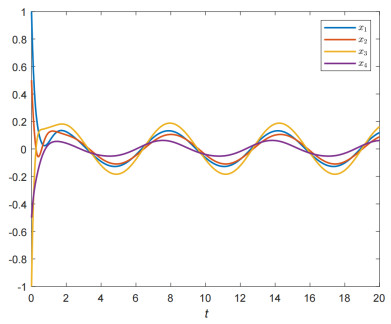
Global exponential periodicity of nonlinear neural networks with multiple time-varying delays is investigated. Such neural networks cannot be written in the vector-matrix form because of the existence of the multiple delays. It is noted that although the neural network with multiple time-varying delays has been investigated by Lyapunov-Krasovskii functional method in the literature, the sufficient conditions in the linear matrix inequality form have not been obtained. Two sets of sufficient conditions in the linear matrix inequality form are established by Lyapunov-Krasovskii functional and linear matrix inequality to ensure that two arbitrary solutions of the neural network with multiple delays attract each other exponentially. This is a key prerequisite to prove the existence, uniqueness, and global exponential stability of periodic solutions. Some examples are provided to demonstrate the effectiveness of the established results. We compare the established theoretical results with the previous results and show that the previous results are not applicable to the systems in these examples.
Citation: Huahai Qiu, Li Wan, Zhigang Zhou, Qunjiao Zhang, Qinghua Zhou. Global exponential periodicity of nonlinear neural networks with multiple time-varying delays[J]. AIMS Mathematics, 2023, 8(5): 12472-12485. doi: 10.3934/math.2023626
[1] | Zhigang Zhou, Li Wan, Qunjiao Zhang, Hongbo Fu, Huizhen Li, Qinghua Zhou . Exponential stability of periodic solution for stochastic neural networks involving multiple time-varying delays. AIMS Mathematics, 2024, 9(6): 14932-14948. doi: 10.3934/math.2024723 |
[2] | Yanshou Dong, Junfang Zhao, Xu Miao, Ming Kang . Piecewise pseudo almost periodic solutions of interval general BAM neural networks with mixed time-varying delays and impulsive perturbations. AIMS Mathematics, 2023, 8(9): 21828-21855. doi: 10.3934/math.20231113 |
[3] | Li Wan, Qinghua Zhou, Hongbo Fu, Qunjiao Zhang . Exponential stability of Hopfield neural networks of neutral type with multiple time-varying delays. AIMS Mathematics, 2021, 6(8): 8030-8043. doi: 10.3934/math.2021466 |
[4] | Qian Cao, Xiaojin Guo . Anti-periodic dynamics on high-order inertial Hopfield neural networks involving time-varying delays. AIMS Mathematics, 2020, 5(6): 5402-5421. doi: 10.3934/math.2020347 |
[5] | Qinghua Zhou, Li Wan, Hongbo Fu, Qunjiao Zhang . Exponential stability of stochastic Hopfield neural network with mixed multiple delays. AIMS Mathematics, 2021, 6(4): 4142-4155. doi: 10.3934/math.2021245 |
[6] | Xiaofang Meng, Yongkun Li . Pseudo almost periodic solutions for quaternion-valued high-order Hopfield neural networks with time-varying delays and leakage delays on time scales. AIMS Mathematics, 2021, 6(9): 10070-10091. doi: 10.3934/math.2021585 |
[7] | Nina Huo, Bing Li, Yongkun Li . Global exponential stability and existence of almost periodic solutions in distribution for Clifford-valued stochastic high-order Hopfield neural networks with time-varying delays. AIMS Mathematics, 2022, 7(3): 3653-3679. doi: 10.3934/math.2022202 |
[8] | Er-yong Cong, Li Zhu, Xian Zhang . Global exponential synchronization of discrete-time high-order BAM neural networks with multiple time-varying delays. AIMS Mathematics, 2024, 9(12): 33632-33648. doi: 10.3934/math.20241605 |
[9] | Jin Gao, Lihua Dai . Weighted pseudo almost periodic solutions of octonion-valued neural networks with mixed time-varying delays and leakage delays. AIMS Mathematics, 2023, 8(6): 14867-14893. doi: 10.3934/math.2023760 |
[10] | Qinghua Zhou, Li Wan, Hongshan Wang, Hongbo Fu, Qunjiao Zhang . Exponential stability of Cohen-Grossberg neural networks with multiple time-varying delays and distributed delays. AIMS Mathematics, 2023, 8(8): 19161-19171. doi: 10.3934/math.2023978 |
Global exponential periodicity of nonlinear neural networks with multiple time-varying delays is investigated. Such neural networks cannot be written in the vector-matrix form because of the existence of the multiple delays. It is noted that although the neural network with multiple time-varying delays has been investigated by Lyapunov-Krasovskii functional method in the literature, the sufficient conditions in the linear matrix inequality form have not been obtained. Two sets of sufficient conditions in the linear matrix inequality form are established by Lyapunov-Krasovskii functional and linear matrix inequality to ensure that two arbitrary solutions of the neural network with multiple delays attract each other exponentially. This is a key prerequisite to prove the existence, uniqueness, and global exponential stability of periodic solutions. Some examples are provided to demonstrate the effectiveness of the established results. We compare the established theoretical results with the previous results and show that the previous results are not applicable to the systems in these examples.
