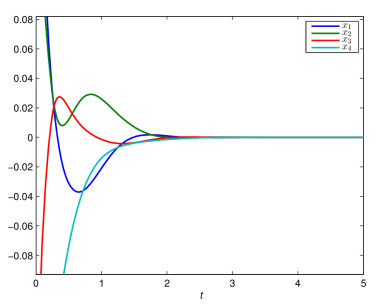
Maybe because Cohen-Grossberg neural networks with multiple time-varying delays and distributed delays cannot be converted into the vector-matrix forms, the stability results of such networks are relatively few and the stability conditions in the linear matrix inequality forms have not been established. So this paper investigates the exponential stability of the networks and gives the sufficient condition in the linear matrix inequality forms. Two examples are provided to demonstrate the effectiveness of the theoretical results.
Citation: Qinghua Zhou, Li Wan, Hongshan Wang, Hongbo Fu, Qunjiao Zhang. Exponential stability of Cohen-Grossberg neural networks with multiple time-varying delays and distributed delays[J]. AIMS Mathematics, 2023, 8(8): 19161-19171. doi: 10.3934/math.2023978
[1] | Yijia Zhang, Tao Xie, Yunlong Ma . Robustness analysis of exponential stability of Cohen-Grossberg neural network with neutral terms. AIMS Mathematics, 2025, 10(3): 4938-4954. doi: 10.3934/math.2025226 |
[2] | Biwen Li, Yibo Sun . Stability analysis of Cohen-Grossberg neural networks with time-varying delay by flexible terminal interpolation method. AIMS Mathematics, 2023, 8(8): 17744-17764. doi: 10.3934/math.2023906 |
[3] | Pratap Anbalagan, Evren Hincal, Raja Ramachandran, Dumitru Baleanu, Jinde Cao, Chuangxia Huang, Michal Niezabitowski . Delay-coupled fractional order complex Cohen-Grossberg neural networks under parameter uncertainty: Synchronization stability criteria. AIMS Mathematics, 2021, 6(3): 2844-2873. doi: 10.3934/math.2021172 |
[4] | Ruoyu Wei, Jinde Cao, Wenhua Qian, Changfeng Xue, Xiaoshuai Ding . Finite-time and fixed-time stabilization of inertial memristive Cohen-Grossberg neural networks via non-reduced order method. AIMS Mathematics, 2021, 6(7): 6915-6932. doi: 10.3934/math.2021405 |
[5] | Tao Xie, Wenqing Zheng . Robustness analysis of Cohen-Grossberg neural network with piecewise constant argument and stochastic disturbances. AIMS Mathematics, 2024, 9(2): 3097-3125. doi: 10.3934/math.2024151 |
[6] | Mohammed D. Kassim . A fractional Halanay inequality for neutral systems and its application to Cohen-Grossberg neural networks. AIMS Mathematics, 2025, 10(2): 2466-2491. doi: 10.3934/math.2025115 |
[7] | Shuting Chen, Ke Wang, Jiang Liu, Xiaojie Lin . Periodic solutions of Cohen-Grossberg-type Bi-directional associative memory neural networks with neutral delays and impulses. AIMS Mathematics, 2021, 6(3): 2539-2558. doi: 10.3934/math.2021154 |
[8] | Huahai Qiu, Li Wan, Zhigang Zhou, Qunjiao Zhang, Qinghua Zhou . Global exponential periodicity of nonlinear neural networks with multiple time-varying delays. AIMS Mathematics, 2023, 8(5): 12472-12485. doi: 10.3934/math.2023626 |
[9] | Qinghua Zhou, Li Wan, Hongbo Fu, Qunjiao Zhang . Exponential stability of stochastic Hopfield neural network with mixed multiple delays. AIMS Mathematics, 2021, 6(4): 4142-4155. doi: 10.3934/math.2021245 |
[10] | Li Wan, Qinghua Zhou, Hongbo Fu, Qunjiao Zhang . Exponential stability of Hopfield neural networks of neutral type with multiple time-varying delays. AIMS Mathematics, 2021, 6(8): 8030-8043. doi: 10.3934/math.2021466 |
Maybe because Cohen-Grossberg neural networks with multiple time-varying delays and distributed delays cannot be converted into the vector-matrix forms, the stability results of such networks are relatively few and the stability conditions in the linear matrix inequality forms have not been established. So this paper investigates the exponential stability of the networks and gives the sufficient condition in the linear matrix inequality forms. Two examples are provided to demonstrate the effectiveness of the theoretical results.
