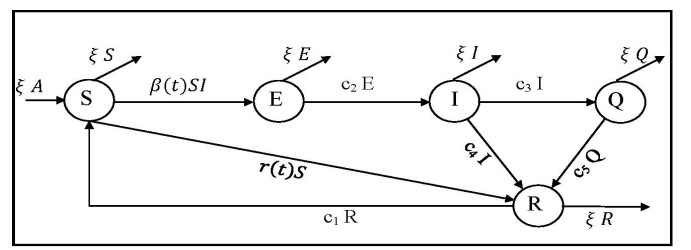
In this paper, an SEIQRS model with a periodic vaccination strategy is studied for the dynamics of the Hand-Foot-and-Mouth Disease (HFMD). This model incorporates a seasonal variation in the disease transmission rate β(t). Our model has a unique disease free periodic solution (DFPS). The basic reproductive number R0 and its lower and upper bounds, Rinf0 and Rsup0 respectively, are defined. We show that the DFPS is globally asymptotically stable when Rsup0<1 and unstable if Rinf0>1. Computer simulations of our model have been conducted using a novel periodic function of the contact rate. This novel function imitates the seasonality in the observed, multi-peaks pattern, data. Clear and good matching between real data and the obtained simulation results are shown. The obtained simulation results give a good prediction and possible control of the disease dynamics.
Citation: I. A. Moneim, G. A. Mosa. A realistic model for the periodic dynamics of the hand-foot-and-mouth disease[J]. AIMS Mathematics, 2022, 7(2): 2585-2601. doi: 10.3934/math.2022145
[1] | Nauman Ahmed, Ali Raza, Ali Akgül, Zafar Iqbal, Muhammad Rafiq, Muhammad Ozair Ahmad, Fahd Jarad . New applications related to hepatitis C model. AIMS Mathematics, 2022, 7(6): 11362-11381. doi: 10.3934/math.2022634 |
[2] | Tuersunjiang Keyoumu, Wanbiao Ma, Ke Guo . Existence of positive periodic solutions for a class of in-host MERS-CoV infection model with periodic coefficients. AIMS Mathematics, 2022, 7(2): 3083-3096. doi: 10.3934/math.2022171 |
[3] | Sireepatch Sangsawang, Usa Wannasingha Humphries, Amir Khan, Puntani Pongsumpun . Sensitivity analysis of cassava mosaic disease with saturation incidence rate model. AIMS Mathematics, 2023, 8(3): 6233-6254. doi: 10.3934/math.2023315 |
[4] | Salma M. Al-Tuwairqi, Asma A. Badrah . Modeling the dynamics of innate and adaptive immune response to Parkinson's disease with immunotherapy. AIMS Mathematics, 2023, 8(1): 1800-1832. doi: 10.3934/math.2023093 |
[5] | San-Xing Wu, Xin-You Meng . Dynamics of a delayed predator-prey system with fear effect, herd behavior and disease in the susceptible prey. AIMS Mathematics, 2021, 6(4): 3654-3685. doi: 10.3934/math.2021218 |
[6] | Ashraf Adnan Thirthar, Hamadjam Abboubakar, Aziz Khan, Thabet Abdeljawad . Mathematical modeling of the COVID-19 epidemic with fear impact. AIMS Mathematics, 2023, 8(3): 6447-6465. doi: 10.3934/math.2023326 |
[7] | Attaullah, Muhammad Jawad, Sultan Alyobi, Mansour F. Yassen, Wajaree Weera . A higher order Galerkin time discretization scheme for the novel mathematical model of COVID-19. AIMS Mathematics, 2023, 8(2): 3763-3790. doi: 10.3934/math.2023188 |
[8] | Miled El Hajji . Periodic solutions for chikungunya virus dynamics in a seasonal environment with a general incidence rate. AIMS Mathematics, 2023, 8(10): 24888-24913. doi: 10.3934/math.20231269 |
[9] | Ping Zhu . Dynamics of the positive almost periodic solution to a class of recruitment delayed model on time scales. AIMS Mathematics, 2023, 8(3): 7292-7309. doi: 10.3934/math.2023367 |
[10] | Mohamed El-Borhamy, Essam Eddin M. Rashad, Arafa A. Nasef, Ismail Sobhy, Samah M. Elkholy . On the construction of stable periodic solutions for the dynamical motion of AC machines. AIMS Mathematics, 2023, 8(4): 8902-8927. doi: 10.3934/math.2023446 |
In this paper, an SEIQRS model with a periodic vaccination strategy is studied for the dynamics of the Hand-Foot-and-Mouth Disease (HFMD). This model incorporates a seasonal variation in the disease transmission rate β(t). Our model has a unique disease free periodic solution (DFPS). The basic reproductive number R0 and its lower and upper bounds, Rinf0 and Rsup0 respectively, are defined. We show that the DFPS is globally asymptotically stable when Rsup0<1 and unstable if Rinf0>1. Computer simulations of our model have been conducted using a novel periodic function of the contact rate. This novel function imitates the seasonality in the observed, multi-peaks pattern, data. Clear and good matching between real data and the obtained simulation results are shown. The obtained simulation results give a good prediction and possible control of the disease dynamics.
Hand-foot-and-mouth disease (HFMD) is a disease that mainly affects children younger than five years of age. Symptoms start within three to six days of contact according to the Center for Disease Control and Prevention (CDC), an initial fever and generalized malaise followed by painful sores in the mouth that blister and ulcerate [1].
HFMD causes a major public health problem, especially in Asia. Although most cases of HFMD are mild, there are some severe cases that may develop heart and lung failure and probably leads to death [1,2,3]. In the last decade, many severe HFMD outbreaks have occurred in East Asia and Southeast Asia. A vaccine for HFMD was approved and applied in China in 2016. However, several HFMD outbreaks occurred recently [4,5]. This guides the decision makers to focus more on effective prevention and control strategies.
A distinct seasonal pattern in tropical and subtropical regions with more than two peaks may occur within a year [6,7,8]. Many research studies have confirmed that climatological changes in humidity and temperature have an important effect on the incidence of HFMD despite the inconsistent results from different studies [9,10,11]. Another factor that affects the variations in the number of HFMD incidences is the opening and closing of the daycare centers or schools [12,13].