When we design a nonlinear delayed neural network and apply it to some practical applications including collect computation, associative memory, pattern recognition, learning theory, and optimization [1,2,3], we have to consider the existence and stability of the equilibrium point of the delayed neural network. This is because the stable equilibrium point plays an important role in these applications and can avoid some suboptimal responses. Some stability results of various types of nonlinear delayed neural network have been published, see [4,5,6,7,8,9,10,11,12,13,14,15,16] and the references cited.
The periodic solution is also an important dynamical behavior of the nonlinear delayed neural networks. It is clear that an equilibrium point is a special case of the periodic solution because it can be viewed as a special periodic solution with an arbitrary period. So the analysis of the periodic solution of the nonlinear delayed neural network is more meaningful and complicated. The periodic solution of a class of nonlinear delayed neural networks has been used to learning theory because learning often needs to be repeated [17]. The neural network studied in this paper is a class of recurrent neural networks. As known, periodic solution of recurrent neural network can be used to store acoustic characteristics in speech recognition. The reason why the periodic solutions of a recurrent neural network can store the acoustic characteristics is that the periodic solution contains the information for a time series, and the length of the time series usually corresponds to the length of the acoustic characteristics. In practice, a time series of an audio signal is fed as input into the network, if the weights of the network meet our result, then the output is the periodic solution of the time series. We can calculate the average power spectral density (PSD) of the periodic solution and compare it with the PSD of the original audio signal. If the difference between them is small, then the periodic solution can be considered as effectively storing the acoustic characteristics. Some interesting and valuable results of periodic solutions of various types of nonlinear delayed neural networks have been obtained, see [18,19,20,21,22,23,24,25,26,27,28] and the references cited.
After reading the references cited above, we notice that neural networks with multiple delays τij(t) cannot be converted into the vector-matrix form because of the existence of the multiple delays τij(t). What's more, we notice that although the neural network with multiple delays τij(t) have been actually investigated by Lyapunov-Krasovskii functional method in the literature, to the best of our knowledge, the sufficient conditions in the linear matrix inequality form have not been obtained. That is to say, Lyapunov-Krasovskii functional method and linear matrix inequality method have not been simultaneously used to study the neural network that cannot be converted into the vector-matrix form. Therefore, we naturally wonder whether we can use two methods together to study the neural networks with multiple delays.
The innovations of this paper are listed in the following.
1) By using Lyapunov-Krasovskii functional and linear matrix inequality, we establish two sets of sufficient conditions in the linear matrix inequality form to ensure that two arbitrary solutions of the neural network with multiple delays attracts each other exponentially. This is a key prerequisite to prove the periodic solution of the neural network with multiple delays is existent, unique and globally exponentially stable.
2) The established sufficient conditions can also ensure the exponential stability of the equilibrium point of the neural network with multiple delays.
3) We confirm that Lyapunov-Krasovskii functional and linear matrix inequality can also be applicable to the neural networks which cannot be written in the vector-matrix form. So Lyapunov-Krasovskii functional and linear matrix inequality can be applicable to the neural networks that can or cannot be written in the vector-matrix form.
4) We provide several examples to show that compared with the existing results derived by differential inequality, matrix theory, Laypunov functional and Halanay inequality, the established sufficient conditions are less conservative.
The nonlinear neural system with multiple time-varying delays studied in this paper can be described as the following mathematical expression
˙xi(t)=−cixi(t)+n∑j=1aijfj(xj(t))+n∑j=1bijgj(xj(t−τij(t)))+ui(t),t≥0, | (2.1) |
in which i=1,⋯,n,ci>0, the nonlinear activation functions fi(⋅),gi(⋅) and the multiple time-varying delays τij(t) satisfy that there exist some constants α−i,α+i,β−i,β+i,τ and ˜τ such that for all z1,z2∈R(z1≠z2), α−i≤α+i,β−i≤β+i and t≥0,
α−i≤fi(z1)−fi(z2)z1−z2≤α+i,β−i≤gi(z1)−gi(z2)z1−z2≤β+i, | (2.2) |
0≤τij(t)≤τ,˙τij(t)≤˜τ<1. | (2.3) |
The initial conditions are xi(t)=ξi(t),t∈[−τ,0],i=1,⋯,n. Let ξ={(ξ1(t),⋯,ξn(t))T:t∈[−τ,0]} be C([−τ,0],Rn)-valued function with
||ξ||2=sup−τ≤t≤0‖ξ(t)‖2<∞, |
in which C([−τ,0],Rn) denotes the space of all continuous Rn-valued functions defined on [−τ,0].