Cohen-Grossberg neural networks, proposed in 1983, have been applied in parallel memory and optimization [1,2]. These applications depend on the stability of equilibrium points of Cohen-Grossberg neural networks. In addition, from the perspective of the model structure, the model of Cohen-Grossberg neural networks includes some famous neural networks such as cellular neural networks and Hopfield neural networks as its special cases. So it is important to investigate the stability of Cohen-Grossberg neural networks.
In implementation of neural networks, time delays are unavoidable because of various reasons such as the finite switching speed of amplifiers. Usually, time-varying delays in models of delayed feedback systems serve as a good approximation in many circuits having a small number of cells. Moreover, neural networks usually have a spatial extent due to the presence of a multitude of parallel pathways with a variety of axon sizes and lengths, and hence there is a distribution of propagation delays over a period of time. So time-varying delays and distributed delays should be incorporated in the models of neural networks. In addition, it is worth noting that a time delay in the response of a neuron can influence the stability of a network and deteriorate the dynamical performance creating oscillatory and unstable characteristics [3]. Therefore, the stability and its related dynamic analysis have received much attention for various types of delayed neural networks, for example, see [4,5,6,7,8,9,10,11,12,13,14,15,16] and references therein.
As is well known, the stability condition in the linear matrix inequality forms contains some non-system parameters to be determined and the stability conditions derived by matrix theory, the method of variation of parameters and differential inequality technique completely depend on the system parameters. So the stability condition in the linear matrix inequality forms is usually less conservative. However, as far as our knowledge, perhaps because Cohen-Grossberg neural networks with multiple delays cannot be transformed into the vector-matrix form, there is relatively little research on the exponential stability of such neural networks and the stability condition in the linear matrix inequality forms has not been obtained. Therefore, this paper aims at deriving the sufficient condition in the linear matrix inequality forms for the exponential stability of Cohen-Grossberg neural networks with multiple discrete time-varying delays and multiple distributed time-varying delays.
The innovations of this paper are listed in the following.
1) By using Lyapunov-Krasovskii functional and linear matrix inequality simultaneously, the sufficient conditions in the linear matrix inequality forms are derived to ensure the exponential stability of Cohen-Grossberg neural networks with multiple discrete time-varying delays and multiple distributed time-varying delays.
2) It is confirmed that Lyapunov-Krasovskii functional and linear matrix inequality can be used simultaneously to investigate the neural networks with multiple delays that cannot be transformed into the vector-matrix form.
3) Two examples are provided to show that the sufficient condition established here is better than the existing results derived by matrix theory [17], the method of variation of parameters and differential inequality technique [18].