Many previous efforts have been done to study the dynamics of HFMD. A number of these studies used models without any vaccination. For example, [14] and [15] used an SIR model to analyze the epidemic of HFMD in Taiwan and Malaysia respectively and they found that the disease spread quite rapidly and the number of susceptible persons is the only controllable parameter. Moreover, some studies for the dynamical behaviors of HFMD considered more complicated models including SEIQR models with and without vaccination [16,17,18,19,20,21,22,23]. For example, Zhu [23] used a SEIQR with sinusoidal periodic contact rate, to investigate the spread of seasonal HFMD in Wenzhou and he found that HFMD becomes an endemic disease in Wenzhou. Some other previous studies [17,18,24,25,26,27,28] used the sinusoidal function of period one year (β(t)=β0+βsin(ωt+ϕ)) for simulating the seasonal varying transmission rate. Unfortunately, they failed to simulate the one year multi-peaks pattern which appeared in the reported data of HFMD.
However, our study focuses on analysing and simulating the effect of the above factors and clarify their relations with the observed multi-peaks, (in a one year) pattern. We study an SEIQRS model and consider these factors in the dynamics of HFMD. We try to determine the effect of the periodic transmission and vaccination rates on the spread of HFMD.
In this paper, we formulate an SEIQRS model with seasonal contact rate and periodic vaccination strategy. We find that this model has no disease free equilibrium point. But a disease free periodic solution (DFPS) is possible for this model. The reproduction number R0 is derived. An upper bound Rsup0 and a lower bound Rinf0 of the reproduction number R0 are derived as well. This DFPS is globally asymptotically stable if Rsup0<1 and unstable when Rinf0>1.
In our simulation we use a novel form of periodic contact and vaccination rates with period one year as follows:
β(t)={β10+β11e−β12(mod(t,52))20<mod(t,52)≤26;β20+β21e−β22(mod(t,52)−26)226<mod(t,52)≤52. |
and
r(t)={ρ10+ρ11e−ρ12(mod(t,52))20<mod(t,52)≤26;ρ20+ρ21e−ρ22(mod(t,52)−26)226<mod(t,52)≤52. |
We designed these functions to fit the multi-peaks pattern of the real data. Our new form of periodic functions shows a nicer fitting with the real data, compared with the previous simulation results [23]. These more realistic simulations give a wider insight to the future of the spread of the HFMD. We plot and compare our obtained simulation results with both previous works and real data. On the other hand, our simulation results confirm our analytical results obtained in this paper.
In the paper, we shall study a more realistic SEIQRS model with periodic vaccination and loss of immunity. Periodic vaccination gives a more applicable realistic strategy to control the dynamics of the disease.
We assume that the population is mixed uniformly and homogeneously with a constant population size A. The population number A is divided into five groups, S(t), E(t), I(t), Q(t), and R(t) which represent the susceptible, exposed, infected, quarantined, and recovered individuals respectively. Figure 1 illustrates the flowchart of the dynamics of the spread of HFMD. This figure describes the flow of inputs/outputs into each of the five compartments of our model. A proportion of the susceptible individuals who have received the vaccine move to the recovered group, the rest get infected and join the latent period or died for any reason. on the other hand, there is a flux of newborns entering the susceptible class. The latent population in their latency period do not show any symptom. Some of the latent died naturally but after about 3∼7 days the latent individuals become infectious. A part of the infectious people will be hospitalized for treatment and isolated then they get recovered or died. Another proportion of those infectious moves to the recovery class, while some have died. Finally, some of the recovered lose their immunity and join the susceptibles again, another proportion died.
The model parameters are defined and listed as follows :
Parameter | Definition |
β(t) | The annual periodic transmission rate |
r(t) | The time dependent annual periodic vaccination rate |
c1 | The progression rate of the recovered (by losing immunity). |
1c2 | The average incubation period |
c3 | The quarantine rate |
c4 | The infectious recovery rate |
c5 | The quarantined recovery rate |
ξ | The natural birth and death rate |
Note that, β(t) and r(t) are time dependent bounded seasonally varied functions with period one year and all parameter values are non negative. Using our assumptions and following [9,18,19] we can write our SEIQRS model as a set of five coupled non-linear ordinary differential equations as follows:
dS(t)dt=ξA−β(t)I(t)S(t)−(ξ+r(t))S(t)+c1R(t), | (2.1) |
dE(t)dt=β(t)I(t)S(t)−(ξ+c2)E(t), | (2.2) |
dI(t)dt=c2E(t)−(ξ+c3+c4)I(t), | (2.3) |
dQ(t)dt=c3I(t)−(ξ+c5)Q(t), | (2.4) |
anddR(t)dt=r(t)S(t)+c4I(t)+c5Q(t)−(ξ+c1)R(t), | (2.5) |
withS(t)+E(t)+I(t)+Q(t)+R(t)=A. |
Equations (2.1)–(2.5) represent a nonlinear first order system of differential equations that describes the spread of HFMD disease.
The disease transmission rate β(t) and the vaccination rate r(t) are nonconstant, positive, and bounded periodic functions. These periodic functions β(t) and r(t) are designed to imitate the seasonal variation in the reported cases for HFMD infectious disease due to climatological changes or opening and closing schools [12,13].
As we assume that, the vaccination rate r(t) is a nonconstant function therefore, the system (2.1)–(2.5) could not have any equilibrium point. On the other hand, a disease free periodic solution (DFPS) is still possible when E(t)=I(t)=Q(t)=0.
Define the region D⊆R5 where,
D={(S,E,I,Q,R)∈[0,A]5:S+E+I+Q+R=A}. |
The five dimensional set D, is obviously positively invariant, as the right-hand side of the system (2.1)–(2.5) is differentiable.
