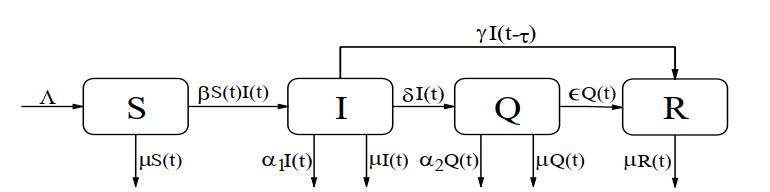
Citation: Tasnim Fatima, Ekeoma Ijioma, Toshiyuki Ogawa, Adrian Muntean. Homogenization and dimension reduction of filtration combustion in heterogeneous thin layers[J]. Networks and Heterogeneous Media, 2014, 9(4): 709-737. doi: 10.3934/nhm.2014.9.709
[1] | Marte K.R. Kjøllesdal, Gerd Holmboe-Ottesen . Dietary Patterns and Birth Weight—a Review. AIMS Public Health, 2014, 1(4): 211-225. doi: 10.3934/Publichealth.2014.4.211 |
[2] | Carla Gonçalves, Helena Moreira, Ricardo Santos . Systematic review of mediterranean diet interventions in menopausal women. AIMS Public Health, 2024, 11(1): 110-129. doi: 10.3934/publichealth.2024005 |
[3] | Hanan E. Badr, Travis Saunders, Omar Bayoumy, Angelie Carter, Laura Reyes Castillo, Marilyn Barrett . Reversal for metabolic syndrome criteria following the CHANGE program: What are the driving forces? Results from an intervention community-based study. AIMS Public Health, 2025, 12(1): 162-184. doi: 10.3934/publichealth.2025011 |
[4] | Truong Tuyet Mai, Tran Thu Trang, Tran Thi Hai . Effectiveness of germinated brown rice on metabolic syndrome: A randomized control trial in Vietnam. AIMS Public Health, 2020, 7(1): 33-43. doi: 10.3934/publichealth.2020005 |
[5] | Jürgen Vormann . Magnesium: Nutrition and Homoeostasis. AIMS Public Health, 2016, 3(2): 329-340. doi: 10.3934/publichealth.2016.2.329 |
[6] | Yu Li, Zhehui Luo, Claudia Holzman, Hui Liu, Claire E. Margerison . Paternal race/ethnicity and risk of adverse birth outcomes in the United States, 1989–2013. AIMS Public Health, 2018, 5(3): 312-323. doi: 10.3934/publichealth.2018.3.312 |
[7] | Jennifer L Lemacks, Laurie S Abbott, Cali Navarro, Stephanie McCoy, Tammy Greer, Sermin Aras, Michael B Madson, Jacqueline Reese-Smith, Chelsey Lawrick, June Gipson, Byron K Buck, Marcus Johnson . Passive recruitment reach of a lifestyle management program to address obesity in the deep south during the COVID-19 pandemic. AIMS Public Health, 2023, 10(1): 116-128. doi: 10.3934/publichealth.2023010 |
[8] | Trong Hung Nguyen, Thi Hang Nga Nguyen, Hung Le Xuan, Phuong Thao Nguyen, Kim Cuong Nguyen, Tuyet Nhung Le Thi . Nutritional status and dietary intake before hospital admission of pulmonary tuberculosis patients. AIMS Public Health, 2023, 10(2): 443-455. doi: 10.3934/publichealth.2023031 |
[9] | Ragnar Rylander . Treatment with Magnesium in Pregnancy. AIMS Public Health, 2015, 2(4): 804-809. doi: 10.3934/publichealth.2015.4.804 |
[10] | John P. Elliott, John C. Morrison, James A. Bofill . Risks and Benefits of Magnesium Sulfate Tocolysis in Preterm Labor (PTL). AIMS Public Health, 2016, 3(2): 348-356. doi: 10.3934/publichealth.2016.2.348 |
Coronavirus disease 2019 (COVID-19) remains an on-going global pandemic at present. The World Health Organization declared COVID-19 as a Public Health Emergency of International Concern (PHEIC) on January 30, 2020. According to data released by Johns Hopkins University, there are 37, 213, 592 confirmed cases and 1, 072, 959 deaths in 188 countries and regions around the world by October 11, 2020 [1]. The disease is caused by a novel coronavirus named severe acute respiratory syndrome coronavirus 2 (SARS-CoV-2) [2]. Although most SARS-CoV-2-infected cases have asymptomatic or mild-to-moderate diseases, around 10$ \% $ of those infected may develop severe pneumonia and other associated organ malfunctions [3].
In recent investigations, many scholars studied different epidemic models of COVID-19. In Zhang's study [4], a new mathematical model (SEIRD) was proposed, which is constructed with five classes including susceptible, exposed, infected, recovered and deaths to describe the possibility of transmission in a given general population. Bhadauria et al. [5] studied the SIQ model by using the stability theory of nonlinear ordinary differential equations. Li et al. [6] developed a numerical method preserving positivity for a stochastic SIQS epidemic model. In Ref. [7], Higazy proved the existence of a stable solution for the fractional order COVID-19 SIDARTHE model. A $ \theta {\rm{ - }} $SEIHQRD model which is more relevant to COVID-19 was developed by Ramos et al. [8]. Nisar et al. [9] constructed the SIRD model and verified the correctness. Batistela et al. [10] proposed an SIRSi model of COVID-19. Especially, Paré et al. [11] proposed traditional group models, continuous-time and discrete-time versions of the models with non-trivial networks on simple SIR-based models, and supported the need for networked models through presenting a set of simulations.
In the propagation process of COVID-19, we consider that there is a time-delay from infection to recovery. At present, there are some researches on epidemic models with time-delay. Zhu et al. [12] constructed a time delay reaction-diffusion model that is closer to the actual spread of the COVID-19 epidemic with considering the time delay effect of infected persons during the spread of the epidemic. In Ref. [13], the mathematical modeling of COVID-19 fatality trends was constructed by Scheiner et al., which was based on infection-to-death delay rule. Wei et al. [14] considered the time delay from susceptible individuals to infected individuals, thus, proposed a new SVEIR epidemic disease model with time delay, and analyzed the dynamic behavior of the model under pulse vaccination. In Ref. [15], Mukherjee considered an S-I epidemic model with time delay, the time delay is the immune period and the incubation period, and gave an estimate on the length of delays for system which is stable in the absence of delays remains stable.
At the same time, many researchers analyzed the stability of the COVID-19 model. In Ref. [16], Araz dealt with a mathematical model about COVID-19 spread, and analyzed global and local stability for the considered model. Annas et al. [17] carried out the stability analysis and numerical simulation of the SEIR model on the spread of COVID-19 in the research. Besides, some scholars conducted mathematical analysis on the principle of COVID-19 infection. Almocera et al. [18] studied an in-host model, and the stability of a unique positive equilibrium point, with viral load $ {{V}^{\rm{*}}} $, suggested that the virus may replicate fast enough to overcome T cell response and cause infection. In Ref. [19], Samanta analyzed the stability of the proposed model to control the epidemic.
And some scholars predicted the epidemic situation. León et al. [20] proposed an SEIARD mathematical model and attempted to forecast the evolution of the outbreak. Youssef et al. [21] carried out numerical verification and predictions of the proposed SEIR model, and compared the results with the real data due to the spreading of the COVID-19 in Saudi Arabia.
In addition, some scholars used the models with several compartments to put forward analysis and opinions on epidemic prevention and control. In Ref. [22], Carli et al. proposed a multi-region SIRQTHE model and an optimal control approach, which supported governments in defining the most effective strategies to be adopted during post-lockdown mitigation phases in a multi-region scenario. Giordano et al. [23] established a SIDARTHE model that predicted the course of the epidemic to help plan an effective control strategy. On the basis of the expected utility theory, Odagaki [24] carried out a theoretical framework to find out an optimum strategy for minimizing the maximum number of infeced and for controlling the outbreak of pandemic. In Ref. [25], Saldaña et al. developed a compartmental epidemic model of the COVID-19 epidemic outbreak to evaluate the theoretical impact of plausible control interventions.
In this paper, we establish the COVID-19 epidemic model based on the SIQR model. However, these large deviations between model predictions and the actually recorded numbers stem from the uncertainty of the underlying SIQR model parameters: they may not be sufficiently well known for the novel COVID-19 pandemic yet. Generally, considering the latent period gives rise to models with the incorporation of delays to solve problems. Therefore, time-delay has important biologic meaning in epidemic models. Differently, by describing the time-delay from the infection period to the recovery period, we propose a new delay SIQR epidemic model with horizontal transmission in our paper, and obtain the critical treatment time through calculation and stability analysis, which can contribute greatly to medicine.