In this paper, we consider the following Cohen-Grossberg neural networks with multiple time-varying delays and distributed delays:
˙xi(t)=di(xi(t)){−ci(xi(t))+n∑j=1aijfj(xj(t))+n∑j=1bijgj(xj(t−τij(t)))+n∑j=1∫tt−ρij(t)dijhj(xj(s))ds},i=1,⋯,n, | (2.1) |
in which aij,bij and dij are some constants, other functions satisfy the following assumption:
(A1): For i,j=1,⋯,n,ci(0)=fi(0)=gi(0)=hi(0)=σij(0,0)=0 and there exist some constants c_i,d_i,¯di,f−i,f+i,g−i,g+i,h−i,h+i,τ,ρ and ˉτ such that for t≥0 and every x,y∈R,
0≤τij(t)≤τ,0≤ρij(t)≤ρ,˙τij(t)≤ˉτ<1,0<di_≤di(x)≤¯di, |
0<c_i≤ci(x)−ci(y)x−y=|ci(x)−ci(y)||x−y|,h−i≤hi(x)−hi(y)x−y≤h+i,x≠y, |
f−i≤fi(x)−fi(y)x−y≤f+i,g−i≤gi(x)−gi(y)x−y≤g+i,x≠y. |
The initial conditions associated with (2.1) are of the form: xi(s)=ξi(s),s∈[−max{τ,ρ},0], and ξ={(ξ1(s),⋯,ξ1(s))T:−max{τ,ρ}≤s≤0} is C([−max{τ,ρ},0];Rn)-valued function satisfying
||ξ||2=sup−max{τ,ρ}≤t≤0‖ξ(t)‖2<∞, |
in which C([−max{τ,ρ},0];Rn) denotes the space of all continuous Rn-valued functions defined on [−max{τ,ρ},0], ‖⋅‖ denotes the Euclidean norm. It is easy to see that by changing the functions of system (2.1), system (2.1) can convert into the following neural networks studied in [17,18]:
˙xi(t)=−cixi(t)+n∑j=1aijfj(xj(t))+n∑j=1bijgj(xj(t−τj(t))), | (2.2) |
˙xi(t)=−cixi(t)+n∑j=1aijfj(xj(t))+n∑j=1bijfj(xj(t−τij(t))). | (2.3) |
Theorem 3.1. The origin of system (2.1) is globally exponentially stable provided that there exist some positive constants p1,⋯,pn,ui1,⋯,uin(i=1,2,3) such that
Δ=(Δ1U1F1U2G1U3H1∗−2U1+A200%∗∗−2U2+11−ˉτB20∗∗∗−2U3+ρ2D2)<0, | (3.1) |
in which Δ<0 denotes that matrix Δ is symmetric negative definite, ∗ means the symmetric terms of the symmetric matrix Δ,
Δ1=−2Pd_C+P2ˉdA1+P2ˉdB1+P2ˉdD1−2U1F2−2U2G2−2U3H2, |
Ui=diag{ui1,⋯,uin}(i=1,2,3),C=diag{c_1,⋯,c_n}, |
ˉd=diag{ˉd1,⋯,ˉdn},d_=diag{d_1,⋯,d_n},P=diag{p1,⋯,pn}, |
A1=diag{n∑j=1|a1j|,⋯,n∑j=1|anj|},A2=diag{n∑j=1ˉdj|aj1|,⋯,n∑j=1ˉdj|ajn|}, |
