and CPs were computed.
(3) The BEs were then calculated using the proposed LOF through the M-H algorithm, as described in subsection (3.4). The values of q were assumed to be (−4,4). For the different measures of entropy, the 95% HPD intervals, ALs, and CPs were calculated at ζ=1.5 and 0.5.
(4)To enhance the stability of the model and simplify its complexity, fixed values for the hyperparameters were chosen as
a1=0.6,b1=1.2,a2=2,andb2=0.4, |
based on prior evidence supporting these values. The parameters n, r, T1, and T2 were selected according to Table 1, and steps (1–4) were repeated 1000 times. The MSEs of the various entropy estimates were then computed. The outcomes of the simulation study are recorded in Tables 2–9.
n | r | T1 | T2 |
250 | 200 | (2, 5) | 7 |
150 | 120 | (2, 5) | 7 |
250 | (200,170) | 0.2 | 1.2 |
150 | (120, 80) | 0.2 | 1.2 |
250 | 200 | 1.5 | (3, 7) |
150 | 120 | 1.5 | (3, 7) |
Entropy | n | r | MLE | SE | LINEX | GE | ||
q=(−4) | q=(4) | q=(−4) | q=(4) | |||||
E1 | 150 | 120 | 1.36712 | 1.51529 | 1.57698 | 1.4603 | 1.54341 | 1.46821 |
0.60911 | 0.61993 | 0.78749 | 0.47828 | 0.69251 | 0.5022 | |||
E2 | 0.19348 | 0.14388 | 0.15158 | 0.13615 | 0.17786 | 0.01169 | ||
0.0026 | 0.00428 | 0.00363 | 0.00507 | 0.00157 | 0.03013 | |||
E3 | 0.29022 | 0.21582 | 0.23314 | 0.19842 | 0.26679 | 0.01753 | ||
0.0059 | 0.00964 | 0.00754 | 0.01239 | 0.00353 | 0.0678 | |||
E4 | 0.33029 | 0.24562 | 0.26804 | 0.22307 | 0.30362 | 0.01995 | ||
0.0077 | 0.01249 | 0.00946 | 0.0166 | 0.00457 | 0.08781 | |||
E1 | 150 | 80 | 1.45558 | 1.59925 | 1.70715 | 1.51007 | 1.64329 | 1.52503 |
.43429 | 0.88746 | 1.21708 | 0.63845 | 1.01106 | 0.68956 | |||
E2 | 0.14164 | 0.12597 | 0.13823 | 0.1136 | 0.18205 | 0.00373 | ||
0.00183 | 0.00796 | 0.00644 | 0.00983 | 0.00175 | 0.03244 | |||
E3 | 0.21247 | 0.18895 | 0.2165 | 0.16107 | 0.27307 | 0.00559 | ||
0.0041 | 0.01791 | 0.01305 | 0.02451 | 0.00394 | 0.07299 | |||
E4 | 0.2418 | 0.21504 | 0.2507 | 0.17889 | 0.31078 | 0.00636 | ||
0.00532 | 0.0232 | 0.0162 | 0.03311 | 0.0051 | 0.09454 | |||
E1 | 250 | 200 | 1.41556 | 1.47743 | 1.51066 | 1.44628 | 1.49357 | 1.45048 |
0.42562 | 0.49233 | 0.57613 | 0.41596 | 0.53207 | 0.42718 | |||
E2 | 0.18265 | 0.16257 | 0.1671 | 0.15803 | 0.1816 | 0.05219 | ||
0.0188 | 0.0166 | 0.0147 | 0.019 | 0.0093 | 0.02149 | |||
E3 | 0.27397 | 0.24386 | 0.25404 | 0.23364 | 0.2724 | 0.07828 | ||
0.0423 | 0.0374 | 0.0312 | 0.0457 | 0.021 | 0.04836 | |||
E4 | 0.3118 | 0.27753 | 0.29072 | 0.26429 | 0.31001 | 0.08909 | ||
0.00547 | 0.0484 | 0.0395 | 0.0609 | 0.0272 | 0.06263 | |||
E1 | 250 | 170 | 1.43521 | 1.02243 | 1.03456 | 1.01037 | 1.03126 | 1.00721 |
0.7071 | 0.57443 | 0.59289 | 0.55637 | 0.58775 | 0.55186 | |||
E2 | 0.17722 | 0.46139 | 0.46597 | 0.45683 | 0.46873 | 0.44847 | ||
0.00349 | 0.0779 | 0.08047 | 0.07539 | 0.08202 | 0.07092 | |||
E3 | 0.26582 | 0.69208 | 0.70242 | 0.68185 | 0.7031 | 0.67271 | ||
0.00784 | 0.17527 | 0.184 | 0.16684 | 0.18454 | 0.15957 | |||
E4 | 0.30253 | 0.78764 | 0.80104 | 0.77439 | 0.80017 | 0.76559 | ||
0.01016 | 0.22701 | 0.23991 | 0.21462 | 0.23902 | 0.20668 |
Entropy | n | T1 | MLE | SE | LINEX | GE | ||
q=(−4) | q=(4) | q=(−4) | q=(4) | |||||
E1 | 150 | 2 | 1.42207 | 1.10074 | 1.12712 | 1.07561 | 1.11805 | 1.07101 |
0.74852 | 0.71389 | 0.75988 | 0.67162 | 0.74317 | 0.66525 | |||
E2 | 0.18175 | 0.33537 | 0.3417 | 0.32909 | 0.34903 | 0.304 | ||
0.00373 | 0.02404 | 0.02599 | 0.02218 | 0.02829 | 0.01683 | |||
E3 | 0.27262 | 0.50306 | 0.51733 | 0.48895 | 0.52355, | 0.45599 | ||
0.00838 | 0.0541 | 0.06077 | 0.0479 | 0.06366 | 0.03786 | |||
E4 | 0.31026 | 0.57252 | 0.59102 | 0.55425 | 0.59583 | 0.51895 | ||
0.01086 | 0.07006 | 0.07995 | 0.06098 | 0.08245 | 0.04904 | |||
E1 | 150 | 5 | 1.4257 | 1.09124 | 1.11732 | 1.06638 | 1.10857 | 1.06145 |
0.35491 | 0.696 | 0.74062 | 0.65491 | 0.72485 | 0.64805 | |||
E2 | 0.1727 | 0.33467 | 0.34102 | 0.32838 | 0.34835 | 0.30641 | ||
0.00347 | 0.02359 | 0.02554 | 0.02173 | 0.02785 | 0.01618 | |||
E3 | 0.25905 | 0.50201 | 0.51631 | 0.48787 | 0.52252 | 0.45961 | ||
0.0078 | 0.05307 | 0.05974 | 0.04687 | 0.06266 | 0.0364 | |||
E4 | 0.29482 | 0.57132 | 0.58986 | 0.55302 | 0.59467 | 0.52307 | ||
0.01011 | 0.06874 | 0.07862 | 0.05965 | 0.08116 | 0.04714 | |||
E1 | 250 | 2 | 1.42515 | 1.08207 | 1.09661 | 1.06792 | 1.09196 | 1.06535 |
0.5497 | 0.67419 | 0.69842 | 0.65104 | 0.6904 | 0.64724 | |||
E2 | 0.1791 | 0.35346 | 0.35724 | 0.34969 | 0.36132 | 0.33912 | ||
0.00256 | 0.02935 | 0.03065 | 0.02808 | 0.03207 | 0.02472 | |||
E3 | 0.26866 | 0.53018 | 0.53871 | 0.52171 | 0.54198 | 0.50868 | ||
0.00575 | 0.06603 | 0.07045 | 0.06178 | 0.07215 | 0.05562 | |||
E4 | 0.30575 | 0.60339 | 0.61444 | 0.59241 | 0.61682 | 0.57892 | ||
0.00745 | 0.08552 | 0.09206 | 0.07928 | 0.09345 | 0.07204 | |||
E1 | 250 | 5 | 1.43201 | 1.074 | 1.08855 | 1.05981 | 1.08398 | 1.0570 |
0.36655 | 0.66264 | 0.68669 | 0.63962 | 0.67882 | 0.63567 | |||
E2 | 0.16956 | 0.35109 | 0.35487 | 0.34733 | 0.35901 | 0.33668 | ||
0.00258 | 0.02859 | 0.02987 | 0.02734 | 0.03129 | 0.02401 | |||
E3 | 0.25435 | 0.52664 | 0.53516 | 0.51817 | 0.53851 | 0.50502 | ||
0.00581 | 0.06432 | 0.06868 | 0.06014 | 0.07039 | 0.05403 | |||
E4 | 0.28946 | 0.59935 | 0.6104 | 0.58839 | 0.61286 | 0.57474 | ||
0.00752 | 0.08331 | 0.08975 | 0.07717 | 0.09117 | 0.06998 |
Entropy | n | T2 | MLE | SE | LINEX | GE | ||
q=(−4) | q=(4) | q=(−4) | q=(4) | |||||
E1 | 150 | 3 | 1.44174 | 1.10164 | 1.12749 | 1.07697 | 1.11865 | 1.07244 |
0.69463 | 0.71086 | 0.7555 | 0.66966 | 0.73956 | 0.66315 | |||
E2 | 0.17451 | 0.34042 | 0.34679 | 0.33409 | 0.35394 | 0.31117 | ||
0.00411 | 0.0254 | 0.02744 | 0.02346 | 0.02978 | 0.01788 | |||
E3 | 0.26176 | 0.51063 | 0.525 | 0.49642 | 0.53092 | 0.46676 | ||
0.00925 | 0.05716 | 0.06412 | 0.05069 | 0.067 | 0.04023 | |||
E4 | 0.2979 | 0.58113 | 0.59976 | 0.56274 | 0.60422 | 0.5312 | ||
0.01198 | 0.07404 | 0.08435 | 0.06454 | 0.08678 | 0.05211 | |||
E1 | 150 | 7 | 1.45241 | 1.09892 | 1.12479 | 1.07423 | 1.116 | 1.06958 |
0.41651 | 0.70499 | 0.74941 | 0.664 | 0.73368 | 0.65728 | |||
E2 | 0.16415 | 0.3389 | 0.34527 | 0.33257 | 0.35247 | 0.31123 | ||
0.0043 | 0.02485 | 0.02687 | 0.02293 | 0.02921 | 0.01722 | |||
E3 | 0.24623 | 0.50835 | 0.52272 | 0.49412 | 0.5287 | 0.46684 | ||
0.00967 | 0.05592 | 0.06282 | 0.0495 | 0.06572 | 0.03875 | |||
E4 | 0.28022 | 0.57853 | 0.59716 | 0.56012 | 0.6017 | 0.5313 | ||
0.01253 | 0.07243 | 0.08265 | 0.0630 | 0.08512 | 0.05019 | |||
E1 | 250 | 3 | 1.43076 | 1.0857 | 1.10019 | 1.07159 | 1.09552 | 1.06909 |
0.36052 | 0.67711 | 0.70126 | 0.654 | 0.69327 | 0.65021 | |||
E2 | 0.1783 | 0.35307 | 0.35684 | 0.34933 | 0.36091 | 0.33882 | ||
0.00213 | 0.02927 | 0.03056 | 0.02801 | 0.03197 | 0.02468 | |||
E3 | 0.26745 | 0.52961 | 0.5381 | 0.52119 | 0.54137 | 0.50823 | ||
0.00479 | 0.06585 | 0.07024 | 0.06164 | 0.07194 | 0.05553 | |||
E4 | 0.30438 | 0.60274 | 0.61373 | 0.59183 | 0.61612 | 0.5784 | ||
0.00621 | 0.0853 | 0.09178 | 0.0791 | 0.09317 | 0.07192 | |||
E1 | 250 | 7 | 1.43755 | 1.09109 | 1.10581 | 1.07676 | 1.10102 | 1.07429 |
0.35688 | 0.68555 | 0.71023 | 0.66196 | 0.702 | 0.6582 | |||
E2 | 0.17673 | 0.35121 | 0.35498 | 0.34746 | 0.35909 | 0.33684 | ||
0.00222 | 0.02865 | 0.02993 | 0.02741 | 0.03134 | 0.02408 | |||
E3 | 0.2651 | 0.52681 | 0.53531 | 0.51838 | 0.53864 | 0.50526 | ||
0.00499 | 0.06447 | 0.06882 | 0.0603 | 0.07052 | 0.05418 | |||
E4 | 0.3017 | 0.59955 | 0.61056 | 0.58863 | 0.61301 | 0.57502 | ||
0.00646 | 0.0835 | 0.08993 | 0.07737 | 0.09134 | 0.07018 |
Entropy | n | r | MLE | SE | LINEX | GE | ||
q=(−4) | q=(4) | q=(−4) | q=(4) | |||||
E1 | 150 | 80 | 1.44582 | 1.603 | 1.73021 | 1.50082 | 1.65364 | 1.51755 |
0.40585 | 0.92552 | 1.32941 | 0.63469 | 1.06945 | 0.69625 | |||
E2 | 0.44828 | 0.39371 | 0.41826 | 0.37158 | 0.43654 | 0.25012 | ||
0.00698 | 0.01149 | 0.00913 | 0.01474 | 0.00655 | 0.06768 | |||
E3 | 0.22414 | 0.19686 | 0.20282 | 0.19119 | 0.21827 | 0.12506 | ||
0.00174 | 0.00287 | 0.00255 | 0.00326 | 0.00164 | 0.01692 | |||
E4 | 0.54112 | 0.47525 | 0.51149 | 0.4433 | 0.52695 | 0.30192 | ||
0.01017 | 0.01675 | 0.0128 | 0.02258 | 0.00955 | 0.09861 | |||
E1 | 150 | 120 | 1.43487 | 1.5157 | 1.57724 | 1.46066 | 1.54378 | 1.46853 |
0.37627 | 0.61997 | 0.78671 | 0.47843 | 0.69239 | 0.50217 | |||
E2 | 0.44775 | 0.41609 | 0.42997 | 0.40302 | 0.43997 | 0.36094 | ||
0.00522 | 0.00589 | 0.00495 | 0.00714 | 0.00406 | 0.01896 | |||
E3 | 0.22388 | 0.20805 | 0.21146 | 0.20473 | 0.21998 | 0.18047 | ||
0.00131 | 0.00147 | 0.00134 | 0.00162 | 0.00101 | 0.00474 | |||
E4 | 0.54049 | 0.50227 | 0.52262 | 0.48332 | 0.53109 | 0.43569 | ||
0.00761 | 0.00859 | 0.00699 | 0.01083 | 0.00591 | 0.02762 | |||
E1 | 250 | 170 | 1.44429 | 1.01809 | 1.03028 | 1.00595 | 1.027 | 1.0027 |
0.39576 | 0.5683 | 0.58679 | 0.5502 | 0.58168 | 0.5456 | |||
E2 | 0.43933 | 0.99617 | 1.02445 | 0.97078 | 1.0163 | 0.96342 | ||
0.00699 | 0.29079 | 0.32224 | 0.26396 | 0.31289 | 0.2566 | |||
E3 | 0.21966 | 0.49809 | 0.50495 | 0.49158 | 0.50815 | 0.48171 | ||
0.00175 | 0.0727 | 0.07646 | 0.06922 | 0.07822 | 0.06415 | |||
E4 | 0.53032 | 1.20248 | 1.24422 | 1.16586 | 1.22679 | 1.16296 | ||
0.01019 | 0.42372 | 0.48007 | 0.37722 | 0.45592 | 0.3739 | |||
E1 | 250 | 200 | 1.42666 | 1.07694 | 1.09132 | 1.06292 | 1.08678 | 1.06027 |
0.5947 | 0.46296 | 0.48666 | 0.44027 | 0.47897 | 0.43631 | |||
E2 | 0.4528 | 0.75476 | 0.76948 | 0.74095 | 0.76889 | 0.73139 | ||
0.00397 | 0.08851 | 0.09748 | 0.08049 | 0.09706 | 0.07525 | |||
E3 | 0.2264 | 0.37738 | 0.381 | 0.37387 | 0.38445 | 0.3657 | ||
0.00099 | 0.02213 | 0.02322 | 0.0211 | 0.02427 | 0.01881 | |||
E4 | 0.54658 | 0.91107 | 0.93268 | 0.89108 | 0.92814 | 0.88287 | ||
0.00578 | 0.12897 | 0.14495 | 0.11502 | 0.14143 | 0.10965 |
Entropy | n | T1 | MLE | SE | LINEX | GE | ||
q=(−4) | q=(4) | q=(−4) | q=(4) | |||||
E1 | 150 | 2 | 1.43621 | 1.08769 | 1.11309 | 1.0634 | 1.10461 | 1.05858 |
0.38391 | 0.68731 | 0.73053 | 0.64734 | 0.71542 | 0.64047 | |||
E2 | 0.44669 | 0.74201 | 0.76687 | 0.71968 | 0.76569 | 0.70293 | ||
0.00752 | 0.08279 | 0.09771 | 0.07048 | 0.09677 | 0.06223 | |||
E3 | 0.22335 | 0.37101 | 0.37704 | 0.36528 | 0.38284 | 0.35146 | ||
0.00188 | 0.0207 | 0.02247 | 0.01909 | 0.02419 | 0.01556 | |||
E4 | 0.5392 | 0.89569 | 0.93237 | 0.86347 | 0.92427 | 0.84851 | ||
0.01096 | 0.12064 | 0.14747 | 0.09936 | 0.141 | 0.09068 | |||
E1 | 150 | 5 | 1.45913 | 1.11113 | 1.13795 | 1.08562 | 1.12859 | 1.08114 |
0.33642 | 0.63146 | 0.67869 | 0.58809 | 0.66129 | 0.58194 | |||
E2 | 0.44216 | 0.7227 | 0.74673 | 0.70098 | 0.74627 | 0.68352 | ||
0.00626 | 0.07177 | 0.08517 | 0.06067 | 0.08473 | 0.05273 | |||
E3 | 0.22108 | 0.36134 | 0.36719 | 0.35579 | 0.37313 | 0.34175 | ||
0.00157 | 0.01794 | 0.01953 | 0.01649 | 0.02118 | 0.01318 | |||
E4 | 0.53374 | 0.87237 | 0.90781 | 0.84102 | 0.90082 | 0.82508 | ||
0.00913 | 0.10457 | 0.12865 | 0.08539 | 0.12345 | 0.07684 | |||
E1 | 250 | 2 | 1.42519 | 1.08909 | 1.10364 | 1.07491 | 1.09892 | 1.07243 |
0.44944 | 0.68529 | 0.70973 | 0.66191 | 0.70155 | 0.65823 | |||
E2 | 0.46296 | 0.75583 | 0.77058 | 0.74198 | 0.76997 | 0.73241 | ||
0.00409 | 0.08983 | 0.0989 | 0.08172 | 0.09843 | 0.07648 | |||
E3 | 0.23148 | 0.37791 | 0.38154 | 0.3744 | 0.38498 | 0.3662 | ||
0.00102 | 0.02246 | 0.02356 | 0.02142 | 0.02461 | 0.01912 | |||