The rest of the paper is organized as follows: In Section 2, considering the treatment time of epidemic, we present a delayed differential equation for COVID-19. In Section 3, we analyze the existence and stability of equilibria and the existence Hopf bifurcation of the system. In Section 4, we derive the normal form of Hopf bifurcation for above model, then determine the direction of Hopf bifurcation and the stability of bifurcating periodic solutions. In Section 5, we present the simulated results to verify the correctness of the theoretical analysis, and show the effect of treatment time on epidemic. Finally, some conclusions are shown in Section 6.
COVID-19 is similar to other infectious diseases. The susceptible population can be infected by COVID-19 carriers. Some of the infected people are quarantined, and some are killed by COVID-19. Both infected and quarantined people may be cured after treatment. A feature of COVID-19 can be obtained by combining a large number of cases, that is, cured infected people are difficult to be infected again. In Ref. [26], Liu et al. proposed an infectious disease model as follows:
$ {dSdt=Λ−μS−βSIN,dIdt=βSIN−(μ+γ+δ+α)I,dQdt=δI−(μ+ϵ+α)Q,dRdt=γI+ϵQ−μR, $
|
(2.1) |
where $ S $, $ I $, $ Q $, $ R $ denote the numbers of susceptible, infective, quarantined and removed, $ N = S + I + Q + R $ is the number of total population individuals. The parameter $ \Lambda $ is the recruitment rate of $ S $ corresponding to births and immigration; $ \beta $ denotes the average number of adequate contacts; $ \mu $ is the natural death rate; $ \gamma $ and $ \epsilon $ denote the recover rates from group $ I $, $ Q $ to $ R $, respectively; $ \delta $ denotes the removal rate from $ I $; $ \alpha $ is the disease-caused death rate of $ I $ and $ Q $. The parameters involved in system (2.1) are all positive constants.
On the basis of reference [26], we define $ \beta $ as the contact rate, which eliminates the calculation of the total population number $ N $ and simplifies the form of the equation. It is a good way to reduce the error of the model results by reducing the parameters. Combined with practical reports, it is known that some quarantined patients will receive treatment, and the mortality of treated patients will be greatly reduced. Therefore, unlike the model in Ref.[26], we believe that the COVID-19 mortality of infected and quarantined person is different. Meanwhile, the uncertainty of the model parameters is also the main reason for the difference between the predicted results and the actual. Here, we consider the time-delay ($ \tau $) from infection to recovery process on the basis of the model in Ref.[26], and the critical treatment time obtained by the calculation with time-delay is helpful to the treatment of COVID-19, which is rarely studied before and should be paid attention to in severe epidemic areas. In particular, the model can change the parameters according to the situation of different regions to get the corresponding critical treatment time, and take reasonable measures to control the epidemic effectively. Finally, we present a new COVID-19 epidemic model, and the relationships between the four populations (susceptible population($ S $), infected population($ I $), quarantined population($ Q $), recovered population($ R $)) are obtained, as shown in Figure 1.
Thus, we construct the following COVID-19 epidemic model:
$ {˙S=Λ−μS−βSI,˙I=βSI−δI−μI−α1I−γI(t−τ),˙Q=δI−ϵQ−α2Q−μQ,˙R=γI(t−τ)+ϵQ−μR, $
|
(2.2) |
where $ \Lambda, \; \mu, \; \beta, \; \delta, \; \gamma, \; \epsilon, \; \alpha_1, \; \alpha _2 $ are parameters; $ S, \; I, \; Q, \; R $ are control variables and $ \tau $ is the time-delay. The specific definitions are given in the Table 1.
Symbol | Definition |
$ S $ | Number of susceptible people |
$ I $ | Number of infected people |
$ Q $ | Number of quarantined people |
$ R $ | Number of recovered people |
$ \Lambda $ | Natural increase of population |
$ \beta $ | Transition rate from $ S $ to $ I $ |
$ \delta $ | Transition rate from $ I $ to $ Q $ |
$ {\gamma} $ | Transition rate from $ I $ to $ R $, the cure rate of infected persons |
$ {\epsilon} $ | Transition rate from $ Q $ to $ R $, the cure rate of quarantined persons |
$ {\alpha _1} $ | COVID-19 mortality rate of infected persons |
$ {\alpha _2} $ | COVID-19 mortality rate of quarantined persons |
$ \mu $ | Natural death of population |
$ \tau $ | The time-delay from infection to recovery process |
In this section, system (2.2) is considered. Obviously, system (2.2) has two equilibria:
$ E_1 = (S_1^*, I_1^*, Q_1^*, R_1^*), \; E_2 = (S_2^*, I_2^*, Q_2^*, R_2^*), $ |
where
$ S_1^* = \frac{\Lambda}{\mu} $, $ I_1^* = 0 $, $ Q_1^* = 0 $, $ R_1^* = 0 $, $ S_2^* $ = $ \frac{{{\gamma}{\rm{ + }}{\alpha _1}{\rm{ + }}\delta {\rm{ + }}\mu }}{\beta } $, $ I_2^* $ = $ \frac{{\Lambda \beta - \mu ({\gamma} + {\alpha _1} + \delta + \mu)}}{{\beta ({\gamma} + {\alpha _1} + \delta + \mu)}} $,
$ Q_2^* $ = $ \frac{{\delta [\Lambda \beta - \mu ({\gamma} + {\alpha _1} + \delta + \mu)]}}{{\beta ({\gamma} + {\alpha _1} + \delta + \mu)({\alpha _2} + {\epsilon} + \mu)}} $, $ R_2^* $ = $ \frac{{[\Lambda \beta - \mu ({\gamma} + {\alpha _1} + \delta + \mu)][{\gamma}({\epsilon} + {\alpha _2} + \mu) + {\epsilon}\delta]}}{{\beta \mu ({\gamma} + {\alpha _1} + \delta + \mu)({\alpha _2} + {\epsilon} + \mu)}} $.
Firstly, we consider the first equilibrium $ E_1 = (S_1^*, I_1^*, Q_1^*, R_1^*) = (\frac{\Lambda}{\mu}, 0, 0, 0) $. Transferring the equilibrium to the origin and linearizing the system around it, we obtain the characteristic equation of the linearized system as follows:
$ (λ+μ)2(λ+ϵ+α2+μ)(λ−βS∗1+δ+α1+μ+γe−λτ)=0, $
|
(3.1) |
where $ S_1^* $ = $ \frac{\Lambda }{\mu } $.
When $ \tau = 0 $, Eq. (3.1) becomes
$ (λ+μ)2(λ+ϵ+α2+μ)(λ−βS∗1+δ+α1+μ+γ)=0. $
|
(3.2) |
Eq. (3.2) has four roots: $ {\lambda _1}{\rm{ = }}{\lambda _2}{\rm{ = - }}\mu, \; {\lambda _3}{\rm{ = - }}{\epsilon} - {\alpha _2} - \mu, \; {\lambda _4} = \beta S_1^* - \delta - {\alpha _1} - \mu- {\gamma} $, due to $ \mu > 0, {\epsilon} > 0, {\alpha _2} > 0 $, so in the actual situation, $ {\lambda _1} < 0 $, $ {\lambda _2} < 0 $, $ {\lambda _3} < 0 $.
We consider the following assumption:
(H1) $ \frac{{\beta \Lambda }}{\mu } < \mu + \delta + {\alpha _1} + {\gamma} $.
When (H1) holds, all the roots of Eq. (3.2) have negative real parts, and the equilibrium $ E_1 $ is locally asymptotically stable when $ \tau = 0 $.
When $ \tau > 0 $, let $ \lambda = \mathrm{i}\omega\; (\omega > 0) $ be a root of Eq. (3.1). Due to $ {\lambda _1} < 0 $, $ {\lambda _2} < 0 $, $ {\lambda _3} < 0 $, actually, we only need to discuss the following equation:
$ λ−βS∗1+δ+α1+μ+γe−λτ=0. $
|
(3.3) |
Substituting $ \lambda = \mathrm{i}\omega\; (\omega > 0) $ into Eq. (3.3) and separating the real and imaginary parts, we obtain
$ {βS∗1−(δ+α1+μ)=γcos(ωτ),ω=γsin(ωτ). $
|
(3.4) |
Eq. (3.4) leads to
$ {cos(ωτ)=βS∗1−(δ+α1+μ)γ,sin(ωτ)=ωγ. $
|
(3.5) |
Adding the square of two equations of Eq. (3.5), we have
$ h(ω)=ω2+(βS∗1−δ−α1−μ)2−γ2=0. $
|
(3.6) |
Therefore, we give the following assumption:
(H2) $ {(\beta S_1^* - \delta - {\alpha _1} - \mu)^2} - \gamma^2 < 0 $.
