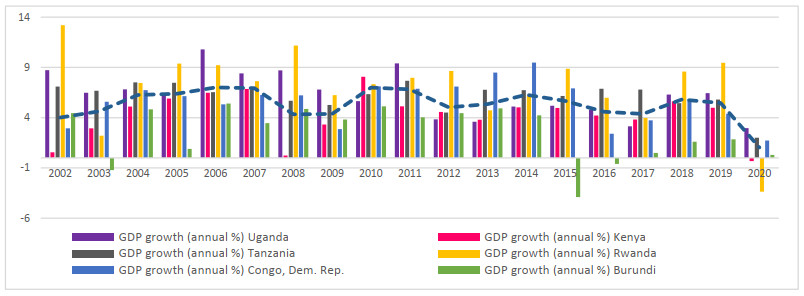
We investigate the following nonlinear system
{−(a+b∫Ω|∇u|2dx)Δu+ϕu=λu+μ|u|2u, x∈Ω,−Δϕ=u2, x∈Ω,u=ϕ=0, x∈∂Ω,
with a,b>0, λ,μ∈R, and Ω⊂R3 is bounded with smooth boundary. Let λ1>0 be the first eigenvalue of (−Δu,H10(Ω)). We get that for certain ˜μ>0 there exists at least one least energy sign-changing solution for the above system if λ<aλ1 and μ>˜μ. In addition, we remark that the nonlinearity λu+μ|u|2u does not satisfy the growth conditions.
Citation: Xia Su, Wen Guan, Xia Li. Least energy sign-changing solutions for Kirchhoff-Schrödinger-Poisson system on bounded domains[J]. Electronic Research Archive, 2023, 31(5): 2959-2973. doi: 10.3934/era.2023149
[1] | Mario Ohlberger, Ben Schweizer, Maik Urban, Barbara Verfürth . Mathematical analysis of transmission properties of electromagnetic meta-materials. Networks and Heterogeneous Media, 2020, 15(1): 29-56. doi: 10.3934/nhm.2020002 |
[2] | Leonid Berlyand, V. V. Zhikov . Preface. Networks and Heterogeneous Media, 2008, 3(3): i-ii. doi: 10.3934/nhm.2008.3.3i |
[3] | Tasnim Fatima, Ekeoma Ijioma, Toshiyuki Ogawa, Adrian Muntean . Homogenization and dimension reduction of filtration combustion in heterogeneous thin layers. Networks and Heterogeneous Media, 2014, 9(4): 709-737. doi: 10.3934/nhm.2014.9.709 |
[4] | Xavier Blanc, Claude Le Bris, Frédéric Legoll, Tony Lelièvre . Beyond multiscale and multiphysics: Multimaths for model coupling. Networks and Heterogeneous Media, 2010, 5(3): 423-460. doi: 10.3934/nhm.2010.5.423 |
[5] | Adrian Muntean, Toyohiko Aiki . Preface to ``The Mathematics of Concrete". Networks and Heterogeneous Media, 2014, 9(4): i-ii. doi: 10.3934/nhm.2014.9.4i |
[6] | Guy Bouchitté, Ben Schweizer . Plasmonic waves allow perfect transmission through sub-wavelength metallic gratings. Networks and Heterogeneous Media, 2013, 8(4): 857-878. doi: 10.3934/nhm.2013.8.857 |
[7] | Antoine Gloria Cermics . A direct approach to numerical homogenization in finite elasticity. Networks and Heterogeneous Media, 2006, 1(1): 109-141. doi: 10.3934/nhm.2006.1.109 |
[8] | Hirofumi Notsu, Masato Kimura . Symmetry and positive definiteness of the tensor-valued spring constant derived from P1-FEM for the equations of linear elasticity. Networks and Heterogeneous Media, 2014, 9(4): 617-634. doi: 10.3934/nhm.2014.9.617 |
[9] | Patrick Henning, Mario Ohlberger . The heterogeneous multiscale finite element method for advection-diffusion problems with rapidly oscillating coefficients and large expected drift. Networks and Heterogeneous Media, 2010, 5(4): 711-744. doi: 10.3934/nhm.2010.5.711 |
[10] | Renata Bunoiu, Claudia Timofte . Homogenization of a thermal problem with flux jump. Networks and Heterogeneous Media, 2016, 11(4): 545-562. doi: 10.3934/nhm.2016009 |
We investigate the following nonlinear system
{−(a+b∫Ω|∇u|2dx)Δu+ϕu=λu+μ|u|2u, x∈Ω,−Δϕ=u2, x∈Ω,u=ϕ=0, x∈∂Ω,
with a,b>0, λ,μ∈R, and Ω⊂R3 is bounded with smooth boundary. Let λ1>0 be the first eigenvalue of (−Δu,H10(Ω)). We get that for certain ˜μ>0 there exists at least one least energy sign-changing solution for the above system if λ<aλ1 and μ>˜μ. In addition, we remark that the nonlinearity λu+μ|u|2u does not satisfy the growth conditions.
The growing trend towards globalisation has resulted in more interconnected financial markets due to the rapid spread of technological advances and innovations which have also boosted financial market development particularly in developing countries. Of grave concern however is the increase in financial and currency crises in such economies as a consequence of financial globalization (Prasad et al., 2003). Moreover evidence suggests that regional integration may have amplified the effects of global crises in sub-Saharan Africa (Price & Elu, 2014). Furthermore, external shocks have been identified as one of the major reasons for macroeconomic instability and poor economic performance of African countries (Barrot et al., 2018; Dieppe et al., 2020; Eberhardt & Presbitero, 2018; International Monetary Fund, 2003; Raddatz, 2007; Varangis et al., 2004). The recent global COVID-19 pandemic (COVID-19) not only had major adverse effects on African economies and financial markets but many developing countries continue to struggle to recover from its devastating effects (Anyanwu & Salami, 2021; Gill, 2022; Katusiime, 2021).
However, despite the proliferation of models of crisis prediction arising from the increased frequency of global crisis such as the ongoing COVID-19 pandemic, few studies investigate the spillover and connectedness dynamics of African financial markets. Among the studies that examine asset co-movement within the context of African financial markets is Assifuah-Nunoo et al. (2022) who re-examined the oil-stock co-movement in the context of oil-exporting countries in Africa, Agyei et al. (2022) who study co-movement between exchange rate returns and stock returns in selected African markets amid COVID-19 in a time and frequency domain and Agyei (2022) who investigate the heterogeneous and asymmetric co-movements between stock market returns from Ghana and Jamaica. Moreover, the intensification of market integration efforts across African countries and the adverse effects of the COVID-19 pandemic suggests an urgency in understanding the connectedness dynamics of these financial markets.
Given existing evidence shows return and volatility connectedness varies over time and frequency domains, analysing connectedness exclusively in the time domain paradigm may obscure valuable information if investor heterogeneity in terms of expectation and risk preference is not considered (Mensi et al., 2021; Owusu Junior et al., 2022). This study thus contributes to the extant literature by examining financial market connectedness and spillovers in developing countries during the COVID-19 pandemic using the time-domain approach of Diebold & Yilmaz (2012) and the frequency-domain approach of Baruník & Křehlík (2018) to measure return and volatility spillovers. Examining return and volatility spillovers and connectedness in both time and frequency domain paradigms offers valuable insight on the connectedness of financial markets and assets across time as well as diverse investment horizons (i.e. short-, medium-, and long-term). Specifically, the study examines financial market time and frequency connectedness within the context of Uganda, Kenya and Tanzania, typical developing countries that are original members of the East African community (EAC) a regional integration block which were hard hit by the COVID-19 pandemic. Overall, static time-domain results reveal a relatively high degree of return and volatility connectedness with the total return and volatility spillover indices for the full sample of 38.5 percent and 44.3 percent respectively. Moreover this high degree of return and volatility connectedness is also reflected in the results of static return and volatility frequency domain connectedness, especially at the longer term frequency bands, an indication that return and volatility shocks are persistent.
The remainder of this paper is organized as follows; Section two provides an overview of the EAC, Section three presents an overview of the literature, Section four the methodology employed while Section five presents the empirical results and Section six the main conclusions of the study.
The EAC is a regional organisation comprising 7 partner states with its headquarters in Arusha, Tanzania. The Treaty for reestablishment of the EAC was signed on 30 November 1999 and entered into force on 7 July 2000 following its ratification by the original three Partner States Kenya, Uganda, and Tanzania. The EAC countries have generally relied on prudent macroeconomic management to address the resultant macroeconomic instability from adverse global and domestic shocks even while they continued to pursue policies that foster financial integration. The pursuit of financial integration by EAC members is aimed at exploiting economies of scale by maximizing financial sector resource mobilisation and allocation in small and fragmented domestic markets across the region to support sustainable financial sector growth and economic development (Kasekende & Ng'eno, 2000). However, increased financial integration and globalisation are recognized potential sources of macroeconomic instability and EAC economies are concerned that this macroeconomic volatility increases uncertainty which distorts efficient resource allocation and renders macroeconomic policy ineffective. Thus an understanding of the dynamics of financial return and volatility spillovers within the context of developing countries as characterises the EAC member states is especially important given recent evidence that shows developing countries remain predominantly a destination, rather than a source, of global financial spillovers as a result of their increasing integration into international financial markets (Agénor & Pereira da Silva, 2018).
In addition, such evidence in the face of increasing intensity and frequency of shocks and financial instability would be useful to policy makers in the management of macroeconomic instability. Further the study of cross market spillovers within the EAC is also critical because domestic financial market imperfections may result in varying magnitudes in spillovers across EAC economies. Indeed evidence shows EAC countries remain susceptible to asymmetric shocks and have country-specific shocks which have been prevalent in the last two decades despite similarities in their economic structures (Drummond et al., 2015). The current global COVID-19 pandemic also significantly adversely affected the EAC region with real GDP growth approximately falling from 5.5 percent in 2019 to 1.0% in 2020 (See Figure 1). This paper thus examines financial market return and volatility spillovers in the EAC financial markets during the COVID-19 pandemic using time-frequency connectedness measures of Diebold & Yilmaz (2012) and Baruník & Křehlík (2018).
Although the investigation of the interconnectedness of financial markets and its implications for systemic risk and macroeconomic stability is not new, the evidence is generally mixed partly because financial connectedness may have adverse effects where it creates additional channels through which shocks quickly propagate and yet it may also support macroeconomic stability where it allows for risk sharing among other economies of scale. In addition, return and volatility spillovers may behave differently before, during and after crisis episodes, increasing in intensity before, during and after economic and financial crises as highlighted in the seminal spillover and financial connectedness study by Diebold & Yilmaz (2012). Diebold & Yilmaz (2012) propose important measures of the total and directional volatility spillovers using the generalized vector autoregressive methodology to characterize daily volatility spillover among four key U.S. asset classes, including stocks, bonds, commodities, and foreign exchange, from January 1999 to January 2010. Their study shows insignificant volatility spillovers before the global financial crisis and considerable volatility spillovers from the stock market to the bonds, commodities, and the foreign exchange markets during financial crisis. The study also highlights the role of the U.S. stock market in the propagation financial shocks by showing that the direction of volatility shock transmission was mainly from the stock market to other markets during the global financial crisis when volatility connectedness increased beyond its pre-crisis level. Another seminal study is by Baruník & Křehlík (2018), who propose a new framework for measuring connectedness among financial variables that arise due to heterogeneous frequency responses to shocks and document rich time-frequency dynamics of volatility connectedness in U.S. financial institutions. Specifically, the study finds that economically, periods in which connectedness is created at high frequencies are periods when stock markets seem to process information rapidly and calmly, and a shock to one asset in the system will have an impact mainly in the short term while connectedness is created at lower frequencies, suggests that shocks are persistent and are being transmitted for longer periods.
The predominant portion of the literature examines international rather than domestic spillovers. In addition, a large portion of this extant literature focuses on advanced, emerging, and global perspectives when investigating spillovers. For instance Bagheri & Ebrahimi (2020) investigate the directional volatility and return network connectedness among stock, commodity, bond, currency, and cryptocurrency markets using Diebold and Yilmaz (2012) over the period February 2006 to August 2018 and find that markets are highly connected, especially during 2008–2009 with Asian stock markets as net receivers of shocks, while European and American stock markets are net transmitters of shocks to other markets. The pairwise connectedness results suggest that among stock markets, DAX-CAC 40, FTSE 100-CAC 40 and S & P 500-S & P_TSX index are more integrated through connectedness than the others while for other markets, WTI crude oil-Brent crude oil, 30-Year bond and 10-Year bond, Dollar Index futures-EUR/USD have notable connections. On the other hand, cryptocurrencies contribute insignificantly to other markets and are highly integrated with each other, but gold and cryptocurrencies seem to be good choices for investors to hedge during a crisis. Minoiu et al. (2015) examine the ability of connectedness in the global network of financial linkages to predict systemic banking crises during the 1978–2010 period and find that increases in a country's own connectedness and decreases in its neighbours' connectedness are associated with a higher probability of banking crises after controlling for macroeconomic fundamentals. The study concludes that financial interconnectedness has early warning potential, especially for the 2007–2010 wave of systemic banking crises.
In the context of advanced and emerging markets, Li et al. (2021) explore the dynamics of the return connectedness across six major commodity assets (crude oil, gold and corn) and financial assets (stock, bond and currency) in China and the US during recent COVID-19 pandemic and find that the total return connectedness of the US commodity and financial assets is stronger than that of the Chinese commodity and financial assets in most cases and total return connectedness both in China and US markets increases rapidly after the outbreak of COVID-19. Interestingly, the study finds that crude oil shifts from a net transmitter to a net receiver of shocks in China after the outbreak of COVID-19 but remains a net transmitter of shocks in the US while stock and currency become net transmitters of shocks in both markets after the outbreak of COVID-19. The study suggests policy makers and investors apply flexible regulatory and portfolio allocation strategies in view of the interchangeable roles of the commodity and financial assets. Similarly Lin & Chen (2021) measure the dynamic connectedness of financial asset returns in major financial markets in China and America and find that major financial markets of China and America are highly connected and that the US stock market is at the core of information spillover, although most financial markets only resonate with domestic financial markets and a few financial markets are relatively independent. Uluceviz & Yilmaz (2021) analyse the connectedness between the real and the financial sectors of the U.S. economy using the weekly ADS index of the Philadelphia Fed (the widely used business conditions indicator) and a derived real activity index (RAI) to represent the real side and find that for during times of financial distress and business cycle turning points, the direction of connectedness runs from the real sector to financial markets the ADS index while connectedness from the real side to financial markets weakens substantially, while the connectedness from financial markets to the real side becomes more pronounced when RAI is included to represent the real side.
Ogbuabor et al. (2020) are one of the few studies that examine the African context, and their study examines the dynamics of the Common Market for Eastern and Southern Africa's (COMESA) output connectedness with the global economy from 1970Q1 to 2016Q4 using the Diebold and Yilmaz (2009) network approach. The study finds that COMESA's output connectedness with the rest of the world is quite sizeable, with a total connectedness index of 73percent, with the USA, EU, Japan, China, Canada, Indonesia, and UK exerting the most dominant output influence on COMESA region. In addition, the effect of non-COMESA African economies in COMESA's real activities is minimal relative to idiosyncratic conditions and contributions from the rest of the global economy. Overall, the study's findings indicate that COMESA economies are considerably open, deeply interconnected, and sensitive to international output shocks and as such policymakers in COMESA ought to consider output shocks originating from mentioned dominant sources.
