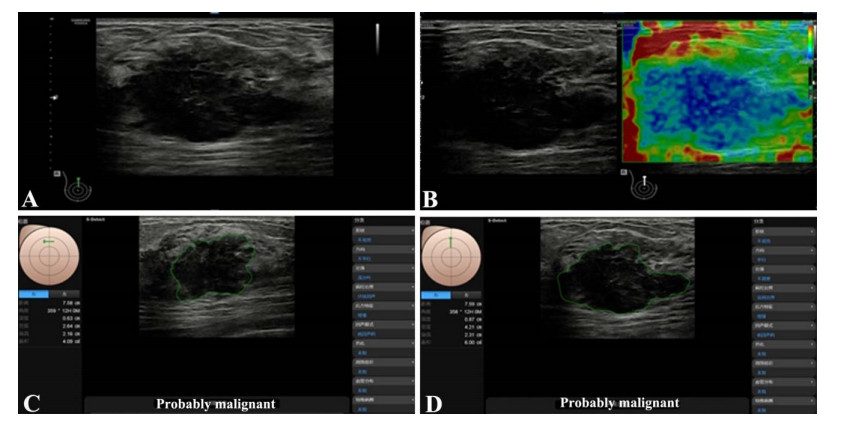
Listeria monocytogenes poses a significant threat to food safety worldwide, including in African countries. This bacterium is capable of causing severe infections, particularly in vulnerable populations. In this review, we provide an overview of the prevalence, transmission, and control measures of L. monocytogenes in the food chain across North Africa. Various factors contribute to the persistence and spread of this pathogen in food production and distribution systems, including environmental contamination, improper handling, and inadequate hygiene practices. Understanding the dynamics of L. monocytogenes in the North African food chain is crucial for implementing effective control strategies to mitigate the risk of contamination and protect public health. This review highlights the rise of virulence genes in L. monocytogenes from food production, especially milk production, over the past five years and their subsequent identification in human L. monocytogenes isolates from listeriosis cases. This underscores the persistent challenges that L. monocytogenes may pose to food safety and public health in North African countries.
Citation: Mohamed-Yousif Ibrahim Mohamed, Ihab Habib. Listeria monocytogenes in food products, and its virulence in North Africa[J]. AIMS Agriculture and Food, 2025, 10(1): 97-128. doi: 10.3934/agrfood.2025006
[1] | Bo An . Construction and application of Chinese breast cancer knowledge graph based on multi-source heterogeneous data. Mathematical Biosciences and Engineering, 2023, 20(4): 6776-6799. doi: 10.3934/mbe.2023292 |
[2] | Jiajia Jiao, Xiao Xiao, Zhiyu Li . dm-GAN: Distributed multi-latent code inversion enhanced GAN for fast and accurate breast X-ray image automatic generation. Mathematical Biosciences and Engineering, 2023, 20(11): 19485-19503. doi: 10.3934/mbe.2023863 |
[3] | Lingli Gan, Xiaoling Yin, Jiating Huang, Bin Jia . Transcranial Doppler analysis based on computer and artificial intelligence for acute cerebrovascular disease. Mathematical Biosciences and Engineering, 2023, 20(2): 1695-1715. doi: 10.3934/mbe.2023077 |
[4] | Yali Ouyang, Zhuhuang Zhou, Weiwei Wu, Jin Tian, Feng Xu, Shuicai Wu, Po-Hsiang Tsui . A review of ultrasound detection methods for breast microcalcification. Mathematical Biosciences and Engineering, 2019, 16(4): 1761-1785. doi: 10.3934/mbe.2019085 |
[5] | Jun Gao, Qian Jiang, Bo Zhou, Daozheng Chen . Convolutional neural networks for computer-aided detection or diagnosis in medical image analysis: An overview. Mathematical Biosciences and Engineering, 2019, 16(6): 6536-6561. doi: 10.3934/mbe.2019326 |
[6] | Muhammad Bilal Shoaib Khan, Atta-ur-Rahman, Muhammad Saqib Nawaz, Rashad Ahmed, Muhammad Adnan Khan, Amir Mosavi . Intelligent breast cancer diagnostic system empowered by deep extreme gradient descent optimization. Mathematical Biosciences and Engineering, 2022, 19(8): 7978-8002. doi: 10.3934/mbe.2022373 |
[7] | Jian-xue Tian, Jue Zhang . Breast cancer diagnosis using feature extraction and boosted C5.0 decision tree algorithm with penalty factor. Mathematical Biosciences and Engineering, 2022, 19(3): 2193-2205. doi: 10.3934/mbe.2022102 |
[8] | Xiao Zou, Jintao Zhai, Shengyou Qian, Ang Li, Feng Tian, Xiaofei Cao, Runmin Wang . Improved breast ultrasound tumor classification using dual-input CNN with GAP-guided attention loss. Mathematical Biosciences and Engineering, 2023, 20(8): 15244-15264. doi: 10.3934/mbe.2023682 |
[9] | Xiaoli Zhang, Ziying Yu . Pathological analysis of hesperetin-derived small cell lung cancer by artificial intelligence technology under fiberoptic bronchoscopy. Mathematical Biosciences and Engineering, 2021, 18(6): 8538-8558. doi: 10.3934/mbe.2021423 |
[10] | Xi Lu, Xuedong Zhu . Automatic segmentation of breast cancer histological images based on dual-path feature extraction network. Mathematical Biosciences and Engineering, 2022, 19(11): 11137-11153. doi: 10.3934/mbe.2022519 |
Listeria monocytogenes poses a significant threat to food safety worldwide, including in African countries. This bacterium is capable of causing severe infections, particularly in vulnerable populations. In this review, we provide an overview of the prevalence, transmission, and control measures of L. monocytogenes in the food chain across North Africa. Various factors contribute to the persistence and spread of this pathogen in food production and distribution systems, including environmental contamination, improper handling, and inadequate hygiene practices. Understanding the dynamics of L. monocytogenes in the North African food chain is crucial for implementing effective control strategies to mitigate the risk of contamination and protect public health. This review highlights the rise of virulence genes in L. monocytogenes from food production, especially milk production, over the past five years and their subsequent identification in human L. monocytogenes isolates from listeriosis cases. This underscores the persistent challenges that L. monocytogenes may pose to food safety and public health in North African countries.
The breast tumor is a common clinical disease in women. Ultrasound has always been considered the most appropriate method for breast examination and tumor screening [1]. However, ultrasound has a strong technical dependence on the examiners, and the results of different examiners are different, which has been considered the shortcomings of ultrasound [2]. With the imaging technology development, more and more methods have been used to objectively analyze ultrasound images to help diagnose breast tumors [3], such as elastic ultrasound, computer-aided diagnosis technology, etc.
The S-Detect artificial intelligence system is a set of auxiliary ultrasound imaging in diagnosing a deep learning system [4,5,6]. This system extracts the morphological features from the breast imaging reporting data system recommended by the American Society of Radiology (BI-RADS). Combined with pathological results of mass, the information can be used to diagnose breast lumps automatically. Two-dimensional breast ultrasound is widely used in diagnosing breast lesions. Still, due to its strong operator dependence, the accuracy and repeatability of BI-RADS classification results between different operators need to be improved [7,8,9]. Artificial intelligence-assisted diagnosis system eliminates the influence of many human factors. It can carry out image analysis objectively and efficiently to improve doctors' working efficiency and diagnostic efficiency [10,11,12].
One of the main problems encountered in ultrasound diagnosis is that senior doctors' diagnostic level is significantly higher than that of junior doctors. The training of a junior doctor often takes four years or more. The use of AI will significantly reduce the difficulty of training doctors. The purpose of this study was to evaluate the value of the S-Detect artificial intelligence system in the auxiliary diagnosis of benign and malignant breast masses.
From November 2019 to June 2020, the patients with breast masses undergoing ultrasound examination in the ultrasound imaging department of our hospital were randomly collected.
Inclusion criteria: The diagnosis had definite pathological support; adult patients; hospitalized patients.
Exclusion criteria: The mass boundary was not clear; there were blood flow, arrow, and text on the image; patients who can't cooperate with the exam.
Finally, 40 patients were enrolled in the study cohort. All the patients were female, with an age of (50.9 ± 13.9) years old. These patients were diagnosed with single or multiple breast lesions. However, only one typical image was selected from a patient. This study was approved by the ethics committee of the First People's Hospital of Anqing City. The written informed consent was obtained from patients.
The Samsung RS80A ultrasonic diagnostic instrument was used to detect the breast, with an L3-12A probe, frequency 3~12MHz.The patient was placed in the supine position, with his hands over his head, to expose the mammary glands and axilla's scanning area as large as possible. The scan was executed by two ultrasound doctors (engaged in breast ultrasound > 3 years) for regular breast two-dimensional (2D) gray-scale and color doppler ultrasound. The 2D images were collected, and the location, size, shape, boundary, internal echo, rear and side, blood flow, and spectrum of the echo were observed. The information was evaluated by the BI-RADS classification.
