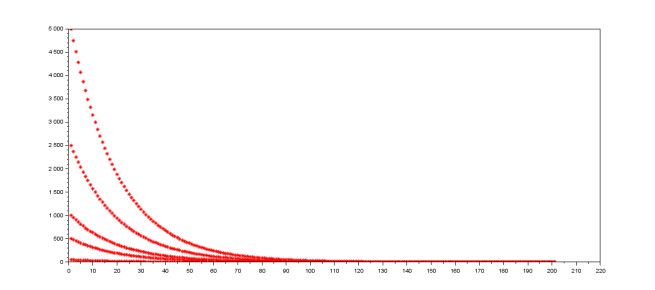
This paper is concerned with the robust synchronization analysis of delayed fractional order neural networks with uncertain parameters (DFNNUPs). Firstly, the DFNNUPs drive system model and response system model are established. Secondly, using multiple matrix quadratic Lyapunov function approach and inequality analysis technique, the robust synchronization conditions are derived in the form of the matrix inequalities. Finally, the correctness of the theoretical results is verified by an example.
Citation: Xinxin Zhang, Yunpeng Ma, Shan Gao, Jiancai Song, Lei Chen. Robust synchronization analysis of delayed fractional order neural networks with uncertain parameters[J]. AIMS Mathematics, 2022, 7(10): 18883-18896. doi: 10.3934/math.20221040
[1] | Zubair Ahmad, Zahra Almaspoor, Faridoon Khan, Sharifah E. Alhazmi, M. El-Morshedy, O. Y. Ababneh, Amer Ibrahim Al-Omari . On fitting and forecasting the log-returns of cryptocurrency exchange rates using a new logistic model and machine learning algorithms. AIMS Mathematics, 2022, 7(10): 18031-18049. doi: 10.3934/math.2022993 |
[2] | Naif Alotaibi, A. S. Al-Moisheer, Ibrahim Elbatal, Salem A. Alyami, Ahmed M. Gemeay, Ehab M. Almetwally . Bivariate step-stress accelerated life test for a new three-parameter model under progressive censored schemes with application in medical. AIMS Mathematics, 2024, 9(2): 3521-3558. doi: 10.3934/math.2024173 |
[3] | Jumanah Ahmed Darwish, Saman Hanif Shahbaz, Lutfiah Ismail Al-Turk, Muhammad Qaiser Shahbaz . Some bivariate and multivariate families of distributions: Theory, inference and application. AIMS Mathematics, 2022, 7(8): 15584-15611. doi: 10.3934/math.2022854 |
[4] | Alanazi Talal Abdulrahman, Khudhayr A. Rashedi, Tariq S. Alshammari, Eslam Hussam, Amirah Saeed Alharthi, Ramlah H Albayyat . A new extension of the Rayleigh distribution: Methodology, classical, and Bayes estimation, with application to industrial data. AIMS Mathematics, 2025, 10(2): 3710-3733. doi: 10.3934/math.2025172 |
[5] | Baishuai Zuo, Chuancun Yin . Stein’s lemma for truncated generalized skew-elliptical random vectors. AIMS Mathematics, 2020, 5(4): 3423-3433. doi: 10.3934/math.2020221 |
[6] | Aisha Fayomi, Ehab M. Almetwally, Maha E. Qura . A novel bivariate Lomax-G family of distributions: Properties, inference, and applications to environmental, medical, and computer science data. AIMS Mathematics, 2023, 8(8): 17539-17584. doi: 10.3934/math.2023896 |
[7] | Walid Emam . Benefiting from statistical modeling in the analysis of current health expenditure to gross domestic product. AIMS Mathematics, 2023, 8(5): 12398-12421. doi: 10.3934/math.2023623 |
[8] | Qian Li, Qianqian Yuan, Jianhua Chen . An efficient relaxed shift-splitting preconditioner for a class of complex symmetric indefinite linear systems. AIMS Mathematics, 2022, 7(9): 17123-17132. doi: 10.3934/math.2022942 |
[9] | Yanting Xiao, Wanying Dong . Robust estimation for varying-coefficient partially linear measurement error model with auxiliary instrumental variables. AIMS Mathematics, 2023, 8(8): 18373-18391. doi: 10.3934/math.2023934 |
[10] | Guangshuai Zhou, Chuancun Yin . Family of extended mean mixtures of multivariate normal distributions: Properties, inference and applications. AIMS Mathematics, 2022, 7(7): 12390-12414. doi: 10.3934/math.2022688 |
This paper is concerned with the robust synchronization analysis of delayed fractional order neural networks with uncertain parameters (DFNNUPs). Firstly, the DFNNUPs drive system model and response system model are established. Secondly, using multiple matrix quadratic Lyapunov function approach and inequality analysis technique, the robust synchronization conditions are derived in the form of the matrix inequalities. Finally, the correctness of the theoretical results is verified by an example.
Variational inequality is a powerful and well-known mathematical tool which has the direct, natural, unified and easily formulation to apply in mathematics such as linear and nonlinear analysis and optimization problem, etc. In addition, it has been used as a tool for studying in many fields such as engineering, industry economics, transportation, social and pure and applied science, see [17,21,30] and the reference therein. As previously mentioned, this implies many researchers developed the variational inequality in the various aspects. For example, the mixed variational inequality has been generalized from the variational inequality where the variational inequality is a special case of the mixed variational inequality and was presented by Lescarret [19] and Browder [2]. Later, Konnov and Volotskaya [16] applied the mixed variational inequality into the general economic equilibrium problems and oligopolistic equilibrium problem. So, many researchers have applied the mixed variational inequality in many fields such as optimization, game theory, control theory, etc., see [1,4,29]. On the other hand, in the study and development of problems, the inverse problem is the interesting one. Because the formatting of a problem from one problem to another is possible, and moreover, the solutions of the two problems are also related. For this reason, some problems, which cannot solve in direct, we can take the concept of the inverse problems for solving that results. Therefore, the inverse problems are applied in many fields such as engineering, finance, economics, transportation and science etc. For example, H. Kunze et al. [13,14,15] studied the inverse problems on many problems, such as the optimization problem, the differential equation and the variational equation etc. In these works, Kunze used the Collage theorem technique for solving these inverse problems and also presented some applications in economics and applied sciences. With the interest mentioned above, we would like to study the variational inequality in the aspect of the inverse problem which many researches of the inverse variational inequality are applied in many branches such as traffic network, economic, telecommunication networks. For example, in 2008, Yang [35] considered and analyzed the dynamic power price problem on both the discrete and evolutionary cases and, moreover, described the characterization of the optimal price by the solution of the inverse variational inequality. In 2010, He et al. [7] proposed some problems in the formulation of the inverse variational inequality on a normative control problem for solving the network equilibrium state in a linearly constrained set, etc. Furthermore, the inverse variational inequality is further developed and studied, where the inverse mixed variational inequality is one that has been improved from the inverse variational inequality and has the inverse variational inequality as a special case, see [3,12]. Later, in 2014, Li et al. [20] studied the inverse mixed variational inequality problem and applied this problem to the traffic network equilibrium problem and the traffic equilibrium control problem. They used the generalized f-projection operators to obtain their results and proposed the properties of the generalized f-projection operator to obtain the convergence of the generalized f-projection algorithm for inverse mixed variational inequality. In 2016, Li and Zou [23] extended the inverse mixed variational inequality into a new class of inverse mixed quasi variational inequality. All of the above, we are interested in studying and developing the problem which is generalized from the inverse mixed variational inequality that, in this paper, will be called the generalized inverse mixed variational inequality and used a generalized f-projection operator for solving our results.
