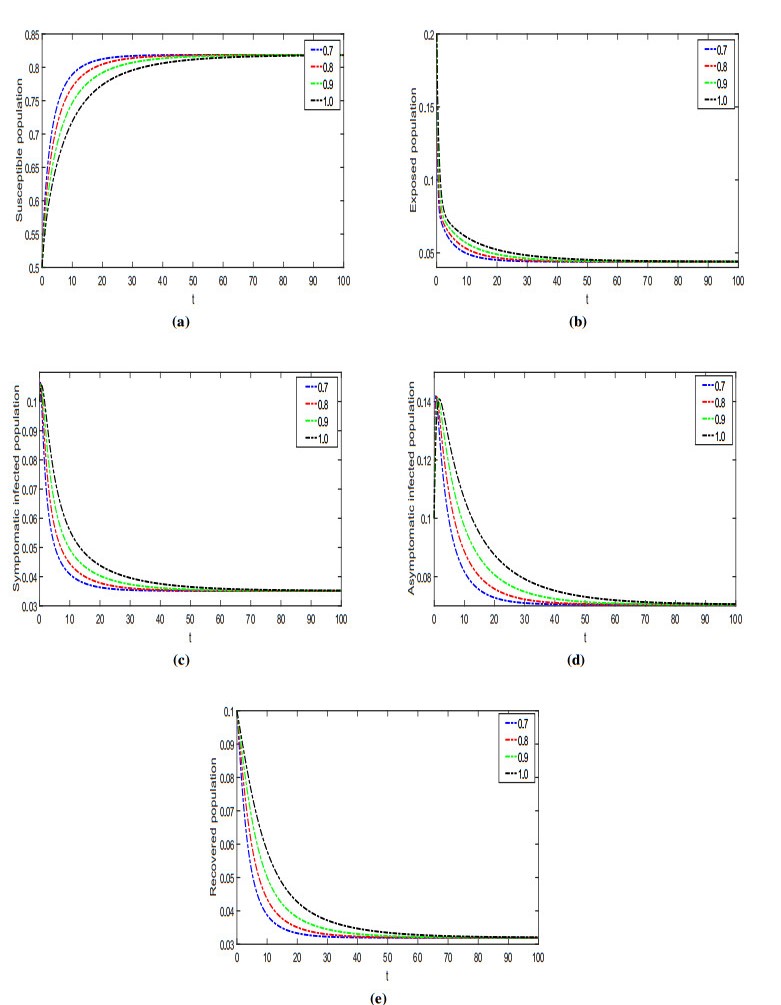
The majority of infectious illnesses, such as HIV/AIDS, Hepatitis, and coronavirus (2019-nCov), are extremely dangerous. Due to the trial version of the vaccine and different forms of 2019-nCov like beta, gamma, delta throughout the world, still, there is no control on the transmission of coronavirus. Delay factors such as social distance, quarantine, immigration limitations, holiday extensions, hospitalizations, and isolation are being utilized as essential strategies to manage the outbreak of 2019-nCov. The effect of time delay on coronavirus disease transmission is explored using a non-linear fractional order in the Caputo sense in this paper. The existence theory of the model is investigated to ensure that it has at least one and unique solution. The Ulam-Hyres (UH) stability of the considered model is demonstrated to illustrate that the stated model's solution is stable. To determine the approximate solution of the suggested model, an efficient and reliable numerical approach (Adams-Bashforth) is utilized. Simulations are used to visualize the numerical data in order to understand the behavior of the different classes of the investigated model. The effects of time delay on dynamics of coronavirus transmission are shown through numerical simulations via MATLAB-17.
Citation: Lei Zhang, Mati Ur Rahman, Shabir Ahmad, Muhammad Bilal Riaz, Fahd Jarad. Dynamics of fractional order delay model of coronavirus disease[J]. AIMS Mathematics, 2022, 7(3): 4211-4232. doi: 10.3934/math.2022234
[1] | Hashem Najafi, Abdallah Bensayah, Brahim Tellab, Sina Etemad, Sotiris K. Ntouyas, Shahram Rezapour, Jessada Tariboon . Approximate numerical algorithms and artificial neural networks for analyzing a fractal-fractional mathematical model. AIMS Mathematics, 2023, 8(12): 28280-28307. doi: 10.3934/math.20231447 |
[2] | Shabir Ahmad, Aman Ullah, Mohammad Partohaghighi, Sayed Saifullah, Ali Akgül, Fahd Jarad . Oscillatory and complex behaviour of Caputo-Fabrizio fractional order HIV-1 infection model. AIMS Mathematics, 2022, 7(3): 4778-4792. doi: 10.3934/math.2022265 |
[3] | K. A. Aldwoah, Mohammed A. Almalahi, Mansour A. Abdulwasaa, Kamal Shah, Sunil V. Kawale, Muath Awadalla, Jihan Alahmadi . Mathematical analysis and numerical simulations of the piecewise dynamics model of Malaria transmission: A case study in Yemen. AIMS Mathematics, 2024, 9(2): 4376-4408. doi: 10.3934/math.2024216 |
[4] | Sara Salem Alzaid, Badr Saad T. Alkahtani . Real-world validation of fractional-order model for COVID-19 vaccination impact. AIMS Mathematics, 2024, 9(2): 3685-3706. doi: 10.3934/math.2024181 |
[5] | Ateq Alsaadi . Advancing water quality management: A synergistic approach using fractional differential equations and neural networks. AIMS Mathematics, 2025, 10(3): 5332-5352. doi: 10.3934/math.2025246 |
[6] | Iqbal M. Batiha, Abeer A. Al-Nana, Ramzi B. Albadarneh, Adel Ouannas, Ahmad Al-Khasawneh, Shaher Momani . Fractional-order coronavirus models with vaccination strategies impacted on Saudi Arabia's infections. AIMS Mathematics, 2022, 7(7): 12842-12858. doi: 10.3934/math.2022711 |
[7] | Tiecheng Zhang, Liyan Wang, Yuan Zhang, Jiangtao Deng . Finite-time stability for fractional-order fuzzy neural network with mixed delays and inertial terms. AIMS Mathematics, 2024, 9(7): 19176-19194. doi: 10.3934/math.2024935 |
[8] | Weerawat Sudsutad, Chatthai Thaiprayoon, Jutarat Kongson, Weerapan Sae-dan . A mathematical model for fractal-fractional monkeypox disease and its application to real data. AIMS Mathematics, 2024, 9(4): 8516-8563. doi: 10.3934/math.2024414 |
[9] | Mohammed A. Almalahi, Satish K. Panchal, Fahd Jarad, Mohammed S. Abdo, Kamal Shah, Thabet Abdeljawad . Qualitative analysis of a fuzzy Volterra-Fredholm integrodifferential equation with an Atangana-Baleanu fractional derivative. AIMS Mathematics, 2022, 7(9): 15994-16016. doi: 10.3934/math.2022876 |
[10] | Ranbir Kumar, Sunil Kumar, Jagdev Singh, Zeyad Al-Zhour . A comparative study for fractional chemical kinetics and carbon dioxide CO2 absorbed into phenyl glycidyl ether problems. AIMS Mathematics, 2020, 5(4): 3201-3222. doi: 10.3934/math.2020206 |
The majority of infectious illnesses, such as HIV/AIDS, Hepatitis, and coronavirus (2019-nCov), are extremely dangerous. Due to the trial version of the vaccine and different forms of 2019-nCov like beta, gamma, delta throughout the world, still, there is no control on the transmission of coronavirus. Delay factors such as social distance, quarantine, immigration limitations, holiday extensions, hospitalizations, and isolation are being utilized as essential strategies to manage the outbreak of 2019-nCov. The effect of time delay on coronavirus disease transmission is explored using a non-linear fractional order in the Caputo sense in this paper. The existence theory of the model is investigated to ensure that it has at least one and unique solution. The Ulam-Hyres (UH) stability of the considered model is demonstrated to illustrate that the stated model's solution is stable. To determine the approximate solution of the suggested model, an efficient and reliable numerical approach (Adams-Bashforth) is utilized. Simulations are used to visualize the numerical data in order to understand the behavior of the different classes of the investigated model. The effects of time delay on dynamics of coronavirus transmission are shown through numerical simulations via MATLAB-17.
Coronaviruses are members of the corona Miridae family of viruses. The virus's size ranges from 65 to 125 nanometers. They're 22–26 kilobases of single-stranded RNA with a single nucleus. Alpha, beta, gamma, and delta are the other members of the coronavirus family. Influenza and the Middle East Respiratory Syndrome Coronavirus (MERS-COV) are two of the most frequent pulmonary diseases that can be fatal. They have the potential to induce pulmonary failure. Because the illness's primary hosts were camels, bats, and monkeys, it was once thought to be an animal-only sickness. It was later passed on to humans, and it has now become a global disaster. The features, locations, and transmission of the coronavirus in the human population were discovered in the literature [1]. A fatal coronavirus has been spreading in Wuhan, China, since December 2019. The number of people who have died is increasing all the time. 70,000 people were infected in the first 50 days of the illness. Coronaviruses of the beta group have been identified as the source of the infection. The virus has been named Wuhan virus or new Coronavirus 2019 by Chinese experts. SARS-COV-2, COVID-19 is the designation given to the virus by the International Committee of the Red Cross (ICRC). Using mathematical techniques, Zhao et al. investigated the coronavirus outbreak in China [2]. The worst-affected regions owing to coronavirus include Africa, America, Southeast Asia, Europe, the Eastern Mediterranean, and the Western Pacific. According to the World Health Organization (WHO), around thirty million individuals have been infected with the virus. One lakh and forty-four thousand people have been reported dead, while seven lakh and sixty-five thousand people have been reported recovered. There are 11,000 2019-nCov carriers in Pakistan, with 237 fatalities. Sadly, testing kits and lab tools are only available only around 2 percent of the time. As a result, governments used delaying tactics such as social distance, self-quarantine, and travel restrictions to combat the worldwide epidemic. The 2019-nCov pandemic has now been declared a worldwide concern by the WHO. Shim et al. examined the coronavirus's transmission potential and severity in South Korea [3]. Using the mathematical model of coronavirus, Kucharski et al. discovered a few control methods [4]. Jiang et al. proposed a machine learning model for coronavirus prediction based on data [5]. Fanelli et al. studied and forecast the COVID 19 spreading in China, Italy and France via integer order mathematical model [6]. Altan introduced new model of COVID 19 consisting of 2D curvelet transform, chaotic salp swarm algorithm and deep learning technique [7]. By incorporating delay factors into the system of differential equations, the mathematical modeling of the coronavirus model is in good accord with the real events. The factor of delay has been included in this model. Quarantine, isolation, or immunization, for example, are all delay causes. In most epidemiological models, if the infection rate is kept under control, the illness will eventually settle into a stable state. Controlling infection in the current scenario of 2019-nCov is almost difficult, therefore delaying measures, such as social distance, quarantine, isolation, and others, have been used to combat the pandemic of 2019-nCov. Fortunately, the modeling's delay factors or delaying strategies are self-contained and unaffected by other transmission rates. Keeping these views, Naveed et al. proposed a new delay integer order model of the coronavirus disease [8]. The detail of the delay model is given below.