B1=diag{n∑j=1|b1j|,⋯,n∑j=1|bnj|},B2=diag{n∑j=1ˉdj|bj1|,⋯,n∑j=1ˉdj|bjn|}, |
D1=diag{n∑j=1|d1j|,⋯,n∑j=1|dnj|},D2=diag{n∑j=1ˉdj|dj1|,⋯,n∑j=1ˉdj|djn|}, |
F1=diag{|f−1+f+1|,⋯,|f−n+f+n|},F2=diag{f−1f+1,⋯,f−nf+n}, |
G1=diag{|g−1+g+1|,⋯,|g−n+g+n|},G2=diag{g−1g+1,⋯,g−ng+n}, |
H1=diag{|h−1+h+1|,⋯,|h−n+h+n|},H2=diag{h−1h+1,⋯,h−nh+n}. |
Proof. It follows from (3.1) that there exists a positive constant λ such that ˜Δ<0, in which
˜Δ=(˜Δ1U1F1U2G1U3H1∗−2U1+A200%∗∗−2U2+eλτ1−ˉτB20∗∗∗−2U3+ρ2eλρD2), |
˜Δ1=λP−2Pd_C+P2ˉdA1+PˉdB1+PˉdD1−2U1F2−2U2G2−2U3H2. |
Lyapunov-Krasovskii functional V(t) is defined as follows:
V(t)=V1(t)+V2(t)+V3(t), | (3.2) |
in which
V1(t)=eλtn∑i=1pix2i(t),V2(t)=n∑i=1n∑j=1∫tt−τij(t)eλ(s+τ)1−ˉτˉdi|bij|g2j(xj(s))ds, |
V3(t)=∫0−ρ∫tt+sn∑i=1n∑j=1ˉdi|dij|ρeλ(θ+ρ)h2j(xj(θ))dθds. |
Along the trajectory of system (2.1), we obtain
˙V1(t)=λeλtn∑i=1pix2i(t)+eλtn∑i=1{−2pixi(t)di(xi(t))ci(xi(t))+n∑j=12pixi(t)di(xi(t))aijfj(xj(t))+n∑j=12pixi(t)di(xi(t))bijgj(xj(t−τij(t)))+n∑j=12pixi(t)di(xi(t))dij∫tt−ρij(t)hj(xj(s))ds}≤eλtn∑i=1{λpix2i(t)−2pid_ic_ix2i(t)+n∑j=12piˉdi|aij||xi(t)fj(xj(t))|+n∑j=12piˉdi|bij||xi(t)gj(xj(t−τij(t)))|+n∑j=12piˉdi|dij||xi(t)∫tt−ρij(t)hj(xj(s))ds|}≤eλtn∑i=1{λpix2i(t)−2pid_ic_ix2i(t)+n∑j=1ˉdi|aij|[p2ix2i(t)+f2j(xj(t))]+n∑j=1ˉdi|bij|[p2ix2i(t)+g2j(xj(t−τij(t)))]+n∑j=1ˉdi|dij|[p2ix2i(t)+(∫tt−ρij(t)hj(xj(s))ds)2]}≤eλtn∑i=1{λpix2i(t)−2pid_ic_ix2i(t)+n∑j=1ˉdi|aij|[p2ix2i(t)+f2j(xj(t))]+n∑j=1ˉdi|bij|[p2ix2i(t)+g2j(xj(t−τij(t)))]+n∑j=1ˉdi|dij|[p2ix2i(t)+ρ∫tt−ρh2j(xj(s))ds]}, | (3.3) |
˙V2(t)=n∑i=1n∑j=1{eλ(t+τ)1−ˉτˉdi|bij|g2j(xj(t))−(1−˙τij(t))eλ(t−τij(t)+τ)1−ˉτˉdi|bij|g2j(xj(t−τij(t)))}≤n∑i=1n∑j=1{eλ(t+τ)1−ˉτˉdi|bij|g2j(xj(t))−eλtˉdi|bij|g2j(xj(t−τij(t)))}£ | (3.4) |
˙V3(t)=n∑i=1n∑j=1ˉdi|dij|ρ{ρeλ(t+ρ)h2j(xj(t))−∫0−ρeλ(t+s+ρ)h2j(xj(t+s))ds}=n∑i=1n∑j=1ˉdi|dij|ρ{ρeλ(t+ρ)h2j(xj(t))−∫tt−ρeλ(s+ρ)h2j(xj(s))ds}≤n∑i=1n∑j=1ˉdi|dij|ρ{ρeλ(t+ρ)h2j(xj(t))−eλt∫tt−ρh2j(xj(s))ds}. | (3.5) |