If (H2) holds, then Eq. (3.6) has one positive root $ \omega_0 = \sqrt {\gamma^2 - {{(\beta S_1^* - \delta - {\alpha _1} - \mu)}^2}} $. Substituting $ \omega_0 $ into Eq. (3.5), we get
$ τ(j)1={1ω0[arccos(P0)+2jπ],Q0≥0,1ω0[2π−arccos(P0)+2jπ],Q0<0,j=0,1,2,⋯, $
|
(3.7) |
where
$ Q0=sin(ω0τ(j)1)=ω0γ,P0=cos(ω0τ(j)1)=βS∗1−(δ+α1+μ)γ. $
|
Lemma 3.1. If (H2) holds, when $ \tau = \tau_1^{(j)}\; (\; j = 0, 1, 2, \cdots) $, then Eq. (3.1) has a pair of pure imaginary roots $ \pm \mathrm{i}\omega_0 $, and all the other roots of Eq. (3.1) have nonzero real parts.
Furthermore, let $ \lambda(\tau) = \alpha(\tau)+\mathrm{i}\omega(\tau) $ be the root of Eq. (3.1) satisfying $ \alpha(\tau_1^{(j)}) = 0 $, $ \omega(\tau_1^{(j)}) = \omega_0\; (\; j = 0, 1, 2, \cdots) $.
Lemma 3.2. If (H2) holds, we have the following transversality conclusions:
$ {\rm{Re}}(\frac{{{\rm{d}}\tau }}{{{\rm{d}}\lambda }})\left| {_{\tau = \tau _1^{(j)}}} \right. = {\rm{Re}}{(\frac{{{\rm{d}}\lambda }}{{{\rm{d}}\tau }})^{ - 1}}\left| {_{\tau = \tau _1^{(j)}}} \right. = \frac{1}{{\gamma^2}} > 0 $, where $ j = 0, 1, 2, \cdots $.
Secondly, for the other equilibrium $ E_2 $ = $ (S_2^*, I_2^*, Q_2^*, R_2^*) $ of the system (2.2), similarly, transferring the equilibrium to the origin and linearizing the system around it, we obtain the characteristic equation of the linearized system as follows:
$ (λ+μ)(λ+ϵ+α2+μ)[(λ+μ+βI∗2)(λ−γ+γe−λτ)+β2I∗2S∗2]=0, $
|
(3.8) |
where $ S_2^* $ = $ \frac{{{\gamma}{\rm{ + }}{\alpha _1}{\rm{ + }}\delta {\rm{ + }}\mu }}{\beta } $, $ I_2^* $ = $ \frac{{\Lambda \beta - \mu ({\gamma} + {\alpha _1} + \delta + \mu)}}{{\beta ({\gamma} + {\alpha _1} + \delta + \mu)}} $.
When $ \tau = 0 $, Eq. (3.8) becomes
$ (λ+μ)(λ+ϵ+α2+μ)[λ2+(μ+βI∗2)λ+β2I∗2S∗2]=0. $
|
(3.9) |
Eq. (3.9) has four roots: $ {\lambda _1}{\rm{ = - }}\mu $, $ {\lambda _2}{\rm{ = - }}{\epsilon} - {\alpha _2} - \mu $, $ {\lambda _3} = \frac{{ - \mu - \beta S_2^* + \sqrt {{{(\mu + \beta S_2^*)}^2} - 4{\beta ^2}S_2^*I_2^*} }}{2}4 $, $ {\lambda _4} = \frac{{ - \mu - \beta S_2^* - \sqrt {{{(\mu + \beta S_2^*)}^2} - 4{\beta ^2}S_2^*I_2^*} }}{2} $. Due to $ \mu > 0 $, $ {\epsilon} > 0 $, $ {\alpha _2} > 0 $, in the actual situation, $ {\lambda _1} < 0 $, $ {\lambda _2} < 0 $.
Note that $ \beta ^2S_2^*I_2^* > 0 $, we consider the following assumption:
(H3) $ \frac{{\beta \Lambda }}{\mu } > \mu + \delta + {\alpha _1} + {\gamma} $.
Therefore, under the assumption (H3), all the roots of Eq. (3.9) have negative real parts, and the equilibrium $ E_2 = (S_2^*, I_2^*, Q_2^*, R_2^*) $ is locally asymptotically stable when $ \tau = 0 $.
When $ \tau > 0 $, let $ \lambda = \mathrm{i}\omega\; (\omega > 0) $ be a root of Eq. (3.8). Due to $ \mu > 0 $, $ {\epsilon} > 0 $, $ {\alpha _2} > 0 $, actually, we only need to consider the equation:
$ (λ+μ+βI∗2)(λ−γ+γe−λτ)+β2I∗2S∗2=0. $
|
(3.10) |
Substituting $ \lambda = \mathrm{i}\omega\; (\omega > 0) $ into Eq. (3.10) and separating the real and imaginary parts, we have
$ {ω2+γμ+γβI∗2−β2S∗2I∗2=(γμ+γβI∗2)cosωτ+γωsinωτ,ω(μ−γ+βI∗2)=−γωcosωτ+(γμ+γβI∗2)sinωτ. $
|
(3.11) |
Eq. (3.11) leads to
$ {cosωτ=(ω2+γμ+γβI∗2−β2S∗2I∗2)(γμ+γβI∗2)−γω2(μ−γ+βI∗2)(γμ+γβI∗2)2+γ2ω2,sinωτ=γω(μ+βI∗2)(μ−γ+βI∗2)+γω(ω2+γμ+γβI∗2−β2S∗2I∗2)(γμ+γβI∗2)2+γ2ω2. $
|
(3.12) |
Adding the square of two equations of Eq. (3.12), let $ z = \omega^2 $, then
$ h(z)=z2+c1z+c0=0, $
|
(3.13) |
where $ {c_1} = {(\mu + \beta I_2^*)^2} - 2{\beta ^2}S_2^*I_2^* $, $ {c_0} = {({\gamma}\mu + {\gamma}\beta I_2^* - {\beta ^2}S_2^*I_2^*)^2} - {({\gamma}\mu + {\gamma}\beta I_2^*)^2} $. Therefore, we give the following assumptions:
(H4) $ {c_0} < 0. $
(H5) $ c_1^2 - 4{c_0} > 0 $, $ {c_1} < 0, {c_0} > 0 $.
If (H4) holds, then Eq. (3.13) has only one positive real root $ z_1 $. If (H5) holds, then Eq. (3.13) has two positive real roots $ z_2 $ and $ z_3 $. Substituting $ \omega_k = \sqrt{z_k}\; (\; k = 1, 2, 3) $ into Eq. (3.12), we get
$ τ(j)2,k={1ωk[arccos(Pk)+2jπ],Qk≥0,1ωk[2π−arccos(Pk)+2jπ],Qk<0,k=1,2,3,j=0,1,2,⋯, $
|
(3.14) |
where
$ Qk=sin(ωkτ(j)2,k)=γωk(μ+βI∗2)(μ−γ+βI∗2)+γωk(ω2k+γμ+γβI∗2−β2S∗2I∗2)(γμ+γβI∗2)2+γ2ω2k,Pk=cos(ωkτ(j)2,k)=(ω2k+γμ+γβI∗2−β2S∗2I∗2)(γμ+γβI∗2)−γω2k(μ−γ+βI∗2)(γμ+γβI∗2)2+γ2ω2k. $
|
Lemma 3.3. If (H4) or (H5) holds, when $ \tau = \tau_{2, k}^{(j)}\; (\; k = 1, 2, 3;\; j = 0, 1, 2, \cdots) $, then Eq. (3.8) has a pair of pure imaginary roots $ \pm \mathrm{i}\omega_k $, and all the other roots of Eq. (3.8) have nonzero real parts.
Furthermore, let $ \lambda(\tau) = \alpha(\tau)+\mathrm{i}\omega(\tau) $ be the root of Eq. (3.8) satisfying $ \alpha(\tau_{2, k}^{(j)}) = 0 $, $ \omega(\tau_{2, k}^{(j)}) = \omega_k\; (\; k = 1, 2, 3;\; j = 0, 1, 2, \cdots) $.