E4 | 0.55884 | 0.91237 | 0.93402 | 0.89231 | 0.92943 | 0.88409 | ||
0.00996 | 0.13089 | 0.14704 | 0.11679 | 0.14342 | 0.11143 | |||
E1 | 250 | 5 | 1.43915 | 1.08467 | 1.09935 | 1.07035 | 1.09464 | 1.06776 |
0.3813 | 0.67743 | 0.70194 | 0.65398 | 0.69382 | 0.65014 | |||
E2 | 0.44599 | 0.74805 | 0.76262 | 0.73437 | 0.76216 | 0.72469 | ||
0.0048 | 0.08519 | 0.09392 | 0.0774 | 0.09356 | 0.07223 | |||
E3 | 0.22299 | 0.37402 | 0.37761 | 0.37055 | 0.38108 | 0.36234 | ||
0.0012 | 0.0213 | 0.02236 | 0.0203 | 0.02339 | 0.01806 | |||
E4 | 0.53835 | 0.90297 | 0.92436 | 0.88316 | 0.92001 | 0.87478 | ||
0.007 | 0.12414 | 0.13968 | 0.11058 | 0.13633 | 0.10525 |
Entropy | n | T2 | MLE | SE | LINEX | GE | ||
q=(−4) | q=(4) | q=(−4) | q=(4) | |||||
E1 | 150 | 3 | 1.4405 | 1.10344 | 1.12943 | 1.07871 | 1.12043 | 1.07437 |
0.924 | 0.71613 | 0.76153 | 0.67439 | 0.745 | 0.66824 | |||
E2 | 0.17586 | 0.34206 | 0.34844 | 0.33572 | 0.35556 | 0.31302 | ||
0.00344 | 0.02604 | 0.02809 | 0.02408 | 0.03042 | 0.01859 | |||
E3 | 0.26378 | 0.5131 | 0.52749 | 0.49886 | 0.53337 | 0.46953 | ||
0.00774 | 0.0586 | 0.06561 | 0.05207 | 0.06846 | 0.04182 | |||
E4 | 0.3002 | 0.58394 | 0.60258 | 0.56552 | 0.607 | 0.53439 | ||
0.01002 | 0.07589 | 0.08627 | 0.06632 | 0.08866 | 0.05418 | |||
E1 | 150 | 7 | 1.44209 | 1.10927 | 1.13531 | 1.08448 | 1.12627 | 1.08014 |
0.79386, | 0.62524 | 0.67072 | 0.58337 | 0.65418 | 0.57719 | |||
E2 | 0.17903 | 0.34127 | 0.34767 | 0.33492 | 0.35482 | 0.31269 | ||
0.00275 | 0.02572 | 0.02778 | 0.02376 | 0.03012 | 0.01796 | |||
E3 | 0.26854 | 0.51191 | 0.52633 | 0.49764 | 0.53222 | 0.46903 | ||
0.00844 | 0.05787 | 0.0649 | 0.05134 | 0.06777 | 0.04041 | |||
E4 | 0.30562 | 0.58259 | 0.60129 | 0.56412 | 0.60571 | 0.53379 | ||
0.01093 | 0.07496 | 0.08536 | 0.06537 | 0.08778 | 0.05234 | |||
E1 | 250 | 3 | 1.42874 | 1.08905 | 1.10354 | 1.07494 | 1.09884 | 1.07249 |
0.55561 | 0.78152 | 0.77575 | 0.67835 | 0.79771 | 0.6946 | |||
E2 | 0.18196 | 0.3545 | 0.35827 | 0.35075 | 0.36231 | 0.34031 | ||
0.00272 | 0.02977 | 0.03107 | 0.0285 | 0.03248 | 0.02516 | |||
E3 | 0.27294 | 0.53175 | 0.54025 | 0.52332 | 0.54347 | 0.51047 | ||
0.00611 | 0.06697 | 0.0714 | 0.06272 | 0.07309 | 0.0566 | |||
E4 | 0.31062 | 0.60517 | 0.61618 | 0.59426 | 0.61851 | 0.58095 | ||
0.00792 | 0.08675 | 0.09329 | 0.08049 | 0.09466 | 0.07331 | |||
E1 | 250 | 7 | 1.43871 | 1.094 | 1.10877 | 1.07963 | 1.10393 | 1.07719 |
0.38096 | 0.6932 | 0.71814 | 0.66939 | 0.70972 | 0.66574 | |||
E2 | 0.17714 | 0.3506 | 0.35439 | 0.34684 | 0.35853 | 0.33616 | ||
0.00238 | 0.02842 | 0.0297 | 0.02718 | 0.03111 | 0.02385 | |||
E3 | 0.26571 | 0.5259 | 0.53443 | 0.51744 | 0.53779 | 0.50424 | ||
0.00535 | 0.06395 | 0.06829 | 0.05977 | 0.07001 | 0.05365 | |||
E4 | 0.3024 | 0.59852 | 0.60956 | 0.58756 | 0.61204 | 0.57386 | ||
0.00693 | 0.08282 | 0.08925 | 0.07669 | 0.09067 | 0.06949 |
Entropy | n | ACI | HPD | |||||
Interval | AL | CP | Interval | AL | CP | |||
E1 | 150 | r=120 | 1.32397(1.54377) | 0.2198 | 0.92 | 1.204 (1.8658) | 0.6618 | 0.9 |
E2 | 0.07005 (0.27138) | 0.20133 | 0.96 | 0.02202 (0.26484) | 0.24282 | 0.94 | ||
E3 | 0.10508 (0.40707) | 0.30199 | 0.96 | 0.03303 (0.39726) | 0.36423 | 0.94 | ||
E4 | 0.11958 (0.46327) | 0.34369 | 0.96 | 0.03759 (0.45212) | 0.41452 | 0.94 | ||
E1 | 150 | r=80 | 1.31453 (1.58589) | 0.27136 | 0.953 | 1.2048 (2.05354) | 0.84873 | 0.935 |
E2 | 0.04964 (0.29784) | 0.2482 | 0.97 | -0.02797 (0.27811) | 0.30608 | 0.96 | ||
E3 | 0.07447 (0.44676) | 0.3723 | 0.97 | -0.04195 (0.41717) | 0.45912 | 0.96 | ||
E4 | 0.08475 (0.50845) | 0.4237 | 0.97 | -0.04774 (0.47477) | 0.52251 | 0.96 | ||
E1 | 250 | r=200 | 1.33008 (1.50104) | 0.17096 | 0.912 | 1.24128 (1.73582) | 0.49454 | 0.89 |
E2 | 0.10495 (0.26035) | 0.1554 | 0.93 | 0.06898 (0.25542) | 0.18644 | 0.925 | ||
E3 | 0.15742 (0.39053) | 0.23311 | 0.93 | 0.10347 (0.38313) | 0.27967 | 0.925 | ||
E4 | 0.17916 (0.44445) | 0.26529 | 0.93 | 0.11776 (0.43603) | 0.31828 | 0.925 | ||
E1 | 250 | r=170 | 1.30827 (1.56216) | 0.25389 | 0.95 | 0.87087 (1.17569) | 0.30482 | 0.930 |
E2 | 0.0614 (0.29303) | 0.23163 | 0.97 | 0.36874 (0.5565) | 0.18775 | 0.942 | ||
E3 | 0.0921 (0.43955) | 0.34745 | 0.97 | 0.55311 (0.83474) | 0.28163 | 0.943 | ||
E4 | 0.10481 (0.50024) | 0.39543 | 0.97 | 0.62948(0.95) | 0.32051 | 0.942 | ||
E1 | 150 | T1=5 | 1.28738(1.55676) | 0.26939 | 0.952 | 0.88645 (1.33038) | 0.44393 | 0.92 |
E2 | 0.05928 (0.30422) | 0.24494 | 0.971 | 0.22668(0.4469) | 0.22022 | 0.91 | ||
E3 | 0.08892 (0.45633) | 0.36741 | 0.971 | 0.34002(0.67035) | 0.33033 | 0.912 | ||
E4 | 0.10119 (0.51934) | 0.41814 | 0.971 | 0.38697(0.76291) | 0.37594 | 0.912 | ||
E1 | 150 | T1=2 | 1.29124 (1.56016) | 0.26892 | 0.94 | 0.87814 (1.32003) | 0.44189 | 0.94 |
E2 | 0.04976 (0.29565) | 0.24589 | 0.98 | 0.22572 (0.44618) | 0.22046 | 0.95 | ||
E3 | 0.07464 (0.44347) | 0.36883 | 0.98 | 0.33858 (0.66927) | 0.3307 | 0.95 | ||
E4 | 0.08494 (0.5047) | 0.41976 | 0.98 | 0.38532 (0.76168) | 0.37636 | 0.95 | ||
E1 | 250 | T1=5 | 1.32001 (1.53028) | 0.21027 | 0.88 | 0.92047 (1.25225) | 0.33179 | 0.92 |
E2 | 0.08331 (0.2749) | 0.19158 | 0.92 | 0.26901 (0.43942) | 0.17041 | 0.92 | ||
E3 | 0.12497 (0.41235) | 0.28738 | 0.92 | 0.40351 (0.65913) | 0.25562 | 0.92 | ||
E4 | 0.14222 (0.46928) | 0.32705 | 0.92 | 0.45923(0.75014) | 0.29091 | 0.92 | ||
E1 | 250 | T1=2 | 1.32681 (1.53722) | 0.21041 | 0.931 | 0.91238 (1.24443) | 0.33205 | 0.94 |
E2 | 0.07314 (0.26599) | 0.19285 | 0.96 | 0.26679 (0.43719) | 0.1704 | 0.94 | ||
E3 | 0.10971 (0.39898) | 0.28927 | 0.96 | 0.40018 (0.65578) | 0.25561 | 0.94 | ||
E4 | 0.12486 (0.45407) | 0.32921 | 0.96 | 0.45543 (0.74633) | 0.2909 | 0.94 | ||
E1 | 150 | T2=7 | 1.30623 (1.57724) | 0.27101 | 0.93 | 0.88947 (1.3293) | 0.43983 | 0.92 |
E2 | 0.05067 (0.29834) | 0.24767 | 0.95 | 0.23143 (0.45226) | 0.22083 | 0.94 | ||
E3 | 0.076 (0.44751) | 0.37151 | 0.95 | 0.34715 (0.67839) | 0.33124 | 0.94 | ||
E4 | 0.0865 (0.5093) | 0.42281 | 0.95 | 0.39508 (0.77205) | 0.37697 | 0.94 | ||
E1 | 150 | T2=3 | 1.31687 (1.58794) | 0.27108 | 0.93 | 0.88647 (1.32694) | 0.44047 | 0.93 |
E2 | 0.03946 (0.28884) | 0.24937 | 0.94 | 0.22951 (0.45066) | 0.22115 | 0.92 | ||
E3 | 0.0592 (0.43326) | 0.37406 | 0.94 | 0.34427 (0.67599) | 0.33172 | 0.92 | ||
E4 | 0.06737 (0.49308) | 0.42571 | 0.94 | 0.39181 (0.76933) | 0.37752 | 0.92 | ||
E1 | 250 | T2=7 | 1.3256 (1.53593) | 0.21033 | 0.93 | 0.92428 (1.25566) | 0.33138 | 0.94 |
E2 | 0.08241 (0.27419) | 0.19178 | 0.95 | 0.26889 (0.43892) | 0.17003 | 0.951 | ||
E3 | 0.12362 (0.41129) | 0.28767 | 0.95 | 0.40334 (0.65838) | 0.25504 | 0.951 | ||
E4 | 0.14068 (0.46808) | 0.32739 | 0.95 | 0.45903(0.74928) | 0.29025 | 0.951 | ||
E1 | 250 | T2=3 | 1.33245 (1.54265) | 0.2102 | 0.952 | 0.92852 (1.26253) | 0.33402 | 0.972 |
E2 | 0.08078 (0.27268) | 0.1919 | 0.98 | 0.26694 (0.437) | 0.17005 | 0.973 | ||
E3 | 0.12118 (0.40902) | 0.28784 | 0.98 | 0.40042 (0.6555) | 0.25508 | 0.97 | ||
E4 | 0.13791 (0.4655) | 0.32759 | 0.98 | 0.4557 (0.746) | 0.2903 | 0.972 |
Entropy | n | ACI | HPD | |||||
Interval | AL | CP | Interval | AL | CP | |||
E1 | 150 | r=120 | 1.32451 (1.54522) | 0.22071 | 0.912 | 1.20353 (1.86439) | 0.66086 | 0.94 |
E2 | 0.31014 (0.58536) | 0.27522 | 0.93 | 0.26572 (0.58693) | 0.3212 | 0.952 | ||
E3 | 0.15507 (0.29268) | 0.13761 | 0.93 | 0.13286 (0.29346) | 0.1606 | 0.951 | ||
E4 | 0.37438 (0.7066) | 0.33222 | 0.93 | 0.32076 (0.70848) | 0.38773 | 0.952 | ||
E1 | 150 | r=80 | 1.30054 (1.59109) | 0.29055 | 0.92 | 1.18112 (2.09479) | 0.91367 | 0.94 |
E2 | 0.2672 (0.62936) | 0.36215 | 0.95 | 0.19976 (0.62205) | 0.42229 | 0.96 | ||
E3 | 0.1336(0.31468) | 0.18108 | 0.95 | 0.09988 (0.31103) | 0.21114 | 0.96 | ||
E4 | 0.32254 (0.7597) | 0.43716 | 0.95 | 0.24114 (0.75088) | 0.50975 | 0.960 | ||
E1 | 250 | r=200 | 1.32132 (1.53199) | 0.21067 | 0.90 | 0.91591 (1.24612) | 0.33021 | 0.938 |
E2 | 0.32109 (0.58451) | 0.26342 | 0.96 | 0.60057 (0.93122) | 0.33065 | 0.941 | ||
E3 | 0.16055 (0.29225) | 0.13171 | 0.96 | 0.30029 (0.46561) | 0.16532 | 0.94 | ||
E4 | 0.38759 (0.70556) | 0.31797 | 0.96 | 0.72496 (1.12409) | 0.39913 | 0.941 | ||
E1 | 250 | r=170 | 1.31742 (1.57116) | 0.25374 | 0.951 | 0.86563 (1.17167) | 0.30604 | 0.95 |
E2 | 0.28194 (0.59672) | 0.31479 | 0.98 | 0.78845 (1.24102) | 0.45257 | 0.95 | ||
E3 | 0.14097 (0.29836) | 0.15739 | 0.98 | 0.39422(0.62051) | 0.22629 | 0.951 | ||
E4 | 0.34033 (0.72031) | 0.37998 | 0.98 | 0.95174 (1.49804) | 0.5463 | 0.951 | ||
E1 | 150 | T1=5 | 1.32346(1.5948) | 0.27135 | 0.292 | 0.89618 (1.34279) | 0.44661 | 0.90 |
E2 | 0.27375 (0.61058) | 0.33683 | 0.95 | 0.53076 (0.94919) | 0.41843 | 0.94 | ||
E3 | 0.13687(0.30529) | 0.16842 | 0.95 | 0.26536(0.47461) | 0.20924 | 0.94 | ||
E4 | 0.33044 (0.73704) | 0.40659 | 0.95 | 0.64065 (1.14576) | 0.5051 | 0.94 | ||
E1 | 150 | T1=3 | 1.30039 (1.57202) | 0.27163 | 0.891 | 0.87685 (1.31329) | 0.43644 | 0.88 |
E2 | 0.27726 (0.61612) | 0.33886 | 0.94 | 0.54827 (0.9726) | 0.42433 | 0.93 | ||
E3 | 0.13863 (0.30806) | 0.16943 | 0.94 | 0.27414 (0.4863) | 0.21217 | 0.93 | ||
E4 | 0.33468 (0.74373) | 0.40905 | 0.94 | 0.66182 (1.17403) | 0.51221 | 0.93 | ||
E1 | 250 | T1=5 | 1.31995 (1.53043) | 0.21048 | 0.94 | 0.92732 (1.25933) | 0.33201 | 0.97 |
E2 | 0.33064 (0.59527) | 0.26462 | 0.97 | 0.60102 (0.93213) | 0.33111 | 0.96 | ||
E3 | 0.16532 (0.29763) | 0.13231 | 0.97 | 0.30051 (0.46607) | 0.16556 | 0.96 | ||
E4 | 0.39912 (0.71855) | 0.31943 | 0.97 | 0.72549 (1.12518) | 0.39969 | 0.96 | ||
E1 | 250 | T1=2 | 1.33397 (1.54433) | 0.21036 | 0.88 | 0.92234 (1.25581) | 0.33347 | 0.89 |
E2 | 0.31502 (0.57695) | 0.26194 | 0.91 | 0.59443 (0.92381) | 0.32939 | 0.92 | ||
E3 | 0.15751 (0.28848) | 0.13097 | 0.91 | 0.29721 (0.46191) | 0.16469 | 0.92 | ||
E4 | 0.38026 (0.69645) | 0.31619 | 0.91 | 0.71753 (1.11514) | 0.3976 | 0.92 | ||
E1 | 150 | T2=7 | 1.30473 (1.57627) | 0.27154 | 0.93 | 0.89139 (1.33151) | 0.44012 | 0.94 |
E2 | 0.05189 (0.29982) | 0.24793 | 0.96 | 0.23277 (0.45373) | 0.22096 | 0.95 | ||
E3 | 0.07784 (0.44973) | 0.37189 | 0.96 | 0.34912 (0.68059) | 0.33146 | 0.95 | ||
E4 | 0.08858 (0.51183) | 0.42324 | 0.96 | 0.39734 (0.77451) | 0.37717 | 0.95 | ||
E1 | 150 | T2=3 | 1.30623 (1.57796) | 0.27172 | 0.94 | 0.89652 (1.33842) | 0.44189 | 0.93 |
E2 | 0.05519 (0.30286) | 0.24767 | 0.97 | 0.23189 (0.45337) | 0.22149 | 0.94 | ||
E3 | 0.08279 (0.45429) | 0.3715 | 0.97 | 0.34783 (0.68006) | 0.33223 | 0.94 | ||
E4 | 0.09422 (0.51701) | 0.42279 | 0.97 | 0.39585 (0.77396) | 0.37811 | 0.94 | ||
E1 | 250 | T2=7 | 1.32349 (1.53398) | 0.21048 | 0.92 | 0.92772 (1.25923) | 0.33151 | 0.956 |
E2 | 0.08622 (0.2777) | 0.19149 | 0.96 | 0.27025 (0.44047) | 0.17022 | 0.981 | ||
E3 | 0.12932 (0.41655) | 0.28723 | 0.96 | 0.40537 (0.6607) | 0.25533 | 0.981 | ||
E4 | 0.14718 (0.47407) | 0.32689 | 0.96 | 0.46134 (0.75193) | 0.29058 | 0.951 | ||
E1 | 250 | T2=3 | 1.33356 (1.54387) | 0.21031 | 0.91 | 0.93109 (1.26572) | 0.33463 | 0.899 |
E2 | 0.08117 (0.27311) | 0.19194 | 0.945 | 0.26625 (0.43669) | 0.17044 | 0.91 | ||
E3 | 0.12176 (0.40967) | 0.28791 | 0.945 | 0.39937 (0.65503) | 0.25566 | 0.912 | ||
E4 | 0.13857 (0.46623) | 0.32766 | 0.945 | 0.45451 (0.74547) | 0.29096 | 0.912 |
Here are some observations on the MLEs and BEs of entropy measure performance as shown in Tables 2–9 above.