On the other hand, a neural network (also known as a dynamical system in the mathematical literature) is the problem related to time and is a powerful tool which is used to apply in the signal processing, pattern recognition, associative memory and other engineering or scientific field, see [5,18,24,27,39]. By the characteristic of nature of parallelization and distributed information process, the neural networks have served as the promising computational models for real time applications. So, the neural networks have been designed to solve the mathematic programming and the related optimization problems, see [22,33,38] and the reference therein. From the foregoing, it will be interesting to study and develop the neural network further and can be also seen from the continuous development of research in artificial neural networks such as the following research: In 1996, A. Nagurney [28] studied the projected dynamical system and variational inequalities and also presented some applications of these problems in economics and transportation. In 2002, Xia et al. [34] presented a neural network, which has a single-layer structure and has amenable to parallel implementation and proposed the equivalence of the neural network and the variational inequality for solving the nonlinear formulation and the stability of such network. In 2015, Zou et al. [37] presented a neural network which possesses a simple one-layer structures for solving the inverse variational inequality problem and proved the stability of such network and, moreover, proposed some numerical examples. In the same year, M. A. Noor et al. [26] proposed the dynamical systems for the extended general quasi variational inequalities and proved the convergence of globally exponentially of the dynamical system. In 2021, Vuong et al. [31] considered the projected neural network for solving inverse variational inequalities and proposed the stability of the neural network. Moreover, they presented the applications of such neural network in transportation science. Later, in 2022, D. Hu et al. [6] used the neural network for solving about the optimization problems by proposing a modified projection neural network and used this network to solve the non-smooth, nonlinear and constrained convex optimization problems. Then, the existence of the solution and the stability in the Lyapunov sense of the modified projection neural network was proved. In addition, the application of the neural network in the optimization problem is not the only one mentioned here, there are many other studies that have applied the neural network into the optimization problem, see [11,36] and the reference therein. All of these neural networks, we see that it is important and interesting tool to study and develop further applications. Therefore, in this paper, we are interested to propose a neural network which associated with a generalization of the inverse mixed variational inequality problem and consider the stability of such neural network.
Based on the above, we would like to present the main objectives of this article as follows:
● The generalized inverse mixed variational inequality problem is presented and the existence and uniqueness of the problem are proved.
● The neural network associated with the generalized inverse mixed variational inequality is proposed. The existence and stability of such neural network are proved.
● Finally, we introduce the iterative methods which arises from the previous neural network and also display a numerical example by using such iterative methods.
The paper is organized as follows: In Section 2, we recall some basic definitions and theorems of the generalized f-projector operator and the neural network. In Section 3, we consider and study a generalized inverse mixed variational inequality by using the generalized f-projection operator for solving the existence and uniqueness of the generalized inverse mixed variational inequality. In Section 4, the neural network of the generalized inverse mixed variational inequality is proposed and the Wiener-Hopf equation, which the solution of the equation is equivalent to the solution of the generalized inverse mixed variational inequality, is also considered. Then, the existence and stability of such neural network are proved. Finally, author will present some iterative schemes which are constructed from the neural network and display a numerical example by using such algorithms to understand all of our theorems in this paper in Sections 5 and 6.
Throughout for this paper, we let H be a real Hilbert space whose inner product and norm are denoted by ⟨⋅,⋅⟩ and ‖⋅‖, respectively. Let 2H be denoted for the class of all nonempty subset of H and K be a nonempty closed and convex subset of H. For each K⊆H we denote by d(⋅,K) for the usual distance function on H to K, that is d(u,K)=infv∈K‖u−v‖, for all u∈H. In this paper, we will study the generalized inverse mixed variational inequality which is a generalization of the variational inequality, so we will use the generalization of the projection operator for considering this results. Then, we will introduce the concept of the generalized f-projector operator which was introduced by Wu and Huang [32].
Definition 2.1. [32] Let H be a real Hilbert space and K be a nonempty closed and convex subset of H. We say that Pf,ρK:H→2K is a generalized f-projection operator if
Pf,ρK(x)={u∈K⏐G(x,u)=infξ∈KG(x,ξ)},for allx∈H |
where G:H×K→R∪{+∞} is a functional defined as follows:
G(x,ξ)=‖x‖2−2⟨x,ξ⟩+‖ξ‖2+2ρf(ξ), |
with x∈H,ξ∈K,ρ is a positive number and f:K→R∪{+∞} is a proper, convex and lower semicontinuous function for the set of real numbers denoted by R.
Remark 2.1. By the definition of a generalized f-projection operator, if we let f=0 then the Pf,ρK is the usual projection operator. That is, if f=0 then G(x,ξ)=‖x‖2−2⟨x,ξ⟩+‖ξ‖2=‖x−ξ‖2. This implies Pf,ρK(x)={u∈K⏐G(x,u)=infξ∈KG(x,ξ)}={u∈K⏐G(x,u)=infξ∈K‖x−ξ‖}=PK(x).
Later, in 2014, Li et al. [20] presented the properties of the operator Pf,ρK in Hilbert spaces as follows.
Lemma 2.1. [20] Let H be a real Hilbert space and K be a nonempty closed and convex subset of H. Then, the following statements hold:
(i) Pf,ρK(x) is nonempty and Pf,ρK is a single valued mapping;
(ii) for all x∈H,x∗=Pf,ρK(x) if and only if
⟨x∗−x,y−x∗⟩+ρf(y)−ρf(x∗)≥0,∀y∈K; |
(iii) Pf,ρK is continuous.
Theorem 2.1. [20] Let H be a real Hilbert space and K be a nonempty closed and convex subset of H. Let f:K→R∪{+∞} be a proper, convex and lower semicontinuous function. Then, the following statements hold:
‖(v−Pf,ρK(v))−(u−Pf,ρK(u))‖2≤‖v−u‖2−‖Pf,ρK(v)−Pf,ρK(u)‖2, |
and
‖(v−Pf,ρK(v))−(u−Pf,ρK(u))‖≤‖v−u‖, |
for all u,v∈H.