It is easy to see that the mathematical expression of system (2.1) cannot be transformed into a vector system. By changing some functions in the mathematical expression, the following systems studied in [4,18,19] are some special cases of system (2.1):
˙xi(t)=−cixi(t)+n∑j=1aijfj(xj(t))+n∑j=1bijgj(xj(t−τj(t)))+ui(t), | (2.4) |
˙xi(t)=−cixi(t)+n∑j=1aijfj(xj(t))+n∑j=1bijfj(xj(t−τij(t)))+ui, | (2.5) |
˙xi(t)=−cixi(t)+n∑j=1aijfj(xj(t))+n∑j=1bijfj(xj(t−τij(t)))+ui(t). | (2.6) |
Let x(t,ξ) and y(t,ψ) be the solutions of system (2.1) with arbitrary initial conditions ξ and ψ, respectively. For simplicity and convenience, two arbitrary solutions x(t,ξ) and y(t,ψ) denote by x(t) and y(t), respectively. In addition, Σ<0 denotes matrix Σ is symmetric negative definite, ∗ means the symmetric terms of symmetric matrix Σ. Define
A=(aij)n×n,C=diag{c1,⋯,cn},P=diag{p1,⋯,pn}, |
K1=diag{k11,⋯,k1n},K2=diag{k21,⋯,k2n}, |
B1=diag{n∑j=1|b1j|,⋯,n∑j=1|bnj|},B2=diag{n∑j=1pj|bj1|,⋯,n∑j=1pj|bjn|}, |
B3=diag{n∑j=1|bj1|,⋯,n∑j=1|bjn|}, |
˜α=diag{α−1α+1,⋯,α−nα+n},ˉα=diag{α−1+α+1,⋯,α−n+α+n}, |
˜β=diag{β−1β+1,⋯,β−nβ+n},ˉβ=diag{β−1+β+1,⋯,β−n+β+n}. |
We first show that two arbitrary solutions x(t) and y(t) of system (2.1) attract each other exponentially.
Theorem 1. Suppose that there exist some positive constants p1,⋯,pn,ki1,⋯,kin (i=1,2) such that
Σ=(−2PC+PB1−2K1˜α−2K2˜βPA+K1ˉαK2ˉβ∗−2K10∗∗−2K2+(1−˜τ)−1B2)<0. |
Then, there exist two positive constants λ and M>1 such that
‖x(t)−y(t)‖2≤Me−λt‖ξ−ψ‖2,t≥0. | (3.1) |
Proof. It follows from Σ<0 that there exists a constant λ>0 such that
ˉΣ=(λP−2PC+PB1−2K1˜α−2K2˜βPA+K1ˉαK2ˉβ∗−2K10∗∗−2K2+(1−˜τ)−1eλτB2)<0. | (3.2) |
We construct the following Lyapunov-Krasovskii functional
V(t)=eλtn∑i=1pi[xi(t)−yi(t)]2+(1−˜τ)−1n∑i=1n∑j=1∫tt−τij(t)eλ(s+τ)pi|bij|[gj(xj(s))−gj(yj(s))]2ds, | (3.3) |
and derive
˙V(t)=λeλtn∑i=1pi[xi(t)−yi(t)]2+eλtn∑i=12pi[xi(t)−yi(t)][˙xi(t)−˙yi(t)]+(1−˜τ)−1n∑i=1n∑j=1(eλ(t+τ)pi|bij|[gj(xj(t))−gj(yj(t))]2−(1−˙τij(t))eλ(t−τij(t)+τ)pi|bij|[gj(xj(t−τij(t)))−gj(yj(t−τij(t)))]2)=λeλtn∑i=1pi[xi(t)−yi(t)]2+2eλtn∑i=1pi[xi(t)−yi(t)](−ci[xi(t)−yi(t)]+n∑j=1aij[fj(xj(t))−fj(yj(t))]+n∑j=1bij[gj(xj(t−τij(t)))−gj(yj(t−τij(t)))])+(1−˜τ)−1n∑i=1n∑j=1(eλ(t+τ)pi|bij|[gj(xj(t))−gj(yj(t))]2−(1−˙τij(t))eλ(t−τij(t)+τ)pi|bij|[gj(xj(t−τij(t)))−gj(yj(t−τij(t)))]2)≤λeλtn∑i=1pi[xi(t)−yi(t)]2+eλtn∑i=1(−2cipi[xi(t)−yi(t)]2+n∑j=12aijpi[xi(t)−yi(t)][fj(xj(t))−fj(yj(t))]+n∑j=1|bij|pi[xi(t)−yi(t)]2+n∑j=1|bij|pi[gj(xj(t−τij(t)))−gj(yj(t−τij(t)))]2)+n∑i=1n∑j=1(eλ(t+τ)(1−˜τ)−1pi|bij|[gj(xj(t))−gj(yj(t))]2−eλtpi|bij|[gj(xj(t−τij(t)))−gj(yj(t−τij(t)))]2)=eλtn∑i=1([λpi−2cipi+n∑j=1|bij|pi][xi(t)−yi(t)]2+n∑j=12aijpi[xi(t)−yi(t)][fj(xj(t))−fj(yj(t))])+n∑i=1n∑j=1eλ(t+τ)(1−˜τ)−1pj|bji|[gi(xi(t))−gi(yi(t))]2=eλt{[x(t)−y(t)]T(λP−2PC+PB1)[x(t)−y(t)]+2[x(t)−y(t)]TPA[f(x(t))−f(y(t))]+eλτ(1−˜τ)−1[g(x(t))−g(y(t))]TB2[g(x(t))−g(y(t))]}, | (3.4) |
