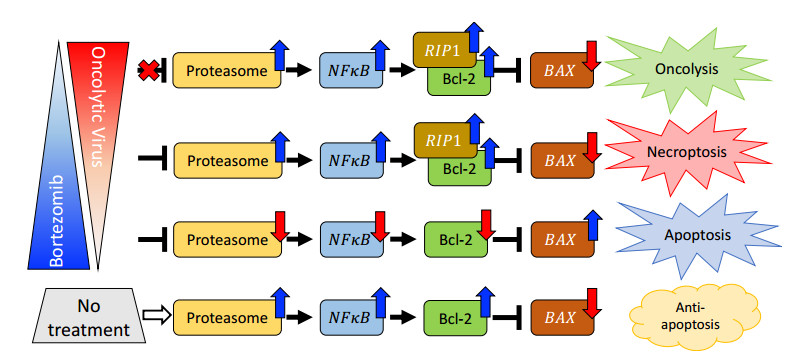
The paper examines the concept of place branding in rural areas, highlighting its significance in increasing reputation, attracting investment, improving competitiveness, and fostering a sense of pride and belonging among local populations. This article provides a literature review of rural branding, examining the trends of the main topics, analyses, and methodological approaches used in investigating place branding. The analysis is based on 45 articles from the Scopus and Web of Science databases, organized into seven main topics: tourism, rural identity, rural and regional development, sustainability, food and gastronomy, stakeholders' involvement, and landscape. Additionally, the study integrates a conceptual framework that outlines the interplay between these themes, emphasizing the theoretical grounding of rural place branding. The findings indicate a positive trend in the number of articles published on rural place branding, a predominance of qualitative research methods, and a strong focus on theoretical framework analysis. The study also highlights the role of sustainability in rural place branding, demonstrating how the synergy of cultural heritage preservation, stakeholder collaboration, and landscape branding significantly enhances the local economy, fosters community pride, and promotes regional agri-food products and gastronomy. These elements contribute to the sustainable growth of rural regions while reinforcing their unique identity within competitive global markets.
Citation: Fuad Jafarli, Maurizio Canavari. Place branding in rural areas: A literature review[J]. AIMS Agriculture and Food, 2025, 10(1): 129-152. doi: 10.3934/agrfood.2025007
[1] | Khaphetsi Joseph Mahasa, Rachid Ouifki, Amina Eladdadi, Lisette de Pillis . A combination therapy of oncolytic viruses and chimeric antigen receptor T cells: a mathematical model proof-of-concept. Mathematical Biosciences and Engineering, 2022, 19(5): 4429-4457. doi: 10.3934/mbe.2022205 |
[2] | Elzbieta Ratajczyk, Urszula Ledzewicz, Maciej Leszczynski, Avner Friedman . The role of TNF-α inhibitor in glioma virotherapy: A mathematical model. Mathematical Biosciences and Engineering, 2017, 14(1): 305-319. doi: 10.3934/mbe.2017020 |
[3] | Taeyong Lee, Adrianne L. Jenner, Peter S. Kim, Jeehyun Lee . Application of control theory in a delayed-infection and immune-evading oncolytic virotherapy. Mathematical Biosciences and Engineering, 2020, 17(3): 2361-2383. doi: 10.3934/mbe.2020126 |
[4] | Zizi Wang, Zhiming Guo, Hal Smith . A mathematical model of oncolytic virotherapy with time delay. Mathematical Biosciences and Engineering, 2019, 16(4): 1836-1860. doi: 10.3934/mbe.2019089 |
[5] | Lu Gao, Yuanshun Tan, Jin Yang, Changcheng Xiang . Dynamic analysis of an age structure model for oncolytic virus therapy. Mathematical Biosciences and Engineering, 2023, 20(2): 3301-3323. doi: 10.3934/mbe.2023155 |
[6] | Peter S. Kim, Joseph J. Crivelli, Il-Kyu Choi, Chae-Ok Yun, Joanna R. Wares . Quantitative impact of immunomodulation versus oncolysis with cytokine-expressing virus therapeutics. Mathematical Biosciences and Engineering, 2015, 12(4): 841-858. doi: 10.3934/mbe.2015.12.841 |
[7] | Prathibha Ambegoda, Hsiu-Chuan Wei, Sophia R-J Jang . The role of immune cells in resistance to oncolytic viral therapy. Mathematical Biosciences and Engineering, 2024, 21(5): 5900-5946. doi: 10.3934/mbe.2024261 |
[8] | Jianjun Paul Tian . The replicability of oncolytic virus: Defining conditions in tumor virotherapy. Mathematical Biosciences and Engineering, 2011, 8(3): 841-860. doi: 10.3934/mbe.2011.8.841 |
[9] | Joseph Malinzi, Rachid Ouifki, Amina Eladdadi, Delfim F. M. Torres, K. A. Jane White . Enhancement of chemotherapy using oncolytic virotherapy: Mathematical and optimal control analysis. Mathematical Biosciences and Engineering, 2018, 15(6): 1435-1463. doi: 10.3934/mbe.2018066 |
[10] | Nada Almuallem, Dumitru Trucu, Raluca Eftimie . Oncolytic viral therapies and the delicate balance between virus-macrophage-tumour interactions: A mathematical approach. Mathematical Biosciences and Engineering, 2021, 18(1): 764-799. doi: 10.3934/mbe.2021041 |
The paper examines the concept of place branding in rural areas, highlighting its significance in increasing reputation, attracting investment, improving competitiveness, and fostering a sense of pride and belonging among local populations. This article provides a literature review of rural branding, examining the trends of the main topics, analyses, and methodological approaches used in investigating place branding. The analysis is based on 45 articles from the Scopus and Web of Science databases, organized into seven main topics: tourism, rural identity, rural and regional development, sustainability, food and gastronomy, stakeholders' involvement, and landscape. Additionally, the study integrates a conceptual framework that outlines the interplay between these themes, emphasizing the theoretical grounding of rural place branding. The findings indicate a positive trend in the number of articles published on rural place branding, a predominance of qualitative research methods, and a strong focus on theoretical framework analysis. The study also highlights the role of sustainability in rural place branding, demonstrating how the synergy of cultural heritage preservation, stakeholder collaboration, and landscape branding significantly enhances the local economy, fosters community pride, and promotes regional agri-food products and gastronomy. These elements contribute to the sustainable growth of rural regions while reinforcing their unique identity within competitive global markets.
Cancer is characterized by abnormal cell growth and several steps of genetic mutations. Glioblastoma multiforme (GBM), a type of brain tumor, is one of the most fatal human cancers, with a low survival rate attributed to its aggressive growth and rapid, pervasive brain invasion [1,2]. Despite recent progress in cancer treatment, toxicity and corresponding side effects such as cancer-related cognitive changes [3,4] are common in conventional anticancer therapies such as chemotherapy and radiation therapy. Targeted cancer therapy, a promising choice with recent advances, can be a great way of eradicating cancer cells by controlling its dynamics and players in the signaling pathways that enable tumor growth [5]. Thus, there is a need for optimal control of promotion or inhibition of these intracellular molecules in cancer treatment including chemo- and oncolytic virus (OV) therapies.
The ubiquitin–proteasome system within a cell regulates the degradation of proteins, thus controlling cellular function and maintaining homeostasis [6,7]. Therefore, proteasome inhibitors can be used to treat cancer due its inhibition of cancer cells's increased need of protein synthesis and degradation in cancer progression [6,8]. By interfering with appropriate degradation and recycling of proteins, a critical component for cell viability [9], proteasome inhibitors induce accumulation of ubiquitin-tagged proteins, resulting in endoplasmic reticulum (ER) stress [10] and, thus, apoptosis, i.e., programmed cell death, of cancer cells. Bortezomib, the first proteasome inhibitor agent in cancer treatment [11], was approved for multiple myeloma and lymphoma by Food and Drug Administration (FDA) [6]. Despite minimal undesirable side effects such as accumulation of anti-apoptotic proteins, bortezomib was shown to consistently overcome the well-known apoptotic resistance of cancer cells such as melanoma cells [12], especially when combined with other anti-cancer agents [7,13,14].
NFκB, a master regulator that controls inflammation and immune responses as well as cell proliferation, stays usually in an inactive mode by its suppressor, IκB [15]. Various regulators such as ER stress can activate NFκB by degrading IκB which induces the translocation of NFκB to the nucleus and subsequent activation of various key genes, including the anti-apoptotic gate keeper, Bcl2 and suppression of the final apoptosis gene BAX (Figure 1) [16,17,18,19,20]. While the systemic homeostasis can be maintained by this production of IκB and the feedback control loop in normal tissue [21], a poor management of NFκB by IκB, or a constitutively active NFκB state, can allow the critical resistance of tumor cells to conventional anti-cancer therapy [22,23,24,25,26]. Therefore, agents targeting BAX showed a great potential of killing cancer cells by up-regulating BAX, thus, inducing apoptosis [17,27]. While apoptosis is a typical cell death program, necroptosis is another form of cell death process that is caspase-independent and causes massive cell killing. This is executed by up-regulation of reactive oxygen species (ROS) from activated RIP1, a receptor-interacting-protein kinase [28]. RIP1, a key activator of necroptosis, is not involved in the apoptotic signaling pathway [28,29]. Up-regulation of both Bcl2 and RIP1 as well as down-regulation of caspase-8 were associated with necroptosis in glioma cancer cells [30].
An emerging treatment option with rapid advances for cancer patients is oncolytic virotherapy (OV) that uses various forms of cancer-lysing viruses as a targeted therapy [31,32,33]. OVs can target and kill cancer cells by infection and destruction of cell structure while avoiding unnecessary damages to normal cells in tumor microenvironment (TME) [34,35]. While these viruses can be eliminated by the immune system on the way to the target tumor [36], the high degree of replications within a cancer cell and boosting effect of OV-induced immune activities within TME [31,37] can compensate the loss by immune cells, which enables these viruses to spread throughout the tumor from the infected tumoral region [38]. Clinical trials for various types of OVs as well as combination therapies have been conducted and are under way to test their anti-tumor efficacy and safety [6,31,39]. Some of them were approved by FDA for clinical use, for instance, T-Vec, a modified herpes simplex virus 1 (oHSV), for melanoma patients [6,37,40]. However, virus clearance due to OV-induced immunity prevents an OV therapy from efficient anti-tumor efficacy [41]. Combination therapies including OVs were suggested as an alternative method to overcome this low efficacy and several chemotherapeutic candidates are being tested for synergistic impact on cancer cell killing [37,42].
Yoo et al. [6] demonstrated that bortezomib was not only effective in killing cancer cells but promotes synergistic anti-tumor efficacy by inducing unfolded protein response in cancer cells, which then leads to the nuclear localization of the oHSV and a significant increase in viral replication. A follow-up study [43] then showed that the OV-bortezomib combination therapy can induce necroptosis through stimulation of the secretion of various cytokines associated with inflammation. While bortezomib treatment alone initiated a typical apoptosis, the combination treatment induced necroptosis by diverting to different signaling pathways [20,44]. Kim et al. [45] developed a mathematical model of the nonlinear dynamics of complex interactions between cancer cells and immune cells in OV–bortezomib therapy based on the experimental observations including those reported in [6,43]. It was found that both injection of exogenous natural killer (NK) cells and deletion of endogenous NK cells can lead to better anti-tumor efficacy, illustrating the complex role of TME such as immune cells in regulation of dynamics of OV-tumor interaction in a combination anti-cancer therapy. In a follow-up study [46], Kim et al. investigated the intracellular signaling pathway that governs the cancer cell death mechanism in response to various levels of bortezomib with OV therapy. Anti-apoptosis status and two different cellular death mechanisms, i.e., apoptosis and necroptosis in response to bortezomib treatment, were characterized by up- or down-regulation of three key intracellular molecules (NFkB/Bcl2, BAX, RIP1) [46], which was consistent with experimental observations [6,20,43,44,45]. These works [45,46,47] showed that the treatment protocols significantly affect the anti-tumor efficacy in the combination therapy and illustrated the importance of the detailed analysis of both apoptotic and necroptotic death of cancer cells in bortezomib-OV therapies. Various types of mathematical models by other groups have been developed for oncolytic virus therapy [48,49,50,51,52,53,54,55,56,57,58] and bortezomib treatment [59,60,61] in various cancers.