At the same time, we can also obtain
0≤−2n∑i=1u1i[fi(xi(t))−f+ixi(t)][fi(xi(t))−f−ixi(t)]=−2n∑i=1u1i[f2i(xi(t))−(f+i+f−i)xi(t)fi(xi(t))+f+if−ix2i(t)]≤−2n∑i=1u1if2i(xi(t))+2n∑i=1u1i|f+i+f−i||xi(t)||fi(xi(t))|−2n∑i=1u1if+if−ix2i(t)=−2˜fT(x(t))U1˜f(x(t))+2˜fT(x(t))U1F1˜x(t)−2˜xT(t)U1F2˜x(t), | (3.6) |
0≤−2n∑i=1u2i[gi(xi(t))−g+ixi(t)][gi(xi(t))−g−ixi(t)]≤−2˜gT(x(t))U2˜g(x(t))+2˜gT(x(t))U2G1˜x(t)−2˜xT(t)U2G2˜x(t) | (3.7) |
and
0≤−2n∑i=1u3i[hi(xi(t))−h+ixi(t)][hi(xi(t))−h−ixi(t)]≤−2˜hT(x(t))U3˜h(x(t))+2˜hT(x(t))U3H1˜x(t)−2˜xT(t)U3H2˜x(t), | (3.8) |
in which
˜x(t)=(|x1(t)|,⋯,|xn(t)|)T,˜f(x(t))=(|f1(x1(t))|,⋯,|fn(xn(t))|)T, |
˜g(x(t))=(|g1(x1(t))|,⋯,|gn(xn(t))|)T,˜h(x(t))=(|h1(x1(t))|,⋯,|hn(xn(t))|)T. |
So, from (3.3)–(3.8), we have
˙V(t)≤eλt{˜xT(t)(λP−2Pd_C+P2ˉdA1+P2ˉdB1+P2ˉdD1−2U1F2−2U2G2−2U3H2)˜x(t)+˜fT(x(t))(−2U1+A2)˜f(x(t))+˜gT(x(t))(−2U2+eλτ1−ˉτB2)˜g(x(t)+˜hT(x(t))(−2U3+ρ2eλρD2)˜h(x(t))+2˜xT(t)U1F1˜f(x(t))+2˜xT(t)U2G1˜g(x(t))+2˜xT(t)U3H1˜h(x(t))}=eλtyT(t)˜Δy(t)<0, | (3.9) |
in which y(t)=(˜xT(t),˜fT(x(t)),˜gT(x(t)),˜hT(x(t)))T.
Integrating from 0 to t for (3.9) and using (3.2), we obtain
eλtmin1≤i≤n{pi}‖x(t)‖2≤V(t)≤V(0)≤{max1≤i≤n{pi}‖x(0)‖2+n∑i=1n∑j=1∫0−τeλ(s+τ)ˉdi|bij|ˉg2j1−ˉτx2j(s)ds+∫0−ρ∫0sn∑i=1n∑j=1ˉdi|dij|ρeλ(θ+ρ)ˉh2jx2j(θ)dθds}≤{max1≤i≤n{pi}+eλττ1−ˉτmax1≤i≤n{n∑j=1ˉdj|bji|ˉg2i}+eλρρ3max1≤i≤n{n∑j=1ˉdj|dji|ˉh2i}}‖ξ‖2, |
which implies the origin of system (2.1) is exponentially stable, in which ˉgj=max{|g−j|,|g+j|}, ˉhj=max{|h−j|,|h+j|},j=1,⋯,n.
For the systems (2.2) and (2.3), we obtain the following results from Theorem 3.1.
Corollary 3.1. The origin of system (2.2) is globally exponentially stable provided that there exist some positive constants p1,⋯,pn,ui1,⋯,uin(i=1,2) such that
Δ=(Δ1U1F1U2G1∗−2U1+A20%∗∗−2U2+11−ˉτB2)<0, | (3.10) |
in which
Δ1=−2PC+P2A1+P2B1−2U1F2−2U2G2,C=diag{c1,⋯,cn}, |
A2=diag{n∑j=1|aj1|,⋯,n∑j=1|ajn|},B2=diag{n∑j=1|bj1|,⋯,n∑j=1|bjn|}, |
the other symbols are the same as Theorem 3.1.