In our model, the vaccination rate r(t) is a periodic function, this force the system (2.1)–(2.5) to have no equilibrium point. On the other hand we expect that, there is a disease free periodic solution (DFPS) for the system (2.1)–(2.5) at (ˆS(t),0,0,0,ˆR(t)). Similar to [29,30,31] this DFPS is given by
dSdt=Aξ−(ξ+r(t))S(t)+c1R(t)=A(ξ+c1)−(ξ+r(t)+c1)S. | (3.1) |
with the initial condition S(t0)∈R+, integrating equation (3.1) we get,
S(t)=(S(t0)+A(ξ+c1)∫tt0e((ξ+c1)(κ−t0)+∫κt0r(τ)dτ)dκ)e((ξ+c1)(t−t0)−∫tt0r(τ)dτ) | (3.2) |
So,
S(t0+(n+1)T)=(S(t0+nT)+A(ξ+c1)∫t0+Tt0e((ξ+c1)(κ−t0)+∫κt0r(τ)dτ)dκ)e((ξ+c1)T+∫t0+Tt0r(τ)dτ) | (3.3) |
Equation (3.3) represents a recurrence relation between the susceptibles at times (t0+nT;n=1,2,....). Now define the mapping G such that
G(Sn)=Sn+1 |
where Sn=S(t0+nT). If Sn1≠Sn2 we have that,
|G(Sn1)−G(Sn2)|≤|Sn1−Sn2|exp(−ξT). |
Therefore, the mapping G is a contraction mapping and it has a unique fixed point S∗ [32]. Moreover S∗; is depending on t0; such that,
S∗(t0)=A(ξ+c1)∫t0+Tt0e((ξ+c1)(κ−t0)+∫κt0r(τ)dτ)dκe((ξ+c1)T+∫t0+Tt0r(τ)dτ)−1. | (3.4) |
Hence, S∗(t0+T)=S∗(t0). So, S∗ is a periodic function of t. From (3.4), we have that, S∗(t0) is continuously differentiable with respect to t0. On the other hand, we have
∫tt0e((ξ+c1)(κ−t0)+∫κt0r(τ)dτ)dκ⩽∫tt0e((ξ+c1)(κ−t0)+∫κt0r(τ)dτ)(ξ+c1+r(κ))dκξ+c1+rmin=e(ξ+c1)(t−t0)+∫tt0r(τ)dτ−1ξ+c1+rmin, | (3.5) |
where rmin=mint∈[0,T]r(t). Substituting (3.4) and (3.5) into (3.2), we obtain
S∗(t)⩽A(ξ+c1)ξ+c1+rmin(1+(e(ξ+c1)(t−t0)+∫tt0(r(τ))dτ−1))e(ξ+c1)(t−t0)+∫tt0r(τ)dτ=A(ξ+c1)ξ+c1+rmin<A |
Hence, ˆS(t)=S∗(t),ˆE(t)=ˆI(t)=ˆQ(t)=0, and ˆR(t)=R∗(t)=A−S∗(t) is a disease-free periodic solution of the system (2.1)–(2.5).
The basic reproduction number R0 is defined as the average number of new cases produced by a single infected individual entered into a pure susceptible population. Now, we can drive a form of the basic reproduction number R0 of the system (2.1)–(2.5) using a similar scenario to [33,34]. By linearizing the system (2.1)–(2.5) around (ˆS(t),0,0,0,ˆR(t)), we find that:
dE(t)dt=β(t)I(t)ˆS(t)−(ξ+c2)E(t), | (4.1) |
dI(t)dt=c2E(t)−(ξ+c3+c4)I(t), | (4.2) |
anddQ(t)dt=c3I(t)−(ξ+c5)Q(t), | (4.3) |
which can be written in the matrix form as:
dxdt=(F(t)−V)x |
where,
x=(EIQ), F(t)=(0β(t)ˆS(t)0000000)andV=(c2+ξ00−c2c3+c4+ξ00−c3c5+ξ) |
Now, let y(t)=β(t)ˆS(t). Note that y(t) is a nonzero positive periodic function with period T, so ¯y(t)=1T∫T0β(τ)ˆS(τ)dτ is average of Y(t) over the time T. Now, arguing similar to [33], we can obtain the value of the basic reproduction number R0 as follows:
R0=1T∫T0c2β(τ)ˆS(τ)dτ(ξ+c2)(ξ+c3+c4)=c2¯y(t)(ξ+c2)(ξ+c3+c4) | (4.4) |
where ˆS(t) is a periodic solution of period T and it is the only disease free solution of the system of differential equations (2.1)–(2.5).
Now, we can define Rsup0 as an upper bound and Rinf0 a lower one for the value R0 respectively where,
Rsup0=c2(ξ+c2)(ξ+c3+c4)supt∈[0,T]∫T0(ξ+c2)β(t−ϕ)ˆS(t−ϕ)e−(ξ+c2)ϕdϕ1−e−(ξ+c2)T | (4.5) |
and
Rinf0=c2(ξ+c2)(ξ+c3+c4)inft∈[0,T]∫T0(ξ+c2)β(t−ϕ)ˆS(t−ϕ)e−(ξ+c2)ϕdϕ1−e−(ξ+c2)T | (4.6) |
In this section, we extend the results obtained by [29,30,31]. We show first that if Rsup0<1 the DFPS (ˆS(t),0,0,0,ˆR(t)) is globally asymptotically stable. In another section we shall prove that the DFPS is unstable when Rinf0>1.
Now, we show that the DFPS is globally asymptotically stable when Rsup0<1. We use an argument similar to that used in the proof of Theorem 1 [31] with some modifications to fit our SEIQRS model.
Lemma 1.
lim supt→∞(S(t)−ˆS(t))≤0. |
Proof
From Equation (2.1)
dSdt=ξA−β(t)SI−(ξ+r(t))S+c1R,≤A(ξ+c1)−(ξ+r(t)+c1)S. |
As (ˆS(t),0,0,0,ˆR(t)) is a solution of the system (2.1)–(2.5) when E(t)=I(t)=Q(t)=0 then arguing similar to [31] we deduce that,
lim supt→∞(S(t)−ˆS(t))≤0, |
and the proof of Lemma 1 is completed.
Now we go directly to the first main result which is the global stability of the disease free solution when Rsup0<1. Theorem 1 proves that DFPS is globally asymptotically stable (GAS) when Rsup0<1. Following Theorem 1 in [31], we give proof of the next Theorem.