Then, the S-Detect mammary gland mode was selected to display the 2D horizontal and vertical gray-scale section of the mass. After clicking the center of lesion, the system automatically outlined the lesion boundary as the region of interest. If the border drawn automatically did not match the mass's solid edge, the operator could readjust and sketch the frame. After selecting the most appropriate boundary, the system automatically listed the various characteristics of the mass (size, depth, shape, boundary, internal echo, and so on) and the diagnostic results, which probably benign or malignant (Figures 1–3).
Finally, the model was converted to breast elasticity, and the patient was asked to hold their breath without pressure. Satisfactory elastic images were collected. The color of elastography was divided into green and blue, which reflects the hardness of the tissue. If the lesion is very hard, it is dark blue and tends to be malignant. The lesions were soft, light green, and managed to be benign.
The BI-RADS classification results for each breast mass were divided into the senior physician (engaged in breast ultrasound > 15 years) and the junior group. Both of them were classified into two groups based on whether the auxiliary S-Detect AI were used. One group was the BI-RADS initial classification of each mass by sonographers based on conventional ultrasound images; another group was the BI-RADS classification for each mass again performed for the physician combined with S-Detect assisted diagnosis.
Since each mass was randomly examined more than twice before the final surgical biopsy, the multiple BI-RADS classifications for all lesions obtained by multiple sonographers were defined in this study as a junior set of results. The S-Detect system diagnosis results were also combined with the junior set of products. The BI-RADS classification diagnosis results Category ≤ 4a was defined as benign lesions, while Category ≥ 4b was defined as malignant lesions.
The S-Detect diagnosis of each breast mass in both transverse and longitudinal sections was recorded as B/B, and both areas were likely benign. The diagnosis results of the two regions are different, which was denoted as M/B. Both sections are likely malignant, denoted as M/M. The S-Detect diagnostic result B/B was defined as benign lesions, while the M/B or M/M were defined as malignant lesions.
According to the standards of elastic imaging Guidelines, the Tsukuba score (Elasticity Score) was performed for each breast mass, with the ES value as the ES group data. The ES ≤ 3 points of Tsukuba were defined as benign lesions, while ES ≥ 4 points were defined as malignant lesions.
The SPSS 21.0 software was used for statistical analysis of the data. The qualitative data were presented in terms of rate and the results of different diagnostic methods for the same lesion were compared by paired chi-square test.
Based on the pathological results, the receiver operator characteristics (ROC) curves were drawn for multiple groups of diagnostic products to obtain the area under curve area (AUC), sensitivity, specificity, and diagnostic accuracy. Kappa test was used to analyze the consistency of diagnostic results of different groups of data. The accuracy was calculated by the following formula:
accuracy=a+dn×100%(1) |
In the formula, the "a" represents the true positive number, "d" represents the true negative number, and "n" represents the total number.
Among the 40 patients, benign lesions accounted for 40.0% (16/40), and malignant lesions accounted for 60.0% (24/40). In the benign lesions, there were 4 cases of mammary gland disease, 8 cases of fibroadenoma, 2 cases of papilloma, and 2 cases of other benign tumors. In the malignant lesions, there were 17 cases of invasive ductal carcinoma, 1 case of mucinous carcinoma, 1 case of papillary carcinoma, 3 case of intraductal carcinoma, 1 cases of invasive lobular carcinoma, and 1 case of intraductal carcinoma in situ..
When the results of pathological examination were used as the gold standard, the S-Detect AI system itself had high sensitivity, specificity, and accuracy (95.8%, 93.8%, 89.6%) for the identification of benign and malignant breast masses. The diagnostic efficiency of two-dimensional gray-scale ultrasound was the lowest, with the sensitivity and specificity was lower than 80%. The elastic image had a relatively high specificity (93.8%) and a relatively lower sensitivity (79.2%). The detail was shown in Table 1 and Figure 4.
Group | AUC | Sensitivity (%) | Specificity (%) | Accuracy (%) |
AI | 0.948 | 95.8 | 93.8 | 89.6 |
Elastic image | 0.865 | 79.2 | 93.8 | 73.0 |
Gray-scale | 0.719 | 75.0 | 68.8 | 43.8 |
Before the S-Detect AI system was used, the BI-RADS classification of 40 patients was category 3 in 8 cases, Category 4a in 13 cases, category 4b in 11 cases, and category 4c in 6 cases, and category 5 in 2 cases. From Table 2, doctors primarily use the S-Detect auxiliary system for escalation and degradation of category 4a and class 4b. For BI-RADS3, 4C, and 5 breast lesions, the S-Detect system diagnostic difference did not substantially affect the judgment of doctor.
Before assistance | S-Detect | After assistance |
Category 8 | B/B 7 | Category 3 8 |
M/B 1 | ||
Category4a 13 | B/B 6 | Category 3 3 |
M/B 4 | Category 4a 4 | |
M/M 3 | Category 4b 4 | |
Category 4c 2 | ||
Category 4b 11 | B/B 2 | Category 4a 2 |
M/B 4 | Category 4b 6 | |
M/M 5 | Category 4c 3 | |
Category 4c 6 | M/B 2 | Category 4c 6 |
M/M 4 | ||
Category 5 2 | M/M 2 | Category 5 2 |
With the assistance of S-Detect AI system, the accuracy of BI-RADS classification was improved significantly. The detail was showed in Table 3 and Figure 5.
Group | Area under ROC curve | Sensitivity (%) | Specificity (%) | Accuracy (%) |
Senior | 0.802 | 79.2 | 81.3 | 60.5 |
Junior | 0.719 | 75.0 | 68.8 | 43.8 |
Senior+AI | 0.969 | 100.0 | 93.8 | 93.8 |
Junior+AI | 0.948 | 95.8 | 93.8 | 89.6 |
The S-Detect artificial intelligence system can effectively differentiate benign from malignant breast lesions. With the assistance of this technology, the BI-RADS classification diagnostic performance of senior sonographers has been on the rise [13]. The S-Detect auxiliary diagnosis results combined with the results of random ultrasound examination can significantly improve diagnostic accuracy. Therefore, this clinical diagnosis technique can enhance the quality of random breast ultrasound examinations obtained by patients and reduce missed diagnoses and misdiagnosis. The S-Detect technique and the elasticity scoring technique can improve the sonographer's diagnostic efficiency in breast cancer diagnosis. Still, there are some differences between the diagnostic ability and the auxiliary diagnostic ability [14].
Artificial intelligence technology based on big data and deep learning algorithm is widely integrated into the medical field [15,16,17]. Combining medical imaging with it can reduce the work burden of imaging physicians and improve the overall work efficiency. Computer aided diagnosis system has the advantages of objectivity, stability and high repeatability, and has been used in the early research and application of lung mass, breast mass and cardiovascular disease. The S-Detect auxiliary diagnostic system is intelligent and efficient, using the physico-acoustic characteristics of 2D gray-scale images of breast lesions for rapid differential diagnosis. In this study, the S-Detect technique has a high diagnostic efficiency, with differential diagnosis sensitivity, specificity and accuracy exceeding 90%. With the aid of this technology, the diagnostic efficiency of senior doctors has an upward trend. As a result, the S-Detect technology can provide effective advice and increase diagnostic confidence for junior sonographer in the diagnosis of 2D gray-scale images.
According to the BI-RADS classification standards recommended by the American Society of Radiology, Category3mass for the possibility of malignant 2% or less, Category 4a mass is the possibility of malignant is 2~10%. In this study, of the 16 benign groups, 93.8% (15/16) were classified as Category 3 or 4a, and the pathological findings were mostly adenopathy or fibroadenoma of the breast. The clinician will perform a pathological biopsy or surgical excision of masses classified as Category 4a or above, most of which pathologically turn out benign. The S-Detect technology has higher diagnostic performance for BI-RADS4a and 4B mass upgrades and downgrades, improving the accuracy of the random ultrasound surgeon's diagnosis and reducing unnecessary biopsies and surgeries to BI-RADS4a diagnosis. The operators in 2D ultrasound examination of the breast are highly dependent. The operation technique and image discrimination ability of ultrasound doctors with different years of experience lead to poor repeatability among the operators [18,19,20]. The S-Detect system automatically analyzes a 2D gray-scale image of a breast mass, allowing for more objective identification of a breast mass, regardless of the operator's years of service, experience, resolution, etc. In this study, to our hospital patients randomized to breast ultrasound, diagnostic accuracy was less than 80%. With the help of artificial intelligence, the joint diagnosis accuracy up to 90%, both in the senior and junior doctor. The results reduced the low qualification doctor with high qualification doctor diagnosis efficiency of the differences, thus improving the hospital's overall quality [10].
Elasticity score technology reflects the hardness characteristics of breast masses [21,22]. It uses the elasticity information of breast masses to improve the diagnostic efficiency of ultrasound doctors. The S-Detect technique uses the same physical-acoustic information and diagnostic classification as conventional breast ultrasound complements and optimizes the two-dimensional information of conventional ultrasound. In this study, the elasticity scoring technique was less sensitive than the S-Detect technique, and the accuracy was similar to the S-Detect technique. Still, the combination of the elasticity scoring with routine breast ultrasound was slightly better than the S-Detect technique, possibly due to the complementarity of physical information from different directions.