Although global and regional financial integration in the EAC may increase the potential for the financial transmission of external shocks, empirical evidence on this point is non-existent. Moreover, existing evidence suggests an amplification of financial sector shock transmission during crisis periods (Katusiime, 2019; Mokni & Mansouri, 2017). The study thus investigates dynamic connectedness in developing countries financial markets, specifically the EAC financial markets before and during the COVID-19 epidemic crisis.
The Diebold & Yilmaz (2012) spillover index approach features prominently in extant literature on financial connectedness, having been widely accepted as standard measures of time connectedness not least because of its suitability for evaluating the degree of interdependence and connectedness within and across countries and regions. The Baruník & Křehlík (2018) technique is basically an extension to the popular spillover index approach proposed and developed over time by Diebold & Yilmaz(2009, 2012) that measures the frequency dynamics of connectedness and systemic risk. While a vast majority of research has used multivariate Generalized AutoRegressive Conditional Heteroskedasticity (GARCH) models, cointegration, structural vector autoregressions (VAR) models or AutoRegressive Conditional Heteroskedasticity (ARCH) type models to study spillovers (Palanska, 2020), these models have very limited ability to quantify spillovers due to their lack of spillover dynamics (Baruník et al., 2015)1. The study thus employs the Diebold & Yilmaz (2012) and Baruník & Křehlík (2018) framework to examine return and volatility spillovers and their frequency dynamics in Uganda, Kenya and Tanzania 's money and foreign exchange interbank markets, before and during the COVID-19 pandemic.
1A number of studies explore time-frequency connectedness using wavelet analysis which combines time and frequency domain approaches in the study of the time series in the time-frequency domain (Hung & Vo, 2021; Umar & Gubareva, 2020; Vacha & Barunik, 2012).
The underlying framework for the DY spillover indexes relies on variance decomposition associated with an N-variable vector auto-regression and considers a covariance stationary N-variable vector auto-regression. The forecast error variances of each variable parse into parts attributable to the various system shocks using variance decompositions. The generalized variance decomposition (GVD) framework of Koop et al. (1996) and Pesaran & Shin (1998) is useful when studying directional connectedness as it produces variance decompositions that are invariant to ordering. In a covariance stationary VAR (P) model, the generalized forecast error variance decomposition (GFEVD) of each variable parse into parts attributable to the various system shock for a forecast horizon H can be computed as:
θgij(H)=σ−1jj∑H−1h=0(e'iAh∑ej)2∑H−1h=0(e'iAh∑A'hei) | (1) |
where
Ci←⋅(H)=∑nj=1,j≠iθ gij(H)∑Ni,j=1θ gij(H)×100=∑nj=1,j≠iθ gij(H)N×100 | (2) |
This is the directional connectedness from all other variables to variable i. Similarly, the measure of directional volatility spillovers transmitted by market i to all other markets j is:
C⋅←i(H)=∑nj=1,j≠iθ gji(H)∑Ni,j=1θ gji(H)×100=∑nj=1,j≠iθ gji(H)N×100 | (3) |
This is the directional connectedness from variable i to all other variables. We also quantify the net volatility spillover from market i to all other markets j as:
Ci(H)=C⋅←i(H)−Ci←⋅(H) | (4) |
This is essentially the net directional connectedness from variable i to all other variables which is simply the difference between gross volatility shocks transmitted to and gross volatility shocks received from all other markets. It is also of interest to examine pairwise connectedness of any two markets, we normalize each entry of the matric and name it pairwise directional connectedness from variable j to variable i or volatility spillover from variable j to variable i" using
Ci←j(H)=θ gij(H)θgij(H)∑Nj=1θgij(H) | (5) |
It measures the sum proportion of volatility shocks from variable i generating to other variables in the total forecast error variance for each variable. The net pairwise spillover index is the difference between the volatility spillover from i to j and the volatility spillover from j to i. If we add up all the non-diagonal
C(H)=∑ni,j=1,i≠jθ gji(H)∑Ni,j=1θ gij(H)=∑ni,j=1,i≠jθ gji(H)N | (6) |
Baruník & Křehlík (2018) measure frequency dynamics (long term, medium term, or short term) of connectedness by use of spectral representation of variance decompositions based on frequency responses to shocks. The scaled generalized variance decomposition on the frequency band
( θd)j,k=(θd)j,k∑k(θ∞)j,k | (7) |
Where
Cϰd=100⋅(∑ θd∑ θ∞−Tr{ θd}∑ θ∞) | (8) |
Which decomposes the total connectedness in Equation (8). Short term, medium term and long-term connectedness will be accessible if we frequency band
The study employs monthly data on financial markets variables and Brent crude oil prices (Brent) for the period January 2005 to January 2022. The sample period considered reflects major global crisis events and allows for the measure of return spillovers and volatility spillovers pre and during the COVID 19 pandemics. Data on the EAC variables of interest were obtained from Bank of Uganda's database while Brent oil data was sourced from the World Bank Commodity Price Data. The financial markets variables considered include the overall interbank money market interest rate and nominal exchange rates for Kenya, Uganda, and Tanzania. The descriptive statistics presented in Table 1 show that all the variables witnessed some variability as indicated by the standard deviations. The gap between the maximum and minimum also reflects a significant level of dispersion from the average return in a market, especially for interbank interest rates. In addition, as indicated in Table 1, Tanzania's interbank rate has marginally more variability than its EAC counterparts Kenya and Uganda while Uganda and Tanzania's exchange rates are more depreciated and experience more variability when compared to Kenya's exchange rate.
Variable | Description | Mean | Maximum | Minimum | Std. Dev. | Observations |
Interbank Kenya | Overall Interbank Interest Rate Kenya | 6.755728 | 28.9 | 0.98 | 4.026051 | 206 |
Interbank Uganda | Overall Interbank Interest Rate Uganda | 9.164757 | 26.68 | 2.65 | 3.971696 | 206 |
Interbank Tanzania | Overall Interbank Interest Rate Tanzania | 6.99466 | 29.72 | 0.87 | 4.738687 | 206 |
KSHS/USD | Natural Log of Nominal Average Kenya Shilling per US dollar Exchange rate (KSHS/USD) | 4.475495 | 4.730754 | 4.125508 | 0.159064 | 206 |
UGX/USD | Natural Log of Average Nominal Uganda Shilling per US dollar Exchange rate (UGX/USD) | 7.87166 | 8.253352 | 7.378221 | 0.293194 | 206 |
TZS/USD | Natural Log of Average Nominal Tanzania Shilling per US dollar Exchange rate (TZS/USD) | 7.424048 | 7.740391 | 6.956364 | 0.259825 | 206 |
Brent | Natural Log of Crude oil (Brent) | 4.24742 | 4.896892 | 3.150169 | 0.344239 | 206 |
The results of unit root tests based on the augmented Dickey–Fuller (ADF) test (Dickey & Fuller, 1979), Phillip-Perron (PP) (Phillips & Perron, 1988), and Kwiatkowski, Phillips, Schmidt, and Shin (KPSS) (Kwiatkowski et al., 1992) are presented in Table 2 above. The results indicate that the variables have mixed orders of integration, that is we have a mix of I(0) and I(1) variables. I(1) variables that indicated non-stationary behaviour are first differenced to make them stationary or I(0) before including them in analyses. Returns series analysed in the study are defined as
Variables | Augmented Dicky Fuller (ADF) test | Phillips-Peron (PP) test | Kwiatkowski-Phillips-Schmidt-Shin | |||
Level | First Difference | Level | First Difference | Level | First Difference | |
Overall Interbank Interest Rate Kenya | −3.965 | −4.042 | 0.199 | |||
Overall Interbank Interest Rate Uganda | −4.185 | −3.124 | 0.173 | |||
Overall Interbank Interest Rate Tanzania | −3.242 | −5.473 | 0.167 | |||
Natural Log of Nominal Average Kenya Shilling per US dollar Exchange rate (KSHS/USD) | −0.472 | −9.979 | −0.437 | −10.051 | 1.667 | 0.118 |
Natural Log of Average Nominal Uganda Shilling per US dollar Exchange rate (UGX/USD) | −1.163 | −9.260 | −0.974 | −8.695 | 1.736 | 0.162 |
Natural Log of Average Nominal Tanzania Shilling per US dollar Exchange rate (TZS/USD) | −1.092 | −9.922 | −1.387 | −9.966 | 1.767 | 0.150 |
Natural Log of Crude oil (Brent) | −3.171 | −2.678 | −9.074 | 0.340 | ||
Asymptotic critical values | ||||||
Significance level | ADF | PP | KPSS | |||
1% | −3.462 | −3.462 | 0.739 | |||
5% | −2.875 | −2.875 | 0.463 | |||
10% | −2.574 | −2.574 | 0.347 |
We examine spillovers and connectedness in the EAC region using the Diebold & Yilmaz (2012) time domain and Baruník & Křehlík (2018) frequency domain standard approaches. The applied analytical framework consists of both the spillover tables which provide static analyses of spillovers and connectedness over the full sample and dynamic spillover analyses which extracts return and volatility spillovers using rolling window analysis.
Tables 3 shows the static return and volatility spillover effects among EAC countries' interbank interest rates and exchange rates over the full sample.
Interbank Kenya | Interbank Uganda | Interbank Tanzania | KSHS/USD | UGX/USD | TZS/USD | Brent | FROM | |
Interbank Kenya | 45.45 | 23.66 | 3.05 | 3.65 | 2.4 | 5.14 | 16.65 | 7.79 |
Interbank Uganda | 14.45 | 61.96 | 5.02 | 1.91 | 2.15 | 4.87 | 9.65 | 5.43 |
Interbank Tanzania | 12.38 | 21.76 | 48.79 | 1.13 | 2.85 | 8.52 | 4.57 | 7.32 |
KSHS/USD | 3.77 | 2.88 | 0.47 | 68.97 | 16.94 | 2.93 | 4.04 | 4.43 |
UGX/USD | 0.79 | 5.11 | 1.66 | 14.19 | 66.13 | 8.24 | 3.88 | 4.84 |
TZS/USD | 1.42 | 2.31 | 3.39 | 7.03 | 8.8 | 75.51 | 1.53 | 3.5 |
Brent | 0.04 | 2.5 | 2.6 | 14.4 | 10.87 | 5.57 | 64.02 | 5.14 |
TO | 4.69 | 8.32 | 2.31 | 6.04 | 6.29 | 5.04 | 5.76 | 38.45 |
NET | −3.10 | 2.88 | −5.00 | 1.61 | 1.45 | 1.54 | 0.62 | |
PANEL B | Interbank Kenya | Interbank Uganda | Interbank Tanzania | KSHS/USD | UGX/USD | TZS/USD | Brent | FROM |
Interbank Kenya | 28.29 | 40.59 | 2.41 | 9.57 | 12.46 | 6.54 | 0.14 | 10.24 |
Interbank Uganda | 7.45 | 57.57 | 3.23 | 13.17 | 14.11 | 4.16 | 0.3 | 6.06 |
Interbank Tanzania | 10.81 | 25.32 | 33.66 | 5.57 | 9.99 | 14.34 | 0.32 | 9.48 |
KSHS/USD | 5.71 | 18.82 | 1.92 | 58.1 | 6.06 | 8.85 | 0.53 | 5.99 |
UGX/USD | 3.57 | 6.4 | 0.57 | 19.31 | 49.63 | 19.8 | 0.71 | 7.2 |
TZS/USD | 0.88 | 0.73 | 1.62 | 11.37 | 6.66 | 76.1 | 2.63 | 3.41 |
Brent | 0.45 | 3.02 | 0.37 | 5.83 | 0.52 | 2.9 | 86.91 | 1.87 |
TO | 4.13 | 13.55 | 1.45 | 9.26 | 7.11 | 8.08 | 0.66 | 44.25 |
NET | −6.12 | 7.49 | −8.03 | 3.28 | −0.08 | 4.67 | −1.21 |
In the Table 3, the elements on the diagonal of the table are own variance shares, and the off-diagonal elements represent the cross-variance shares or spillovers between variables. The off-diagonal column sums and row sums depict directional spillovers 'TO' others and 'FROM' others, while the difference between the TO and FROM are the NET volatility spillovers. The total volatility spillover index is shown in the lower right corner of the table. It is apparent in Table 3 that the total volatility spillover index for the full sample of 44.3 percent was larger than the total return spillover index of 38.5 percent. This is an indication that 38.5 percent of the return forecast error variance comes from return spillovers across the combined system of variables over the sample period whereas the remaining 61.6 percent is explained by idiosyncratic shocks and likewise, on average volatility spillovers account for almost 50 percent of the total forecast error variance of the system of considered variables with 44.3 percent of the volatility forecast error variance in our sample attributed to spillovers while the remaining 55.8 percent can be explained by idiosyncratic shocks. Overall, static time-domain results reveal a relatively high degree of return and volatility spillovers within the system of considered variables, an indication that EAC interbank and foreign exchange markets are characterised by a relatively high level of connectedness with each other and thus offer moderate diversification opportunities for investors.
As shown in Table 3 panel A, the directional return spillovers from all other asset markets to one specific market (FROM) reveals that gross directional return spillovers from others to the Kenyan interbank interest rate is moderately high, at 7.8 percent, closely followed by the Tanzanian interbank interest rate for which gross directional return spillovers from others explain 7.3 percent of the forecast error variance while the TZS/USD receives the lowest contribution of return spillovers from others of about 3.5 percent. This suggests that the return forecast error variance of the TZS/USD exchange rate is predominantly explained by own shocks. The study finds that the highest contributor of return shock spillovers TO other variables' forecast error variance is the Ugandan interbank interest rate with 8.3 percent, followed by the UGX/USD with gross directional return spillovers to others of 6.3 percent. Similarly, considering volatility spillovers, the FROM column in Table 3 panel B which shows gross directional volatility spillovers from others to a specific variable indicates that the highest recipient of gross directional volatility spillovers from others is Kenyan interbank interest rate at 10.2 percent, closely followed by the Tanzanian interbank interest rate with 9.5 percent while the Brent Crude oil receives the lowest contribution of return spillovers from others of about 1.9 percent. In other words, shocks to other market variables account for a significant percentage of the return and volatility forecast error variances of the Kenyan interbank interest rate and Tanzanian interbank interest rate. The gross directional volatility spillovers TO other variables indicates that the highest contributor of volatility shock spillovers to other variables is the Ugandan interbank interest rate with 13.6 percent, followed by the KSHS/USD with gross directional volatility spillovers to other variables of 9.3 percent.