Theorem 1. The DFPS, (ˆS,0,0,0,ˆR) is GAS if Rsup0<1,
Proof
From Equations (2.3) and (2.4) we have
dI(t)dt=c2E(t)−(ξ+c3+c4)I(t), |
and
dQ(t)dt=c3I(t)−(ξ+c5)Q(t), |
arguing similar to [31] and [35] we can easily find that
I∞≤c2E∞(ξ+c3+c4). |
and
Q∞≤c3I∞(ξ+c5)≤c3c2E∞(ξ+c5)(ξ+c3+c4). |
The idea behind this proof is simple as that, from Lemma 1 we have that, given ϵ>0 there exists t1 such that S(t)≤ˆS(t)+ϵ, I(t)≤I∞+ϵ and Q(t)≤Q∞+ϵ for all t≥t1.
Using Eq (2.2), E(t) can be bounded above, then we use this upper bound to show that E∞=0. Now similar to Theorem 1 [31], suppose that E∞>0. By integrating Eq (2.2) we have that for t≥t0>t1,
E(t)≤E(t0)e−(ξ+c2)(t−t0)+(I∞+ϵ)e−(ξ+c2)t∫tt0β(η)(ˆS(η)+ϵ)e(ξ+c2)ηdη. | (5.1) |
Now, recall that, y(η)=β(η)ˆS(η). Therefore,
e−(ξ+c2)t∫tt0y(η)e(ξ+c2)ηdη=∫t−t00y(t−u)e−(ξ+c2)udu | (5.2) |
Suppose that (k+1)T≥t−t0≥kT, then we have,
∫t−t00y(t−u)e−(ξ+c2)udu=∫T0y(t−u)e−(ξ+c2)udu(1+e−(ξ+c2)T+e−(μ+σ)2T+⋯+e−(ξ+c2)(k−1)T)+∫t−t0kTy(t−u)e−(ξ+c2)udu≤∫T0y(t−u)e−(ξ+c2)udu(1+e−(ξ+c2)T+e−(ξ+c2)2T+⋯+e−(ξ+c2)kT)<∫T0y(t−ζ)e−(ξ+c2)ζdζ1−e−(ξ+c2)T | (5.3) |
So, for t≥t0 we find that
E(t)≤E(t0)e−(ξ+c2)(t−t0)+I∞+ϵξ+c2∫T0(ξ+c2)β(t−ζ)(ˆS(t−ζ)+ϵ)e−(ξ+c2)ζdζ1−e−(ξ+c2)T≤Ae−(ξ+c2)(t−t0)+I∞+ϵξ+c2(supt∈[0,T]∫T0(ξ+c2)y(t−ζ)e−(ξ+c2)ζdζ1−e−(ξ+c2)T+ϵ∫T0(ξ+c2)β(t−ζ)e−(ξ+c2)ζdζ1−e−(ξ+c2)T) | (5.4) |
Choose t2>t0 large enough so that for t≥t2; Ae−(ξ+c2)(t−t0)<ϵ: Then for t≥t2,
E(t)≤Rsup0E∞+ϵ(1+supt∈[0,T]∫T0y(t−ζ)e−(ξ+c2)ζdζ1−e−(ξ+c2)T+(I∞+ϵξ+c2)βmax), | (5.5) |
where βmax=supu∈[0,T]β(u). Now choose ϵ small enough so that,
ϵ(1+supt∈[0,T]∫T0y(t−ζ)e−(ξ+c2)ζdζ1−e−(ξ+c2)T+(I∞+ϵξ+c2)βmax)<ψE∞, | (5.6) |
where Rsup0+ψ<1 and ψ>0. Hence for t≥t2, we find that E(t)≤(Rsup0+ψ)E∞. Thus, 0≤E∞≤(Rsup0+ψ)E∞. then ,E∞=0. Hence also I∞=0 this leads to Q∞=0 Moreover E(t)→0, I(t)→0 and Q(t)→0 as t→∞. Finally we go to show that (S(t)−ˆS(t))→0 and (R(t)−ˆR(t))→0 as t→∞. As ˆR(t) is a solution of Eq (2.5) when E(t)=I(t)=Q(t)=0, we find that,
d(R−ˆR)dt=r(t)(S−ˆS)+c4I+c5Q−(ξ+c1)(R−ˆR). | (5.7) |
Given ϵ1>0 using Lemma 1, there exists t3 such that E(t)+I(t)+Q(t)≤ϵ1 and S(t)≤S(t)+ϵ1 for all t≥t3. So for t≥t3
d(R−R)dt≤(rmax+c4+c5)ϵ1−(ξ+c1)(R−ˆR) |
where rmax=supu∈[0,T]r(u). By integrating this inequality, we have that
(R(t)−ˆR(t))≤(R(t3)−ˆR(t3))e−(ξ+c1)(t−t3)+ϵ1(rmax+c4+c5ξ+c1)(1−e−(ξ+c1)(t−t3))≤Ae−(ξ+c1)(t−t3)+ϵ1(rmax+c4+c5ξ+c1) |
Now we can show that, given ϵ2>0, there exists t4 such that R(t)−ˆR(t)≤ϵ2 for t≥t4. Therefore, S(t)=A−R(t)−Q(t)−I(t)−E(t)≥A−ˆR(t)−ϵ1−ϵ2 for t≥t4. From Lemma 1, we find that S(t)→ˆS(t) as t→∞. Since R(t)=A−S(t)−I(t)−Q(t)−E(t), then we must have, R(t)→A−ˆS(t)=ˆR(t) at t→∞. This completes the proof of Theorem 1. Thus if Rsup0<1, then DFPS is GAS.
Here Rinf0 is a lower bound for R0. We use an argument consisting of a mixture of those used in [30], [36], and [37]. So, we can show that if Rinf0>1 the (DFPS) is not stable and the disease will fire up.
The first case when the infection is not present initially, I(0)=E(0)=Q(0)=0 and S+R=A, then by Theorem 1, the system (2.1)–(2.5) tends to the DFPS as t→∞ whatever the value of R0. The second case is that, all of E(0)>0, I(0)>0, and Q(0)>0 we shall prove that DFPS is unstable by using an argument similar to [30] and [31].
Theorem 2. If Rinf0>1, then the DFPS is unstable.