In this study, we employ a modified mathematical model of bortezomib-OV cancer therapy, integrating cell-death pathways based on Kim et al. [46]. This refined model enables the design of optimally controlled treatment strategies. Our modeling framework utilizes optimal control theory, a mathematical tool that deals with complex biological systems that can be controlled by an external agent [62]. Optimal control theory has been used to determine effective administration schedules of anti-cancer agents in various cancer types [63,64,65,66,67] including brain tumors [47,68,69,70,71] and lung cancers [72,73]. For example, an optimal control method was utilized to prevent aggressive glioma cell invasion by up-regulating miR-451 [71] and controlling cell cycle [70] within cancer cells, or to optimize the anti-tumor efficacy in a nonlinear immune microenvironment [47]. Optimal control theory along mathematical models was used in various fields including epidemiology for COVID-19 [74] and MERS [75] and cancer research. In particular, optimal control scheme was used to construct cost-effective therapy protocols against cancer development, from earlier works [64,65,66] to recent works [76]. These studies investigated the optimal strategies in controlling tumor growth under various treatments (chemotherapy [64,65,66,69,76,77,78], immunotherapy [79,80,81,82], and review [63] by minimizing the tumor size and costs. In previous studies [45,46], authors investigated the nonlinear dynamics of NK cells in a combination therapy (OVs + bortezomib) as well as anti-tumor efficacy via the intracellular signaling pathway (NFκB-Bcl-2-BAX-RIP1) and invasion pattern of GBM cells in brain in response to OVs and bortezomib. GBM is a serious brain cancer with very low survival rate and major cause of death is regrowth of invasive tumor cells in different locations after surgery. In [46], Kim et al explores tumor growth and invasion patterns in GBM patients based on the injection location of bortezomib and OVs. These studies focus mainly on anti-tumor efficacy of NK therapy and invasion pattern of tumor cells in the presence of bortezomib and OVs through constant treatment protocols. These approaches present challenges in translating the findings into practical clinical applications. In particular, in order to better understand the OV-mediated cell death program and provide personalized care for each cancer patient, it is necessary to develop optimally controlled injection strategies. Due to the safety issues, injection amount of OVs is usually limited and high doses of bortezomib can cause serious side effects. For example, local or IV-administration of OVs can cause adverse reactions such as influenza-like symptoms (vomiting, myalgia, headache, fatigue, elevated body temperature, nausea) and local reactions (pain, rash, erythema, peripheral edema) [83,84]. Despite the high degrees of anti-tumor effects, bortezomib can also induce serious side effects such as nausea, diarrhea, tiredness, low platelets, fever, and low white blood cell count [85,86,87]. On the other hand, low level of OVs and bortezomib decreases the overall anti-tumor efficacy due to virus clearance and blood-brain barrier (BBB). Therefore, appropriate level of OVs and bortezomib need to be controlled for injection into patient's body in order to avoid serious side effects and to obtain maximal clinical outcomes. In our framework based on the detailed hybrid type of mathematical model, we used an optimal control theory in order to maximize the anti-tumor efficacy and minimize the side effects and costs associated with administration at clinics by controlling the intracellular cell death program. We also investigated the dynamical systems of intracellular module in terms of apoptosis, oncolysis, and necroptosis in much more detail. This analysis provides a platform of developing more efficient next generation of OVs and another drugs targeting the necroptosis signaling pathway. Hence, we aim to obtain optimal treatment regimens (including timing and dosages) for OV and bortezomib, striking a balance where cancer cell eradication is maximized, while concurrently minimizing undesirable side effects and financial expenditures. The OV/bortezomib-mediated intracellular signaling pathways of apoptosis, necroptosis, and oncolysis in cell death programs are very complex biological system itself, providing fundamentally different mechanism of eradicating tumor cells. The necroptosis pathway plays a key role in cancer therapy including OV therapy. For example, anti-cancer drugs such as doxorubicin can increase oncolytic effect significantly (> 100-fold in breast cancer) by enhancing OV replication and inducing apoptosis and necroptosis [88]. Other research groups found that a radiation therapy can induce necroptosis in cancer progression [89]. However, it is poorly understood how these cell death programs work in OV therapy in detail. Thus, better understanding of this complex cell-death program itself can shed light into developing a new, more effective anti-cancer drug targeting NFκB-Bcl2-BAX-RIP1 in an OV combination therapy by manipulating the signaling network. Our findings enable the proposal of sophisticated optimal treatment strategies in terms of controlling the precise components at the gene level within a tumor cell rather than a population level (cf. [47]). Furthermore, detailed mathematical analysis of the intracellular cell death cascade and optimally controlled injection schedule can provide a general framework for the pharmaceutical companies to find new compounds targeting signaling pathways in OV therapy. We employed an optimal control formulation to strategically reduce tumor size by triggering both necroptosis and apoptosis through modulating intracellular signaling pathways (NFκB/Bcl2, BAX, RIP1), while managing the cumulative administration costs of bortezomib and OVs. Our results provide efficient and cost-effective therapeutic management schemes for OVs and bortezomib, taking into account overall anti-tumor efficacy.
In this work, we investigate the complex interactions between tumor cells and other therapeutic agents such as bortezomib (B) and oncolytic virus (v) incorporating an intracellular pathway using a mathematical model. Tumor cells are classified as : Uninfected (x), infected (y), and necrotic tumor (n) cells. Four intracellular components involved in the cell-death program are considered: IκB (S), NFκB (F), BAX (A), and RIP1 (R). The regulatory network in Figure 2 describes the complex interactions among the cellular components and intracellular modules. In the model, we consider a tumor microenvironment where (ⅰ) initially uninfected tumor cells (x) grow (i.e., initial condition of x is always positive.) (ⅱ) uninfected tumor cells (x) become infected tumor cells (y) under intracellular oncolysis condition in response OV treatment (ⅲ) bortezomib alone can kill tumor cells under intracellular apoptosis condition (ⅳ) uninfected tumor cells (x) become infected tumor cells (y) under intracellular necroptosis condition in response both OV treatment and bortezomib, leading to necroptotic death.
Following administration, OVs navigate toward the tumor site and infiltrate the target cancer cells. Within these cells, OVs vigorously replicate generating a substantial viral population. As the infected cancer cells disintegrate, OVs are released, instigating the infection-elimination process anew.
Bortezomib alone, can induce cancer cell death through a typical programmed cell-death pathways, involving apoptotic signaling cascade. Conversely, the combined therapy of bortezomib and OV orchestrates a synergistic assault on cancer cells, harnessing necroptotic signaling pathways to achieve a heightened level of cell destruction.
The network of the model is shown in Figure 2 and the corresponding model dynamics can be described by a system of ordinary differential equations in a dimensionless form as follows:
dSdt=λBB1+αvk+v+σ1σ29σ29+σ4F2−S, | (2.1) |
dFdt=σ7+σ2σ210σ210+σ5S2−ω1(D)F, | (2.2) |
dAdt=σ8+σ3σ211σ211+σ6F2−ω2A, | (2.3) |
dRdt=σ12+σ13[oHSV]F−ω3R, | (2.4) |
dxdt=λx(1−xx0)−β1xBIapop−β2xvIoncol−β3xvInecrop, | (2.5) |
dydt=−δy+β2xvIoncol+β3xvInecrop, | (2.6) |
dndt=δy−μn, | (2.7) |
dvdt=uV+bδy(1+α1B)−γv, | (2.8) |
dBdt=uB−(μ1x+μ2y)BkB+B−μBB. | (2.9) |
Here, λB is the signaling activation rate of IκB in response to bortezomib signal B, which is inhibited by the presence of OVs. So, [oHSV] indicates a response switch function of OVs (v) in the form, [oHSV] = vk+v with a Hill-type parameter k. This IκB activity is inhibited by NFκB (F) with inhibition strength σ4, autocatalytic enhancement parameter σ1, and Hill-type parameters σ9. In a similar fashion, the inhibition processes of NFκB (F) and BAX (A) by IκB and NFκB, are represented by corresponding parameters σ5,σ2,σ10 and σ6,σ3,σ11, respectively. Here, ω1(t) may depend on drugs that enhance NFκB degradation, i.e., ω1(D)=ω†1eD where D is a concentration of a NFκB inhibitor. Here, the drug concentration D is a constant. The signaling sources and decay rates of NFκB, BAX, and RIP1 are represented by σ7,σ8,σ12 and ω1,ω2, and ω3, respectively. The third term on the right hand side of Eq (2.1) indicates the dimensionless natural decay of IκB. The same response switch function [oHSV] in Eq (2.4) is used for activation of RIP1 in the presence of OVs in the system. Population dynamics of uninfected tumor cells in Eq (2.5) consists of logistic growth with the growth rate λ and carrying capacity x0, bortezomib-induced cell killing with the apoptotic death rate β1, infection process with infection rate β2, and massive tumor cell killing with necroptotic death rate β3. An uninfected tumor cell is infected when an oncolytic virus (OV) or a group of OVs enter this tumor cell and replicate upto 10,000-fold, initiating cell death program (either oncolysis and necroptosis) in response to OV therapy. Therefore, the indicator functions Ioncol and Inecrop reflect this infection process and the transition x→y. After infection and confirmation of the cell fate (either oncolysis or necroptosis), the infected tumor cells go through the subsequent, cell death process which is reflected in the y→n transition in the model. Here, Iapop,Ioncol,Inecrop are indicator functions for apoptotic, oncolytic, and necroptotic status, respectively, based on up- or down-regulation of NFκB, BAX, and RIP1:
Iapop={1if apoptosis 0otherwise ,Ioncol={1if oncolysis 0otherwise ,Inecrop={1if necroptosis 0otherwise . | (2.10) |
The exact definition of these status will be defined in the next section. Infected tumor cells (y) from the uninfected tumor population are cleared to dying tumor population (n) at a death rate δ and these necrotic population are cleared from the population system at a death rate μ. OVs are supplied at a rate uV and are able to replicate from lysis of infected cancer cells at a rate bδy with doubling number b in the absence of bortezomib (B). Injection of bortezomib in addition to OV therapy enhances viral replication at additional rate α1B, inducing synergistic anti-cancer effect. OVs are cleared from the system at a rate γ. Bortezomib is injected at a rate μB, internalized in uninfected and infected cancer cells at degradation rates μ1 and μ2, respectively, and cleared from the system at a decay rate μB.
A dimensional version of equations corresponding to Eqs (2.1)–(2.9) and the nondimensionalization provided in the Supplementary material (Supporting information S1 File). Matlab (Mathworks) software is used to simulate the transient dynamics of the model and to obtain the numerical results for the optimal control problems. Table 1 provides the full list of parameters, its meaning and corresponding values used in the model.
Par | Description | Values | Ref |
Intracellular dynamics | |||
λB | Bortezomib signaling scaling factor | 1.9 | [46] |
α | Inhibition strength of bortezomib by oHSV | 30 | [46] |
σ1 | Autocatalytic enhancement rate of IκB | 4 | [46] |
σ9 | Hill-type parameter | 1.0 | [46] |
σ4 | Inhibition strength of IκB by the NFκB-Bcl-2 complex | 2.2 | [46] |
σ7 | Signaling strength of the NFκB-Bcl-2 complex | 0.07 | [46] |
σ2 | Autocatalytic enhancement rate of the NFκB-Bcl-2 complex | 1.33 | [46] |
σ10 | Hill-type parameter | 1.0 | [46] |
σ5 | Inhibition strength of the NFκB-Bcl-2 complex by IκB | 1 | [46] |
ω1 | Decay rate of NFκB-Bcl-2 complex | 0.3 | [107,108] |
σ8 | Signaling strength of BAX | 0.0033 | [46] |
σ3 | Autocatalytic enhancement rate of BAX | 0.111 | [46] |
σ11 | Hill-type parameter | 1.0 | [46] |
σ6 | Inhibition strength of BAX by the NFκB-Bcl-2 complex | 1 | [46] |
ω2 | Decay rate of BAX | 0.02 | [107,108,109] |
σ12 | Signaling strength of RIP1 | 0.1 | [46] |
σ13 | Activation rate of RIP1 in the presence of OVs | 0.13 | [46] |
ω3 | Decay rate of RIP1 | 0.139 | [107,108,110] |
k | Hill type parameter of oHSV switching | 0.01 v∗ | [46] |
Cancer cells | |||
λ | proliferation rate of tumor cells | 4×10−3 | [46,57] |
x0 | Carrying capacity of uninfected tumor cells | 1 | [38,45,46,57] |
β1 | Bortezomib-induced apoptosis rate of tumor cells | 2×10−4 | [46] |
β2 | Oncolysis rate of tumor cells | 3×10−4 | [45,46,57] |
β3 | Necroptosis rate of tumor cells | 5×10−4 | [46] |
δ | infected cell lysis rate | 4.4×10−3 | [45,57] |
μ | Removal rate of dead cells | 2.3×10−3 | [45,46,57] |
Anticancer drugs | |||
b | Burst size of infected cells | 50 | [46,57] |
α1 | bortezomib-induced viral replication rate | 1 | [45,46] |
γ | clearance rate of viruses | 1.8×10−3 | [45,57] |
μ1 | consumption rate of bortezomib by uninfected tumor cells | 0.2075 | [45,46] |
μ2 | consumption rate of bortezomib by infected tumor cells | 0.2075 | [45,46] |
kB | Hill-type parameter | 1 | [45,46] |
μB | decay rate of bortezomib | 0.03 | [46,111,112] |
Reference values of main variables | |||
S∗ | Concentration of IκB | 0.05 μM | [113,114] |
F∗ | Concentration of the NFκB-Bcl2 complex | 0.5 μM | [113,114,115,116] |
A∗ | Concentration of BAX | 0.1 μM | [117] |
R∗ | Concentration of RIP1 | 5.0 μM | [118] |
x∗ | Uninfected cell density | 106cells/mm3 | [45,57,119] |
y∗ | Infected cell density | =x∗ | [45,57,119] |
n∗ | Dead cell density | =x∗ | [45,57,119] |
v∗ | Virus concentration | 2.2×108virus/mm3 | [45,57,119] |
B∗ | Bortezomib concentration | 1.0×10−11g/mm3 | [6,43,45] |
The optimal control theory was used to yield an optimal administration strategies of OVs and bortezomib that minimizes tumor cell populations (uninfected and infected tumor cells) and associated costs. Our goal is to obtain feasible therapeutic schedule of uV and uB to reduce the tumor size with minimal administrative costs. The controls uV and uB represent dosages of OVs and bortezomib, respectively. These controls are assumed to be bounded. That is, the control sets are defined as
uV(t)∈[0,umaxV]anduB(t)∈[0,umaxB]for allt∈[ts,tf], | (2.11) |
where umaxV and umaxB are the maximum dosage of OVs and bortezomib, respectively, which could be administered in a given treatment period [ts,tf] (where ts is the start time of treatment with optimal control applied, and tf is the end time).
Two distinct cost functionals are formulated to facilitate alternating administrations of OVs and bortezomib, enhancing their synergistic efficacy against cancer cells. In the first formulation, both integrands involve linear combination of uninfected x(t), infected y(t) and quadratic control. This quadratic form guarantees a strictly convex Hamiltonian and a unique minimizer, rendering the mathematical problem more tractable. Incorporating quadratic controls within the cost function effectively simulates the detrimental repercussions of excessive drug administration, a strategy observed in multiple studies [32,82,90,91,92,93]. However, the use of such quadratic functionals lacks robust biological justification, raising concerns about the validity of its simplifying assumptions [94]. The quadratic functional types may not be commonly motivated by biological systems making its simplifying model assumptions bit questionable and ambiguous [94]. However, its use also showed qualitatively significant outcomes in terms of incorporating the intrinsic nonlinearity of the given problem [90,95]. Thus, the quadratic controls in the cost function has been used in controlling the adverse effects of use of too much therapeutic drugs in various works [32,82,90,91,92,93]. In cancer immunotherapy, a type of isoperimetric optimal controls has been applied [79,80,81]. This leads to the formulation of an alternative cost functional, where linear controls are involved, supplemented by a penalty function serving as a guide for controlled implemented.