Lemma 3.4. If (H4) or (H5) holds, and $ {z_k} = \omega _k^2 $, $ h'({z_k}) \ne 0 $, then we have the following transversality conclusions:
$ {\rm{Re}}(\frac{{{\rm d}\tau }}{{{\rm d}\lambda }}) \left| {_{\tau = \tau _{2, k}^{(j)}}} \right. = {\mathop{\rm Re}\nolimits} {(\frac{{{\rm d}\lambda }}{{{\rm d}\tau }})^{ - 1}}\left| {_{\tau = \tau _{2, k}^{(j)}}} \right. = \frac{{h'(z_k)}}{{\gamma^2[{{(\mu + \beta I_2^*)}^2} + {\omega _k^2}]}}\ne 0 $, $ k = 1, 2, 3, \; j = 0, 1, 2, \cdots $.
Theorem 3.1. We show the conclusion associated with two equilibria of the system (2.2).
(1) If the assumptions (H1) and (H2) hold, the equilibrium $ E_1 $ of the system (2.2) undergoes Hopf bifurcation at $ \tau = \tau_1^{(j)}\; (\; j = 0, 1, 2, \cdots) $, where $ \tau_1^{(j)} $ is given by Eq. (3.7), and we have: when $ \tau \in [0, \tau _1^{(0)}) $, the equilibrium $ E_1 $ is locally asymptotically stable, and the equilibrium $ E_1 $ is unstable when $ \tau > \tau _1^{(0)} $.
(2) If the assumptions (H4) or (H5) holds, the equilibrium $ E_2 $ of the system (2.2) undergoes Hopf bifurcation at $ \tau = \tau_{2, k}^{(j)}\; (\; k = 1, 2, 3;\; j = 0, 1, 2, \cdots) $, where $ \tau_{2, k}^{(j)} $ is given by Eq. (3.14), and
(a) If the assumptions (H3) and (H4) hold, $ h(z) $ has one positive root $ z_1 $, then when $ \tau \in [0, \tau _{2, 1}^{(0)}) $, the equilibrium $ E_2 $ is locally asymptotically stable, and the equilibrium $ E_2 $ is unstable when $ \tau > \tau _{2, 1}^{(0)} $.
(b) If the assumptions (H3) and (H5) hold, $ h(z) $ has two positive roots $ z_2 $ and $ z_3 $, we suppose $ z_2 < z_3 $, then $ h'({z_2}) < 0, h'({z_3}) > 0 $, note that $ \tau _{2, 2}^{(0)} > \tau _{2, 3}^{(0)} $. Then $ \exists $ $ m \in N $ makes $ 0 < \tau _{2, 3}^{(0)} < \tau _{2, 2}^{(0)} < \tau _{2, 3}^{(1)} < \tau _{2, 2}^{(1)} < \cdots < \tau _{2, 2}^{(m-1)} < \tau _{2, 3}^{(m)} < \tau _{2, 3}^{(m + 1)} $. When $ \tau \in [0, \tau _{2, 3}^{(0)})\cup \bigcup\limits_{l = 1}^m {} (\tau _{2, 2}^{(l-1)}, \tau _{2, 3}^{(l)}) $, the equilibrium $ E_2 $ of the system (2.2) is locally asymptotically stable, and when $ \tau \in \bigcup\limits_{l = 0}^{m-1} {}(\tau _{2, 3}^{(l)}, \tau _{2, 2}^{(l)}) \cup (\tau _{2, 3}^{(m)}, + \infty) $, the equilibrium $ E_2 $ of the system (2.2) is unstable.
In this section, we discuss the normal form of Hopf bifurcation for the system (2.2) by using the multiple time scales method. Combining with actual situation, we concern about the impact of treatment time on epidemic control. Therefore, we consider the time-delay $ \tau $ as a bifurcation parameter, let $ \tau {\rm{ = }}{\tau _c} + \varepsilon {\tau _\varepsilon } $, where $ \tau_c $ is the critical value of Hopf bifurcation given in Eq. (3.7) or Eq. (3.14) respectively, $ \tau _\varepsilon $ is the disturbance parameter, and $ \varepsilon $ is the dimensionless scale parameter. We suppose the characteristic Eq. (3.1) and Eq. (3.8) have eigenvalue $ \lambda = {\rm i}\omega^{(k)}\; (\; k = 1, 2) $, where $ \omega^{(1)} = \omega_0 $, $ \omega^{(2)} = \omega_1 $, $ \omega_2 $ or $ \omega_3 $, at which system (2.2) undergoes a Hopf bifurcation at equilibrium $ E_k = (S_k^*, I_k^*, Q_k^*, R_k^*) $, $ k = 1, 2 $, respectively.
Then system (2.2) can be written as
$ ˙X(t)=AX(t)+BX(t−τ)+F[X(t),X(t−τ)], $
|
(4.1) |
where $ X(t) = {(S_k, I_k, Q_k, R_k)^{\rm T}} $, $ X(t - \tau) = {(S_k(t - \tau), I_k(t - \tau), Q_k(t - \tau), R_k(t - \tau))^{\rm T}} $,
$ A=(−μ−βI∗k−βS∗k00βI∗kβS∗k−(δ+μ+α1)000δ−(ϵ+α2+μ)000−ϵ−μ),B=(00000−γ0000000−γ00), $
|
$ F(X(t),X(t−τ))=(FSFIFQFR)=(Λ−μS∗k−βS∗kI∗k−βSkIkβSkIk+βS∗kI∗k−(δ+μ+α1+γ)I∗kδI∗k−(ϵ+α2+μ)Q∗kγI∗k−ϵQ∗k−μR∗k). $
|
We suppose $ h_k $, $ h_k^* $($ k = 1, 2 $) are the eigenvector of the corresponding eigenvalue $ \lambda = {\rm i}\omega^{(k)} $, $ \lambda = -{\rm i}\omega^{(k)} $ respectively of Eq. (4.1) for equilibrium $ E_k $, and satisfies $ \langle {h_k^*, h_k} \rangle = {\overline {h_k^*} ^{\rm T}}h_k = 1 $. By simple calculation, we can get:
$ hk=(hk1,hk2,hk3,hk4)T=(1,−λ+μ+βI∗kβS∗k,−δ(λ+μ+βI∗k)(λ+ϵ+α2+μ)βS∗k,δϵ(λ+μ+βI∗k)−γ(λ+μ+βI∗k)(λ+ϵ+α2+μ)e−λτ(λ+μ)(λ+ϵ+α2+μ)βS∗k)T,h∗k=(h∗k1,h∗k2,h∗k3,h∗k4)T=dk(1,−λ+μ+βI∗kβI∗k,0,0)T, $
|
(4.2) |
where $ {d_k} = \frac{{{\beta ^2}S_k^*I_k^*}}{{{\beta ^2}S_k^*I_k^* - {{(-\lambda + \mu + \beta I_k^*)}^2}}}, \; k = 1, 2. $
We suppose the solution of Eq. (4.1) as follows:
$ X(t)=X(T0,T1,T2,⋯)=∞∑k=1εkXk(T0,T1,T2,⋯), $
|
(4.3) |
where $ X({T_0}, {T_1}, {T_2}, \cdots) = {[S({T_0}, {T_1}, {T_2}, \cdots), I({T_0}, {T_1}, {T_2}, \cdots), Q({T_0}, {T_1}, {T_2}, \cdots), R({T_0}, {T_1}, {T_2}, \cdots)]^{\rm T}}, \\ {X_k}({T_0}, {T_1}, {T_2}, \cdots) = {[{S_k}({T_0}, {T_1}, {T_2}, \cdots), {I_k}({T_0}, {T_1}, {T_2}, \cdots), {Q_k}({T_0}, {T_1}, {T_2}, \cdots), {R_k}({T_0}, {T_1}, {T_2}, \cdots)]^{\rm T}}. $