Proof
In this case, we suppose that the DFPS is stable when Rinf0>1 and we get a contradiction with our assumption. So if (S,E,I,Q,R) starts near (ˆS,0,0,0,ˆR) we have that (S,E,I,Q,R) must stay near (ˆS,0,0,0,ˆR) for the time being large. Therefore (S,E,I,Q,R)→(ˆS,0,0,0,ˆR) as t→∞. So, given ε=(1/2)(1−(1/Rinf0))>0 there exists t1>0 such that |S(t)−ˆS|≤εˆS, |E(t)−0|≤ε, |I(t)−0|≤ε, |Q(t)−0|≤ε and |R(t)−ˆR|≤ε for all t≥t1. Choose ε1>0 such that (Rinf0+1)(1−ε1)>2.
From Eq (4.6) recall Rinf0,
Rinf0=c2(ξ+c2)(ξ+c3+c4)inft∈[0,T]∫T0(ξ+c2)y(t−ϕ)e−(ξ+c2)ϕdϕ1−e−(ξ+c2)T, |
Therefore, there exists an integer k such that
c2(ξ+c2)(ξ+c3+c4)inft∈[0,T]∫T0(ξ+c2)y(t−ϕ)e−(ξ+c2)ϕdϕ(1+e−(ξ+c2)T+e−(ξ+c2)2T+⋯+e−(ξ+c2)(k−1)T)>Rinf0(1−ε1). | (5.8) |
Choose t2≥t1 such that, E(t2)≥E(0)e−(ξ+c2)t2>0, I(t2)≥I(0)e−(ξ+c3+c4)t2>0 and Q(t2)≥Q(0)e−(ξ+c5)t2>0 as E(0)>0, I(0)>0 and Q(0)>0. Now define ε2 and η as follows:
0<ε2<min{12I(t2)e−(ξ+c3+c4)kT,(ξ+c5)2c3Q(t2)e−(ξ+c5)kT,c22(ξ+c3+c4)E(t2)e−(ξ+c2)kT}, |
η=inf{ϕ≥0:I(t2+δ)≥ε2,Q(t2+δ)≥c3(ξ+c5)ε2andE(t2+δ)≥ξ+c3+c4c2ε2,forδ∈[0,ϕ]}. |
By continuity η>0 and if η<∞ then I(t2+η)=ε2, Q(t2+η)=(c3/(ξ+c5))ε2 or E(t2+η)=((ξ+c3+c4)/c2)ε2. This leads to a contradiction.
Now from Eq (2.3) and by the definition of ε2 we find that,
I(t2+η)=I(t2)e−(ξ+c3+c4)η+e−(ξ+c3+c4)(t2+η)∫t2+ηt2c2E(χ)e(ξ+c3+c4)χdχ,≥I(t2)e−(ξ+c3+c4)η+ε2(1−e−(ξ+c3+c4)η)>ε2, |
similarly, from Eq (2.4) we find that,
Q(t2+η)=Q(t2)e−(ξ+c5)η+e−(ξ+c5)(t2+η)∫t2+ηt2c3I(χ)e(ξ+c5)χdχ,≥Q(t2)e−(ξ+c5)η+ε2(1−e−(ξ+c5)η)>c3(ξ+c5)ε2, |
but on the other hand from Eq (2.2) we find that
E(t2+η)≥E(t2)e−(ξ+c2)η+e−(ξ+c2)(t2+η)∫t2+ηt2β(χ)S(χ)I(χ)e(ξ+c2)χdχ,≥E(t2)e−(ξ+c2)η+e−(ξ+c2)(t2+η)ε2(1−ε)∫t2+ηt2y(χ)e(ξ+c2)χdχ. | (5.9) |
Now
e−(ξ+c2)(t2+η)∫t2+ηt2y(χ)e(ξ+c2)χdχ=∫t2+ηt2y(χ)e−(ξ+c2)(t2+η−χ)dχ,=∫η0y(t2+η−ϕ)e−(ξ+c2)ϕdϕ. |
If η≤kT then
E(t2+η)≥E(t2)e−(ξ+c2)kT>(ξ+c3+c4)c2ε2. |
If η≥kT then
∫η0y(t0+η−ϕ)e−(ξ+c2)ϕ]dϕ=∫T0y(t0+η−ϕ)e−(ξ+c2)ϕdϕ(1+e−(ξ+c2)T+e−(ξ+c2)2T+⋯+e−(ξ+c2)(k−1)T)+∫ηkTe−(ξ+c2)ϕdϕ,≥inft∈[0,T]∫T0y(t−ϕ)e−(ξ+c2)ϕdϕ(1+e−(ξ+c2)T+e−(ξ+c2)2T+⋯+e−(ξ+c2)(k−1)T),>ξ+c3+c4c2Rinf0(1−ϵ1),usingEq(5.8). |
As Rinf0>1 and ε=(Rinf0−1)2Rinf0>0 then using Eq (5.9)
E(t2+η)≥E(t2)e−(ξ+c2)η+ε212(1Rinf0+1)ξ+c3+c4c2Rinf0(1−ε1),=E(t2)e−(ξ+c2)η+(ξ+c3+c4)2c2ε2(Rinf0+1)(1−ε1),>(ξ+c3+c4)c2ε2,as$(Rinf0+1)(1−ε1)>2$. |
Hence η<∞ leads to a contradiction so η=∞ and I(t2+δ)≥ε2, Q(t2+δ)≥(c3/(ξ+c5))ε2 and E(t2+δ)≥((ξ+c3+c4)/c2)ε2 for all δ≥0. This contradicts the fact that the trajectory tends to the DFPS. Hence the DFPS cannot be stable for Rinf0>1, and the proof is completed.
These results prove that the disease free solution for our SEIQRS model represented by the system (2.1)–(2.5) with a nonconstant periodic transmission and vaccination rates r(t) in [0,T], is globally asymptotically stable if Rsup0<1 and not stable if Rinf0>1. from these results we deduce that,
supt∈[0,T]∫T0y(t−ϕ)e−(ξ+c2)ϕdϕ1−e−(ξ+c2)T<ξ+c3+c4c2, | (5.10) |
is the sufficient condition to keep the DFPS globally asymptotically stable.