1) Quadratic controls This strategy proposes a sequential administration of OV and bortezomib, minimizing both their dosages and the levels of uninfected (x(t)) and infected cancer cells (y(t)). Employing quadratic controls lead to the following cost functional
J(uV(t),uB(t))=∫tfts(x(t)+y(t)+C1u2V(t)2)dt+∫t′ft′s(x(t)+y(t)+C2u2B(t)2)dt. | (2.12) |
2) Linear controls with constraint In this approach, linear controls are employed to emulate a biologically plausible regulatory mechanism. To achieve the desired effect of inducing necroptosis, a sigmoid function is integrated that ensures a sustained and optimal concentration of bortezomib. The cost functional yields
J(uV(t),uB(t))=∫tfts(x(t)+y(t)+C3uV(t))dt+∫t′ft′s(C4uB(t)+B51+ep(B−thB))dt. | (2.13) |
Our goal is to find optimal administration of both OV and bortezomib (u∗V(t) and u∗B(t)), identifying optimal dosages under the framework of optimal control theory. The principle technique for such an optimal control problem is to solve a set of "necessarycondition" that an optimal control and corresponding state must satisfy. The necessary conditions we derived were developed by Pontryagin. He introduced the concept of 'adjoint functions' to incorporate differential equations into the objective function. Adjoint functions serve a similar purpose to the Lagrange multiplier of multivariate calculus, which adds constraints to functions of several variables that are being maximized or minimized [62,96]. We perform numerical simulations by employing a fourth-order iterative Runge-Kutta method. Commencing with the initial conditions and an initial control estimate, we solve the state equations using the forward scheme. Furthermore, the adjoint equations are solved using the backward scheme, ensuring compliance with the transversality conditions. Control updates involve a convex combination of the previous controls and values derived from the characterizations. This methodology, recognized as the Forward-Backward Sweep Method (FBSM), has demonstrated convergence [94]. See supporting information S1 File for more details.
The fundamental structure of four phenotypic modes in response to various bortezomib levels in the absence and presence of OVs are represented in Figure 3. Specifically, Figure 3(A), (B) illustrate the steady states of three key intracellular variables: NFκB (represented by blue solid curve), BAX (depicted by the dashed red curve), and RIP1 (shown as the dotted yellow curve). These plots delineate the behavior of these variables under varying bortezomib (B) concentrations, when OVs are absent (v=0 in Figure 3(A)) and present (v=1 in Figure 3(B)).
In the absence of OV within the system, we observe elevated levels of NFκB, coupled with low levels of both BAX and RIP1 in response to lower levels of bortezomib. This dynamic pattern prevents the initiation of bortezomib-induced apoptosis, as the key apoptotic gene, BAX, remains suppressed. As the bortezomib concentration increases, a shift occurs: NFκB is down-regulated, while RIP1 maintains a low level; moreover, the BAX level transitions to an overexpression state, triggering programmed cell death, specifically apoptosis (Figure 3(A)). However, the intracellular dynamics of NFκB, BAX, and RIP1 undergo a significant transformation in the presence of OV when responding to bortezomib stimuli. Both NFκB and RIP1 consistently sustain high levels irrespective of bortezomib concentrations, while the BAX level remains consistently low (Figure 3(B)). This particular state signifies the activation of necroptotic signaling (depicted within the pink box in Figure 3(B)) under conditions of elevated bortezomib levels. Conversely, when bortezomib stimulus is limited, cell demise ensues through oncolysis (illustrated by the green box in Figure 3(B)), rather than necroptosis.
By defining thresholds for these intracellular variables, thF=1.7 for NFκB, thA=1.7 for BAX, thR=1.7 for RIP1, and thB=0.1 for bortezomib, we can delineatefour different phenotypic modes, namely the anti-apoptotic (Tt), apoptotic (Ta), necroptotic (Tn), and oncolysis (To) phases as follows:
Tt={(F,A,R)∈R3:F>thF,A<thA,R<thR}, | (3.1) |
Ta={(F,A,R)∈R3:F<thF,A>thA,R<thR}, | (3.2) |
Tn={(F,A,R,B)∈R4:F>thF,A<thA,R>thR,B>thB}, | (3.3) |
To={(F,A,R,B)∈R4:F>thF,A<thA,R>thR,B<thB}. | (3.4) |
Figure 3(C) illustrates the anti-apoptosis (yellow cube), apoptosis (blue cube), necroptosis (red cube), and oncolysis (green cube) modules in a NFκB-BAX-RIP1 state space. Figure 3(D), (E) show temporal dynamics of concentrations of NFκB and BAX, respectively, with various fixed bortezomib levels (0≤B≤1), illustrating convergent behaviors of two key intracellular variables. Sharp contrast in expression levels at final time (t=200) in both NFκB and BAX reflects different modes: anti-apoptosis (yellow cube in Figure 3(C)) for low bortezomib levels (0≤B≤0.46) and apoptosis (blue cube in Figure 3(C)) phases for high bortezomib levels (0.46≤B≤1). In Figure 3(F)–(I), we investigate the temporal dynamics of the intracellular module in response to a fluctuating bortezomib stimulus (B(t)=−0.5cos(π500t+0.5)). The concentration of IκB undergoes a sudden increase surpassing that of B(t), and reaches its peak coinciding with that of bortezomib's maximum concentration. Conversely, as B(t) decreases, the concentration of IκB takes a downward course, undergoing an abrupt reduction that surpasses the decline in bortezomib levels. This sequence culminates a cycle of bortezomib-triggered activation and deactivation. The inherent mutual inhibition between IκB and NFκB, as described in Eqs (2.1) and (2.2), gives rise to a pivotal dynamic. Specifically, the upward shift (from down-regulation to up-regulation) of IκB during the initial stimulus phase (0≤t≤500) induces a downward transition (from up-regulation to down-regulation) in NFκB and brings about an upward transition (from down-regulation to up-regulation) in BAX. This orchestrated sequence results in a significant phenotypic switch, steering the system from an anti-apoptosis state to an apoptosis mode (as depicted in Figure 3(G)).
Bortezomib has been demonstrated to induce cancer cell elimination through diverse mechanisms, including the suppression of the NFκB pathway. Furthermore, it effectively inhibits the NFκB-mediated tumor growth enhancement by suppressing the degradation of IκB, thereby manifesting its multifaceted antitumor effects [97,98,99,100,101]. Thus, bortezomib can successfully induce apoptotic death of cancer cells in the absence of OV as illustrated in Figure 3(F)–(H) and corroborated by experimental evidences [97,98,99,100,101]. As the value of B(t) is reduced during the second half of the period (500≤t≤1000), the backward switch (NFκB (low→high); BAX (high→low)) follows, leading to the reverse (Ta→Tt) transition (Figure 3(G)). This loop of forward and backward transitions with initial (red asterisk) and mid point (blue circle) on the trajectory curve is shown in Figure 3(H). This illustrates the dynamic movement of concentrations of key intracellular molecules in the bifurcation diagram, forming a loop of that characterizes a cell death program (Tt→Ta→Tt, Figure 3(H)), in response to a fluctuating bortezomib stimulus (Figure 3(I)).
In Figure 4, we investigate the effect of anti-NFκB antibodies on the dynamic progression of apoptotic cell death within cancer cells. These antibodies serve as valuable tools to study the activation and regulation of NFκB signaling pathways in various biological contexts, including cancer, inflammation, and immune responses. In therapeutic applications, targeting NFκB with specific antibodies may have potential applications in treating diseases characterized by aberrant NFκB activity, such as certain autoimmune disorders and cancers [102,103]. Figure 4(A)–(C) depict the steady states of intracellular variables (NFκB, BAX, and RIP1) for various bortezomib stimuli in the absence (Figure 4(A), D=0) and presence (D=0.45 in Figure 4(B) and D=1 in Figure 4(C)) of anti-NFκB drug in the system. The results demonstrate that the area of apoptosis expands as the dose of NFκB antibody increase from 0 to 1. This suggests that apoptosis can be induced even with a small amount of bortezomib, and its effect becomes more prominent as the dose of NFκB antibody increases. Figure 4(D), (E) show the time courses of concentrations of NFκB (Figure 4(D)) and BAX (Figure 4(E)) in response to various drug levels (0≤D≤1) when B=0.1 with nitial condition S(0)=0,F(0)=4.5,A(0)=0.5,R(0)=0.7. Figure 4(G), (H) illustrate the time courses of concentrations of NFκB (Figure 4(G)) and BAX (Figure 4(H)) in response to various drug levels (0≤D≤1) when B=0.1 with initial condition S(0)=5,F(0)=0.5,A(0)=4.5,R(0)=0.7. Figure 4(F) depicts the trajectories of solutions in the F−A plane when D=0.45,B=0.1. Initial conditions used for the red curve are S(0)=1.7,F(0)=0.82,A(0)=0.5,R(0)=0.7 while for the blue curve are S(0)=1.7,F(0)=0.83,A(0)=0.5,R(0)=0.7. The bistability region exist when the level of NFκB antibody is intermediate. Indeed, near the bistable point, small changes in the initial values can lead to convergence in opposite directions, resulting to different treatment outcomes. Therefore, when proposing a treatment strategy in the early stages, it is crucial to carefully consider the condition of intracellular signaling. The sensitivity to initial conditions near the bistable point highlights the need for precision and thorough analysis when designing therapeutic interventions to achieve the desired treatment outcomes. Figure 4(I) shows the characterization of apoptosis, bistability, anti-apoptosis regions in the B−D plane.
Within oncolytic virotherapy (OV therapy), the mere presence of active OVs proves adept at eradicating cancer cells through oncolysis, that avoids the initiation of necroptosis signaling pathways, even in the absence of bortezomib. Intriguingly, empirical evidence has illuminated the amalgamation of therapies, wherein OVs and bortezomib are combined, induces a synergistic impact, notably enhancing the efficacy of cancer cell eradication [6,43]. We investigate the temporal dynamics of intracellular variables in Eqs (2.1)–(2.4) in response to fluctuating OV supply in the absence and presence of bortezomib. In response to increasing OV input described by v(t)=−225cosπ100t+0.0008t+0.28 (Figure 5(A)), the system starting from the same apoptotic status (red asterisks in Figure 5(B), (C)) converges to oncolytic phenotype (blue asterisk in Figure 5(B)) and necroptotic mode (blue asterisk in Figure 5(C)) in the absence (B=0) and presence (B=1) of bortezomib, respectively. In other words, the typical apoptotic cell death program in response to bortezomib transits to more severe cell death program, i.e., necroptosis (red asterisk → blue asterisk in Figure 5(C)), when oncolytic virus is present in the tumor microenvironment. The phenotypic transition between apoptosis and necroptosis is bidirectional. When OV stimulus fluctuates according to v(t)=−12cosπ250t+0.5, the initial curve in v(t) leads to the first transition from the apoptotic mode (red asterisk in Figure 5(F)) to the necroptotic mode (green circle in Figure 5(F)) but it is followed by back-transition to the apoptotic mode (blue triangle in Figure 5(F)) in response to the declining OV level. The second wave of OV stimuli also induces a loop of transition series Ta→Tn→Ta by the forward and backward movements.
The fundamental structures of four phenotypic modes in response to various bortezomib levels in the absence and presence of OVs are represented in Figure 3. Here, we further scrutinized the fundamental structures of four phenotypic modes, discerning their responses to diverse levels of oncolytic viruses in both the absence and presence of bortezomib. Specifically, Figure 6 illustrates the steady states of three key intracellular variables (NFκB, BAX, and RIP1). These plots delineate the behavior of these variables under varying [oHSV] conditions in the absence (B=0 in Figure 6(A)) and presence (B=1 in Figure 6(B)) of bortezomib.
NFκB consistently sustains high levels irrespective of [oHSV], while the BAX level remains consistently low (Figure 6(A)). However, the level of RIP1 increases as the [oHSV] increases. This particular state signifies the activation of oncolysis signaling (depicted within the green box in Figure 6(A)) under conditions of elevated [oHSV] levels. Conversely, when [oHSV] stimulation is limited, the cell death program is not initiated, leading to the anti-apoptosis phenotype (illustrated by the yellow box in Figure 6(A)). However, the intracellular dynamics of NFκB, BAX, and RIP1 undergoes a significant transformation in the presence of bortezomib in response to [oHSV] stimuli. In the presence of bortezomib within the system, we observe elevated levels of BAX, coupled with low levels of both NFκB and RIP1 in response to lower levels of [oHSV] (Figure 6(B)). This dynamic pattern prevents the initiation of bortezomib-induced apoptosis, as the key apoptotic gene, BAX, remains suppressed. As the concentration of [oHSV] increases, we have a dramatic shift: NFκB and RIP1 are up-regulated, while the level of BAX remains low, leading anti-apoptosis (yellow box in Figure 6(B)). As the [oHSV] increases further, the RIP1 level transits to an overexpression state, triggering necroptosis (pink box in Figure 6(B)).
In the main text model, certain parameters lack experimental data and may influence simulation results. We considered all parameters in the intracellular signaling (B, v, λB, α, σ1, σ9, σ4, σ7, σ2, σ10, σ5, ω1, σ8, σ3, σ11, σ6, ω2, σ12, σ13, ω3) for sensitivity analysis. We examined the sensitivity of intracellular molecules' activities (IκB, NFκB, BAX, and RIP1) at t = 0.1, 1, 10,100 to these parameters. A range was selected for each parameter, divided into 10,000 intervals of uniform length. For each of the 20 parameters of interest, a partial rank correlation coefficient (PRCC) value was calculated. PRCC values range between -1 and 1, with the sign determining whether an increase in the parameter value will decrease (-) or increase (+) activities of the intracellular dynamics at a given time. The sensitivity analysis, as described below, followed the method outlined in [104]. In Figure 7, The BAX (activities of apoptosis inducer) level is positively correlated to ω1, σ8, σ3, σ11 but negatively correlated to ω2. In a similar fashion, the RIP1 (activities of necroptosis or oncolysis inducer) activity is positively correlated to σ7, σ2, σ10, σ12, σ13 but negatively correlated to ω1, ω3. These results are summarized in Table 2.