in which
x(t)−y(t)=(x1(t)−y1(t),⋯,xn(t)−yn(t))T, |
f(x(t))−f(y(t))=(f1(x1(t))−f1(y1(t)),⋯,fn(xn(t))−fn(yn(t)))T, |
g(x(t))−g(y(t))=(g1(x1(t))−g1(y1(t)),⋯,gn(xn(t))−gn(yn(t)))T. |
In addition, it is easy to deduce the following inequalities from (2.2):
0≤−2n∑i=1k1i[(fi(xi(t))−fi(yi(t)))−α+i(xi(t)−yi(t))][(fi(xi(t))−fi(yi(t)))−α−i(xi(t)−yi(t))] |
=−2n∑i=1k1i(fi(xi(t))−fi(yi(t)))2−2n∑i=1k1iα+iα−i(xi(t)−yi(t))2+2(α+i+α−i)n∑i=1k1i(fi(xi(t))−fi(yi(t)))(xi(t)−yi(t))=−2[f(x(t))−f(y(t))]TK1[f(x(t))−f(y(t))]−2[x(t)−y(t)]TK1˜α[x(t)−y(t)]+2[f(x(t))−f(y(t))]TK1ˉα[x(t)−y(t)], | (3.5) |
and
0≤−2n∑i=1k2i[(gi(xi(t))−gi(yi(t)))−β+i(xi(t)−yi(t))][(gi(xi(t))−gi(yi(t)))−β−i(xi(t)−yi(t))]=−2[g(x(t))−g(y(t))]TK2[g(x(t))−g(y(t))]−2[x(t)−y(t)]TK2˜β[x(t)−y(t)]+2[g(x(t))−g(y(t))]TK2ˉβ[x(t)−y(t)]. | (3.6) |
Let γ(t)=([x(t)−y(t)]T,[f(x(t))−f(y(t))]T,[g(x(t))−g(y(t))]T)T,βi=max{|β−i|,|β+i|},i=1,2,⋯,n. Then, from (3.2)–(3.6), we deduce
eλtmin1≤i≤n{pi}‖x(t)−y(t)‖2≤V(t)≤V(0)+∫t0˙V(s)ds≤V(0)+∫t0eλsγT(s)ˉΣγ(s)ds≤V(0)≤max1≤i≤n{pi}‖x(0)−y(0)‖2+(1−˜τ)−1n∑i=1n∑j=1∫0−τeλ(s+τ)pi|bij|[gj(xj(s))−gj(yj(s))]2ds≤(max1≤i≤n{pi}+(1−˜τ)−1eλττmax1≤i≤n{n∑j=1pj|bji|β2i})‖ξ−ψ‖2. |
So (3.1) holds and the proof is finished.
Remark 1. Although Theorem 1 gives the sufficient conditions in the linear matrix inequality form, MATLAB executables that solve the matrices P, K1, and K2 are difficultly written because the matrix B2 contains all elements of the undetermined matrix P. Therefore, it is more expected to obtain some easily verified sufficient conditions.
Theorem 2. Inequality (3.1) holds provided that there exist some positive constants p1,⋯,pn,ki1,⋯,kin (i=1,2) such that
Σ=(−2PC+P2B1−2K1˜α−2K2˜βPA+K1ˉαK2ˉβ∗−2K10∗∗−2K2+(1−˜τ)−1B3)<0. | (3.7) |
Proof. It follows from Σ<0 that there exists a constant λ>0 such that
ˉΣ=(λP−2PC+P2B1−2K1˜α−2K2˜βPA+K1ˉαK2ˉβ∗−2K10∗∗−2K2+(1−˜τ)−1eλτB3)<0. |
We construct the following Lyapunov-Krasovskii functional
V(t)=eλtn∑i=1pi[xi(t)−yi(t)]2+(1−˜τ)−1n∑i=1n∑j=1∫tt−τij(t)eλ(s+τ)|bij|[gj(xj(s))−gj(yj(s))]2ds, |
and derive
˙V(t)≤λeλtn∑i=1pi[xi(t)−yi(t)]2+eλtn∑i=1(−2cipi[xi(t)−yi(t)]2+n∑j=12aijpi[xi(t)−yi(t)][fj(xj(t))−fj(yj(t))]+n∑j=1|bij|p2i[xi(t)−yi(t)]2+n∑j=1|bij|[gj(xj(t−τij(t)))−gj(yj(t−τij(t)))]2)+n∑i=1n∑j=1(eλ(t+τ)(1−˜τ)−1|bij|[gj(xj(t))−gj(yj(t))]2−eλt|bij|[gj(xj(t−τij(t)))−gj(yj(t−τij(t)))]2)=eλt{[x(t)−y(t)]T(λP−2PC+P2B1)[x(t)−y(t)]+2[x(t)−y(t)]TPA[f(x(t))−f(y(t))]+eλτ(1−˜τ)−1[g(x(t))−g(y(t))]TB3[g(x(t))−g(y(t))]}. |
The rest proof is similar to that of Theorem 1 and is omitted.