The derivative with respect to t is transformed into:
$ \frac{d}{{dt}} = \frac{\partial }{{\partial {T_0}}} + {\varepsilon }\frac{\partial }{{\partial {T_1}}} + \varepsilon ^2\frac{\partial }{{\partial {T_2}}} + \cdots = {D_0} + {\varepsilon }{D_1} + \varepsilon ^2{D_2} + \cdots, $ |
where $ {D_i} = \frac{\partial }{{\partial {T_i}}} $, $ i = 0, 1, 2, \cdots. $
We make $ {X_j} = {({S_j}, {I_j}, {Q_j}, {R_j})^{\rm T}} = {X_j}({T_0}, {T_1}, {T_2}, \cdots) $, $ {X_{j, {\tau _c}}} = {({S_{j, {\tau _c}}}, {I_{j, {\tau _c}}}, {Q_{j, \tau _c}}, {R_{j, \tau _c}})^{\rm T}} = {X_j}({T_0} - {\tau _c}, {T_1}, {T_2}, \cdots) $, $ j = 1, 2, 3, \cdots. $
From Eq. (4.3) we can get:
$ ˙X(t)=εD0X1+ε2D1X1+ε3D2X1+ε2D0X2+ε3D0X3+⋯. $
|
(4.4) |
The Taylor expansion of $ X(t - \tau) $ is carried out:
$ X(t−τ)=εX1,τc+ε2X2,τc+ε3X3,τc−ε2τεD0X1,τc−ε3τεD0X2,τc−ε2τcD1X1,τc−ε3τεD1X1,τc−ε3τcD2X1,τc−ε3τcD1X2,τc+⋯, $
|
(4.5) |
where $ {X_{j, {\tau _c}}} = {X_j}({T_0} - {\tau _c}, {T_1}, {T_2}, \cdots), \; j = 1, 2, 3, \cdots. $
Substituting Eqs. (4.3) $ \sim $ (4.5) into Eq. (4.1), and balancing the coefficients before $ \varepsilon $ on both sides of the equation, the following expression is obtained:
$ D0Sk1+μSk1+βI∗kSk1+βS∗kIk1=0,D0Ik1−βI∗kSk1−βS∗kIk1+(δ+μ+α1)Ik1+γIk1,τc=0,D0Qk1−δIk1+(ϵ+α2+μ)Qk1=0,D0Rk1−γIk1,τc+ϵQk1+μRk1=0,k=1,2. $
|
(4.6) |
Thus Eq. (4.6) has the following solution form:
$ X1(T1,T2,T3,⋯)=G(T1,T2,T3,⋯)eiω(k)T0hk+ˉG(T1,T2,T3,⋯)e−iω(k)T0ˉhk,k=1,2. $
|
(4.7) |
The expression of the coefficient before $ {\varepsilon ^2} $ is as follows:
$ D0Sk2+μSk2+βI∗kSk2+βS∗kIk2=−D1Sk1−βSk1Ik1,D0Ik2−βI∗kSk2−βS∗kIk2+(δ+μ+α1)Ik2+γIk2,τc=−D1Ik1+βSk1Ik1+γ(τεD0Ik1,τc+τcD1Ik1,τc),D0Qk2−δIk2+(ϵ+α2+μ)Qk2=−D1Qk1,D0Rk2−γIk2,τc+ϵQk2+μRk2=−D1Rk1−γ(τεD0Ik1,τc+τcD1Ik1,τc),k=1,2. $
|
(4.8) |
Substituting Eq. (4.7) into the right hand side of Eq. (4.8), and the coefficient vector of $ {{\rm e}^{{\rm i}\omega^{(k)} {T_0}}} $ is denoted by $ m_1 $. According to the solvable condition $ \langle {h_k^*, {m_1}} \rangle = 0 $, the expression of $ \frac{{\partial G}}{{\partial {T_1}}} $ can be obtained as follows:
$ ∂G∂T1=NkτεG, $
|
(4.9) |
where $ {N_k} = \frac{{{{({\rm i}\omega^{(k)} + \mu + \beta I_k^*)}^2}{\gamma} \cdot {\rm i}\omega^{(k)} {{\rm e}^{ - {\rm i}\omega^{(k)} {\tau _c}}}}}{{{{({\rm i}\omega^{(k)} + \mu + \beta I_k^*)}^2} - {\beta ^2}S_k^*I_k^* - {{({\rm i}\omega^{(k)} + \mu + \beta I_k^*)}^2}{\gamma}{\tau _c}{{\rm e}^{ - {\rm i}\omega^{(k)} {\tau _c}}}}}, \; k = 1, 2. $
Since $ \tau_\varepsilon $ is a disturbance parameter, we only consider its effect on the linear part. It has little effect on the high order, so it can be ignored. Therefore, we ignore the part containing $ \tau_\varepsilon $ in the higher order. We suppose:
$ Sk2=gk1e2iω(k)T0G2+ˉgk1e−2iω(k)T0ˉG2+lk1GˉG,Ik2=gk2e2iω(k)T0G2+ˉgk2e−2iω(k)T0ˉG2+lk2GˉG,Qk2=gk3e2iω(k)T0G2+ˉgk3e−2iω(k)T0ˉG2+lk3GˉG,Rk2=gk4e2iω(k)T0G2+ˉgk4e−2iω(k)T0ˉG2+lk4GˉG, $
|
(4.10) |
where
$ gk1=−βhk2(2iω(k)+δ+μ+α1+γe−2iω(k)τc)J,gk2=(2iω(k)+μ)βhk2J,gk3=(2iω(k)+μ)δβhk2(2iω(k)+μ+α2+ϵ)J,gk4=(2iω(k)+μ)βhk2[ϵ(2iω(k)+μ+α2+ϵ)−δϵ](2iω(k)+μ+α2+ϵ)(2iω(k)+μ)J,lk1=−β(hk2+ˉhk2)(δ+μ+α1+γ)V,lk2=βμ(hk2+ˉhk2)V,lk3=δβμ(hk2+ˉhk2)(μ+α2+ϵ)V,lk4=γβμ(hk2+ˉhk2)(μ+α2+ϵ)−ϵδβμ(hk2+ˉhk2)μ(μ+α2+ϵ)V,J=(2iω(k)+μ+βI∗k)(2iω(k)+δ+μ+α1+γe−2iω(k)τc)−βS∗k(2iω(k)+μ),V=(μ+βI∗k)(δ+μ+α1+γ)+βμS∗k,hk2=−iω(k)+μ+βI∗kβS∗k,k=1,2. $
|
(4.11) |
The expression of the coefficient before $ {\varepsilon ^3} $ is:
$ D0Sk3+μSk3+βI∗kSk3+βS∗kIk3=−D2Sk1−D1Sk2−βSk2Ik1−βSk1Ik2,D0Ik3−βI∗kSk3−βS∗kIk3+(δ+μ+α1)Ik3+γIk3,τc=−D2Ik1−D1Ik2+βSk2Ik1+βSk1Ik2+γ(τcD1Ik2,τc+τcD2Ik1,τc),D0Qk3−δIk3+(ϵ+α2+μ)Qk3=−D2Qk1−D1Qk2,D0Rk3−γIk3,τc+ϵQk3+μRk3=−D2Rk1−D1Rk2−γ(τcD1Ik2,τc+τcD2Ik1,τc),k=1,2. $
|
(4.12) |
Substituting Eq. (4.7), Eq. (4.10) and Eq. (4.11) into the right hand side of Eq. (4.12), and the coefficient vector of $ {{\rm e}^{{\rm i}\omega^{(k)} {T_0}}} $ is denoted by $ m_2 $. According to the solvable condition $ \langle {h_k^*, {m_2}} \rangle = 0 $, the expression of $ \frac{{\partial G}}{{\partial {T_2}}} $ can be obtained as follows:
$ ∂G∂T2=ξkG2ˉG, $
|
(4.13) |
where
$ ξk=β(lk2+gk2+lk1hk2+gk1ˉhk2)(iω(k)+μ)βI∗k−(iω(k)+μ+βI∗k)(γτce−iω(k)τc−1)hk2,k=1,2. $
|
Let $ G \to G/{\varepsilon } $, then the deduce to third-order normal form of Hopf bifurcation of system (2.2) is:
$ ˙G=NkτεG+ξkG2ˉG, $
|
(4.14) |
where $ N_k $ is given in Eq. (4.9), and $ \xi _k $ is given in Eq. (4.13), $ k = 1, 2 $.
By substituting $ G = r{{\rm e}^{{\rm i}\theta }} $ into Eq. (4.14), the following normal form of Hopf bifurcation in polar coordinates can be obtained:
$ {˙r=Re(Nk)τεr+Re(ξk)r3,˙θ=Im(Nk)τε+Im(ξk)r2, $
|
(4.15) |
where $ N_k $ is given in Eq. (4.9), and $ \xi _k $ is given in Eq. (4.13), $ k = 1, 2 $.