The obtained results, for our SEIQRS model with nonconstant periodic vaccination rate r(t), extend the corresponding results obtained by [30], [31], [37], and [35]. These results are original for an SEIQRS model with periodicity in the transmission and vaccination rates.
Now we look numerically at the behavior of the system (2.1)–(2.5). The software package Mathematica12 is used to solve our system of nonlinear ordinary differential equations (2.1)–(2.5). Real data about HFMD in Egypt dose not available. So we use data taken from the district of Wenzhou China (Table 1 [23]). We choose Wenzhou China as a subtropical area that is similar to the climate of Egypt. Simulations are performed using parameters from the literature [23]. We compare our simulation results with the reported data of HFMD in Wenzhou from March 2010, to December 2013. Also, we compare our results with the simulation results of [23].
The parameters estimated in [23] are used in our simulation to give a fair comparison with their results. The parameters are set to the following values:
Parameter | value |
c1 | 0.015. |
1c2 | 0.571 |
c3 | 0.007177 |
c4 | 0.875 |
c5 | 0.4375 |
ξ | 0.00642 |
As stated before our novel periodic transmission rate β(t) is designed to fit the yearly multi-peaks pattern in the reported data. Recall β(t) as follows:
β(t)={β10+β11e−β12(mod(t,52))20<mod(t,52)≤26;β20+β21e−β22(mod(t,52)−26)226<mod(t,52)≤52;. |
By using the least-square fitting of the numbers of I(t), we can estimate the values of βij:i,j=0,1,2.
Figure 2 shows that our simulation results are often closer to the real data than the results obtained by [23]. Clear multiple peaks, within a year, are appeared in our simulation which gives a good fitting to the real data. These results show that our model is more realistic than [23]. In Figure 2 we use the parameters' values of the transmission periodic function which are estimated from the least square fitting of I(t) during the period from 2010 to 2013, as follows: β10 = 0.64, β11 = 0.665, β12 = 0.0035, β20 = 0.63, β21 = 0.445, β22 = 0.0017.
Figure 3 shows that there is a decrease in the height of the peaks of the number of infected persons I(t) over time. However, this solution has a one year multi-peaks similar to those of the reported real data. Figure 3 predicts that the number of the new HFMD cases will fluctuate around a decreasing level.
Figure 4 plots the number of infected against time in weeks when R0>1. This figure shows that our simulation results are always very close to the reported data. Our simulation results give a more precise fitting than the results of [23]. Our model produces a multi-peaks, in one period, solution while the model in [23] has a mono peak carve. In Figure 4 the parameters of the transmission periodic function are given estimated values from the least square fitting of I(t) from 2011 to 2012, as follows: β10 = 0.49, β11 = 0.94, β12 = 0.0031, β20 = 0.36, β21 = 0.70, β22 = 0.00065.
Figure 5 shows that there is a stable endemic periodic solution of our model, with a period of one year, when the basic reproductive number (R0>1). This means that the HFMD will take off and becomes endemic when R0 is larger enough than one in value.
Now, when we apply the vaccination strategy of the form:
r(t)={ρ10+ρ11e−ρ12(mod(t,52))20<mod(t,52)≤26;ρ20+ρ21e−ρ22(mod(t,52)−26)226<mod(t,52)≤52;. |
Where the parameters of the vaccination function r(t) are given the following values: ρ10 = 0.00098, ρ11 = 0.00188, ρ12 = 0.0031, ρ20 = 0.00072, ρ21 = 0.0014, ρ22 = 0.00065. In this case, we find that the susceptibles and the infected populations tend to the DFPS as shown in Figure 5.
Figure 6 (a) shows that in the absence of vaccination the HFMD fire up when R0>1. While applying our vaccination strategy forces the disease to die out and (b) show that without vaccination the susceptible population stays high enough to provide a flow of new case which give the disease the chance to persist in the population. On the other hand, applying our vaccination strategy forces the susceptibles to be small enough to fluctuate close to the DFPS and keep R0 to be less than one.
We study an SEIQRS model for the dynamics of HFMD. Our model introducing a seasonality in both the contact and vaccination rates. In section 1 we give a brief introduction to the dynamics of HFMD as a public health problem. In section 2 we formulate our model and define the model parameters. The exitance of the DFPS is proved in section 3 and the formula of the basic reproduction number R0 is derived in section 4. We define an upper bound of Rsup0 and a lower bound Rinf0 in this section as well. We use these lower and upper bounds to prove that, the disease free periodic solution DFPS, is globally asymptotically stable when Rsup0<1 and unstable if Rinf0>1. Section 5 contains the stability results. We use our novel form of periodic contact and vaccination rates during all of our simulations in section 6. Previous works used a sinusoidal form of periodic transmission rate which is failed to simulate the yearly multi-peaks pattern in the reported data. We claim that our suggested transmission periodic function forces our model to have a multi-peaks pattern. This result gives our model more realistic behaviours and produces more accurate simulation results. Our simulation results are original for this kind of periodic functions.
In our study, the population is assumed to be homogeneously mixed and has a constant size. This assumption is unrealistic, so in future work, one can use network epidemic models to simulate the fact that, peoples have different chances to catch the disease [38,39,40]. Another future work is to study the behaviour of the endemic solution when the reproduction number R0>1.
This project is funded by the Academy of Scientific Research and Technology (ASRT), Egypt, Grant No(6739), (ASRT) is the 2nd affiliation of this research.
The authors declare that they have no competing interests.