If pi=p(i=1,⋯,n) in Theorem 1, then we obtain the following easily verified condition.
Theorem 3. Inequality (3.1) holds provided that there exist some positive constants p,ki1,⋯,kin(i=1,2) such that
Σ=(−2pC+pB1−2K1˜α−2K2˜βpA+K1ˉαK2ˉβ∗−2K10∗∗−2K2+(1−˜τ)−1pB3)<0. | (3.8) |
It is obvious that the matrices P, K1, and K2 in (3.7) and (3.8) can be easily determined by MATLAB LMI Control Toolbox. The focus of this paper is to establish (3.7) or (3.8) to ensure (3.1) holds. Based on (3.1), we can define a Poincarˊe mapping and use the contraction mapping principle to show the periodic solution of system (2.1) is existent, unique and globally exponentially stable. The proof is common and similar to that of [19], so it is omitted.
Theorem 4. Let ui(t) and τij(t)(i,j=1,⋯,n) be ω-periodic functions. Then, under the conditions of Theorem 2 (or Theorem 3), there exists a unique and globally exponentially stable ω-periodic solution of system (2.1).
Remark 2. Let ui(t)=ui(i=1,⋯,n) in (2.1). Then, under the conditions of Theorem 2 (or Theorem 3), there exists a unique and globally exponentially stable equilibrium point of system (2.1).
For system (2.5) and system (2.6), we can easily derive the following result.
Theorem 5. Let ui(t) and τij(t)(i,j=1,⋯,n) be ω-periodic functions. Then, system (2.6) has a unique and globally exponentially stable periodic solution provided that one of the following conditions is true:
(ⅰ) There exist some positive constants p1,⋯,pn,ki1,⋯,kin (i=1,2) such that
Σ=(−2PC+P2B1−2(K1+K2)˜αPA+K1ˉαK2ˉα∗−2K10∗∗−2K2+(1−˜τ)−1B3)<0. |
(ⅱ) There exist some positive constants p,ki1,⋯,kin(i=1,2) such that
Σ=(−2pC+pB1−2(K1+K2)˜αpA+K1ˉαK2ˉα∗−2K10∗∗−2K2+(1−˜τ)−1pB3)<0. |
In particular, system (2.5) has a unique and globally exponentially stable equilibrium point if one of the above conditions is true.
Remark 3. In [18], the author has used the theory of fixed point and differential inequality technique to prove there exists exactly one ω-periodic solution of system (2.4) if α=ξ||A||2+η||B||2c0<1, where ξ=max1≤i≤n{supxi≠0fi(xi)xi},η=max1≤i≤n{supxi≠0gi(xi)xi},c0=min1≤i≤n{ci},||A||2 denotes the square root of the largest eigenvalue of ATA. For system (2.4) in Example 1, we demonstrate Theorem 4 is effective and calculate α=5.0145>1 which implies that the result in [18] is not applicable.
Remark 4. In [4], the authors have used Brouwer's fixed point theorem, matrix theory and inequality analysis to prove system (2.5) has exactly one equilibrium which is globally exponentially stable if ρ(K)<1, where ρ(K) denotes spectral radius of matrix K=(kij)n×n, kij=c−1i(|aij|+|bij|)pj,pj=max{|α−j|,|α+j|} in this paper. For system (2.5) in Example 2, we demonstrate Theorem 5 is effective and calculate ρ(K)=1 which implies that the result in [4] is not applicable.
Remark 5. In [19], the authors have used Laypunov functional and Halanay inequality to prove system (2.6) is globally exponentially periodic provided that di−Li∑nj=1(|aji|+|bji|)>0 or di−∑nj=1(|aij|+|bij|)Lj>0,i=1,⋯,n, where di=ci and Lj=max{|α−j|,|α+j|} in this paper. For system (2.6) in Example 3, we demonstrate Theorem 5 is effective and calculate di−Li∑4j=1(|aji|+|bji|)=di−∑4j=1(|aij|+|bij|)Lj=0,i=1,⋯,n, which implies that the results in [19] are not applicable.
Example 1. Consider system (2.4) with the following parameters and functions:
A=(aij)4×4=(1−1−11−11−1−111−11−1−1−1−1),B=(bij)4×4=(−11−11−1−11−11−1−1−1−1−1−11), |
C=diag{6,6,5,5},fi(x)=0.5tanh(xi),gi(xi)=0.8tanh(xi),ui=sint, τi(t)=0.2sint+0.2,i=1,2,3,4.
We calculate that B1=B3=4I,˜α=˜β=0,ˉα=I,ˉβ=0.8I,˜τ=0.2, where I denotes identity matrix.