Next, the following definition is mappings which are used for solving our results.
Definition 2.2. [23] Let H be a real Hilbert space and g,A:H→H be two single valued mappings.
(i) A is said to be a λ-strongly monotone on H if there exists a constant λ>0 such that
⟨Ax−Ay,x−y⟩≥λ‖x−y‖2,∀x,y∈H. |
(ii) A is said to be a γ-Lipschitz continuous on H if there exists a constant γ>0 such that
‖Ax−Ay‖≤γ‖x−y‖,∀x,y∈H. |
(iii) (A,g) is said to be a μ-strongly monotone couple on H if there exists a positive constant μ>0 such that
⟨Ax−Ay,g(x)−g(y)⟩≥μ‖x−y‖2,∀x,y∈H. |
On the other hand, we will recall the following well known concepts of the neural network (also known as dynamical system in the literature).
A dynamical system
˙x=f(x),for allx∈H, | (2.1) |
where f is a continuous function form H into H. A solution of (2.1) is a differentiable function x:I→H where I is some intervals of R such that for all t∈I,
˙x(t)=f(x(t)). |
The following definitions, we will propose the equilibrium and the stability of the solution of the neural network as follows.
Definition 2.3. [9]
a) A point x∗ is an equilibrium point for (2.1) if f(x∗)=0;
b) An equilibrium point x∗ of (2.1) is stable if, for any ε>0, there exists δ>0 such that, for every x0∈B(x∗,δ), the solution x(t) of the dynamical system with x(0)=x0 exists and is contained in B(x∗,ε) for all t>0, where B(x∗,r) denotes the open ball with center x∗ and radius r;
c) A stable equilibrium point x∗ of (2.1) is asymptotically stable if there exists δ>0 such that, for every solution x(t) with x(0)∈B(x∗,δ), one has
limt→∞x(t)=x∗. |
Definition 2.4. [28] Let x(t) in (2.1). For any x∗∈K, where K is a closed convex set, let L be a real continuous function defined on a neighborhood N(x∗) of x∗, and differentiable everywhere on N(x∗) except possibly at x∗. L is called a Lyapunov function at x∗, if it satisfies:
i) L(x∗)=0 and L(x)>0, for all x≠x∗,
ii) ˙L(x)≤0 for all x≠x∗ where
˙L(x)=ddtL(x(t))⏐t=0. | (2.2) |
Notice that, the equilibrium point x, which satisfies Definition 2.4 ii), is stable in the sense of Lyapunov.
Definition 2.5. [26] A neural network is said to be globally convergent to the solution set X of (2.1) if, irrespective of initial point, the trajectory of neural network satisfies
limt→∞d(x(t),X)=0. | (2.3) |
If the set X has a unique point x∗, then (2.3) satisfies limt→∞x(t)=x∗. If the neural network is still stable at x∗ in the Lyapunov sense, then the neural network is globally asymptotically stable at x∗.
Definition 2.6. [26] The neural network is said to be globally exponentially stable with degree ω at x∗ if, irrespective of the initial point, the trajectory of the neural network x(t) satisfies
‖x(t)−x∗‖≤c0‖x(t0)−x∗‖exp−ω(t−t0) |
for all t≥t0, where c0 and ω are positive constants independent of initial point. Notice that, if it is a globally exponentially stability then it is a globally asymptotically stable and the neural network converges arbitrarily fast.
Lemma 2.2. [25](Gronwall) Let ˆu and ˆv be real valued nonnegative continuous functions with domain {t⏐t≥t0} and let α(t)=α0(|t−t0|), where α0 is a monotone increasing function. If for all t≥t0,
ˆu(t)≤α(t)+∫tt0ˆu(s)ˆv(s)ds, |
then,
ˆu(t)≤α(t)exp∫tt0ˆv(s)ds. |
In this section, we will propose the generalized inverse mixed variational inequality. Let g,A:H→H be two continuous mappings and f:K→R∪{+∞} be a proper, convex and lower semicontinuous function. The generalized inverse mixed variational inequality is: to find an x∗∈H such that A(x∗)∈K and
⟨g(x∗),y−A(x∗)⟩+ρf(y)−ρf(A(x∗))≥0,for ally∈K. | (3.1) |
Remark 3.1. The generalized inverse mixed variational inequality (3.1) can be reduced to the following problems:
(i) If g is the identity mapping. Then, (3.1) collapses to the inverse mixed variational inequality which was studied by Li et al. [20] as follows: find an x∗∈H such that A(x∗)∈K and
⟨x∗,y−A(x∗)⟩+ρf(y)−ρf(A(x∗))≥0,∀y∈K. |
(ii) If H=Rn, where Rn denotes the real n-dimensional Euclidean space, g is the identity mapping and f(x)=0 for all x∈Rn, then (3.1) collapses to the following inverse variational inequality: find an x∗∈Rn such that A(x∗)∈K and
⟨x∗,y−A(x∗)⟩≥0,∀y∈K, |
which was proposed by He and Liu [8].
(iii) If H=Rn,A is the identity mapping and f(x)=0 for all x∈Rn, then (3.1) becomes the classic variational inequality, that is, to find an x∗∈Rn such that
⟨g(x∗),y−x∗⟩≥0,∀y∈K. |
By using Lemma 2.1 (ii), we obtain the following t heorem.
Theorem 3.1. Let H be a real Hilbert space and K be a nonempty closed and convex subset of H. Let f:K→R∪{+∞} be a proper, convex and lower semicontinuous function. Then x∗ is a solution of the generalized inverse mixed variational inequality (3.1) if and only if x∗ satisfies
A(x∗)=Pf,ρK[A(x∗)−g(x∗)]. | (3.2) |
Proof. (⇒) Let x∗ be a solution of (3.1), that is, A(x∗)∈K and
⟨g(x∗),y−A(x∗)⟩+ρf(y)−ρf(Ax∗)≥0 |
for all y∈K. We have
⟨Ax∗−Ax∗+g(x∗),y−Ax∗⟩+ρf(y)−ρf(Ax∗)≥0 |
for all y∈K. By Lemma 2.1 (ii), we obtain
Ax∗=Pf,ρK(Ax∗−g(x∗)). |
(⇐) Let Ax∗=Pf,ρK(Ax∗−g(x∗)). By Lemma 2.1 (ii), we have
⟨Ax∗−(Ax∗+g(x∗)),y−Ax∗⟩+ρf(y)−ρf(Ax∗)≥0 |
for all y∈K. This implies that
⟨g(x∗),y−A(x∗)⟩+ρf(y)−ρf(Ax∗)≥0 |
for all y∈K. We conclude that x∗ is a solution of (3.1).
The next theorem, we consider the existence and uniqueness of the generalized inverse mixed variational inequality (3.1) as follows.