In summary, the S-Detect artificial intelligence system can improve breast cancer diagnosis accuracy by ultrasound physicians and improve the quality of routine breast ultrasound diagnosis. In the future, multi-center and more extensive sample size studies are needed to verify the artificial intelligence system's clinical application prospect. The S-Detect technology has its limitations. Its characteristic analysis does not include important information such as blood flow signals, calcifications, and mass hardness, and the system has errors in the identification of masses < 1 cm in diameter.
All the doctors in the ultrasound department of the first people's Hospital of Anqing city were acknowledged for their data collection contribution.
All authors declare no conflicts of interest in this paper.
[1] |
EFSA (2022) The European Union One Health 2021 Zoonoses Report. EFSA J 20: e07666. https://doi.org/10.2903/j.efsa.2022.7666 doi: 10.2903/j.efsa.2022.7666
![]() |
[2] |
Townsend A, Strawn LK, Chapman BJ, et al. (2021) A systematic review of Listeria species and Listeria monocytogenes prevalence, persistence, and diversity throughout the fresh produce supply chain. Foods 10: 1427. https://doi.org/10.3390/foods10061427 doi: 10.3390/foods10061427
![]() |
[3] |
Tack DM, Ray L, Griffin PM, et al. (2020) Preliminary incidence and trends of infections with pathogens transmitted commonly through food—Foodborne diseases active surveillance network, 10 U.S. Sites, 2016–2019. MMWR Morb Mortal Wkly Rep 69: 509–514. https://doi.org/10.15585/mmwr.mm6917a1 doi: 10.15585/mmwr.mm6917a1
![]() |
[4] |
Santos dos. JS, Biduski B, Santos dos. LR (2021) Listeria monocytogenes: health risk and a challenge for food processing establishments. Arch Microbiol 203: 5907–5919. https://doi.org/10.1007/s00203-021-02590-2 doi: 10.1007/s00203-021-02590-2
![]() |
[5] | Habib I, Mohamed MYI (2022) Chapter 3—Foodborne infections in the Middle East. In: Savvaidis IN, Osaili TM (Eds.), Food Safety in the Middle East, Elsevier, 71–107. https://doi.org/10.1016/B978-0-12-822417-5.00005-2 |
[6] |
Habib I, Mohamed MYI, Khan M (2021) Current state of Salmonella, Campylobacter and Listeria in the food chain across the arab countries: A descriptive review. Foods 10: 2369. https://doi.org/10.3390/foods10102369 doi: 10.3390/foods10102369
![]() |
[7] |
Elafify M, Darwish WS, El-Toukhy M, et al. (2022) Prevalence of multidrug resistant Salmonella spp. in dairy products with the evaluation of the inhibitory effects of ascorbic acid, pomegranate peel extract, and D-tryptophan against Salmonella growth in cheese. Int J Food Microbiol 364: 109534. https://doi.org/10.1016/j.ijfoodmicro.2022.109534 doi: 10.1016/j.ijfoodmicro.2022.109534
![]() |
[8] |
Zahli R, Scheu AK, Abrini J, et al. (2022) Salmonella spp: Prevalence, antimicrobial resistance and molecular typing of strains isolated from poultry in Tetouan-Morocco. LWT 153: 112359. https://doi.org/10.1016/j.lwt.2021.112359 doi: 10.1016/j.lwt.2021.112359
![]() |
[9] |
El Kenawy A, McCabe M, Vicente-Serrano S, et al. (2016) Recent changes in continentality and aridity conditions over the Middle East and North Africa region, and their association with circulation patterns. Clim Res 69: 25–43. https://doi.org/10.3354/cr01389 doi: 10.3354/cr01389
![]() |
[10] |
Mohamed M-YI (2024) Campylobacteriosis in North Africa. AIMS Agric Food 9: 801–821. https://doi.org/10.3934/agrfood.2024043 doi: 10.3934/agrfood.2024043
![]() |
[11] |
Mohamed M-YI, Khalifa HO, Habib I (2025) Food pathways of Salmonella and its ability to cause gastroenteritis in North Africa. Foods 14: 253. https://doi.org/10.3390/foods14020253 doi: 10.3390/foods14020253
![]() |
[12] |
Gray J, Chandry PS, Kaur M, et al. (2021) Colonisation dynamics of Listeria monocytogenes strains isolated from food production environments. Sci Rep 11: 12195. https://doi.org/10.1038/s41598-021-91503-w doi: 10.1038/s41598-021-91503-w
![]() |
[13] |
Rafei R, Hawli M, Osman M, et al. (2020) Distribution of EMM types and macrolide resistance determinants among group A Streptococci in the Middle East and North Africa region. J Glob Antimicrob Resist 22: 334–348. https://doi.org/10.1016/j.jgar.2020.02.005 doi: 10.1016/j.jgar.2020.02.005
![]() |
[14] |
Spanu C, Jordan K (2020) Listeria monocytogenes environmental sampling program in ready‐to‐eat processing facilities: A practical approach. Compr Rev Food Sci Food Saf 19: 2843–2861. https://doi.org/10.1111/1541-4337.12619 doi: 10.1111/1541-4337.12619
![]() |
[15] | WHO (2015) WHO Estimates of the Global Burden of Foodborne Diseases: Foodborne Disease Burden Epidemiology Reference Group 2007–2015; World Health Organization: Geneva, Switzerland. |
[16] |
Dufailu OA, Yaqub MO, Owusu-Kwarteng J, et al. (2021) Prevalence and characteristics of Listeria species from selected African countries. Trop Dis Travel Med Vaccines 7: 26. https://doi.org/10.1186/s40794-021-00151-5 doi: 10.1186/s40794-021-00151-5
![]() |
[17] | Mascellino MT (2020) Prologue: Escherichia coli, Listeria, and Salmonella, New Insight into Brucella Infection and Foodborne Diseases, IntechOpen. |
[18] |
Osek J, Lachtara B, Wieczorek K (2022) Listeria monocytogenes in foods-From culture identification to whole‐genome characteristics. Food Sci Nutr 10: 2825–2854. https://doi.org/10.1002/fsn3.2910 doi: 10.1002/fsn3.2910
![]() |
[19] |
Chen M, Cheng J, Zhang J, et al. (2019) Isolation, potential virulence, and population diversity of Listeria monocytogenes from meat and meat products in China. Front Microbiol 10: 946. https://doi.org/10.3389/fmicb.2019.00946 doi: 10.3389/fmicb.2019.00946
![]() |
[20] |
Lasagabaster A, Jiménez E, Lehnherr T, et al. (2020) Bacteriophage biocontrol to fight Listeria outbreaks in seafood. Food Chem Toxicol 145: 111682. https://doi.org/10.1016/j.fct.2020.111682 doi: 10.1016/j.fct.2020.111682
![]() |
[21] |
Miceli A, Settanni L (2019) Influence of agronomic practices and pre-harvest conditions on the attachment and development of Listeria monocytogenes in vegetables. Ann Microbiol 69: 185–199. https://doi.org/10.1007/s13213-019-1435-6 doi: 10.1007/s13213-019-1435-6
![]() |
[22] |
Inoue T, Itani T, Inomata N, et al. (2017) Listeria monocytogenes septicemia and meningitis caused by Listeria enteritis complicating ulcerative colitis. Int Med 56: 2655–2659. https://doi.org/10.2169/internalmedicine.8654-16 doi: 10.2169/internalmedicine.8654-16
![]() |
[23] |
Turner LH, Kinder JM, Wilburn A, et al. (2017) Preconceptual Zika virus asymptomatic infection protects against secondary prenatal infection. PLoS Pathog 13: e1006684. https://doi.org/10.1371/journal.ppat.1006684 doi: 10.1371/journal.ppat.1006684
![]() |
[24] |
Reda WW, Abdel-Moein K, Hegazi A, et al. (2016) Listeria monocytogenes: An emerging food-borne pathogen and its public health implications. J Infect Dev Countries 10: 149–154. https://doi.org/10.3855/jidc.6616 doi: 10.3855/jidc.6616
![]() |
[25] |
King MT, Huh I, Shenai A, et al. (2018) Structural basis of VHH-mediated neutralization of the food-borne pathogen Listeria monocytogenes. J Biol Chem 293: 13626–13635. https://doi.org/10.1074/jbc.RA118.003888 doi: 10.1074/jbc.RA118.003888
![]() |
[26] |
Nouichi S, Mezali L, Hamdi TM (2022) Distribution of Salmonella virulence factors originated from sheep and cattle in Algerian slaughterhouses. J Hell Vet Med Soc 73: 5013–5020. https://doi.org/10.12681/jhvms.29148 doi: 10.12681/jhvms.29148