By using MATLAB LMI Control Toolbox, we obtain P=diag{4.8363,4.8363,7.6076,7.6076}, K1=diag{17.0542,17.0542,20.6096,20.6096},K2=22.1420I and P=0.1564I,K1=diag{0.4524,0.4509,0.4781,0.4770},K2=diag{0.7300,0.7301,0.7325,0.7326} which satisfy the conditions of Theorem 2 and Theorem 3, respectively. So our theoretical results are effective. Figure 1 shows the solution trajectories of system (2.4) move periodically. Figure 2 shows the average PSD of xi(t) and ui(t)=sint are relatively close, which implies the periodic solution x(t) stores effectively the acoustic characteristics of u(t).
On the other hand, we calculate ξ=1,η=0.8,||A||2=√8,||B||2=√7.4641,c0=min1≤i≤4{ci}=5 and
α=ξ||A||2+η||B||2c0=5.0145>1 |
defined in Remark 3. Therefore, the result proposed in [18] is not applicable to system (2.4) in this example.
Example 2. Consider system (2.5) with C=diag{5,5,5,5},fi(xi)=0.5tanh(xi),ui=1,τii(t)=0.2sint+0.2, τij(t)=0.2cost+0.2,i≠j,i,j=1,2,3,4, the matrices A and B are the same as Example 1.
We calculate that B1=B3=4I,˜α=0,ˉα=0.5I,˜τ=0.2. By using MATLAB LMI Control Toolbox, we obtain P=diag{6.2027,6.2027,7.2511,7.2511},K1=diag{22.3647,22.3647,24.8109,24.8109},K2=25.3107I and P=2.4441I,K1=diag{9.6738,9.6738,10.5625,10.5625},K2=14.5619I which satisfy the condition (ⅰ) and the condition (ⅱ) of Theorem 5, respectively. Figure 3 shows the solution trajectories of system (2.5) tend to a point.
On the other hand, we calculate that pi=0.5,i=1,2,3,4, and ρ(K)=1 defined in Remark 4. Therefore, the result proposed in [4] is not applicable to system (2.5) in this example.
Example 3. Consider system (2.6) with C=diag{4,4,4,4},ui=sint, the matrices A and B are the same as Example 1 and other functions are defined in Example 2.
We calculate that B1=B3=4I,˜α=0,ˉα=0.5I,˜τ=0.2. By using MATLAB LMI Control Toolbox, we obtain P=diag{7.5477,7.5477,9.3961,9.3961},K1=diag{21.5649,21.5649,24.6456,24.6456},K2=24.7554I and P=0.1679I,K1=diag{0.5707,0.5707,0.6318,0.6318},K2=0.9065I which satisfy the condition (ⅰ) and the condition (ⅱ) of Theorem 5, respectively. Figure 4 shows the solution trajectories of system (2.6) move periodically.
On the other hand, we calculate that di=4,Li=0.5,di−Li∑4j=1(|aji|+|bji|)= di−∑4j=1(|aij|+|bij|)Lj=0,i=1,2,3,4, defined in Remark 5. Therefore, the results proposed in [19] are not applicable to system (2.6) in this example.
This paper has investigated the global exponential periodicity of nonlinear neural networks with multiple time-varying delays. This type of neural system cannot be transformed into the vector-matrix form because the multiple time-varying delays can not be expressed by the vector form. We note that in many existing references, although the neural networks with multiple delays τij(t) have been actually investigated by Lyapunov-Krasovskii functional method, the linear matrix inequality approach has not been used for this type of neural system that cannot be written in vector-matrix form. So we want to know whether we can use two methods together to study the neural networks with multiple delays. Two sets of sufficient conditions in the linear matrix inequality form are established to ensure two arbitrary solutions of nonlinear neural networks with multiple time-varying delays attract each other exponentially which can lead us to prove the periodic solution of the neural networks with multiple delays is existent, unique and globally exponentially stable by the contraction mapping principle. So the focus of this paper is to establish (3.7) or (3.8) which ensure (3.1) holds. Three examples are given to demonstrate our results are effective. In these examples, we compare our results with the existing results derived by differential inequality, matrix theory, Laypunov functional and Halanay inequality. Three existing results are not applicable to the systems in the three examples, respectively.
We would like to thank the editor and the reviewers for their detailed comments and valuable suggestions. This work was supported by the National Natural Science Foundation of China (Nos: 12271416, 11971367, 11826209 and 11271295), the Natural Science Foundation of Guangdong Province (2018A030313536).
The authors declare no conflicts of interest.