Theorem 3.2. Let H be a real Hilbert space and K be a nonempty closed convex subset of H,g,A:H→H be Lipschitz continuous on H with constants α and β, respectively. Let f:K→R∪{+∞} be a proper, convex and lower semicontinuous function. Assume that
(i) g is a λ-strongly monotone and (A,g) is a μ-strongly monotone couple on H;
(ii) the following condition holds
√β2−2μ+α2+√1−2λ+α2<1, |
where μ<β2+α22 and λ<1+α22.
Then, the generalized inverse mixed variational inequality (3.1) has a unique solution in H.
Proof. Let F:H→H be defined as follows: for any u∈H,
F(u)=u−Au+Pf,ρK(Au−g(u)). |
For any x,y∈H, denote ˉx=Ax−g(x) and ˉy=Ay−g(y), we have
‖F(x)−F(y)‖=‖x−Ax+Pf,ρK(Ax−g(x))−y+Ay−Pf,ρK(Ay−g(y))‖=‖x−y−g(x)+g(y)−(ˉx−Pf,ρK(ˉx)−[ˉy−Pf,ρK(ˉy)])‖≤‖x−y−g(x)+g(y)‖+‖ˉx−Pf,ρK(ˉx)−[ˉy−Pf,ρK(ˉy)]‖. |
Since g is a λ-strongly monotone and α-Lipschitz continuous, we see that
‖x−y−g(x)+g(y)‖2=‖x−y‖2−2⟨g(x)−g(y),x−y⟩+‖g(x)−g(y)‖2≤(1−2λ+α2)‖x−y‖2, | (3.3) |
and, by Theorem 2.1, we obtain
‖(ˉx−Pf,ρK(ˉx))−(ˉy−Pf,ρK(ˉy))‖≤‖ˉx−ˉy‖=‖A(x)−g(x)−A(y)+g(y)‖. |
Since A is a β-Lipschitz continuous, g is a α-Lipschitz continuous and (A,g) is a μ-strongly monotone couple on H. Then,
‖A(x)−g(x)−A(y)+g(y)‖2=‖A(x)−A(y)‖2−2⟨A(x)−A(y),g(x)−g(y)⟩+‖g(x)−g(y)‖2≤β2‖x−y‖2−2μ‖x−y‖2+α2‖x−y‖2=(β2−2μ+α2)‖x−y‖2. | (3.4) |
By (3.3) and (3.4), then
‖F(x)−F(y)‖≤√1−2λ+α2‖x−y‖+√β2−2μ+α2‖x−y‖=(√1−2λ+α2+√β2−2μ+α2)‖x−y‖=θ‖x−y‖ |
where θ=√1−2λ+α2+√β2−2μ+α2. By the assumption (ii), we have 0<θ<1. This implies that F is a contraction mapping in H. So, F has a unique fixed point in H. Therefore if x∗ is a fixed point, then
x∗=x∗−Ax∗+Pf,ρK(Ax∗−g(x∗)). |
Hence, Ax∗=Pf,ρK(Ax∗−g(x∗)). By Theorem 3.1, we conclude that x∗ is a solution of the generalized inverse mixed variational inequality (3.1).
In this part, firstly, we will propose the Wiener Hopf equation which the solution of the equation is equivalent to the solution of the generalized inverse mixed variational inequality (3.1). Then, we will present the neural network associated with the generalized inverse mixed variational inequality. Finally, the existence and stability of the solution of such neural network are proved as follows.
Let g,A:H→H be two continuous mappings and K be a nonempty closed and convex subset of H. Let f:K→R∪{+∞} be a proper, convex and lower semicontinuous function. The Wiener Hopf Equation which is equivalent to the generalized inverse mixed variational inequality (3.1) as follows: find x∗∈H such that
QK(A(x∗)−g(x∗))+g(x∗)=0, | (4.1) |
where QK=I−Pf,ρK with I is an identity operator.
The following lemma, we will present the equivalent solution of the Wiener Hopf Equation (4.1) and the generalized inverse mixed variational inequality (3.1) problem.
Lemma 4.1. x∗ is a solution of the generalized inverse mixed variational inequality (3.1) if and only if x∗ is a solution of the Wiener Hopf Equation (4.1).
Proof. (⇒) Assume that x∗∈H is a solution of (3.1). By Theorem 3.2, we obtain that
A(x∗)=Pf,ρK(A(x∗)−g(x∗)). |
Since QK=I−Pf,ρK, we have
QK(A(x∗)−g(x∗))=(I−Pf,ρK)(A(x∗)−g(x∗))=A(x∗)−g(x∗)−Pf,ρK(A(x∗)−g(x∗))=A(x∗)−g(x∗)−A(x∗)=−g(x∗). |
Then, QK(A(x∗)−g(x∗))+g(x∗)=0.
(⇐) Since x∗∈H is a solution of the Wiener Hopf equation (4.1), that is,
QK(A(x∗)−g(x∗))+g(x∗)=0. |
Since QK=I−Pf,ρK, we get
(I−Pf,ρK)(A(x∗)−g(x∗))+g(x∗)=0. |
Then,
A(x∗)=Pf,ρK(A(x∗)−g(x∗)). |
Therefore, x∗ is a solution of (3.1).
Next, we will propose the neural network (known as dynamical system in the literature) associated with the generalized inverse mixed variational inequality. Let H be a real Hilbert space and K be a nonempty closed and convex subset of H. Let A:H→K be a Lipschitz continuous with constants β and g:H→H be a Lipschitz continuous with constants α and f:K→R∪{+∞} be a proper, convex and lower semicontinuous function. By Theorem 3.2, we know that the solution of the generalized inverse mixed variational inequality (3.1) exists and Lemma 4.1, we have the equivalence of the solution of (3.1) with the solution of Wiener Hopf equation (4.1). So, we obtain the following result.
Since QK(A(x∗)−g(x∗))+g(x∗)=0 and QK=I−Pf,ρK, we have
(I−Pf,ρK)(A(x∗)−g(x∗))+g(x∗)=0. |
This implies that
A(x∗)−Pf,ρK(A(x∗)−g(x∗))=0. |
Now, we define the residue vector R(x) by the relation
R(x)=A(x)−Pf,ρK(A(x)−g(x)). | (4.2) |
Then, by the previous article, we see that x∈K is a solution of the generalized inverse mixed variational inequality if and only if x∈K is a zero of the equation
R(x)=0. | (4.3) |
By the equivalent formulation (3.2), we will propose the neural network associated with the generalized inverse mixed variational inequality as follows:
dxdt=η{Pf,ρK(A(x)−g(x))−A(x)}, | (4.4) |
which x(t0)=x0 and η is a positive constant with a positive real number t0.
Notice that the right-hand side is related to the projection operator and is discontinuous of the boundary of K. It is clear from the definition that the solution to the neural network associated with the generalized inverse mixed variational inequality always stay in K. This implies that the qualitative results such as the existence of the solution on the given data to such neural network can be studied.