(1) The MSEs of MLEs and BEs decrease when T1 increases for all entropy measures in most cases and this satisfies Case 1 in the GT-Ⅱ HCS.
(2) The MSEs of MLEs and BEs decrease when r increases for all entropy measures in most cases and this satisfies Case 2 in the GT-Ⅱ HCS.
(3) The MSEs of MLEs and BEs decrease when T2 increases for all entropy measures in most cases and this satisfies Case 3 in the GT-Ⅱ HCS (see Tables 2–7). Additional clarification is available in Figures 3–6.
(4) In most circumstances, the BEs of all entropy measurements under LINEX LOF provide the best values and are greater than data gathered under other LOFs (see Tables 2–7).
(5)The MSE at ζ=1.5 is smaller than the MSE at ζ=0.5 for most entropy measurements, and as the value of ζ increases, the BEs of all entropy measurements improve (see Tables 2–7).
(6) The BEs of all entropy measurements under LINEX LOF and GE LOF (q=−4) provide more information, exhibiting smaller MSE values and consequently less uncertainty in most cases (see Tables 2–7). Further explanation is available in Figure 7.
(7)Estimating entropy measures for the Weibull distribution aids in analyzing reliability data, enabling better decision-making regarding product reliability and risk assessment. Bayesian entropy estimates can also be utilized to analyze financial data involving Weibull distributions, helping evaluate financial risk and time-to-event outcomes.
(8) The developed estimators can be applied to analyze survival data in medical studies, contributing to understanding disease progression and patient outcomes.
(9) As seen in Tables 8 and 9, the length of the interval decreases and the CP values drop as the values of r,T1, and T2 increase. The CPs of the BEs for the entropy measures are smaller than those corresponding to the MLEs.
This paper is structured as a case study in which we look at some fiber strength data. The sample consists of experimental data from the National Physical Laboratory in England on the strength of 1.5-cm-long glass fibers. The data set is obtained from Alizadeh et al. [35]:
0.55, 0.74, 0.77, 0.81, 0.84, 0.93, 1.04, 1.11, 1.13, 1.24, 1.25, 1.27, 1.28, 1.29, 1.30, 1.36, 1.39, 1.42, 1.48, 1.48, 1.49, 1.49, 1.50, 1.50, 1.51, 1.52, 1.53, 1.54, 1.55, 1.55, 1.58, 1.59, 1.60, 1.61, 1.61, 1.61, 1.61, 1.62, 1.62, 1.63, 1.64, 1.66, 1.66, 1.66, 1.67, 1.68, 1.68, 1.69, 1.70, 1.70, 1.73, 1.76, 1.76, 1.77, 1.78, 1.81, 1.82, 1.84, 1.84, 1.89, 2.00, 2.01, 2.24.
According to the Kolmogorov-Smirnov goodness of fit test applied to this genuine data, the Weibull distribution matches the data where the p-value = 0.53 and the statistic value = 0.0311. Figure 8 illustrates the estimated PDF and CDF of the Weibull distribution.
We will now examine what occurs if the data are censored. Using this data set, we produce three artificial GT-Ⅱ HCS sets in the manner described below:
Case1:T1=1.5,T2=2,r=30whereD=32,C=T1=1.5.Case2:T1=1.5,T2=2,r=40whereD=40,C=xr=1.63.Case3:T1=1.5,T2=2,r=60whereD=55,C=T2=2. |
For entropy measurements in these situations, we applied ML and Bayesian techniques. We employed the MCMC algorithm with a dataset of N=10000 observations and M=1000 as burn-in at various LOFs. To compute the BEs, we utilize a non-informative prior because we do not know anything about the priors. We take a1=b1=a2=b2=0.0001, which are almost identical to Jeffrey's prior as mentioned by Congdon [36]. The value of ζ is selected as ζ=1.5.
The BE of entropy via LINEX LOF and the GE LOF at q=−4 have a large value as shown in Table 10. In the end, it is concluded that the actual data matches the simulated research findings.
Entropy | MLE | SE | LINEX | GE | |||
q=(4) | q=(−4) | q=(4) | q=(−4) | ||||
E1 | 0.87621 | 0.80134 | 0.81222 | 0.79066 | 0.81134 | 0.78419 | |
E2 | Case 1 | 0.43147 | 0.49738 | 0.50214 | 0.49264 | 0.50444 | 0.48498 |
E3 | 0.64721 | 0.74759 | 0.75809 | 0.7372 | 0.75794 | 0.72962 | |
E4 | 0.73657 | 0.85118 | 0.86477 | 0.83793 | 0.8629 | 0.83111 | |
E1 | 0.8699 | 0.74041 | 0.75034 | 0.73028 | 0.75034 | 0.72235 | |
E2 | Case 2 | 0.32401 | 0.43615 | 0.4409 | 0.43133 | 0.44414 | 0.42118 |
E3 | 0.48601 | 0.65422 | 0.66488 | 0.64335 | 0.66621 | 0.63178 | |
E4 | 0.55312 | 0.74455 | 0.75835 | 0.73046 | 0.7582 | 0.71901 | |
E1 | 0.77377 | 0.61321 | 0.6271 | 0.5993 | 0.62968 | 0.58181 | |
E2 | Case 3 | 0.32039 | 0.37454 | 0.38353 | 0.36519 | 0.39153 | 0.3149 |
E3 | 0.48059 | 0.56181 | 0.58189 | 0.54052 | 0.5873 | 0.47235 | |
E4 | 0.54695 | 0.63938 | 0.6653 | 0.61165 | 0.66839 | 0.53757 |
Entropy is a useful metric for measuring information uncertainty. Likewise, in the fields of survival analysis and reliability engineering, the Weibull distribution is a crucial lifetime model. Thus this work examines the maximum likelihood and Bayesian estimators of Shannon entropy, ζ-entropy, Arimoto entropy, and Havrda and Charvat entropy for the Weibull distribution using the GT-Ⅱ HCS. The Weibull distribution's entropy expressions are established in Section 2. In classical estimation, the ML estimators of parameters are first derived, next the ML entropy estimators may be acquired through the invariance property, and then the ACIs are calculated in terms of their average length and CPs. In Bayesian estimation, the SLOF and the ASLOF are selected. Nevertheless, computing the forms of Bayesian estimators and HPD is challenging due to their complexity. This issue is resolved by applying the MCMC techniques, specifically employing the M-H algorithm. The numerical results lead to the following conclusions:
● The MLEs and BEs for different entropy measurements show a decreasing trend in their MSEs as the termination time increases in most scenarios.
● Bayesian estimates under different LOFs outperform the MLEs for all entropy measurements in the majority of cases, indicating superior performance.
● Bayesian estimates of entropy measurements using LINEX and GE LOFs at q=−4 exhibit a high level of uncertainty, suggesting potential challenges in estimating entropy under these conditions.
● The CPs of the BEs for the entropy measures are smaller than that corresponding to the MLEs and the average length of the intervals decreases when the sample size increases.
● The results obtained from the analysis of real data examples align with those from the simulated data, indicating the reliability and validity of the findings across different data sets.
One of the study's drawbacks is that it only considers the Weibull distribution when utilizing both classical and Bayesian estimation approaches under the GT-Ⅱ HCS. Furthermore, simulation studies are conducted using large sample sizes. Future research could explore alternative probability distributions beyond the Weibull distribution and investigate the performance of different loss functions in entropy estimation. Additionally, alternative methods such as the Lindley and Tierney-Kadane approximation methods could be considered for calculating entropy measures. Furthermore, conducting simulation studies with both small and large sample sizes would provide a comprehensive understanding of the behavior of entropy estimation methods across different data scenarios.
Amal S. Hassan, Najwan Alsadat and Baria A. Helmy: Conceptualization, Original draft, Methodology, Formal analysis, Writing; Oluwafemi Samson Balogun: Conceptualization, Original draft, Methodology, Formal analysis. All authors have read and approved the final version of the manuscript for publication.
The authors gratefully thank the editor and the anonymous referees for their valuable comments on an earlier version of this manuscript. Their insightful feedback has significantly improved the quality of the final version. This research is supported by the Researchers Supporting Project number (RSPD2024R548), King Saud University, Riyadh, Saudi Arabia.
The authors declare no conflicts of interest.