Parameter | B | v | λB | α | σ1 | σ9 |
PRCC(S, 0.1 h) | 0.28187 | −0.03828 | 0.26953 | −0.33367 | 0.94918 | 0.50929 |
PRCC(F, 0.1 h) | −0.02305 | 0.01961 | −0.02248 | 0.04351 | −0.27969 | −0.04106 |
PRCC(A, 0.1 h) | 0.00822 | 0.00416 | −0.01449 | −0.01347 | −0.01005 | −0.00355 |
PRCC(R, 0.1 h) | −0.00527 | 0.13183 | −0.00513 | 0.02775 | −0.06686 | −0.01338 |
PRCC(S, 1 h) | 0.26056 | −0.02550 | 0.25868 | −0.29694 | 0.82422 | 0.73676 |
PRCC(F, 1 h) | −0.07284 | 0.02242 | −0.08059 | 0.09171 | −0.48167 | −0.32707 |
PRCC(A, 1 h) | 0.02008 | 0.00165 | 0.01746 | −0.02042 | 0.11314 | 0.05378 |
PRCC(R, 1 h) | −0.03627 | 0.11745 | −0.04266 | 0.06355 | −0.27849 | −0.14751 |
PRCC(S, 10 h) | 0.37318 | −0.03499 | 0.35211 | −0.35990 | 0.55805 | 0.62897 |
PRCC(F, 10 h) | −0.09981 | 0.02151 | −0.10770 | 0.12735 | −0.32377 | −0.35500 |
PRCC(A, 10 h) | 0.04337 | 0.00249 | 0.04355 | −0.04101 | 0.16069 | 0.14905 |
PRCC(R, 10 h) | −0.06094 | 0.07032 | −0.07396 | 0.10494 | −0.23546 | −0.24755 |
PRCC(S, 100 h) | 0.38143 | −0.03454 | 0.36085 | −0.36390 | 0.51007 | 0.58888 |
PRCC(F, 100 h) | −0.09180 | 0.01882 | −0.10493 | 0.12160 | −0.27286 | −0.30714 |
PRCC(A, 100 h) | 0.04653 | −0.00022 | 0.02574 | −0.01787 | 0.11811 | 0.10076 |
PRCC(R, 100 h) | −0.04955 | 0.05732 | −0.06608 | 0.09572 | −0.17990 | −0.19896 |
Minimum | 0 | 0 | 0.19 | 3 | 0.4 | 0.1 |
Baseline | 0.5 | 0.01 | 1.9 | 30 | 4 | 1 |
Maximum | 5 | 0.1 | 5.7 | 60 | 8 | 5 |
Parameter | σ4 | σ7 | σ2 | σ10 | σ5 | ω1 |
PRCC(S, 0.1 h) | −0.17767 | −0.06597 | −0.24638 | −0.03404 | −0.00584 | 0.02214 |
PRCC(F, 0.1 h) | 0.00846 | 0.63726 | 0.96485 | 0.53649 | −0.19325 | −0.15541 |
PRCC(A, 0.1 h) | −0.01288 | −0.03460 | −0.19312 | −0.03172 | −0.00515 | 0.00624 |
PRCC(R, 0.1 h) | 0.02074 | 0.26024 | 0.79329 | 0.14504 | −0.05250 | −0.04657 |
PRCC(S, 1 h) | −0.40421 | −0.20533 | −0.50535 | −0.30275 | 0.12012 | 0.16696 |
PRCC(F, 1 h) | 0.12788 | 0.52405 | 0.85069 | 0.68899 | −0.35946 | −0.55150 |
PRCC(A, 1 h) | −0.02169 | −0.16545 | −0.45533 | −0.24162 | 0.08285 | 0.14085 |
PRCC(R, 1 h) | 0.06123 | 0.32027 | 0.72956 | 0.46364 | −0.19797 | −0.28509 |
PRCC(S, 10 h) | −0.36729 | −0.27416 | −0.40630 | −0.34399 | 0.15168 | 0.53178 |
PRCC(F, 10 h) | 0.18956 | 0.49155 | 0.67193 | 0.55311 | −0.26960 | −0.81199 |
PRCC(A, 10 h) | −0.06678 | −0.25292 | −0.36120 | −0.29522 | 0.11705 | 0.42062 |
PRCC(R, 10 h) | 0.13050 | 0.35427 | 0.54956 | 0.43078 | −0.19729 | −0.69605 |
PRCC(S, 100 h) | −0.33893 | −0.24777 | −0.38422 | −0.30025 | 0.12914 | 0.56675 |
PRCC(F, 100 h) | 0.17244 | 0.45223 | 0.63376 | 0.50030 | −0.23642 | −0.82047 |
PRCC(A, 100 h) | −0.06158 | −0.17745 | −0.25095 | −0.20439 | 0.06632 | 0.38276 |
PRCC(R, 100 h) | 0.11273 | 0.30119 | 0.48277 | 0.35914 | −0.15850 | −0.70620 |
Minimum | 0.22 | 0.007 | 0.133 | 0.1 | 0.1 | 0.03 |
Baseline | 2.2 | 0.07 | 1.33 | 1 | 1 | 0.3 |
Maximum | 6.6 | 1.4 | 6.65 | 5 | 5 | 1.5 |
Parameter | σ8 | σ3 | σ11 | σ6 | ω2 | σ12 |
PRCC(S, 0.1 h) | 0.00418 | 0.00133 | 0.00721 | −0.00730 | 0.00880 | −0.00095 |
PRCC(F, 0.1 h) | 0.00491 | −0.02376 | −0.00479 | −0.01447 | 0.00070 | 0.00029 |
PRCC(A, 0.1 h) | 0.94584 | 0.94967 | 0.43179 | −0.14146 | −0.02909 | 0.01632 |
PRCC(R, 0.1 h) | −0.00100 | −0.00278 | 0.00123 | 0.00560 | 0.00341 | 0.99028 |
PRCC(S, 1 h) | −0.00087 | 0.00042 | −0.00427 | 0.00687 | 0.00177 | 0.00779 |
PRCC(F, 1 h) | 0.00618 | −0.00739 | 0.00563 | −0.01924 | 0.00263 | −0.00287 |
PRCC(A, 1 h) | 0.91506 | 0.83297 | 0.64729 | −0.35019 | −0.17798 | 0.00315 |
PRCC(R, 1 h) | 0.00334 | −0.01925 | 0.00145 | 0.00094 | 0.00290 | 0.71206 |
PRCC(S, 10 h) | −0.00308 | −0.01061 | −0.00714 | 0.00995 | −0.01861 | 0.01484 |
PRCC(F, 10 h) | 0.01215 | −0.00269 | 0.00844 | −0.01508 | 0.00412 | −0.01259 |
PRCC(A, 10 h) | 0.88966 | 0.56291 | 0.46346 | −0.27714 | −0.70996 | 0.00142 |
PRCC(R, 10 h) | 0.01655 | −0.01123 | 0.00754 | −0.01035 | −0.00245 | 0.30818 |
PRCC(S, 100 h) | −0.00690 | −0.01292 | −0.00574 | 0.00764 | −0.01664 | 0.01243 |
PRCC(F, 100 h) | 0.01736 | 0.00037 | 0.00739 | −0.01191 | 0.00101 | −0.01150 |
PRCC(A, 100 h) | 0.81841 | 0.37472 | 0.30075 | −0.17214 | −0.87775 | −0.00371 |
PRCC(R, 100 h) | 0.02093 | −0.00869 | 0.00635 | −0.01199 | −0.00584 | 0.25393 |
Minimum | 0.00033 | 0.0111 | 0.1 | 0.1 | 0.002 | 0.01 |
Baseline | 0.0033 | 0.111 | 1 | 1 | 0.02 | 0.1 |
Maximum | 0.99 | 1.11 | 5 | 5 | 0.2 | 1 |
Parameter | σ13 | ω3 | ||||
PRCC(S, 0.1 h) | 0.01202 | −0.00260 | ||||
PRCC(F, 0.1 h) | 0.01100 | −0.00583 | ||||
PRCC(A, 0.1 h) | 0.00190 | 0.00014 | ||||
PRCC(R, 0.1 h) | 0.84920 | −0.25007 | ||||
PRCC(S, 1 h) | 0.00730 | 0.00780 | ||||
PRCC(F, 1 h) | 0.01072 | −0.01173 | ||||
PRCC(A, 1 h) | 0.01013 | 0.00761 | ||||
PRCC(R, 1 h) | 0.82148 | −0.49732 | ||||
PRCC(S, 10 h) | 0.00154 | 0.01567 | ||||
PRCC(F, 10 h) | 0.00974 | −0.01014 | ||||
PRCC(A, 10 h) | 0.00512 | 0.01810 | ||||
PRCC(R, 10 h) | 0.68681 | −0.75844 | ||||
PRCC(S, 100 h) | −0.00381 | 0.01276 | ||||
PRCC(F, 100 h) | 0.01531 | −0.00586 | ||||
PRCC(A, 100 h) | 0.00191 | −0.00058 | ||||
PRCC(R, 100 h) | 0.61909 | −0.74965 | ||||
Minimum | 0.013 | 0.0139 | ||||
Baseline | 0.13 | 0.139 | ||||
Maximum | 1.3 | 1.39 |
To investigate the effect of injection sequence, we consider two administration schedules: (ⅰ) Bortezomib + oncolytic-virus (bortezomib first) (Figure 8(A)–(D)) and (ⅱ) oncolytic-virus + Bortezomib (OV first) (Figure 8(E)–(H)). The approach involves partitioning the 30-day interval evenly, allocating 15 days for the first therapeutic administration followed by an additional 15 days for the subsequent treatment. The objective is to discern the therapeutic administration sequence that yields a synergistic effect in the eradication of cancer cells. In these cases, the cumulative amounts of OV and bortezomib are the same (∑t=30t=0uV=0.5 and ∑t=30t=0uB=0.75). Figure 8(A), (B) show the time courses of injection rate and concentration of OV (red curves) and bortezomib (blue curves) of the case where bortezomib is administered first. Figure 8(C) shows the time courses of intracellular signaling (NFκB (F), BAX (A), and RIP1 (R)) in response to bortezomib first administration. Simulations reveal a scenario where cancer cells might elude the apoptotic pathway over the 15-day course of bortezomib administration (as depicted in Figure 8(C)). Notably, the initiation of oncolysis becomes feasible only upon the subsequent implementation of oncolytic virus therapy (as illustrated in Figure 8(D)). This observation prompted to explore an alternative scenario, involving the administration of oncolytic virus (OV) prior to bortezomib. Figure 8(E), (F) display the time courses of injection rate and concentration of OV (red curves) and bortezomib (blue curves) of the OV first administration. Corresponding time course evolutions of intracellular signaling variables (NFκB (F), BAX (A), and RIP1 (R)) are depicted in Figure 8(G). Significantly, the induction of oncolysis ensues as a result of OV administration, subsequently leading to necroptosis following bortezomib administration. Despite the equal administration of OV and bortezomib, distinct profiles emerge within the cancer cell population (depicted in Figure 8(D), (H)) as well as intracellular signaling dynamics (illustrated in Figure 8(C), (G)). In the scenario where bortezomib is administered first, cancer cells can evade the apoptotic phenotype. Conversely, when OV takes precedence in administration before bortezomib, oncolysis followed by necroptosis can be promoted. Consequently, the synergistic effect of these therapeutic agents is achieved by administering OV before bortezomib. However, constant administration of bortezomib and OV, as illustrated in Figure 8, poses challenges within a clinical context, encompassing concerns related to drug toxicity and practical feasibility. Consequently, we intend to explore a scenario in which the administration durations for both OV and bortezomib are confined to a single day, with a sole administration of OV.
We examined a periodic bortezomib administration and a single OV administration within the time interval [0,30]. The 30-day duration is partitioned where OV is introduced for a single day, followed by a 14-day interval of rest. Subsequently, the remaining days are divided into five segments, during which bortezomib is administered five times. Given its inherent replication capacity, OV is administered solely on the initial phase within a 15-day interval. We have examined six distinct injection schedules, comprising a single administration of OV and five instances of bortezomib. The total amount of OV and bortezomib is same as in the constant sequential OV and bortezomib administration above. We denote OV and bortezomib as 'V' and 'B', respectively. For instance, 'BBBBBV' reflects five administration of bortezomib within the first 15 days and one time OV administration in the remaining 15 days. Figure 9(A)–(D) show the time courses of injection rates (uB,uV), concentration of OV and bortezomib, concentration of intracellular signaling variables (NFκB, BAX, and RIP1), and cancer cells (uninfected, infected, and total cancer cells) of the 'BBBBBV', respectively. Observe that in Figure 9(C), there are two phenotypes: apoptosis (blue region) and oncolysis (green region). Remarkably, despite employing an equivalent quantity of bortezomib as seen in the constant sequential administration of OV and bortezomib shown in Figure 8, distinct phenotypic outcomes can be elicited. Hence, administering a substantial quantity separately proves more effective than delivering a smaller dose over an extended period. Figure 9(E)–(H) showcase the outcomes for the 'VBBBBB' scenario, revealing a clear manifestation of the synergistic effect between OV and bortezomib. Upon each bortezomib injection (from day 15th), a rapid increase in the level of OV becomes evident (depicted in Figure 9(F)). This phenomenon arises from the combined action of OV and bortezomib treatment, which induces a necroptotic phenotype and consequently enhances viral infections. As a result, the replication term (second term in Eq (2.8)) contributes to the substantial increase in OV levels. In Figure 9(G), a distinct alternation between necroptosis and oncolysis emerges starting at the 15-day mark, coinciding with bortezomib injections. However, the discernible effect on the cancer cell population remains limited, primarily attributed to the supplementary role played by bortezomib, which acts as an enhancer, while OV alone displays only modest infectivity towards cancer cells (as demonstrated in Figures 8(H) and 9(H)). In the context of single administering OV and five bortezomib injections, an intriguing query arises: Should the OV injection be administered solely at the beginning or the end of bortezomib administration? To address this concern, we strategically place the OV injection between the bortezomib administrations. The temporal dynamics of phenotypic changes corresponding to diverse injection schedules are illustrated in Figure 9(I). Figure 9(I) illustrates the discrete phenotypic states of cancer cells resulting from various injection schedules. Figure 9(J) displays the normalized cancer cell (green: uninfected cancer cells; pink: infected cancer cells; red: total cancer cells (uninfected + infected) outcomes across all scenarios at the final time point. Evidently, the 'BBBBBV' scenario exhibits the least effectiveness in eradicating cancer cells. Conversely, the 'VBBBBB' scenario emerges as the most potent in targeting uninfected cancer cells for elimination. Generally, the strategy commencing with OV demonstrates a superior anti-cancer effect compared to the approach initiated with bortezomib. Nonetheless, an important query arises regarding the optimality of the strategy derived from these findings. Up to this point, the injection timings have been manually set, and the cumulative dosage of anti-cancer drugs has been predetermined. Furthermore, it's noteworthy that the bortezomib dosage surpasses our reference threshold of 1, consequently impacting patient toxicity levels.