Now, we will present the existence and uniqueness of the solution of the neural network associated with the generalized inverse mixed variational inequality (4.4).
Theorem 4.1. Let g:H→H be a Lipschitz continuous with constants α and A:H→K be a Lipschitz continuous with constants β. Let f:K→R∪{+∞} be a proper, convex and lower semicontinuous function. Assume that all of assumption of Theorem 3.2 hold. Then, for each x0∈H, there exists the unique continuous solution x(t) of the neural network associated with the generalized inverse mixed variational inequality (4.4) with x(t0)=x0 over the interval [t0,∞).
Proof. Let η be a positive constant and define the mapping F:H→K by
F(x)=η{Pf,ρK[A(x)−g(x)]−A(x)}, |
for all x∈H. By using Theorem 2.1 and (3.4), we obtain
‖F(x)−F(y)‖=‖η{Pf,ρK[A(x)−g(x)]−A(x)}−η{Pf,ρK[A(y)−g(y)]−A(y)}‖=η‖{Pf,ρK[A(x)−g(x)]−A(x)}−{Pf,ρK[A(y)−g(y)]−A(y)}‖=η‖[(A(y)−g(y))−Pf,ρK[A(y)−g(y)]]−[(A(x)−g(x))−Pf,ρK[A(x)−g(x)]]+g(y)−g(x)‖≤η{‖[(A(y)−g(y))−Pf,ρK[A(y)−g(y)]]−[(A(x)−g(x))−Pf,ρK[A(x)−g(x)]]‖+‖g(y)−g(x)‖}≤η{‖(A(y)−g(y))−(A(x)−g(x))‖+‖g(y)−g(x)‖}=η(√β2−2μ+α2+α)‖x−y‖. |
By the assumption (ii) of Theorem 3.2, we know that η(√β2−2μ+α2+α)>0. Thus, F is a Lipschitz continuous. This implies that, for each x0∈H, there exists a unique continuous solution x(t) of (4.4), defined in initial t0≤t<Γ with the initial condition x(t0)=x0.
Let [t0,Γ) be its maximal interval of existence, we will show that Γ=∞. Under the assumption, we obtain that (3.1) has a unique solution (say x∗) such that A(x∗)∈K and
A(x∗)=Pf,ρK[A(x∗)−g(x∗)]. |
Let x∈H. We have
‖F(x)‖=‖η{Pf,ρK[A(x)−g(x)]−A(x)}‖=η‖Pf,ρK[A(x)−g(x)]−Pf,ρK[A(x∗)−g(x∗)]+A(x∗)−A(x)‖=η‖Pf,ρK[A(x)−g(x)]−Pf,ρK[A(x∗)−g(x∗)]+A(x∗)−g(x∗)−A(x)+g(x)+g(x∗)−g(x)‖≤η{‖[(A(x∗)−g(x∗))−Pf,ρK[A(x∗)−g(x∗)]−[(A(x)−g(x))−Pf,ρK[A(x)−g(x)]]‖+‖g(x∗)−g(x)‖}≤η{‖A(x∗)−g(x∗)−A(x)+g(x)‖+‖g(x∗)−g(x)‖}≤η{(√β2−2μ+α2)‖x∗−x‖+α‖x∗−x‖}=η{(√β2−2μ+α2+α)‖x∗−x‖}≤η(√β2−2μ+α2+α)‖x∗‖+η(√β2−2μ+α2+α)‖x‖. |
Hence,
‖x(t)‖≤‖x(t0)‖+∫tt0‖F(s)‖ds≤‖x(t0)‖+∫tt0η(√β2−2μ+α2+α)‖x∗‖ds+∫tt0η(√β2−2μ+α2+α)‖x(s)‖ds=‖x(t0)‖+η(√β2−2μ+α2+α)‖x∗‖(t−t0)+η(√β2−2μ+α2+α)∫tt0‖x(s)‖ds=‖x(t0)‖+k1(t−t0)+k2∫tt0‖x(s)‖ds, |
where k1=η(√β2−2μ+α2+α)‖x∗‖ and k2=η(√β2−2μ+α2+α). By Gronwall's Lemma, we obtain that
‖x(t)‖≤{‖x(t0)‖+k1(t−t0)}expk2(t−t0), |
where t∈[t0,Γ). Therefore, the solution x(t) is bounded on [t0,Γ), if Γ is finite. We conclude that Γ=∞.
Theorem 4.2. Assume that all of the assumptions of Theorem 4.1 hold and satisfy the following condition
√β2−2μ+α2<λ. | (4.5) |
Then, the neural network associated with the generalized inverse mixed variational inequality (4.4) is globally exponentially stable and also globally asymptotically stable to the solution of the generalized inverse mixed variational inequality (3.1).
Proof. By Theorem 4.1, we known that (3.1) has a unique continuous solution x(t) over [t0,Γ) for any fixed x0∈H. Let x0(t)=x(t,t0;x0) be the solution of the initial value problem (4.4) and x∗(t) be a solution of (3.1).
Define the Lyapunov function L:H→R by
L(x)=12‖x−x∗‖2, |
for all x∈H. We obtain that
dLdx=dLdx⋅dxdt=⟨x−x∗,dxdt⟩=⟨x−x∗,η{Pf,ρK[A(x)−g(x)]−A(x)}⟩=η⟨x−x∗,Pf,ρK[A(x)−g(x)]−A(x)⟩=η⟨x−x∗,Pf,ρK[A(x)−g(x)]−A(x∗)+A(x∗)−A(x)⟩=η⟨x−x∗,(A(x∗)−g(x∗))−Pf,ρK[A(x∗)−g(x∗)]−(A(x)−g(x))+Pf,ρK[A(x)−g(x)]⟩+η⟨x−x∗,g(x∗)−g(x)⟩≤η‖x−x∗‖‖(A(x∗)−g(x∗))−Pf,ρK[A(x∗)−g(x∗)]−(A(x)−g(x))+Pf,ρK[A(x)−g(x)]‖−η⟨x−x∗,g(x)−g(x∗)⟩≤η‖x−x∗‖‖(A(x∗)−g(x∗))−(A(x)−g(x))‖−ηλ‖x−x∗‖2≤η√β2−2μ+α2‖x−x∗‖2−ηλ‖x−x∗‖2=η(√β2−2μ+α2−λ)‖x−x∗‖2. |
By the assumption (4.5), we obtain that √β2−2μ+α2−λ<0. We have
‖x(t)−x∗‖≤‖x0−x∗‖+∫tt0‖L(x(s))‖ds≤‖x0−x∗‖+∫tt0η(√β2−2μ+α2−λ)‖x(s)−x∗‖ds≤‖x0−x∗‖expθ(t−t0), |
where θ=η(√β2−2μ+α2−λ). Since θ<0, this implies that (4.4) is a globally exponentially stable with degree −θ at x∗. Moreover, we see that L(x) is a global Lyapunov function for the (4.4) and (4.4) is stable in the sense of Lyapunov. Thus, the neural network is also globally asymptotically stable. We conclude that the solution of the (4.4) converges to the unique solution of (3.1).