[1] |
D. Molodtsov, Soft set theory-first results, Comput. Math. Appl., 37 (1999), 19–31. https://doi.org/10.1016/S0898-1221(99)00056-5 doi: 10.1016/S0898-1221(99)00056-5
![]() |
[2] |
M. Yazdi, E. Zarei, S. Adumene, R. Abbassi, P. Rahnamayiezekavat, Uncertainty modeling in risk assessment of digitalized process systems, Meth. Chem. Proc. Safety, 6 (2022), 389–416. https://doi.org/10.1016/bs.mcps.2022.04.005 doi: 10.1016/bs.mcps.2022.04.005
![]() |
[3] | H. Li, M. Yazdi, Stochastic game theory approach to solve system safety and reliability decision-making problem under uncertainty, In: Advanced Decision-Making Methods and Applications in System Safety and Reliability Problems. Studies in Systems, Decision and Control, Springer, 211 (2022). https://doi.org/10.1007/978-3-031-07430-1_8 |
[4] | E. Zarei, M. Yazdi, R. Moradi, A. BahooToroody, Expert judgment and uncertainty in sociotechnical systems analysis, In: in Safety Causation Analysis in Sociotechnical Systems: Advanced Models and Techniques. Studies in Systems, Decision and Control, Springer, 541 (2024). https://doi.org/10.1007/978-3-031-62470-4_18 |
[5] |
P. K. Maji, R. Biswas, A. J. Roy, Soft set theory, Comput. Math. Appl., 45 (2003), 555–562. https://doi.org/10.1016/S0898-1221(03)00016-6 doi: 10.1016/S0898-1221(03)00016-6
![]() |
[6] |
M. I. Ali, F. Feng, X. Liu, W. K. Min, M. Shabir, On some new operations in soft set theory, Comput. Math. Appl., 57 (2009), 1547–1553. https://doi.org/10.1016/j.camwa.2008.11.009 doi: 10.1016/j.camwa.2008.11.009
![]() |
[7] |
J. C. R. Alcantud, A. Z. Khameneh, G. Santos-Garcia, M. Akram, A systematic literature review of soft set theory, Neural Comput. Applic., 36 (2024), 8951–8975. https://doi.org/10.1007/s00521-024-09552-x doi: 10.1007/s00521-024-09552-x
![]() |
[8] |
N. Çağman, S. Karataş, S. Enginoğlu, Soft topology, Comput. Math. Appl., 62 (2011), 351–358. https://doi.org/10.1016/j.camwa.2011.05.016 doi: 10.1016/j.camwa.2011.05.016
![]() |
[9] |
M. Shabir, M. Naz, On soft topological spaces, Comput. Math. Appl., 61 (2011), 1786–1799. https://doi.org/10.1016/j.camwa.2011.02.006 doi: 10.1016/j.camwa.2011.02.006
![]() |
[10] | S. Nazmul, S. K. Samanta, Neighbourhood properties of soft topological spaces, Ann. Fuzzy Math. Inform., 6 (2013), 1–15. |
[11] |
S. Hussain, B. Ahmad, Some properties of soft topological spaces, Comput. Math. Appl., 62 (2011), 4058–4067. https://doi.org/10.1016/j.camwa.2011.09.051 doi: 10.1016/j.camwa.2011.09.051
![]() |
[12] |
W. K. Min, A note on soft topological spaces, Comput. Math. Appl., 61 (2011), 3524–3528. https://doi.org/10.1016/j.camwa.2011.08.068 doi: 10.1016/j.camwa.2011.08.068
![]() |
[13] |
M. Terepeta, On separating axioms and similarity of soft topological spaces, Soft Comput., 23 (2019), 1049–1057. https://doi.org/10.1007/s00500-017-2824-z doi: 10.1007/s00500-017-2824-z
![]() |
[14] |
T. M. Al-shami, M. E. El-Shafei, Partial belong relation on soft separation axioms and decision-making problem, two birds with one stone, Soft Comput., 24 (2020), 5377–5387. https://doi.org/10.1007/s00500-019-04295-7 doi: 10.1007/s00500-019-04295-7
![]() |
[15] |
H. Hazra, P. Majumdar, S. K. Samanta, Soft topology, Fuzzy Inform. Eng., 4 (2012), 105–115. https://doi.org/10.1007/s12543-012-0104-2 doi: 10.1007/s12543-012-0104-2
![]() |
[16] |
A. Aygünoğlu, H. Aygün, Some notes on soft topological spaces, Neural Comput. Applic., 21 (2012), 113–119. https://doi.org/10.1007/s00521-011-0722-3 doi: 10.1007/s00521-011-0722-3
![]() |
[17] | I. Zorlutuna, M. Akdag, W. K. Min, S. Atmaca, Remarks on soft topological spaces, Ann. Fuzzy Math. Inform., 3 (2012), 171–185. |
[18] | S. Das, S. K. Samanta, Soft metric, Ann. Fuzzy Math. Inform., 6 (2013), 77–94. |
[19] | T. M. Al-shami, L. D. R. Kočinac, The equivalence between the enriched and extended soft topologies, Appl. Comput. Math., 18 (2019), 149–162. |
[20] |
J. C. R. Alcantud, Soft open bases and a novel construction of soft topologies from bases for topologies, Mathematics, 8 (2020), 672. https://doi.org/10.3390/math8050672 doi: 10.3390/math8050672
![]() |
[21] |
J. C. R. Alcantud, An operational characterization of soft topologies by crisp topologies, Mathematics, 9 (2021), 1656. https://doi.org/10.3390/math9141656 doi: 10.3390/math9141656
![]() |
[22] |
M. Matejdes, Methodological remarks on soft topology, Soft Comput., 25 (2021), 4149–4156. https://doi.org/10.1007/s00500-021-05587-7 doi: 10.1007/s00500-021-05587-7
![]() |
[23] |
G. Ali, M. Akram, Decision-making method based on fuzzy N-soft expert sets, Arabian J. Sci. Eng., 45 (2020), 10381–10400. https://doi.org/10.1007/s13369-020-04733-x doi: 10.1007/s13369-020-04733-x
![]() |
[24] |
A. Adeel, M. Akram, N. Çağman, Decision-making analysis based on hesitant fuzzy N-soft ELECTRE-I approach, Soft Comput., 26 (2022), 11849–11863. https://doi.org/10.1007/s00500-022-06981-5 doi: 10.1007/s00500-022-06981-5
![]() |
[25] | J. C. R. Alcantud, G. Santos-Garcia, M. Akram, A novel methodology for multi-agent decision-making based on N-soft sets, Soft Comput., 2023. https://doi.org/10.1007/s00500-023-08522-0 |
[26] | A. Kerckhoffs, La cryptographie militaire, J. Sci. Milit., 9 (1883), 5–38. |
[27] |
R. L. Rivest, A. Shamir, L. Adleman, A method for obtaining digital signatures and public-key cryptosystems, Commun. ACM, 21 (1978), 120–126. https://doi.org/10.1145/359340.359342 doi: 10.1145/359340.359342
![]() |
[28] | W. Diffie, M. E. Hellman, Multiuser cryptographic techniques, In: AFIPS National Computer Conference, New York, 1976. https://doi.org/10.1145/1499799.1499815 |
[29] | M. O. Rabin, Digitalized signatures and public key functions as intractable as factorisation, In: MIT/LCS/TR-212 MIT Laboratory for Computer Science, 1979. |
[30] |
H. Aktaş, M. Kalkan, An application of the crystography, J. Math. Comput. Sci., 11 (2014), 147–158. http://dx.doi.org/10.22436/jmcs.011.02.07 doi: 10.22436/jmcs.011.02.07
![]() |
[31] |
N. Çağman, S. Enginoğlu, Soft matrix theory and its decision making, Comput. Math. Appl., 59 (2010), 3308–3314. https://doi.org/10.1016/j.camwa.2010.03.015 doi: 10.1016/j.camwa.2010.03.015
![]() |
[32] |
F. Feng, J. Cho, W. Pedrycz, H. Fujita, T. Herawan, Soft set based association rule mining, Knowledge-Based Syst., 111 (2016), 268–282. https://doi.org/10.1016/j.knosys.2016.08.020 doi: 10.1016/j.knosys.2016.08.020
![]() |
[33] | M. Kalkan, Z. Zararsız, Kriptografi, steganografi ve kodlama teorisinin grup teorisi ile ilişkisinin incelenmesi ve bazı uygulamaların geliştirilmesi, Ph.D thesis, Nevşehir Hacıbektaş University, 2021. |
[34] |
Z. Liu, J. C. R. Alcantud, K. Qin, L. Xiong, The soft sets and fuzzy sets-based neural networks and application, IEEE Access, 111 (2020), 268–282. https://doi.org/10.1109/ACCESS.2020.2976731 doi: 10.1109/ACCESS.2020.2976731
![]() |
[35] | B. K. Tripathy, T. R. Sooraj, R. K. Mohanty, Rough set and soft set models in image processing, In: Intelligent Multimedia Data Analysis, Berlin, Boston, De Gruyter, 2019, 123–144. https://doi.org/10.1515/9783110552072-006 |
[36] |
E. Aygün, Soft matrix product and soft cryptosystem, Filomat, 32 (2019), 6519–6530. https://doi.org/10.2298/FIL1819519A doi: 10.2298/FIL1819519A
![]() |
[37] |
E. Aygün, AES encryption and a cryptosystem obtained with soft set Ⅱ, Cumhur. Sci. J., 40 (2019), 69–78. https://doi.org/10.17776/csj.416395 doi: 10.17776/csj.416395
![]() |
[38] |
B. Paik, S. K. Mondal, Introduction to soft cryptosystem and its application, Wirel. Pers. Commun., 125 (2022), 1801–1826. https://doi.org/10.1007/s11277-022-09635-9 doi: 10.1007/s11277-022-09635-9
![]() |
[39] | İ. Yılmaz, Esnek kümeler ve vernam şifreleme üzerine, MSc. thesis, Erciyes University, 2023. |
[40] | M. Matsui, Linear cryptanalysis method for DES cipher, Lect. Notes Comput. Sci., 765 (1994), 386–397. |
[41] | D. R. Stinson, Cryptography theory and practice, Boca Raton: Chapman & Hall/CRC, 2006. |
n | r | T1 | T2 |
250 | 200 | (2, 5) | 7 |
150 | 120 | (2, 5) | 7 |
250 | (200,170) | 0.2 | 1.2 |
150 | (120, 80) | 0.2 | 1.2 |
250 | 200 | 1.5 | (3, 7) |
150 | 120 | 1.5 | (3, 7) |
Entropy | n | r | MLE | SE | LINEX | GE | ||
q=(−4) | q=(4) | q=(−4) | q=(4) | |||||
E1 | 150 | 120 | 1.36712 | 1.51529 | 1.57698 | 1.4603 | 1.54341 | 1.46821 |
0.60911 | 0.61993 | 0.78749 | 0.47828 | 0.69251 | 0.5022 | |||
E2 | 0.19348 | 0.14388 | 0.15158 | 0.13615 | 0.17786 | 0.01169 | ||
0.0026 | 0.00428 | 0.00363 | 0.00507 | 0.00157 | 0.03013 | |||
E3 | 0.29022 | 0.21582 | 0.23314 | 0.19842 | 0.26679 | 0.01753 | ||
0.0059 | 0.00964 | 0.00754 | 0.01239 | 0.00353 | 0.0678 | |||
E4 | 0.33029 | 0.24562 | 0.26804 | 0.22307 | 0.30362 | 0.01995 | ||
0.0077 | 0.01249 | 0.00946 | 0.0166 | 0.00457 | 0.08781 | |||
E1 | 150 | 80 | 1.45558 | 1.59925 | 1.70715 | 1.51007 | 1.64329 | 1.52503 |
.43429 | 0.88746 | 1.21708 | 0.63845 | 1.01106 | 0.68956 | |||
E2 | 0.14164 | 0.12597 | 0.13823 | 0.1136 | 0.18205 | 0.00373 | ||
0.00183 | 0.00796 | 0.00644 | 0.00983 | 0.00175 | 0.03244 | |||
E3 | 0.21247 | 0.18895 | 0.2165 | 0.16107 | 0.27307 | 0.00559 | ||
0.0041 | 0.01791 | 0.01305 | 0.02451 | 0.00394 | 0.07299 | |||
E4 | 0.2418 | 0.21504 | 0.2507 | 0.17889 | 0.31078 | 0.00636 | ||
0.00532 | 0.0232 | 0.0162 | 0.03311 | 0.0051 | 0.09454 | |||
E1 | 250 | 200 | 1.41556 | 1.47743 | 1.51066 | 1.44628 | 1.49357 | 1.45048 |
0.42562 | 0.49233 | 0.57613 | 0.41596 | 0.53207 | 0.42718 | |||
E2 | 0.18265 | 0.16257 | 0.1671 | 0.15803 | 0.1816 | 0.05219 | ||
0.0188 | 0.0166 | 0.0147 | 0.019 | 0.0093 | 0.02149 | |||
E3 | 0.27397 | 0.24386 | 0.25404 | 0.23364 | 0.2724 | 0.07828 | ||
0.0423 | 0.0374 | 0.0312 | 0.0457 | 0.021 | 0.04836 | |||
E4 | 0.3118 | 0.27753 | 0.29072 | 0.26429 | 0.31001 | 0.08909 | ||
0.00547 | 0.0484 | 0.0395 | 0.0609 | 0.0272 | 0.06263 | |||
E1 | 250 | 170 | 1.43521 | 1.02243 | 1.03456 | 1.01037 | 1.03126 | 1.00721 |
0.7071 | 0.57443 | 0.59289 | 0.55637 | 0.58775 | 0.55186 | |||
E2 | 0.17722 | 0.46139 | 0.46597 | 0.45683 | 0.46873 | 0.44847 | ||
0.00349 | 0.0779 | 0.08047 | 0.07539 | 0.08202 | 0.07092 | |||
E3 | 0.26582 | 0.69208 | 0.70242 | 0.68185 | 0.7031 | 0.67271 | ||
0.00784 | 0.17527 | 0.184 | 0.16684 | 0.18454 | 0.15957 | |||
E4 | 0.30253 | 0.78764 | 0.80104 | 0.77439 | 0.80017 | 0.76559 | ||
0.01016 | 0.22701 | 0.23991 | 0.21462 | 0.23902 | 0.20668 |
Entropy | n | T1 | MLE | SE | LINEX | GE | ||
q=(−4) | q=(4) | q=(−4) | q=(4) | |||||
E1 | 150 | 2 | 1.42207 | 1.10074 | 1.12712 | 1.07561 | 1.11805 | 1.07101 |
0.74852 | 0.71389 | 0.75988 | 0.67162 | 0.74317 | 0.66525 | |||
E2 | 0.18175 | 0.33537 | 0.3417 | 0.32909 | 0.34903 | 0.304 | ||