![]() |
[27] |
Eckstein S, Ehmann R, Gritli A, et al. (2022) Viral and Bacterial Zoonotic Agents in Dromedary Camels from Southern Tunisia: A Seroprevalence Study. Microorganisms 10: 727. https://doi.org/10.3390/microorganisms10040727 doi: 10.3390/microorganisms10040727
![]() |
[28] |
Paré G, Trudel MC, Jaana M, et al. (2015) Synthesizing information systems knowledge: A typology of literature reviews. Inf Manage 52: 183–199. https://doi.org/10.1016/j.im.2014.08.008 doi: 10.1016/j.im.2014.08.008
![]() |
[29] |
Geteneh A, Biset S, Tadesse S, et al. (2022) A vigilant observation to pregnancy associated listeriosis in Africa: Systematic review and meta-analysis. PLOS Global Public Health 2: e0001023. https://doi.org/10.1371/journal.pgph.0001023 doi: 10.1371/journal.pgph.0001023
![]() |
[30] |
Mohamed M-YI, Abu J, Aziz SA, et al. (2022) Occurrence of antibiotic resistant C. jejuni and E. coli in wild birds, chickens, humans, and the environment in Malay villages, Kedah, Malaysia. Vet Med (Praha) 67: 298–308. https://doi.org/10.17221/102/2021-VETMED doi: 10.17221/102/2021-VETMED
![]() |
[31] |
Oueslati W, Ridha Rjeibi M, Benyedem H, et al. (2023) Serotype occurrence, virulence profiles, antimicrobial resistance and molecular characterization of Salmonella isolated from hospitalized patients with gastroenteritis in great Tunisia between 2010 and 2020. Antibiotics 12: 526. https://doi.org/10.3390/antibiotics12030526 doi: 10.3390/antibiotics12030526
![]() |
[32] |
Benhalima L, Merad T, Bensouilah M, et al. (2019) Listeria monocytogenes and other Listeria species in raw milk and sausage in East Algeria. Asian J Dairy Food Res 38: 7–11. https://doi.org/10.18805/ajdfr.DR-128 doi: 10.18805/ajdfr.DR-128
![]() |
[33] |
Mohamed M-YI, Habib I (2023) Pathogenic E. coli in the food chain across the Arab Countries: A Descriptive Review. Foods 12: 3726. https://doi.org/10.3390/foods12203726 doi: 10.3390/foods12203726
![]() |
[34] |
Mohamed M-YI, Lakshmi GB, Sodagari H, et al. (2024) A one health perspective on camel meat hygiene and zoonoses: Insights from a decade of research in the Middle East. Vet Sci 11: 344. https://doi.org/10.3390/vetsci11080344 doi: 10.3390/vetsci11080344
![]() |
[35] |
Sarr M, Alou MT, Padane A, et al. (2023) A review of the literature of Listeria monocytogenes in Africa highlights breast milk as an overlooked human source. Front Microbiol 14: 1213953. https://doi.org/10.3389/fmicb.2023.1213953 doi: 10.3389/fmicb.2023.1213953
![]() |
[36] |
Eleftherios Mylonakis MD, Maria Paliou MD, Elizabeth L. Hohmann MD, et al. (2002) Listeriosis during pregnancy a case series and review of 222 cases. Lippincott Williams & Wilkins, Inc 81: 260–269. https://doi.org/10.1097/00005792-200207000-00002 doi: 10.1097/00005792-200207000-00002
![]() |
[37] |
EL-Naenaeey E-S, Abdelwahab A, Merwad A, et al. (2019) Prevalence of Listeria species in dairy cows and pregnant women with reference to virulotyping of Listeria monocytogenes in Egypt. Zagazig Vet J 47: 248–258. https://doi.org/10.21608/zvjz.2019.12896.1042 doi: 10.21608/zvjz.2019.12896.1042
![]() |
[38] |
Koopmans MM, Brouwer MC, Vázquez-Boland JA, et al. (2023) Human listeriosis. Clin Microbiol Rev 36: No.1. https://doi.org/10.1128/cmr.00060-19 doi: 10.1128/cmr.00060-19
![]() |
[39] |
Dapgh AN, ELGedawy AA, Abouelhag HA, et al. (2020) Advanced identification and characterization of Listeria species in Egyptian local soft cheese. South Asian J Res Microbiol 8: 11–18. https://doi.org/10.9734/sajrm/2020/v8i230188 doi: 10.9734/sajrm/2020/v8i230188
![]() |
[40] |
Martinez-Rios V, Gkogka E, Dalgaard P (2020) Predicting growth of Listeria monocytogenes at dynamic conditions during manufacturing, ripening and storage of cheeses—Evaluation and application of models. Food Microbiol 92: 103578. https://doi.org/10.1016/j.fm.2020.103578 doi: 10.1016/j.fm.2020.103578
![]() |
[41] |
Abdeen EE, Mousa WS, Harb OlaH, et al. (2021) Prevalence, antibiogram and genetic characterization of Listeria monocytogenes from food products in Egypt. Foods 10: 1381. https://doi.org/10.3390/foods10061381 doi: 10.3390/foods10061381
![]() |
[42] |
Mahmoud H, Karmi M, Maky M (2019) Occurrence and characterization of Listeria species isolated from processed meat in Qena, Egypt. Zagazig Vet J 47: 267–276. https://doi.org/10.21608/zvjz.2019.13161.1044 doi: 10.21608/zvjz.2019.13161.1044
![]() |
[43] | Ahmed HA, Hussein l MA, El-Ashram AMM (2013) Seafood a potential source of some zoonotic bacteria in Zagazig, Egypt, with the molecular detection of Listeria monocytogenes virulence genes. Vet Ital 49: 299–308. |
[44] |
Saleh SO, Hussien AA, Youseef AG, et al. (2024) Prevalence, antibiotic resistance, and phylogenetic analysis of Listeria monocytogenes isolated from various sources in Egypt: fish, vegetables, and humans. Iraqi J Vet Sci 38: 15–27. https://doi.org/10.33899/ijvs.2023.140242.3033 doi: 10.33899/ijvs.2023.140242.3033
![]() |
[45] |
Alegbeleye O, Sant'Ana AS (2022) Impact of temperature, soil type and compost amendment on the survival, growth and persistence of Listeria monocytogenes of non-environmental (food-source associated) origin in soil. Sci Total Environ 843: 157033. https://doi.org/10.1016/j.scitotenv.2022.157033 doi: 10.1016/j.scitotenv.2022.157033
![]() |
[46] |
Gartley S, Anderson-Coughlin B, Sharma M, et al. (2022) Listeria monocytogenes in irrigation water: An assessment of outbreaks, sources, prevalence, and persistence. Microorganisms 10: 1319. https://doi.org/10.3390/microorganisms10071319 doi: 10.3390/microorganisms10071319
![]() |
[47] |
Osek J, Lachtara B, Wieczorek K (2022) Listeria monocytogenes – how this pathogen survives in food-production environments? Front Microbiol 13: 866462. https://doi.org/10.3389/fmicb.2022.866462 doi: 10.3389/fmicb.2022.866462
![]() |
[48] |
Osman KM, Kappell AD, Fox EM, et al. (2019) Prevalence, pathogenicity, virulence, antibiotic resistance, and phylogenetic analysis of biofilm-producing Listeria monocytogenes isolated from different ecological niches in Egypt: food, humans, animals, and environment. Pathogens 9: 5. https://doi.org/10.3390/pathogens9010005 doi: 10.3390/pathogens9010005
![]() |
[49] |
Kulesh R, Shinde SV, Khan WA, et al. (2022) The occurrence of Listeria monocytogenes in goats, farm environment and invertebrates. Biol Rhythm Res 53: 831–840. https://doi.org/10.1080/09291016.2019.1660836 doi: 10.1080/09291016.2019.1660836
![]() |
[50] |
Shafik S, Abdelrahman MA (2019) Molecular characterization of Listeria monocytogenes isolated from some abattoirs in Dakahlia, Egypt. Assiut Vet Med J 65: 95–103. https://doi.org/10.21608/avmj.2019.168762 doi: 10.21608/avmj.2019.168762
![]() |
[51] | ECDC/EFSA/EMA (2015) ECDC/EFSA/EMA (European Centre for Disease Prevention and Control, European Food Safety Authority and European Medicines Agency) (2015) First joint report on the integrated analysis of the consumption of antimicrobial agents and occurrence of antimicrobial resistance in bacteria from humans and food-producing animals. EFSA J13: 4006. https://doi.org/10.2903/j.efsa.2015.4006 |
[52] |