According to the normal form of Hopf bifurcation in polar coordinates, we just need to consider the first equation in system (4.15). Thus, there is the following theorem:
Theorem 4.1. For the system (4.15), when $ \frac{{{\mathop{\rm Re}\nolimits} (N_k){\tau _\varepsilon }}}{{{\mathop{\rm Re}\nolimits} ({\xi _k})}} < 0\; (\; k = 1, 2) $, there is a nontrivial fixed point $ r = \sqrt { - \frac{{{\mathop{\rm Re}\nolimits} ({N_k}){\tau _\varepsilon }}}{{{\mathop{\rm Re}\nolimits} ({\xi _k})}}} $, and system (2.2) has periodic solution:
(1) If $ {\mathop{\rm Re}\nolimits} (N_k){\tau _\varepsilon } < 0 $, then the periodic solution reduced on the center manifold is unstable.
(2) If $ {\mathop{\rm Re}\nolimits} (N_k){\tau _\varepsilon } > 0 $, then the periodic solution reduced on the center manifold is stable.
In this section, according to the data presented in Refs. [27] and [28], we let $ \Lambda = 10.48 $, $ \beta = 0.0004 $, $ \delta = 0.7 $, $ {\alpha _1} = 0.0484 $, $ {\alpha _2} = 0.022778 $, $ {\gamma} = 0.42386 $, $ {\epsilon} = 0.475 $, $ \mu = 0.00714 $. Then, system (2.2) only has one nonnegative equilibrium $ E_1 = (S_1^{\rm{*}}, I_1^{\rm{*}}, Q_1^{\rm{*}}, R_1^{\rm{*}}) \approx (1467.8, 0, 0, 0). $ Obviously, the assumption (H1) holds, thus the equilibrium $ E_1 $ is locally asymptotically stable when $ \tau $ = 0.
Substituding these parameter values into the Eqs. (3.5)$ \sim $(3.7), using MATLAB, we can obtain $ {\omega _0}\approx0.3900 $, $ Q_0\approx 0.9201 $, $ P_0\approx-0.4284 $, $ \tau _1^{(0)}\approx5.1629 $. According to the Theorem 3.1, the equilibrium $ E_1 $ is locally asymptotically stable at $ \tau \in [0, \tau _1^{(0)}) $, and when $ \tau = \tau _1^{(0)} $, Hopf bifurcation occurs near the equilibrium $ E_1 $. Then, we obtain $ {\mathop{\rm Re}\nolimits} (N_1) > 0 $, $ {\mathop{\rm Re}\nolimits} (\xi_1) > 0 $ from Eqs. (4.9)$ \sim $(4.13), thus according to the Theorem 4.1, the system (2.2) has backward periodic solution and the periodic solution is unstable when $ \tau_\varepsilon < 0 $.
When $ \tau = 0 $, we choose the initial value $ (1473, 1, 2, 1) $ and the corresponding locally asymptotically stable equilibrium is shown in Figure 2.
When $ \tau = 4.2 \in [0, \tau _1^{(0)}) $, we choose the initial value $ (1473, 1, 2, 1) $, the equilibrium $ E_1 $ is also locally asymptotically stable (see Figure 3). Thus, the epidemic will be controlled eventually.
When $ \tau = 5.5 \in (\tau _1^{(0)}, +\infty) $, we choose the initial value $ (1473, 1, 2, 1) $, and the equilibrium $ E_1 $ is unstable shown in Figure 4.
It can be seen from Figure 2$ \sim $ Figure 4, when $ \tau \in [0, \tau _1^{(0)}) $, the equilibrium $ E_1 $ of system (2.2) is locally asymptotically stable (see Fig. 2 and Fig. 3). When $ \tau \in (\tau _1^{(0)}, +\infty) $, the equilibrium $ E_1 $ of system (2.2) is unstable, and accompanied by the fluctuation with increased amplitude (see Fig. 4). There is no periodic solution, so we believe the theoretical analysis is correct.
Remark 1: Through numerical simulations, it can be found that when the treatment time $ \tau < \tau_1^{(0)} $, the shorter treatment time is, the faster the epidemic tends to be stable. If $ \tau > \tau_1^{(0)} $, the epidemic cannot be controlled effectively, and accompanied by the fluctuation with increased amplitude with time. Thus, we obtain the critical treatment time $ \tau_1^{(0)} $ for the epidemic.
According to the data presented in Refs. [27] and [28], we choose another set of parameters, $ \Lambda = 2.48 $, $ \beta = 0.86834 $, $ \delta = 0.3 $, $ {\alpha _1} = 0.0484 $, $ {\alpha _2} = 0.022778 $, $ {\gamma} = 0.42386 $, $ {\epsilon} = 0.475 $, $ \mu = 0.00714 $. We obtain $ E_1 = (S_1^{\rm{*}}, I_1^{\rm{*}}, Q_1^{\rm{*}}, R_1^{\rm{*}})\approx(347.3, 0, 0, 0) $, $ E_2 = (S_2^{\rm{*}}, I_2^{\rm{*}}, Q_2^{\rm{*}}, R_2^{\rm{*}})\approx (0.8976, 3.1737, 1.8857, 313.8526) $. Apparently, the assumption (H1) does not hold, but the assumption (H3) holds, therefore, the equilibrium $ E_1 $ is unstable and the equilibrium $ E_2 $ is locally asymptotically stable when $ \tau = 0 $.
Substituding these parameter values into the Eq. (3.13), we get $ c_1\approx3.3383 $, $ c_0\approx-0.4174 $. It satisfies the assumption (H4), therefore, Eq. (3.13) has only one positive root $ z_1\approx0.1204 $. Substituting these parameter values into the Eqs. (3.12)$ \sim $(3.14), using MATLAB, we can obtain $ {\omega _1}\approx0.3474 $, $ Q_1\approx 0.5926 $, $ P_1\approx-0.8055 $, $ \tau _{2, 1}^{(0)}\approx7.2175 $. According to the Theorem 3.1, the equilibrium $ E_2 $ is locally asymptotically stable at $ \tau \in [0, \tau _{2, 1}^{(0)}) $, and when $ \tau = \tau _{2, 1}^{(0)} $, Hopf bifurcation occurs near the equilibrium $ E_2 $. Then we obtain $ {\mathop{\rm Re}\nolimits} (N_2) > 0 $, $ {\mathop{\rm Re}\nolimits} (\xi_2) < 0 $ by Eqs. (4.9)$ \sim $(4.13), according to the Theorem 4.1, the system (2.2) has stable periodic solution near $ E_2 $, and the periodic solution reduced on the center manifold is stable.
When $ \tau $ = 0, we choose the initial value $ (0.85, 3, 1.9, 300) $ and the corresponding locally asymptotically stable equilibrium is shown in Figure 5.
When $ \tau = 4 \in [0, \tau _{2, 1}^{(0)}) $, we choose the initial value $ (0.85, 3, 1.9, 300) $, and the periodic solution of this model is locally asymptotically stable shown in Figure 6.
When $ \tau = 5 \in [0, \tau _{2, 1}^{(0)}) $, we choose the initial value $ (0.85, 3, 1.9, 300) $, the equilibrium $ E_2 $ has a stable equilibrium is shown in Figure 7.
It can be seen from Figure 5$ \sim $Figure 7, when $ \tau \in [0, \tau _{2, 1}^{(0)}) $, the equilibrium $ E_2 $ of system (2.2) is locally asymptotically stable. Especially, if $ \tau $ is larger, the time required for the system (2.2) to be controlled stable is longer.
When $ \tau = 7.23 > \tau _{2, 1}^{(0)} = 7.2175 $, we choose the initial value $ (0.85, 3, 1.9, 300) $, the model has stable periodic solution shown in Figure 8.
When $ \tau = 7.5 > 7.23 $, we choose the initial value $ (0.85, 3, 1.9, 300) $, and the equilibrium $ E_2 $ is unstable shown in Figure 9.
When $ \tau = 7.9 > 7.5 $, we choose the initial value $ (0.85, 3, 1.9, 300) $, and the equilibrium $ E_2 $ is unstable shown in Figure 10.
It can be seen from the Figure 8$ \sim $Figure 10, when $ \tau \in (\tau _{2, 1}^{(0)}, +\infty) $, the equilibrium $ E_2 $ of system (2.2) is unstable. When $ \tau $ approaches the critical value $ \tau _{2, 1}^{(0)} $, the equilibrium $ E_2 $ of system (2.2) exhibits periodic fluctuation and bifurcates stable periodic solutions (see Fig. 8). As $ \tau $ increasing, the fluctuation phenomenon lasts for a period of time, and shows increasing volatility trends (see Fig. 9) and disappears eventually (see Fig. 10). According to the Theorem 3.1 and Theorem 4.1, we know that the theoretical analysis is correct.