[1] |
P. N. Suganthan, E. K. Teoh, D. P. Mital, Pattern recognition by homomorphic graph matching using Hopfield neural networks, Image Vis. Comput., 13 (1995), 45–60. https://doi.org/10.1016/0262-8856(95)91467-R doi: 10.1016/0262-8856(95)91467-R
![]() |
[2] |
T. Deb, A. K. Ghosh, A. Mukherjee, Singular value decomposition applied to associative memory of Hopfield neural network, Mater. Today, 5 (2018), 2222–2228. https://doi.org/10.1016/j.matpr.2017.09.222 doi: 10.1016/j.matpr.2017.09.222
![]() |
[3] |
V. Donskoy, BOMD: Building optimization models from data (neural networks based approach), Quant. Finance Econ., 3 (2019), 608–623. https://doi.org/10.3934/QFE.2019.4.608 doi: 10.3934/QFE.2019.4.608
![]() |
[4] |
L. H. Huang, C. X. Huang, B. W. Liu, Dynamics of a class of cellular neural networks with time-varying delays, Phys. Lett. A, 345 (2005), 330–344. https://doi.org/10.1016/j.physleta.2005.07.039 doi: 10.1016/j.physleta.2005.07.039
![]() |
[5] |
X. D. Li, D. O'Regan, H. Akca, Global exponential stabilization of impulsive neural networks with unbounded continuously distributed delays, IMA J. Appl. Math., 80 (2015), 85–99. https://doi.org/10.1093/imamat/hxt027 doi: 10.1093/imamat/hxt027
![]() |
[6] |
S. Zhang, Y. G. Yu, Q. Wang, Stability analysis of fractional-order Hopfield neural networks with discontinuous activation functions, Neurocomputing, 171 (2016), 1075–1084. https://doi.org/10.1016/j.neucom.2015.07.077 doi: 10.1016/j.neucom.2015.07.077
![]() |
[7] |
C. J. Xu, P. L. Li, Global exponential convergence of neutral-type Hopfield neural networks with multi-proportional delays and leakage delays, Chaos Soliton. fract., 96 (2017), 139–144. https://doi.org/10.1016/j.chaos.2017.01.012 doi: 10.1016/j.chaos.2017.01.012
![]() |
[8] |
Y. H. Zhou, C. D. Li, H. Wang, Stability analysis on state-dependent impulsive Hopfield neural networks via fixed-time impulsive comparison system method, Neurocomputing, 316 (2018), 20–29. https://doi.org/10.1016/j.neucom.2018.07.047 doi: 10.1016/j.neucom.2018.07.047
![]() |
[9] |
S. Arik, A modified Lyapunov functional with application to stability of neutral-type neural networks with time delays, J. Franklin Inst., 356 (2019), 276–291. https://doi.org/10.1016/j.jfranklin.2018.11.002 doi: 10.1016/j.jfranklin.2018.11.002
![]() |
[10] |
F. X. Wang, X. G. Liu, M. L. Tang, L. F. Chen, Further results on stability and synchronization of fractional-order Hopfield neural networks, Neurocomputing, 346 (2019), 12–19. https://doi.org/10.1016/j.neucom.2018.08.089 doi: 10.1016/j.neucom.2018.08.089
![]() |
[11] |
W. Q. Shen, X. Zhang, Y. T. Wang, Stability analysis of high order neural networks with proportional delays, Neurocomputing, 372 (2020), 33–39. https://doi.org/10.1016/j.neucom.2019.09.019 doi: 10.1016/j.neucom.2019.09.019
![]() |
[12] |
O. Faydasicok, A new Lyapunov functional for stability analysis of neutral-type Hopfield neural networks with multiple delays, Neural Networks, 129 (2020), 288–297. https://doi.org/10.1016/j.neunet.2020.06.013 doi: 10.1016/j.neunet.2020.06.013
![]() |
[13] |
H. Wang, G. L. Wei, S. Wen, T. Huang, Generalized norm for existence, uniqueness and stability of Hopfield neural networks with discrete and distributed delays, Neural Networks, 128 (2020), 288–293. https://doi.org/10.1016/j.neunet.2020.05.014 doi: 10.1016/j.neunet.2020.05.014
![]() |
[14] |
Q. K. Song, Y. X. Chen, Z. J. Zhao, Y. R. Liu, F. E. Alsaadi, Robust stability of fractional-order quaternion-valued neural networks with neutral delays and parameter uncertainties, Neurocomputing, 420 (2021), 70–81. https://doi.org/10.1016/j.neucom.2020.08.059 doi: 10.1016/j.neucom.2020.08.059
![]() |
[15] |
Y. K. Deng, C. X. Huang, J. D. Cao, New results on dynamics of neutral type HCNNs with proportional delays, Math. Comput. Simul., 187 (2021), 51–59. https://doi.org/10.1016/j.matcom.2021.02.001 doi: 10.1016/j.matcom.2021.02.001
![]() |
[16] |
Q. K. Song, R. T. Zeng, Z. J. Zhao, Y. R. Liu, F. E. Alsaadi, Mean-square stability of stochastic quaternion-valued neural networks with variable coefficients and neutral delays, Neurocomputing, 471 (2022), 130–138. https://doi.org/10.1016/j.neucom.2021.11.033 doi: 10.1016/j.neucom.2021.11.033