Habib I, Mohteshamuddin K, Mohamed M-YI, et al. (2023) Domestic pets in the United Arab Emirates as reservoirs for antibiotic-resistant bacteria: A comprehensive analysis of extended-spectrum beta-lactamase producing Escherichia coli prevalence and risk factors. Animals 13: 1587. https://doi.org/10.3390/ani13101587 doi: 10.3390/ani13101587
![]() |
[53] |
Djomgoue NG, Fonbah LJ, Mbulli AI, et al. (2023) Risk factors and associated outcomes of virulence genes eae, entB, and pipD carriage in Escherichia coli, Klebsiella pneumoniae, and Salmonella spp. from HIV-1 and HIV-negative gastroenteritis patients in the Dschang Regional Hospital Annex. Cureus 15: e42329. https://doi.org/10.7759/cureus.42329 doi: 10.7759/cureus.42329
![]() |
[54] | Abdellaoui L, Bouayad L, Bensefia SA, et al. (2020) Serotyping and antibiotic sensitivity of Listeria monocytogenes isolated from cheeses produced in the region of Algiers (Algeria). Veterinaria 69: 43–49. |
[55] |
Hamdi TM, Naïm M, Martin P, et al. (2007) Identification and molecular characterization of Listeria monocytogenes isolated in raw milk in the region of Algiers (Algeria). Int J Food Microbiol 116: 190–193. https://doi.org/10.1016/j.ijfoodmicro.2006.12.038 doi: 10.1016/j.ijfoodmicro.2006.12.038
![]() |
[56] |
Bouayad L, Hamdi T-M (2012) Prevalence of Listeria spp. in ready to eat foods (RTE) from Algiers (Algeria). Food Control 23: 397–399. https://doi.org/10.1016/j.foodcont.2011.08.006 doi: 10.1016/j.foodcont.2011.08.006
![]() |
[57] |
Zakaria AI, Sabala RF (2024) Potential public health hazards related to consumption of poultry contaminated with antibiotic resistant Listeria monocytogenes in Egypt. BMC Microbiol 24: 41. https://doi.org/10.1186/s12866-024-03183-x doi: 10.1186/s12866-024-03183-x
![]() |
[58] |
Abou-Khadra S, El-Azzouny M, EL-Sheikh S (2024) Occurrence, serodiagnosis and antimicrobial resistance of Listeria monocytogenes isolated from different types of meat products and milk. Alex J Vet Sci 80: 55. https://doi.org/10.5455/ajvs.184100 doi: 10.5455/ajvs.184100
![]() |
[59] |
Abou Elez RMM, Elsohaby I, Al-Mohammadi A-R, et al. (2023) Antibacterial and anti-biofilm activities of probiotic Lactobacillus plantarum against Listeria monocytogenes isolated from milk, chicken and pregnant women. Front Microbiol 14: 1201201. https://doi.org/10.3389/fmicb.2023.1201201 doi: 10.3389/fmicb.2023.1201201
![]() |
[60] |
Radwan M, Helmy N, Mousa M (2022) Assessment of proteomic and molecular techniques in isolation of Listeria monocytogenes in minced meat. Alex J Vet Sci 74: 31. https://doi.org/10.5455/ajvs.19829 doi: 10.5455/ajvs.19829
![]() |
[61] |
Elsayed MM, Elkenany RM, Zakaria AI, et al. (2022) Epidemiological study on Listeria monocytogenes in Egyptian dairy cattle farms' insights into genetic diversity of multi-antibiotic-resistant strains by ERIC-PCR. Environ Sci Pollut 29: 54359–54377. https://doi.org/10.1007/s11356-022-19495-2 doi: 10.1007/s11356-022-19495-2
![]() |
[62] |
Gamal B, El-Malt LM, Abdel Hameed KG, et al. (2022) Evaluation the antibacterial effect of hydroalcoholic coffee extract on L. monocytogenes isolated from milk and milk products. SVU-Int J Vet Sci 5: 38–51. https://doi.org/10.21608/svu.2022.131641.1190 doi: 10.21608/svu.2022.131641.1190
![]() |
[63] | Ahmed SA, Mostafa AHM, El-Sherbini M, et al. (2022) Assessment of microbial safety and quality of market raw milk and pasteurized milk sold in Dakahlia governorate, Egypt. J Adv Vet Res 12: 456–461. |
[64] |
Ewida RM, Hasan WS, Elfaruk MS, et al. (2022) Occurrence of Listeria spp. in soft cheese and ice cream: effect of probiotic Bifidobacterium spp. On survival of Listeria monocytogenes in soft cheese. Foods 11: 3443. https://doi.org/10.3390/foods11213443 doi: 10.3390/foods11213443
![]() |
[65] |
Mohamed HMA, Katreen KG, Abd Al-Azeem MW, et al. (2022) Molecular detection of Listeria species isolated from raw milk with special reference to virulence determinants and antimicrobial resistance in Listeria monocytogenes. J Anim Health Prod 10: 492–505. https://doi.org/10.17582/journal.jahp/2022/10.4.492.505 doi: 10.17582/journal.jahp/2022/10.4.492.505
![]() |
[66] |
Dapgh AN, Salem RL (2022) Molecular detection of Listeria monocytogenes in milk and some milk products. Int J Vet Sci 514–519. https://doi.org/10.47278/journal.ijvs/2021.128 doi: 10.47278/journal.ijvs/2021.128
![]() |
[67] |
Badawy B, Gwida M, Sadat A, et al. (2022) Prevalence and antimicrobial resistance of virulent Listeria monocytogenes and Cronobacter sakazakii in dairy cattle, the environment, and dried milk with the in vitro application of natural alternative control. Antibiotics 11: 1087. https://doi.org/10.3390/antibiotics11081087 doi: 10.3390/antibiotics11081087
![]() |
[68] |
El-Baz AH, Elsayed MM (2021) Prevalence of Listeria monocytogens in farm milk and some dairy products with comparing its sensitivity to different antibiotics and H2O2 loaded on silver nanoparticles in Dakahlia governorate, Egypt. Alex J Vet Sci 68: 71–81. https://doi.org/10.5455/ajvs.30487 doi: 10.5455/ajvs.30487
![]() |
[69] |
Saleh E, El-Boudy A, Elsayed A, et al. (2021) Molecular characterization of Listeria monocytogenes isolated from raw milk and some dairy products at local markets in Damanhour city, Egypt. Damanhour J Vet Sci 6: 1–6. https://doi.org/10.21608/djvs.2021.187849 doi: 10.21608/djvs.2021.187849
![]() |
[70] |
Hassanien A, Shaker E (2021) Virulence potential of Listeria monocytogenes recovered from Ice cream and aborted women samples in Sohag city, Egypt. Adv Anim Vet Sci 9: 2309–3331. https://doi.org/10.17582/journal.aavs/2021/9.11.1829.1837 doi: 10.17582/journal.aavs/2021/9.11.1829.1837
![]() |
[71] |
Baher W, Shalaby M, Abdelghfar S (2021) Prevalence of multidrug-resistant Listeria monocytogenes in retailed goat meat and offal. Damanhour J. Vet. Sci 7: 19–22. https://doi.org/10.21608/djvs.2021.106468.1058 doi: 10.21608/djvs.2021.106468.1058
![]() |
[72] |
Harb O, Elbab G, Shawish R, et al. (2020) Genetic detection of Listeria monocytogenes recovered from fillet fish samples. Alex J Vet Sci 67: 74–79. https://doi.org/10.5455/ajvs.13697 doi: 10.5455/ajvs.13697
![]() |
[73] |
Abdel Aziz SAA, Mohamed MBED (2020) Prevalence, virulence genes, and antimicrobial resistance profile of Listeria monocytogenes isolated from retail poultry shops in Beni-Suef city, Egypt. J Adv Vet Anim Res 7: 710. https://doi.org/10.5455/javar.2020.g472 doi: 10.5455/javar.2020.g472
![]() |
[74] |
Mohamed RI, Abdelmonem MA, Amin HM (2018) Virulence and antimicrobial susceptibility profile of Listeria monocytogenes isolated from frozen vegetables available in the Egyptian market. Afr J Microbiol Res 12: 218–224. https://doi.org/10.5897/AJMR2018.8794 doi: 10.5897/AJMR2018.8794
![]() |
[75] |
Abd El-Tawab AA, Homouda SN, El-Haw SE (2018) Prevalence and molecular studies on Listeria monocytogenes isolated from chicken in El-Gharbia Governorate. Benha Vet Med J 34: 430–442. https://doi.org/10.21608/bvmj.2018.54506 doi: 10.21608/bvmj.2018.54506
![]() |
[76] |
Tahoun ABMB, Abou Elez RMM, Abdelfatah EN, et al. (2017) Listeria monocytogenes in raw milk, milking equipment and dairy workers: Molecular characterization and antimicrobial resistance patterns. J Glob Antimicrob Resist 10: 264–270. https://doi.org/10.1016/j.jgar.2017.07.008 doi: 10.1016/j.jgar.2017.07.008
![]() |
[77] |