By the previous article, we proposed the neural network associated with the generalized inverse mixed variational inequality. Next, we will suggest and analyze some iterative schemes which will be used for solving the solution of the generalized inverse mixed variational inequality (3.1). By using the concept of the forward difference scheme, we obtain the discretization of the neural network (4.4) with respect to the time variable t, with step size hn>0 and initial point x0∈H such that
xn+1(t)−xn(t)hn=η{Pf,ρK[A(xn(t))−g(xn(t))]−A(xn(t))}, | (5.1) |
where x(t0)=x0 and η is a positive constant with a positive real number t0.
If we let hn=1 then, by (5.1), we obtain the following iterative scheme:
xn+1(t)=xn(t)+η{Pf,ρK[A(xn(t))−g(xn(t))]−A(xn(t))}, | (5.2) |
where x(t0)=x0 and η is a positive constant with a positive real number t0. Hence, we will consider the following algorithm which is introduced by (5.2).
Algorithm 1: Choose the starting point x0∈H with A(x0)∈K and fixed ρ,η are positive constants.
Then, we compute {xn} through the following iterative scheme: Set n=0.
Step 1: Compute
xn+1=xn+η{Pf,ρK[A(xn)−g(xn)]−A(xn)}. |
If xn+1=xn, then STOP and xn is a solution. Otherwise, update n to n+1 and go to Step 1.
Furthermore, if we use the inertial type predictor and corrector technique. Then, Algorithm 1 can be written in the following algorithm.
Algorithm 2: Choose the starting point x0,x1∈H with
y1=x1+ω1(x1−x0)∈H |
where 0≤ω1≤1 and A(y1)∈K. Then, we compute {xn} through the following iterative scheme: Set n=1.
Step 1: Compute: fixed ρ and η are positive constants,
xn+1=yn+η{Pf,ρK[A(yn)−g(yn)]−A(yn)}. |
If xn+1=xn, then STOP and xn is a solution. Otherwise, go to next step.
Step 2: Set
yn+1=xn+1+ωn+1(xn+1−xn) |
where 0≤ωn≤1 and update n to n+1 and go to Step 1.
The following theorem, we will present the convergence of the previous algorithms which converges to the solution of (3.1).
Theorem 5.1. Assume that all of the assumptions of Theorem 3.2 hold and satisfy the following condition:
Δ+η(Δ+α)22<λ<Δ+η(Δ+α)22+12η | (5.3) |
where Δ=√β2−2μ+α2. Then, the sequence {xn} generated by Algorithm 1 converges strongly to the unique solution of the generalized inverse mixed variational inequality (3.1).
Proof. By the assumption of Theorem 3.2, we have (3.1) has a unique solution and we let x∗ be a unique solution of (3.1). By Algorithm 1, we have
xn+1=xn+η{Pf,ρK[A(xn)−g(xn)]−A(xn)}. |
Then,
‖xn+1−x∗‖2=‖xn+η{Pf,ρK[A(xn)−g(xn)]−A(xn)}−x∗‖2=‖(xn−x∗)+η{Pf,ρK[A(xn)−g(xn)]−A(xn)}‖2=‖xn−x∗‖2+2η⟨xn−x∗,Pf,ρK[A(xn)−g(xn)]−A(xn)⟩+η2‖Pf,ρK(xn)[A(xn)−g(xn)]−A(xn)‖2. |
By Theorem 2.1, (3.4) and g is an α-Lipschitz continuous, we have
‖Pf,ρK[A(xn)−g(xn)]−A(xn)‖=‖Pf,ρK[A(xn)−g(xn)]−A(x∗)+A(x∗)−A(xn)‖≤‖(A(x∗)−g(x∗))−Pf,ρK[A(x∗)−g(x∗)]−(A(xn)−g(xn))+Pf,ρK[A(xn)−g(xn)]‖+‖g(xn)−g(x∗)‖≤√β2−2μ+α2‖xn−x∗‖+α‖xn−x∗‖=(√β2−2μ+α2+α)‖xn−x∗‖, | (5.4) |
and, by (3.4) and g is a λ-strongly monotone, we get that
⟨xn−x∗,Pf,ρK[A(xn)−g(xn)]−A(xn)⟩=⟨xn−x∗,Pf,ρK[A(xn)−g(xn)]−Pf,ρK[A(x∗)−g(x∗)]+A(x∗)−A(xn)⟩=⟨xn−x∗,(A(x∗)−g(x∗))−Pf,ρK[A(x∗)−g(x∗)]−[(A(xn)−g(xn))−Pf,ρK[A(xn)−g(xn)]]⟩+⟨xn−x∗,g(x∗)−g(xn)⟩≤‖xn−x∗‖‖(A(x∗)−g(x∗))−Pf,ρK[A(x∗)−g(x∗)]−(A(xn)−g(xn))+Pf,ρK[A(xn)−g(xn)]‖−⟨xn−x∗,g(xn)−g(x∗)⟩≤‖xn−x∗‖‖A(x∗)−g(x∗)−A(xn)+g(xn)‖−λ‖xn−x∗‖2≤√β2−2μ+α2‖xn−x∗‖2−λ‖xn−x∗‖2=(√β2−2μ+α2−λ)‖xn−x∗‖2. | (5.5) |
Hence, by (5.4) and (5.5), we obtain that
‖xn+1−x∗‖2≤‖xn−x∗‖2+2η(√β2−2μ+α2−λ)‖xn−x∗‖2+η2(√β2−2μ+α2+α)2‖xn−x∗‖2=[1+2η(√β2−2μ+α2−λ)+η2(√β2−2μ+α2+α)2]‖xn−x∗‖2=[1+2η(Δ−λ)+η2(Δ+α)2]‖xn−x∗‖2, |
where Δ=√β2−2μ+α2. This implies that
‖xn+1−x∗‖2≤Θ‖xn−x∗‖2, |
where Θ=1+2η(Δ−λ)+η2(Δ+α)2. By this processing, we obtain that
‖xn+1−x∗‖2≤Θ‖xn−x∗‖2≤Θ2‖xn−1−x∗‖2≤Θ3‖xn−2−x∗‖2⋮≤Θn+1‖x0−x∗‖2. |
By the condition (5.3), we see that 0<Θ<1. Then, ‖xn+1−x∗‖→0 as n→∞. We conclude that {xn} converges to the solution of (3.1).