As a result, the subsequent section will elucidate how the application of optimal control theory can yield favorable outcomes, further utilizing minimal quantities of OV and bortezomib.
Now, we investigate the optimal control problems for various objective functions in Eqs (2.12) and (2.13). As observed from the previous results, it is evident that administering OV first is significantly more effective compared to administering bortezomib first. The simulation outcomes and analysis support the idea that initiating the treatment with OV leads to superior therapeutic results in combating cancer progression and inducing necroptotic phenotype. This finding can have important implications for designing optimized treatment strategies in clinical settings, prioritizing OV administration for improved efficacy and better patient outcomes. Regarding the objective function, as described in the previous section, we will examine the following results using two different objective functions (Eqs (2.12) and (2.13)). Each objective function represents a distinct therapeutic goal, and by analyzing the outcomes based on these different criteria, we aim to gain comprehensive insights into the effectiveness of the treatment strategies in different scenarios. These objective functions allow us to assess the impact of the interventions from multiple perspectives, providing a more comprehensive evaluation of the treatment outcomes.
1) Quadratic controls
In this therapeutic strategy, we investigate the optimal control problem for objective function Eq (2.12). Figure 10(A), (B) shows the time courses of the injection rate and concentration of OV and bortezomib, respectively. We set that the state stays at necroptotic phenotype after injecting bortezomib (day 15th). That is, the level of bortezomib never falls under the threshold (thB=0.1). In Figure 9(G), necroptotic and oncolytic mode alternately appeared even though the total amount of bortezomib is 0.75. However, with the application of optimal control theory, necroptotic phenotype is maintained from the moment bortezomib is injected (Figure 10(C)). It is worth noting that with optimal control, total amount of bortezomib is just 0.6733. Because we set the upper bound of the control when applying the optimal control theory, the level of bortezomib does not exceed the reference value (Figure 10(B)). However, in this case, one-time OV is coupled with frequent bortezomib administrations, the inconvenience arises from the need for repetitive hospital visits solely for the purpose of bortezomib injections. Hence, we present an alternative approach, incorporating intervals of respite between bortezomib injections (depicted in Figure 10(E)–(H)). This strategy involves a one-time OV coupled with intermittent bortezomib administration. In this latter scenario, the injection intervals are designed, ensuring that the bortezomib dosage threshold will not be exceeded that could potentially lead to toxicity. The total amount of bortezomib, in this case, is calculated at 0.2823, significantly lower by over fifty percent compared to the previous scenario's value of 0.6733. Furthermore, there is a notable reduction in the total amount of OV, diminishing from 0.5 as seen in Figures 8 and 9, to 0.2938 as demonstrated in Figure 10. In the second scenario, the injection strategy of bortezomib shows four injections in 15 days. The possible actual administration of bortezomib could be bolus intravenous injection twice weekly for two weeks (days 1, 4, 8, and 11) followed by a ten-day rest period [105,106]. Hence, our mathematical model utilizing optimal control theory, aligns with the practical drug administration protocols and has the potential to provide valuable insights into optimizing drug utilization within real treatment scenarios.
2) Linear controls with constraint
In this therapeutic approach, we consider the impact of initially administering OV followed by bortezomib, employing a sigmoid function to effectively steer cells toward a necroptotic phenotype. We divided the entire time into two periods: the period of injecting only OV and the period of injecting only bortezomib. In the first integration term (in Eq (2.13)), a term for uninfected cancer cells (x(t)) is added because the main purpose is to infect cancer cells through OV. The objective function of the second integration term (in Eq (2.13)) considers a sigmoid term for bortezomib to keep the cells in the necroptotic phenotype. Figure 11 are the results for Eq (2.13). Figure 11(A), (B) shows the time courses of injection rate (uV and uB) and concentration of OV (v) and bortezomib (B), respectively. Figure 11(C), (D) illustrate the time courses of intracellular signaling (NFκB, BAX, RIP1) and cancer cell population (uninfected, infected, and total cancer cells), respectively. Bortezomib continues to maintain a level that satisfies the necroptotic phenotype (blue curves in Figure 11(B)). As a result, after 15 days, the necroptosis phenotype is maintained and more cancer cells can be infected (Figure 11(C), (D)). The mechanism of quadratic controls necessitates the dynamic adjustment of infusion rates over time, as both uV and uB exhibit continuous dynamics when the necessary conditions for optimal control are computed. In contrast, linear controls result to either 0 or the maximum injection rate which is due to the calculation of necessary conditions in the optimal control formulation. Consequently, linear controls provide practical administration protocol as it do not require temporal adjustments to the injection rate.
The optimal control framework herein is based on the mathematical models developed by [45,46] describing the intracellular dynamics and tumor suppression in response to a combination therapy involving bortezomib and OV in cancer patients. While bortezomib is quite effective in killing cancer cells in general, it can cause strong toxicity and serious side effects. One of our goals of this study is to analyze the cellular system behaviors in response to bortezomib-OV combination therapy via a delicate intracellular signaling pathway (NFkB-Bcl-2-BAX-RIP1). Another goal is to find optimal strategies that effectively kill cancer cells by OV and bortezomib through both apoptotic and necroptotic phenotype with minimal costs (side effects + administrative costs) using optimal control theory [46]. The associated optimal control problems are formulated such that the overall cancer cell population and the total cost of the two anticancer drugs are minimized.
Yoo et al. showed that OV replication, thus anti-tumor efficacy, can be improved by the unfolded protein response (UPR) after bortezomib treatment, inducing synergistic cancer cell killing [6]. Kim et al. [46] introduced the apoptosis and necroptosis cell death mechanism involved in this combination (OV + bortezomib) therapy. In this work, a detailed analysis of the model in this work suggests that injection of OVs followed by bortezomib can effectively increase anti-tumor efficacy due to the higher infection rate of cancer cells from the oncolytic mode and superior killing capacity of tumor cells from combined cell death (necroptosis + oncolysis) phase while the OV treatment at a latter time induces only oncolysis or a mild killing phase (apoptosis + oncolysis), leading to lower killing rates (Figures 8 and 9). Treatment of a tumor with OVs at an earlier time usually leads to better anti-tumor efficacy [6] and our study supports the idea of effectiveness of initial OV treatment even in the combination therapy by revealing the dynamics of detailed sub-cellular cell death program at an intracellular level. Relative order of OVs relative to bortezomib treatment in a combination therapy induces the cellular transition from the severe ON (oncolysis + necroptosis) mode to the intermediate AON phase (apoptosis + oncolysis + necroptosis), and to mild AO (apoptosis + oncolysis) phase (Figure 9). Therefore, delays in OV treatment decrease anti-tumor efficacy, suggesting the importance of OV injection time. Excessive use of OVs and bortezomib also has to be limited due to safety issues and drug-associated side effects, respectively, in addition to a need to minimize administrative costs. Anti-cancer strategies with optimal control in our work suggest that both the necroptosis-dominant phase from frequent injection of bortezomib and a mixed (necroptosis + oncolysis) mode from less frequent injection mode of bortezomib can effectively kill cancer cells in the 2nd cell death program a combination therapy with initial administration of OVs (Figures 10 and 11). Optimally controlled injection strategy of OVs and bortezomib in this work may lead to better understanding of fundamental mechanisms of the cell death program and effective anti-cancer efficacy in various cancers [105,106].
The authors declare they have not used Artificial Intelligence (AI) tools in the creation of this article.
This work was supported by the National Research Foundation of Korea (NRF) grant funded by the Korea government (MSIT) (No. NRF-2021R1A2C1010891) (Y.K.).
The authors declare there is no conflict of interest.
[1] |
Kumar N, Panda RK (2019) Place branding and place marketing: a contemporary analysis of the literature and usage of terminology. Int Rev Publ Nonprofit Mark 16: 255–292. https://doi.org/10.1007/s12208-019-00230-6 doi: 10.1007/s12208-019-00230-6
![]() |
[2] |
Swain S, Jebarajakirthy C, Sharma BK, et al. (2024) Place branding: A systematic literature review and future research agenda. J Travel Res 63: 535–564. https://doi.org/10.1177/00472875231168620 doi: 10.1177/00472875231168620
![]() |
[3] |
Mohamed B (2019) Exploring place branding: State of the art and future research directions. China-USA Bus Rev 18: 23–33. https://doi.org/10.17265/1537-1514/2019.02.003 doi: 10.17265/1537-1514/2019.02.003
![]() |
[4] |
Vela J de SE (2013) Fundamentos conceptuales y teóricos para marcas de territorio. Boletín de la Asociación de Geógrafos españoles. https://doi.org/10.21138/bage.1575 doi: 10.21138/bage.1575
![]() |
[5] |
Anholt S (2008) Place branding: Is it marketing, or isn't it? Place Branding Publ Diplomacy 4: 1–6. https://doi.org/10.1057/palgrave.pb.6000088 doi: 10.1057/palgrave.pb.6000088
![]() |
[6] | Eraydın Z (2014) The global image of the city: Impacts of place branding on the image of Ankara. A thesis submitted to the graduate school of natural and applied sciences of Middle East Technical University. |
[7] |
Horlings LG (2012) Place branding by building coalitions; lessons from rural–urban regions in the Netherlands. Place Branding Publ Diplomacy 8: 295–309. https://doi.org/10.1057/pb.2012.21 doi: 10.1057/pb.2012.21
![]() |
[8] |
Inkinen T, Heikkonen M, Makkonen T, et al. (2024) Multilayered spatial categories in tourism marketing and branding. J Destination Mark Manage 31: 100867. https://doi.org/10.1016/j.jdmm.2024.100867 doi: 10.1016/j.jdmm.2024.100867
![]() |
[9] |
Zhang S, Sun Y, Yu X, et al. (2023) Geographical indication, agricultural products export and urban–rural income gap. Agriculture 13: 378. https://doi.org/10.3390/agriculture13020378 doi: 10.3390/agriculture13020378
![]() |
[10] |
Kavaratzis M, Hatch MJ (2013) The dynamics of place brands: An identity-based approach to place branding theory. Marketing Theory 13: 69–86. https://doi.org/10.1177/1470593112467268 doi: 10.1177/1470593112467268