[1] | Hand, Foot, and Mouth Disease (HFMD). Available from: https://www.cdc.gov/hand-foot-mouth/index.html. |
[2] | J. Wang, T. Hu, D. Sun, S. Ding, M. J. Carr, W. Xing, et al., Epidemiological characteristics of hand, foot, and mouth disease in Shandong, China, 2009-2016, Sci Rep 7, 8900 (2017). doi: 10.1038/s41598-017-09196-z. |
[3] |
K. Kaminska, G. Martinetti, R. Lucchini, G. Kaya, C. Mainetti, Coxsackievirus A6 and hand, foot, and mouth disease: three case reports of familial child-to-immunocompetent adult transmission and a literature review, Case Rep. Dermatol., 5 (2013), 203–209. doi: 10.1159/000354533. doi: 10.1159/000354533
![]() |
[4] |
Q. Y. Mao, Y. Wang, L. Bian, M. Xu, Zh. Liang, EV71 vaccine, a new tool to control outbreaks of hand, foot and mouth disease (HFMD), Expert Rev. Vacc., 15 (2016), 599–606. doi: 10.1586/14760584.2016.1138862. doi: 10.1586/14760584.2016.1138862
![]() |
[5] |
B. Yang, F. Liu, Q. Liao, P. Wu, Zh. Chang, J. Huang, et al., Epidemiology of hand, foot and mouth disease in China, 2008 to 2015 prior to the introduction of EV-A71 vaccine, Euro Surveill., 22 (2017), 1–10. doi: 10.2807/1560-7917.ES.2017.22.50.16-00824. doi: 10.2807/1560-7917.ES.2017.22.50.16-00824
![]() |
[6] |
F. Gou, X. Liu, J. He, D. Liu, Y. Cheng, H. Liu, et al., Different responses of weather factors on hand, foot and mouth disease in three different climate areas of Gansu, China, BMC Infect. Dis., 18 (2018), 15. doi: 10.1186/s12879-017-2860-4. doi: 10.1186/s12879-017-2860-4
![]() |
[7] |
J. Jia, F. Kong, X. Xin, J. Liang, H. Xin, L. Dong, et al., Epidemiological Characteristics of Hand, Foot, and Mouth Disease Outbreaks in Qingdao, 2009-2018, Iran J Public Health, 50 (2021), 999–1008. doi:10.18502/ijph.v50i5.6117. doi: 10.18502/ijph.v50i5.6117
![]() |
[8] |
P. Wang, H. Zhao, F. You, H. Zhou, W. B. Goggins, Seasonal modeling of hand, foot, and mouth disease as a function of meteorological variations in Chongqing, China Int. J. Biometeorol, 61 (2017), 1411–1419. doi: 10.1007/s00484-017-1318-0. doi: 10.1007/s00484-017-1318-0
![]() |
[9] |
C. X. Dai, Z. Wang, W. M. Wang, Y. Q. Li, K. F. Wang, Epidemics and underlying factors of multiple-peak pattern on hand, foot and mouth disease in Wenzhou, China, Math. Biosci. Eng., 16 (2019), 2168–2188. doi: 10.3934/mbe.2019106. doi: 10.3934/mbe.2019106
![]() |
[10] |
Y. L. Hii, J. Rocklov, N. Ng, Short term effects of weather on hand, foot and mouth disease, PLoS ONE, 6 (2011), 1–6. doi: 10.1371/journal.pone.0016796. doi: 10.1371/journal.pone.0016796
![]() |
[11] |
W. Dong, X. Li, P. Yang, H. Liao, X. Wang, Q. Wang, The effects of weather factors on hand, foot and mouth disease in Beijing, Sci. Rep., 6 (2016), 1–9. doi: 10.1038/srep19247. doi: 10.1038/srep19247
![]() |
[12] |
L. Sun, H. Lin, J. Lin, J. He, A. Deng, M. Kang, et al., Evaluating the transmission routes of hand, foot, and mouth disease in Guangdong, China, Am. J. Infect. Control., 44 (2016), e13–e14. doi: 10.1016/j.ajic.2015.04.202. doi: 10.1016/j.ajic.2015.04.202
![]() |
[13] |
Y. H. Xie, V. Chongsuvivatwong, Y. Tan, Zh. Zh. Tang, V. Sornsrivichai, E. B. McNeil, Important roles of public playgrounds in the transmission of hand, foot, and mouth disease, Epidemiol. Infect., 143 (2015), 1432–1441. doi: 10.1017/S0950268814002301. doi: 10.1017/S0950268814002301
![]() |
[14] | Y. C. Wang, F. C. Sung, Modeling the infections for Enteroviruses in Taiwan, Institute of Environmental Health, China Medical University College of Public Health, Taipei, 2004. |
[15] | F. C. S. Tiing, J. Labadin, A simple deterministic model for the spread of hand, foot and mouth disease (HFMD) in Sarawak, 2008 Second Asia International Conference on Modelling and Simulation, (2008), 947–952. doi: 10.1109/AMS.2008.139. |
[16] | N. Roy, Mathematical modeling of hand-foot-mouth disease: quarantine as a control measure, Int. J. Adv. Sci. Eng. Technol. Res., 1 (2012), 1–11. |
[17] |
Y. Ma, M. Liu, Q. Hou, J. Zhao, Modelling seasonal HFMD with the recessive infection in Shandong, China, Math. Biosci. Eng., 10 (2013), 1159–1171. doi: 10.3934/mbe.2013.10.1159. doi: 10.3934/mbe.2013.10.1159
![]() |
[18] |
J. Liu, Threshold dynamics for a HFMD epidemic model with periodic transmission rate, Nonlinear Dynam., 64 (2011), 89–95. doi: 10.1007/s11071-010-9848-6. doi: 10.1007/s11071-010-9848-6
![]() |
[19] |
J. Y. Yang, Y. Chen, F. Q. Zhang, Stability analysis and optimal control of a hand-foot-mouth disease (HFMD) model, J. Appl. Math. Comput., 41 (2013), 99–117. doi: 10.1007/s12190-012-0597-1. doi: 10.1007/s12190-012-0597-1
![]() |
[20] |
G. P. Samanta, Analysis of a delayed hand-foot-mouth disease epidemic model with pulse vaccination, Syst. Sci. Control Eng., 2 (2014), 61–73. doi: 10.1080/21642583.2014.880827. doi: 10.1080/21642583.2014.880827
![]() |
[21] |
R. Viriyapong, S. Wichaino, Mathematical modeling of hand, foot and mouth disease in the Northern Thailand, Far East J. Math. Sci., 100 (2016), 805–820. doi: 10.17654/MS100050805. doi: 10.17654/MS100050805
![]() |
[22] |