Remark 5.1 1.) By Theorem 5.1, if we let xn+1=yn−ηA(yn)+ηPf,ρK[A(yn)−g(yn)] (in Algorithm 2), then we also obtain that Algorithm 2 converges to the solution of (3.1).
2.) Moreover, by the condition (5.3), we see that the choice of η affect to λ, this means that if we have the value of a suitable α,λ,μ,β, then we can find the value of suitable η.
In this section, we will propose the example for understanding the previous theorems and algorithms as follows.
Example 6.1. Let H=[0,∞) and K=H. Assume that A(x)=x2,g(x)=3x2 and f(x)=x2+2x+1. Here, we fix ρ=1 and, by the condition (5.3), we can choose η=0.1.
It is easy to show that g is a Lipschitz continuous with constant 32 and strongly monotone with constant 32 and A is a Lipschitz continuous with constant 12. Moreover, we can show that the definition of g and A satisfies a (A,g)34-strongly monotone couple on H. So, we obtain α=32,β=12,λ=32 and μ=34. It is easy to show that the solution of (3.1) is 0 and 0 is also the solution of the neural network associated with the generalized inverse mixed variational inequality.
The following results, we considered the numerical example by using Algorithm 1. Firstly, if we chose x0=50 and by the definition of A, it is easily to see that A(x)∈K where x∈K. The computation in SCILAB program and the computer system used was a ASUS located at the Pibulsongkham Rajabhat University at Phitsanulok, Thailand. We had the following results: It was convergent to x∗=4.45×10−323 in the 14514 iterations and, moreover, when we assign x0 to any other value, we obtain the following results:
If we chose x0=500 then, in the 14590 iterations, it was convergent to x∗.
If we chose x0=1000 then, in the 14603 iterations, it was convergent to x∗.
If we chose x0=2500 then, in the 14621 iterations, it was convergent to x∗.
If we chose x0=5000 then, in the 14635 iterations, it was convergent to x∗, see in Figure 1.
On the other hand, we compute this example by using Algorithm 2 and let ωi=1i. If we chose x0=10 and x1=15. Then, we had the following results: it was convergent to the same solution, x∗=4.45×10−323, in the 14517 iterations.
If we chose x0=75 and x1=50 then, in the 14528 iterations, it was convergent to x∗.
If we chose x0=100 and x1=500 then, in the 14591 iterations, it was convergent to x∗.
If we chose x0=500 and x1=400 then, in the 14573 iterations, it was convergent to x∗, see in Figure 2.
Remark 6.1. By the above numerical example:
1). Observe that in Algorithm 2, we must assume that x1≥x02 to guarantee that y1∈H, this implies that xi and yi in H.
2). if we change ρ (such as ρ=0.01,ρ=100), then we will obtain the same results with ρ=1.
In this work, we presented the concept of the generalized inverse mixed variational inequality and the neural network associated with the generalized inverse mixed variational inequality. The existence and uniqueness of both problems were proved. The stability of the neural network was studied by assuming some condition. For considering our results, we proposed some algorithms and used such algorithms to show our numerical example. The results in this work extend and improve the literature paper.
The authors would like to thank Pibulsongkram Rajabhat University.
The authors declare that they have no conflicts of interest.
[1] |
A. M. Alimi, C. Aouiti, E. A. Assali, Finite-time and fixed-time synchronization of a class of inertial neural networks with multi-proportional delays and its application to secure communication, Neurocomputing, 332 (2019), 29–43. https://doi.org/10.1016/j.neucom.2018.11.020 doi: 10.1016/j.neucom.2018.11.020
![]() |
[2] |
L. Lin, J. D. Cao, S. Y. Zhu, P. Shi, Synchronization analysis for stochastic networks through finite fields, IEEE Trans. Automat. Control, 67 (2022), 1016–1022. https://doi.org/10.1109/TAC.2021.3081621 doi: 10.1109/TAC.2021.3081621
![]() |
[3] | R. Nobili, The machine of the mind Part II: The role of synchronization in brain information processing, 2020. |
[4] |
Y. Du, J. E. Clark, J. Whitall, Timing at peak force may be the hidden target controlled in continuation and synchronization tapping, Exp. Brain Res., 235 (2017), 1541–1554. https://doi.org/10.1007/s00221-017-4918-3 doi: 10.1007/s00221-017-4918-3
![]() |
[5] |
J. Y. Xiao, S. P. Wen, X. J. Yang, S. M. Zhong, New approach to global Mittag-Leffler synchronization problem of fractional-order quaternion-valued BAM neural networks based on a new inequality, Neural Networks, 122 (2020), 320–337. https://doi.org/10.1016/j.neunet.2019.10.017 doi: 10.1016/j.neunet.2019.10.017
![]() |
[6] |
U. Kandasamy, X. D. Li, R. Rajan, Quasi-synchronization and bifurcation results on fractional-order quaternion-valued neural networks, IEEE Trans. Neural Networks Learn. Syst., 31 (2019), 4063–4072. https://doi.org/10.1109/TNNLS.2019.2951846 doi: 10.1109/TNNLS.2019.2951846
![]() |
[7] | C. J. Xu, W. Zhang, C. Aouiti, Z. X. Liu, M. X. Liao, P. L. Li, Further investigation on bifurcation and their control of fractional-order bidirectional associative memory neural networks involving four neurons and multiple delays, Math. Methods Appl. Sci., 2021. https://doi.org/10.1002/mma.7581 |
[8] | C. J. Xu, W. Zhang, C. Aouiti, Z. X. Liu, L. Y. Yao, Further analysis on dynamical properties of fractional-order bi-directional associative memory neural networks involving double delays, Math. Methods Appl. Sci., 2022. https://doi.org/10.1002/mma.8477 |
[9] |
F. F. Du, J. G. Lu, New criterion for finite-time synchronization of fractional order memristor-based neural networks with time delay, Appl. Math. Comput., 389 (2021), 125616. https://doi.org/10.1016/j.amc.2020.125616 doi: 10.1016/j.amc.2020.125616
![]() |
[10] |
K. X. Wu, B. Li, Y. W. Du, S. S. Du, Synchronization for impulsive hybrid-coupled reaction-diffusion neural networks with time-varying delays, Commun. Nonlinear Sci. Numer. Simul., 82 (2019), 105031. https://doi.org/10.1016/j.cnsns.2019.105031 doi: 10.1016/j.cnsns.2019.105031