Elshinawa SHH, Meshref AMS, Zeinhom MMA, et al. (2017) Incidence of Listeria species in some dairy products in Beni-Suef governorate. Assiut Vet Med J 63: 5–13. https://doi.org/10.21608/avmj.2016.169210 doi: 10.21608/avmj.2016.169210
![]() |
[78] |
Mohamed Y, Reda WW, Abdel-Moein K, et al. (2016) Prevalence and phylogenetic characterization of Listeria monocytogenes isolated from processed meat marketed in Egypt. J Genet Eng Biotechnol 14: 119–123. https://doi.org/10.1016/j.jgeb.2016.06.001 doi: 10.1016/j.jgeb.2016.06.001
![]() |
[79] | Ebaya MMA, AL-Ashmawy MAM, EL-Sokkary MM, et al. (2016) Comparative study on prevalence and antimicrobial susceptibility of Escherichia coli and Listeria monocytogenes isolated from raw milk in Egypt. New Egypt J Microbiol 43: 145–158. |
[80] |
Meshref AM, Zeinhom MM, Abdel-Atty NS (2015) Occurrence and distribution of Listeria species in some Egyptian foods. Alex J Vet Sci 46: 42–47. https://doi.org/10.5455/ajvs.187647 doi: 10.5455/ajvs.187647
![]() |
[81] |
Shaker EM, Hassanien AA (2015) PCR technique for detection of some virulence associated genes in Listeria monocytogenes isolated from table eggs and clinical human samples. Assiut Vet Med J 61: 219–225. https://doi.org/10.21608/avmj.2015.170040 doi: 10.21608/avmj.2015.170040
![]() |
[82] |
Awadallah MAI, Suelam ⅡA (2014) Characterization of virulent Listeria monocytogenes isolates recovered from ready-to-eat meat products and consumers in Cairo, Egypt. Vet World 7: 788–793. https://doi.org/10.14202/vetworld.2014.788-793 doi: 10.14202/vetworld.2014.788-793
![]() |
[83] | Al-Ashmawy MAM, Abdelgalil MMGKH (2014) Prevalence, detection methods and antimicrobial susceptibility of Listeria monocytogens isolated from milk and soft cheeses and its zoonotic importance. World Appl Sci J 29: 869–878. |
[84] |
Ismaiel AA-R, Ali AE-S, Enan G (2014) Incidence of Listeria in Egyptian meat and dairy samples. Food Sci Biotechnol 23: 179–185. https://doi.org/10.1007/s10068-014-0024-5 doi: 10.1007/s10068-014-0024-5
![]() |
[85] |
Abdellrazeq G, Kamar A, ElHoushy S (2014) Molecular characterization of Listeria species isolated from frozen fish. Alex J Vet Sci 40: 1. https://doi.org/10.5455/ajvs.45443 doi: 10.5455/ajvs.45443
![]() |
[86] | Edris AM, Amany M.S, Fouad MA (2014) Incidence of Listeria monocytogenes in fresh Tilapia Nilotica fish. Benha Vet Med J 26: 120–126. |
[87] |
El-Shenawy M, El-Shenawy M, Mañes J, et al. (2011) Listeria spp. in street-vended ready-to-eat foods. Interdiscip Perspect Infect Dis 2011: 1–6. https://doi.org/10.1155/2011/968031 doi: 10.1155/2011/968031
![]() |
[88] |
ElMalek A, Ali S, Hassanein R, et al. (2010) Occurrence of Listeria species in meat, chicken products and human stools in Assiut city, Egypt with PCR use for rapid identification of Listeria monocytogenes. Vet World 3: 353–359. https://doi.org/10.5455/vetworld.2010.353-359 doi: 10.5455/vetworld.2010.353-359
![]() |
[89] | Sayed M, Abdel-Azeem M, Farghaly M, et al. (2009) Using of PCR assay for identification of Listeria monocytogenes recovered from table eggs. Vet World 2: 453–455. |
[90] | Hesham TN, Hanan LE, Fathi AT, et al. (2017) Prevalence of Listeria spp. among Dairy, Meat and their Products Marketed in Tripoli, Libya. Int J Life Sci Res 5: 19–25. |
[91] |
El-Sharef N, Ghenghesh KS, Abognah YS, et al. (2006) Bacteriological quality of ice cream in Tripoli-Libya. Food Control 17: 637–641. https://doi.org/10.1016/j.foodcont.2005.04.001 doi: 10.1016/j.foodcont.2005.04.001
![]() |
[92] |
Bouymajane A, Rhazi Filali F, Oulghazi S, et al. (2021) Occurrence, antimicrobial resistance, serotyping and virulence genes of Listeria monocytogenes isolated from foods. Heliyon 7: e06169. https://doi.org/10.1016/j.heliyon.2021.e06169 doi: 10.1016/j.heliyon.2021.e06169
![]() |
[93] |
Boukili M, Rhazi Filali F, Lafkih N, et al. (2020) Prevalence, characterization and antimicrobial resistance of Listeria monocytogenes isolated from beef meat in Meknes city, Morocco. Germs 10: 74–80. https://doi.org/10.18683/germs.2020.1180 doi: 10.18683/germs.2020.1180
![]() |
[94] |
Amajoud N, Leclercq A, Soriano JM, et al. (2018) Prevalence of Listeria spp. and characterization of Listeria monocytogenes isolated from food products in Tetouan, Morocco. Food Control 84: 436–441. https://doi.org/10.1016/j.foodcont.2017.08.023 doi: 10.1016/j.foodcont.2017.08.023
![]() |
[95] |
Marnissi E, Bennani L, Cohen N, et al. (2013) Presence of Listeria monocytogenes in raw milk and traditional dairy products marketed in the north-central region of Morocco. Afr J Food Sci 7: 87–91. https://doi.org/10.5897/AJFS2013.0992 doi: 10.5897/AJFS2013.0992
![]() |
[96] |
Ennaji H, Timinouni M, Ennaji MM, et al. (2008) Characterization and antibiotic susceptibility of Listeria monocytogenes isolated from poultry and red meat in Morocco. Infect Drug Resist 1: 45–50. https://doi.org/10.2147/IDR.S3632 doi: 10.2147/IDR.S3632
![]() |
[97] |
Hmaïed F, Helel S, Le berre V, et al. (2014) Prevalence, identification by a DNA microarray-based assay of human and food isolates Listeria spp. from Tunisia. Pathologie Biologie 62: 24–29. https://doi.org/10.1016/j.patbio.2013.10.005 doi: 10.1016/j.patbio.2013.10.005
![]() |
[98] |
Mohamed M-YI, Abu J, Zakaria Z, et al. (2022) Multi-drug resistant pathogenic Escherichia coli isolated from wild birds, chicken, and the environment in Malaysia. Antibiotics 11: 1275. https://doi.org/10.3390/antibiotics11101275 doi: 10.3390/antibiotics11101275
![]() |
[99] |
Lakicevic BZ, Den Besten HMW, De Biase D (2022) Landscape of stress response and virulence genes among Listeria monocytogenes strains. Front Microbiol 12: 738470. https://doi.org/10.3389/fmicb.2021.738470 doi: 10.3389/fmicb.2021.738470
![]() |
[100] | Alía A, Martín I, Andrade MJ, et al. (2024) Chapter 20—Listeria monocytogenes cell-to-cell spread. In: Tang Y, Hindiyeh M, Liu D, et al. (Eds.), Molecular Medical Microbiology, Elsevier, 391–406. https://doi.org/10.1016/B978-0-12-818619-0.00017-4 |
[101] |
Ireton K, Mortuza R, Gyanwali GC, et al. (2021) Role of internalin proteins in the pathogenesis of Listeria monocytogenes. Mol Microbiol 116: 1407–1419. https://doi.org/10.1111/mmi.14836 doi: 10.1111/mmi.14836
![]() |
[102] |
Cheng C, Sun J, Yu H, et al. (2020) Listeriolysin O pore-forming activity is required for ERK1/2 phosphorylation during Listeria monocytogenes infection. Front Immunol 11: 1146. https://doi.org/10.3389/fimmu.2020.01146 doi: 10.3389/fimmu.2020.01146
![]() |
[103] |
Wang J, Cui M, Liu Y, et al. (2024) The mitochondrial carboxylase PCCA interacts with Listeria monocytogenes phospholipase PlcB to modulate bacterial survival. Appl Environ Microbiol 90: No.6. https://doi.org/10.1128/aem.02135-23 doi: 10.1128/aem.02135-23
![]() |
[104] |
Vazquez-Armenta FJ, Hernandez-Oñate MA, Martinez-Tellez MA, et al. (2020) Quercetin repressed the stress response factor (sigB) and virulence genes (prfA, actA, inlA, and inlC), lower the adhesion, and biofilm development of L. monocytogenes. Food Microbiol 87: 103377. https://doi.org/10.1016/j.fm.2019.103377 doi: 10.1016/j.fm.2019.103377
![]() |
[105] |
dos Santos PT, Thomasen RSS, Green MS, et al. (2020) Free fatty acids interfere with the DNA binding activity of the virulence regulator PrfA of Listeria monocytogenes. J Bacteriol 202: No.15. https://doi.org/10.1128/JB.00156-20 doi: 10.1128/JB.00156-20
![]() |
[106] |