Remark 2: Through numerical simulations, it can be found that when the treatment time $ \tau < \tau _{2, 1}^{(0)} $, the epidemic can be controlled effectively, and the smaller the time-delay $ \tau $ is the faster the epidemic is controlled. When the treatment time $ \tau \in (\tau _{2, 1}^{(0)}, +\infty) $ is close to $ \tau _{2, 1}^{(0)} $, the epidemic will repeatedly occur periodically, otherwise, the epidemic cannot be controlled. Since the small variation in treatment time will lead to the epidemic polarization, therefore, whether to grasp the treatment time is essential to control the epidemic.
Remark 3: In Ref. [26], Liu et al. used the Milstein's Higher Order Method mentioned to illustrate their main results. And they obtained that the disease is persistent. Similarly, in our simulation, the epidemic is persistent and tends to stable with time.
In Ref. [29], the study carried out numerical simulation. First of all, they changed the value of parameters to get the "without control'' and "with control'' graphs, from which we can see that different populations tend to be stable in the end. At the same time, through comparison, it is concluded that the control technique is useful in controlling the disease. Our simulation results show that the system is locally asymptotically stable in the critical treatment time, and the disease will be effectively controlled. Then they fit real data with the infected class of their model and found that the number of cases grows exponentially with time, the disease would be serious without applying the proper optimal control strategies. And we conclude that if not treat within the critical time, that is to say, without timely and effective treatment, the epidemic will be uncontrollable.
In a word, although the model studied is different from Ref. [29], and we focus on the impact of time delay on the epidemic situation, but the overall idea and conclusion are consistent, so we can still verify the correctness of our simulation results. Moreover, we emphasize the time-delay of treatment, so we also obtain the critical treatment time, which can take more targeted measures to control the epidemic. To effectively control the epidemic situation, we need to take measures not only in the external environment, but also in the internal medical care, and now there is little research on the treatment direction. Therefore, the critical treatment time we get is helpful for the alleviation of the disease.
In this paper, according to the propagation characteristics of COVID-19, we have constructed the SIRQ epidemic model with time delay for the COVID-19. We have also analyzed the stability of the equilibria and the existence of Hopf bifurcation associated with both equilibria. Then, we have used the multiple time scales method to derive the normal form of Hopf bifurcation for above COVID-19 epidemic model. Finally, We have chosen two groups of parameter values according to the data presented in Refs. [27] and [28] for numerical simulations to verify the correctness of the theoretical analysis. Compared with the numerical simulation results of Refs. [26] and [29], we have got the conclusion that our numerical simulation results were accuracy.
The numerical simulations showed that the small change of time-delay $ \tau $ leads to the epidemic from stable to uncontrollable: the smaller $ \tau $ is the better the epidemic controlled, but with $ \tau $ increasing, the epidemic will occur repeatedly and outbreak eventually. Therefore, it is significant that find the critical treatment time to control the epidemic. In addition, we would predict the critical treatment time of epidemic in different regions according to the relevant parameters, so as to effectively control the epidemic in treatment, and realize the major breakthrough of medicine in the epidemic situation.
As for the part of numerical simulation, according to the opinions of reviewers, we will use more accurate data to get more valuable conclusions in further research.
The authors are extremely grateful to the anonymous referees and editor for their careful reading, valuable comments and helpful suggestions, which have helped us to improve the presentation of this work significantly. This study was funded by Fundamental Research Funds for the Central Universities of China (Grant No. 2572019BC14), the Heilongjiang Provincial Natural Science Foundation of China (Grant No. LH2019A001) and College Students Innovations Special Project funded by Northeast Forestry University of China (No.202010225035).
The Authors declare that this work has no conflict of interest.
[1] |
I. Aganovic, J. Tambaca and Z. Tutek, A note on reduction of dimension for linear elliptic equations, Glasnik Matematicki, 41 (2006), 77-88. doi: 10.3336/gm.41.1.08
![]() |
[2] | B. Alberts, D. Bray, J. Lewis, M. Raff, K. Roberts and J. Watson, Molecular Biology of the Cell, Garland, NY, 2002. |
[3] |
G. Allaire, Homogenization and two-scale convergence, SIAM J. Math. Anal., 23 (1992), 1482-1518. doi: 10.1137/0523084
![]() |
[4] |
G. Allaire and Z. Habibi, Homogenization of a conductive, convective, and radiative heat transfer problem in a heterogeneous domain, SIAM J. Math. Anal., 45 (2013), 1136-1178. doi: 10.1137/110849821
![]() |
[5] | B. Amaziane, L. Pankratov and V. Pytula, Homogenization of one phase flow in a highly heterogeneous porous medium including a thin layer, Asymptotic Analysis, 70 (2010), 51-86. |
[6] | L. Barbu and G. Morosanu, Singularly Perturbed Boundary Value Problems, vol. 156 of International Series of Numerical Mathematics, Birkhäuser, Basel, 2007. |
[7] |
M. Beneš and J. Zeman, Some properties of strong solutions to nonlinear heat and moisture transport in multi-layer porous structures, Nonlinear Anal. RWA, 13 (2012), 1562-1580. doi: 10.1016/j.nonrwa.2011.11.015
![]() |
[8] | G. Chechkin, A. L. Piatnitski and A. S. Shamaev, Homogenization Methods and Applications, vol. 234 of Translations of Mathematical Monographs, AMS, Providence, Rhode Island USA, 2007. |
[9] |
R.-H. Chen, G. B. Mitchell and P. D. Ronney, Diffusive-thermal instability and flame extinction in nonpremixed combustion, in Symposium (International) on Combustion, Elsevier, 24 (1992), 213-221. doi: 10.1016/S0082-0784(06)80030-5
![]() |
[10] |
M. Chipot, $l$ goes to Infinity, Birkhäuser, Basel, 2002. doi: 10.1007/978-3-0348-8173-9
![]() |
[11] |
M. Chipot and S. Guesmia, On some anisotropic, nonlocal, parabolic singular perturbations problems, Applicable Analysis, 90 (2011), 1775-1789. doi: 10.1080/00036811003627542
![]() |
[12] | D. Cioranescu and P. Donato, An Introduction to Homogenization, Oxford University Press, New York, 1999. |
[13] |
D. Cioranescu and J. S. J. Paulin, Homogenization in open sets with holes, J. Math. Anal. Appl., 71 (1979), 590-607. doi: 10.1016/0022-247X(79)90211-7
![]() |
[14] | D. Ciorănescu and A. Oud Hammouda, Homogenization of elliptic problems in perforated domains with mixed boundary conditions, Rev. Roumaine Math. Pures Appl., 53 (2008), 389-406. |
[15] |
D. Ciorănescu and J. Saint Jean Paulin, Homogenization of Reticulated Structures, Springer Verlag, Berlin, 1999. doi: 10.1007/978-1-4612-2158-6
![]() |
[16] |
P. Constantin, A. Kiselev, A. Oberman and L. Ryzhik, Bulk burning rate in passive-reactive diffusion, Arch. Ration. Mech. Anal., 154 (2000), 53-91. doi: 10.1007/s002050000090
![]() |
[17] |
B. Denet and P. Haldenwang, Numerical study of thermal-diffusive instability of premixed flames, Combustion Science and Technology, 86 (1992), 199-221. doi: 10.1080/00102209208947195
![]() |
[18] |
A. Fasano, M. Mimura and M. Primicerio, Modelling a slow smoldering combustion process, Math. Methods Appl. Sci., 33 (2010),1211-1220. doi: 10.1002/mma.1301
![]() |
[19] |
T. Fatima and A. Muntean, Sulfate attack in sewer pipes: Derivation of a concrete corrosion model via two-scale convergence, Nonlinear Analysis: Real World Applications, 15 (2014), 326-344. doi: 10.1016/j.nonrwa.2012.01.019