Let the whole populace is divided into five subclasses, S(t): the susceptible populace, E(t): the exposed populace, I(t): symptomatic populace, A(t): asymptomatic populace and R(t): is the recovered populace, then the transmission dynamics of delayed is governed by the following set of differential equations [8].
dSdt=πs−(β1I(t−τ)S(t−τ)+β2A(t−τ)S(t−τ))e−μτ−μS,dEdt=(β1I(t−τ)S(t−τ)+β2A(t−τ)S(t−τ))e−μτ−(ω1+ω2+ω3+μ)E,dIdt=ω1E−(ω4+μ)I,dAdt=ω2E−(ω5+μ)A,dRdt=ω3E+ω4I+ω5A−μR. | (1.1) |
The above system involves different parameters such as: πs is the rate of recruitment, μ is the rate of mortality due to disease infection, β1 is the symptomatic rate of infection, β2 is the asymptomatic rate of infection, the interaction with infected symptomatic rate is ω1, the interaction with infected asymptomatic rate is ω2, ω3 is the exposed person recovered from disease, ω4 symptomatic person recovered after quarantine and the quarantine of asymptomatic infected persons with the rate of ω5.
From the few decades, fractional calculus (FC) has become a hot research area among researchers due to its wide range of applications in the modeling of physical processes. FC generalizes classical calculus. The first study for the generalization of the ordinary integral and differential operators into fractional derivatives (FD) is done by Riemann-Liouville and Caputo [9,10]. Fractional order differential equation provided a natural framework to study real-world problems, such as viscoelastic system, signal processing, diffusion processes, control processing, interested reader are referred to [11,12,13]. The aforementioned area has been addressed from different aspects such as qualitative analysis that include existence, uniqueness and regularity, the other is numerical and analytical method to test and view the information. The operators of FC are more general than the integers order operator because they have defined nonlocal kernels. The fractional operators (FOs) preserve memory and provide the past history of any physical process. There are many FOs concerned with the convolution of the kernels. The superiority of the FOs over classical operators has been presented in many research papers. We discuss some applications of FOs in the mathematical modeling of real-world physical problems. Nisar et al. investigated the fractional-order mathematical model of the current pandemic disease (COVID-19), which was based on real data [14]. Ameen et al., analyzed the fractional-order human liver based on real clinical data [15]. In literature [16], the authors have studied the blood ethanol model under three different fractional operators. To be more specific, FOs are also used by researchers for modeling bacterial diseases. For instance, Rihan et al. used fractional operators to analyze Salmonella bacterial infection [17]. Baleanu et al. investigated the human liver model via nonsingular fractional operators [18]. Alqahtani et al. solved numerically salmonella bacterial infection under fractional operator [19]. Droghei et al. compare the fractional and empirical model to analyze the dynamics of contaminant density and fluid pressure transients in swelling shales [20]. Falcini et al. apply fractional derivatives of a function concerning another function in order to have a more general and realistic picture of nonlocal effects in morphodynamics and sediment transport models [21]. Garra et al. the authors investigate the role played by the memory effect in the propagation of nonlinear thermoelastic waves using a time-fractional approach [22]. Jahanshahi et al. proposed a fractional-order SIRD model with time-dependent memory indexes for encompassing the multi-fractional characteristics of the COVID-19 [23]. Rajagopal and his coauthors constructed a fractional order model for Italy cases in [24]. We list several other applications of FC in applied sciences in [25,26,27,28].
The new fractional version of delayed system (1.1) is proposed under the time-derivative in sense of Caputo fractional derivative operator. Due to fractional order operator, imbalance of dimension occurs on the left and right sides of equations. According to [29], we can modify the fractional operator by an auxiliary parameter Λ, having the dimension of sec., to ensure the same dimension on both sides. Now the transmission model of the infectious disease for t>0 and 0<h≤1 takes the form
1Λ1−hCDhtS(t)=πs−(β1I(t−τ)S(t−τ)+β2A(t−τ)S(t−τ))e−μτ−μS,1Λ1−hCDhtE(t)=(β1I(t−τ)S(t−τ)+β2A(t−τ)S(t−τ))e−μτ−(ω1+ω2+ω3+μ)E,1Λ1−hCDhtI(t)=ω1E−(ω4+μ)I,1Λ1−hCDhtA(t)=ω2E−(ω5+μ)A,1Λ1−hCDhtR(t)=ω3E+ω4I+ω5A−μR, | (1.2) |
with initial conditions
S(0)=S0, E(0)=E0, I(0)=I0, A(0)=A0, R(0)=R0. |
The rest of paper is organized as: In Section 2, some definitions from fractional calculus are recalled. Section 3 is devoted for qualitative study of the considered model. In Section 4, the numerical solution is obtained for the system under consideration with the aid of Adams-Bashforth iterative technique. In Section 5, the Matlab software is used to perform the numerical simulation for getting the graphical representation of the considered model. In Section 6, conclusion of the paper is given.
This section gives some basic definitions and preliminaries of the fractional calculus theory, which are used in this study [30]. For the sake of simplicity we use RL, FI, and FOD for Reimann-Liouville, fractional integral and fractional order derivative, respectively.
Definition 2.1. Let V∈L1(R+) be a function, the RL FI of order h is defined by
IhtV(t)=1Γ(h)∫t0(t−℘)1−hV(℘)d℘. | (2.1) |
Definition 2.2. The Caputo FOD of V is defined as
CDhtV(t)=1Γ(n−h)∫t0(t−℘)n−h−1Vn(℘)d℘, |
provided that integral part exists, and n−1<h≤n n=[h]+1. If h∈(0,1), then one has
CDhtV(t)=1Γ(1−h)∫t0V′(℘)(t−℘)hd℘. |
Lemma 2.2.1. In the case of fractional differential equations, the following results are valid:
Ih[CDhq](t)=q(t)+η0+η1t+η2t2+...+ηn−1tn−1. |
In this part, we deal with the theory related to positive solution, invariant feasible region and boundedness of solution of the considered model. We find the basic reproduction number (BRN) and two equilibria states. One is disease free equilibrium (DFE) state and the other is endemic equilibrium (EE) state. For further discussion, let
R5+={(S,E,I,A,R)∈R5:S(0)>0,E(0)≥0,I(0)≥0,A(0)≥0,R≥0}, |
and IntR5+ denotes the interior of R5+.
Lemma 3.0.2 (Positivity). If (S(0),E(0),I(0),A(0),R(0))T∈IntR5+, then for any t≥0, the solution of the considered mode are non-negative.
Proof. From the first equation of considered model (2), we have
1Λ1−hCDhtS(t)|S=0=πSCDhtS(t)|S=0=Λ1−hπS≥0. |
Now, from the 2nd equation of (2), we get
1Λ1−hCDhtE(t)|E=0=(β1I(t−τ)S(t−τ)+β2A(t−τ)S(t−τ))e−μτCDhtE(t)|E=0=Λ1−h(β1I(t−τ)S(t−τ)+β2A(t−τ)S(t−τ))e−μτ≥0. |
Similarly
CDhtI(t)|I=0=Λ1−hω1E≥0,CDhtA(t)|A=0=Λ1−hω2E≥0,CDhtR(t)|R=0=ω3E+ω4I+ω5A≥0. |
Thus, the all solutions of the model (2) are non-negative.
Suppose ß={S,E,I,A,R∈R5:S+E+I+A+R≤πsμ}. Now to prove the feasibility of system (3.1), we need to show that the closed set ß is the region.
Theorem 3.1. The closed set ß is positively invariant with respect to fractional system.
Proof. First of all we prove that N=S+E+I+A+R, is bounded and non-negative functions. By adding all the relation of the system (3.1), the total population with the fractional derivative is given as
1Λ1−hCDhtN(t)=πs−μN(t), |
where N=S+E+I+A+R. Now using the Laplace transform to obtain the population as
N(t)=N(0)Eh(−μΛ1−hth)+∫t0πsΛ1−h℘h−1Eh,h(−μΛ1−h℘h)d℘. |
The term N(0) denotes size of human population at t=0 and Eh(.) denotes a Mittag-Leffler function. After doing some basic algebra, one can write
N(t)=N(0)Eh(−μΛ1−hth)+∫t0πsΛ1−h℘h−1∞∑j=0(−1)jμjΛj(1−h)℘jhΓ(jh+h)d℘=πsΛ1−hμΛ1−h+Eh(−μΛ1−hth)(N(0)−πsΛ1−hμΛ1−h)=πsμ+Eh(−dΛ1−hth)(N(0)−πsμ). |
Thus if N(0)≤πsμ then for t>0, N(t)≤πsμ. So, the closed set ß is positively invariant in the frame of fractional derivative.