![]() |
[17] |
S. Townley, A. Ilchmann, M. G. Weiss, W. Mcclements, A. C. Ruiz, D. H. Owens, et al., Existence and learning of oscillations in recurrent neural networks, IEEE Trans. Neural Networks, 11 (2000), 205–214. https://doi.org/10.1109/72.822523 doi: 10.1109/72.822523
![]() |
[18] |
H. Y. Zhao, Global exponential stability and periodicity of cellular neural networks with variable delays, Phys. Lett. A, 336 (2005), 331–341. https://doi.org/10.1016/j.physleta.2004.12.001 doi: 10.1016/j.physleta.2004.12.001
![]() |
[19] |
L. Q. Zhou, G. D. Hu, Global exponential periodicity and stability of cellular neural networks with variable and distributed delays, Appl. Math. Comput., 195 (2008), 402–411. https://doi.org/10.1016/j.amc.2007.04.114 doi: 10.1016/j.amc.2007.04.114
![]() |
[20] |
L. Yang, Y. K. Li, Existence and exponential stability of periodic solution for stochastic Hopfield neural networks on time scales, Neurocomputing, 167 (2015), 543–550. https://doi.org/10.1016/j.neucom.2015.04.038 doi: 10.1016/j.neucom.2015.04.038
![]() |
[21] |
C. Wang, Piecewise pseudo-almost periodic solution for impulsive non-autonomous high-order Hopfield neural networks with variable delays, Neurocomputing, 171 (2016), 1291–1301. https://doi.org/10.1016/j.neucom.2015.07.054 doi: 10.1016/j.neucom.2015.07.054
![]() |
[22] |
A. M. Alimi, C. Aouiti, F. Chérif, F. Dridi, M. S. M'hamdi, Dynamics and oscillations of generalized high-order Hopfield neural networks with mixed delays, Neurocomputing, 321 (2018), 274–295. https://doi.org/10.1016/j.neucom.2018.01.061 doi: 10.1016/j.neucom.2018.01.061
![]() |
[23] |
S. Gao, R. Shen, T. R. Chen, Periodic solutions for discrete-time Cohen-Grossberg neural networks with delays, Phys. Lett. A, 383 (2019), 414–420. https://doi.org/10.1016/j.physleta.2018.11.016 doi: 10.1016/j.physleta.2018.11.016
![]() |
[24] |
F. C. Kong, Q. X. Zhu, K. Wang, J. J. Nieto, Stability analysis of almost periodic solutions of discontinuous BAM neural networks with hybrid time-varying delays and D operator, J. Franklin Inst., 356 (2019), 11605–11637. https://doi.org/10.1016/j.jfranklin.2019.09.030 doi: 10.1016/j.jfranklin.2019.09.030
![]() |
[25] |
M. Abdelaziz, F. Cherif, Piecewise asymptotic almost periodic solutions for impulsive fuzzy Cohen-Grossberg neural networks, Chaos Soliton. Fract., 132 (2020), 109575. https://doi.org/10.1016/j.chaos.2019.109575 doi: 10.1016/j.chaos.2019.109575
![]() |
[26] |
F. C. Kong, Y. Ren, R. Sakthivel, Delay-dependent criteria for periodicity and exponential stability of inertial neural networks with time-varying delays, Neurocomputing, 419 (2021), 261–272. https://doi.org/10.1016/j.neucom.2020.08.046 doi: 10.1016/j.neucom.2020.08.046
![]() |
[27] |
Z. W. Cai, L. H. Huang, Z. Y. Wang X. M. Pan, S. K. Liu, Periodicity and multi-periodicity generated by impulses control in delayed Cohen-Grossberg-type neural networks with discontinuous activations, Neural Networks, 143 (2021), 230–245. https://doi.org/10.1016/j.neunet.2021.06.013 doi: 10.1016/j.neunet.2021.06.013
![]() |
[28] |
H. Li, Y. G. Kao, I. Stamov, C. T. Shao, Global asymptotic stability and S-asymptotic ω-periodicity of impulsive non-autonomous fractional-order neural networks, Appl. Math. Comput., 410 (2021), 126459. https://doi.org/10.1016/j.amc.2021.126459 doi: 10.1016/j.amc.2021.126459
![]() |
1. | Changgui Wu, Liang Zhao, Finite-time adaptive dynamic surface control for output feedback nonlinear systems with unmodeled dynamics and quantized input delays, 2024, 9, 2473-6988, 31553, 10.3934/math.20241518 | |
2. | Wenlong Xue, Yufeng Tian, Zhenghong Jin, A novel nonzero functional method to extended dissipativity analysis for neural networks with Markovian jumps, 2024, 9, 2473-6988, 19049, 10.3934/math.2024927 | |
3. | A. Karnan, G. Soundararajan, G. Nagamani, Ardak Kashkynbayev, Design of an event-triggered extended dissipative state estimator for neural networks with multiple time-varying delays, 2024, 1951-6355, 10.1140/epjs/s11734-024-01240-0 | |
4. | Wenlong Xue, Zhenghong Jin, Yufeng Tian, Stability analysis of delayed neural networks via compound-parameter -based integral inequality, 2024, 9, 2473-6988, 19345, 10.3934/math.2024942 |