Corollary 3.2. The origin of system (2.3) is globally exponentially stable provided that there exist some positive constants p1,⋯,pn,u11,⋯,u1n such that
Δ=(Δ1U1F1∗−2U1+A2+11−ˉτB2%)<0, | (3.11) |
in which
Δ1=−2PC+P2A1+P2B1−2U1F2,C=diag{c1,⋯,cn}, |
A2=diag{n∑j=1|aj1|,⋯,n∑j=1|ajn|},B2=diag{n∑j=1|bj1|,⋯,n∑j=1|bjn|}, |
the other symbols are the same as Theorem 3.1.
Remark 3.1. It is obvious that Corollaries 3.1 and 3.2 can be applicable to the networks (2.2) and (2.3) studied in [17,18], since these networks are some special cases of system (2.1). Therefore, Corollaries 3.1 and 3.2 can be seen as new stability criteria for the networks (2.2) and (2.3).
Remark 3.2. Based on the method of variation of parameters and differential inequality technique, Theorem 2 in [18] shows that the origin of system (2.2) is globally exponentially stable provided that
α=ξ||A||2+η||B||2c0<1, |
in which ξ=max1≤i≤n{supxi≠0fi(xi)xi},η=max1≤i≤n{supxi≠0gi(xi)xi},c0=min1≤i≤n{ci},||A||2 denotes the square root of the largest eigenvalue of ATA. This stability condition completely depends on the parameters of system (2.2) and the stability condition of Corollary 3.1 contains some non-system parameters p1,⋯,pn,ui1,⋯,uin(i=1,2) to be determined. We demonstrate that Corollary 3.1 is applicable to system (2.2) in Example 1 and Theorem 2 in [18] is not. So the stability condition of Corollary 3.1 is better.
Remark 3.3. By using matrix theory and inequality analysis, Theorem 2.4 in [17] shows that zero solution of system (2.3) is globally exponentially stable provided that ρ(K)<1, in which ρ(K) denotes spectral radius of matrix K=(kij)n×n,kij=c−1i(|aij|+|bij|)αj,αj corresponds to max{|f−j|,|f+j|} in this paper. Similarly, this stability condition also depends on the parameters of system (2.3) and the stability condition of Corollary 3.2 contains some non-system parameters p1,⋯,pn,u11,⋯,u1n to be determined. We demonstrate Corollary 3.2 is applicable to system (2.3) in Example 2 and Theorem 2.4 in [17] is not. So the stability condition of Corollary 3.2 is better.
Example 4.1. Consider system (2.2) with the following parameters and functions:
A=(aij)4×4=(1−1−11−11−1−111−11−1−1−1−1),B=(bij)4×4=(−11−11−1−11−11−1−1−1−1−1−11), |
C=diag{6,6,5,5},fi(xi)=tanh(xi),gi(xi)=0.8tanh(xi),τi(t)=0.2sint+0.2,i=1,2,3,4.
We calculate that A1=A2=B1=B2=4I,F1=I,G1=0.8I,F2=G2=0,ˉτ=0.2, in which I denotes identity matrix. By using MATLAB LMI Control Toolbox, we know when
P=diag{258.2100,258.2100,450.2626,450.2626},U1=diag{324.4595,324.4595,317.0773,317.0773},U2=diag{337.2718,337.2718,332.0326,332.0326}, |
the condition of Corollary 3.1 is satisfied and so Corollary 3.1 is applicable to system (2.2). Figure 1 shows the solution trajectories of system (2.2) with the initial value (0.3;0.2;−0.2;−0.3)T tend to 0.
On the other hand, we calculate ξ=1,η=0.8,||A||2=√8,||B||2=√7.4641,c0=min1≤i≤4{ci}=5 and α=5.0145>1 defined in Remark 3.2. Therefore, Theorem 2 in [18] is not applicable to system (2.2) in this example.
Example 4.2. Consider system (2.3) with C=diag{5,5,5,5},fi(xi)=0.5tanh(xi),τii(t)=0.2sint+0.2,τij(t)=0.2cost+0.2,i≠j,i,j=1,2,3,4, the matrices A and B are the same as Example 4.1.