Here we discuss the steady states and the basic reproduction number. The model (2) has two equilibrium points. By solving 1Λ1−hCDht(.)=0,the model (2) two equilibrium points, which satisfies the system as:
πs−(β1I(t−τ)S(t−τ)+β2A(t−τ)S(t−τ))e−μτ−μS=0,(β1I(t−τ)S(t−τ)+β2A(t−τ)S(t−τ))e−μτ−(ω1+ω2+ω3+μ)E=0,ω1E−(ω4+μ)I=0,ω2E−(ω5+μ)A=0,ω3E+ω4I+ω5A−μR=0. | (3.1) |
The DFE point F0 can be easily obtained by putting all states variables equals to zero except susceptible state variable. Thus,
F0=(πSμ,0,0,0,0). |
Also, the EE point F∗ is given by
F1=(S∗,E∗,I∗,A∗,R∗), |
where
S∗=(ω4+μ)(μ+ω1+ω2+ω3)(μ+ω5)β1e−μτω1(ω5+μ)+ω2e−μτβ1(μ+ω4),E∗=πs−μS∗μ+ω1+ω2+ω3,I∗=ω1E∗μ+ω4,A∗=ω2E∗μ+ω5,R∗=ω5A∗+ω3E∗+ω4I∗μ+ω5. |
The basic Reproduction number (BRN): The basic reproduction number of an infection is the expected number of cases directly generated by one case in a population where all individuals are susceptible to infection. The BRN R can be obtained by using next generation matrix. From the considered model (1.1), we take the infectious and recovered class of the human populace as
F=(0β1πSe−μτμβ2πSe−μτμ0000000000000), |
and
V=(ω1+ω2+ω3+μ000−ω1ω4+μ00−ω20ω5+μ0−ω3−ω4−ω5μ). |
Now R is the spectral radius of FV−1 is given by
FV−1=(πse−μτ(β1ω1(μ+ω5)+β2ω2(μ+ω4))μ(ω1+ω2+ω3+μ)(μ+ω4)(μ+ω5)β1πSe−μτμ(ω4+μ)β2πSe−μτμ(ω5+μ)0000000000000). |
Hence R is given by
R=πse−μτ(β1ω1(μ+ω5)+β2ω2(μ+ω4))(μ+ω4)(μ+ω5)(μ+ω3+ω2+ω1)μ. |
Here, we will show the local stability of the BRN.
Theorem 3.2. If R<1, then the DFE of the said problem is locally asymptotically stable, otherwise unstable for R>1.
Proof. The Jacobian matrix for the considered model at DFE may be calculated as
J(F0)=[−μ0−β1πSe−μτμ−β2πSe−μτμ00−(μ+ω1+ω2+ω3)β1πSe−μτμβ2πSe−μτμ00ω1−ω4−μ000ω20−ω5−μ00ω3ω4ω5−μ]. |
From the Jacobian matrix we have obtained the following eigenvalues: λ1=−μ<0, λ2=−μ<0 and
|J(F0)−λI|=|−(ω1+ω2+ω3+μ)−λβ1πSe−μτμβ2πSe−μτμω1−(ω4+μ)−λ0ω20−(ω5+μ)−λ|=0. |
Put c1=(ω1+ω2+ω3+μ)>0, c2=β1πSe−μτμ>0, c3=β2πSe−μτμ>0, c4=ω4+μ>0 and c5=ω5+μ>0.
|J(F0)−λI|=|−c1−λc2c3ω1−c4−λ0ω20−c5−λ|=0. |
We get λ3+λ2[c5+c1+c4]+λ[c5(c1+c4)+c1c4−c2ω1−c3ω2]+(c1c4c5−ω2c3c4−c5c2ω1)=0.
Applying the criteria of Routh-Hurwitz of third order polynomial as [c5+c1+c4]>0, (c1c4c5−ω2c3c4−c5c2ω1)>0, if (c1c4c5−ω2c3c4+c5c2ω1)c1c4c5<1 substituting the values, we have R=πse−μτ(β1ω1(μ+ω5)+β2ω2(μ+ω4))(μ+ω4)(μ+ω5)(μ+ω3+ω2+ω1)μ<1 and [c5+c1+c4]+λ[c5(c1+c4)+c1c4−c2ω1−c3ω2]>(c1c4c5−ω2c3c4−c5c2ω1), if R<1. Therefore, all eigenvalues are non = positive. Thus F0 is locally asymptotically stable.
Theorem 3.3. If R>1, then the EE of F1 is locally asymptotically stable, otherwise unstable if R<1.
Proof. The Jacobian matrix of F1 can be calculated as
J(F1)=[−(β1I∗+β2A∗)e−μτ−μ0−β1πSe−μτμ−β2πSe−μτμ0(β1I∗+β2A∗)e−μτ−(μ+ω1+ω2+ω3)β1πSe−μτμβ2πSe−μτμ00ω1−ω4−μ000ω20−ω5−μ00ω3ω4ω5−μ]. |
We have obtained the following eigenvalues from the Jacobian matrix J(F1):
λ1=−μ<0 and
|J(F1)−λI|=|−d1−μ−λ0−d2−d3b1−b4−λb2b30ω1−b5−λ00ω20−d6−λ|=0, |
where
d1=(β1I∗+β2A∗)e−μτ>0,d2=β1S∗e−μτ>0,d3=β2S∗e−μτ>0, |
d4=ω1+ω2+ω3+μ>0,d5=ω4+μ>0,d6=ω5+μ>0. |
Now
λ4+(d1+d4+d5+d6+μ)λ3+(d3ω2−d1d6−d6μ−d5d6−d4d6+d2ω1−d1d5−d1d4−d5μ−d4μ−d4d5)λ2 |
+(d3d5ω2+d3μω2+d2d6ω1−d1d5d6−d1d4d6−d5d6μ−d4d6μ−d4d5d6+d2μω1−d1d4d5−d4d5μ)λ+ |
(d3d5μω2+d2d6μω1+d1d4d5d6+d4d5d6μ)=0. |
Therefore, n0λ4+n1λ3+n2λ2+n3λ+n4=0, where
n1=(d1+d4+d5+d6+μ),n2=(d3ω2−d1d6−d6μ−d5d6−d4d6+d2ω1−d1d5−d1d4−d5μ−d4μ−d4d5),n3=(d3d5ω2+d3μω2+d2d6ω1−d1d5d6−d1d4d6−d5d6μ−d4d6μ−d4d5d6+d2μω1−d1d4d5−d4d5μ),n4=(d3d5μω2+d2d6μω1+d1d4d5d6+d4d5d6μ). |
Now applying the critera of Routh-Hurwitz for fourth order polynomial as n0>0, n1>0, n1n2−n0n3>0, (n1n2−n0n3)n3−n21n4>0 and n4>0 only if R>1. Hence, all of the eigenvalues are non-positive. Therefore, by Routh Hurwitz criteria F1 is locally asymptotically stable.
Beside the study of non-integer-order differential equations numerically, the qualitative study of such system s of equations has remained the interest of many researchers [31]. Using of a fixed point theory, we construct the existence and uniqueness of proposed model (2). For this, we rewrite the system (2) as
{1Λ1−hCDhtG=Ψ(t,G(t)),fort∈[0,T],0<h≤1,G(t)|t=0=G0≥0. | (3.2) |
only if
{G(t)=(S,E,I,A,R)T,Ψ(t,G(t))=(Yi(t,S,E,I,A,R)), | (3.3) |
for i=1,2,3,4,5, and each Y1,Y2,Y3,Y4,Y5, are defined as
{Y1=πs−(β1I(t−τ)S(t−τ)+β2A(t−τ)S(t−τ))e−μτ−μS,Y2=(β1I(t−τ)S(t−τ)+β2A(t−τ)S(t−τ))e−μτ−(ω1+ω2+ω3+μ)E,Y3=ω1E−(ω4+μ)I,Y4=ω2E−(ω5+μ)A,Y5=ω3E+ω4I+ω5A−μR. |
On applying FI on both side (3.2), we get
G(t)−G(0)=Λ1−hΓ(h)∫t0Ψ(℘,G(℘))(t−℘)h−1d℘. | (3.4) |
In particular for each class we can rewrite (3.4) as
S(t)−S(0)=Λ1−hΓ(h)∫t0(t−℘)h−1Y1d℘,E(t)−E(0)=Λ1−hΓ(h)∫t0(t−℘)h−1Y2d℘,I(t)−I(0)=Λ1−hΓ(h)∫t0(t−℘)h−1Y3d℘,A(t)−A(0)=Λ1−hΓ(h)∫t0(t−℘)h−1Y4d℘,R(t)−R(0)=Λ1−hΓ(h)∫t0(t−℘)h−1Y5d℘. | (3.5) |
In order to prove the kernel Ψ(℘,G(℘)) satisfies the Lipschitz condition and contraction, it is sufficient to prove that all Yi satisfy Lipschitz condition and contraction.
Theorem 3.4. The kernel Yi satisfy the Lipschitz condition and contraction for bounded function G(t), if the given inequality holds
1>Υi≥0, |
for i=1,2,3,4,5.