0.00373 | 0.02404 | 0.02599 | 0.02218 | 0.02829 | 0.01683 | |||
E3 | 0.27262 | 0.50306 | 0.51733 | 0.48895 | 0.52355, | 0.45599 | ||
0.00838 | 0.0541 | 0.06077 | 0.0479 | 0.06366 | 0.03786 | |||
E4 | 0.31026 | 0.57252 | 0.59102 | 0.55425 | 0.59583 | 0.51895 | ||
0.01086 | 0.07006 | 0.07995 | 0.06098 | 0.08245 | 0.04904 | |||
E1 | 150 | 5 | 1.4257 | 1.09124 | 1.11732 | 1.06638 | 1.10857 | 1.06145 |
0.35491 | 0.696 | 0.74062 | 0.65491 | 0.72485 | 0.64805 | |||
E2 | 0.1727 | 0.33467 | 0.34102 | 0.32838 | 0.34835 | 0.30641 | ||
0.00347 | 0.02359 | 0.02554 | 0.02173 | 0.02785 | 0.01618 | |||
E3 | 0.25905 | 0.50201 | 0.51631 | 0.48787 | 0.52252 | 0.45961 | ||
0.0078 | 0.05307 | 0.05974 | 0.04687 | 0.06266 | 0.0364 | |||
E4 | 0.29482 | 0.57132 | 0.58986 | 0.55302 | 0.59467 | 0.52307 | ||
0.01011 | 0.06874 | 0.07862 | 0.05965 | 0.08116 | 0.04714 | |||
E1 | 250 | 2 | 1.42515 | 1.08207 | 1.09661 | 1.06792 | 1.09196 | 1.06535 |
0.5497 | 0.67419 | 0.69842 | 0.65104 | 0.6904 | 0.64724 | |||
E2 | 0.1791 | 0.35346 | 0.35724 | 0.34969 | 0.36132 | 0.33912 | ||
0.00256 | 0.02935 | 0.03065 | 0.02808 | 0.03207 | 0.02472 | |||
E3 | 0.26866 | 0.53018 | 0.53871 | 0.52171 | 0.54198 | 0.50868 | ||
0.00575 | 0.06603 | 0.07045 | 0.06178 | 0.07215 | 0.05562 | |||
E4 | 0.30575 | 0.60339 | 0.61444 | 0.59241 | 0.61682 | 0.57892 | ||
0.00745 | 0.08552 | 0.09206 | 0.07928 | 0.09345 | 0.07204 | |||
E1 | 250 | 5 | 1.43201 | 1.074 | 1.08855 | 1.05981 | 1.08398 | 1.0570 |
0.36655 | 0.66264 | 0.68669 | 0.63962 | 0.67882 | 0.63567 | |||
E2 | 0.16956 | 0.35109 | 0.35487 | 0.34733 | 0.35901 | 0.33668 | ||
0.00258 | 0.02859 | 0.02987 | 0.02734 | 0.03129 | 0.02401 | |||
E3 | 0.25435 | 0.52664 | 0.53516 | 0.51817 | 0.53851 | 0.50502 | ||
0.00581 | 0.06432 | 0.06868 | 0.06014 | 0.07039 | 0.05403 | |||
E4 | 0.28946 | 0.59935 | 0.6104 | 0.58839 | 0.61286 | 0.57474 | ||
0.00752 | 0.08331 | 0.08975 | 0.07717 | 0.09117 | 0.06998 |
Entropy | n | T2 | MLE | SE | LINEX | GE | ||
q=(−4) | q=(4) | q=(−4) | q=(4) | |||||
E1 | 150 | 3 | 1.44174 | 1.10164 | 1.12749 | 1.07697 | 1.11865 | 1.07244 |
0.69463 | 0.71086 | 0.7555 | 0.66966 | 0.73956 | 0.66315 | |||
E2 | 0.17451 | 0.34042 | 0.34679 | 0.33409 | 0.35394 | 0.31117 | ||
0.00411 | 0.0254 | 0.02744 | 0.02346 | 0.02978 | 0.01788 | |||
E3 | 0.26176 | 0.51063 | 0.525 | 0.49642 | 0.53092 | 0.46676 | ||
0.00925 | 0.05716 | 0.06412 | 0.05069 | 0.067 | 0.04023 | |||
E4 | 0.2979 | 0.58113 | 0.59976 | 0.56274 | 0.60422 | 0.5312 | ||
0.01198 | 0.07404 | 0.08435 | 0.06454 | 0.08678 | 0.05211 | |||
E1 | 150 | 7 | 1.45241 | 1.09892 | 1.12479 | 1.07423 | 1.116 | 1.06958 |
0.41651 | 0.70499 | 0.74941 | 0.664 | 0.73368 | 0.65728 | |||
E2 | 0.16415 | 0.3389 | 0.34527 | 0.33257 | 0.35247 | 0.31123 | ||
0.0043 | 0.02485 | 0.02687 | 0.02293 | 0.02921 | 0.01722 | |||
E3 | 0.24623 | 0.50835 | 0.52272 | 0.49412 | 0.5287 | 0.46684 | ||
0.00967 | 0.05592 | 0.06282 | 0.0495 | 0.06572 | 0.03875 | |||
E4 | 0.28022 | 0.57853 | 0.59716 | 0.56012 | 0.6017 | 0.5313 | ||
0.01253 | 0.07243 | 0.08265 | 0.0630 | 0.08512 | 0.05019 | |||
E1 | 250 | 3 | 1.43076 | 1.0857 | 1.10019 | 1.07159 | 1.09552 | 1.06909 |
0.36052 | 0.67711 | 0.70126 | 0.654 | 0.69327 | 0.65021 | |||
E2 | 0.1783 | 0.35307 | 0.35684 | 0.34933 | 0.36091 | 0.33882 | ||
0.00213 | 0.02927 | 0.03056 | 0.02801 | 0.03197 | 0.02468 | |||
E3 | 0.26745 | 0.52961 | 0.5381 | 0.52119 | 0.54137 | 0.50823 | ||
0.00479 | 0.06585 | 0.07024 | 0.06164 | 0.07194 | 0.05553 | |||
E4 | 0.30438 | 0.60274 | 0.61373 | 0.59183 | 0.61612 | 0.5784 | ||
0.00621 | 0.0853 | 0.09178 | 0.0791 | 0.09317 | 0.07192 | |||
E1 | 250 | 7 | 1.43755 | 1.09109 | 1.10581 | 1.07676 | 1.10102 | 1.07429 |
0.35688 | 0.68555 | 0.71023 | 0.66196 | 0.702 | 0.6582 | |||
E2 | 0.17673 | 0.35121 | 0.35498 | 0.34746 | 0.35909 | 0.33684 | ||
0.00222 | 0.02865 | 0.02993 | 0.02741 | 0.03134 | 0.02408 | |||
E3 | 0.2651 | 0.52681 | 0.53531 | 0.51838 | 0.53864 | 0.50526 | ||
0.00499 | 0.06447 | 0.06882 | 0.0603 | 0.07052 | 0.05418 | |||
E4 | 0.3017 | 0.59955 | 0.61056 | 0.58863 | 0.61301 | 0.57502 | ||
0.00646 | 0.0835 | 0.08993 | 0.07737 | 0.09134 | 0.07018 |
Entropy | n | r | MLE | SE | LINEX | GE | ||
q=(−4) | q=(4) | q=(−4) | q=(4) | |||||
E1 | 150 | 80 | 1.44582 | 1.603 | 1.73021 | 1.50082 | 1.65364 | 1.51755 |
0.40585 | 0.92552 | 1.32941 | 0.63469 | 1.06945 | 0.69625 | |||
E2 | 0.44828 | 0.39371 | 0.41826 | 0.37158 | 0.43654 | 0.25012 | ||
0.00698 | 0.01149 | 0.00913 | 0.01474 | 0.00655 | 0.06768 | |||
E3 | 0.22414 | 0.19686 | 0.20282 | 0.19119 | 0.21827 | 0.12506 | ||
0.00174 | 0.00287 | 0.00255 | 0.00326 | 0.00164 | 0.01692 | |||
E4 | 0.54112 | 0.47525 | 0.51149 | 0.4433 | 0.52695 | 0.30192 | ||
0.01017 | 0.01675 | 0.0128 | 0.02258 | 0.00955 | 0.09861 | |||
E1 | 150 | 120 | 1.43487 | 1.5157 | 1.57724 | 1.46066 | 1.54378 | 1.46853 |
0.37627 | 0.61997 | 0.78671 | 0.47843 | 0.69239 | 0.50217 | |||
E2 | 0.44775 | 0.41609 | 0.42997 | 0.40302 | 0.43997 | 0.36094 | ||
0.00522 | 0.00589 | 0.00495 | 0.00714 | 0.00406 | 0.01896 | |||
E3 | 0.22388 | 0.20805 | 0.21146 | 0.20473 | 0.21998 | 0.18047 | ||
0.00131 | 0.00147 | 0.00134 | 0.00162 | 0.00101 | 0.00474 | |||
E4 | 0.54049 | 0.50227 | 0.52262 | 0.48332 | 0.53109 | 0.43569 | ||
0.00761 | 0.00859 | 0.00699 | 0.01083 | 0.00591 | 0.02762 | |||
E1 | 250 | 170 | 1.44429 | 1.01809 | 1.03028 | 1.00595 | 1.027 | 1.0027 |
0.39576 | 0.5683 | 0.58679 | 0.5502 | 0.58168 | 0.5456 | |||
E2 | 0.43933 | 0.99617 | 1.02445 | 0.97078 | 1.0163 | 0.96342 | ||
0.00699 | 0.29079 | 0.32224 | 0.26396 | 0.31289 | 0.2566 | |||
E3 | 0.21966 | 0.49809 | 0.50495 | 0.49158 | 0.50815 | 0.48171 | ||
0.00175 | 0.0727 | 0.07646 | 0.06922 | 0.07822 | 0.06415 | |||
E4 | 0.53032 | 1.20248 | 1.24422 | 1.16586 | 1.22679 | 1.16296 | ||
0.01019 | 0.42372 | 0.48007 | 0.37722 | 0.45592 | 0.3739 | |||
E1 | 250 | 200 | 1.42666 | 1.07694 | 1.09132 | 1.06292 | 1.08678 | 1.06027 |
0.5947 | 0.46296 | 0.48666 | 0.44027 | 0.47897 | 0.43631 | |||
E2 | 0.4528 | 0.75476 | 0.76948 | 0.74095 | 0.76889 | 0.73139 | ||
0.00397 | 0.08851 | 0.09748 | 0.08049 | 0.09706 | 0.07525 | |||
E3 | 0.2264 | 0.37738 | 0.381 | 0.37387 | 0.38445 | 0.3657 | ||
0.00099 | 0.02213 | 0.02322 | 0.0211 | 0.02427 | 0.01881 | |||
E4 | 0.54658 | 0.91107 | 0.93268 | 0.89108 | 0.92814 | 0.88287 | ||
0.00578 | 0.12897 | 0.14495 | 0.11502 | 0.14143 | 0.10965 |
Entropy | n | T1 | MLE | SE | LINEX | GE | ||
q=(−4) | q=(4) | q=(−4) | q=(4) | |||||
E1 | 150 | 2 | 1.43621 | 1.08769 | 1.11309 | 1.0634 | 1.10461 | 1.05858 |
0.38391 | 0.68731 | 0.73053 | 0.64734 | 0.71542 | 0.64047 | |||
E2 | 0.44669 | 0.74201 | 0.76687 | 0.71968 | 0.76569 | 0.70293 | ||
0.00752 | 0.08279 | 0.09771 | 0.07048 | 0.09677 | 0.06223 | |||
E3 | 0.22335 | 0.37101 | 0.37704 | 0.36528 | 0.38284 | 0.35146 | ||
0.00188 | 0.0207 | 0.02247 | 0.01909 | 0.02419 | 0.01556 | |||
E4 | 0.5392 | 0.89569 | 0.93237 | 0.86347 | 0.92427 | 0.84851 | ||
0.01096 | 0.12064 | 0.14747 | 0.09936 | 0.141 | 0.09068 | |||
E1 | 150 | 5 | 1.45913 | 1.11113 | 1.13795 | 1.08562 | 1.12859 | 1.08114 |
0.33642 | 0.63146 | 0.67869 | 0.58809 | 0.66129 | 0.58194 | |||
E2 | 0.44216 | 0.7227 | 0.74673 | 0.70098 | 0.74627 | 0.68352 | ||
0.00626 | 0.07177 | 0.08517 | 0.06067 | 0.08473 | 0.05273 | |||
E3 | 0.22108 | 0.36134 | 0.36719 | 0.35579 | 0.37313 | 0.34175 | ||
0.00157 | 0.01794 | 0.01953 | 0.01649 | 0.02118 | 0.01318 | |||
E4 | 0.53374 | 0.87237 | 0.90781 | 0.84102 | 0.90082 | 0.82508 | ||
0.00913 | 0.10457 | 0.12865 | 0.08539 | 0.12345 | 0.07684 | |||
E1 | 250 | 2 | 1.42519 | 1.08909 | 1.10364 | 1.07491 | 1.09892 | 1.07243 |
0.44944 | 0.68529 | 0.70973 | 0.66191 | 0.70155 | 0.65823 | |||
E2 | 0.46296 | 0.75583 | 0.77058 | 0.74198 | 0.76997 | 0.73241 | ||
0.00409 | 0.08983 | 0.0989 | 0.08172 | 0.09843 | 0.07648 | |||
E3 | 0.23148 | 0.37791 | 0.38154 | 0.3744 | 0.38498 | 0.3662 | ||
0.00102 | 0.02246 | 0.02356 | 0.02142 | 0.02461 | 0.01912 | |||
E4 | 0.55884 | 0.91237 | 0.93402 | 0.89231 | 0.92943 | 0.88409 | ||
0.00996 | 0.13089 | 0.14704 | 0.11679 | 0.14342 | 0.11143 | |||
E1 | 250 | 5 | 1.43915 | 1.08467 | 1.09935 | 1.07035 | 1.09464 | 1.06776 |
0.3813 | 0.67743 | 0.70194 | 0.65398 | 0.69382 | 0.65014 | |||
E2 | 0.44599 | 0.74805 | 0.76262 | 0.73437 | 0.76216 | 0.72469 | ||
0.0048 | 0.08519 | 0.09392 | 0.0774 | 0.09356 | 0.07223 | |||
E3 | 0.22299 | 0.37402 | 0.37761 | 0.37055 | 0.38108 | 0.36234 | ||
0.0012 | 0.0213 | 0.02236 | 0.0203 | 0.02339 | 0.01806 | |||
E4 | 0.53835 | 0.90297 | 0.92436 | 0.88316 | 0.92001 | 0.87478 | ||
0.007 | 0.12414 | 0.13968 | 0.11058 | 0.13633 | 0.10525 |
Entropy | n | T2 | MLE | SE | LINEX | GE | ||
q=(−4) | q=(4) | q=(−4) | q=(4) | |||||
E1 | 150 | 3 | 1.4405 | 1.10344 | 1.12943 | 1.07871 | 1.12043 | 1.07437 |
0.924 | 0.71613 | 0.76153 | 0.67439 | 0.745 | 0.66824 | |||
E2 | 0.17586 | 0.34206 | 0.34844 | 0.33572 | 0.35556 | 0.31302 | ||
0.00344 | 0.02604 | 0.02809 | 0.02408 | 0.03042 | 0.01859 | |||
E3 | 0.26378 | 0.5131 | 0.52749 | 0.49886 | 0.53337 | 0.46953 | ||
0.00774 | 0.0586 | 0.06561 | 0.05207 | 0.06846 | 0.04182 | |||
E4 | 0.3002 | 0.58394 | 0.60258 | 0.56552 | 0.607 | 0.53439 | ||
0.01002 | 0.07589 | 0.08627 | 0.06632 | 0.08866 | 0.05418 | |||
E1 | 150 | 7 | 1.44209 | 1.10927 | 1.13531 | 1.08448 | 1.12627 | 1.08014 |
0.79386, | 0.62524 | 0.67072 | 0.58337 | 0.65418 | 0.57719 | |||
E2 | 0.17903 | 0.34127 | 0.34767 | 0.33492 | 0.35482 | 0.31269 | ||
0.00275 | 0.02572 | 0.02778 | 0.02376 | 0.03012 | 0.01796 | |||
E3 | 0.26854 | 0.51191 | 0.52633 | 0.49764 | 0.53222 | 0.46903 | ||
0.00844 | 0.05787 | 0.0649 | 0.05134 | 0.06777 | 0.04041 | |||
E4 | 0.30562 | 0.58259 | 0.60129 | 0.56412 | 0.60571 | 0.53379 | ||
0.01093 | 0.07496 | 0.08536 | 0.06537 | 0.08778 | 0.05234 | |||
E1 | 250 | 3 | 1.42874 | 1.08905 | 1.10354 | 1.07494 | 1.09884 | 1.07249 |
0.55561 | 0.78152 | 0.77575 | 0.67835 | 0.79771 | 0.6946 | |||
E2 | 0.18196 | 0.3545 | 0.35827 | 0.35075 | 0.36231 | 0.34031 | ||
0.00272 | 0.02977 | 0.03107 | 0.0285 | 0.03248 | 0.02516 | |||
E3 | 0.27294 | 0.53175 | 0.54025 | 0.52332 | 0.54347 | 0.51047 | ||
0.00611 | 0.06697 | 0.0714 | 0.06272 | 0.07309 | 0.0566 | |||
E4 | 0.31062 | 0.60517 | 0.61618 | 0.59426 | 0.61851 | 0.58095 | ||
0.00792 | 0.08675 | 0.09329 | 0.08049 | 0.09466 | 0.07331 | |||
E1 | 250 | 7 | 1.43871 | 1.094 | 1.10877 | 1.07963 | 1.10393 | 1.07719 |
0.38096 | 0.6932 | 0.71814 | 0.66939 | 0.70972 | 0.66574 | |||
E2 | 0.17714 | 0.3506 | 0.35439 | 0.34684 | 0.35853 | 0.33616 | ||
0.00238 | 0.02842 | 0.0297 | 0.02718 | 0.03111 | 0.02385 | |||
E3 | 0.26571 | 0.5259 | 0.53443 | 0.51744 | 0.53779 | 0.50424 | ||
0.00535 | 0.06395 | 0.06829 | 0.05977 | 0.07001 | 0.05365 | |||