Net spillovers provide a clear summation of the direction and magnitude of return and volatility spillovers, indicating whether a market is a net receiver or a transmitter of return and volatility shocks. The study finds that in terms of net return spillovers, the Ugandan interbank interest rate, KSHS/USD, TZS/USD, UGX/USD, and Brent Crude oil for which spillovers from other variables to them were less than spillovers from them to other variables were net transmitters while the Kenyan interbank interest rate and Tanzanian interbank interest rate whose net spillovers were negative were net receivers of return shocks. In magnitude, the Ugandan interbank interest rate was the biggest net transmitter of return shock spillovers at 2.9 percent while the Tanzanian interbank interest rate was the biggest net receiver of return spillovers at −5.0 percent, followed by the Kenyan interbank interest rate at −3.1 percent. As regards net volatility spillovers, Ugandan interbank interest rate, KSHS/USD and TZS/USD are net transmitters of volatility spillovers the while the Kenyan interbank interest rate, Tanzanian interbank interest rate, Brent Crude oil and UGX/USD were net recipients of volatility spillovers. The largest positive magnitude of net volatility spillovers is recorded for Ugandan interbank interest rate at 7.5 percent which makes it the leading net transmitter of volatility shocks while the largest negative value is recorded for the Tanzanian interbank interest rate at −8.0 percent followed by the Kenyan interbank interest rate at −6.1 percent, which makes these variables major net recipients of volatility shocks mostly generated by Ugandan interbank interest rate. As evidenced by the values of the diagonal entries in Table 3, Kenya and Tanzania's money markets have a relatively low level of own return and volatility connectedness while Kenya and Uganda's foreign exchange markets have relatively low levels of own return and volatility connectedness, an indication that own shocks explain a limited proportion of these variables' return and volatility forecast error variance. These results suggest that Kenya's financial sector is the most open of the three considered EAC partner economies.
According to the results of the net pairwise spillovers present in Table 4, the biggest positive magnitude of net pairwise return spillovers is recorded between the Kenyan interbank interest rate and Brent Crude oil of 2.4 percent, indicating that the Kenyan interbank interest rate is a net transmitter of return shocks with respect to Brent Crude oil. This may be an indication that return shocks to the Kenyan interbank interest rate affect Brent Crude oil price returns via the impact on investment and consumption in Kenya, an oil importing nation whose dependence on oil is reflected in the huge reliance on the supply and affordability oil for among other things transport, power generation, manufacturing, and agricultural sectors. Also evident is the largest negative magnitude of net pairwise return spillovers recorded is between the Ugandan interbank interest rate and Tanzanian interbank interest rate at −2.4 percent, indicating that the Ugandan interbank interest rate is a net receiver of return shocks with respect to Tanzanian interbank interest rate. In terms of the net pairwise directional volatility spillovers, the largest positive net pairwise volatility spillover is recorded between the Kenyan interbank interest rate and Ugandan interbank interest rate at 4.7 percent, indicating that the Kenyan interbank interest rate transmits more volatility to the Ugandan interbank interest rate. Similarly, the largest negative net pairwise volatility spillover is recorded between Ugandan interbank interest rate and Tanzanian interbank interest rate at −3.2 percent, an indication that Tanzanian interbank interest rate is a net transmitter of volatility shocks to the Ugandan interbank interest rate. By and large, all EAC exchange rates are net receivers of return shocks from Brent Crude oil whereas Brent Crude oil except for the KSHS/USD at −0.8 percent is not a source of volatility spillovers in the EAC considered economies. Of interest is the finding that amongst the overall interbank market interest rates in all three EAC countries, Tanzania is a consistent net transmitter of pairwise return and volatility spillovers while Uganda is a consistent net recipient of pairwise return and volatility shock spillovers.
Time Based Pairwise Spillovers | Frequency Based Pairwise Spillovers | |||||||
return | volatility | return1 | return2 | return3 | volatility1 | volatility2 | volatility3 | |
Interbank Kenya-Interbank Uganda | 1.32 | 4.73 | 0.01 | 0.06 | 1.25 | 0.00 | 0.25 | 4.49 |
Interbank Kenya-Interbank Tanzania | −1.33 | −1.20 | −0.02 | −0.07 | −1.24 | −0.93 | −0.07 | −0.19 |
Interbank Kenya-KSHS/USD | −0.02 | 0.55 | 0.02 | −0.04 | 0.00 | −0.51 | −0.13 | 1.18 |
Interbank Kenya-UGX/USD | 0.23 | 1.27 | −0.01 | 0.01 | 0.23 | 0.05 | −0.07 | 1.29 |
Interbank Kenya-TZS/USD | 0.53 | 0.81 | −0.06 | 0.01 | 0.59 | 0.04 | 0.13 | 0.64 |
Interbank Kenya-Brent | 2.37 | −0.04 | 0.00 | 0.00 | 2.37 | −0.01 | −0.01 | −0.02 |
Interbank Uganda-Interbank Tanzania | −2.39 | −3.16 | −0.05 | −0.08 | −2.26 | −0.05 | −0.01 | −3.09 |
Interbank Uganda-KSHS/USD | −0.14 | −0.81 | −0.04 | −0.08 | −0.03 | −0.14 | −0.48 | −0.19 |
Interbank Uganda-UGX/USD | −0.42 | 1.10 | −0.20 | −0.10 | −0.12 | 0.03 | −0.04 | 1.11 |
Interbank Uganda-TZS/USD | 0.37 | 0.49 | −0.10 | −0.03 | 0.50 | 0.00 | 0.01 | 0.48 |
Interbank Uganda-Brent | 1.02 | −0.39 | 0.00 | 0.00 | 1.02 | 0.00 | −0.07 | −0.32 |
Interbank Tanzania-KSHS/USD | 0.09 | 0.52 | 0.02 | 0.02 | 0.06 | 0.00 | −0.02 | 0.54 |
Interbank Tanzania-UGX/USD | 0.17 | 1.35 | −0.06 | −0.03 | 0.26 | 0.39 | 0.06 | 0.90 |
Interbank Tanzania-TZS/USD | 0.73 | 1.82 | 0.09 | 0.07 | 0.57 | 0.10 | 0.46 | 1.26 |
Interbank Tanzania-Brent | 0.28 | −0.01 | 0.01 | 0.01 | 0.26 | 0.00 | 0.01 | −0.02 |
KSHS/USD-UGX/USD | 0.39 | −1.89 | 0.10 | 0.20 | 0.09 | 0.43 | 0.06 | −2.39 |
KSHS/USD-TZS/USD | −0.59 | −0.36 | −0.05 | −0.17 | −0.37 | 0.20 | −0.07 | −0.49 |
KSHS/USD-Brent | −1.48 | −0.76 | 0.26 | 0.10 | −1.84 | 0.02 | −0.03 | −0.75 |
UGX/USD-TZS/USD | −0.08 | 1.88 | −0.04 | 0.00 | −0.04 | −0.04 | 0.13 | 1.78 |
UGX/USD-Brent | −1.00 | 0.03 | 0.22 | 0.12 | −1.34 | 0.01 | 0.01 | 0.01 |
TZS/USD-Brent | −0.58 | −0.04 | 0.06 | 0.05 | −0.69 | 0.00 | −0.03 | −0.01 |
Notes: The net pairwise spillovers is the difference between the spillovers from i to j and the spillovers from j to i, whereby when the value is positive variable i transmits more spillovers to variable j, whereas when it is negative variable j send more spillovers variable i. |
According to Baruník & Křehlík (2018), heterogeneous frequency responses to shocks are simply aggregated in time domain and thus are insufficient to reveal differentiated linkages within different frequency bands amongst the EAC considered asset markets. As such Tables 5 and 6 display the results of static return and volatility frequency domain connectedness in which spillovers in time domain are deconstructed into three different frequency bands corresponding to the in short term (1–3 months) which corresponds to a quarter, medium term (3–6 months) which corresponds to half a year, and long term (more than 6 months), respectively. As shown in Table 5, the total return spillover index, an indicator of overall return connectedness, ranges from 4.1 percent to 29.9 percent at different frequency bands with the level of connectedness strengthening across the considered EAC markets as the frequency band increases. Explicitly, the results indicate that total return spillovers from long-term frequency of 29.9 percent contribute the most to total connectedness, followed by the short-term (4.4 percent) and medium-term (4.1 percent) frequencies. This suggests that shocks to the whole system of selected variables are persistent, lasting more than 6 months, as the market responds slowly to spillover shocks, mainly at low frequencies. These results are inconsistent with the efficiency market hypothesis which posits that asset prices quickly reflect all pertinent information (Degutis & Novickytė, 2014; Langevoort, 1992; Lo, 2008).
1–3 months | Interbank Kenya | Interbank Uganda | Interbank Tanzania | KSHS/USD | UGX/USD | TZS/USD | Brent | FROM_ABS | FROM_WTH |
Interbank Kenya | 6.81 | 0.21 | 0.04 | 0.33 | 0.02 | 0.08 | 0.00 | 0.10 | 0.45 |
Interbank Uganda | 0.17 | 4.75 | 0.48 | 0.11 | 0.24 | 0.09 | 0.03 | 0.16 | 0.72 |
Interbank Tanzania | 0.19 | 0.86 | 12.51 | 0.14 | 0.22 | 1.80 | 0.09 | 0.47 | 2.14 |
KSHS/USD | 0.22 | 0.36 | 0.02 | 34.39 | 5.20 | 0.81 | 1.89 | 1.22 | 5.52 |
UGX/USD | 0.08 | 1.67 | 0.64 | 4.47 | 28.74 | 2.19 | 1.61 | 1.53 | 6.92 |
TZS/USD | 0.52 | 0.78 | 1.15 | 1.15 | 2.47 | 34.37 | 0.43 | 0.93 | 4.22 |
Brent | 0.00 | 0.00 | 0.01 | 0.09 | 0.07 | 0.02 | 1.67 | 0.03 | 0.13 |
TO_ABS | 0.17 | 0.55 | 0.34 | 0.90 | 1.18 | 0.71 | 0.58 | 4.43 | |
TO_WTH | 0.77 | 2.52 | 1.52 | 4.08 | 5.34 | 3.24 | 2.63 | 20.10 | |
NET | 0.07 | 0.40 | −0.14 | −0.32 | −0.35 | −0.22 | 0.55 | ||
3–6 months | Interbank Kenya | Interbank Uganda | Interbank Tanzania | KSHS/USD | UGX/USD | TZS/USD | Brent | FROM_ABS | FROM_WTH |
Interbank Kenya | 5.57 | 0.83 | 0.21 | 0.19 | 0.15 | 0.38 | 0.02 | 0.25 | 1.82 |
Interbank Uganda | 0.41 | 4.59 | 0.39 | 0.02 | 0.15 | 0.24 | 0.02 | 0.18 | 1.26 |
Interbank Tanzania | 0.70 | 0.91 | 8.66 | 0.14 | 0.16 | 1.16 | 0.11 | 0.45 | 3.27 |
KSHS/USD | 0.44 | 0.55 | 0.02 | 15.60 | 4.90 | 0.83 | 1.03 | 1.11 | 8.00 |
UGX/USD | 0.08 | 0.87 | 0.37 | 3.53 | 14.84 | 2.18 | 1.00 | 1.15 | 8.26 |
TZS/USD | 0.33 | 0.46 | 0.64 | 2.04 | 2.16 | 17.19 | 0.44 | 0.87 | 6.26 |
Brent | 0.00 | 0.02 | 0.03 | 0.32 | 0.19 | 0.07 | 2.04 | 0.09 | 0.65 |
TO_ABS | 0.28 | 0.52 | 0.24 | 0.89 | 1.10 | 0.69 | 0.38 | 4.10 | |
TO_WTH | 2.02 | 3.74 | 1.72 | 6.42 | 7.92 | 5.00 | 2.71 | 29.53 | |
NET | 0.03 | 0.34 | −0.22 | −0.22 | −0.05 | −0.17 | 0.29 | ||
Beyond 6 months | Interbank Kenya | Interbank Uganda | Interbank Tanzania | KSHS/USD | UGX/USD | TZS/USD | Brent | FROM_ABS | FROM_WTH |
Interbank Kenya | 33.07 | 22.62 | 2.80 | 3.13 | 2.23 | 4.68 | 16.62 | 7.44 | 11.61 |
Interbank Uganda | 13.87 | 52.62 | 4.15 | 1.78 | 1.75 | 4.55 | 9.60 | 5.10 | 7.96 |
Interbank Tanzania | 11.50 | 19.99 | 27.63 | 0.84 | 2.47 | 5.56 | 4.37 | 6.39 | 9.97 |
KSHS/USD | 3.11 | 1.98 | 0.43 | 18.99 | 6.84 | 1.29 | 1.11 | 2.11 | 3.29 |
UGX/USD | 0.63 | 2.57 | 0.65 | 6.18 | 22.54 | 3.87 | 1.27 | 2.17 | 3.38 |
TZS/USD | 0.57 | 1.06 | 1.60 | 3.84 | 4.16 | 23.96 | 0.65 | 1.70 | 2.65 |
Brent | 0.04 | 2.48 | 2.55 | 13.98 | 10.62 | 5.47 | 60.31 | 5.02 | 7.84 |
TO_ABS | 4.24 | 7.24 | 1.74 | 4.25 | 4.01 | 3.63 | 4.80 | 29.92 | |
TO_WTH | 6.62 | 11.30 | 2.72 | 6.64 | 6.26 | 5.67 | 7.50 | 46.70 | |
NET | −3.20 | 2.14 | −4.65 | 2.15 | 1.84 | 1.93 | −0.22 | ||
Notes: FROM_WTH and TO_WTH capture 'within' connectedness which refers to the connectedness effect that occurs within the frequency band and is weighted by the power of the series on the given frequency band exclusively while FROM_ABS and TO_ABS refer to the frequency connectedness decomposition of the original Diebold & Yilmaz (2012) connectedness measures into distinct parts that when summed provide the original connectedness measures. Hence FROM ABS is the measure of frequency connectedness in absolute sense while FROM WTH is the measure of 'within' connectedness. |
1–3 months | Interbank Kenya | Interbank Uganda | Interbank Tanzania | KSHS/USD | UGX/USD | TZS/USD | Brent | FROM_ABS | FROM_WTH | ||||||||||
Interbank Kenya | 13.61 | 0.86 | 0.47 | 0.41 | 1.95 | 0.40 | 0.01 | 0.59 | 4.07 | ||||||||||
Interbank Uganda | 0.88 | 1.30 | 0.34 | 0.20 | 0.26 | 0.02 | 0.00 | 0.24 | 1.70 | ||||||||||
Interbank Tanzania | 7.01 | 0.72 | 10.32 | 0.26 | 2.76 | 0.71 | 0.02 | 1.64 | 11.39 | ||||||||||
KSHS/USD | 3.94 | 1.18 | 0.25 | 17.01 | 4.09 | 1.82 | 0.17 | 1.64 | 11.37 | ||||||||||
UGX/USD | 1.58 | 0.07 | 0.02 | 1.06 | 8.93 | 0.39 | 0.12 | 0.46 | 3.20 | ||||||||||
TZS/USD | 0.12 | 0.01 | 0.04 | 0.41 | 0.63 | 7.27 | 0.01 | 0.17 | 1.22 | ||||||||||
Brent | 0.09 | 0.04 | 0.01 | 0.06 | 0.07 | 0.04 | 8.81 | 0.04 | 0.30 | ||||||||||
TO_ABS | 1.95 | 0.41 | 0.16 | 0.34 | 1.40 | 0.48 | 0.05 | 4.78 | |||||||||||
TO_WTH | 13.52 | 2.86 | 1.12 | 2.37 | 9.69 | 3.35 | 0.33 | 33.24 | |||||||||||
NET | 1.36 | 0.17 | −1.48 | −1.30 | 0.93 | 0.31 | 0.01 | ||||||||||||
3–6 months | Interbank Kenya | Interbank Uganda | Interbank Tanzania | KSHS/USD | UGX/USD | TZS/USD | Brent | FROM_ABS | FROM_WTH | ||||||||||
Interbank Kenya | 4.39 | 2.68 | 0.66 | 0.08 | 0.25 | 0.97 | 0.01 | 0.66 | 5.19 | ||||||||||
Interbank Uganda | 0.96 | 3.39 | 0.72 | 0.19 | 0.09 | 0.12 | 0.01 | 0.30 | 2.32 | ||||||||||
Interbank Tanzania | 1.18 | 0.80 | 8.72 | 0.05 | 0.44 | 3.62 | 0.13 | 0.89 | 6.94 | ||||||||||
KSHS/USD | 0.96 | 3.56 | 0.17 | 7.99 | 1.45 | 0.50 | 0.16 | 0.97 | 7.60 | ||||||||||
UGX/USD | 0.77 | 0.34 | 0.05 | 1.02 | 6.02 | 1.23 | 0.23 | 0.52 | 4.08 | ||||||||||
TZS/USD | 0.09 | 0.03 | 0.39 | 1.01 | 0.31 | 10.97 | 0.18 | 0.29 | 2.24 | ||||||||||
Brent | 0.11 | 0.48 | 0.09 | 0.34 | 0.15 | 0.37 | 21.07 | 0.22 | 1.72 | ||||||||||
TO_ABS | 0.58 | 1.13 | 0.30 | 0.38 | 0.38 | 0.97 | 0.10 | 3.85 | |||||||||||
TO_WTH | 4.54 | 8.81 | 2.32 | 3.01 | 2.99 | 7.62 | 0.80 | 30.10 | |||||||||||
NET | −0.08 | 0.83 | −0.59 | −0.59 | −0.14 | 0.69 | −0.12 | ||||||||||||
Beyond 6 months | Interbank Kenya | Interbank Uganda | Interbank Tanzania | KSHS/USD | UGX/USD | TZS/USD | Brent | FROM_ABS | FROM_WTH | ||||||||||
Interbank Kenya | 10.28 | 37.06 | 1.28 | 9.09 | 10.26 | 5.17 | 0.12 | 9.00 | 12.35 | ||||||||||
Interbank Uganda | 5.62 | 52.88 | 2.17 | 12.77 | 13.77 | 4.02 | 0.30 | 5.52 | 7.58 | ||||||||||
Interbank Tanzania | 2.62 | 23.80 | 14.63 | 5.27 | 6.78 | 10.01 | 0.17 | 6.95 | 9.54 | ||||||||||
KSHS/USD | 0.81 | 14.08 | 1.49 | 33.10 | 0.53 | 6.53 | 0.20 | 3.38 | 4.64 | ||||||||||
UGX/USD | 1.23 | 5.98 | 0.50 | 17.24 | 34.69 | 18.18 | 0.36 | 6.21 | 8.53 | ||||||||||
TZS/USD | 0.67 | 0.69 | 1.19 | 9.95 | 5.72 | 57.87 | 2.44 | 2.95 | 4.05 | ||||||||||
Brent | 0.26 | 2.50 | 0.28 | 5.43 | 0.30 | 2.49 | 57.03 | 1.61 | 2.21 | ||||||||||
TO_ABS | 1.60 | 12.02 | 0.99 | 8.53 | 5.34 | 6.63 | 0.51 | 35.62 | |||||||||||
TO_WTH | 2.20 | 16.50 | 1.36 | 11.72 | 7.33 | 9.10 | 0.70 | 48.90 | |||||||||||
NET | −7.39 | 6.49 | −5.96 | 5.16 | −0.88 | 3.68 | −1.10 | ||||||||||||