![]() |
[11] |
Oguztimur S, Akturan U (2016) Synthesis of city branding literature (1988–2014) as a research domain. Int J Tourism Res 18: 357–372. https://doi.org/10.1002/jtr.2054 doi: 10.1002/jtr.2054
![]() |
[12] |
Lucarelli A, Olof Berg P (2011) City branding: a state‐of‐the‐art review of the research domain. J Place Manage Dev 4: 9–27. https://doi.org/10.1108/17538331111117133 doi: 10.1108/17538331111117133
![]() |
[13] |
Gulisova B, Horbel C, Bjørnshave Noe E (2023) Rural place branding processes: actor engagement in service ecosystems. J Place Manage Dev 16: 415–439. https://doi.org/10.1108/jpmd-11-2022-0105 doi: 10.1108/jpmd-11-2022-0105
![]() |
[14] | OECD (2024) The new rural paradigm. In OECD Rural Policy Reviews. https://doi.org/10.1787/9789264023918-en |
[15] |
Liu Y, Li Y (2017) Revitalize the world's countryside. Nature 548: 275–277. https://doi.org/10.1038/548275a doi: 10.1038/548275a
![]() |
[16] |
Shen J, Chou R-J (2022) Rural revitalization of Xiamei: The development experiences of integrating tea tourism with ancient village preservation. J Rural Stud 90: 42–52. https://doi.org/10.1016/j.jrurstud.2022.01.006 doi: 10.1016/j.jrurstud.2022.01.006
![]() |
[17] |
Vuorinen M, Vos M (2013) Challenges in joint place branding in rural regions. Place Branding Publ Diplomacy 9: 154–163. https://doi.org/10.1057/pb.2013.18 doi: 10.1057/pb.2013.18
![]() |
[18] |
Mittilä T, Lepistö T (2013) The role of artists in place branding: A case study. Place Branding Publ Diplomacy 9: 143–153. https://doi.org/10.1057/pb.2013.15 doi: 10.1057/pb.2013.15
![]() |
[19] |
Gulisova B (2021) Rural place branding processes: A meta-synthesis. Place Branding Publ Diplomacy 17: 368–381. https://doi.org/10.1057/s41254-020-00187-y doi: 10.1057/s41254-020-00187-y
![]() |
[20] |
Rinaldi C (2017) Food and gastronomy for sustainable place development: A multidisciplinary analysis of different theoretical approaches. Sustainability 9: 1748. https://doi.org/10.3390/su9101748 doi: 10.3390/su9101748
![]() |
[21] |
Rovira M, Garay L, Górriz-Mifsud E, et al. (2022) Territorial marketing based on non-wood Forest products (NWFPs) to enhance sustainable tourism in rural areas: A literature review. Forests 13: 1231. https://doi.org/10.3390/f13081231 doi: 10.3390/f13081231
![]() |
[22] |
Andersson I (2014) Placing place branding: an analysis of an emerging research field in human geography. Geografisk Tidsskrift-Danish J Geo 114: 143–155. https://doi.org/10.1080/00167223.2014.895954 doi: 10.1080/00167223.2014.895954
![]() |
[23] |
Zenker S (2024) Size does matter: City branding versus small city, town, and rural place branding. Place Branding Publ Diplomacy 20: 40–43. https://doi.org/10.1057/s41254-024-00379-w doi: 10.1057/s41254-024-00379-w
![]() |
[24] |
Ramezi M, Yeganeh M, Bemanian M (2022) The role of place branding in promoting social capital in urban areas (case study: Ahvaz, Iran). Front Built Environ 8: 889139. https://doi.org/10.3389/fbuil.2022.889139 doi: 10.3389/fbuil.2022.889139
![]() |
[25] |
Clark J, Rice G (2020) Revitalising rural Scotland: Loch Fyne, branding and belonging. J Place Manage Dev 13: 18–29. https://doi.org/10.1108/jpmd-06-2019-0044 doi: 10.1108/jpmd-06-2019-0044
![]() |
[26] |
Tobias S, Wahl PM (2013) Can place branding support landscape conservation in city-regions? A case study from Switzerland. Land Use Policy 30: 266–275. https://doi.org/10.1016/j.landusepol.2012.03.021 doi: 10.1016/j.landusepol.2012.03.021
![]() |
[27] |
Alsayel A, Fransen J, de Jong M (2023) City branding in a multi-level governance context: comparing branding performance across five institutional models for urban development in Saudi Arabia. J Place Manage Development 16: 267–290. https://doi.org/10.1108/jpmd-07-2022-0061 doi: 10.1108/jpmd-07-2022-0061
![]() |
[28] |
Moilanen T, Rainisto SK (2009) How to brand nations, cities and destinations. A planning book for place branding. Springer. https://doi.org/10.1057/9780230584594 doi: 10.1057/9780230584594
![]() |
[29] |
Mbah M, Franz A (2021) Revitalization and branding of rural communities in Cameroon using a circular approach for sustainable development—A proposal for the Batibo Municipality. Sustainability 13: 6908. https://doi.org/10.3390/su13126908 doi: 10.3390/su13126908
![]() |
[30] |
Logar E (2022) Place branding as an approach to the development of rural areas: A case study of the brand» Babica in Dedek «from the Škofja Loka Hills, Slovenia. Acta geographica Slovenica 62: 120–133. https://doi.org/10.3986/ags.10883 doi: 10.3986/ags.10883
![]() |
[31] | Kavaratzis M, Braun E, Zenker S (2010) My city—my brand: The role of residents in place branding. In: 50th European Regional Science Association Congress, Jönköping, 19–23. |
[32] |
Braun E, Kavaratzis M, Zenker S (2013) My city–my brand: The different roles of residents in place branding. J Place Manage Dev 6: 18–28. https://doi.org/10.1108/17538331311306087 doi: 10.1108/17538331311306087
![]() |
[33] |
Aasetre J, Carlsson E, Haugum M (2020) Developing local community: Municipal policies oriented towards place branding. Scand J Publ Adm 24: 43–63. https://doi.org/10.58235/sjpa.v24i2.8617 doi: 10.58235/sjpa.v24i2.8617
![]() |
[34] |
Gulisova B, Horbel C, Noe E (2021) Rural place branding from a multi-level perspective: A Danish example. Place Branding Publ Diplomacy 17: 231–248. https://doi.org/10.1057/s41254-021-00204-8 doi: 10.1057/s41254-021-00204-8
![]() |
[35] |
Chen M, Long Y (2024) Empowering Rural Revitalization: Unleashing the Potential of E-commerce for Sustainable Industrial Integration. J Knowl Econ 15: 18813–18831. https://doi.org/10.1007/s13132-024-01813-3 doi: 10.1007/s13132-024-01813-3
![]() |
[36] |
Manoharan AP, Hohensinn L, Wu HC (2024) Public branding in the digital age: An empirical investigation of US cities. Int J Publ Adm 1–12. https://doi.org/10.1080/01900692.2024.2311385 doi: 10.1080/01900692.2024.2311385
![]() |
[37] |
Lee AH, Wall G, Kovacs JF (2015) Creative food clusters and rural development through place branding: Culinary tourism initiatives in Stratford and Muskoka, Ontario, Canada. J Rural Stud 39: 133–144. https://doi.org/10.1016/j.jrurstud.2015.05.001 doi: 10.1016/j.jrurstud.2015.05.001
![]() |
[38] |
Snyder H (2019) Literature review as a research methodology: An overview and guidelines. J Bus Res 104: 333–339. https://doi.org/10.1016/j.jbusres.2019.07.039 doi: 10.1016/j.jbusres.2019.07.039
![]() |
[39] | PRISMA (2024) Available from: http://www.prisma-statement.org/. |
[40] |
Moher D, Shamseer L, Clarke M, et al. (2015) Preferred reporting items for systematic review and meta-analysis protocols (PRISMA-P) 2015 statement. Syst Rev 4: 1–9. https://doi.org/10.1186/2046-4053-4-1 doi: 10.1186/2046-4053-4-1
![]() |
[41] |
Page MJ, McKenzie JE, Bossuyt PM, et al. (2021) The PRISMA 2020 statement: An updated guideline for reporting systematic reviews. J Clin Epidemiol 134: 103–112. https://doi.org/10.1016/j.jclinepi.2021.02.003 doi: 10.1016/j.jclinepi.2021.02.003
![]() |
[42] | Briner RB, Denyer D (2012) Chapter 7—Systematic review and evidence synthesis as a practice and scholarship tool. In: The Oxford Handbook of Evidence-Based Management, 112–129. https://doi.org/10.1093/oxfordhb/9780199763986.013.0007 |
[43] | Chadegani AA, Salehi H, Yunus MM, et al. (2013) A comparison between two main academic literature collections: Web of Science and Scopus databases. arXiv preprint arXiv: 13050377. 9: 18–26. https://doi.org/10.5539/ass.v9n5p18 |
[44] |
Singh VK, Singh P, Karmakar M, et al. (2021) The journal coverage of Web of Science, Scopus and Dimensions: A comparative analysis. Scientometrics 126: 5113–5142. https://doi.org/10.1007/s11192-021-03948-5 doi: 10.1007/s11192-021-03948-5
![]() |
[45] |
Carrión-Mero P, Montalván-Burbano N, Paz-Salas N, et al. (2020) Volcanic geomorphology: a review of worldwide research. Geosciences 10: 347. https://doi.org/10.3390/geosciences10090347 doi: 10.3390/geosciences10090347
![]() |
[46] |
Bornmann L, Haunschild R, Hug SE (2018) Visualizing the context of citations referencing papers published by Eugene Garfield: A new type of keyword co-occurrence analysis. Scientometrics 114: 427–437. https://doi.org/10.1007/s11192-017-2591-8 doi: 10.1007/s11192-017-2591-8
![]() |
[47] | Araújo T, Abreu A, Louçã F (2023) The evolution of Complexity co-occurring keywords: bibliometric analysis and network approach. arXiv preprint arXiv: 230800992. Advances in Complex Systems27: NO. 04n05. https://doi.org/10.1142/s0219525924500061 |
[48] | VOSviewer (2024) Visualizing Scientific Landscapes. Available from: https://www.vosviewer.com/. |
[49] |
Hagen L, Keller TE, Yerden X, et al. (2019) Open data visualizations and analytics as tools for policy-making. Gov Inf Q 36: 101387. https://doi.org/10.1016/j.giq.2019.06.004 doi: 10.1016/j.giq.2019.06.004
![]() |
[50] |
Moral-Muñoz JA, Herrera-Viedma E, Santisteban-Espejo A, et al. (2020) Software tools for conducting bibliometric analysis in science: An up-to-date review. Profesional de la Información 29: e290103. https://doi.org/10.3145/epi.2020.ene.03 doi: 10.3145/epi.2020.ene.03
![]() |
[51] |
Bukar UA, Sayeed MS, Razak SFA, et al. (2023) A method for analyzing text using VOSviewer. MethodsX 11: 102339. https://doi.org/10.1016/j.mex.2023.102339 doi: 10.1016/j.mex.2023.102339
![]() |
[52] |
Xu Z, Ge Z, Wang X, et al. (2021) Bibliometric analysis of technology adoption literature published from 1997 to 2020. Technol Forecast Soc Change 170: 120896. https://doi.org/10.1016/j.techfore.2021.120896 doi: 10.1016/j.techfore.2021.120896
![]() |
[53] |
Sang S (2021) Reconstructing the place branding model from the perspective of Peircean semiotics. Ann Tourism Res 89: 103209. https://doi.org/10.1016/j.annals.2021.103209 doi: 10.1016/j.annals.2021.103209
![]() |
[54] |
Senyao S, Ha S (2022) How social media influences resident participation in rural tourism development: A case study of Tunda in Tibet. J Tourism Cultural Change 20: 386–405. https://doi.org/10.1080/14766825.2020.1849244 doi: 10.1080/14766825.2020.1849244
![]() |
[55] |
Vegnuti R (2020) Cinque Terre, Italy-a case of place branding: From opportunity to problem for tourism. Worldwide Hospitality Tourism Themes 12: 471–483. https://doi.org/10.1108/whatt-05-2020-0032 doi: 10.1108/whatt-05-2020-0032
![]() |
[56] |
Jarratt D, Phelan C, Wain J, et al. (2019) Developing a sense of place toolkit: Identifying destination uniqueness. Tourism Hospitality Res 19: 408–421. https://doi.org/10.1177/1467358418768678 doi: 10.1177/1467358418768678
![]() |
[57] |
Jones T, Nagata S, Nakajima M, et al. (2009) Prefectural branding in Japan–Tourism, national parks and the Shinshu brand. Place Branding Publ Diplomacy 5: 192–201. https://doi.org/10.1057/pb.2009.13 doi: 10.1057/pb.2009.13
![]() |
[58] | Lee AH, Wall G, Kovacs J, et al. (2016) Food clusters and creative tourism development: A conceptual framework. The J Rural Community Dev 11: 72–88. |
[59] |
Ducros HB (2017) Confronting sustainable development in two rural heritage valorization models. J Sustainable Tourism 25: 327–343. https://doi.org/10.1080/09669582.2016.1206552 doi: 10.1080/09669582.2016.1206552
![]() |
[60] |
Zhao M, Lu H, Liang J, et al. (2021) Evaluating green resource branding using user-generated content data: The case study of a greenway in eastern Guangzhou, China. Urban Forest Urban Greening 66: 127395. https://doi.org/10.1016/j.ufug.2021.127395 doi: 10.1016/j.ufug.2021.127395