E4 | 0.3024 | 0.59852 | 0.60956 | 0.58756 | 0.61204 | 0.57386 | ||
0.00693 | 0.08282 | 0.08925 | 0.07669 | 0.09067 | 0.06949 |
Entropy | n | ACI | HPD | |||||
Interval | AL | CP | Interval | AL | CP | |||
E1 | 150 | r=120 | 1.32397(1.54377) | 0.2198 | 0.92 | 1.204 (1.8658) | 0.6618 | 0.9 |
E2 | 0.07005 (0.27138) | 0.20133 | 0.96 | 0.02202 (0.26484) | 0.24282 | 0.94 | ||
E3 | 0.10508 (0.40707) | 0.30199 | 0.96 | 0.03303 (0.39726) | 0.36423 | 0.94 | ||
E4 | 0.11958 (0.46327) | 0.34369 | 0.96 | 0.03759 (0.45212) | 0.41452 | 0.94 | ||
E1 | 150 | r=80 | 1.31453 (1.58589) | 0.27136 | 0.953 | 1.2048 (2.05354) | 0.84873 | 0.935 |
E2 | 0.04964 (0.29784) | 0.2482 | 0.97 | -0.02797 (0.27811) | 0.30608 | 0.96 | ||
E3 | 0.07447 (0.44676) | 0.3723 | 0.97 | -0.04195 (0.41717) | 0.45912 | 0.96 | ||
E4 | 0.08475 (0.50845) | 0.4237 | 0.97 | -0.04774 (0.47477) | 0.52251 | 0.96 | ||
E1 | 250 | r=200 | 1.33008 (1.50104) | 0.17096 | 0.912 | 1.24128 (1.73582) | 0.49454 | 0.89 |
E2 | 0.10495 (0.26035) | 0.1554 | 0.93 | 0.06898 (0.25542) | 0.18644 | 0.925 | ||
E3 | 0.15742 (0.39053) | 0.23311 | 0.93 | 0.10347 (0.38313) | 0.27967 | 0.925 | ||
E4 | 0.17916 (0.44445) | 0.26529 | 0.93 | 0.11776 (0.43603) | 0.31828 | 0.925 | ||
E1 | 250 | r=170 | 1.30827 (1.56216) | 0.25389 | 0.95 | 0.87087 (1.17569) | 0.30482 | 0.930 |
E2 | 0.0614 (0.29303) | 0.23163 | 0.97 | 0.36874 (0.5565) | 0.18775 | 0.942 | ||
E3 | 0.0921 (0.43955) | 0.34745 | 0.97 | 0.55311 (0.83474) | 0.28163 | 0.943 | ||
E4 | 0.10481 (0.50024) | 0.39543 | 0.97 | 0.62948(0.95) | 0.32051 | 0.942 | ||
E1 | 150 | T1=5 | 1.28738(1.55676) | 0.26939 | 0.952 | 0.88645 (1.33038) | 0.44393 | 0.92 |
E2 | 0.05928 (0.30422) | 0.24494 | 0.971 | 0.22668(0.4469) | 0.22022 | 0.91 | ||
E3 | 0.08892 (0.45633) | 0.36741 | 0.971 | 0.34002(0.67035) | 0.33033 | 0.912 | ||
E4 | 0.10119 (0.51934) | 0.41814 | 0.971 | 0.38697(0.76291) | 0.37594 | 0.912 | ||
E1 | 150 | T1=2 | 1.29124 (1.56016) | 0.26892 | 0.94 | 0.87814 (1.32003) | 0.44189 | 0.94 |
E2 | 0.04976 (0.29565) | 0.24589 | 0.98 | 0.22572 (0.44618) | 0.22046 | 0.95 | ||
E3 | 0.07464 (0.44347) | 0.36883 | 0.98 | 0.33858 (0.66927) | 0.3307 | 0.95 | ||
E4 | 0.08494 (0.5047) | 0.41976 | 0.98 | 0.38532 (0.76168) | 0.37636 | 0.95 | ||
E1 | 250 | T1=5 | 1.32001 (1.53028) | 0.21027 | 0.88 | 0.92047 (1.25225) | 0.33179 | 0.92 |
E2 | 0.08331 (0.2749) | 0.19158 | 0.92 | 0.26901 (0.43942) | 0.17041 | 0.92 | ||
E3 | 0.12497 (0.41235) | 0.28738 | 0.92 | 0.40351 (0.65913) | 0.25562 | 0.92 | ||
E4 | 0.14222 (0.46928) | 0.32705 | 0.92 | 0.45923(0.75014) | 0.29091 | 0.92 | ||
E1 | 250 | T1=2 | 1.32681 (1.53722) | 0.21041 | 0.931 | 0.91238 (1.24443) | 0.33205 | 0.94 |
E2 | 0.07314 (0.26599) | 0.19285 | 0.96 | 0.26679 (0.43719) | 0.1704 | 0.94 | ||
E3 | 0.10971 (0.39898) | 0.28927 | 0.96 | 0.40018 (0.65578) | 0.25561 | 0.94 | ||
E4 | 0.12486 (0.45407) | 0.32921 | 0.96 | 0.45543 (0.74633) | 0.2909 | 0.94 | ||
E1 | 150 | T2=7 | 1.30623 (1.57724) | 0.27101 | 0.93 | 0.88947 (1.3293) | 0.43983 | 0.92 |
E2 | 0.05067 (0.29834) | 0.24767 | 0.95 | 0.23143 (0.45226) | 0.22083 | 0.94 | ||
E3 | 0.076 (0.44751) | 0.37151 | 0.95 | 0.34715 (0.67839) | 0.33124 | 0.94 | ||
E4 | 0.0865 (0.5093) | 0.42281 | 0.95 | 0.39508 (0.77205) | 0.37697 | 0.94 | ||
E1 | 150 | T2=3 | 1.31687 (1.58794) | 0.27108 | 0.93 | 0.88647 (1.32694) | 0.44047 | 0.93 |
E2 | 0.03946 (0.28884) | 0.24937 | 0.94 | 0.22951 (0.45066) | 0.22115 | 0.92 | ||
E3 | 0.0592 (0.43326) | 0.37406 | 0.94 | 0.34427 (0.67599) | 0.33172 | 0.92 | ||
E4 | 0.06737 (0.49308) | 0.42571 | 0.94 | 0.39181 (0.76933) | 0.37752 | 0.92 | ||
E1 | 250 | T2=7 | 1.3256 (1.53593) | 0.21033 | 0.93 | 0.92428 (1.25566) | 0.33138 | 0.94 |
E2 | 0.08241 (0.27419) | 0.19178 | 0.95 | 0.26889 (0.43892) | 0.17003 | 0.951 | ||
E3 | 0.12362 (0.41129) | 0.28767 | 0.95 | 0.40334 (0.65838) | 0.25504 | 0.951 | ||
E4 | 0.14068 (0.46808) | 0.32739 | 0.95 | 0.45903(0.74928) | 0.29025 | 0.951 | ||
E1 | 250 | T2=3 | 1.33245 (1.54265) | 0.2102 | 0.952 | 0.92852 (1.26253) | 0.33402 | 0.972 |
E2 | 0.08078 (0.27268) | 0.1919 | 0.98 | 0.26694 (0.437) | 0.17005 | 0.973 | ||
E3 | 0.12118 (0.40902) | 0.28784 | 0.98 | 0.40042 (0.6555) | 0.25508 | 0.97 | ||
E4 | 0.13791 (0.4655) | 0.32759 | 0.98 | 0.4557 (0.746) | 0.2903 | 0.972 |
Entropy | n | ACI | HPD | |||||
Interval | AL | CP | Interval | AL | CP | |||
E1 | 150 | r=120 | 1.32451 (1.54522) | 0.22071 | 0.912 | 1.20353 (1.86439) | 0.66086 | 0.94 |
E2 | 0.31014 (0.58536) | 0.27522 | 0.93 | 0.26572 (0.58693) | 0.3212 | 0.952 | ||
E3 | 0.15507 (0.29268) | 0.13761 | 0.93 | 0.13286 (0.29346) | 0.1606 | 0.951 | ||
E4 | 0.37438 (0.7066) | 0.33222 | 0.93 | 0.32076 (0.70848) | 0.38773 | 0.952 | ||
E1 | 150 | r=80 | 1.30054 (1.59109) | 0.29055 | 0.92 | 1.18112 (2.09479) | 0.91367 | 0.94 |
E2 | 0.2672 (0.62936) | 0.36215 | 0.95 | 0.19976 (0.62205) | 0.42229 | 0.96 | ||
E3 | 0.1336(0.31468) | 0.18108 | 0.95 | 0.09988 (0.31103) | 0.21114 | 0.96 | ||
E4 | 0.32254 (0.7597) | 0.43716 | 0.95 | 0.24114 (0.75088) | 0.50975 | 0.960 | ||
E1 | 250 | r=200 | 1.32132 (1.53199) | 0.21067 | 0.90 | 0.91591 (1.24612) | 0.33021 | 0.938 |
E2 | 0.32109 (0.58451) | 0.26342 | 0.96 | 0.60057 (0.93122) | 0.33065 | 0.941 | ||
E3 | 0.16055 (0.29225) | 0.13171 | 0.96 | 0.30029 (0.46561) | 0.16532 | 0.94 | ||
E4 | 0.38759 (0.70556) | 0.31797 | 0.96 | 0.72496 (1.12409) | 0.39913 | 0.941 | ||
E1 | 250 | r=170 | 1.31742 (1.57116) | 0.25374 | 0.951 | 0.86563 (1.17167) | 0.30604 | 0.95 |
E2 | 0.28194 (0.59672) | 0.31479 | 0.98 | 0.78845 (1.24102) | 0.45257 | 0.95 | ||
E3 | 0.14097 (0.29836) | 0.15739 | 0.98 | 0.39422(0.62051) | 0.22629 | 0.951 | ||
E4 | 0.34033 (0.72031) | 0.37998 | 0.98 | 0.95174 (1.49804) | 0.5463 | 0.951 | ||
E1 | 150 | T1=5 | 1.32346(1.5948) | 0.27135 | 0.292 | 0.89618 (1.34279) | 0.44661 | 0.90 |
E2 | 0.27375 (0.61058) | 0.33683 | 0.95 | 0.53076 (0.94919) | 0.41843 | 0.94 | ||
E3 | 0.13687(0.30529) | 0.16842 | 0.95 | 0.26536(0.47461) | 0.20924 | 0.94 | ||
E4 | 0.33044 (0.73704) | 0.40659 | 0.95 | 0.64065 (1.14576) | 0.5051 | 0.94 | ||
E1 | 150 | T1=3 | 1.30039 (1.57202) | 0.27163 | 0.891 | 0.87685 (1.31329) | 0.43644 | 0.88 |
E2 | 0.27726 (0.61612) | 0.33886 | 0.94 | 0.54827 (0.9726) | 0.42433 | 0.93 | ||
E3 | 0.13863 (0.30806) | 0.16943 | 0.94 | 0.27414 (0.4863) | 0.21217 | 0.93 | ||
E4 | 0.33468 (0.74373) | 0.40905 | 0.94 | 0.66182 (1.17403) | 0.51221 | 0.93 | ||
E1 | 250 | T1=5 | 1.31995 (1.53043) | 0.21048 | 0.94 | 0.92732 (1.25933) | 0.33201 | 0.97 |
E2 | 0.33064 (0.59527) | 0.26462 | 0.97 | 0.60102 (0.93213) | 0.33111 | 0.96 | ||
E3 | 0.16532 (0.29763) | 0.13231 | 0.97 | 0.30051 (0.46607) | 0.16556 | 0.96 | ||
E4 | 0.39912 (0.71855) | 0.31943 | 0.97 | 0.72549 (1.12518) | 0.39969 | 0.96 | ||
E1 | 250 | T1=2 | 1.33397 (1.54433) | 0.21036 | 0.88 | 0.92234 (1.25581) | 0.33347 | 0.89 |
E2 | 0.31502 (0.57695) | 0.26194 | 0.91 | 0.59443 (0.92381) | 0.32939 | 0.92 | ||
E3 | 0.15751 (0.28848) | 0.13097 | 0.91 | 0.29721 (0.46191) | 0.16469 | 0.92 | ||
E4 | 0.38026 (0.69645) | 0.31619 | 0.91 | 0.71753 (1.11514) | 0.3976 | 0.92 | ||
E1 | 150 | T2=7 | 1.30473 (1.57627) | 0.27154 | 0.93 | 0.89139 (1.33151) | 0.44012 | 0.94 |
E2 | 0.05189 (0.29982) | 0.24793 | 0.96 | 0.23277 (0.45373) | 0.22096 | 0.95 | ||
E3 | 0.07784 (0.44973) | 0.37189 | 0.96 | 0.34912 (0.68059) | 0.33146 | 0.95 | ||
E4 | 0.08858 (0.51183) | 0.42324 | 0.96 | 0.39734 (0.77451) | 0.37717 | 0.95 | ||
E1 | 150 | T2=3 | 1.30623 (1.57796) | 0.27172 | 0.94 | 0.89652 (1.33842) | 0.44189 | 0.93 |
E2 | 0.05519 (0.30286) | 0.24767 | 0.97 | 0.23189 (0.45337) | 0.22149 | 0.94 | ||
E3 | 0.08279 (0.45429) | 0.3715 | 0.97 | 0.34783 (0.68006) | 0.33223 | 0.94 | ||
E4 | 0.09422 (0.51701) | 0.42279 | 0.97 | 0.39585 (0.77396) | 0.37811 | 0.94 | ||
E1 | 250 | T2=7 | 1.32349 (1.53398) | 0.21048 | 0.92 | 0.92772 (1.25923) | 0.33151 | 0.956 |
E2 | 0.08622 (0.2777) | 0.19149 | 0.96 | 0.27025 (0.44047) | 0.17022 | 0.981 | ||
E3 | 0.12932 (0.41655) | 0.28723 | 0.96 | 0.40537 (0.6607) | 0.25533 | 0.981 | ||
E4 | 0.14718 (0.47407) | 0.32689 | 0.96 | 0.46134 (0.75193) | 0.29058 | 0.951 | ||
E1 | 250 | T2=3 | 1.33356 (1.54387) | 0.21031 | 0.91 | 0.93109 (1.26572) | 0.33463 | 0.899 |
E2 | 0.08117 (0.27311) | 0.19194 | 0.945 | 0.26625 (0.43669) | 0.17044 | 0.91 | ||
E3 | 0.12176 (0.40967) | 0.28791 | 0.945 | 0.39937 (0.65503) | 0.25566 | 0.912 | ||
E4 | 0.13857 (0.46623) | 0.32766 | 0.945 | 0.45451 (0.74547) | 0.29096 | 0.912 |
Entropy | MLE | SE | LINEX | GE | |||
q=(4) | q=(−4) | q=(4) | q=(−4) | ||||
E1 | 0.87621 | 0.80134 | 0.81222 | 0.79066 | 0.81134 | 0.78419 | |
E2 | Case 1 | 0.43147 | 0.49738 | 0.50214 | 0.49264 | 0.50444 | 0.48498 |
E3 | 0.64721 | 0.74759 | 0.75809 | 0.7372 | 0.75794 | 0.72962 | |
E4 | 0.73657 | 0.85118 | 0.86477 | 0.83793 | 0.8629 | 0.83111 | |
E1 | 0.8699 | 0.74041 | 0.75034 | 0.73028 | 0.75034 | 0.72235 | |
E2 | Case 2 | 0.32401 | 0.43615 | 0.4409 | 0.43133 | 0.44414 | 0.42118 |
E3 | 0.48601 | 0.65422 | 0.66488 | 0.64335 | 0.66621 | 0.63178 | |
E4 | 0.55312 | 0.74455 | 0.75835 | 0.73046 | 0.7582 | 0.71901 | |
E1 | 0.77377 | 0.61321 | 0.6271 | 0.5993 | 0.62968 | 0.58181 | |
E2 | Case 3 | 0.32039 | 0.37454 | 0.38353 | 0.36519 | 0.39153 | 0.3149 |
E3 | 0.48059 | 0.56181 | 0.58189 | 0.54052 | 0.5873 | 0.47235 | |
E4 | 0.54695 | 0.63938 | 0.6653 | 0.61165 | 0.66839 | 0.53757 |
n | r | T1 | T2 |
250 | 200 | (2, 5) | 7 |
150 | 120 | (2, 5) | 7 |
250 | (200,170) | 0.2 | 1.2 |
150 | (120, 80) | 0.2 | 1.2 |
250 | 200 | 1.5 | (3, 7) |
150 | 120 | 1.5 | (3, 7) |
Entropy | n | r | MLE | SE | LINEX | GE | ||
q=(−4) | q=(4) | q=(−4) | q=(4) | |||||
E1 | 150 | 120 | 1.36712 | 1.51529 | 1.57698 | 1.4603 | 1.54341 | 1.46821 |
0.60911 | 0.61993 | 0.78749 | 0.47828 | 0.69251 | 0.5022 | |||
E2 | 0.19348 | 0.14388 | 0.15158 | 0.13615 | 0.17786 | 0.01169 | ||
0.0026 | 0.00428 | 0.00363 | 0.00507 | 0.00157 | 0.03013 | |||
E3 | 0.29022 | 0.21582 | 0.23314 | 0.19842 | 0.26679 | 0.01753 | ||
0.0059 | 0.00964 | 0.00754 | 0.01239 | 0.00353 | 0.0678 | |||
E4 | 0.33029 | 0.24562 | 0.26804 | 0.22307 | 0.30362 | 0.01995 | ||
0.0077 | 0.01249 | 0.00946 | 0.0166 | 0.00457 | 0.08781 | |||
E1 | 150 | 80 | 1.45558 | 1.59925 | 1.70715 | 1.51007 | 1.64329 | 1.52503 |
.43429 | 0.88746 | 1.21708 | 0.63845 | 1.01106 | 0.68956 | |||
E2 | 0.14164 | 0.12597 | 0.13823 | 0.1136 | 0.18205 | 0.00373 | ||
0.00183 | 0.00796 | 0.00644 | 0.00983 | 0.00175 | 0.03244 | |||
E3 | 0.21247 | 0.18895 | 0.2165 | 0.16107 | 0.27307 | 0.00559 | ||
0.0041 | 0.01791 | 0.01305 | 0.02451 | 0.00394 | 0.07299 | |||
E4 | 0.2418 | 0.21504 | 0.2507 | 0.17889 | 0.31078 | 0.00636 | ||
0.00532 | 0.0232 | 0.0162 | 0.03311 | 0.0051 | 0.09454 | |||