![]() |
[20] |
B. Gustafsson and J. Mossino, Non-periodic explicit homogenization and reduction of dimension: the linear case, IMA J. Appl. Math., 68 (2003), 269-298. doi: 10.1093/imamat/68.3.269
![]() |
[21] | Z. Habibi, Homogéneisation et Convergence à Deux Échelles lors D'échanges Thermiques Stationnaires et Transitoires. Application Aux Coeurs des Réacteurs Nucléaires à Caloporteur gaz., PhD thesis, École Polytechnique, Paris, 2011. |
[22] |
U. Hornung, Homogenization and Porous Media, Springer-Verlag New York, 1997. doi: 10.1007/978-1-4612-1920-0
![]() |
[23] |
U. Hornung and W. Jäger, Diffusion, convection, absorption, and reaction of chemicals in porous media, J. Diff. Eqs., 92 (1991), 199-225. doi: 10.1016/0022-0396(91)90047-D
![]() |
[24] | E. R. Ijioma, Homogenization approach to filtration combustion of reactive porous materials: Modeling, simulation and analysis, PhD thesis, Meiji University, Tokyo, Japan, 2014. |
[25] |
E. R. Ijioma, A. Muntean and T. Ogawa, Pattern formation in reverse smouldering combustion: A homogenisation approach, Combustion Theory and Modelling, 17 (2013), 185-223. doi: 10.1080/13647830.2012.734860
![]() |
[26] | K. Ikeda and M. Mimura, Mathematical treatment of a model for smoldering combustion, Hiroshima Math. J., 38 (2008), 349-361. |
[27] |
L. Kagan and G. Sivashinsky, Pattern formation in flame spread over thin solid fuels, Combust. Theory Model., 12 (2008), 269-281. doi: 10.1080/13647830701639462
![]() |
[28] | CASA Report. |
[29] |
V. Kurdyumov and E. Fernández-Tarrazo, Lewis number effect on the propagation of premixed laminar flames in narrow open ducts, Combustion and Flame, 128 (2002), 382-394, URL http://www.sciencedirect.com/science/article/pii/S00102180010 03583. doi: 10.1016/S0010-2180(01)00358-3
![]() |
[30] |
K. Kuwana, G. Kushida and Y. Uchida, Lewis number effect on smoldering combustion of a thin solid, Combustion Science and Technology, 186 (2014), 466-474. doi: 10.1080/00102202.2014.883220
![]() |
[31] | J. L. Lions, Quelques Méthodes de Résolution des Problemes Aux Limites Nonlinéaires, Dunod, Paris, 1969. |
[32] |
Z. Lu and Y. Dong, Fingering instability in forward smolder combustion, Combustion Theory and Modelling, 15 (2011), 795-815. doi: 10.1080/13647830.2011.564658
![]() |
[33] | S. Monsurro, Homogenization of a two-component composite with interfacial thermal barrier, Adv. Math. Sci. Appl., 13 (2003), 43-63. |
[34] |
S. Neukamm and I. Velcic, Derivation of a homogenized von-Karman plate theory from 3D nonlinear elasticity, Mathematical Models and Methods in Applied Sciences, 23 (2013), 2701-2748. doi: 10.1142/S0218202513500449
![]() |
[35] | M. Neuss-Radu, Some extensions of two-scale convergence, C. R. Acad. Sci. Paris. Mathematique, 322 (1996), 899-904. |
[36] |
M. Neuss-Radu and W. Jäger, Effective transmission conditions for reaction-diffusion processes in domains separated by an interface, SIAM J. Math. Anal., 39 (2007), 687-720. doi: 10.1137/060665452
![]() |
[37] |
G. Nguestseng, A general convergence result for a functional related to the theory of homogenization, SIAM J. Math. Anal, 20 (1989), 608-623. doi: 10.1137/0520043
![]() |
[38] |
A. Oliveira and M. Kaviany, Nonequilibrium in the transport of heat and reactants in combustion in porous media, Progress in Energy and Combustion Science, 27 (2001), 523-545. doi: 10.1016/S0360-1285(00)00030-7
![]() |
[39] |
S. Olson, H. Baum and T. Kashiwagi, Finger-like smoldering over thin cellulose sheets in microgravity, Twenty-Seventh Symposium (International) on Combustion, 27 (1998), 2525-2533. doi: 10.1016/S0082-0784(98)80104-5
![]() |
[40] |
I. Ozdemir, W. A. M. Brekelmans and M. G. D. Geers, Computational homogenization for heat conduction in heterogeneous solids, International Journal for Numerical Methods in Engineering, 73 (2008), 185-204. doi: 10.1002/nme.2068
![]() |
[41] |
A. Bourgeat, G. A. Chechkin and A. L. Piatnitski, Singular double porosity model, Applicable Analysis, 82 (2003), 103-116. doi: 10.1080/0003681031000063739
![]() |
[42] | P. Ronney, E. Roegner and J. Greenberg, Lewis number effects on flame spreading over thin solid fuels, Combust. Flame, 90 (1992), 71-83. |
[43] |
M. Sahraoui and M. Kaviany, Direct simulation vs volume-averaged treatment of adiabatic premixed flame in a porous medium, Int. J. Heat Mass Transf., 37 (1994), 2817-2834. doi: 10.1016/0017-9310(94)90338-7
![]() |
[44] | H. F. W. Taylor, Cement Chemistry, London: Academic Press, 1990. |
[45] |
R. Temam and A. Miranville, Mathematical Modeling in Continuum Mechanics, Cambridge University Press, 2005. doi: 10.1017/CBO9780511755422
![]() |
[46] | S. Turns, An Introduction to Combustion: Concepts and Applications, McGraw-Hill Series in Mechanical Engineering, McGraw-Hill, 2000. |
[47] | Advances in Chemical Engineering, 1-45. |
[48] |
C. J. van Duijn and I. S. Pop, Crystal dissolution and precipitation in porous media: Pore scale analysis, J. Reine Angew. Math, 577 (2004), 171-211. doi: 10.1515/crll.2004.2004.577.171
![]() |
[49] |
J.-P. Vassal, L. Orgéas, D. Favier and J.-L. Auriault, Upscaling the diffusion equations in particulate media made of highly conductive particles. I. Theoretical aspects, Physical Review E, 77 (2008), 011302, 10pp. doi: 10.1103/PhysRevE.77.011302
![]() |
[50] |
F. Yuan and Z. Lu, Structure and stability of non-adiabatic reverse smolder waves, Applied Mathematics and Mechanics, 34 (2013), 657-668. doi: 10.1007/s10483-013-1698-8
![]() |
[51] |
O. Zik, Z. Olami and E. Moses, Fingering instability in combustion, Phys. Rev. Lett., 81 (1998), 3868-3871. doi: 10.1103/PhysRevLett.81.3868
![]() |
1. | Yong Li, Huixin Li, Xiangwen Chen, Yingying Wang, Lei Chen, Man Yang, Jingguang Tang, 2023, Review of Interruption Control Technologies for Cascading Faults in Power Systems, 979-8-3503-0389-6, 426, 10.1109/ACFPE59335.2023.10455331 | |
2. | Jinlong Fu, Yan Song, Yike Feng, Rumor Spreading Model Considering the Roles of Online Social Networks and Information Overload, 2023, 11, 2169-3536, 123947, 10.1109/ACCESS.2023.3328396 | |
3. | Yanchao Liu, Pengzhou Zhang, Lei Shi, Junpeng Gong, A Survey of Information Dissemination Model, Datasets, and Insight, 2023, 11, 2227-7390, 3707, 10.3390/math11173707 | |
4. | Junnan Jiang, 2024, Topic Prediction and Trend Analysis in Social Media Data Mining, 9798400710148, 165, 10.1145/3702879.3702908 |
Symbol | Definition |
$ S $ | Number of susceptible people |
$ I $ | Number of infected people |
$ Q $ | Number of quarantined people |
$ R $ | Number of recovered people |
$ \Lambda $ | Natural increase of population |
$ \beta $ | Transition rate from $ S $ to $ I $ |
$ \delta $ | Transition rate from $ I $ to $ Q $ |
$ {\gamma} $ | Transition rate from $ I $ to $ R $, the cure rate of infected persons |
$ {\epsilon} $ | Transition rate from $ Q $ to $ R $, the cure rate of quarantined persons |
$ {\alpha _1} $ | COVID-19 mortality rate of infected persons |
$ {\alpha _2} $ | COVID-19 mortality rate of quarantined persons |
$ \mu $ | Natural death of population |
$ \tau $ | The time-delay from infection to recovery process |
Symbol | Definition |
$ S $ | Number of susceptible people |
$ I $ | Number of infected people |
$ Q $ | Number of quarantined people |
$ R $ | Number of recovered people |
$ \Lambda $ | Natural increase of population |
$ \beta $ | Transition rate from $ S $ to $ I $ |
$ \delta $ | Transition rate from $ I $ to $ Q $ |
$ {\gamma} $ | Transition rate from $ I $ to $ R $, the cure rate of infected persons |
$ {\epsilon} $ | Transition rate from $ Q $ to $ R $, the cure rate of quarantined persons |
$ {\alpha _1} $ | COVID-19 mortality rate of infected persons |
$ {\alpha _2} $ | COVID-19 mortality rate of quarantined persons |
$ \mu $ | Natural death of population |
$ \tau $ | The time-delay from infection to recovery process |