![]() |
[61] |
Cleave E, Arku G (2015) Community branding and brand images in Ontario, Canada. Place Branding Publ Diplomacy 11: 65–82. https://doi.org/10.1057/pb.2014.5 doi: 10.1057/pb.2014.5
![]() |
[62] |
Botschen G, Promberger K, Bernhart J (2017) Brand-driven identity development of places. J Place Manage Dev 10: 152–172. https://doi.org/10.1108/jpmd-07-2016-0051 doi: 10.1108/jpmd-07-2016-0051
![]() |
[63] |
McManus P, Connell J (2014) Putting places on the map? Marketing rural and regional Australia. J Destination Market Manage 3: 105–113. https://doi.org/10.1016/j.jdmm.2014.01.001 doi: 10.1016/j.jdmm.2014.01.001
![]() |
[64] |
Jansson D (2012) Branding Åland, branding Ålanders: Reflections on place identity and globalization in a Nordic archipelago. Place Branding Publ Diplomacy 8: 119–132. https://doi.org/10.1057/pb.2012.3 doi: 10.1057/pb.2012.3
![]() |
[65] |
Basile G, Cavallo A (2020) Rural identity, authenticity, and sustainability in Italian inner areas. Sustainability 12: 1272. https://doi.org/10.3390/su12031272 doi: 10.3390/su12031272
![]() |
[66] | Ilieș G, Ilieș M (2015) Identity based geo-and tourism branding strategies derived from rural Maramureș land (Romania). GeoJ Tourism Geosites 8: 179–186. |
[67] | Kavoura A, Bitsani E (2013) E-branding of rural tourism in Carinthia, Austria. Tourism: An Int Interdisciplinary J 61: 289–312. |
[68] |
Mettepenningen E, Vandermeulen V, Van Huylenbroeck G, et al. (2012) Exploring synergies between place branding and agricultural landscape management as a rural development practice. Soc Ruralis 52: 432–452. https://doi.org/10.1111/j.1467-9523.2012.00575.x doi: 10.1111/j.1467-9523.2012.00575.x
![]() |
[69] |
Lopes C, Leitão J, Rengifo-Gallego J (2022) Place-branded foods with responsible and sustainable management: A la carte serving in regional restaurants. Sustainability 14: 6615. https://doi.org/10.3390/su14116615 doi: 10.3390/su14116615
![]() |
[70] |
Donner M, Horlings L, Fort F, et al. (2017) Place branding, embeddedness and endogenous rural development: Four European cases. Place Branding Publ Diplomacy 13: 273–292. https://doi.org/10.1057/s41254-016-0049-z doi: 10.1057/s41254-016-0049-z
![]() |
[71] |
Moreno-Fernandez S, Castelo-Branco SE-S (2022) Celtic music festivals in Portugal: Europeanisation, Urban Regeneration, and Regional Development. Yearb Tradit Music 54: 99–118. https://doi.org/10.1017/ytm.2022.29 doi: 10.1017/ytm.2022.29
![]() |
[72] |
Domínguez García MD, Horlings L, Swagemakers P, et al. (2013) Place branding and endogenous rural development. Departure points for developing an inner brand of the River Minho estuary. Place Branding Publ Diplomacy 9: 124–140. https://doi.org/10.1057/pb.2013.10 doi: 10.1057/pb.2013.10
![]() |
[73] |
Kuhlman T, Farrington J (2010) What is sustainability? Sustainability 2: 3436–3448. https://doi.org/10.3390/su2113436 doi: 10.3390/su2113436
![]() |
[74] |
Strezov V, Evans A, Evans TJ (2017) Assessment of the economic, social and environmental dimensions of the indicators for sustainable development. Sustainable Dev 25: 242–253. https://doi.org/10.1002/sd.1649 doi: 10.1002/sd.1649
![]() |
[75] |
Rogers DS, Duraiappah AK, Antons DC, et al. (2012) A vision for human well-being: transition to social sustainability. Curr Opin Environ Sustainability 4: 61–73. https://doi.org/10.1016/j.cosust.2012.01.013 doi: 10.1016/j.cosust.2012.01.013
![]() |
[76] |
Barile S, Quattrociocchi B, Calabrese M, et al. (2018) Sustainability and the viable systems approach: opportunities and issues for the governance of the territory. Sustainability 10: 790. https://doi.org/10.3390/su10030790 doi: 10.3390/su10030790
![]() |
[77] |
Gulisova B, Horbel C, Noe E (2021) Place branding and sustainable rural communities: qualitative evidence from rural areas in Denmark. J Strategic Mark 1–22. https://doi.org/10.1080/0965254x.2021.2006274 doi: 10.1080/0965254x.2021.2006274
![]() |
[78] |
Ryan MM, Mizerski K (2010) Place branding for sustainable futures: A case study. Place Branding Publ Diplomacy 6: 49–57. https://doi.org/10.1057/pb.2010.2 doi: 10.1057/pb.2010.2
![]() |
[79] |
Blichfeldt BS, Halkier H (2014) Mussels, tourism and community development: a case study of place branding through food festivals in rural North Jutland, Denmark. Eur Plann Stud 22: 1587–1603. https://doi.org/10.1080/09654313.2013.784594 doi: 10.1080/09654313.2013.784594
![]() |
[80] |
Muñiz-Martinez N, Florek M (2023) Food-based place branding as holistic place ecosystems: The case of Basque Gastronomic Ecosystem. Place Branding Publ Diplomacy 19: 155–166. https://doi.org/10.1057/s41254-021-00222-6 doi: 10.1057/s41254-021-00222-6
![]() |
[81] |
Bowen R, Bennett S (2020) Selling places: a community-based model for promoting local food. The case of Rhondda Cynon Taf. J Place Manage Dev 13: 215–228. https://doi.org/10.1108/jpmd-10-2018-0081 doi: 10.1108/jpmd-10-2018-0081
![]() |
[82] |
Ruban DA, Ermolaev VA (2021) Readiness of food producers to reflect regions in their names and trademarks: a perspective for regional branding with sunflower oil. Helia 44: 89–100. https://doi.org/10.1515/helia-2020-0026 doi: 10.1515/helia-2020-0026
![]() |
[83] |
Bisani S, Daye M, Mortimer K (2022) Multi-stakeholder perspective on the role of universities in place branding. J Place Manage Dev 15: 112–129. https://doi.org/10.1108/jpmd-05-2020-0039 doi: 10.1108/jpmd-05-2020-0039
![]() |
[84] | Wachter M, Wytrzens K (2016) The impact of a local thematic focus on community building activities in rural villages. Erschienen im Jahrbuch der osterreichischen Gesellschaft fur Agrarokonomie. |
[85] |
Muñiz Martínez N (2016) Towards a network place branding through multiple stakeholders and based on cultural identities: The case of "The Coffee Cultural Landscape" in Colombia. J Place Manage Dev 9: 73–90. https://doi.org/10.1108/jpmd-11-2015-0052 doi: 10.1108/jpmd-11-2015-0052
![]() |
[86] | Cavicchi A, Rinaldi C, Corsi M (2013) Higher education institutions as managers of wicked problems: place branding and rural development in Marche Region, Italy. Int Food Agribus Manage Rev 16: 51–68. |
[87] |
de San Eugenio-Vela J, Barniol-Carcasona M (2015) The relationship between rural branding and local development. A case study in the Catalonia's countryside: Territoris Serens (El Lluçanès). J Rural Stud 37: 108–119. https://doi.org/10.1016/j.jrurstud.2015.01.001 doi: 10.1016/j.jrurstud.2015.01.001
![]() |
[88] | Mabillard V, Pasquier M, Vuignier R (2024) Place branding and marketing from a policy perspective: Building effective strategies for places. Taylor Francis, London: Routledge, 352. https://doi.org/10.4324/9781003286189 |
1. | Muhammad Khan, Xiuting Huang, Xiaoxin Ye, Donghui Zhang, Baiyao Wang, Anan Xu, Rong Li, Anbang Ren, Chengcong Chen, Jingjing Song, Rong Zheng, Yawei Yuan, Jie Lin, Necroptosis-based glioblastoma prognostic subtypes: implications for TME remodeling and therapy response, 2024, 56, 0785-3890, 10.1080/07853890.2024.2405079 | |
2. | Donggu Lee, Sunju Oh, Sean Lawler, Yangjin Kim, Bistable dynamics of TAN-NK cells in tumor growth and control of radiotherapy-induced neutropenia in lung cancer treatment, 2025, 22, 1551-0018, 744, 10.3934/mbe.2025028 |
Par | Description | Values | Ref |
Intracellular dynamics | |||
λB | Bortezomib signaling scaling factor | 1.9 | [46] |
α | Inhibition strength of bortezomib by oHSV | 30 | [46] |
σ1 | Autocatalytic enhancement rate of IκB | 4 | [46] |
σ9 | Hill-type parameter | 1.0 | [46] |
σ4 | Inhibition strength of IκB by the NFκB-Bcl-2 complex | 2.2 | [46] |
σ7 | Signaling strength of the NFκB-Bcl-2 complex | 0.07 | [46] |
σ2 | Autocatalytic enhancement rate of the NFκB-Bcl-2 complex | 1.33 | [46] |
σ10 | Hill-type parameter | 1.0 | [46] |
σ5 | Inhibition strength of the NFκB-Bcl-2 complex by IκB | 1 | [46] |
ω1 | Decay rate of NFκB-Bcl-2 complex | 0.3 | [107,108] |
σ8 | Signaling strength of BAX | 0.0033 | [46] |
σ3 | Autocatalytic enhancement rate of BAX | 0.111 | [46] |
σ11 | Hill-type parameter | 1.0 | [46] |
σ6 | Inhibition strength of BAX by the NFκB-Bcl-2 complex | 1 | [46] |
ω2 | Decay rate of BAX | 0.02 | [107,108,109] |
σ12 | Signaling strength of RIP1 | 0.1 | [46] |
σ13 | Activation rate of RIP1 in the presence of OVs | 0.13 | [46] |
ω3 | Decay rate of RIP1 | 0.139 | [107,108,110] |
k | Hill type parameter of oHSV switching | 0.01 v∗ | [46] |
Cancer cells | |||
λ | proliferation rate of tumor cells | 4×10−3 | [46,57] |
x0 | Carrying capacity of uninfected tumor cells | 1 | [38,45,46,57] |
β1 | Bortezomib-induced apoptosis rate of tumor cells | 2×10−4 | [46] |
β2 | Oncolysis rate of tumor cells | 3×10−4 | [45,46,57] |
β3 | Necroptosis rate of tumor cells | 5×10−4 | [46] |
δ | infected cell lysis rate | 4.4×10−3 | [45,57] |
μ | Removal rate of dead cells | 2.3×10−3 | [45,46,57] |
Anticancer drugs | |||
b | Burst size of infected cells | 50 | [46,57] |
α1 | bortezomib-induced viral replication rate | 1 | [45,46] |
γ | clearance rate of viruses | 1.8×10−3 | [45,57] |
μ1 | consumption rate of bortezomib by uninfected tumor cells | 0.2075 | [45,46] |
μ2 | consumption rate of bortezomib by infected tumor cells | 0.2075 | [45,46] |
kB | Hill-type parameter | 1 | [45,46] |
μB | decay rate of bortezomib | 0.03 | [46,111,112] |
Reference values of main variables | |||
S∗ | Concentration of IκB | 0.05 μM | [113,114] |
F∗ | Concentration of the NFκB-Bcl2 complex | 0.5 μM | [113,114,115,116] |
A∗ | Concentration of BAX | 0.1 μM | [117] |
R∗ | Concentration of RIP1 | 5.0 μM | [118] |
x∗ | Uninfected cell density | 106cells/mm3 | [45,57,119] |
y∗ | Infected cell density | =x∗ | [45,57,119] |
n∗ | Dead cell density | =x∗ | [45,57,119] |
v∗ | Virus concentration | 2.2×108virus/mm3 | [45,57,119] |
B∗ | Bortezomib concentration | 1.0×10−11g/mm3 | [6,43,45] |
Parameter | B | v | λB | α | σ1 | σ9 |
PRCC(S, 0.1 h) | 0.28187 | −0.03828 | 0.26953 | −0.33367 | 0.94918 | 0.50929 |
PRCC(F, 0.1 h) | −0.02305 | 0.01961 | −0.02248 | 0.04351 | −0.27969 | −0.04106 |
PRCC(A, 0.1 h) | 0.00822 | 0.00416 | −0.01449 | −0.01347 | −0.01005 | −0.00355 |
PRCC(R, 0.1 h) | −0.00527 | 0.13183 | −0.00513 | 0.02775 | −0.06686 | −0.01338 |
PRCC(S, 1 h) | 0.26056 | −0.02550 | 0.25868 | −0.29694 | 0.82422 | 0.73676 |
PRCC(F, 1 h) | −0.07284 | 0.02242 | −0.08059 | 0.09171 | −0.48167 | −0.32707 |
PRCC(A, 1 h) | 0.02008 | 0.00165 | 0.01746 | −0.02042 | 0.11314 | 0.05378 |
PRCC(R, 1 h) | −0.03627 | 0.11745 | −0.04266 | 0.06355 | −0.27849 | −0.14751 |
PRCC(S, 10 h) | 0.37318 | −0.03499 | 0.35211 | −0.35990 | 0.55805 | 0.62897 |
PRCC(F, 10 h) | −0.09981 | 0.02151 | −0.10770 | 0.12735 | −0.32377 | −0.35500 |
PRCC(A, 10 h) | 0.04337 | 0.00249 | 0.04355 | −0.04101 | 0.16069 | 0.14905 |
PRCC(R, 10 h) | −0.06094 | 0.07032 | −0.07396 | 0.10494 | −0.23546 | −0.24755 |
PRCC(S, 100 h) | 0.38143 | −0.03454 | 0.36085 | −0.36390 | 0.51007 | 0.58888 |
PRCC(F, 100 h) | −0.09180 | 0.01882 | −0.10493 | 0.12160 | −0.27286 | −0.30714 |
PRCC(A, 100 h) | 0.04653 | −0.00022 | 0.02574 | −0.01787 | 0.11811 | 0.10076 |
PRCC(R, 100 h) | −0.04955 | 0.05732 | −0.06608 | 0.09572 | −0.17990 | −0.19896 |
Minimum | 0 | 0 | 0.19 | 3 | 0.4 | 0.1 |
Baseline | 0.5 | 0.01 | 1.9 | 30 | 4 | 1 |
Maximum | 5 | 0.1 | 5.7 | 60 | 8 | 5 |