We calculate that A1=A2=B1=B2=4I,F1=0.5I,F2=0,ˉτ=0.2. By using MATLAB LMI Control Toolbox, we know when P=76.1324I,U1=72.4840I, the condition of Corollary 3.2 is satisfied. Figure 2 shows the solution trajectories of system (2.3) with the initial value (0.3;0.2;−0.2;−0.3)T tend to 0.
On the other hand, we calculate αi=0.5,kij=0.2(i=1,2,3,4) and ρ(K)=1 defined in Remark 3.3. Therefore, Theorem 2.4 in [17] is not applicable to system (2.3) in this example.
This paper has investigated the exponential stability of Cohen-Grossberg neural networks with multiple discrete time-varying delays and multiple distributed time-varying delays. Maybe because such networks cannot be converted into the vector-matrix forms, the stability results of the networks are relatively few and the stability conditions in the linear matrix inequality forms have not been established. By using Lyapunov-Krasovskii functional and linear matrix inequality simultaneously, the sufficient conditions in the linear matrix inequality forms of ensuring the exponential stability are derived. It is confirmed that Lyapunov-Krasovskii functional and linear matrix inequality can be used simultaneously to investigate the neural networks with multiple delays that cannot be transformed into the vector-matrix form.
The authors declare they have not used Artificial Intelligence (AI) tools in the creation of this article.
The authors would like to thank the editor and the reviewers for their detailed comments and valuable suggestions. This work was supported by the National Natural Science Foundation of China (No: 12271416, 11971367, 11826209, 11501499, 61573011 and 11271295), the Natural Science Foundation of Guangdong Province (2018A030313536).
The authors declare no conflicts of interest.
[1] |
M. A. Cohen, S. Grossberg, Absolute stability of global pattern formation and parallel memory storage by competitive neural networks, IEEE Trans. Syst. Man. Cy. B, 13 (1983), 815–826. https://doi.org/10.1109/TSMC.1983.6313075 doi: 10.1109/TSMC.1983.6313075
![]() |
[2] |
Y. Takahashi, Solving optimization problems with variable-constraint by an extended Cohen-Grossberg model, Theor. Comput. Sci., 158 (1996), 279–341. https://doi.org/10.1016/0304-3975(95)00085-2 doi: 10.1016/0304-3975(95)00085-2
![]() |
[3] | J. H. Wu, Introduction to Neural Dynamics and Signal Transmission Delay, Berlin: Walter de Gruyter, 2001. |
[4] |
S. Gao, R. Shen, T. R. Chen, Periodic solutions for discrete-time Cohen-Grossberg neural networks with delays, Phys. Lett. A, 383 (2019), 414–420. https://doi.org/10.1016/j.physleta.2018.11.016 doi: 10.1016/j.physleta.2018.11.016
![]() |
[5] |
B. Sun, Y. T. Cao, Z. Y. Guo, Z. Yan, S. P. Wen, Synchronization of discrete-time recurrent neural networks with time-varying delays via quantized sliding mode control, Appl. Math. Comput., 375 (2020), 125093. https://doi.org/10.1016/j.amc.2020.125093 doi: 10.1016/j.amc.2020.125093
![]() |
[6] |
Y. X. Wang, Y. T. Cao, Z. Y. Guo, T. W. Huang, S. P. Wen, Event-based sliding-mode synchronization of delayed memristive neural networks via continuous/periodic sampling algorithm, Appl. Math. Comput., 383 (2020), 125379. https://doi.org/10.1016/j.amc.2020.125379 doi: 10.1016/j.amc.2020.125379
![]() |
[7] |