E1 | 250 | 200 | 1.41556 | 1.47743 | 1.51066 | 1.44628 | 1.49357 | 1.45048 |
0.42562 | 0.49233 | 0.57613 | 0.41596 | 0.53207 | 0.42718 | |||
E2 | 0.18265 | 0.16257 | 0.1671 | 0.15803 | 0.1816 | 0.05219 | ||
0.0188 | 0.0166 | 0.0147 | 0.019 | 0.0093 | 0.02149 | |||
E3 | 0.27397 | 0.24386 | 0.25404 | 0.23364 | 0.2724 | 0.07828 | ||
0.0423 | 0.0374 | 0.0312 | 0.0457 | 0.021 | 0.04836 | |||
E4 | 0.3118 | 0.27753 | 0.29072 | 0.26429 | 0.31001 | 0.08909 | ||
0.00547 | 0.0484 | 0.0395 | 0.0609 | 0.0272 | 0.06263 | |||
E1 | 250 | 170 | 1.43521 | 1.02243 | 1.03456 | 1.01037 | 1.03126 | 1.00721 |
0.7071 | 0.57443 | 0.59289 | 0.55637 | 0.58775 | 0.55186 | |||
E2 | 0.17722 | 0.46139 | 0.46597 | 0.45683 | 0.46873 | 0.44847 | ||
0.00349 | 0.0779 | 0.08047 | 0.07539 | 0.08202 | 0.07092 | |||
E3 | 0.26582 | 0.69208 | 0.70242 | 0.68185 | 0.7031 | 0.67271 | ||
0.00784 | 0.17527 | 0.184 | 0.16684 | 0.18454 | 0.15957 | |||
E4 | 0.30253 | 0.78764 | 0.80104 | 0.77439 | 0.80017 | 0.76559 | ||
0.01016 | 0.22701 | 0.23991 | 0.21462 | 0.23902 | 0.20668 |
Entropy | n | T1 | MLE | SE | LINEX | GE | ||
q=(−4) | q=(4) | q=(−4) | q=(4) | |||||
E1 | 150 | 2 | 1.42207 | 1.10074 | 1.12712 | 1.07561 | 1.11805 | 1.07101 |
0.74852 | 0.71389 | 0.75988 | 0.67162 | 0.74317 | 0.66525 | |||
E2 | 0.18175 | 0.33537 | 0.3417 | 0.32909 | 0.34903 | 0.304 | ||
0.00373 | 0.02404 | 0.02599 | 0.02218 | 0.02829 | 0.01683 | |||
E3 | 0.27262 | 0.50306 | 0.51733 | 0.48895 | 0.52355, | 0.45599 | ||
0.00838 | 0.0541 | 0.06077 | 0.0479 | 0.06366 | 0.03786 | |||
E4 | 0.31026 | 0.57252 | 0.59102 | 0.55425 | 0.59583 | 0.51895 | ||
0.01086 | 0.07006 | 0.07995 | 0.06098 | 0.08245 | 0.04904 | |||
E1 | 150 | 5 | 1.4257 | 1.09124 | 1.11732 | 1.06638 | 1.10857 | 1.06145 |
0.35491 | 0.696 | 0.74062 | 0.65491 | 0.72485 | 0.64805 | |||
E2 | 0.1727 | 0.33467 | 0.34102 | 0.32838 | 0.34835 | 0.30641 | ||
0.00347 | 0.02359 | 0.02554 | 0.02173 | 0.02785 | 0.01618 | |||
E3 | 0.25905 | 0.50201 | 0.51631 | 0.48787 | 0.52252 | 0.45961 | ||
0.0078 | 0.05307 | 0.05974 | 0.04687 | 0.06266 | 0.0364 | |||
E4 | 0.29482 | 0.57132 | 0.58986 | 0.55302 | 0.59467 | 0.52307 | ||
0.01011 | 0.06874 | 0.07862 | 0.05965 | 0.08116 | 0.04714 | |||
E1 | 250 | 2 | 1.42515 | 1.08207 | 1.09661 | 1.06792 | 1.09196 | 1.06535 |
0.5497 | 0.67419 | 0.69842 | 0.65104 | 0.6904 | 0.64724 | |||
E2 | 0.1791 | 0.35346 | 0.35724 | 0.34969 | 0.36132 | 0.33912 | ||
0.00256 | 0.02935 | 0.03065 | 0.02808 | 0.03207 | 0.02472 | |||
E3 | 0.26866 | 0.53018 | 0.53871 | 0.52171 | 0.54198 | 0.50868 | ||
0.00575 | 0.06603 | 0.07045 | 0.06178 | 0.07215 | 0.05562 | |||
E4 | 0.30575 | 0.60339 | 0.61444 | 0.59241 | 0.61682 | 0.57892 | ||
0.00745 | 0.08552 | 0.09206 | 0.07928 | 0.09345 | 0.07204 | |||
E1 | 250 | 5 | 1.43201 | 1.074 | 1.08855 | 1.05981 | 1.08398 | 1.0570 |
0.36655 | 0.66264 | 0.68669 | 0.63962 | 0.67882 | 0.63567 | |||
E2 | 0.16956 | 0.35109 | 0.35487 | 0.34733 | 0.35901 | 0.33668 | ||
0.00258 | 0.02859 | 0.02987 | 0.02734 | 0.03129 | 0.02401 | |||
E3 | 0.25435 | 0.52664 | 0.53516 | 0.51817 | 0.53851 | 0.50502 | ||
0.00581 | 0.06432 | 0.06868 | 0.06014 | 0.07039 | 0.05403 | |||
E4 | 0.28946 | 0.59935 | 0.6104 | 0.58839 | 0.61286 | 0.57474 | ||
0.00752 | 0.08331 | 0.08975 | 0.07717 | 0.09117 | 0.06998 |
Entropy | n | T2 | MLE | SE | LINEX | GE | ||
q=(−4) | q=(4) | q=(−4) | q=(4) | |||||
E1 | 150 | 3 | 1.44174 | 1.10164 | 1.12749 | 1.07697 | 1.11865 | 1.07244 |
0.69463 | 0.71086 | 0.7555 | 0.66966 | 0.73956 | 0.66315 | |||
E2 | 0.17451 | 0.34042 | 0.34679 | 0.33409 | 0.35394 | 0.31117 | ||
0.00411 | 0.0254 | 0.02744 | 0.02346 | 0.02978 | 0.01788 | |||
E3 | 0.26176 | 0.51063 | 0.525 | 0.49642 | 0.53092 | 0.46676 | ||
0.00925 | 0.05716 | 0.06412 | 0.05069 | 0.067 | 0.04023 | |||
E4 | 0.2979 | 0.58113 | 0.59976 | 0.56274 | 0.60422 | 0.5312 | ||
0.01198 | 0.07404 | 0.08435 | 0.06454 | 0.08678 | 0.05211 | |||
E1 | 150 | 7 | 1.45241 | 1.09892 | 1.12479 | 1.07423 | 1.116 | 1.06958 |
0.41651 | 0.70499 | 0.74941 | 0.664 | 0.73368 | 0.65728 | |||
E2 | 0.16415 | 0.3389 | 0.34527 | 0.33257 | 0.35247 | 0.31123 | ||
0.0043 | 0.02485 | 0.02687 | 0.02293 | 0.02921 | 0.01722 | |||
E3 | 0.24623 | 0.50835 | 0.52272 | 0.49412 | 0.5287 | 0.46684 | ||
0.00967 | 0.05592 | 0.06282 | 0.0495 | 0.06572 | 0.03875 | |||
E4 | 0.28022 | 0.57853 | 0.59716 | 0.56012 | 0.6017 | 0.5313 | ||
0.01253 | 0.07243 | 0.08265 | 0.0630 | 0.08512 | 0.05019 | |||
E1 | 250 | 3 | 1.43076 | 1.0857 | 1.10019 | 1.07159 | 1.09552 | 1.06909 |
0.36052 | 0.67711 | 0.70126 | 0.654 | 0.69327 | 0.65021 | |||
E2 | 0.1783 | 0.35307 | 0.35684 | 0.34933 | 0.36091 | 0.33882 | ||
0.00213 | 0.02927 | 0.03056 | 0.02801 | 0.03197 | 0.02468 | |||
E3 | 0.26745 | 0.52961 | 0.5381 | 0.52119 | 0.54137 | 0.50823 | ||
0.00479 | 0.06585 | 0.07024 | 0.06164 | 0.07194 | 0.05553 | |||
E4 | 0.30438 | 0.60274 | 0.61373 | 0.59183 | 0.61612 | 0.5784 | ||
0.00621 | 0.0853 | 0.09178 | 0.0791 | 0.09317 | 0.07192 | |||
E1 | 250 | 7 | 1.43755 | 1.09109 | 1.10581 | 1.07676 | 1.10102 | 1.07429 |
0.35688 | 0.68555 | 0.71023 | 0.66196 | 0.702 | 0.6582 | |||
E2 | 0.17673 | 0.35121 | 0.35498 | 0.34746 | 0.35909 | 0.33684 | ||
0.00222 | 0.02865 | 0.02993 | 0.02741 | 0.03134 | 0.02408 | |||
E3 | 0.2651 | 0.52681 | 0.53531 | 0.51838 | 0.53864 | 0.50526 | ||
0.00499 | 0.06447 | 0.06882 | 0.0603 | 0.07052 | 0.05418 | |||
E4 | 0.3017 | 0.59955 | 0.61056 | 0.58863 | 0.61301 | 0.57502 | ||
0.00646 | 0.0835 | 0.08993 | 0.07737 | 0.09134 | 0.07018 |
Entropy | n | r | MLE | SE | LINEX | GE | ||
q=(−4) | q=(4) | q=(−4) | q=(4) | |||||
E1 | 150 | 80 | 1.44582 | 1.603 | 1.73021 | 1.50082 | 1.65364 | 1.51755 |
0.40585 | 0.92552 | 1.32941 | 0.63469 | 1.06945 | 0.69625 | |||
E2 | 0.44828 | 0.39371 | 0.41826 | 0.37158 | 0.43654 | 0.25012 | ||
0.00698 | 0.01149 | 0.00913 | 0.01474 | 0.00655 | 0.06768 | |||
E3 | 0.22414 | 0.19686 | 0.20282 | 0.19119 | 0.21827 | 0.12506 | ||
0.00174 | 0.00287 | 0.00255 | 0.00326 | 0.00164 | 0.01692 | |||
E4 | 0.54112 | 0.47525 | 0.51149 | 0.4433 | 0.52695 | 0.30192 | ||
0.01017 | 0.01675 | 0.0128 | 0.02258 | 0.00955 | 0.09861 | |||
E1 | 150 | 120 | 1.43487 | 1.5157 | 1.57724 | 1.46066 | 1.54378 | 1.46853 |
0.37627 | 0.61997 | 0.78671 | 0.47843 | 0.69239 | 0.50217 | |||
E2 | 0.44775 | 0.41609 | 0.42997 | 0.40302 | 0.43997 | 0.36094 | ||
0.00522 | 0.00589 | 0.00495 | 0.00714 | 0.00406 | 0.01896 | |||
E3 | 0.22388 | 0.20805 | 0.21146 | 0.20473 | 0.21998 | 0.18047 | ||
0.00131 | 0.00147 | 0.00134 | 0.00162 | 0.00101 | 0.00474 | |||
E4 | 0.54049 | 0.50227 | 0.52262 | 0.48332 | 0.53109 | 0.43569 | ||
0.00761 | 0.00859 | 0.00699 | 0.01083 | 0.00591 | 0.02762 | |||
E1 | 250 | 170 | 1.44429 | 1.01809 | 1.03028 | 1.00595 | 1.027 | 1.0027 |
0.39576 | 0.5683 | 0.58679 | 0.5502 | 0.58168 | 0.5456 | |||
E2 | 0.43933 | 0.99617 | 1.02445 | 0.97078 | 1.0163 | 0.96342 | ||
0.00699 | 0.29079 | 0.32224 | 0.26396 | 0.31289 | 0.2566 | |||
E3 | 0.21966 | 0.49809 | 0.50495 | 0.49158 | 0.50815 | 0.48171 | ||
0.00175 | 0.0727 | 0.07646 | 0.06922 | 0.07822 | 0.06415 | |||
E4 | 0.53032 | 1.20248 | 1.24422 | 1.16586 | 1.22679 | 1.16296 | ||
0.01019 | 0.42372 | 0.48007 | 0.37722 | 0.45592 | 0.3739 | |||
E1 | 250 | 200 | 1.42666 | 1.07694 | 1.09132 | 1.06292 | 1.08678 | 1.06027 |
0.5947 | 0.46296 | 0.48666 | 0.44027 | 0.47897 | 0.43631 | |||
E2 | 0.4528 | 0.75476 | 0.76948 | 0.74095 | 0.76889 | 0.73139 | ||
0.00397 | 0.08851 | 0.09748 | 0.08049 | 0.09706 | 0.07525 | |||
E3 | 0.2264 | 0.37738 | 0.381 | 0.37387 | 0.38445 | 0.3657 | ||
0.00099 | 0.02213 | 0.02322 | 0.0211 | 0.02427 | 0.01881 | |||
E4 | 0.54658 | 0.91107 | 0.93268 | 0.89108 | 0.92814 | 0.88287 | ||
0.00578 | 0.12897 | 0.14495 | 0.11502 | 0.14143 | 0.10965 |
Entropy | n | T1 | MLE | SE | LINEX | GE | ||
q=(−4) | q=(4) | q=(−4) | q=(4) | |||||
E1 | 150 | 2 | 1.43621 | 1.08769 | 1.11309 | 1.0634 | 1.10461 | 1.05858 |
0.38391 | 0.68731 | 0.73053 | 0.64734 | 0.71542 | 0.64047 | |||
E2 | 0.44669 | 0.74201 | 0.76687 | 0.71968 | 0.76569 | 0.70293 | ||
0.00752 | 0.08279 | 0.09771 | 0.07048 | 0.09677 | 0.06223 | |||
E3 | 0.22335 | 0.37101 | 0.37704 | 0.36528 | 0.38284 | 0.35146 | ||
0.00188 | 0.0207 | 0.02247 | 0.01909 | 0.02419 | 0.01556 | |||
E4 | 0.5392 | 0.89569 | 0.93237 | 0.86347 | 0.92427 | 0.84851 | ||
0.01096 | 0.12064 | 0.14747 | 0.09936 | 0.141 | 0.09068 | |||
E1 | 150 | 5 | 1.45913 | 1.11113 | 1.13795 | 1.08562 | 1.12859 | 1.08114 |
0.33642 | 0.63146 | 0.67869 | 0.58809 | 0.66129 | 0.58194 | |||
E2 | 0.44216 | 0.7227 | 0.74673 | 0.70098 | 0.74627 | 0.68352 | ||
0.00626 | 0.07177 | 0.08517 | 0.06067 | 0.08473 | 0.05273 | |||
E3 | 0.22108 | 0.36134 | 0.36719 | 0.35579 | 0.37313 | 0.34175 | ||
0.00157 | 0.01794 | 0.01953 | 0.01649 | 0.02118 | 0.01318 | |||
E4 | 0.53374 | 0.87237 | 0.90781 | 0.84102 | 0.90082 | 0.82508 | ||
0.00913 | 0.10457 | 0.12865 | 0.08539 | 0.12345 | 0.07684 | |||
E1 | 250 | 2 | 1.42519 | 1.08909 | 1.10364 | 1.07491 | 1.09892 | 1.07243 |
0.44944 | 0.68529 | 0.70973 | 0.66191 | 0.70155 | 0.65823 | |||
E2 | 0.46296 | 0.75583 | 0.77058 | 0.74198 | 0.76997 | 0.73241 | ||
0.00409 | 0.08983 | 0.0989 | 0.08172 | 0.09843 | 0.07648 | |||
E3 | 0.23148 | 0.37791 | 0.38154 | 0.3744 | 0.38498 | 0.3662 | ||
0.00102 | 0.02246 | 0.02356 | 0.02142 | 0.02461 | 0.01912 | |||
E4 | 0.55884 | 0.91237 | 0.93402 | 0.89231 | 0.92943 | 0.88409 | ||
0.00996 | 0.13089 | 0.14704 | 0.11679 | 0.14342 | 0.11143 | |||
E1 | 250 | 5 | 1.43915 | 1.08467 | 1.09935 | 1.07035 | 1.09464 | 1.06776 |
0.3813 | 0.67743 | 0.70194 | 0.65398 | 0.69382 | 0.65014 | |||
E2 | 0.44599 | 0.74805 | 0.76262 | 0.73437 | 0.76216 | 0.72469 | ||
0.0048 | 0.08519 | 0.09392 | 0.0774 | 0.09356 | 0.07223 | |||
E3 | 0.22299 | 0.37402 | 0.37761 | 0.37055 | 0.38108 | 0.36234 | ||
0.0012 | 0.0213 | 0.02236 | 0.0203 | 0.02339 | 0.01806 | |||
E4 | 0.53835 | 0.90297 | 0.92436 | 0.88316 | 0.92001 | 0.87478 | ||
0.007 | 0.12414 | 0.13968 | 0.11058 | 0.13633 | 0.10525 |
Entropy | n | T2 | MLE | SE | LINEX | GE | ||
q=(−4) | q=(4) | q=(−4) | q=(4) | |||||
E1 | 150 | 3 | 1.4405 | 1.10344 | 1.12943 | 1.07871 | 1.12043 | 1.07437 |
0.924 | 0.71613 | 0.76153 | 0.67439 | 0.745 | 0.66824 | |||
E2 | 0.17586 | 0.34206 | 0.34844 | 0.33572 | 0.35556 | 0.31302 | ||
0.00344 | 0.02604 | 0.02809 | 0.02408 | 0.03042 | 0.01859 | |||
E3 | 0.26378 | 0.5131 | 0.52749 | 0.49886 | 0.53337 | 0.46953 | ||
0.00774 | 0.0586 | 0.06561 | 0.05207 | 0.06846 | 0.04182 | |||
E4 | 0.3002 | 0.58394 | 0.60258 | 0.56552 | 0.607 | 0.53439 | ||
0.01002 | 0.07589 | 0.08627 | 0.06632 | 0.08866 | 0.05418 | |||
E1 | 150 | 7 | 1.44209 | 1.10927 | 1.13531 | 1.08448 | 1.12627 | 1.08014 |
0.79386, | 0.62524 | 0.67072 | 0.58337 | 0.65418 | 0.57719 | |||