Parameter | σ4 | σ7 | σ2 | σ10 | σ5 | ω1 |
PRCC(S, 0.1 h) | −0.17767 | −0.06597 | −0.24638 | −0.03404 | −0.00584 | 0.02214 |
PRCC(F, 0.1 h) | 0.00846 | 0.63726 | 0.96485 | 0.53649 | −0.19325 | −0.15541 |
PRCC(A, 0.1 h) | −0.01288 | −0.03460 | −0.19312 | −0.03172 | −0.00515 | 0.00624 |
PRCC(R, 0.1 h) | 0.02074 | 0.26024 | 0.79329 | 0.14504 | −0.05250 | −0.04657 |
PRCC(S, 1 h) | −0.40421 | −0.20533 | −0.50535 | −0.30275 | 0.12012 | 0.16696 |
PRCC(F, 1 h) | 0.12788 | 0.52405 | 0.85069 | 0.68899 | −0.35946 | −0.55150 |
PRCC(A, 1 h) | −0.02169 | −0.16545 | −0.45533 | −0.24162 | 0.08285 | 0.14085 |
PRCC(R, 1 h) | 0.06123 | 0.32027 | 0.72956 | 0.46364 | −0.19797 | −0.28509 |
PRCC(S, 10 h) | −0.36729 | −0.27416 | −0.40630 | −0.34399 | 0.15168 | 0.53178 |
PRCC(F, 10 h) | 0.18956 | 0.49155 | 0.67193 | 0.55311 | −0.26960 | −0.81199 |
PRCC(A, 10 h) | −0.06678 | −0.25292 | −0.36120 | −0.29522 | 0.11705 | 0.42062 |
PRCC(R, 10 h) | 0.13050 | 0.35427 | 0.54956 | 0.43078 | −0.19729 | −0.69605 |
PRCC(S, 100 h) | −0.33893 | −0.24777 | −0.38422 | −0.30025 | 0.12914 | 0.56675 |
PRCC(F, 100 h) | 0.17244 | 0.45223 | 0.63376 | 0.50030 | −0.23642 | −0.82047 |
PRCC(A, 100 h) | −0.06158 | −0.17745 | −0.25095 | −0.20439 | 0.06632 | 0.38276 |
PRCC(R, 100 h) | 0.11273 | 0.30119 | 0.48277 | 0.35914 | −0.15850 | −0.70620 |
Minimum | 0.22 | 0.007 | 0.133 | 0.1 | 0.1 | 0.03 |
Baseline | 2.2 | 0.07 | 1.33 | 1 | 1 | 0.3 |
Maximum | 6.6 | 1.4 | 6.65 | 5 | 5 | 1.5 |
Parameter | σ8 | σ3 | σ11 | σ6 | ω2 | σ12 |
PRCC(S, 0.1 h) | 0.00418 | 0.00133 | 0.00721 | −0.00730 | 0.00880 | −0.00095 |
PRCC(F, 0.1 h) | 0.00491 | −0.02376 | −0.00479 | −0.01447 | 0.00070 | 0.00029 |
PRCC(A, 0.1 h) | 0.94584 | 0.94967 | 0.43179 | −0.14146 | −0.02909 | 0.01632 |
PRCC(R, 0.1 h) | −0.00100 | −0.00278 | 0.00123 | 0.00560 | 0.00341 | 0.99028 |
PRCC(S, 1 h) | −0.00087 | 0.00042 | −0.00427 | 0.00687 | 0.00177 | 0.00779 |
PRCC(F, 1 h) | 0.00618 | −0.00739 | 0.00563 | −0.01924 | 0.00263 | −0.00287 |
PRCC(A, 1 h) | 0.91506 | 0.83297 | 0.64729 | −0.35019 | −0.17798 | 0.00315 |
PRCC(R, 1 h) | 0.00334 | −0.01925 | 0.00145 | 0.00094 | 0.00290 | 0.71206 |
PRCC(S, 10 h) | −0.00308 | −0.01061 | −0.00714 | 0.00995 | −0.01861 | 0.01484 |
PRCC(F, 10 h) | 0.01215 | −0.00269 | 0.00844 | −0.01508 | 0.00412 | −0.01259 |
PRCC(A, 10 h) | 0.88966 | 0.56291 | 0.46346 | −0.27714 | −0.70996 | 0.00142 |
PRCC(R, 10 h) | 0.01655 | −0.01123 | 0.00754 | −0.01035 | −0.00245 | 0.30818 |
PRCC(S, 100 h) | −0.00690 | −0.01292 | −0.00574 | 0.00764 | −0.01664 | 0.01243 |
PRCC(F, 100 h) | 0.01736 | 0.00037 | 0.00739 | −0.01191 | 0.00101 | −0.01150 |
PRCC(A, 100 h) | 0.81841 | 0.37472 | 0.30075 | −0.17214 | −0.87775 | −0.00371 |
PRCC(R, 100 h) | 0.02093 | −0.00869 | 0.00635 | −0.01199 | −0.00584 | 0.25393 |
Minimum | 0.00033 | 0.0111 | 0.1 | 0.1 | 0.002 | 0.01 |
Baseline | 0.0033 | 0.111 | 1 | 1 | 0.02 | 0.1 |
Maximum | 0.99 | 1.11 | 5 | 5 | 0.2 | 1 |
Parameter | σ13 | ω3 | ||||
PRCC(S, 0.1 h) | 0.01202 | −0.00260 | ||||
PRCC(F, 0.1 h) | 0.01100 | −0.00583 | ||||
PRCC(A, 0.1 h) | 0.00190 | 0.00014 | ||||
PRCC(R, 0.1 h) | 0.84920 | −0.25007 | ||||
PRCC(S, 1 h) | 0.00730 | 0.00780 | ||||
PRCC(F, 1 h) | 0.01072 | −0.01173 | ||||
PRCC(A, 1 h) | 0.01013 | 0.00761 | ||||
PRCC(R, 1 h) | 0.82148 | −0.49732 | ||||
PRCC(S, 10 h) | 0.00154 | 0.01567 | ||||
PRCC(F, 10 h) | 0.00974 | −0.01014 | ||||
PRCC(A, 10 h) | 0.00512 | 0.01810 | ||||
PRCC(R, 10 h) | 0.68681 | −0.75844 | ||||
PRCC(S, 100 h) | −0.00381 | 0.01276 | ||||
PRCC(F, 100 h) | 0.01531 | −0.00586 | ||||
PRCC(A, 100 h) | 0.00191 | −0.00058 | ||||
PRCC(R, 100 h) | 0.61909 | −0.74965 | ||||
Minimum | 0.013 | 0.0139 | ||||
Baseline | 0.13 | 0.139 | ||||
Maximum | 1.3 | 1.39 |
Par | Description | Values | Ref |
Intracellular dynamics | |||
λB | Bortezomib signaling scaling factor | 1.9 | [46] |
α | Inhibition strength of bortezomib by oHSV | 30 | [46] |
σ1 | Autocatalytic enhancement rate of IκB | 4 | [46] |
σ9 | Hill-type parameter | 1.0 | [46] |
σ4 | Inhibition strength of IκB by the NFκB-Bcl-2 complex | 2.2 | [46] |
σ7 | Signaling strength of the NFκB-Bcl-2 complex | 0.07 | [46] |
σ2 | Autocatalytic enhancement rate of the NFκB-Bcl-2 complex | 1.33 | [46] |
σ10 | Hill-type parameter | 1.0 | [46] |
σ5 | Inhibition strength of the NFκB-Bcl-2 complex by IκB | 1 | [46] |
ω1 | Decay rate of NFκB-Bcl-2 complex | 0.3 | [107,108] |
σ8 | Signaling strength of BAX | 0.0033 | [46] |
σ3 | Autocatalytic enhancement rate of BAX | 0.111 | [46] |
σ11 | Hill-type parameter | 1.0 | [46] |
σ6 | Inhibition strength of BAX by the NFκB-Bcl-2 complex | 1 | [46] |
ω2 | Decay rate of BAX | 0.02 | [107,108,109] |
σ12 | Signaling strength of RIP1 | 0.1 | [46] |
σ13 | Activation rate of RIP1 in the presence of OVs | 0.13 | [46] |
ω3 | Decay rate of RIP1 | 0.139 | [107,108,110] |
k | Hill type parameter of oHSV switching | 0.01 v∗ | [46] |
Cancer cells | |||
λ | proliferation rate of tumor cells | 4×10−3 | [46,57] |
x0 | Carrying capacity of uninfected tumor cells | 1 | [38,45,46,57] |
β1 | Bortezomib-induced apoptosis rate of tumor cells | 2×10−4 | [46] |
β2 | Oncolysis rate of tumor cells | 3×10−4 | [45,46,57] |
β3 | Necroptosis rate of tumor cells | 5×10−4 | [46] |
δ | infected cell lysis rate | 4.4×10−3 | [45,57] |
μ | Removal rate of dead cells | 2.3×10−3 | [45,46,57] |
Anticancer drugs | |||
b | Burst size of infected cells | 50 | [46,57] |
α1 | bortezomib-induced viral replication rate | 1 | [45,46] |
γ | clearance rate of viruses | 1.8×10−3 | [45,57] |
μ1 | consumption rate of bortezomib by uninfected tumor cells | 0.2075 | [45,46] |
μ2 | consumption rate of bortezomib by infected tumor cells | 0.2075 | [45,46] |
kB | Hill-type parameter | 1 | [45,46] |
μB | decay rate of bortezomib | 0.03 | [46,111,112] |
Reference values of main variables | |||
S∗ | Concentration of IκB | 0.05 μM | [113,114] |
F∗ | Concentration of the NFκB-Bcl2 complex | 0.5 μM | [113,114,115,116] |
A∗ | Concentration of BAX | 0.1 μM | [117] |
R∗ | Concentration of RIP1 | 5.0 μM | [118] |
x∗ | Uninfected cell density | 106cells/mm3 | [45,57,119] |
y∗ | Infected cell density | =x∗ | [45,57,119] |
n∗ | Dead cell density | =x∗ | [45,57,119] |
v∗ | Virus concentration | 2.2×108virus/mm3 | [45,57,119] |
B∗ | Bortezomib concentration | 1.0×10−11g/mm3 | [6,43,45] |
Parameter | B | v | λB | α | σ1 | σ9 |
PRCC(S, 0.1 h) | 0.28187 | −0.03828 | 0.26953 | −0.33367 | 0.94918 | 0.50929 |
PRCC(F, 0.1 h) | −0.02305 | 0.01961 | −0.02248 | 0.04351 | −0.27969 | −0.04106 |
PRCC(A, 0.1 h) | 0.00822 | 0.00416 | −0.01449 | −0.01347 | −0.01005 | −0.00355 |
PRCC(R, 0.1 h) | −0.00527 | 0.13183 | −0.00513 | 0.02775 | −0.06686 | −0.01338 |
PRCC(S, 1 h) | 0.26056 | −0.02550 | 0.25868 | −0.29694 | 0.82422 | 0.73676 |
PRCC(F, 1 h) | −0.07284 | 0.02242 | −0.08059 | 0.09171 | −0.48167 | −0.32707 |
PRCC(A, 1 h) | 0.02008 | 0.00165 | 0.01746 | −0.02042 | 0.11314 | 0.05378 |
PRCC(R, 1 h) | −0.03627 | 0.11745 | −0.04266 | 0.06355 | −0.27849 | −0.14751 |
PRCC(S, 10 h) | 0.37318 | −0.03499 | 0.35211 | −0.35990 | 0.55805 | 0.62897 |
PRCC(F, 10 h) | −0.09981 | 0.02151 | −0.10770 | 0.12735 | −0.32377 | −0.35500 |
PRCC(A, 10 h) | 0.04337 | 0.00249 | 0.04355 | −0.04101 | 0.16069 | 0.14905 |
PRCC(R, 10 h) | −0.06094 | 0.07032 | −0.07396 | 0.10494 | −0.23546 | −0.24755 |
PRCC(S, 100 h) | 0.38143 | −0.03454 | 0.36085 | −0.36390 | 0.51007 | 0.58888 |
PRCC(F, 100 h) | −0.09180 | 0.01882 | −0.10493 | 0.12160 | −0.27286 | −0.30714 |
PRCC(A, 100 h) | 0.04653 | −0.00022 | 0.02574 | −0.01787 | 0.11811 | 0.10076 |
PRCC(R, 100 h) | −0.04955 | 0.05732 | −0.06608 | 0.09572 | −0.17990 | −0.19896 |
Minimum | 0 | 0 | 0.19 | 3 | 0.4 | 0.1 |
Baseline | 0.5 | 0.01 | 1.9 | 30 | 4 | 1 |
Maximum | 5 | 0.1 | 5.7 | 60 | 8 | 5 |
Parameter | σ4 | σ7 | σ2 | σ10 | σ5 | ω1 |
PRCC(S, 0.1 h) | −0.17767 | −0.06597 | −0.24638 | −0.03404 | −0.00584 | 0.02214 |
PRCC(F, 0.1 h) | 0.00846 | 0.63726 | 0.96485 | 0.53649 | −0.19325 | −0.15541 |
PRCC(A, 0.1 h) | −0.01288 | −0.03460 | −0.19312 | −0.03172 | −0.00515 | 0.00624 |
PRCC(R, 0.1 h) | 0.02074 | 0.26024 | 0.79329 | 0.14504 | −0.05250 | −0.04657 |
PRCC(S, 1 h) | −0.40421 | −0.20533 | −0.50535 | −0.30275 | 0.12012 | 0.16696 |
PRCC(F, 1 h) | 0.12788 | 0.52405 | 0.85069 | 0.68899 | −0.35946 | −0.55150 |
PRCC(A, 1 h) | −0.02169 | −0.16545 | −0.45533 | −0.24162 | 0.08285 | 0.14085 |
PRCC(R, 1 h) | 0.06123 | 0.32027 | 0.72956 | 0.46364 | −0.19797 | −0.28509 |
PRCC(S, 10 h) | −0.36729 | −0.27416 | −0.40630 | −0.34399 | 0.15168 | 0.53178 |
PRCC(F, 10 h) | 0.18956 | 0.49155 | 0.67193 | 0.55311 | −0.26960 | −0.81199 |
PRCC(A, 10 h) | −0.06678 | −0.25292 | −0.36120 | −0.29522 | 0.11705 | 0.42062 |
PRCC(R, 10 h) | 0.13050 | 0.35427 | 0.54956 | 0.43078 | −0.19729 | −0.69605 |
PRCC(S, 100 h) | −0.33893 | −0.24777 | −0.38422 | −0.30025 | 0.12914 | 0.56675 |
PRCC(F, 100 h) | 0.17244 | 0.45223 | 0.63376 | 0.50030 | −0.23642 | −0.82047 |
PRCC(A, 100 h) | −0.06158 | −0.17745 | −0.25095 | −0.20439 | 0.06632 | 0.38276 |
PRCC(R, 100 h) | 0.11273 | 0.30119 | 0.48277 | 0.35914 | −0.15850 | −0.70620 |
Minimum | 0.22 | 0.007 | 0.133 | 0.1 | 0.1 | 0.03 |
Baseline | 2.2 | 0.07 | 1.33 | 1 | 1 | 0.3 |
Maximum | 6.6 | 1.4 | 6.65 | 5 | 5 | 1.5 |
Parameter | σ8 | σ3 | σ11 | σ6 | ω2 | σ12 |
PRCC(S, 0.1 h) | 0.00418 | 0.00133 | 0.00721 | −0.00730 | 0.00880 | −0.00095 |
PRCC(F, 0.1 h) | 0.00491 | −0.02376 | −0.00479 | −0.01447 | 0.00070 | 0.00029 |
PRCC(A, 0.1 h) | 0.94584 | 0.94967 | 0.43179 | −0.14146 | −0.02909 | 0.01632 |
PRCC(R, 0.1 h) | −0.00100 | −0.00278 | 0.00123 | 0.00560 | 0.00341 | 0.99028 |
PRCC(S, 1 h) | −0.00087 | 0.00042 | −0.00427 | 0.00687 | 0.00177 | 0.00779 |
PRCC(F, 1 h) | 0.00618 | −0.00739 | 0.00563 | −0.01924 | 0.00263 | −0.00287 |
PRCC(A, 1 h) | 0.91506 | 0.83297 | 0.64729 | −0.35019 | −0.17798 | 0.00315 |
PRCC(R, 1 h) | 0.00334 | −0.01925 | 0.00145 | 0.00094 | 0.00290 | 0.71206 |
PRCC(S, 10 h) | −0.00308 | −0.01061 | −0.00714 | 0.00995 | −0.01861 | 0.01484 |
PRCC(F, 10 h) | 0.01215 | −0.00269 | 0.00844 | −0.01508 | 0.00412 | −0.01259 |
PRCC(A, 10 h) | 0.88966 | 0.56291 | 0.46346 | −0.27714 | −0.70996 | 0.00142 |
PRCC(R, 10 h) | 0.01655 | −0.01123 | 0.00754 | −0.01035 | −0.00245 | 0.30818 |
PRCC(S, 100 h) | −0.00690 | −0.01292 | −0.00574 | 0.00764 | −0.01664 | 0.01243 |
PRCC(F, 100 h) | 0.01736 | 0.00037 | 0.00739 | −0.01191 | 0.00101 | −0.01150 |
PRCC(A, 100 h) | 0.81841 | 0.37472 | 0.30075 | −0.17214 | −0.87775 | −0.00371 |
PRCC(R, 100 h) | 0.02093 | −0.00869 | 0.00635 | −0.01199 | −0.00584 | 0.25393 |
Minimum | 0.00033 | 0.0111 | 0.1 | 0.1 | 0.002 | 0.01 |
Baseline | 0.0033 | 0.111 | 1 | 1 | 0.02 | 0.1 |
Maximum | 0.99 | 1.11 | 5 | 5 | 0.2 | 1 |
Parameter | σ13 | ω3 | ||||
PRCC(S, 0.1 h) | 0.01202 | −0.00260 | ||||
PRCC(F, 0.1 h) | 0.01100 | −0.00583 | ||||
PRCC(A, 0.1 h) | 0.00190 | 0.00014 | ||||
PRCC(R, 0.1 h) | 0.84920 | −0.25007 | ||||
PRCC(S, 1 h) | 0.00730 | 0.00780 | ||||
PRCC(F, 1 h) | 0.01072 | −0.01173 | ||||
PRCC(A, 1 h) | 0.01013 | 0.00761 | ||||
PRCC(R, 1 h) | 0.82148 | −0.49732 | ||||
PRCC(S, 10 h) | 0.00154 | 0.01567 | ||||
PRCC(F, 10 h) | 0.00974 | −0.01014 | ||||
PRCC(A, 10 h) | 0.00512 | 0.01810 | ||||
PRCC(R, 10 h) | 0.68681 | −0.75844 | ||||
PRCC(S, 100 h) | −0.00381 | 0.01276 | ||||
PRCC(F, 100 h) | 0.01531 | −0.00586 | ||||
PRCC(A, 100 h) | 0.00191 | −0.00058 | ||||
PRCC(R, 100 h) | 0.61909 | −0.74965 | ||||
Minimum | 0.013 | 0.0139 | ||||
Baseline | 0.13 | 0.139 | ||||
Maximum | 1.3 | 1.39 |