Wiktorczyk-Kapischke N, Skowron K, Wałecka-Zacharska E (2023) Genomic and pathogenicity islands of Listeria monocytogenes—overview of selected aspects. Front Mol Biosci 10: 1161486. https://doi.org/10.3389/fmolb.2023.1161486 doi: 10.3389/fmolb.2023.1161486
![]() |
[107] |
Lecuit M (2020) Listeria monocytogenes, a model in infection biology. Cell Microbiol 22: 13186. https://doi.org/10.1111/cmi.13186 doi: 10.1111/cmi.13186
![]() |
[108] |
Hochnadel I, Hoenicke L, Petriv N, et al. (2022) Safety and efficacy of prophylactic and therapeutic vaccine based on live-attenuated Listeria monocytogenes in hepatobiliary cancers. Oncogene 41: 2039–2053. https://doi.org/10.1038/s41388-022-02222-z doi: 10.1038/s41388-022-02222-z
![]() |
[109] |
Edosa TT, Jo YH, Keshavarz M, et al. (2020) TmAtg6 plays an important role in anti-microbial defense against Listeria monocytogenes in the mealworm, Tenebrio Molitor. Int J Mol Sci 21: 1232. https://doi.org/10.3390/ijms21041232 doi: 10.3390/ijms21041232
![]() |
[110] |
Gergis AI, Hafez MR, Mohammed ZA, et al. (2024) Phenotypic and genotypic characterization of Listeria monocytogenes isolated from raw milk in Assiut city. Assiut Vet Med J 70: 232–243. https://doi.org/10.21608/avmj.2024.253310.1209 doi: 10.21608/avmj.2024.253310.1209
![]() |
[111] |
Sotohy SA, Elnaker YF, Omar AM, et al. (2024) Prevalence, antibiogram and molecular characterization of Listeria monocytogenes from ruminants and humans in New Valley and Beheira Governorates, Egypt. BMC Vet Res 20: 297. https://doi.org/10.1186/s12917-024-04138-0 doi: 10.1186/s12917-024-04138-0
![]() |
[112] |
Hasan AM (2023) Phenotypic and genotypic of Listeria monocytogenes isolated from raw milk in Assiut governorate. Int J Adv Sci Res Innovation 6: 92–113. https://doi.org/10.21608/ijasri.2023.246924.1013 doi: 10.21608/ijasri.2023.246924.1013
![]() |
[113] |
El-Demerdash AS, Raslan MT (2019) Molecular characterization of Listeria monocytogenes isolated from different animal-origin food Items from urban and rural areas. Adv Anim Vet Sci 7: 51–56. https://doi.org/10.17582/journal.aavs/2019/7.s2.51.56 doi: 10.17582/journal.aavs/2019/7.s2.51.56
![]() |
[114] |
Elsayed ME, Abd El-Hamid MI, El-Gedawy A, et al. (2022) New Insights into Listeria monocytogenes antimicrobial resistance, virulence attributes and their prospective correlation. Antibiotics 11: 1447. https://doi.org/10.3390/antibiotics11101447 doi: 10.3390/antibiotics11101447
![]() |
[115] |
Elbar S, Elkenany R, Elhadidy M, et al. (2020) Prevalence, virulence and antibiotic susceptibility of Listeria monocytogenes isolated from sheep. Mansoura Vet Med J 21: 48–52. https://doi.org/10.35943/mvmj.2020.21.2.207 doi: 10.35943/mvmj.2020.21.2.207
![]() |
[116] | Eldaly EA, El-Gaml AM, Elkenawy ME (2013) Incidence of Listeria monocytogenes in some slaughtered animals at Dakahlia province slaughterhouses—Egypt. Available from: https://www.researchgate.net/profile/Elsaid-Eldaly/publication/236681822_Incidence_of_Listeria_monocytogenes_in_some_slaughtered_animals_at_Dakahlia_province_slaughterhouses_-Egypt/links/00b7d518fd450f133b000000/Incidence-of-Listeria-monocytogenes-in-some-slaughtered-animals-at-Dakahlia-province-slaughterhouses-Egypt.pdf. |
[117] |
ECDC, EFSA (2022) The European Union Summary Report on Antimicrobial Resistance in zoonotic and indicator bacteria from humans, animals and food in 2019–2020. EFSA J 20: e07209. https://doi.org/10.2903/j.efsa.2022.7209 doi: 10.2903/j.efsa.2022.7209
![]() |
[118] |
Stavropoulos C, Tolentino B, Woods K, et al. (2021) Listeria rhomboencephalitis in an immunocompetent host: Treatment with trimethoprim-sulfamethoxazole and ampicillin: A case report and review of treatment options. Inf Dis Clin Pract 29: e204–e207. https://doi.org/10.1097/IPC.0000000000001024 doi: 10.1097/IPC.0000000000001024
![]() |
[119] |
Sutter JP, Kocheise L, Kempski J, et al. (2024) Gentamicin combination treatment is associated with lower mortality in patients with invasive listeriosis: A retrospective analysis. Infection 52: 1601–1606. https://doi.org/10.1007/s15010-024-02330-w doi: 10.1007/s15010-024-02330-w
![]() |
[120] |
Khalifa HO, Shikoray L, Mohamed M-YI, et al. (2024) Veterinary drug residues in the food chain as an emerging public health threat: Sources, analytical methods, health impacts, and preventive measures. Foods 13: 1629. https://doi.org/10.3390/foods13111629 doi: 10.3390/foods13111629
![]() |
[121] |
Andriyanov PA, Zhurilov PA, Liskova EA, et al. (2021) Antimicrobial resistance of Listeria monocytogenes strains isolated from humans, animals, and food products in Russia in 1950–1980, 2000–2005, and 2018–2021. Antibiotics 10: 1206. https://doi.org/10.3390/antibiotics10101206 doi: 10.3390/antibiotics10101206
![]() |
[122] |
Ermolaeva SA, Karpova TI, Andriyanov PA, et al. (2022) Distribution of antimicrobial resistance among clinical and food Listeria monocytogenes isolated in Moscow in 2019–2021. Clin Microbiol Antimicrob Chemother 24: 156–164. https://doi.org/10.36488/cmac.2022.2.156-164 doi: 10.36488/cmac.2022.2.156-164
![]() |
[123] |
Rodríguez-Villodres Á, Lepe JA, Blázquez J, et al. (2020) Effect of subinhibitory concentrations of ampicillin on Listeria monocytogenes. Enferm Infecc Microbiol Clin 38: 72–75. https://doi.org/10.1016/j.eimc.2019.03.010 doi: 10.1016/j.eimc.2019.03.010
![]() |
[124] |
Habib I, Elbediwi M, Mohamed M-YI, et al. (2023) Enumeration, antimicrobial resistance and genomic characterization of extended-spectrum β-lactamases producing Escherichia coli from supermarket chicken meat in the United Arab Emirates. Int J Food Microbiol 398: 110224. https://doi.org/10.1016/j.ijfoodmicro.2023.110224 doi: 10.1016/j.ijfoodmicro.2023.110224
![]() |
[125] |
Ibrahim MJ, Abdul-Aziz S, Bitrus AA, et al. (2018) Occurrence of multidrug resistant (MDR) Campylobacter species isolated from retail chicken meats in Selangor, Malaysia and their associated risk factors. Malays J Microbiol 14: 261–272. https://doi.org/10.21161/mjm.107717 doi: 10.21161/mjm.107717
![]() |
[126] |
El-Gohary FA, Abdel-Hafez LJM, Zakaria AI, et al. (2020) Enhanced antibacterial activity of silver nanoparticles combined with hydrogen peroxide against multidrug-resistant pathogens isolated from dairy farms and beef slaughterhouses in Egypt. Infect Drug Resist Volume 13: 3485–3499. https://doi.org/10.2147/IDR.S271261 doi: 10.2147/IDR.S271261
![]() |
[127] | Mohamed M-YI, Abdul-Aziz S, Jalila A, et al. (2019) Occurrence of antibiotic resistant Campylobacter in wild birds and poultry. Malays J Microbiol 15: 2. |
[128] |
Habib I, Mohamed MYI, Lakshmi GB, et al. (2022) Quantification of Campylobacter contamination on chicken carcasses sold in retail markets in the United Arab Emirates. Int J Food Contam 9: 9. https://doi.org/10.1186/s40550-022-00095-4 doi: 10.1186/s40550-022-00095-4
![]() |
[129] |
AbdelRahman MAA, Roshdy H, Samir AH, et al. (2020) Antibiotic resistance and extended-spectrum β-lactamase in Escherichia coli isolates from imported 1-day-old chicks, ducklings, and turkey poults. Vet World 13: 1037–1044. https://doi.org/10.14202/vetworld.2020.1037-1044 doi: 10.14202/vetworld.2020.1037-1044
![]() |
[130] |
Mohamed M-YI, Habib I, Khalifa HO (2024) Salmonella in the food chain within the Gulf Cooperation Council countries. AIMS Microbiol 10: 468–488. https://doi.org/10.3934/microbiol.2024023 doi: 10.3934/microbiol.2024023