Proof. We will start from S and S1, one can get
‖Y1(t,S)−Y1(t,S1)‖=‖(β1IS+β2AS)e−μτ−μS‖≤e−πτβ1‖I‖‖S(t)−S1(t)‖+e−πτβ2‖A‖‖S(t)−S1(t)‖−μ‖S(t)−S1(t)‖≤[e−πτ(‖β1I+β2A‖)]‖S(t)−S1(t)‖≤[e−πτ(‖β1a3+β2a4‖)−μ]‖S−S1‖. |
Let Υ1=e−πτ(‖β1a3+β2a4‖−μ), where I≤a3 and A≤a4 is a bounded function, thus
‖Y1(t,S)−Y1(t,S1)‖≤Υ1‖S−S1‖. | (3.6) |
Hence, for Y1 the Lipschitz condition is obtained and if 1>e−πτ(‖β1a3+β2a4‖)≥0, then Y1 is a contraction. Similarly, one can show that Yi, i=2,3,4,5 also fulfills the Lipschitz condition which is given as
{‖Y2(t,E)−Y2(t,E1)‖≤Υ2‖E−E1‖,‖Y3(t,I)−Y3(t,I1)‖≤Υ3‖I−I1‖,‖Y4(t,A)−Y4(t,A1)‖≤Υ4‖A−A1‖,‖Y5(t,R)−Y5(t,R1)‖≤Υ5‖R−R1‖, | (3.7) |
where Υ2=ω1+ω2+ω3+μ, Υ3=ω4+μ, Υ4=ω5+μ and Υ5=μ.
According to system (3.5), consider the following recursive form:
Ψ1n=Sn(t)−Sn−1(t)=Λ1−hΓ(h)∫t0(Y1(℘,Sn−1)−Y1(℘,Sn−2))(t−℘)h−1d℘,Ψ2n=En(t)−En−1(t)=Λ1−hΓ(h)∫t0(Y2(℘,En−1)−Y2(℘,En−2))(t−℘)h−1d℘,Ψ3n=In(t)−In−1(t)=Λ1−hΓ(h)∫t0(Y3(℘,In−1)−Y3(℘,In−2))(t−℘)h−1d℘,Ψ4n=An(t)−An−1(t)=Λ1−hΓ(h)∫t0(Y4(℘,An−1)−Y4(℘,An−2))(t−℘)h−1d℘,Ψ5n=Rn(t)−Rn−1(t)=Λ1−hΓ(h)∫t0(Y5(℘,Rn−1)−Y5(℘,Rn−2))(t−℘)h−1d℘, |
with initial conditions S(0)=S0, E(0)=E0, I(0)=I0, A(0)=A0 and R(0)=R0. Applying the norm to the first equation of the above system, we obtain
‖Ψ1n‖=‖Sn(t)−Sn−1(t)‖=‖Λ1−hΓ(h)∫t0(Y1(℘,Sn−1)−Y1(℘,Sn−2))(t−℘)h−1d℘‖,≤Λ1−hΓ(h)∫t0‖(Y1(℘,Sn−1)−Y1(℘,Sn−2))(t−℘)h−1‖d℘, |
using Lipchitz condition, we have
‖Ψ1n‖≤Λ1−hΓ(h)Υ1∫t0‖Ψ1(n−1)(℘)‖d℘. | (3.8) |
Similarly, we obtained
‖Ψ2n‖≤Λ1−hΓ(h)Υ2∫t0‖Ψ2(n−1)(℘)‖d℘,‖Ψ3n‖≤Λ1−hΓ(h)Υ3∫t0‖Ψ3(n−1)(℘)‖d℘,‖Ψ4n‖≤Λ1−hΓ(h)Υ4∫t0‖Ψ4(n−1)(℘)‖d℘,‖Ψ5n‖≤Λ1−hΓ(h)Υ5∫t0‖Ψ5(n−1)(℘)‖d℘. | (3.9) |
Hence, we can write
Sn(t)=n∑k=1Ψ1k(t), En(t)=n∑k=1Ψ2k(t), In(t)=n∑k=1Ψ3k(t), An(t)=n∑k=1Ψ4k(t),Rn(t)=n∑k=1Ψ5k(t). |
Next, we will show the existence of solution of the model (2).
Theorem 3.5. The solution of system (2) is exists for finite time t1, if
Λ1−hΓ(h)t1Υi<1. |
Proof. Using recursive technique and from Eq (3.8) and Eq (3.9), we deduce that
‖Ψ1n‖≤‖Sn(0)‖[Λ1−hΓ(h)Υ1t]n,‖Ψ2n‖≤‖En(0)‖[Λ1−hΓ(h)Υ2t]n,‖Ψ3n‖≤‖In(0)‖[Λ1−hΓ(h)Υ3t]n,‖Ψ4n‖≤‖An(0)‖[Λ1−hΓ(h)Υ4t]n,‖Ψ5n‖≤‖Rn(0)‖[Λ1−hΓ(h)Υ5t]n. | (3.10) |
Hence, the given system possess at least one solution and also it is continuous. Further, we will prove that the above function construct solution for the system (2), assume that
S(t)−S(0)=Sn(t)−J1n(t),E(t)−E(0)=En(t)−J2n(t),I(t)−I(0)=In(t)−J3n(t),A(t)−A(0)=An(t)−J4n(t),R(t)−R(0)=Rn(t)−J5n(t). |
Now
‖J1n(t)‖=‖Λ1−hΓ(h)∫t0(Y1(℘,S)−Y1(℘,Sn−1))d℘‖≤Λ1−hΓ(h)∫t0‖(Y1(℘,S)−Y1(℘,Sn−1))‖d℘≤Λ1−hΓ(h)Υ1‖S−Sn−1‖t. |
After repeating the method again, we have
‖J1n(t)‖≤[Λ1−hΓ(h)t]n+1Υn+11h. |
At t1, we obtain
‖J1n(t)‖≤[Λ1−hΓ(h)t1]n+1Υn+11h. |
Applying limit to the above equation as n goes to ∞, we get ‖J1n(t)‖→0. On the same fashion, we can also show that ‖Jin(t)‖,i=2,3,4,5. Thus, we complete the proof.
Next we show the uniqueness of the solution of the model (2). Let us suppose that S1(t),E1(t),I1(t),A1(t), and R1(t) be another solution of the model (2). Consider
S(t)−S1(t)=Λ1−hΓ(h)∫t0(Y1(℘,S)−Y1(℘,S1))d℘, | (3.11) |
taking norm of the Eq (3.11), we have
‖S(t)−S1(t)‖=Λ1−hΓ(h)∫t0‖(Y1(℘,S)−Y1(℘,S1))‖d℘. |
On using Lipschitz condition, we obtain
‖S(t)−S1(t)‖≤Λ1−hΓ(h)Υ1t‖S(t)−S1(t)‖. |
Hence,
‖S(t)−S1(t)‖(1−Λ1−hΓ(h)Υ1t)≤0. | (3.12) |
Theorem 3.6. The considered system possess a unique solution if the condition given below is satisfied.
1−Λ1−hΓ(h)Υ1t>0. |
Proof. Let the following condition holds
‖S(t)−S1(t)‖(1−Λ1−hΓ(h)Υ1t)≤0. |
Then, ‖S(t)−S1(t)‖=0. Thus, we get S(t)=S1(t). Similarly, we can show the same equality for E,I,A,R. Next, we show the Ulam-Hyers (UH) stability for the considered system.
Definition 3.7. The considered system is said to be UH stable if ∃ some constants δi>0, i∈N5 and for each fi>0, i∈N5, for
|S(t)−Λ1−hΓ(h)∫t0(t−℘)1−hY1(℘,S(℘))d℘|≤f1,|E(t)−Λ1−hΓ(h)∫t0(t−℘)1−hY2(℘,E(℘))d℘|≤f2,|I(t)−Λ1−hΓ(h)∫t0(t−℘)1−hY3(℘,I(℘))d℘|≤f3,|A(t)−Λ1−hΓ(h)∫t0(t−℘)1−hY4(℘,A(℘))d℘|≤f4,|R(t)−Λ1−hΓ(h)∫t0(t−℘)1−hY5(℘,R(℘))d℘|≤f5, |
and there exist {˙S,˙E,˙I,˙A,˙R} satisfy the following
˙S(t)=Λ1−hΓ(h)∫t0(t−℘)1−hY1(℘,˙S(℘))d℘,˙E(t)=Λ1−hΓ(h)∫t0(t−℘)1−hY2(℘,˙E(℘))d℘,˙I(t)=Λ1−hΓ(h)∫t0(t−℘)1−hY3(℘,˙I(℘))d℘,˙A(t)=Λ1−hΓ(h)∫t0(t−℘)1−hY4(℘,˙A(℘))d℘,˙R(t)=Λ1−hΓ(h)∫t0(t−℘)1−hY5(℘,˙R(℘))d℘, | (3.13) |
such that
|S−˙S|≤δ1f1, |E−˙E|≤δ2f2, |I−˙I|≤δ3f3, |A−˙A|≤δ4f4, |R−˙R|≤δ5f5. |
Assumption: Let us assume a Banach space on a real valued function B(U) and U=[0,b] and U=B(U)×B(U)×B(U)×B(U)×B(U) prescribe a norm ‖˙S,˙E,˙I,˙A,˙R‖=sup
Theorem 3.8. The considered system is UH stable with the above assumption.