Notes: FROM_WTH and TO_WTH capture 'within' connectedness which refers to the connectedness effect that occurs within the frequency band and is weighted by the power of the series on the given frequency band exclusively while FROM_ABS and TO_ABS refer to the frequency connectedness decomposition of the original Diebold & Yilmaz (2012) connectedness measures into distinct parts that when summed provide the original connectedness measures. Hence FROM ABS is the measure of frequency connectedness in absolute sense while FROM WTH is the measure of 'within' connectedness. |
The net return spillover analysis in Table 5 reveals that while overall net return spillovers are very small in magnitude in the short to medium term frequency bands, they increase considerably and are sizeable at the long-term frequency. In the long term, the biggest net transmitters of shocks to the system of considered variables are the KSHS/USD exchange rate and Ugandan interbank interest rate with net return spillovers of 2.1 percent respectively, followed by TZS/USD and UGX/USD exchange rates at 1.9 percent and 1.8 percent respectively. These results are consistent with existing evidence that suggests flexible exchange rates may not always insulate small open economies from external shocks (López & Perrotini, 2006; Mahadeva & Sterne, 2012; Tobin & de Macedo, 1979) as well as assertions by Mahadeva and Sterne (2012) that exchange rates in small open economies may be a potential source of shocks. On the other hand, the biggest net receiver of return shock spillovers across the system in the long term is the Tanzanian interbank interest rate at −4.6 percent followed by the Kenyan interbank interest rate at −3.2 percent. Interestingly, crude oil is a net transmitter of return shock spillovers at the short to medium term frequency but switches in the long term to being a recipient of return shock spillovers although the magnitude of its net effect is rather small. Further it appears the money and foreign exchange markets of Kenya and Uganda are the most closely connected to developments in crude oil, especially in the long term, which is consistent with their heavy reliance on imported oil.
Similarly, Table 6 reveals the increase frequency domain total connectedness of the system of considered EAC variables as the frequency band increases with the total volatility spillover index ranging from 3.9 percent to 35.6 percent. Overall, total volatility spillovers are highest in the long-term at 35.6 percent followed by the short-term frequency at 4.8 percent and lastly in the medium-term at 3.9 percent. This is an indication that volatility shocks are persistent. Specifically, the observation that high connectedness of the system is driven by low-frequency responses to shocks suggests that volatility shocks generate increased uncertainty which translates into long-term uncertainty due to the persistence of shocks and investor uncertainty, thus increasing volatility in the long term (Baruník & Křehlík, 2018). Likewise, net volatility spillovers are very small in magnitude, less than 1 percent at the medium-term frequency band and in the short term for Brent crude oil, Ugandan interbank interest rate, TZS/USD and UGX/USD as shown in Table 6. The biggest net transmitter of volatility shocks in the long term is Ugandan interbank interest rate at 6.5 percent followed by the KSHS/USD exchange rate at 5.2 percent and TZS/USD while the largest net recipient of volatility shock spillovers is the Kenyan interbank interest rate at -7.4 percent, followed by Tanzanian interbank interest rate at −6.0 percent, then Brent crude oil and the UGX/USD exchange rate at −1.1 percent and −0.9 percent respectively. In contrast, in the short-term the Kenyan interbank interest rate at 1.4 percent is the biggest net transmitter of volatility shocks, followed by UGX/USD exchange rate at 0.9 percent while the Tanzanian interbank interest rate and KSHS/USD exchange rate at −1.5 percent and −1.3 percent respectively are the largest net receivers of volatility spillover shocks.
In Table 4, except for the long-term frequency band, the analysis of frequency domain net pairwise return and volatility spillovers show that net pairwise return and volatility spillovers are generally small in magnitude, close to zero. In the long term, the biggest net transmitter of return spillovers is the Kenyan interbank interest rate with respect to Brent crude oil with a magnitude of net pairwise return spillovers of 2.4 percent, followed by the respective net pairwise transmission of return spillovers from the Kenyan interbank interest rate and Ugandan interbank interest rate to the Ugandan interbank interest and Brent crude oil of 1.3 percent and 1.0 percent respectively. Conversely, the largest pairwise net recipient of return spillovers is the Ugandan interbank interest with respect to the Tanzanian interbank interest rate at −2.3 percent, followed by the KSHS/USD exchange rate, UGX/USD exchange rate and Kenyan interbank interest rate with respect to Brent crude oil, Brent crude oil and the Tanzanian interbank interest rate at −1.8 percent, −1.3 percent, and −1.2 percent respectively. Consistent with the findings of frequency domain net pairwise return spillover analysis, net pairwise return spillovers are greater in magnitude in the long term than in the short to medium term. As shown in Table 4, in the long term, the biggest magnitude of net pairwise volatility spillovers recorded is between the Kenyan interbank interest rate and Ugandan interbank interest rate of 4.5 percent, an indication that the Kenyan interbank interest rate is a net transmitter of volatility shocks with respect to the Ugandan interbank interest rate. In addition, the UGX/USD is net transmitter of pairwise volatility shocks with respect to TZS/USD at 1.8 percent, the Tanzanian interbank interest rate is net transmitter of pairwise volatility shocks with respect to TZS/USD at 1.3 percent, the Ugandan interbank interest rate is net transmitter of pairwise volatility shocks with respect to UGX/USD at 1.1 percent, while the Kenyan interbank interest rate is net transmitter of pairwise volatility shocks with respect to UGX/USD and KSHS/USD at 1.3 percent and 1.2 percent respectively. Conversely, the largest net pairwise receiver of volatility spillovers is the Ugandan interbank interest with respect to the Tanzanian interbank interest rate at −3.1 percent, followed by the KSHS/USD exchange rate with respect to the UGX/USD exchange rate at −2.4 percent.
The study measures dynamic time connectedness by estimating the total return and volatility spillovers using rolling samples analysis to capture the evolution of return volatility spillovers over time. Overall, total return and volatility spillover plots presented in Figures 2 and 3 reveal significant variation in the evolution of spillovers over time. In Figure 2, the total return spillover index shows fluctuate around an average of 51.8 percent over the sample period except for the significant peaks in return spillovers registered in 2012 and 2016 that coincide with key global shocks. In 2012, the EAC economies experienced adverse shocks emanating from the European debt crisis, due to deep financial linkages in trade, aid, and investments between the EAC member states and Eurozone(The EastAfrican, 2012). In 2016 EAC countries experienced another exogenous shock following the largely unanticipated shock result in June 2016 of the Brexit referendum vote in the United Kingdom (UK) that reverberated across the world, renewing volatility in global financial markets (Juma, 2016; Wearden & Fletcher, 2016). As indicated in Figure 2, although there was a marked increase in return spillovers during the COVID-19 pandemic, the impact was gradual, less pronounced but persistent when compared to previous global shocks. This may be due to the tireless efforts by EAC governments to control its spread and unprecedented negative economic effects. Indeed, return spillovers drop to 44.7 percent following the lifting of COVID-19 restrictions and lockdowns in 2022. Generally, the time-varying behaviour of the total return spillover index reveals an amplification of return shock spillover during periods of high uncertainty, such as sovereign debt crisis in Europe and the Brexit shock.
Regarding the evolution of the total volatility spillover index in Figure 3, the total return spillover index also shows significant fluctuation, averaging 67.5 percent over the sample period. Consistent with the findings of the static time domain analysis, average Total volatility spillovers are greater than Total return spillovers for the considered sample period. The peak in volatility spillovers in 2012 coincides with the adverse shocks to EAC economies emanating from the European debt crisis because of the close trade, aid, and investment ties between the EAC member states and Eurozone (The EastAfrican, 2012). The peak in late 2015 of total volatility spillovers was prompted by a commodity price shock. Although the initial fall in oil prices from mid-2014 to early 2015 was primarily driven by supply factors, deteriorating demand prospects played a role as well, particularly from mid-2015 to early 2016 (Stocker et al., 2018). In addition, despite the brief decline in early 2016, volatility spillovers continued to gradually rise throughout 2016. The period 2017 to 2019 was characterised by intermittent episodes of financial and macroeconomic volatility owing largely to geopolitical tensions and policy uncertainty. The significant rise in total volatility spillovers registered in 2017 may be attributed to heightened political tensions, increased macroeconomic risk and uncertainty as well as the accompanying slowdown in economic activity arising from the protracted violent and hotly contested Kenyan elections of 2017, in which President Uhuru Kenyatta was declared the winner on October 26, 2017, following Supreme Court's nullification of the original election on August 8, 2017 (Moore, 2017; The World Bank, 2017). Total volatility spillovers rise and remain elevated throughout 2018 to 2019 except for the period November to December 2018. This was largely on account of intermittent episodes of heightened global financial market volatility arising from escalating trade tensions between the United States and China, prolonged uncertainty on Brexit, concerns that the Federal Reserve was raising interest rates too quickly, worries about peace and stability in the Middle East and currency instability in some major emerging market economies (Bank of Uganda, 2019; UNECA, 2018). Importantly, the significant effect of the COVID-19 pandemic and accompanying severe economic impact can be seen in the marked increase in total volatility spillovers in early 2020 following the implementation of lockdowns and other stringent measures to contain the COVID-19 pandemic in EAC economies. Total volatility spillovers fall moderately and remain stable, averaging 74.2 percent in the period August 2020 to October 2021. This drop coincides with the end of Kenya's the nationwide lockdown in October 2021 that had been in place since March 2020 to curb the COVID- 19 pandemic in Kenya (Al Jazeera, 2021). The subsequent decline in total volatility spillovers in the period November 2021 to January 2022 averaging 50.3 percent can be attributed to the lifting of Uganda's Lockdown in January 2022 (Daily Monitor, 2022).
The graphical representation of net directional return and volatility connectedness presented in Figure 4 and Figure 5 respectively show significant variation in return and volatility spillovers over time and across countries. Net return spillovers show the difference between the gross return shocks that are transmitted to and those receives from all other markets Diebold & Yilmaz (2012). In the case of the Kenya interbank interest rate, the evolution of net return spillover index in Figure 4 shows that is has predominantly been a net receiver of return spillovers from others even the magnitude of return shock spillovers was moderate averaging under 5 percent for a bigger portion of the sample period. The Kenya interbank interest rate was a net transmitter of return shock spillovers during the European debt crisis in 2012 as indicated by the marked increase in return connectedness and spillovers of close to 15 percent in 2012. In the period after early 2012 until 2021, the Kenyan interbank interest rate was predominantly a net receiver of return shocks. Albeit, in 2019 there was a short-lived episode with marked reduction in shock spillovers received due to intermittent episodes of heightened global financial market instability and volatility arising from geopolitical uncertainty and trade tensions the plagued 2019. Similarly, the Tanzania interbank interest rate was predominantly a net receiver of return shocks over the sample period except for a brief period in early 2016 where it was a net transmitter of return shocks which can be attributed to commodity price shocks the began in mid-2014 to early 2015 all through to early 2016. In addition, there is marked reduction in shock spillovers received in 2019 largely on account of geopolitical uncertainty and trade tensions the plagued 2019 causing intermittent episodes of heightened global financial market instability and volatility. In contrast, the Ugandan interbank interest rate was a net transmitter of return shocks to all other markets from the start of the sample period up to early 2019, with spillovers increasing significantly during the European debt crisis in 2012 as indicated by the marked increase in return connectedness and spillovers of over 25 percent in 2012 and except for a brief period in early 2016 where it was a net recipient of return shocks which can be attributed to commodity price shocks. This situation changes in 2019 with the Ugandan interbank interest rate becoming a net receiver of return shocks from all other markets in 2019 largely on account of geopolitical uncertainty and trade tensions the plagued 2019 causing intermittent episodes of heightened global financial market instability and volatility. During the COVID-19 pandemic, the Ugandan interbank interest rate remained a net receiver of return shocks although the magnitude of shock spillovers was quite low in some periods until the end of the lockdown period in 2021 when it turned into a net transmitter of return shock spillovers.