![]() |
[131] |
Mohamed M-YI, Abu J, Abdul-Aziz S, et al. (2019) Occurrence of antibiotic resistant C. jejuni and E. coli in wild birds, chickens, environment and humans from Orang Asli Villages in Sungai Siput, Perak, Malaysia. Am J Anim Vet Sci 14: 158–169. https://doi.org/10.3844/ajavsp.2019.158.169 doi: 10.3844/ajavsp.2019.158.169
![]() |
[132] |
Habib I, Elbediwi M, Mohteshamuddin K, et al. (2023) Genomic profiling of extended-spectrum β-lactamase-producing Escherichia coli from pets in the United Arab Emirates: Unveiling colistin resistance mediated by mcr-1.1 and its probable transmission from chicken meat—A One Health perspective. J Infect Public Health 16: 163–171. https://doi.org/10.1016/j.jiph.2023.10.034 doi: 10.1016/j.jiph.2023.10.034
![]() |
1. | Peng Xue, Mingyu Si, Dongxu Qin, Bingrui Wei, Samuel Seery, Zichen Ye, Mingyang Chen, Sumeng Wang, Cheng Song, Bo Zhang, Ming Ding, Wenling Zhang, Anying Bai, Huijiao Yan, Le Dang, Yuqian Zhao, Remila Rezhake, Shaokai Zhang, Youlin Qiao, Yimin Qu, Yu Jiang, Unassisted Clinicians Versus Deep Learning–Assisted Clinicians in Image-Based Cancer Diagnostics: Systematic Review With Meta-analysis, 2023, 25, 1438-8871, e43832, 10.2196/43832 | |
2. | Anastasia-Maria Leventi-Peetz, Kai Weber, Probabilistic machine learning for breast cancer classification, 2022, 20, 1551-0018, 624, 10.3934/mbe.2023029 | |
3. | Peng-fei Lyu, Yu Wang, Qing-Xiang Meng, Ping-ming Fan, Ke Ma, Sha Xiao, Xun-chen Cao, Guang-Xun Lin, Si-yuan Dong, Mapping intellectual structures and research hotspots in the application of artificial intelligence in cancer: A bibliometric analysis, 2022, 12, 2234-943X, 10.3389/fonc.2022.955668 | |
4. | Xiaolei Wang, Shuang Meng, Diagnostic accuracy of S-Detect to breast cancer on ultrasonography: A meta-analysis (PRISMA), 2022, 101, 1536-5964, e30359, 10.1097/MD.0000000000030359 | |
5. | Samara Acosta-Jiménez, Javier Camarillo-Cisneros, Abimael Guzmán-Pando, Susana Aideé González-Chávez, Jorge Issac Galván-Tejada, Graciela Ramírez-Alonso, César Francisco Pacheco-Tena, Rosa Elena Ochoa-Albiztegui, 2023, Chapter 8, 978-3-031-18255-6, 83, 10.1007/978-3-031-18256-3_8 | |
6. | Yuqun Wang, Lei Tang, Pingping Chen, Man Chen, The Role of a Deep Learning-Based Computer-Aided Diagnosis System and Elastography in Reducing Unnecessary Breast Lesion Biopsies, 2022, 15268209, 10.1016/j.clbc.2022.12.016 | |
7. | Peizhen Huang, Bin Zheng, Mengyi Li, Lin Xu, Sajjad Rabbani, Abdulilah Mohammad Mayet, Chengchun Chen, Beishu Zhan, He Jun, Ateeq Ur Rehman, The Diagnostic Value of Artificial Intelligence Ultrasound S-Detect Technology for Thyroid Nodules, 2022, 2022, 1687-5273, 1, 10.1155/2022/3656572 | |
8. | Qiyu Liu, Meijing Qu, Lipeng Sun, Hui Wang, Accuracy of ultrasonic artificial intelligence in diagnosing benign and malignant breast diseases, 2021, 100, 0025-7974, e28289, 10.1097/MD.0000000000028289 | |
9. | N.N. Bayandina, E.N. Slavnova, A cytological method in the early diagnosis of cervical cancer: evolution, principles, technologies, prospects, 2023, 12, 2305-218X, 49, 10.17116/onkolog20231202149 | |
10. | Peng Sun, 2023, Chapter 35, 978-981-19-9372-5, 323, 10.1007/978-981-19-9373-2_35 | |
11. | Hee Jeong Kim, Hak Hee Kim, Ki Hwan Kim, Ji Sung Lee, Woo Jung Choi, Eun Young Chae, Hee Jung Shin, Joo Hee Cha, Woo Hyun Shim, Use of a commercial artificial intelligence-based mammography analysis software for improving breast ultrasound interpretations, 2024, 34, 1432-1084, 6320, 10.1007/s00330-024-10718-3 | |
12. | E. L. Teodozova, E. Yu. Khomutova, Artificial intelligence in radial diagnostics of breast cancer, 2023, 3, 2782-3024, 26, 10.61634/2782-3024-2023-12-26-35 | |
13. | Xiao Zou, Jintao Zhai, Shengyou Qian, Ang Li, Feng Tian, Xiaofei Cao, Runmin Wang, Improved breast ultrasound tumor classification using dual-input CNN with GAP-guided attention loss, 2023, 20, 1551-0018, 15244, 10.3934/mbe.2023682 | |
14. | Lu Li, Hongyan Deng, Xinhua Ye, Yong Li, Jie Wang, Comparison of the diagnostic efficacy of mathematical models in distinguishing ultrasound imaging of breast nodules, 2023, 13, 2045-2322, 10.1038/s41598-023-42937-x | |
15. | Na Li, Wanling Liu, Yunyun Zhan, Yu Bi, Xiabi Wu, Mei Peng, Value of S-Detect combined with multimodal ultrasound in differentiating malignant from benign breast masses, 2024, 55, 2090-4762, 10.1186/s43055-023-01183-x | |
16. | Pengjie Song, Li Zhang, Longmei Bai, Qing Wang, Yanlei Wang, Diagnostic performance of ultrasound with computer-aided diagnostic system in detecting breast cancer, 2023, 9, 24058440, e20712, 10.1016/j.heliyon.2023.e20712 | |
17. | Qing Dan, Ziting Xu, Hannah Burrows, Jennifer Bissram, Jeffrey S. A. Stringer, Yingjia Li, Diagnostic performance of deep learning in ultrasound diagnosis of breast cancer: a systematic review, 2024, 8, 2397-768X, 10.1038/s41698-024-00514-z | |
18. | Panpan Zhang, Min Zhang, Menglin Lu, Chaoying Jin, Gang Wang, Xianfang Lin, Comparative Analysis of the Diagnostic Value of S-Detect Technology in Different Planes Versus the BI-RADS Classification for Breast Lesions, 2024, 10766332, 10.1016/j.acra.2024.08.005 | |
19. | Zhuohua Lin, Ligang Cui, Yan Xu, Qiang Fu, Youjing Sun, Feasibility and potential of intraoperative ultrasound in arthroscopy of femoroacetabular impingement, 2024, 2054-8397, 10.1093/jhps/hnad050 | |
20. | Manisha Bahl, Jung Min Chang, Lisa A. Mullen, Wendie A. Berg, Artificial Intelligence for Breast Ultrasound: AJR Expert Panel Narrative Review, 2024, 0361-803X, 1, 10.2214/AJR.23.30645 | |
21. | Jie He, Nan Liu, Li Zhao, New progress in imaging diagnosis and immunotherapy of breast cancer, 2025, 16, 1664-3224, 10.3389/fimmu.2025.1560257 |
Group | AUC | Sensitivity (%) | Specificity (%) | Accuracy (%) |
AI | 0.948 | 95.8 | 93.8 | 89.6 |
Elastic image | 0.865 | 79.2 | 93.8 | 73.0 |
Gray-scale | 0.719 | 75.0 | 68.8 | 43.8 |
Before assistance | S-Detect | After assistance |
Category 8 | B/B 7 | Category 3 8 |
M/B 1 | ||
Category4a 13 | B/B 6 | Category 3 3 |
M/B 4 | Category 4a 4 | |
M/M 3 | Category 4b 4 | |
Category 4c 2 | ||
Category 4b 11 | B/B 2 | Category 4a 2 |
M/B 4 | Category 4b 6 | |
M/M 5 | Category 4c 3 | |
Category 4c 6 | M/B 2 | Category 4c 6 |
M/M 4 | ||
Category 5 2 | M/M 2 | Category 5 2 |
Group | Area under ROC curve | Sensitivity (%) | Specificity (%) | Accuracy (%) |
Senior | 0.802 | 79.2 | 81.3 | 60.5 |
Junior | 0.719 | 75.0 | 68.8 | 43.8 |
Senior+AI | 0.969 | 100.0 | 93.8 | 93.8 |
Junior+AI | 0.948 | 95.8 | 93.8 | 89.6 |
Group | AUC | Sensitivity (%) | Specificity (%) | Accuracy (%) |
AI | 0.948 | 95.8 | 93.8 | 89.6 |
Elastic image | 0.865 | 79.2 | 93.8 | 73.0 |
Gray-scale | 0.719 | 75.0 | 68.8 | 43.8 |
Before assistance | S-Detect | After assistance |
Category 8 | B/B 7 | Category 3 8 |
M/B 1 | ||
Category4a 13 | B/B 6 | Category 3 3 |
M/B 4 | Category 4a 4 | |
M/M 3 | Category 4b 4 | |
Category 4c 2 | ||
Category 4b 11 | B/B 2 | Category 4a 2 |
M/B 4 | Category 4b 6 | |
M/M 5 | Category 4c 3 | |
Category 4c 6 | M/B 2 | Category 4c 6 |
M/M 4 | ||
Category 5 2 | M/M 2 | Category 5 2 |
Group | Area under ROC curve | Sensitivity (%) | Specificity (%) | Accuracy (%) |
Senior | 0.802 | 79.2 | 81.3 | 60.5 |
Junior | 0.719 | 75.0 | 68.8 | 43.8 |
Senior+AI | 0.969 | 100.0 | 93.8 | 93.8 |
Junior+AI | 0.948 | 95.8 | 93.8 | 89.6 |