Proof. The system has a unique solution, we have
\begin{eqnarray} \|\mathbb{S}-\dot{\mathbb{S}}\|& = &\frac{\Lambda^{1-\mathcal{h}}}{\Gamma(\mathcal{h})}\int_0^t(t-\wp)^{1-\mathcal{h}}\|\mathcal{Y}_1(\wp,{\mathbb{S}}(\wp))-\mathcal{Y}_1(\wp,\dot{\mathbb{S}}(\wp))\|d\wp\\ &\leq&\psi_1\|\mathbb{S}-\dot{\mathbb{S}}\|. \end{eqnarray} | (3.14) |
\begin{eqnarray} \|\mathbb{E}-\dot{\mathbb{E}}\|& = &\frac{\Lambda^{1-\mathcal{h}}}{\Gamma(\mathcal{h})}\int_0^t(t-\wp)^{1-\mathcal{h}}\|\mathcal{Y}_2(\wp,{\mathbb{E}}(\wp))-\mathcal{Y}_2(\wp,\dot{\mathbb{E}}(\wp))\|d\wp\\ &\leq&\psi_2\|\mathbb{E}-\dot{\mathbb{E}}\|. \end{eqnarray} | (3.15) |
\begin{eqnarray} \|\mathbb{I}-\dot{\mathbb{I}}\|& = &\frac{\Lambda^{1-\mathcal{h}}}{\Gamma(\mathcal{h})}\int_0^t(t-\wp)^{1-\mathcal{h}}\|\mathcal{Y}_3(\wp,{\mathbb{I}}(\wp))-\mathcal{Y}_3(\wp,\dot{\mathbb{I}}(\wp))\|d\wp\\ &\leq&\psi_3\|\mathbb{I}-\dot{\mathbb{I}}\|. \end{eqnarray} | (3.16) |
\begin{eqnarray} \|\mathbb{A}-\dot{\mathbb{A}}\|& = &\frac{\Lambda^{1-\mathcal{h}}}{\Gamma(\mathcal{h})}\int_0^t(t-\wp)^{1-\mathcal{h}}\|\mathcal{Y}_4(\wp,{\mathbb{A}}(\wp))-\mathcal{Y}_4(\wp,\dot{\mathbb{A}}(\wp))\|d\wp\\ &\leq&\psi_4\|\mathbb{A}-\dot{\mathbb{A}}\|. \end{eqnarray} | (3.17) |
\begin{eqnarray} \|\mathbb{R}-\dot{\mathbb{R}}\|& = &\frac{\Lambda^{1-\mathcal{h}}}{\Gamma(\mathcal{h})}\int_0^t(t-\wp)^{1-\mathcal{h}}\|\mathcal{Y}_5(\wp,{\mathbb{R}}(\wp))-\mathcal{Y}_5(\wp,\dot{\mathbb{R}}(\wp))\|d\wp\\ &\leq&\psi_5\|\mathbb{R}-\dot{\mathbb{R}}\|. \end{eqnarray} | (3.18) |
Using \mathbb{f}_i = \psi_i and \frac{\Lambda^{1-\mathcal{h}}}{\Gamma(\mathcal{h})} = \delta_i , we have
\begin{eqnarray} \|\mathbb{S}-\dot{\mathbb{S}}\|\leq\mathbb{f}_1\delta_1. \end{eqnarray} | (3.19) |
Similarly, for the rest of the classes, we have the following
\begin{eqnarray} \begin{split} \|\mathbb{E}-\dot{\mathbb{E}}\|\leq\mathbb{f}_2\delta_2,\\ \|\mathbb{I}-\dot{\mathbb{I}}\|\leq\mathbb{f}_3\delta_3,\\ \|\mathbb{A}-\dot{\mathbb{A}}\|\leq\mathbb{f}_3\delta_4,\\ \|\mathbb{R}-\dot{\mathbb{R}}\|\leq\mathbb{f}_5\delta_5. \end{split} \end{eqnarray} | (3.20) |
Thus, we have completed the proof.
In this part, we will utilize the Adams-Bashforth approach to develop a general algorithm for solving the considered system. Consider (3.5)
\begin{eqnarray} \begin{split} \mathbb{S}(t)-\mathbb{S}(0)& = \frac{\Lambda^{1-\mathcal{h}}}{\Gamma(\mathcal{h})}\int_0^t(t-\wp)^{\mathcal{h}-1}\mathcal{Y}_1(\wp,\mathbb{S},\mathbb{E},\mathbb{I},\mathbb{A},\mathbb{R})d\wp,\\ \mathbb{E}(t)-\mathbb{E}(0)& = \frac{\Lambda^{1-\mathcal{h}}}{\Gamma(\mathcal{h})}\int_0^t(t-\wp)^{\mathcal{h}-1}\mathcal{Y}_2(\wp,\mathbb{S},\mathbb{E},\mathbb{I},\mathbb{A},\mathbb{R})d\wp,\\ \mathbb{I}(t)-\mathbb{I}(0)& = \frac{\Lambda^{1-\mathcal{h}}}{\Gamma(\mathcal{h})}\int_0^t(t-\wp)^{\mathcal{h}-1}\mathcal{Y}_3(\wp,\mathbb{S},\mathbb{E},\mathbb{I},\mathbb{A},\mathbb{R})d\wp,\\ \mathbb{A}(t)-\mathbb{A}(0)& = \frac{\Lambda^{1-\mathcal{h}}}{\Gamma(\mathcal{h})}\int_0^t(t-\wp)^{\mathcal{h}-1}\mathcal{Y}_4(\wp,\mathbb{S},\mathbb{E},\mathbb{I},\mathbb{A},\mathbb{E})d\wp,\\ \mathbb{R}(t)-\mathbb{R}(0)& = \frac{\Lambda^{1-\mathcal{h}}}{\Gamma(\mathcal{h})}\int_0^t(t-\wp)^{\mathcal{h}-1}\mathcal{Y}_5(\wp,\mathbb{S},\mathbb{E},\mathbb{I},\mathbb{A},\mathbb{R})d\wp. \end{split} \end{eqnarray} | (4.1) |
First, we construct the numerical scheme for the first equation of the above system. For this we take
\begin{equation} \mathbb{S}(t)-\mathbb{S}(0) = \frac{\Lambda^{1-\mathcal{h}}}{\Gamma(\mathcal{h})}\int_0^t(t-\wp)^{\mathcal{h}-1}\mathcal{Y}_1(\wp,\mathbb{S},\mathbb{E},\mathbb{I},\mathbb{A},\mathbb{R})d\wp. \end{equation} | (4.2) |
Let us denote t_{v} = v\Delta, \quad v = 0, 1, 2, \cdots, J, where \Delta = \frac{T}{J} is the step size and J is a positive integer and T > 0 . At t = t_{v+1}, \; v = 0, 1, 2, \cdots, the Eq (4.2) becomes
\begin{equation} \mathbb{S}(t_{v+1})-\mathbb{S}(0) = \frac{\Lambda^{1-\mathcal{h}}}{\Gamma(\mathcal{h})}\int_{0}^{t_{v+1}}\left(t_{v+1}-t\right)^{\mathcal{h}-1}\mathcal{Y}_1(\mathbb{S},\mathbb{E},\mathbb{I},\mathbb{A},\mathbb{R},t)dt , \end{equation} | (4.3) |
and
\begin{equation} \mathbb{S}(t_{v})-\mathbb{S}(0) = \frac{\Lambda^{1-\mathcal{h}}}{\Gamma(\mathcal{h})}\int_{0}^{t_{v}}\left(t_{v}-t\right)^{\mathcal{h}-1}\mathcal{Y}_1(\mathbb{S},\mathbb{E},\mathbb{I},\mathbb{A},\mathbb{R},t)dt . \end{equation} | (4.4) |
By subtracting (4.4) from (4.3), we obtain
\begin{eqnarray*} \mathbb{S}(t_{v+1})& = &\mathbb{S}(t_{v})+\frac{\Lambda^{1-\mathcal{h}}}{\Gamma(\mathcal{h})}\int_{0}^{t_{v+1}}\left(t_{v+1}-t\right)^{\mathcal{h}-1}\mathcal{Y}_1(\mathbb{S},\mathbb{E},\mathbb{I},\mathbb{A},\mathbb{R},t)dt\\ &&+\frac{\Lambda^{1-\mathcal{h}}}{\Gamma(\mathcal{h})}\int_{0}^{t_{v}}\left(t_{v}-t\right)^{\mathcal{h}-1}\mathcal{Y}_1(\mathbb{S},\mathbb{E},\mathbb{I},\mathbb{A},\mathbb{R},t)dt. \end{eqnarray*} |
Let us write the above equation into the following form
\begin{equation} \mathbb{S}(t_{v+1}) = \mathbb{S}(t_{v})+\mathscr{H}_{\mathcal{h},1}+\mathscr{H}_{\mathcal{h},2}, \end{equation} | (4.5) |
where
\begin{align*} \mathscr{H}_{\mathcal{h},1}& = \frac{\Lambda^{1-\mathcal{h}}}{\Gamma(\mathcal{h})}\int_{0}^{t_{v+1}}\left(t_{v+1}-t\right)^{\mathcal{h}-1}\mathcal{Y}_1(\mathbb{S},\mathbb{E},\mathbb{I},\mathbb{A},\mathbb{R},t)dt,\\ \mathscr{H}_{\mathcal{h},2}& = \frac{\Lambda^{1-\mathcal{h}}}{\Gamma(\mathcal{h})}\int_{0}^{t_{v}}\left(t_{v}-t\right)^{\mathcal{h}-1}\mathcal{Y}_1(\mathbb{S},\mathbb{E},\mathbb{I},\mathbb{A},\mathbb{R},t)dt. \end{align*} |
On using the Lagrangian polynomial interpolation, one can get the approximation the function \mathcal{Y}_1(\mathbb{S}, \mathbb{E}, \mathbb{I}, \mathbb{A}, \mathbb{R}, t) as
\begin{align} \bf{Z}(t)& = \frac{t-t_{v-1}}{t_{v}-t_{v-1}}\mathcal{Y}_{1}(\mathbb{S}_{v},\mathbb{E}_{v},\mathbb{I}_{v},\mathbb{A}_{v},\mathbb{R}_{v},t_{v})+\frac{t-t_{v}}{t_{v-1}-t_{v}}\mathcal{Y}_{1}(\mathbb{S}_{v-1},\mathbb{E}_{v-1},\mathbb{I}_{v-1},\mathbb{A}_{v-1},\mathbb{R}_{v-1},t_{v-1}) \\ & = \frac{\mathcal{Y}_{1}(\mathbb{S}_{v},\mathbb{E}_{v},\mathbb{I}_{v},\mathbb{A}_{v},\mathbb{R}_{v},t_{v})}{\Delta}(t-t_{v-1})-\frac{\mathcal{Y}_{1}(\mathbb{S}_{v-1},\mathbb{E}_{v-1},\mathbb{I}_{v-1},\mathbb{A}_{v-1},\mathbb{R}_{v-1},t_{v-1})}{\Delta}(t-t_{v}) , \end{align} | (4.6) |
thus,
\begin{eqnarray*} \mathscr{H}_{\mathcal{h},1}& = &\frac{\mathcal{Y}_{1}(\mathbb{S}_{v},\mathbb{E}_{v},\mathbb{I}_{v},\mathbb{A}_{v},\mathbb{R}_{v},t_{v})}{\Delta\Gamma(\mathcal{h})}\int_{0}^{t_{v+1}}\left(t_{v+1}-t\right)^{\mathcal{h}-1}\left(t-t_{v-1}\right)dt\\ &&-\frac{\mathcal{Y}_{1}(\mathbb{S}_{v-1},\mathbb{E}_{v-1},\mathbb{I}_{v-1},\mathbb{A}_{v-1},\mathbb{R}_{v-1},t_{v-1})}{\Delta\Gamma(\mathcal{h})}\int_{0}^{t_{v+1}}\left(t_{v+1}-t\right)\left(t-t_{v}\right)dt. \end{eqnarray*} |
Simplifying the integral of the above equation, one can obtain