W. Q. Shen, X. Zhang, Y. T. Wang, Stability analysis of high order neural networks with proportional delays, Neurocomputing, 372 (2020), 33–39. https://doi.org/10.1016/j.neucom.2019.09.019 doi: 10.1016/j.neucom.2019.09.019
![]() |
[8] |
O. Faydasicok, A new Lyapunov functional for stability analysis of neutral-type Hopfield neural networks with multiple delays, Neural Networks, 129 (2020), 288–297. https://doi.org/10.1016/j.neunet.2020.06.013 doi: 10.1016/j.neunet.2020.06.013
![]() |
[9] |
L. Wan, Q. H. Zhou, Stability analysis of neutral-type Cohen-Grossberg neural networks with multiple time-varying delays, IEEE Access, 8 (2020), 27618–27623. https://doi.org/10.1109/ACCESS.2020.2971839 doi: 10.1109/ACCESS.2020.2971839
![]() |
[10] |
H. M. Wang, G. L. Wei, S. P. Wen, T. W. Huang, Generalized norm for existence, uniqueness and stability of Hopfield neural networks with discrete and distributed delays, Neural Networks, 128 (2020), 288–293. https://doi.org/10.1016/j.neunet.2020.05.014 doi: 10.1016/j.neunet.2020.05.014
![]() |
[11] |
Q. K. Song, Y. X. Chen, Z. J. Zhao, Y. R. Liu, F. E. Alsaadi, Robust stability of fractional-order quaternion-valued neural networks with neutral delays and parameter uncertainties, Neurocomputing, 420 (2021), 70–81. https://doi.org/10.1016/j.neucom.2020.08.059 doi: 10.1016/j.neucom.2020.08.059
![]() |
[12] |
L. Wan, Q. H. Zhou, H. B. Fu, Q. J. Zhang, Exponential stability of Hopfield neural networks of neutral type with multiple time-varying delays, AIMS Mathematics, 6 (2021), 8030–8043. https://doi.org/10.3934/math.2021466 doi: 10.3934/math.2021466
![]() |
[13] |
L. Wan, Q. H. Zhou, Exponential stability of neutral-type Cohen-Grossberg neural networks with multiple time-varying delays, IEEE Access, 9 (2021), 48914–48922. https://doi.org/10.1109/ACCESS.2021.3068191 doi: 10.1109/ACCESS.2021.3068191
![]() |
[14] |
Y. K. Deng, C. X. Huang, J. D. Cao, New results on dynamics of neutral type HCNNs with proportional delays, Math. Comput. Simul., 187 (2021), 51–59. https://doi.org/10.1016/j.matcom.2021.02.001 doi: 10.1016/j.matcom.2021.02.001
![]() |
[15] |
Z. J. Zhang, X. Zhang, T. T. Yu, Global exponential stability of neutral-type Cohen-Grossberg neural networks with multiple time-varying neutral and discrete delays, Neurocomputing, 490 (2022), 124–131. https://doi.org/10.1016/j.neucom.2022.03.068 doi: 10.1016/j.neucom.2022.03.068
![]() |
[16] |
R. V. Aravind, P. Balasubramaniam, Stability criteria for memristor-based delayed fractional-order Cohen-Grossberg neural networks with uncertainties, J. Comput. Appl. Math., 420 (2023), 114764. https://doi.org/10.1016/j.cam.2022.114764 doi: 10.1016/j.cam.2022.114764
![]() |
[17] |
L. H. Huang, C. X. Huang, B. W. Liu, Dynamics of a class of cellular neural networks with time-varying delays, Phys. Lett. A, 345 (2005), 330–344. https://doi.org/10.1016/j.physleta.2005.07.039 doi: 10.1016/j.physleta.2005.07.039
![]() |
[18] |
H. Y. Zhao, Global exponential stability and periodicity of cellular neural networks with variable delays, Phys. Lett. A, 336 (2005), 331–341. https://doi.org/10.1016/j.physleta.2004.12.001 doi: 10.1016/j.physleta.2004.12.001
![]() |
1. | A. Karnan, G. Soundararajan, G. Nagamani, Ardak Kashkynbayev, Design of an event-triggered extended dissipative state estimator for neural networks with multiple time-varying delays, 2024, 1951-6355, 10.1140/epjs/s11734-024-01240-0 |