E2 | 0.17903 | 0.34127 | 0.34767 | 0.33492 | 0.35482 | 0.31269 | ||
0.00275 | 0.02572 | 0.02778 | 0.02376 | 0.03012 | 0.01796 | |||
E3 | 0.26854 | 0.51191 | 0.52633 | 0.49764 | 0.53222 | 0.46903 | ||
0.00844 | 0.05787 | 0.0649 | 0.05134 | 0.06777 | 0.04041 | |||
E4 | 0.30562 | 0.58259 | 0.60129 | 0.56412 | 0.60571 | 0.53379 | ||
0.01093 | 0.07496 | 0.08536 | 0.06537 | 0.08778 | 0.05234 | |||
E1 | 250 | 3 | 1.42874 | 1.08905 | 1.10354 | 1.07494 | 1.09884 | 1.07249 |
0.55561 | 0.78152 | 0.77575 | 0.67835 | 0.79771 | 0.6946 | |||
E2 | 0.18196 | 0.3545 | 0.35827 | 0.35075 | 0.36231 | 0.34031 | ||
0.00272 | 0.02977 | 0.03107 | 0.0285 | 0.03248 | 0.02516 | |||
E3 | 0.27294 | 0.53175 | 0.54025 | 0.52332 | 0.54347 | 0.51047 | ||
0.00611 | 0.06697 | 0.0714 | 0.06272 | 0.07309 | 0.0566 | |||
E4 | 0.31062 | 0.60517 | 0.61618 | 0.59426 | 0.61851 | 0.58095 | ||
0.00792 | 0.08675 | 0.09329 | 0.08049 | 0.09466 | 0.07331 | |||
E1 | 250 | 7 | 1.43871 | 1.094 | 1.10877 | 1.07963 | 1.10393 | 1.07719 |
0.38096 | 0.6932 | 0.71814 | 0.66939 | 0.70972 | 0.66574 | |||
E2 | 0.17714 | 0.3506 | 0.35439 | 0.34684 | 0.35853 | 0.33616 | ||
0.00238 | 0.02842 | 0.0297 | 0.02718 | 0.03111 | 0.02385 | |||
E3 | 0.26571 | 0.5259 | 0.53443 | 0.51744 | 0.53779 | 0.50424 | ||
0.00535 | 0.06395 | 0.06829 | 0.05977 | 0.07001 | 0.05365 | |||
E4 | 0.3024 | 0.59852 | 0.60956 | 0.58756 | 0.61204 | 0.57386 | ||
0.00693 | 0.08282 | 0.08925 | 0.07669 | 0.09067 | 0.06949 |
Entropy | n | ACI | HPD | |||||
Interval | AL | CP | Interval | AL | CP | |||
E1 | 150 | r=120 | 1.32397(1.54377) | 0.2198 | 0.92 | 1.204 (1.8658) | 0.6618 | 0.9 |
E2 | 0.07005 (0.27138) | 0.20133 | 0.96 | 0.02202 (0.26484) | 0.24282 | 0.94 | ||
E3 | 0.10508 (0.40707) | 0.30199 | 0.96 | 0.03303 (0.39726) | 0.36423 | 0.94 | ||
E4 | 0.11958 (0.46327) | 0.34369 | 0.96 | 0.03759 (0.45212) | 0.41452 | 0.94 | ||
E1 | 150 | r=80 | 1.31453 (1.58589) | 0.27136 | 0.953 | 1.2048 (2.05354) | 0.84873 | 0.935 |
E2 | 0.04964 (0.29784) | 0.2482 | 0.97 | -0.02797 (0.27811) | 0.30608 | 0.96 | ||
E3 | 0.07447 (0.44676) | 0.3723 | 0.97 | -0.04195 (0.41717) | 0.45912 | 0.96 | ||
E4 | 0.08475 (0.50845) | 0.4237 | 0.97 | -0.04774 (0.47477) | 0.52251 | 0.96 | ||
E1 | 250 | r=200 | 1.33008 (1.50104) | 0.17096 | 0.912 | 1.24128 (1.73582) | 0.49454 | 0.89 |
E2 | 0.10495 (0.26035) | 0.1554 | 0.93 | 0.06898 (0.25542) | 0.18644 | 0.925 | ||
E3 | 0.15742 (0.39053) | 0.23311 | 0.93 | 0.10347 (0.38313) | 0.27967 | 0.925 | ||
E4 | 0.17916 (0.44445) | 0.26529 | 0.93 | 0.11776 (0.43603) | 0.31828 | 0.925 | ||
E1 | 250 | r=170 | 1.30827 (1.56216) | 0.25389 | 0.95 | 0.87087 (1.17569) | 0.30482 | 0.930 |
E2 | 0.0614 (0.29303) | 0.23163 | 0.97 | 0.36874 (0.5565) | 0.18775 | 0.942 | ||
E3 | 0.0921 (0.43955) | 0.34745 | 0.97 | 0.55311 (0.83474) | 0.28163 | 0.943 | ||
E4 | 0.10481 (0.50024) | 0.39543 | 0.97 | 0.62948(0.95) | 0.32051 | 0.942 | ||
E1 | 150 | T1=5 | 1.28738(1.55676) | 0.26939 | 0.952 | 0.88645 (1.33038) | 0.44393 | 0.92 |
E2 | 0.05928 (0.30422) | 0.24494 | 0.971 | 0.22668(0.4469) | 0.22022 | 0.91 | ||
E3 | 0.08892 (0.45633) | 0.36741 | 0.971 | 0.34002(0.67035) | 0.33033 | 0.912 | ||
E4 | 0.10119 (0.51934) | 0.41814 | 0.971 | 0.38697(0.76291) | 0.37594 | 0.912 | ||
E1 | 150 | T1=2 | 1.29124 (1.56016) | 0.26892 | 0.94 | 0.87814 (1.32003) | 0.44189 | 0.94 |
E2 | 0.04976 (0.29565) | 0.24589 | 0.98 | 0.22572 (0.44618) | 0.22046 | 0.95 | ||
E3 | 0.07464 (0.44347) | 0.36883 | 0.98 | 0.33858 (0.66927) | 0.3307 | 0.95 | ||
E4 | 0.08494 (0.5047) | 0.41976 | 0.98 | 0.38532 (0.76168) | 0.37636 | 0.95 | ||
E1 | 250 | T1=5 | 1.32001 (1.53028) | 0.21027 | 0.88 | 0.92047 (1.25225) | 0.33179 | 0.92 |
E2 | 0.08331 (0.2749) | 0.19158 | 0.92 | 0.26901 (0.43942) | 0.17041 | 0.92 | ||
E3 | 0.12497 (0.41235) | 0.28738 | 0.92 | 0.40351 (0.65913) | 0.25562 | 0.92 | ||
E4 | 0.14222 (0.46928) | 0.32705 | 0.92 | 0.45923(0.75014) | 0.29091 | 0.92 | ||
E1 | 250 | T1=2 | 1.32681 (1.53722) | 0.21041 | 0.931 | 0.91238 (1.24443) | 0.33205 | 0.94 |
E2 | 0.07314 (0.26599) | 0.19285 | 0.96 | 0.26679 (0.43719) | 0.1704 | 0.94 | ||
E3 | 0.10971 (0.39898) | 0.28927 | 0.96 | 0.40018 (0.65578) | 0.25561 | 0.94 | ||
E4 | 0.12486 (0.45407) | 0.32921 | 0.96 | 0.45543 (0.74633) | 0.2909 | 0.94 | ||
E1 | 150 | T2=7 | 1.30623 (1.57724) | 0.27101 | 0.93 | 0.88947 (1.3293) | 0.43983 | 0.92 |
E2 | 0.05067 (0.29834) | 0.24767 | 0.95 | 0.23143 (0.45226) | 0.22083 | 0.94 | ||
E3 | 0.076 (0.44751) | 0.37151 | 0.95 | 0.34715 (0.67839) | 0.33124 | 0.94 | ||
E4 | 0.0865 (0.5093) | 0.42281 | 0.95 | 0.39508 (0.77205) | 0.37697 | 0.94 | ||
E1 | 150 | T2=3 | 1.31687 (1.58794) | 0.27108 | 0.93 | 0.88647 (1.32694) | 0.44047 | 0.93 |
E2 | 0.03946 (0.28884) | 0.24937 | 0.94 | 0.22951 (0.45066) | 0.22115 | 0.92 | ||
E3 | 0.0592 (0.43326) | 0.37406 | 0.94 | 0.34427 (0.67599) | 0.33172 | 0.92 | ||
E4 | 0.06737 (0.49308) | 0.42571 | 0.94 | 0.39181 (0.76933) | 0.37752 | 0.92 | ||
E1 | 250 | T2=7 | 1.3256 (1.53593) | 0.21033 | 0.93 | 0.92428 (1.25566) | 0.33138 | 0.94 |
E2 | 0.08241 (0.27419) | 0.19178 | 0.95 | 0.26889 (0.43892) | 0.17003 | 0.951 | ||
E3 | 0.12362 (0.41129) | 0.28767 | 0.95 | 0.40334 (0.65838) | 0.25504 | 0.951 | ||
E4 | 0.14068 (0.46808) | 0.32739 | 0.95 | 0.45903(0.74928) | 0.29025 | 0.951 | ||
E1 | 250 | T2=3 | 1.33245 (1.54265) | 0.2102 | 0.952 | 0.92852 (1.26253) | 0.33402 | 0.972 |
E2 | 0.08078 (0.27268) | 0.1919 | 0.98 | 0.26694 (0.437) | 0.17005 | 0.973 | ||
E3 | 0.12118 (0.40902) | 0.28784 | 0.98 | 0.40042 (0.6555) | 0.25508 | 0.97 | ||
E4 | 0.13791 (0.4655) | 0.32759 | 0.98 | 0.4557 (0.746) | 0.2903 | 0.972 |
Entropy | n | ACI | HPD | |||||
Interval | AL | CP | Interval | AL | CP | |||
E1 | 150 | r=120 | 1.32451 (1.54522) | 0.22071 | 0.912 | 1.20353 (1.86439) | 0.66086 | 0.94 |
E2 | 0.31014 (0.58536) | 0.27522 | 0.93 | 0.26572 (0.58693) | 0.3212 | 0.952 | ||
E3 | 0.15507 (0.29268) | 0.13761 | 0.93 | 0.13286 (0.29346) | 0.1606 | 0.951 | ||
E4 | 0.37438 (0.7066) | 0.33222 | 0.93 | 0.32076 (0.70848) | 0.38773 | 0.952 | ||
E1 | 150 | r=80 | 1.30054 (1.59109) | 0.29055 | 0.92 | 1.18112 (2.09479) | 0.91367 | 0.94 |
E2 | 0.2672 (0.62936) | 0.36215 | 0.95 | 0.19976 (0.62205) | 0.42229 | 0.96 | ||
E3 | 0.1336(0.31468) | 0.18108 | 0.95 | 0.09988 (0.31103) | 0.21114 | 0.96 | ||
E4 | 0.32254 (0.7597) | 0.43716 | 0.95 | 0.24114 (0.75088) | 0.50975 | 0.960 | ||
E1 | 250 | r=200 | 1.32132 (1.53199) | 0.21067 | 0.90 | 0.91591 (1.24612) | 0.33021 | 0.938 |
E2 | 0.32109 (0.58451) | 0.26342 | 0.96 | 0.60057 (0.93122) | 0.33065 | 0.941 | ||
E3 | 0.16055 (0.29225) | 0.13171 | 0.96 | 0.30029 (0.46561) | 0.16532 | 0.94 | ||
E4 | 0.38759 (0.70556) | 0.31797 | 0.96 | 0.72496 (1.12409) | 0.39913 | 0.941 | ||
E1 | 250 | r=170 | 1.31742 (1.57116) | 0.25374 | 0.951 | 0.86563 (1.17167) | 0.30604 | 0.95 |
E2 | 0.28194 (0.59672) | 0.31479 | 0.98 | 0.78845 (1.24102) | 0.45257 | 0.95 | ||
E3 | 0.14097 (0.29836) | 0.15739 | 0.98 | 0.39422(0.62051) | 0.22629 | 0.951 | ||
E4 | 0.34033 (0.72031) | 0.37998 | 0.98 | 0.95174 (1.49804) | 0.5463 | 0.951 | ||
E1 | 150 | T1=5 | 1.32346(1.5948) | 0.27135 | 0.292 | 0.89618 (1.34279) | 0.44661 | 0.90 |
E2 | 0.27375 (0.61058) | 0.33683 | 0.95 | 0.53076 (0.94919) | 0.41843 | 0.94 | ||
E3 | 0.13687(0.30529) | 0.16842 | 0.95 | 0.26536(0.47461) | 0.20924 | 0.94 | ||
E4 | 0.33044 (0.73704) | 0.40659 | 0.95 | 0.64065 (1.14576) | 0.5051 | 0.94 | ||
E1 | 150 | T1=3 | 1.30039 (1.57202) | 0.27163 | 0.891 | 0.87685 (1.31329) | 0.43644 | 0.88 |
E2 | 0.27726 (0.61612) | 0.33886 | 0.94 | 0.54827 (0.9726) | 0.42433 | 0.93 | ||
E3 | 0.13863 (0.30806) | 0.16943 | 0.94 | 0.27414 (0.4863) | 0.21217 | 0.93 | ||
E4 | 0.33468 (0.74373) | 0.40905 | 0.94 | 0.66182 (1.17403) | 0.51221 | 0.93 | ||
E1 | 250 | T1=5 | 1.31995 (1.53043) | 0.21048 | 0.94 | 0.92732 (1.25933) | 0.33201 | 0.97 |
E2 | 0.33064 (0.59527) | 0.26462 | 0.97 | 0.60102 (0.93213) | 0.33111 | 0.96 | ||
E3 | 0.16532 (0.29763) | 0.13231 | 0.97 | 0.30051 (0.46607) | 0.16556 | 0.96 | ||
E4 | 0.39912 (0.71855) | 0.31943 | 0.97 | 0.72549 (1.12518) | 0.39969 | 0.96 | ||
E1 | 250 | T1=2 | 1.33397 (1.54433) | 0.21036 | 0.88 | 0.92234 (1.25581) | 0.33347 | 0.89 |
E2 | 0.31502 (0.57695) | 0.26194 | 0.91 | 0.59443 (0.92381) | 0.32939 | 0.92 | ||
E3 | 0.15751 (0.28848) | 0.13097 | 0.91 | 0.29721 (0.46191) | 0.16469 | 0.92 | ||
E4 | 0.38026 (0.69645) | 0.31619 | 0.91 | 0.71753 (1.11514) | 0.3976 | 0.92 | ||
E1 | 150 | T2=7 | 1.30473 (1.57627) | 0.27154 | 0.93 | 0.89139 (1.33151) | 0.44012 | 0.94 |
E2 | 0.05189 (0.29982) | 0.24793 | 0.96 | 0.23277 (0.45373) | 0.22096 | 0.95 | ||
E3 | 0.07784 (0.44973) | 0.37189 | 0.96 | 0.34912 (0.68059) | 0.33146 | 0.95 | ||
E4 | 0.08858 (0.51183) | 0.42324 | 0.96 | 0.39734 (0.77451) | 0.37717 | 0.95 | ||
E1 | 150 | T2=3 | 1.30623 (1.57796) | 0.27172 | 0.94 | 0.89652 (1.33842) | 0.44189 | 0.93 |
E2 | 0.05519 (0.30286) | 0.24767 | 0.97 | 0.23189 (0.45337) | 0.22149 | 0.94 | ||
E3 | 0.08279 (0.45429) | 0.3715 | 0.97 | 0.34783 (0.68006) | 0.33223 | 0.94 | ||
E4 | 0.09422 (0.51701) | 0.42279 | 0.97 | 0.39585 (0.77396) | 0.37811 | 0.94 | ||
E1 | 250 | T2=7 | 1.32349 (1.53398) | 0.21048 | 0.92 | 0.92772 (1.25923) | 0.33151 | 0.956 |
E2 | 0.08622 (0.2777) | 0.19149 | 0.96 | 0.27025 (0.44047) | 0.17022 | 0.981 | ||
E3 | 0.12932 (0.41655) | 0.28723 | 0.96 | 0.40537 (0.6607) | 0.25533 | 0.981 | ||
E4 | 0.14718 (0.47407) | 0.32689 | 0.96 | 0.46134 (0.75193) | 0.29058 | 0.951 | ||
E1 | 250 | T2=3 | 1.33356 (1.54387) | 0.21031 | 0.91 | 0.93109 (1.26572) | 0.33463 | 0.899 |
E2 | 0.08117 (0.27311) | 0.19194 | 0.945 | 0.26625 (0.43669) | 0.17044 | 0.91 | ||
E3 | 0.12176 (0.40967) | 0.28791 | 0.945 | 0.39937 (0.65503) | 0.25566 | 0.912 | ||
E4 | 0.13857 (0.46623) | 0.32766 | 0.945 | 0.45451 (0.74547) | 0.29096 | 0.912 |
Entropy | MLE | SE | LINEX | GE | |||
q=(4) | q=(−4) | q=(4) | q=(−4) | ||||
E1 | 0.87621 | 0.80134 | 0.81222 | 0.79066 | 0.81134 | 0.78419 | |
E2 | Case 1 | 0.43147 | 0.49738 | 0.50214 | 0.49264 | 0.50444 | 0.48498 |
E3 | 0.64721 | 0.74759 | 0.75809 | 0.7372 | 0.75794 | 0.72962 | |
E4 | 0.73657 | 0.85118 | 0.86477 | 0.83793 | 0.8629 | 0.83111 | |
E1 | 0.8699 | 0.74041 | 0.75034 | 0.73028 | 0.75034 | 0.72235 | |
E2 | Case 2 | 0.32401 | 0.43615 | 0.4409 | 0.43133 | 0.44414 | 0.42118 |
E3 | 0.48601 | 0.65422 | 0.66488 | 0.64335 | 0.66621 | 0.63178 | |
E4 | 0.55312 | 0.74455 | 0.75835 | 0.73046 | 0.7582 | 0.71901 | |
E1 | 0.77377 | 0.61321 | 0.6271 | 0.5993 | 0.62968 | 0.58181 | |
E2 | Case 3 | 0.32039 | 0.37454 | 0.38353 | 0.36519 | 0.39153 | 0.3149 |
E3 | 0.48059 | 0.56181 | 0.58189 | 0.54052 | 0.5873 | 0.47235 | |
E4 | 0.54695 | 0.63938 | 0.6653 | 0.61165 | 0.66839 | 0.53757 |