\begin{eqnarray*} \mathscr{H}_{\mathcal{h},1}& = &\frac{\mathcal{Y}_{1}(\mathbb{S}_{v},\mathbb{E}_{v},\mathbb{I}_{v},\mathbb{A}_{v},\mathbb{R}_{v},t_{v})}{\Delta\Gamma(\mathcal{h})}\left\{\frac{2\Delta}{\mathcal{h}}t_{v+1}^{\mathcal{h}}-\frac{t_{v+1}^{\mathcal{h}+1}}{\mathcal{h}+1}\right\} \\ &-&\frac{\mathcal{Y}_{1}(\mathbb{S}_{v-1},\mathbb{E}_{v-1},\mathbb{I}_{v-1},\mathbb{A}_{v-1},\mathbb{R}_{v-1},t_{v-1})}{\Delta\Gamma(\mathcal{h})}\left\{\frac{\Delta}{\mathcal{h}}t_{v+1}^{\mathcal{h}}-\frac{t_{v+1}^{\mathcal{h}+1}}{\mathcal{h}+1}\right\} . \end{eqnarray*} |
Similarly
\begin{eqnarray*} \mathscr{H}_{\mathcal{h},2}& = &\frac{\mathcal{Y}_{1}(\mathbb{S}_{v},\mathbb{E}_{v},\mathbb{I}_{v},\mathbb{A}_{v},\mathbb{R}_{v},t_{v})}{\Delta\Gamma(\mathcal{h})}\left\{\frac{\Delta}{\mathcal{h}}t_{v}^{\mathcal{h}}-\frac{t_{v}^{\mathcal{h}+1}}{\mathcal{h}+1}\right\}\\ &&+\frac{\mathcal{Y}_{1}(\mathbb{S}_{v-1},\mathbb{E}_{v-1},\mathbb{I}_{v-1},\mathbb{A}_{v-1},\mathbb{R}_{v-1},t_{v-1})}{\Delta\Gamma(\mathcal{h}+1)}t_{v}^{\mathcal{h}+1}. \end{eqnarray*} |
Putting the values of \mathscr{H}_{\mathcal{h}, 1} and \mathscr{H}_{\mathcal{h}, 2} in Eq (4.5), we obtain the following approximate solution for the first equation as
\begin{equation} \begin{cases} \mathbb{S}(t_{v+1})& = \mathbb{S}(t_{v})+{\Lambda^{1-\mathcal{h}}}\bigg[\frac{\mathcal{Y}_{1}(\mathbb{S}_{v},\mathbb{E}_{v},\mathbb{I}_{v},\mathbb{A}_{v},\mathbb{R}_{v},t_{v})}{\Delta\Gamma(\mathcal{h})}\Delta^{\mathcal{h}+1}\left\{\frac{2(v+1)^{\mathcal{h}}+v^{\mathcal{h}}}{\mathcal{h}}-\frac{(v+1)^{\mathcal{h}+1}+v^{\mathcal{h}+1}}{\mathcal{h}+1}\right\} \\ &+\frac{\mathcal{Y}_{1}(\mathbb{S}_{v-1},\mathbb{E}_{v-1},\mathbb{I}_{v-1},\mathbb{A}_{v-1},\mathbb{R}_{v-1},t_{v-1})}{\Delta\Gamma(\mathcal{h})}\Delta^{\mathcal{h}+1} \times\left\{\frac{(v+1)^{\mathcal{h}+1}}{\mathcal{h}}-\frac{(v+1)^{\mathcal{h}+1}}{\mathcal{h}+1}+\frac{v^{\mathcal{h}}}{\mathcal{h}+1}\right\}\bigg]. \end{cases} \end{equation} | (4.7) |
Using the above procedure, one can get the numerical scheme for other compartments as
\begin{equation} \begin{cases} \mathbb{E}(t_{v+1})& = \mathbb{E}(t_{v})+{\Lambda^{1-\mathcal{h}}}\bigg[\frac{\mathcal{Y}_{2}(\mathbb{S}_{v},\mathbb{E}_{v},\mathbb{I}_{v},\mathbb{A}_{v},\mathbb{R}_{v},t_{v})}{\Delta\Gamma(\mathcal{h})}\Delta^{\mathcal{h}+1}\left\{\frac{2(v+1)^{\mathcal{h}}+v^{\mathcal{h}}}{\mathcal{h}}-\frac{(v+1)^{\mathcal{h}+1}+v^{\mathcal{h}+1}}{\mathcal{h}+1}\right\} \\ &+\frac{\mathcal{Y}_{2}(\mathbb{S}_{v-1},\mathbb{E}_{v-1},\mathbb{I}_{v-1},\mathbb{A}_{v-1},\mathbb{R}_{v-1},t_{v-1})}{\Delta\Gamma(\mathcal{h})}\Delta^{\mathcal{h}+1} \times\left\{\frac{(v+1)^{\mathcal{h}+1}}{\mathcal{h}}-\frac{(v+1)^{\mathcal{h}+1}}{\mathcal{h}+1}+\frac{v^{\mathcal{h}}}{\mathcal{h}+1}\right\}\bigg]. \end{cases} \end{equation} | (4.8) |
\begin{equation} \begin{cases} \mathbb{I}(t_{v+1})& = \mathbb{I}(t_{v})+{\Lambda^{1-\mathcal{h}}}\bigg[\frac{\mathcal{Y}_{3}(\mathbb{S}_{v},\mathbb{E}_{v},\mathbb{I}_{v},\mathbb{A}_{v},\mathbb{R}_{v},t_{v})}{\Delta\Gamma(\mathcal{h})}\Delta^{\mathcal{h}+1}\left\{\frac{2(v+1)^{\mathcal{h}}+v^{\mathcal{h}}}{\mathcal{h}}-\frac{(v+1)^{\mathcal{h}+1}+v^{\mathcal{h}+1}}{\mathcal{h}+1}\right\} \\ &+\frac{\mathcal{Y}_{3}(\mathbb{S}_{v-1},\mathbb{E}_{v-1},\mathbb{I}_{v-1},\mathbb{A}_{v-1},\mathbb{R}_{v-1},t_{v-1})}{\Delta\Gamma(\mathcal{h})}\Delta^{\mathcal{h}+1} \times\left\{\frac{(v+1)^{\mathcal{h}+1}}{\mathcal{h}}-\frac{(v+1)^{\mathcal{h}+1}}{\mathcal{h}+1}+\frac{v^{\mathcal{h}}}{\mathcal{h}+1}\right\}\bigg]. \end{cases} \end{equation} | (4.9) |
\begin{equation} \begin{cases} \mathbb{A}(t_{v+1})& = \mathbb{A}(t_{v})+{\Lambda^{1-\mathcal{h}}}\bigg[\frac{\mathcal{Y}_{4}(\mathbb{S}_{v},\mathbb{E}_{v},\mathbb{I}_{v},\mathbb{A}_{v},\mathbb{R}_{v},t_{v})}{\Delta\Gamma(\mathcal{h})}\Delta^{\mathcal{h}+1}\left\{\frac{2(v+1)^{\mathcal{h}}+v^{\mathcal{h}}}{\mathcal{h}}-\frac{(v+1)^{\mathcal{h}+1}+v^{\mathcal{h}+1}}{\mathcal{h}+1}\right\} \\ &+\frac{\mathcal{Y}_{4}(\mathbb{S}_{v-1},\mathbb{E}_{v-1},\mathbb{I}_{v-1},\mathbb{A}_{v-1},\mathbb{R}_{v-1},t_{v-1})}{\Delta\Gamma(\mathcal{h})}\Delta^{\mathcal{h}+1} \times\left\{\frac{(v+1)^{\mathcal{h}+1}}{\mathcal{h}}-\frac{(v+1)^{\mathcal{h}+1}}{\mathcal{h}+1}+\frac{v^{\mathcal{h}}}{\mathcal{h}+1}\right\}\bigg]. \end{cases} \end{equation} | (4.10) |
\begin{equation} \begin{cases} \mathbb{R}(t_{v+1})& = \mathbb{R}(t_{v})+{\Lambda^{1-\mathcal{h}}}\bigg[\frac{\mathcal{Y}_{5}(\mathbb{S}_{v},\mathbb{E}_{v},\mathbb{I}_{v},\mathbb{A}_{v},\mathbb{R}_{v},t_{v})}{\Delta\Gamma(\mathcal{h})}\Delta^{\mathcal{h}+1}\left\{\frac{2(v+1)^{\mathcal{h}}+v^{\mathcal{h}}}{\mathcal{h}}-\frac{(v+1)^{\mathcal{h}+1}+v^{\mathcal{h}+1}}{\mathcal{h}+1}\right\} \\ &+\frac{\mathcal{Y}_{5}(\mathbb{S}_{v-1},\mathbb{E}_{v-1},\mathbb{I}_{v-1},\mathbb{A}_{v-1},\mathbb{R}_{v-1},t_{v-1})}{\Delta\Gamma(\mathcal{h})}\Delta^{\mathcal{h}+1} \times\left\{\frac{(v+1)^{\mathcal{h}+1}}{\mathcal{h}}-\frac{(v+1)^{\mathcal{h}+1}}{\mathcal{h}+1}+\frac{v^{\mathcal{h}}}{\mathcal{h}+1}\right\}\bigg]. \end{cases} \end{equation} | (4.11) |
Here we visualize the effect of fractional order and delay term on the transmission of coronavirus disease. In the given part, we provide the graphical representation by numerical simulation for verification of our considered fractional numerical scheme. For this, we chose some initial values for all the three compartments of our said problem having fractional order \mathcal{h} . The initial conditions used for numerical simulations are: \mathbb{S}(0) = 0.5 , \mathbb{E}(0) = 0.2 , \mathbb{I}(0) = 0.1 , \mathbb{A}(0) = 0.1 , \mathbb{R}(0) = 0.1 . We have taken the parameter values as: \Lambda = 0.99 , \omega_4 = 0.0987 , \beta_1 = 1.05 , \mu = 0.5 , \beta_2 = 1.05 , \omega_1 = 0.4787 , \omega_3 = 0.0854 , \pi_S = 0.5 , \omega_5 = 0.1234 , \omega_2 = 1.0004 . For 2019-nCov, we displayed each compartment of the delayed model without the time delay factor in Figure 1 for various values of \mathcal{h} . From Figure 1 (a)–(e), it is observed the susceptible population increases with the passage of time. Then exposed population must be decreases because all population remains in the susceptible class and will not expose to the infection. However, there is a little increase in the symptomatic and Asymptomatic population in a very short interval of time, then it goes down decreases and become stable. Also, recovered population decreases with passage of time. The impact of fractional order on the dynamical behaviour of proposed model is clearly observed in the Figure 1. All the compartment fatly attain their maximum or minimum at lower fractional order. At \mathcal{h} = 1 , the black color curves represent the dynamics of the integer model (1). Thus, fractional order model provide fast history about all compartments of the proposed model. Thus, our model (2) generalizes the model (1).