The graphical representation of net directional return and volatility connectedness presented in Figure 4 and Figure 5 respectively show significant variation in return and volatility spillovers over time and across countries. Net return spillovers show the difference between the gross return shocks that are transmitted to and those receives from all other markets Diebold & Yilmaz (2012). In the case of the Kenya interbank interest rate, the evolution of net return spillover index in Figure 4 shows that is has predominantly been a net receiver of return spillovers from others even the magnitude of return shock spillovers was moderate averaging under 5 percent for a bigger portion of the sample period. The Kenya interbank interest rate was a net transmitter of return shock spillovers during the European debt crisis in 2012 as indicated by the marked increase in return connectedness and spillovers of close to 15 percent in 2012. In the period after early 2012 until 2021, the Kenyan interbank interest rate was predominantly a net receiver of return shocks. Albeit, in 2019 there was a short-lived episode with marked reduction in shock spillovers received due to intermittent episodes of heightened global financial market instability and volatility arising from geopolitical uncertainty and trade tensions the plagued 2019. Similarly, the Tanzania interbank interest rate was predominantly a net receiver of return shocks over the sample period except for a brief period in early 2016 where it was a net transmitter of return shocks which can be attributed to commodity price shocks the began in mid-2014 to early 2015 all through to early 2016. In addition, there is marked reduction in shock spillovers received in 2019 largely on account of geopolitical uncertainty and trade tensions the plagued 2019 causing intermittent episodes of heightened global financial market instability and volatility. In contrast, the Ugandan interbank interest rate was a net transmitter of return shocks to all other markets from the start of the sample period up to early 2019, with spillovers increasing significantly during the European debt crisis in 2012 as indicated by the marked increase in return connectedness and spillovers of over 25 percent in 2012 and except for a brief period in early 2016 where it was a net recipient of return shocks which can be attributed to commodity price shocks. This situation changes in 2019 with the Ugandan interbank interest rate becoming a net receiver of return shocks from all other markets in 2019 largely on account of geopolitical uncertainty and trade tensions the plagued 2019 causing intermittent episodes of heightened global financial market instability and volatility. During the COVID-19 pandemic, the Ugandan interbank interest rate remained a net receiver of return shocks although the magnitude of shock spillovers was quite low in some periods until the end of the lockdown period in 2021 when it turned into a net transmitter of return shock spillovers.
Intriguingly, the KSHS/USD exchange rate was a net transmitter of return shocks spillovers from the start of the time sample until the year 2022 except for brief periods in early 2012 and 2016 which coincide with the European debt crisis in 2012 and commodity price shocks respectively. In addition, there is a marked increase in spillovers to other markets in the period corresponding to the amplification of geopolitical uncertainty and trade tensions in 2019 and a slight drop in the magnitude of spillovers transmitted to other markets during the COVID-19 pandemic. In contrast the UGX/USD exchange rate from the start of the sample to early 2019 registers low net return spillover values that fluctuate around zero suggesting that during this period it was more impacted by own connectedness except for the brief episodes in 2012 and 2016 during which it was a net receiver of return shock spillovers that coincide with the European debt crisis in 2012 and commodity price shocks respectively. Conversely, net return spillovers for the TZS/USD exchange were low and fluctuate around zero in the period 2011 to 2015 except for the episode in early 2012 that corresponds to the 2012 European debt crisis during which it was a net receiver of return shock spillovers from other markets. Net return spillovers rise mid 2015 which coincide with the early stages of the commodity price shock, turning the TZS/USD exchange into a net transmitter of return shock spillovers. This is followed by a brief episode in early 2016 during which it was a net receiver of return shocks spillovers which corresponds to the peak of the commodity price shocks. There after the TZS/USD exchange remains a net transmitter of shock spillovers which increased markedly during the period of geopolitical uncertainty and trade tensions in 2019, remaining elevated except for a brief fall at the start of the covid 19 pandemic and rise to levels of close to 20 percent until the end of the pandemic in early 2022 when net return spillovers drop close to zero. Brent crude oil return are net transmitters of shock spillover especially during crisis events, as indicated by the marked increase in net return shock spillover transmission in early 2012 which corresponds to the European debt crisis, early 2016 which coincide with the commodity price shock, 2019 which corresponds to the period of global geopolitical uncertainty and trade tensions and the start of the COVID-19 induced lockdowns in 2020. Outside these episodes, Brent crude oil's net return spillovers are small and fluctuate close zero except for brief episodes where it is a net receiver of return shock spillovers from other markets.
The time varying evolution of net directional volatility spillovers for the considered variables shown in Figure 5 are calculated by subtracting directional 'TO' spillovers from directional 'FROM' spillovers, with positive (negative) values indicating a transmitter (receiver) of volatility to (from) other markets.
As shown in Figure 5, the Kenya interbank interest rate is largely a net receiver of volatility shock spillovers from other markets throughout the sample period except for during crisis periods such as the brief episodes in early 2012 that coincide with the European debt crisis in 2012 and the period covering the commodity price shocks in mid-2015 and early 2016. In addition, volatility shock spillovers gradually rise during the COVID-19 pandemic reducing the magnitude of net volatility spillovers received by the Kenyan interbank interest rate. Similarly, the Tanzania interbank interest rate was predominantly a net receiver of return shocks over the sample period except for a brief period in early 2016 which coincides with the commodity price shocks at the time where it was a net transmitter of return shocks albeit of small magnitude. In general, net directional volatility spillovers to the Tanzania interbank interest rate from other markets tend to increase markedly during crisis periods such as the 2012 European debt crisis, the mid 2015 to 2016 commodity price shocks, the geopolitical and trade tensions in 2018 and the start of the COVID-19 pandemic lockdowns in the neighbouring Kenya and Uganda. In contrast, the Ugandan interbank interest rate was a net transmitter of return shocks to all other markets from the start of the sample period up to mid-2015, a period coinciding with the commodity price shocks, when it briefly became a net receiver of volatility shock spillovers. Thereafter, the Ugandan interbank interest rate net directional volatility spillovers fluctuate close to zero until early 2018, an indication that it was largely explained by own connectedness during this period. In the period 2018 to 2021 which coincides with the amplification of global financial market volatility arising from geopolitical and trade tensions in 2018 to 2019 as well as the COVID-19 induced lockdowns of 2020 to 2021, the Ugandan interbank interest rate was predominantly a net receiver of volatility shock spillovers. In early 2022, the Ugandan interbank interest rate reverted to being a net transmitter of volatility spillovers to other markets following the full lifting of the two-year lockdown. Interestingly the magnitude of net directional volatility spillovers from the Ugandan interbank interest rate is higher than that from the Kenyan and Tanzanian interbank interest rates.
In addition, Figure 5 shows that the KSHS/USD exchange rate was predominantly a net transmitter of volatility shocks spillovers over the sample period except for brief episodes that correspond to the European debt crisis in 2012 and the commodity price shocks in late 2015 to early 2016. Net directional volatility spillovers increase markedly in early 2018 and remain elevated before declining in early 2022, a period covering both the geopolitical and trade tensions of 2018 and 2019 as well as the COVID-19 pandemic of 2020 and 2021. There are also significant spikes that mark the onset of the COVID-19 induced lockdowns. Similarly, the TZS/USD exchange was predominantly a net transmitter of volatility spillovers to other markets, except for brief periods such as the 2012 European debt crisis, the onset of the COVID-19 induced lockdowns and the end of lockdown in January 2022. However, it registered greater magnitudes of net directional volatility spillovers when compared with the KSHS/USD and UGX/USD exchange rates. There is a generally strengthening of connectedness and increase in net directional volatility spillovers after the commodity price shocks in 2015. In contrast, the UGX/USD exchange rate was predominantly a net recipient of volatility shock spillovers except for the period 2016 to 2018. In addition, net volatility spillovers received increase markedly during crisis periods such as the 2012 European debt crisis, the commodity price shocks in mid-2015 to early 2016, the geopolitical and trade tensions of 2018 and 2019 and the COVID-19 pandemic of 2020 and 2021. On the other hand, Brent crude oil is a net transmitter of volatility shock spillovers especially during crisis events, such as European debt crisis in early 2012, the commodity price shocks in mid-2015 to early 2016 and the onset of the COVID-19 induced lockdowns in 2020. Outside these episodes, Brent crude oil's net volatility spillovers are largely small and fluctuate close zero.
This study examines EAC connectedness dynamics pre and during the COVID-19 pandemic using the time-frequency domain approaches of Diebold & Yilmaz (2012) and Baruník & Křehlík (2018) and finds a strong interdependence among the considered EAC markets as indicated by the high values of total return and volatility spillover indices. This result lends further support to the existing evidence on the suitability of the EAC regional economic integration, including the possible eventual establishment of a monetary union. The level of return and volatility connectedness strengthens across the considered EAC markets as the frequency band increases, suggesting that return and volatility shocks are persistent. However, an investigation of the evolution of time varying return and volatility spillovers shows that the considered EAC markets appear to be more sensitive to global shocks. The study contributes to the emergent literature investigating the connectedness in developing financial markets during the COVID-19 pandemic, asserting that external shocks, such as COVID-19, strengthen the return connectedness. Specifically, the study finds that the COVID-19 pandemic had a significant effect on all the considered EAC markets although the magnitude and direction of impact varies across markets and countries. Further the study finds that Brent Crude oil prices are a significant source of return and volatility spillovers to EAC markets especially during crisis periods. Different trade-offs call for context-specific strategies. It explores how these cost-benefit considerations vary across countries at different income levels.