![]() |
[11] |
Z. L. Xu, X. D. Li, P. Y. Duan, Synchronization of complex networks with time-varying delay of unknown bound via delayed impulsive control, Neural Networks, 125 (2020), 224–232. https://doi.org/10.1016/j.neunet.2020.02.003 doi: 10.1016/j.neunet.2020.02.003
![]() |
[12] |
X. D. Hai, G. J. Ren, Y. G. Yu, C. H. Xu, Y. X. Zeng, Pinning synchronization of fractional and impulsive complex networks via event-triggered strategy, Commun. Nonlinear Sci. Numer. Simul., 82 (2020), 105017. https://doi.org/10.1016/j.cnsns.2019.105017 doi: 10.1016/j.cnsns.2019.105017
![]() |
[13] |
Y. L. Zhang, J. S. Zhuang, Y. H. Xia, Y. Z. Bai, J. D. Cao, L. F. Gu, Fixed-time synchronization of the impulsive memristor-based neural networks, Commun. Nonlinear Sci. Numer. Simul., 77 (2019), 40–53. https://doi.org/10.1016/j.cnsns.2019.04.021 doi: 10.1016/j.cnsns.2019.04.021
![]() |
[14] |
Z. B. Wang, H. Q. Wu, Global synchronization in fixed time for semi-Markovian switching complex dynamical networks with hybrid couplings and time-varying delays, Nonlinear Dyn., 95 (2019), 2031–2062. https://doi.org/10.1007/s11071-018-4675-2 doi: 10.1007/s11071-018-4675-2
![]() |
[15] |
W. W. Zhang, J. D. Cao, D. Y. Chen, F. E. Alsaadi, Synchronization in fractional-order complex-valued delayed neural networks, Entropy, 20 (2018), 1–16. https://doi.org/10.3390/e20010054 doi: 10.3390/e20010054
![]() |
[16] |
R. H. Li, H. Q. Wu, J. D. Cao, Impulsive exponential synchronization of fractional-order complex dynamical networks with derivative couplings via feedback control based on discrete time state observations, Acta Math. Sci., 42 (2022), 737–754. https://doi.org/10.1007/s10473-022-0219-4 doi: 10.1007/s10473-022-0219-4
![]() |
[17] |
L. Li, Z. Wang, J. W. Lu, Y. X. Li, Adaptive synchronization of fractional-order complex-valued neural networks with discrete and distributed delays, Entropy, 20 (2018), 1–14. https://doi.org/10.3390/e20020124 doi: 10.3390/e20020124
![]() |
[18] |
Y. J. Gu, H. Wang, Y. G. Yu, Synchronization for commensurate Riemann-Liouville fractional-order memristor-based neural networks with unknown parameters, J. Franklin Inst., 357 (2020), 8870–8898. https://doi.org/10.1016/j.jfranklin.2020.06.025 doi: 10.1016/j.jfranklin.2020.06.025
![]() |
[19] |
Y. G. Sun, Y. H. Liu, Adaptive synchronization control and parameters identification for chaotic fractional neural networks with time-varying delays, Neural Process. Lett., 53 (2021), 2729–2745. https://doi.org/10.1007/s11063-021-10517-7 doi: 10.1007/s11063-021-10517-7
![]() |
[20] |
S. X. Liu, Y. G. Yu, S. Zhang, Robust synchronization of memristor-based fractional-order Hopfield neural networks with parameter uncertainties, Neural Comput. Appl., 31 (2019), 3533–3542. https://doi.org/10.1007/s00521-017-3274-3 doi: 10.1007/s00521-017-3274-3
![]() |
[21] |
L. Li, X. G. Liu, M. L. Tang, S. L. Zhang, X. M. Zhang, Asymptotical synchronization analysis of fractional-order complex neural networks with non-delayed and delayed couplings, Neurocomputing, 445 (2021), 180–193. https://doi.org/10.1016/j.neucom.2021.03.001 doi: 10.1016/j.neucom.2021.03.001
![]() |
[22] | A. A. Kilbas, H. M. Srivastava, J. J. Trujillo, Theory and application of fractional differential equations, Elsevier, 2006. |
[23] |
X. X. Zhang, Y. P. Ma, LMIs conditions to robust pinning synchronization of uncertain fractional-order neural networks with discontinuous activations, Soft Comput., 24 (2020), 15927–15935. https://doi.org/10.1007/s00500-020-05315-7 doi: 10.1007/s00500-020-05315-7
![]() |
[24] |
H. Q. Wu, X. X. Zhang, S. H. Xue, L. F. Wang, Y. Wang, LMI conditions to global Mittag-Leffler stability of fractional-order neural networks with impulses, Neurocomputing, 193 (2016), 148–154. https://doi.org/10.1016/j.neucom.2016.02.002 doi: 10.1016/j.neucom.2016.02.002
![]() |
[25] |
X. Peng, H. Q. Wu, Non-fragile robust finite-time stabilization and H∞ performance analysis for fractional-order delayed neural networks with discontinuous activations under the asynchronous switching, Neural Comput. Appl., 32 (2020), 4045–4071. https://doi.org/10.1007/s00521-018-3682-z doi: 10.1007/s00521-018-3682-z
![]() |
[26] |
E. E. Yaz, Linear matrix inequalities in system and control theory, Proc. IEEE, 86 (1998), 2473–2474. https://doi.org/10.1109/JPROC.1998.735454 doi: 10.1109/JPROC.1998.735454
![]() |
[27] |
P. Liu, M. X. Kong, Z. G. Zeng, Projective synchronization analysis of fractional-order neural networks with mixed time delays, IEEE Trans. Cybernet., 52 (2022), 6798–6808. https://doi.org/10.1109/TCYB.2020.3027755 doi: 10.1109/TCYB.2020.3027755
![]() |
[28] |
F. Lin, Z. Q. Zhang, Global asymptotic synchronization of a class of BAM neural networks with time delays via integrating inequality techniques, J. Syst. Sci. Complex., 33 (2020), 366–382. https://doi.org/10.1007/s11424-019-8121-4 doi: 10.1007/s11424-019-8121-4
![]() |
[29] |
S. L. Zhang, M. L. Tang, X. G. Liu, Synchronization of a Riemann-Liouville fractional time-delayed neural network with two inertial terms, Circuits Syst. Signal Process., 40 (2021), 5280–5308. https://doi.org/10.1007/s00034-021-01717-6 doi: 10.1007/s00034-021-01717-6
![]() |
[30] | M. L. Xu, P. Liu, M. X. Kong, J. W. Sun, Anti-synchronization analysis of fractional-order neural networks with time-varying delays, In: 2020 12th International Conference on Advanced Computational Intelligence (ICACI), 2020. https://doi.org/10.1109/ICACI49185.2020.9177766 |
1. | Xiaolin Qu, Wei Li, Chenkai Xing, Xueping Luo, Stability analysis for set-valued inverse mixed variational inequalities in reflexive Banach spaces, 2023, 2023, 1029-242X, 10.1186/s13660-023-03060-7 | |
2. | Pham Ky Anh, Trinh Ngoc Hai, Regularized dynamics for monotone inverse variational inequalities in hilbert spaces, 2024, 25, 1389-4420, 2295, 10.1007/s11081-024-09882-8 |