In Figure 2 we have simulated each compartment of the proposed model with fractional order \mathcal{h} = 0.7 , and at different values of the delay term \tau . When the delay time increases, the populace of the susceptible class grows while the populace of the exposed class decreases. Increased delaying strategies leads to a reduction in the infected population without a shift in the rate of transmission, according to our results. Despite the fact that the increase in delaying techniques may be seen as an exponential decrease in the number of symptomatic infected people. When \tau = 0.5 , the number of symptomatic infected people drops to zero, as seen in Figure 2(c). According to the graphs, we may overcome the pandemic of 2019-nCov by using delaying strategies such as social distance, quarantine, travel limitations, holiday extensions, hospitalization, and isolation for roughly 100 days.
Mathematical analysis of epidemic illnesses with a time delay effect is a useful tool for studying disease behavior. We've noticed important techniques for overcoming 2019-nCov's present crisis, as well as delaying strategies and delayed elements, all over the world. Reduce the 2019-nCov quickly by making the most of delaying techniques. Quarantine, isolation, social distance, and immigration limitations are the most effective strategies for delaying variables. However, based on the information provided, we may employ delaying techniques for a total of 100 days to achieve the desired results. An integer order model of the delay coronavirus was studied in literature [8]. Due to superiority and advantages over local operators, fractional operators have seen tremendous growth over time. In the literature of fractional calculus, there exist several nonlocal operators. We utilized a nonlocal operator with a power-law kernel in this study. This research examined an essential model of coronavirus with a time delay in the human population. We have developed the existence and uniqueness theory using fixed point theory, which assures that the model has at least one and unique solution. The Ulam-Hyres stability of the model was discussed using the idea of functional analysis. A reliable numerical method (Adams-Basforth technique) was used to find a numerical solution to the problem under consideration. The numerical simulations were used to show the theoretical conclusions of the model and to investigate the influence of fractional order on the dynamics of the model. Numerical simulations were used to investigate the influence of time delay on coronavirus transmission decrease. It is noticed that the delay model of coronavirus produces good results in the reduction of the diseases. People may adopt the delay strategies like social distancing, quarantine, strengthening the immune system to save themselves from getting the infection. Different nonlocal operators can be used to study the present work in the future.
There exist no conflict of interest regarding to this research work.
[1] |
M. D. Shereen, S. Khan, A. Kazmi, N. Bashir, R. Siddique, COVID-19 infection: Origin, transmission and characteristics of human coronaviruses, J. Adv. Res., 24 (2020), 91–98. https://doi.org/10.1016/j.jare.2020.03.005 doi: 10.1016/j.jare.2020.03.005
![]() |
[2] |
S. Zhao, H. Chen, Modeling the epidemic dynamics and control of COVID-19 outbreak in China, Quant. Biol., 11 (2020), 1–9. https://doi.org/10.1007/s40484-020-0199-0 doi: 10.1007/s40484-020-0199-0
![]() |
[3] |
E. Shim, A. Tariq, W. Choi, Y. Lee, G. Chowell, Transmission potential and severity of COVID-19 in South Korea, Int. J. Infect. Dis., 93 (2020), 339–344. https://doi.org/10.1016/j.ijid.2020.03.031 doi: 10.1016/j.ijid.2020.03.031
![]() |
[4] |
A. J. Kucharski, T. W. Russell, C. Diamond, Y. Liu, J. Edmunds, S. Funk, et al., Early dynamics of transmission and control of COVID-19: A mathematical modelling study, Lancet Infect. Dis., 20 (2020), 553–558. https://doi.org/10.1016/S1473-3099(20)30144-4 doi: 10.1016/S1473-3099(20)30144-4
![]() |
[5] |
X. Jiang, M. Coffee, A. Bari, J. Wang, X. Jiang, J. Huang, et al., Towards an artificial intelligence framework for data-driven prediction of coronavirus clinical severity, Comput. Mater. Con., 63 (2020), 537–551. https://doi.org/10.32604/cmc.2020.010691 doi: 10.32604/cmc.2020.010691
![]() |
[6] |
D. Fanelli, F. Piazza, Analysis and forecast of COVID-19 spreading in China, Italy and France, Chaos Soliton. Fract., 134 (2020), 109761. https://doi.org/10.1016/j.chaos.2020.109761 doi: 10.1016/j.chaos.2020.109761
![]() |
[7] |
A. Altan, S. Karasu, Recognition of COVID-19 disease from X-ray images by hybrid model consisting of 2D curvelet transform, chaotic salp swarm algorithm and deep learning technique, Chaos Soliton. Fract., 140 (2020), 110071. https://doi.org/10.1016/j.chaos.2020.110071 doi: 10.1016/j.chaos.2020.110071
![]() |
[8] |
M. Naveed, M. Rafiq, A. Raza, N. Ahmed, I. Khan, K. S. Nisar, et al., Mathematical analysis of novel coronavirus (2019-nCov) delay pandemic model, Comput. Mater. Con., 64 (2020), 1401–1414. https://doi.org/10.32604/cmc.2020.011314 doi: 10.32604/cmc.2020.011314
![]() |
[9] | A. A. Kilbas, H. M. Srivastava, J. J. Trujillo, Theory and applications of fractional differential equations, Elsevier, 2006. |
[10] | D. Baleanu, K. Diethelm, E. Scalas, J. J. Trujillo, Fractional calculus: Models and numerical methods, World Scientific, 2012. |
[11] | D. Baleanu, J. A. T. Machado, A. C. Luo, Fractional dynamics and control, Springer Science & Business Media, 2011. |
[12] | V. Lakshmikantham, S. Leela, J. V. Devi, Theory of fractional dynamic systems, Cambridge Scientific Publishers, 2009. |
[13] |
C. Li, D. Qian, Y. Chen, On Riemann-Liouville and caputo derivatives, Discrete Dyn. Nat. Soc., 2011 (2011), 562494. https://doi.org/10.1155/2011/562494 doi: 10.1155/2011/562494
![]() |
[14] |
K. S. Nisar, S. Ahmad, A. Ullah, K. Shah, H. Alrabaiah, M. Arfan, Mathematical analysis of SIRD model of COVID-19 with Caputo fractional derivative based on real data, Results Phys., 21 (2021), 103772. https://doi.org/10.1016/j.rinp.2020.103772 doi: 10.1016/j.rinp.2020.103772
![]() |
[15] |
I. G. Ameen, N. H. Sweilam, H. M. Ali, A fractional-order model of human liver: Analytic-approximate and numerical solutions comparing with clinical data, Alex. Eng. J., 60 (2021), 4797–4808. https://doi.org/10.1016/j.aej.2021.03.054 doi: 10.1016/j.aej.2021.03.054
![]() |
[16] |
S. Qureshi, A. Yusuf, A. A. Shaikh, M. Inc, D. Baleanu, Fractional modeling of blood ethanol concentration system with real data application, Chaos, 29 (2019), 013143. https://doi.org/10.1063/1.5082907 doi: 10.1063/1.5082907
![]() |
[17] |
F. A. Rihan, D. Baleanu, S. Lakshmanan, R. Rakkiyappan, On fractional SIRC model with salmonella bacterial infection, Abstr. Appl. Anal., 2014 (2014), 136263. https://doi.org/10.1155/2014/136263 doi: 10.1155/2014/136263
![]() |
[18] |
D. Baleanu, A. Jajarmi, H. Mohammadi, S. Rezapour, A new study on the mathematical modelling of human liver with Caputo-Fabrizio fractional derivative, Chaos Soliton. Fract., 134 (2020) 109705. https://doi.org/10.1016/j.chaos.2020.109705 doi: 10.1016/j.chaos.2020.109705
![]() |
[19] | R. T. Alqahtani, M. A. Abdelkawy, An efficient numerical algorithm for solving fractional SIRC model with salmonella bacterial infection, Math. Biosci. Eng., 17 (2020), 3784–3793. http://www.aimspress.com/journal/MBE |