The study's findings have considerable policy relevance for policymakers, investors and industry experts. The study's findings may augment investors' decision making regarding portfolio diversification and hedging strategies at different investment horizons through an understanding the intensity and frequency range of return and volatility spillovers, while policymakers, can foster market stability through the identification and close monitoring of major transmitters and receivers of return and volatility spillovers, especially during periods of market turmoil. More importantly, our findings could be of use for future development of EAC regional financial policies and currency markets regulation rules. This study examines EAC connectedness dynamics pre and during the COVID-19 pandemic using the time-frequency domain approaches of Diebold & Yilmaz (2012) and Baruník & Křehlík (2018). However, future research should consider investigating EAC connectedness dynamics using wavelet analysis.
The author declares no conflicts of interest in this paper.
[1] | G. Kirchhoff, Mechanik, Teubner, Leipzig, 1883. |
[2] |
D. Cassani, Z. Liu, C. Tarsi, J. Zhang, Multiplicity of sign-changing solutions for Kirchhoff-type equations, Nonlinear Anal., 186 (2019), 145–161. https://doi.org/10.1016/j.na.2019.01.025 doi: 10.1016/j.na.2019.01.025
![]() |
[3] |
B. Cheng, X. H. Tang, Ground state sign-changing solutions for asymptotically 3-linear Kirchhoff-type problems, Complex Var. Elliptic Equations, 62 (2017), 1093–1116. https://doi.org/10.1080/17476933.2016.1270272 doi: 10.1080/17476933.2016.1270272
![]() |
[4] |
Y. B. Deng, S. J. Peng, W. Shuai, Existence and asymptotic behavior of nodal solutions for the Kirchhoff-type problems in R3, J. Funct. Anal., 269 (2015), 3500–3527. https://doi.org/10.1016/j.jfa.2015.09.012 doi: 10.1016/j.jfa.2015.09.012
![]() |
[5] |
G. M. Figueiredo, J. R. Santos Júnior, Existence of a least energy nodal solution for a Schrödinger-Kirchhoff equation with potential vanishing at infinity, J. Math. Phys., 56 (2015), 051506. https://doi.org/10.1063/1.4921639 doi: 10.1063/1.4921639
![]() |
[6] |
F. Y. Li, C. Gao, X. Zhu, Existence and concentration of sign-changing solutions to Kirchhoff-type system with Hartree-type nonlinearity, J. Math. Anal. Appl., 448 (2017), 60–80. https://doi.org/10.1016/j.jmaa.2016.10.069 doi: 10.1016/j.jmaa.2016.10.069
![]() |
[7] |
Q. Li, X. Du, Z. Zhao, Existence of sign-changing solutions for nonlocal Kirchhoff-Schrödinger-type equations in R3, J. Math. Anal. Appl., 477 (2019), 174–186. https://doi.org/10.1016/j.jmaa.2019.04.025 doi: 10.1016/j.jmaa.2019.04.025
![]() |
[8] |
S. Lu, Signed and sign-changing solutions for a Kirchhoff-type equation in bounded domains, J. Math. Anal. Appl., 432 (2015), 965–982. https://doi.org/10.1016/j.jmaa.2015.07.033 doi: 10.1016/j.jmaa.2015.07.033
![]() |
[9] |
A. M. Mao, S. X. Luan, Sign-changing solutions of a class of nonlocal quasilinear elliptic boundary value problems, J. Math. Anal. Appl., 383 (2011), 239–243. https://doi.org/10.1016/j.jmaa.2011.05.021 doi: 10.1016/j.jmaa.2011.05.021
![]() |
[10] |
A. M. Mao, Z. T. Zhang, Sign-changing and multiple solutions of Kirchhoff type problems without the P.S. condition, Nonlinear Anal., 70 (2009), 1275–1287. https://doi.org/10.1016/j.na.2008.02.011 doi: 10.1016/j.na.2008.02.011
![]() |
[11] |
M. Shao, A. Mao, Signed and sign-changing solutions of Kirchhoff type problems, J. Fixed. Point Theory Appl., 20 (2018), 1–20. https://doi.org/10.1007/s11784-018-0486-9 doi: 10.1007/s11784-018-0486-9
![]() |
[12] |
W. Shuai, Sign-changing solutions for a class of Kirchhoff-type problem in bounded domains, J. Differ. Equations, 259 (2015), 1256–1274. https://doi.org/10.1016/j.jde.2015.02.040 doi: 10.1016/j.jde.2015.02.040
![]() |
[13] |
J. Sun, L. Li, M. Cencelj, B. Gabrovšek, Infinitely many sign-changing solutions for Kirchhoff type problems in R3, Nonlinear Anal., 186 (2019), 33–54. https://doi.org/10.1016/j.na.2018.10.007 doi: 10.1016/j.na.2018.10.007
![]() |
[14] |
X. H. Tang, B. Cheng, Ground state sign-changing solutions for Kirchhoff type problems in bounded domains, J. Differ. Equations, 261 (2016), 2384–2402. https://doi.org/10.1016/j.jde.2016.04.032 doi: 10.1016/j.jde.2016.04.032
![]() |
[15] |
D. B. Wang, Least energy sign-changing solutions of Kirchhoff-type equation with critical growth, J. Math. Phys., 61 (2020), 011501. https://doi.org/10.1063/1.5074163 doi: 10.1063/1.5074163
![]() |
[16] |
L. Wang, B. L. Zhang, K. Cheng, Ground state sign-changing solutions for the Schrödinger-Kirchhoff equation in R3, J. Math. Anal. Appl., 466 (2018), 1545–1569. https://doi.org/10.1016/j.jmaa.2018.06.071 doi: 10.1016/j.jmaa.2018.06.071
![]() |
[17] |
H. Ye, The existence of least energy nodal solutions for some class of Kirchhoff equations and Choquard equations in RN, J. Math. Anal. Appl., 431 (2015), 935–954. https://doi.org/10.1016/j.jmaa.2015.06.012 doi: 10.1016/j.jmaa.2015.06.012
![]() |
[18] |
Z. T. Zhang, K. Perera, Sign changing solutions of Kirchhoff type problems via invariant sets of descentow, J. Math. Anal. Appl., 317 (2006), 456–463. https://doi.org/10.1016/j.jmaa.2005.06.102 doi: 10.1016/j.jmaa.2005.06.102
![]() |
[19] |
X. Zhong, C. L. Tang, The existence and nonexistence results of ground state nodal solutions for a Kirchhoff type problem, Commun. Pure Appl. Anal., 16 (2017), 611–628. https://doi.org/10.3934/cpaa.2017030 doi: 10.3934/cpaa.2017030
![]() |
[20] |
C. O. Alves, M. A. S. Souto, Existence of least energy nodal solution for a Schrödinger-Poisson system in bounded domains, Z. Angew. Math. Phys., 65 (2014), 1153–1166. https://doi.org/10.1007/s00033-013-0376-3 doi: 10.1007/s00033-013-0376-3
![]() |
[21] |
S. Chen, X. Tang, Ground state sign-changing solutions for a class of Schrödinger-Poisson type problems in R3, Z. Angew. Math. Phys., 67 (2016). https://doi.org/10.1007/s00033-016-0695-2 doi: 10.1007/s00033-016-0695-2
![]() |
[22] |
S. Khoutir, Least energy sign-changing solutions for a class of Schrödinger-Poisson system on bounded domains, J. Math. Phys., 62 (2021), 031509. https://doi.org/10.1063/5.0040741 doi: 10.1063/5.0040741
![]() |
[23] |
S. Kim, J. Seok, On nodal solutions of the nonlinear Schrödinger-Poisson equations, Commun. Contemp. Math., 14 (2012), 1250041. https://doi.org/10.1142/S0219199712500411 doi: 10.1142/S0219199712500411
![]() |
[24] |
Z. Liang, J. Xu, X. Zhu, Revisit to sign-changing solutions for the nonlinear Schrödinger-Poisson system in R3, J. Math. Anal. Appl., 435 (2016), 783–799. https://doi.org/10.1016/j.jmaa.2015.10.076 doi: 10.1016/j.jmaa.2015.10.076
![]() |
[25] |
Z. Liu, Z. Wang, J. Zhang, Infinitely many sign-changing solutions for the nonlinear Schrödinger-Poisson system, Ann. Mat. Pura Appl., 4 (2016), 775–794. https://doi.org/10.1007/s10231-015-0489-8 doi: 10.1007/s10231-015-0489-8
![]() |
[26] |
W. Shuai, Q. Wang, Existence and asymptotic behavior of sign-changing solutions for the nonlinear Schrödinger-Poisson system in R3, Z. Angew. Math. Phys., 66 (2015), 3267–3282. https://doi.org/10.1007/s00033-015-0571-5 doi: 10.1007/s00033-015-0571-5
![]() |
[27] |
D. B. Wang, H. Zhang, W. Guan, Existence of least-energy sign-changing solutions for Schrödinger-Poisson system with critical growth, J. Math. Anal. Appl., 479 (2019), 2284–2301. https://doi.org/10.1016/j.jmaa.2019.07.052 doi: 10.1016/j.jmaa.2019.07.052
![]() |
[28] |
Z. Wang, H. Zhou, Sign-changing solutions for the nonlinear Schrödinger-Poisson system in R3, Calc. Var. Partial Differ. Equations, 52 (2015), 927–943. https://doi.org/10.1007/s00526-014-0738-5 doi: 10.1007/s00526-014-0738-5
![]() |
[29] |
X. Zhong, C. L. Tang, Ground state sign-changing solutions for a Schrödinger-Poisson system with a critical nonlinearity in R3, Nonlinear Anal. Real World Appl., 39 (2018), 166–184. https://doi.org/10.1016/j.nonrwa.2017.06.014 doi: 10.1016/j.nonrwa.2017.06.014
![]() |
[30] |
V. Bobkov, Least energy nodal solutions for elliptic equations with indefinite nonlinearity, Electron. J. Qual. Theory Differ. Equations, 2014 (2014), 1–15. https://doi.org/10.14232/ejqtde.2014.1.56 doi: 10.14232/ejqtde.2014.1.56
![]() |
[31] |
D. Ruiz, The Schrödinger-Poisson equation under the effect of a nonlinear local term, J. Funct. Anal., 237 (2006), 655–674. https://doi.org/10.1016/j.jfa.2006.04.005 doi: 10.1016/j.jfa.2006.04.005
![]() |
[32] | M. Willem, Minimax Theorems, Birkhäuser, Bosten, 1996. |
1. | Élise Fressart, Barbara Verfürth, Wave Propagation in High-Contrast Media: Periodic and Beyond, 2024, 24, 1609-4840, 345, 10.1515/cmam-2023-0066 | |
2. | Tim Keil, Mario Ohlberger, A relaxed localized trust-region reduced basis approach for optimization of multiscale problems, 2024, 58, 2822-7840, 79, 10.1051/m2an/2023089 | |
3. | Barbara Verfürth, Numerical Multiscale Methods for Waves in High-Contrast Media, 2024, 126, 0012-0456, 37, 10.1365/s13291-023-00273-z |
Variable | Description | Mean | Maximum | Minimum | Std. Dev. | Observations |
Interbank Kenya | Overall Interbank Interest Rate Kenya | 6.755728 | 28.9 | 0.98 | 4.026051 | 206 |
Interbank Uganda | Overall Interbank Interest Rate Uganda | 9.164757 | 26.68 | 2.65 | 3.971696 | 206 |
Interbank Tanzania | Overall Interbank Interest Rate Tanzania | 6.99466 | 29.72 | 0.87 | 4.738687 | 206 |
KSHS/USD | Natural Log of Nominal Average Kenya Shilling per US dollar Exchange rate (KSHS/USD) | 4.475495 | 4.730754 | 4.125508 | 0.159064 | 206 |
UGX/USD | Natural Log of Average Nominal Uganda Shilling per US dollar Exchange rate (UGX/USD) | 7.87166 | 8.253352 | 7.378221 | 0.293194 | 206 |
TZS/USD | Natural Log of Average Nominal Tanzania Shilling per US dollar Exchange rate (TZS/USD) | 7.424048 | 7.740391 | 6.956364 | 0.259825 | 206 |
Brent | Natural Log of Crude oil (Brent) | 4.24742 | 4.896892 | 3.150169 | 0.344239 | 206 |
Variables | Augmented Dicky Fuller (ADF) test | Phillips-Peron (PP) test | Kwiatkowski-Phillips-Schmidt-Shin | |||
Level | First Difference | Level | First Difference | Level | First Difference | |
Overall Interbank Interest Rate Kenya | −3.965 | −4.042 | 0.199 | |||
Overall Interbank Interest Rate Uganda | −4.185 | −3.124 | 0.173 | |||
Overall Interbank Interest Rate Tanzania | −3.242 | −5.473 | 0.167 | |||
Natural Log of Nominal Average Kenya Shilling per US dollar Exchange rate (KSHS/USD) | −0.472 | −9.979 | −0.437 | −10.051 | 1.667 | 0.118 |
Natural Log of Average Nominal Uganda Shilling per US dollar Exchange rate (UGX/USD) | −1.163 | −9.260 | −0.974 | −8.695 | 1.736 | 0.162 |
Natural Log of Average Nominal Tanzania Shilling per US dollar Exchange rate (TZS/USD) | −1.092 | −9.922 | −1.387 | −9.966 | 1.767 | 0.150 |
Natural Log of Crude oil (Brent) | −3.171 | −2.678 | −9.074 | 0.340 | ||
Asymptotic critical values | ||||||
Significance level | ADF | PP | KPSS | |||
1% | −3.462 | −3.462 | 0.739 | |||
5% | −2.875 | −2.875 | 0.463 | |||
10% | −2.574 | −2.574 | 0.347 |