S. Sharma, G. P. Samanta, Analysis of a hand-foot-mouth disease model, Int. J. Biomath., 10 (2017), 1750016. doi: 10.1142/S1793524517500164. doi: 10.1142/S1793524517500164
![]() |
[23] |
Y. Zhu, B. Xu, X. Lian, W. Lin, Z. Zhou, W. Wang, A hand-foot-and-mouth disease model with periodic transmission rate in Wenzhou, China, Abstr. Appl. Anal., 2014 (2014), 1–11. doi: 10.1155/2014/234509. doi: 10.1155/2014/234509
![]() |
[24] |
J. Ma, Z. Ma, Epidemic threshold conditions for seasonally forced SEIR models, Math. Biosci. Eng., 3 (2006), 161–172. doi: 10.3934/mbe.2006.3.161. doi: 10.3934/mbe.2006.3.161
![]() |
[25] |
I. A. Moneim, D. Greenhalgh, Use of a periodic vaccination strategy to control the spread of epidemics with seasonally varying contact rate, Math. Biosci. Eng., 2 (2005), 591–611. doi: 10.3934/mbe.2005.2.591. doi: 10.3934/mbe.2005.2.591
![]() |
[26] |
J. Zhang, Z. Jin, G. Q. Sun, X. D. Sun, S. Ruan, Modeling seasonal rabies epidemics in China, Bull. Math. Biol., 74 (2012), 1226–1251. doi: 10.1007/s11538-012-9720-6. doi: 10.1007/s11538-012-9720-6
![]() |
[27] |
J. Wang, Y. Xiao, R. A. Cheke, Modelling the effects of contaminated environments in mainland China on seasonal HFMD infections and the potential benefit of a pulse vaccination strategy, Discrete Cont. Dyn-B, 24 (2019), 5849–5870. doi: 10.3934/dcdsb.2019109. doi: 10.3934/dcdsb.2019109
![]() |
[28] |
Z. Ding, Y. Li, Y. Cai, Y. Dong, W. Wang, Optimal Control Strategies of HFMD in Wenzhou, China, Complexity, 2020 (2020), 1–5. doi: 10.1155/2020/5902698. doi: 10.1155/2020/5902698
![]() |
[29] |
I. A. Moneim, An SEIR Model with Infectious Latent and aPeriodic Vaccination Strategy, Math. Model. Anal., 26 (2021), 236–252. doi: 10.3846/mma.2021.12945. doi: 10.3846/mma.2021.12945
![]() |
[30] |
I. A. Moneim, Modeling and simulation of the spread of H1N1 flu with periodic vaccination, Int. J. Biomath., 9 (2016), 1650003. doi: 10.1142/S1793524516500030. doi: 10.1142/S1793524516500030
![]() |
[31] |
I. A. Moneim, D. Greenhalgh, Threshold and stability results for an SIRSepidemic model with a general periodic vaccination strategy, J. Biol. Syst., 13 (2005), 131–150. doi: 10.1142/S0218339005001446. doi: 10.1142/S0218339005001446
![]() |
[32] | T. A. Burton, Stability and periodic solutions of ordinary and functional differential equations, Academic Press, New York, 1985. |
[33] |
W. Wang, XQ. Zhao, Threshold Dynamics for Compartmental Epidemic Models in Periodic Environments, J. Dyn. Diff. Equat., 20 (2008), 699–717. doi: 10.1007/s10884-008-9111-8. doi: 10.1007/s10884-008-9111-8
![]() |
[34] |
N. Bacaer, S. Guernaoui, The epidemic threshold of vector-borne diseases with seasonality, J. Math. Biol., 53 (2006), 421–436. doi: 10.1007/s00285-006-0015-0. doi: 10.1007/s00285-006-0015-0
![]() |
[35] |
D. Greenhalgh, I. A. Moneim, SIRS epidemic model and simulations using different types of seasonal contact rate, Systems Analysis Modelling Simulation, 43 (2003), 573–600. doi: 10.1080/023929021000008813. doi: 10.1080/023929021000008813
![]() |
[36] |
I. A. Moneim, Seasonally varying epidemics with and without latent period: a comparative simulation study, Math. Med. Biol., 24 (2007), 1–15. doi:10.1093/imammb/dql023. doi: 10.1093/imammb/dql023
![]() |
[37] |
I. A. Moneim, H. A. Khalil1, Modelling and Simulation of the Spread of HBV Disease with Infectious Latent, Appl. Math., 6 (2015), 745–753. doi: 10.4236/am.2015.65070. doi: 10.4236/am.2015.65070
![]() |
[38] |
Y. Wang, J. Cao, Global dynamics of a network epidemic model for waterborne diseases spread, Appl. Math. Comput., 237 (2014), 474–488. doi: 10.1016/j.amc.2014.03.148. doi: 10.1016/j.amc.2014.03.148
![]() |
[39] |
R. Pastor Satorras, C. Castellano, P. Van Mieghem, A. Vespignani, Epidemic processes in complex networks, Rev. Mod. Phys., 87 (2015), 925–979. doi: 10.1103/RevModPhys.87.925. doi: 10.1103/RevModPhys.87.925
![]() |
[40] |
Y. Wang, J. Cao, Final size of network epidemic models: properties and connections, Sci. China Inf. Sci., 64 (2021), 179201. doi: 10.1007/s11432-019-2656-2. doi: 10.1007/s11432-019-2656-2
![]() |
Parameter | Definition |
β(t) | The annual periodic transmission rate |
r(t) | The time dependent annual periodic vaccination rate |
c1 | The progression rate of the recovered (by losing immunity). |
1c2 | The average incubation period |
c3 | The quarantine rate |
c4 | The infectious recovery rate |
c5 | The quarantined recovery rate |
ξ | The natural birth and death rate |
Parameter | value |
c1 | 0.015. |
1c2 | 0.571 |
c3 | 0.007177 |
c4 | 0.875 |
c5 | 0.4375 |
ξ | 0.00642 |
Parameter | Definition |
β(t) | The annual periodic transmission rate |
r(t) | The time dependent annual periodic vaccination rate |
c1 | The progression rate of the recovered (by losing immunity). |
1c2 | The average incubation period |
c3 | The quarantine rate |
c4 | The infectious recovery rate |
c5 | The quarantined recovery rate |
ξ | The natural birth and death rate |
Parameter | value |
c1 | 0.015. |
1c2 | 0.571 |
c3 | 0.007177 |
c4 | 0.875 |
c5 | 0.4375 |
ξ | 0.00642 |