[20] |
R. Droghei, E. Salusti, A comparison of a fractional derivative model with an empirical model for non-linear shock waves in swelling shales, J. Petrol. Sci. Eng., 125 (2015), 181–188. https://doi.org/10.1016/j.petrol.2014.11.017 doi: 10.1016/j.petrol.2014.11.017
![]() |
[21] |
F. Falcini, R. Garra, A nonlocal generalization of the Exner law, J. Hydrol., 603 (2021), 126947. https://doi.org/10.1016/j.jhydrol.2021.126947 doi: 10.1016/j.jhydrol.2021.126947
![]() |
[22] |
R. Garra, E. Salusti, R. Droghei, Memory effects on nonlinear temperature and pressure wave propagation in the boundary between two fluid-saturated porous rocks, Adv. Math. Phys., 2015 (2015), 532150. https://doi.org/10.1155/2015/532150 doi: 10.1155/2015/532150
![]() |
[23] |
H. Jahanshahi, J. M. Munoz-Pacheco, S. Bekiros, N. D. Alotaibi, A fractional-order SIRD model with time-dependent memory indexes for encompassing the multi-fractional characteristics of the COVID-19, Chaos Soliton. Fract., 143 (2021), 110632. https://doi.org/10.1016/j.chaos.2020.110632 doi: 10.1016/j.chaos.2020.110632
![]() |
[24] |
K. Rajagopal, N. Hasanzadeh, F. Parastesh, I. I. Hamarash, S. Jafari, I. Hussain, A fractional-order model for the novel coronavirus (COVID-19) outbreak, Nonlinear Dyn., 101 (2020), 711–718. https://doi.org/10.1007/s11071-020-05757-6 doi: 10.1007/s11071-020-05757-6
![]() |
[25] |
S. Ahmad, A. Ullah, A. Akgül, D. Baleanu, Analysis of the fractional tumour-immune-vitamins model with Mittag-Leffler kernel, Results Phys., 19 (2020) 103559. https://doi.org/10.1016/j.rinp.2020.103559 doi: 10.1016/j.rinp.2020.103559
![]() |
[26] |
D. Kumar, J. Singh, D. Baleanu, On the analysis of vibration equation involving a fractional derivative with Mittag-Leffler law, Math. Method. Appl. Sci., 43 (2020), 443–457. https://doi.org/10.1002/mma.5903 doi: 10.1002/mma.5903
![]() |
[27] |
D. Baleanu, H. Mohammadi, S. Rezapour, A fractional differential equation model for the COVID-19 transmission by using the Caputo-Fabrizio derivative, Adv. Differ. Equ., 2020 (2020), 299. https://doi.org/10.1186/s13662-020-02762-2 doi: 10.1186/s13662-020-02762-2
![]() |
[28] |
S. Kumar, A. Ahmadian, R. Kumar, D. Kumar, J. Singh, D. Baleanu, et al., An efficient numerical method for fractional SIR epidemic model of infectious disease by using Bernstein wavelets, Mathematics, 8 (2020), 558. https://doi.org/10.3390/math8040558 doi: 10.3390/math8040558
![]() |
[29] | J. F. Gómez-Aguilar, J. J. Rosales-García, J. J. Bernal-Alvarado, T. Córdova-Fraga, R. Guzmán-Cabrera, Fractional mechanical oscillators, Rev. mexicana de física, 58 (2012), 348–352. |
[30] | K. S. Miller, B. Ross, An introduction to the fractional calculus and fractional differential equations, Willy, 1993. |
[31] |
H. Khan, Y. Li, A. Khan, A. Khan, Existence of solution for a fractional‐order Lotka-Volterra reaction-diffusion model with Mittag-Leffler kernel, Math. Method. Appl. Sci., 42 (2019), 3377–3387. https://doi.org/10.1002/mma.5590 doi: 10.1002/mma.5590
![]() |
1. | Xuan Liu, Muhammad Arfan, Mati ur Rahman, Bibi Fatima, Analysis of SIQR type mathematical model under Atangana-Baleanu fractional differential operator, 2023, 26, 1025-5842, 98, 10.1080/10255842.2022.2047954 | |
2. | Rahma Al-Masaeed, Banan Maayah, Sana Abu-Ghurra, Adaptive Technique for Solving 1-D Interface Problems of Fractional Order, 2022, 8, 2349-5103, 10.1007/s40819-022-01397-z | |
3. | Manoj Kumar, An Efficient Numerical Scheme for Solving a Fractional-Order System of Delay Differential Equations, 2022, 8, 2349-5103, 10.1007/s40819-022-01466-3 | |
4. | Manuel Caravaca Garratón, María del Carmen García-Onsurbe, Antonio Soto-Meca, A new Network Simulation Method for the characterization of delay differential equations, 2022, 20904479, 102066, 10.1016/j.asej.2022.102066 | |
5. | Azzh Saad Alshehry, Rasool Shah, Nehad Ali Shah, Ioannis Dassios, A Reliable Technique for Solving Fractional Partial Differential Equation, 2022, 11, 2075-1680, 574, 10.3390/axioms11100574 | |
6. | Nassima Nasri, Fatima Aissaoui, Keltoum Bouhali, Assia Frioui, Badreddine Meftah, Khaled Zennir, Taha Radwan, Fractional Weighted Midpoint-Type Inequalities for s-Convex Functions, 2023, 15, 2073-8994, 612, 10.3390/sym15030612 | |
7. | Zaineb Yakoub, Omar Naifar, Messaoud Amairi, Manel Chetoui, Mohamed Aoun, Abdellatif Ben Makhlouf, Jerzy Baranowski, A Bias-Corrected Method for Fractional Linear Parameter Varying Systems, 2022, 2022, 1563-5147, 1, 10.1155/2022/7278157 | |
8. | Manvendra Narayan Mishra, A. F. Aljohani, Mathematical modelling of growth of tumour cells with chemotherapeutic cells by using Yang–Abdel–Cattani fractional derivative operator, 2022, 16, 1658-3655, 1133, 10.1080/16583655.2022.2146572 | |
9. | Khalid Hattaf, On the Stability and Numerical Scheme of Fractional Differential Equations with Application to Biology, 2022, 10, 2079-3197, 97, 10.3390/computation10060097 | |
10. | Chouaib Bounkaicha, Karam Allali, Modelling disease spread with spatio-temporal fractional derivative equations and saturated incidence rate, 2023, 2363-6203, 10.1007/s40808-023-01773-8 | |
11. | N.H. Sweilam, S. Ahmed, Monika Heiner, Two different parallel approaches for a hybrid fractional order Coronavirus model, 2023, 24, 11108665, 100408, 10.1016/j.eij.2023.100408 | |
12. | Nabeela Anwar, Iftikhar Ahmad, Adiqa Kausar Kiani, Muhammad Shoaib, Muhammad Asif Zahoor Raja, Intelligent solution predictive networks for non-linear tumor-immune delayed model, 2024, 27, 1025-5842, 1091, 10.1080/10255842.2023.2227751 | |
13. | Haifa Bin Jebreen, Carlo Cattani, Solving Time-Fractional Partial Differential Equation Using Chebyshev Cardinal Functions, 2022, 11, 2075-1680, 642, 10.3390/axioms11110642 | |
14. | Mati ur Rahman, Saira Tabassum, Ali Althobaiti, Saad Althobaiti, An analysis of fractional piecewise derivative models of dengue transmission using deep neural network, 2024, 18, 1658-3655, 10.1080/16583655.2024.2340871 | |
15. | Manisha Meena, Mridula Purohit, S.D. Purohit, Dumitru Baleanu, D.L. Suthar, A novel fractionalized investigation of tuberculosis disease, 2024, 32, 2769-0911, 10.1080/27690911.2024.2351229 | |
16. | S. Dickson, S. Padmasekaran, K. Lakshmanan, Stability of delayed fractional order SEIQI_cRVW mathematical model for Omicron variant, 2024, 12, 2195-268X, 1392, 10.1007/s40435-023-01287-2 | |
17. | Khushbu Agrawal, Sunil Kumar, Badr Saad T. Alkahtani, Sara S. Alzaid, The use of Hermite wavelet collocation method for fractional cancer dynamical system, 2024, 32, 2769-0911, 10.1080/27690911.2024.2352745 | |
18. | Mati ur Rahman, M.A. El-Shorbagy, Hussam Alrabaiah, Dumitru Baleanu, Manuel De la Sen, Investigating a new conservative 4-dimensional chaotic system, 2023, 53, 22113797, 106969, 10.1016/j.rinp.2023.106969 | |
19. | A. E. Matouk, Ismail Gad Ameen, Yasmeen Ahmed Gaber, Analyzing the dynamics of fractional spatio-temporal \mathrm{SEIR} epidemic model, 2024, 9, 2473-6988, 30838, 10.3934/math.20241489 | |
20. | Agus Suryanto, Isnani Darti, Trisilowati, Bapan Ghosh, Raqqasyi Rahmatullah Musafir, A fractional-order generalized Richards growth model and its implementation to COVID-19 data, 2024, 31, 2576-5299, 345, 10.1080/25765299.2024.2362987 | |
21. | Ateq Alsaadi, Advancing water quality management: A synergistic approach using fractional differential equations and neural networks, 2025, 10, 2473-6988, 5332, 10.3934/math.2025246 |