Interbank Kenya | Interbank Uganda | Interbank Tanzania | KSHS/USD | UGX/USD | TZS/USD | Brent | FROM | |
Interbank Kenya | 45.45 | 23.66 | 3.05 | 3.65 | 2.4 | 5.14 | 16.65 | 7.79 |
Interbank Uganda | 14.45 | 61.96 | 5.02 | 1.91 | 2.15 | 4.87 | 9.65 | 5.43 |
Interbank Tanzania | 12.38 | 21.76 | 48.79 | 1.13 | 2.85 | 8.52 | 4.57 | 7.32 |
KSHS/USD | 3.77 | 2.88 | 0.47 | 68.97 | 16.94 | 2.93 | 4.04 | 4.43 |
UGX/USD | 0.79 | 5.11 | 1.66 | 14.19 | 66.13 | 8.24 | 3.88 | 4.84 |
TZS/USD | 1.42 | 2.31 | 3.39 | 7.03 | 8.8 | 75.51 | 1.53 | 3.5 |
Brent | 0.04 | 2.5 | 2.6 | 14.4 | 10.87 | 5.57 | 64.02 | 5.14 |
TO | 4.69 | 8.32 | 2.31 | 6.04 | 6.29 | 5.04 | 5.76 | 38.45 |
NET | −3.10 | 2.88 | −5.00 | 1.61 | 1.45 | 1.54 | 0.62 | |
PANEL B | Interbank Kenya | Interbank Uganda | Interbank Tanzania | KSHS/USD | UGX/USD | TZS/USD | Brent | FROM |
Interbank Kenya | 28.29 | 40.59 | 2.41 | 9.57 | 12.46 | 6.54 | 0.14 | 10.24 |
Interbank Uganda | 7.45 | 57.57 | 3.23 | 13.17 | 14.11 | 4.16 | 0.3 | 6.06 |
Interbank Tanzania | 10.81 | 25.32 | 33.66 | 5.57 | 9.99 | 14.34 | 0.32 | 9.48 |
KSHS/USD | 5.71 | 18.82 | 1.92 | 58.1 | 6.06 | 8.85 | 0.53 | 5.99 |
UGX/USD | 3.57 | 6.4 | 0.57 | 19.31 | 49.63 | 19.8 | 0.71 | 7.2 |
TZS/USD | 0.88 | 0.73 | 1.62 | 11.37 | 6.66 | 76.1 | 2.63 | 3.41 |
Brent | 0.45 | 3.02 | 0.37 | 5.83 | 0.52 | 2.9 | 86.91 | 1.87 |
TO | 4.13 | 13.55 | 1.45 | 9.26 | 7.11 | 8.08 | 0.66 | 44.25 |
NET | −6.12 | 7.49 | −8.03 | 3.28 | −0.08 | 4.67 | −1.21 |
Time Based Pairwise Spillovers | Frequency Based Pairwise Spillovers | |||||||
return | volatility | return1 | return2 | return3 | volatility1 | volatility2 | volatility3 | |
Interbank Kenya-Interbank Uganda | 1.32 | 4.73 | 0.01 | 0.06 | 1.25 | 0.00 | 0.25 | 4.49 |
Interbank Kenya-Interbank Tanzania | −1.33 | −1.20 | −0.02 | −0.07 | −1.24 | −0.93 | −0.07 | −0.19 |
Interbank Kenya-KSHS/USD | −0.02 | 0.55 | 0.02 | −0.04 | 0.00 | −0.51 | −0.13 | 1.18 |
Interbank Kenya-UGX/USD | 0.23 | 1.27 | −0.01 | 0.01 | 0.23 | 0.05 | −0.07 | 1.29 |
Interbank Kenya-TZS/USD | 0.53 | 0.81 | −0.06 | 0.01 | 0.59 | 0.04 | 0.13 | 0.64 |
Interbank Kenya-Brent | 2.37 | −0.04 | 0.00 | 0.00 | 2.37 | −0.01 | −0.01 | −0.02 |
Interbank Uganda-Interbank Tanzania | −2.39 | −3.16 | −0.05 | −0.08 | −2.26 | −0.05 | −0.01 | −3.09 |
Interbank Uganda-KSHS/USD | −0.14 | −0.81 | −0.04 | −0.08 | −0.03 | −0.14 | −0.48 | −0.19 |
Interbank Uganda-UGX/USD | −0.42 | 1.10 | −0.20 | −0.10 | −0.12 | 0.03 | −0.04 | 1.11 |
Interbank Uganda-TZS/USD | 0.37 | 0.49 | −0.10 | −0.03 | 0.50 | 0.00 | 0.01 | 0.48 |
Interbank Uganda-Brent | 1.02 | −0.39 | 0.00 | 0.00 | 1.02 | 0.00 | −0.07 | −0.32 |
Interbank Tanzania-KSHS/USD | 0.09 | 0.52 | 0.02 | 0.02 | 0.06 | 0.00 | −0.02 | 0.54 |
Interbank Tanzania-UGX/USD | 0.17 | 1.35 | −0.06 | −0.03 | 0.26 | 0.39 | 0.06 | 0.90 |
Interbank Tanzania-TZS/USD | 0.73 | 1.82 | 0.09 | 0.07 | 0.57 | 0.10 | 0.46 | 1.26 |
Interbank Tanzania-Brent | 0.28 | −0.01 | 0.01 | 0.01 | 0.26 | 0.00 | 0.01 | −0.02 |
KSHS/USD-UGX/USD | 0.39 | −1.89 | 0.10 | 0.20 | 0.09 | 0.43 | 0.06 | −2.39 |
KSHS/USD-TZS/USD | −0.59 | −0.36 | −0.05 | −0.17 | −0.37 | 0.20 | −0.07 | −0.49 |
KSHS/USD-Brent | −1.48 | −0.76 | 0.26 | 0.10 | −1.84 | 0.02 | −0.03 | −0.75 |
UGX/USD-TZS/USD | −0.08 | 1.88 | −0.04 | 0.00 | −0.04 | −0.04 | 0.13 | 1.78 |
UGX/USD-Brent | −1.00 | 0.03 | 0.22 | 0.12 | −1.34 | 0.01 | 0.01 | 0.01 |
TZS/USD-Brent | −0.58 | −0.04 | 0.06 | 0.05 | −0.69 | 0.00 | −0.03 | −0.01 |
Notes: The net pairwise spillovers is the difference between the spillovers from i to j and the spillovers from j to i, whereby when the value is positive variable i transmits more spillovers to variable j, whereas when it is negative variable j send more spillovers variable i. |
1–3 months | Interbank Kenya | Interbank Uganda | Interbank Tanzania | KSHS/USD | UGX/USD | TZS/USD | Brent | FROM_ABS | FROM_WTH |
Interbank Kenya | 6.81 | 0.21 | 0.04 | 0.33 | 0.02 | 0.08 | 0.00 | 0.10 | 0.45 |
Interbank Uganda | 0.17 | 4.75 | 0.48 | 0.11 | 0.24 | 0.09 | 0.03 | 0.16 | 0.72 |
Interbank Tanzania | 0.19 | 0.86 | 12.51 | 0.14 | 0.22 | 1.80 | 0.09 | 0.47 | 2.14 |
KSHS/USD | 0.22 | 0.36 | 0.02 | 34.39 | 5.20 | 0.81 | 1.89 | 1.22 | 5.52 |
UGX/USD | 0.08 | 1.67 | 0.64 | 4.47 | 28.74 | 2.19 | 1.61 | 1.53 | 6.92 |
TZS/USD | 0.52 | 0.78 | 1.15 | 1.15 | 2.47 | 34.37 | 0.43 | 0.93 | 4.22 |
Brent | 0.00 | 0.00 | 0.01 | 0.09 | 0.07 | 0.02 | 1.67 | 0.03 | 0.13 |
TO_ABS | 0.17 | 0.55 | 0.34 | 0.90 | 1.18 | 0.71 | 0.58 | 4.43 | |
TO_WTH | 0.77 | 2.52 | 1.52 | 4.08 | 5.34 | 3.24 | 2.63 | 20.10 | |
NET | 0.07 | 0.40 | −0.14 | −0.32 | −0.35 | −0.22 | 0.55 | ||
3–6 months | Interbank Kenya | Interbank Uganda | Interbank Tanzania | KSHS/USD | UGX/USD | TZS/USD | Brent | FROM_ABS | FROM_WTH |
Interbank Kenya | 5.57 | 0.83 | 0.21 | 0.19 | 0.15 | 0.38 | 0.02 | 0.25 | 1.82 |
Interbank Uganda | 0.41 | 4.59 | 0.39 | 0.02 | 0.15 | 0.24 | 0.02 | 0.18 | 1.26 |
Interbank Tanzania | 0.70 | 0.91 | 8.66 | 0.14 | 0.16 | 1.16 | 0.11 | 0.45 | 3.27 |
KSHS/USD | 0.44 | 0.55 | 0.02 | 15.60 | 4.90 | 0.83 | 1.03 | 1.11 | 8.00 |
UGX/USD | 0.08 | 0.87 | 0.37 | 3.53 | 14.84 | 2.18 | 1.00 | 1.15 | 8.26 |
TZS/USD | 0.33 | 0.46 | 0.64 | 2.04 | 2.16 | 17.19 | 0.44 | 0.87 | 6.26 |
Brent | 0.00 | 0.02 | 0.03 | 0.32 | 0.19 | 0.07 | 2.04 | 0.09 | 0.65 |
TO_ABS | 0.28 | 0.52 | 0.24 | 0.89 | 1.10 | 0.69 | 0.38 | 4.10 | |
TO_WTH | 2.02 | 3.74 | 1.72 | 6.42 | 7.92 | 5.00 | 2.71 | 29.53 | |
NET | 0.03 | 0.34 | −0.22 | −0.22 | −0.05 | −0.17 | 0.29 | ||
Beyond 6 months | Interbank Kenya | Interbank Uganda | Interbank Tanzania | KSHS/USD | UGX/USD | TZS/USD | Brent | FROM_ABS | FROM_WTH |
Interbank Kenya | 33.07 | 22.62 | 2.80 | 3.13 | 2.23 | 4.68 | 16.62 | 7.44 | 11.61 |
Interbank Uganda | 13.87 | 52.62 | 4.15 | 1.78 | 1.75 | 4.55 | 9.60 | 5.10 | 7.96 |
Interbank Tanzania | 11.50 | 19.99 | 27.63 | 0.84 | 2.47 | 5.56 | 4.37 | 6.39 | 9.97 |
KSHS/USD | 3.11 | 1.98 | 0.43 | 18.99 | 6.84 | 1.29 | 1.11 | 2.11 | 3.29 |
UGX/USD | 0.63 | 2.57 | 0.65 | 6.18 | 22.54 | 3.87 | 1.27 | 2.17 | 3.38 |
TZS/USD | 0.57 | 1.06 | 1.60 | 3.84 | 4.16 | 23.96 | 0.65 | 1.70 | 2.65 |
Brent | 0.04 | 2.48 | 2.55 | 13.98 | 10.62 | 5.47 | 60.31 | 5.02 | 7.84 |
TO_ABS | 4.24 | 7.24 | 1.74 | 4.25 | 4.01 | 3.63 | 4.80 | 29.92 | |
TO_WTH | 6.62 | 11.30 | 2.72 | 6.64 | 6.26 | 5.67 | 7.50 | 46.70 | |
NET | −3.20 | 2.14 | −4.65 | 2.15 | 1.84 | 1.93 | −0.22 | ||
Notes: FROM_WTH and TO_WTH capture 'within' connectedness which refers to the connectedness effect that occurs within the frequency band and is weighted by the power of the series on the given frequency band exclusively while FROM_ABS and TO_ABS refer to the frequency connectedness decomposition of the original Diebold & Yilmaz (2012) connectedness measures into distinct parts that when summed provide the original connectedness measures. Hence FROM ABS is the measure of frequency connectedness in absolute sense while FROM WTH is the measure of 'within' connectedness. |
1–3 months | Interbank Kenya | Interbank Uganda | Interbank Tanzania | KSHS/USD | UGX/USD | TZS/USD | Brent | FROM_ABS | FROM_WTH | ||||||||||
Interbank Kenya | 13.61 | 0.86 | 0.47 | 0.41 | 1.95 | 0.40 | 0.01 | 0.59 | 4.07 | ||||||||||
Interbank Uganda | 0.88 | 1.30 | 0.34 | 0.20 | 0.26 | 0.02 | 0.00 | 0.24 | 1.70 | ||||||||||
Interbank Tanzania | 7.01 | 0.72 | 10.32 | 0.26 | 2.76 | 0.71 | 0.02 | 1.64 | 11.39 | ||||||||||
KSHS/USD | 3.94 | 1.18 | 0.25 | 17.01 | 4.09 | 1.82 | 0.17 | 1.64 | 11.37 | ||||||||||
UGX/USD | 1.58 | 0.07 | 0.02 | 1.06 | 8.93 | 0.39 | 0.12 | 0.46 | 3.20 | ||||||||||
TZS/USD | 0.12 | 0.01 | 0.04 | 0.41 | 0.63 | 7.27 | 0.01 | 0.17 | 1.22 | ||||||||||
Brent | 0.09 | 0.04 | 0.01 | 0.06 | 0.07 | 0.04 | 8.81 | 0.04 | 0.30 | ||||||||||
TO_ABS | 1.95 | 0.41 | 0.16 | 0.34 | 1.40 | 0.48 | 0.05 | 4.78 | |||||||||||
TO_WTH | 13.52 | 2.86 | 1.12 | 2.37 | 9.69 | 3.35 | 0.33 | 33.24 | |||||||||||
NET | 1.36 | 0.17 | −1.48 | −1.30 | 0.93 | 0.31 | 0.01 | ||||||||||||
3–6 months | Interbank Kenya | Interbank Uganda | Interbank Tanzania | KSHS/USD | UGX/USD | TZS/USD | Brent | FROM_ABS | FROM_WTH | ||||||||||
Interbank Kenya | 4.39 | 2.68 | 0.66 | 0.08 | 0.25 | 0.97 | 0.01 | 0.66 | 5.19 | ||||||||||
Interbank Uganda | 0.96 | 3.39 | 0.72 | 0.19 | 0.09 | 0.12 | 0.01 | 0.30 | 2.32 | ||||||||||
Interbank Tanzania | 1.18 | 0.80 | 8.72 | 0.05 | 0.44 | 3.62 | 0.13 | 0.89 | 6.94 | ||||||||||
KSHS/USD | 0.96 | 3.56 | 0.17 | 7.99 | 1.45 | 0.50 | 0.16 | 0.97 | 7.60 | ||||||||||
UGX/USD | 0.77 | 0.34 | 0.05 | 1.02 | 6.02 | 1.23 | 0.23 | 0.52 | 4.08 | ||||||||||
TZS/USD | 0.09 | 0.03 | 0.39 | 1.01 | 0.31 | 10.97 | 0.18 | 0.29 | 2.24 | ||||||||||
Brent | 0.11 | 0.48 | 0.09 | 0.34 | 0.15 | 0.37 | 21.07 | 0.22 | 1.72 | ||||||||||
TO_ABS | 0.58 | 1.13 | 0.30 | 0.38 | 0.38 | 0.97 | 0.10 | 3.85 | |||||||||||
TO_WTH | 4.54 | 8.81 | 2.32 | 3.01 | 2.99 | 7.62 | 0.80 | 30.10 | |||||||||||
NET | −0.08 | 0.83 | −0.59 | −0.59 | −0.14 | 0.69 | −0.12 | ||||||||||||
Beyond 6 months | Interbank Kenya | Interbank Uganda | Interbank Tanzania | KSHS/USD | UGX/USD | TZS/USD | Brent | FROM_ABS | FROM_WTH | ||||||||||
Interbank Kenya | 10.28 | 37.06 | 1.28 | 9.09 | 10.26 | 5.17 | 0.12 | 9.00 | 12.35 | ||||||||||
Interbank Uganda | 5.62 | 52.88 | 2.17 | 12.77 | 13.77 | 4.02 | 0.30 | 5.52 | 7.58 | ||||||||||
Interbank Tanzania | 2.62 | 23.80 | 14.63 | 5.27 | 6.78 | 10.01 | 0.17 | 6.95 | 9.54 | ||||||||||
KSHS/USD | 0.81 | 14.08 | 1.49 | 33.10 | 0.53 | 6.53 | 0.20 | 3.38 | 4.64 | ||||||||||
UGX/USD | 1.23 | 5.98 | 0.50 | 17.24 | 34.69 | 18.18 | 0.36 | 6.21 | 8.53 | ||||||||||
TZS/USD | 0.67 | 0.69 | 1.19 | 9.95 | 5.72 | 57.87 | 2.44 | 2.95 | 4.05 | ||||||||||
Brent | 0.26 | 2.50 | 0.28 | 5.43 | 0.30 | 2.49 | 57.03 | 1.61 | 2.21 | ||||||||||
TO_ABS | 1.60 | 12.02 | 0.99 | 8.53 | 5.34 | 6.63 | 0.51 | 35.62 | |||||||||||
TO_WTH | 2.20 | 16.50 | 1.36 | 11.72 | 7.33 | 9.10 | 0.70 | 48.90 | |||||||||||
NET | −7.39 | 6.49 | −5.96 | 5.16 | −0.88 | 3.68 | −1.10 | ||||||||||||
Notes: FROM_WTH and TO_WTH capture 'within' connectedness which refers to the connectedness effect that occurs within the frequency band and is weighted by the power of the series on the given frequency band exclusively while FROM_ABS and TO_ABS refer to the frequency connectedness decomposition of the original Diebold & Yilmaz (2012) connectedness measures into distinct parts that when summed provide the original connectedness measures. Hence FROM ABS is the measure of frequency connectedness in absolute sense while FROM WTH is the measure of 'within' connectedness. |
Variable | Description | Mean | Maximum | Minimum | Std. Dev. | Observations |
Interbank Kenya | Overall Interbank Interest Rate Kenya | 6.755728 | 28.9 | 0.98 | 4.026051 | 206 |
Interbank Uganda | Overall Interbank Interest Rate Uganda | 9.164757 | 26.68 | 2.65 | 3.971696 | 206 |
Interbank Tanzania | Overall Interbank Interest Rate Tanzania | 6.99466 | 29.72 | 0.87 | 4.738687 | 206 |
KSHS/USD | Natural Log of Nominal Average Kenya Shilling per US dollar Exchange rate (KSHS/USD) | 4.475495 | 4.730754 | 4.125508 | 0.159064 | 206 |
UGX/USD | Natural Log of Average Nominal Uganda Shilling per US dollar Exchange rate (UGX/USD) | 7.87166 | 8.253352 | 7.378221 | 0.293194 | 206 |
TZS/USD | Natural Log of Average Nominal Tanzania Shilling per US dollar Exchange rate (TZS/USD) | 7.424048 | 7.740391 | 6.956364 | 0.259825 | 206 |
Brent | Natural Log of Crude oil (Brent) | 4.24742 | 4.896892 | 3.150169 | 0.344239 | 206 |
Variables | Augmented Dicky Fuller (ADF) test | Phillips-Peron (PP) test | Kwiatkowski-Phillips-Schmidt-Shin | |||
Level | First Difference | Level | First Difference | Level | First Difference | |
Overall Interbank Interest Rate Kenya | −3.965 | −4.042 | 0.199 | |||
Overall Interbank Interest Rate Uganda | −4.185 | −3.124 | 0.173 | |||
Overall Interbank Interest Rate Tanzania | −3.242 | −5.473 | 0.167 | |||
Natural Log of Nominal Average Kenya Shilling per US dollar Exchange rate (KSHS/USD) | −0.472 | −9.979 | −0.437 | −10.051 | 1.667 | 0.118 |
Natural Log of Average Nominal Uganda Shilling per US dollar Exchange rate (UGX/USD) | −1.163 | −9.260 | −0.974 | −8.695 | 1.736 | 0.162 |
Natural Log of Average Nominal Tanzania Shilling per US dollar Exchange rate (TZS/USD) | −1.092 | −9.922 | −1.387 | −9.966 | 1.767 | 0.150 |
Natural Log of Crude oil (Brent) | −3.171 | −2.678 | −9.074 | 0.340 | ||
Asymptotic critical values | ||||||
Significance level | ADF | PP | KPSS | |||
1% | −3.462 | −3.462 | 0.739 | |||
5% | −2.875 | −2.875 | 0.463 | |||
10% | −2.574 | −2.574 | 0.347 |
Interbank Kenya | Interbank Uganda | Interbank Tanzania | KSHS/USD | UGX/USD | TZS/USD | Brent | FROM | |
Interbank Kenya | 45.45 | 23.66 | 3.05 | 3.65 | 2.4 | 5.14 | 16.65 | 7.79 |
Interbank Uganda | 14.45 | 61.96 | 5.02 | 1.91 | 2.15 | 4.87 | 9.65 | 5.43 |
Interbank Tanzania | 12.38 | 21.76 | 48.79 | 1.13 | 2.85 | 8.52 | 4.57 | 7.32 |
KSHS/USD | 3.77 | 2.88 | 0.47 | 68.97 | 16.94 | 2.93 | 4.04 | 4.43 |
UGX/USD | 0.79 | 5.11 | 1.66 | 14.19 | 66.13 | 8.24 | 3.88 | 4.84 |
TZS/USD | 1.42 | 2.31 | 3.39 | 7.03 | 8.8 | 75.51 | 1.53 | 3.5 |
Brent | 0.04 | 2.5 | 2.6 | 14.4 | 10.87 | 5.57 | 64.02 | 5.14 |
TO | 4.69 | 8.32 | 2.31 | 6.04 | 6.29 | 5.04 | 5.76 | 38.45 |
NET | −3.10 | 2.88 | −5.00 | 1.61 | 1.45 | 1.54 | 0.62 | |
PANEL B | Interbank Kenya | Interbank Uganda | Interbank Tanzania | KSHS/USD | UGX/USD | TZS/USD | Brent | FROM |
Interbank Kenya | 28.29 | 40.59 | 2.41 | 9.57 | 12.46 | 6.54 | 0.14 | 10.24 |
Interbank Uganda | 7.45 | 57.57 | 3.23 | 13.17 | 14.11 | 4.16 | 0.3 | 6.06 |
Interbank Tanzania | 10.81 | 25.32 | 33.66 | 5.57 | 9.99 | 14.34 | 0.32 | 9.48 |
KSHS/USD | 5.71 | 18.82 | 1.92 | 58.1 | 6.06 | 8.85 | 0.53 | 5.99 |
UGX/USD | 3.57 | 6.4 | 0.57 | 19.31 | 49.63 | 19.8 | 0.71 | 7.2 |
TZS/USD | 0.88 | 0.73 | 1.62 | 11.37 | 6.66 | 76.1 | 2.63 | 3.41 |
Brent | 0.45 | 3.02 | 0.37 | 5.83 | 0.52 | 2.9 | 86.91 | 1.87 |
TO | 4.13 | 13.55 | 1.45 | 9.26 | 7.11 | 8.08 | 0.66 | 44.25 |
NET | −6.12 | 7.49 | −8.03 | 3.28 | −0.08 | 4.67 | −1.21 |
Time Based Pairwise Spillovers | Frequency Based Pairwise Spillovers | |||||||
return | volatility | return1 | return2 | return3 | volatility1 | volatility2 | volatility3 | |
Interbank Kenya-Interbank Uganda | 1.32 | 4.73 | 0.01 | 0.06 | 1.25 | 0.00 | 0.25 | 4.49 |
Interbank Kenya-Interbank Tanzania | −1.33 | −1.20 | −0.02 | −0.07 | −1.24 | −0.93 | −0.07 | −0.19 |
Interbank Kenya-KSHS/USD | −0.02 | 0.55 | 0.02 | −0.04 | 0.00 | −0.51 | −0.13 | 1.18 |
Interbank Kenya-UGX/USD | 0.23 | 1.27 | −0.01 | 0.01 | 0.23 | 0.05 | −0.07 | 1.29 |
Interbank Kenya-TZS/USD | 0.53 | 0.81 | −0.06 | 0.01 | 0.59 | 0.04 | 0.13 | 0.64 |
Interbank Kenya-Brent | 2.37 | −0.04 | 0.00 | 0.00 | 2.37 | −0.01 | −0.01 | −0.02 |
Interbank Uganda-Interbank Tanzania | −2.39 | −3.16 | −0.05 | −0.08 | −2.26 | −0.05 | −0.01 | −3.09 |
Interbank Uganda-KSHS/USD | −0.14 | −0.81 | −0.04 | −0.08 | −0.03 | −0.14 | −0.48 | −0.19 |
Interbank Uganda-UGX/USD | −0.42 | 1.10 | −0.20 | −0.10 | −0.12 | 0.03 | −0.04 | 1.11 |
Interbank Uganda-TZS/USD | 0.37 | 0.49 | −0.10 | −0.03 | 0.50 | 0.00 | 0.01 | 0.48 |
Interbank Uganda-Brent | 1.02 | −0.39 | 0.00 | 0.00 | 1.02 | 0.00 | −0.07 | −0.32 |
Interbank Tanzania-KSHS/USD | 0.09 | 0.52 | 0.02 | 0.02 | 0.06 | 0.00 | −0.02 | 0.54 |
Interbank Tanzania-UGX/USD | 0.17 | 1.35 | −0.06 | −0.03 | 0.26 | 0.39 | 0.06 | 0.90 |
Interbank Tanzania-TZS/USD | 0.73 | 1.82 | 0.09 | 0.07 | 0.57 | 0.10 | 0.46 | 1.26 |
Interbank Tanzania-Brent | 0.28 | −0.01 | 0.01 | 0.01 | 0.26 | 0.00 | 0.01 | −0.02 |
KSHS/USD-UGX/USD | 0.39 | −1.89 | 0.10 | 0.20 | 0.09 | 0.43 | 0.06 | −2.39 |
KSHS/USD-TZS/USD | −0.59 | −0.36 | −0.05 | −0.17 | −0.37 | 0.20 | −0.07 | −0.49 |
KSHS/USD-Brent | −1.48 | −0.76 | 0.26 | 0.10 | −1.84 | 0.02 | −0.03 | −0.75 |
UGX/USD-TZS/USD | −0.08 | 1.88 | −0.04 | 0.00 | −0.04 | −0.04 | 0.13 | 1.78 |
UGX/USD-Brent | −1.00 | 0.03 | 0.22 | 0.12 | −1.34 | 0.01 | 0.01 | 0.01 |
TZS/USD-Brent | −0.58 | −0.04 | 0.06 | 0.05 | −0.69 | 0.00 | −0.03 | −0.01 |
Notes: The net pairwise spillovers is the difference between the spillovers from i to j and the spillovers from j to i, whereby when the value is positive variable i transmits more spillovers to variable j, whereas when it is negative variable j send more spillovers variable i. |
1–3 months | Interbank Kenya | Interbank Uganda | Interbank Tanzania | KSHS/USD | UGX/USD | TZS/USD | Brent | FROM_ABS | FROM_WTH |
Interbank Kenya | 6.81 | 0.21 | 0.04 | 0.33 | 0.02 | 0.08 | 0.00 | 0.10 | 0.45 |
Interbank Uganda | 0.17 | 4.75 | 0.48 | 0.11 | 0.24 | 0.09 | 0.03 | 0.16 | 0.72 |
Interbank Tanzania | 0.19 | 0.86 | 12.51 | 0.14 | 0.22 | 1.80 | 0.09 | 0.47 | 2.14 |
KSHS/USD | 0.22 | 0.36 | 0.02 | 34.39 | 5.20 | 0.81 | 1.89 | 1.22 | 5.52 |
UGX/USD | 0.08 | 1.67 | 0.64 | 4.47 | 28.74 | 2.19 | 1.61 | 1.53 | 6.92 |
TZS/USD | 0.52 | 0.78 | 1.15 | 1.15 | 2.47 | 34.37 | 0.43 | 0.93 | 4.22 |
Brent | 0.00 | 0.00 | 0.01 | 0.09 | 0.07 | 0.02 | 1.67 | 0.03 | 0.13 |
TO_ABS | 0.17 | 0.55 | 0.34 | 0.90 | 1.18 | 0.71 | 0.58 | 4.43 | |
TO_WTH | 0.77 | 2.52 | 1.52 | 4.08 | 5.34 | 3.24 | 2.63 | 20.10 | |
NET | 0.07 | 0.40 | −0.14 | −0.32 | −0.35 | −0.22 | 0.55 | ||
3–6 months | Interbank Kenya | Interbank Uganda | Interbank Tanzania | KSHS/USD | UGX/USD | TZS/USD | Brent | FROM_ABS | FROM_WTH |
Interbank Kenya | 5.57 | 0.83 | 0.21 | 0.19 | 0.15 | 0.38 | 0.02 | 0.25 | 1.82 |
Interbank Uganda | 0.41 | 4.59 | 0.39 | 0.02 | 0.15 | 0.24 | 0.02 | 0.18 | 1.26 |
Interbank Tanzania | 0.70 | 0.91 | 8.66 | 0.14 | 0.16 | 1.16 | 0.11 | 0.45 | 3.27 |
KSHS/USD | 0.44 | 0.55 | 0.02 | 15.60 | 4.90 | 0.83 | 1.03 | 1.11 | 8.00 |
UGX/USD | 0.08 | 0.87 | 0.37 | 3.53 | 14.84 | 2.18 | 1.00 | 1.15 | 8.26 |
TZS/USD | 0.33 | 0.46 | 0.64 | 2.04 | 2.16 | 17.19 | 0.44 | 0.87 | 6.26 |
Brent | 0.00 | 0.02 | 0.03 | 0.32 | 0.19 | 0.07 | 2.04 | 0.09 | 0.65 |
TO_ABS | 0.28 | 0.52 | 0.24 | 0.89 | 1.10 | 0.69 | 0.38 | 4.10 | |
TO_WTH | 2.02 | 3.74 | 1.72 | 6.42 | 7.92 | 5.00 | 2.71 | 29.53 | |
NET | 0.03 | 0.34 | −0.22 | −0.22 | −0.05 | −0.17 | 0.29 | ||
Beyond 6 months | Interbank Kenya | Interbank Uganda | Interbank Tanzania | KSHS/USD | UGX/USD | TZS/USD | Brent | FROM_ABS | FROM_WTH |
Interbank Kenya | 33.07 | 22.62 | 2.80 | 3.13 | 2.23 | 4.68 | 16.62 | 7.44 | 11.61 |
Interbank Uganda | 13.87 | 52.62 | 4.15 | 1.78 | 1.75 | 4.55 | 9.60 | 5.10 | 7.96 |
Interbank Tanzania | 11.50 | 19.99 | 27.63 | 0.84 | 2.47 | 5.56 | 4.37 | 6.39 | 9.97 |
KSHS/USD | 3.11 | 1.98 | 0.43 | 18.99 | 6.84 | 1.29 | 1.11 | 2.11 | 3.29 |
UGX/USD | 0.63 | 2.57 | 0.65 | 6.18 | 22.54 | 3.87 | 1.27 | 2.17 | 3.38 |
TZS/USD | 0.57 | 1.06 | 1.60 | 3.84 | 4.16 | 23.96 | 0.65 | 1.70 | 2.65 |
Brent | 0.04 | 2.48 | 2.55 | 13.98 | 10.62 | 5.47 | 60.31 | 5.02 | 7.84 |
TO_ABS | 4.24 | 7.24 | 1.74 | 4.25 | 4.01 | 3.63 | 4.80 | 29.92 | |
TO_WTH | 6.62 | 11.30 | 2.72 | 6.64 | 6.26 | 5.67 | 7.50 | 46.70 | |
NET | −3.20 | 2.14 | −4.65 | 2.15 | 1.84 | 1.93 | −0.22 | ||
Notes: FROM_WTH and TO_WTH capture 'within' connectedness which refers to the connectedness effect that occurs within the frequency band and is weighted by the power of the series on the given frequency band exclusively while FROM_ABS and TO_ABS refer to the frequency connectedness decomposition of the original Diebold & Yilmaz (2012) connectedness measures into distinct parts that when summed provide the original connectedness measures. Hence FROM ABS is the measure of frequency connectedness in absolute sense while FROM WTH is the measure of 'within' connectedness. |
1–3 months | Interbank Kenya | Interbank Uganda | Interbank Tanzania | KSHS/USD | UGX/USD | TZS/USD | Brent | FROM_ABS | FROM_WTH | ||||||||||
Interbank Kenya | 13.61 | 0.86 | 0.47 | 0.41 | 1.95 | 0.40 | 0.01 | 0.59 | 4.07 | ||||||||||
Interbank Uganda | 0.88 | 1.30 | 0.34 | 0.20 | 0.26 | 0.02 | 0.00 | 0.24 | 1.70 | ||||||||||
Interbank Tanzania | 7.01 | 0.72 | 10.32 | 0.26 | 2.76 | 0.71 | 0.02 | 1.64 | 11.39 | ||||||||||
KSHS/USD | 3.94 | 1.18 | 0.25 | 17.01 | 4.09 | 1.82 | 0.17 | 1.64 | 11.37 | ||||||||||
UGX/USD | 1.58 | 0.07 | 0.02 | 1.06 | 8.93 | 0.39 | 0.12 | 0.46 | 3.20 | ||||||||||
TZS/USD | 0.12 | 0.01 | 0.04 | 0.41 | 0.63 | 7.27 | 0.01 | 0.17 | 1.22 | ||||||||||
Brent | 0.09 | 0.04 | 0.01 | 0.06 | 0.07 | 0.04 | 8.81 | 0.04 | 0.30 | ||||||||||
TO_ABS | 1.95 | 0.41 | 0.16 | 0.34 | 1.40 | 0.48 | 0.05 | 4.78 | |||||||||||
TO_WTH | 13.52 | 2.86 | 1.12 | 2.37 | 9.69 | 3.35 | 0.33 | 33.24 | |||||||||||
NET | 1.36 | 0.17 | −1.48 | −1.30 | 0.93 | 0.31 | 0.01 | ||||||||||||
3–6 months | Interbank Kenya | Interbank Uganda | Interbank Tanzania | KSHS/USD | UGX/USD | TZS/USD | Brent | FROM_ABS | FROM_WTH | ||||||||||
Interbank Kenya | 4.39 | 2.68 | 0.66 | 0.08 | 0.25 | 0.97 | 0.01 | 0.66 | 5.19 | ||||||||||
Interbank Uganda | 0.96 | 3.39 | 0.72 | 0.19 | 0.09 | 0.12 | 0.01 | 0.30 | 2.32 | ||||||||||
Interbank Tanzania | 1.18 | 0.80 | 8.72 | 0.05 | 0.44 | 3.62 | 0.13 | 0.89 | 6.94 | ||||||||||
KSHS/USD | 0.96 | 3.56 | 0.17 | 7.99 | 1.45 | 0.50 | 0.16 | 0.97 | 7.60 | ||||||||||
UGX/USD | 0.77 | 0.34 | 0.05 | 1.02 | 6.02 | 1.23 | 0.23 | 0.52 | 4.08 | ||||||||||
TZS/USD | 0.09 | 0.03 | 0.39 | 1.01 | 0.31 | 10.97 | 0.18 | 0.29 | 2.24 | ||||||||||
Brent | 0.11 | 0.48 | 0.09 | 0.34 | 0.15 | 0.37 | 21.07 | 0.22 | 1.72 | ||||||||||
TO_ABS | 0.58 | 1.13 | 0.30 | 0.38 | 0.38 | 0.97 | 0.10 | 3.85 | |||||||||||
TO_WTH | 4.54 | 8.81 | 2.32 | 3.01 | 2.99 | 7.62 | 0.80 | 30.10 | |||||||||||
NET | −0.08 | 0.83 | −0.59 | −0.59 | −0.14 | 0.69 | −0.12 | ||||||||||||
Beyond 6 months | Interbank Kenya | Interbank Uganda | Interbank Tanzania | KSHS/USD | UGX/USD | TZS/USD | Brent | FROM_ABS | FROM_WTH | ||||||||||
Interbank Kenya | 10.28 | 37.06 | 1.28 | 9.09 | 10.26 | 5.17 | 0.12 | 9.00 | 12.35 | ||||||||||
Interbank Uganda | 5.62 | 52.88 | 2.17 | 12.77 | 13.77 | 4.02 | 0.30 | 5.52 | 7.58 | ||||||||||
Interbank Tanzania | 2.62 | 23.80 | 14.63 | 5.27 | 6.78 | 10.01 | 0.17 | 6.95 | 9.54 | ||||||||||
KSHS/USD | 0.81 | 14.08 | 1.49 | 33.10 | 0.53 | 6.53 | 0.20 | 3.38 | 4.64 | ||||||||||
UGX/USD | 1.23 | 5.98 | 0.50 | 17.24 | 34.69 | 18.18 | 0.36 | 6.21 | 8.53 | ||||||||||
TZS/USD | 0.67 | 0.69 | 1.19 | 9.95 | 5.72 | 57.87 | 2.44 | 2.95 | 4.05 | ||||||||||
Brent | 0.26 | 2.50 | 0.28 | 5.43 | 0.30 | 2.49 | 57.03 | 1.61 | 2.21 | ||||||||||
TO_ABS | 1.60 | 12.02 | 0.99 | 8.53 | 5.34 | 6.63 | 0.51 | 35.62 | |||||||||||
TO_WTH | 2.20 | 16.50 | 1.36 | 11.72 | 7.33 | 9.10 | 0.70 | 48.90 | |||||||||||
NET | −7.39 | 6.49 | −5.96 | 5.16 | −0.88 | 3.68 | −1.10 | ||||||||||||
Notes: FROM_WTH and TO_WTH capture 'within' connectedness which refers to the connectedness effect that occurs within the frequency band and is weighted by the power of the series on the given frequency band exclusively while FROM_ABS and TO_ABS refer to the frequency connectedness decomposition of the original Diebold & Yilmaz (2012) connectedness measures into distinct parts that when summed provide the original connectedness measures. Hence FROM ABS is the measure of frequency connectedness in absolute sense while FROM WTH is the measure of 'within' connectedness. |