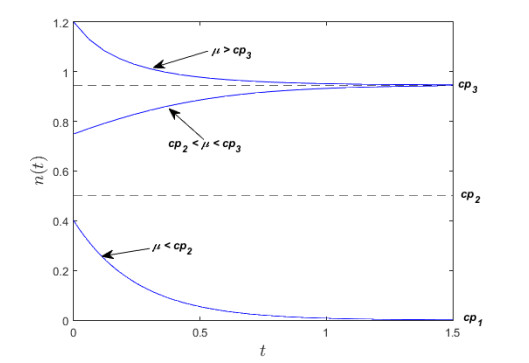
A nonautonomous logistic population model with a feature of an Allee threshold has been investigated in a periodically fluctuating environment. A slow periodicity of the harvesting effort was considered and may arise in response to relatively slow fluctuations of the environment. This assumption permits obtaining the analytical approximate solutions of such model using the perturbation approach based on the slow variation. Thus, the analytical expressions of the population evolution in the situation of subcritical and the supercritical harvesting were obtained and discussed in the framework of the Allee effect. Since the exact solution was not available due to the nonlinearity of the system, the numerical computation was considered to validate our analytical approximation. The comparison between the two methods showed a remarkable agreement as the time progressed, while such agreement fell off when the time was close to the initial density. Moreover, in the absence of the periodicity of the harvesting term, the expressions of the population evolution reduced to the exact solutions but in implicit forms. The finding results were appropriate for a wide range of parameter values, which lead to avoiding extensive recalculations while displaying the population behavior.
Citation: Fahad M. Alharbi. Harvesting a population model with Allee effect in a periodically varying environment[J]. AIMS Mathematics, 2024, 9(4): 8834-8847. doi: 10.3934/math.2024430
[1] | Shanshan Yu, Jiang Liu, Xiaojie Lin . Multiple positive periodic solutions of a Gause-type predator-prey model with Allee effect and functional responses. AIMS Mathematics, 2020, 5(6): 6135-6148. doi: 10.3934/math.2020394 |
[2] | Na Min, Hongyang Zhang, Xiaobin Gao, Pengyu Zeng . Impacts of hunting cooperation and prey harvesting in a Leslie-Gower prey-predator system with strong Allee effect. AIMS Mathematics, 2024, 9(12): 34618-34646. doi: 10.3934/math.20241649 |
[3] | Chengchong Lu, Xinxin Liu, Zhicheng Li . The dynamics and harvesting strategies of a predator-prey system with Allee effect on prey. AIMS Mathematics, 2023, 8(12): 28897-28925. doi: 10.3934/math.20231481 |
[4] | Yaw Chang, Wei Feng, Michael Freeze, Xin Lu, Charles Smith . Elimination, permanence, and exclusion in a competition model under Allee effects. AIMS Mathematics, 2023, 8(4): 7787-7805. doi: 10.3934/math.2023391 |
[5] | Marcello Delitala, Mario Ferraro . Is the Allee effect relevant in cancer evolution and therapy?. AIMS Mathematics, 2020, 5(6): 7649-7660. doi: 10.3934/math.2020489 |
[6] | Fatao Wang, Ruizhi Yang, Yining Xie, Jing Zhao . Hopf bifurcation in a delayed reaction diffusion predator-prey model with weak Allee effect on prey and fear effect on predator. AIMS Mathematics, 2023, 8(8): 17719-17743. doi: 10.3934/math.2023905 |
[7] | Lijuan Chen, Tingting Liu, Fengde Chen . Stability and bifurcation in a two-patch model with additive Allee effect. AIMS Mathematics, 2022, 7(1): 536-551. doi: 10.3934/math.2022034 |
[8] | Jie Liu, Qinglong Wang, Xuyang Cao, Ting Yu . Bifurcation and optimal harvesting analysis of a discrete-time predator–prey model with fear and prey refuge effects. AIMS Mathematics, 2024, 9(10): 26283-26306. doi: 10.3934/math.20241281 |
[9] | Ali Al Khabyah, Rizwan Ahmed, Muhammad Saeed Akram, Shehraz Akhtar . Stability, bifurcation, and chaos control in a discrete predator-prey model with strong Allee effect. AIMS Mathematics, 2023, 8(4): 8060-8081. doi: 10.3934/math.2023408 |
[10] | Yudan Ma, Ming Zhao, Yunfei Du . Impact of the strong Allee effect in a predator-prey model. AIMS Mathematics, 2022, 7(9): 16296-16314. doi: 10.3934/math.2022890 |
A nonautonomous logistic population model with a feature of an Allee threshold has been investigated in a periodically fluctuating environment. A slow periodicity of the harvesting effort was considered and may arise in response to relatively slow fluctuations of the environment. This assumption permits obtaining the analytical approximate solutions of such model using the perturbation approach based on the slow variation. Thus, the analytical expressions of the population evolution in the situation of subcritical and the supercritical harvesting were obtained and discussed in the framework of the Allee effect. Since the exact solution was not available due to the nonlinearity of the system, the numerical computation was considered to validate our analytical approximation. The comparison between the two methods showed a remarkable agreement as the time progressed, while such agreement fell off when the time was close to the initial density. Moreover, in the absence of the periodicity of the harvesting term, the expressions of the population evolution reduced to the exact solutions but in implicit forms. The finding results were appropriate for a wide range of parameter values, which lead to avoiding extensive recalculations while displaying the population behavior.
The population growth behavior has been described through numerous models. For instance, the logistic model [1,2] displays the population evolution from the initial density to the carrying capacity limit, no matter how small the initial population is. However, some populations only evolve when the initial population is large enough, otherwise the population is extinct. Some reasons for this behavior have been described by Courchamp et al. [3] in several categories, such as genetic diseases, demographic stochasticity, etc. Such a phenomenon is called a strong Allee effect [4,5]; for further details, see [6,7,8] and the references therein. There are numerous examples in the real world that adapt the feature of the Allee effect, which have been addressed in [9,10,11,12]. Mathematically, the simplest model that displays this feature [13] is given by
dNdT=PN(t)(1−N(t)K)(N(t)S−1),N(0)=N0, | (1.1) |
where N(T) is the population density at time T≥0, whereas P,K,N0, and S are positive constants of per capita growth rate, carrying capacity, the initial population and Allee threshold, 0<S<K, respectively. Model (1.1) clearly has the property that when the initial population is greater than the Allee threshold, 0<S<N0, the solution travels to the carrying capacity, describing the population at the equilibrium. In contrast, when 0<N0<S, the solution decreases to zero, showing the extinct situation of the population. Since the solution of such model can only be provided in an implicit form, Idlango et al. [14] obtained an analytical approximate expression for the population evolution and showed a very good comparison with the numerical data.
Note that adding a constant effort harvest term that is proportional to the population density, HN(T), into (1.1) gives the logistic population model subjects to the harvesting and the strong Allee effect [15] as follows
dNdT=PN(T)(1−N(T)K)(N(T)S−1)−HN(T),N(0)=N0 | (1.2) |
describing the situation of subcritical and supercritical harvesting under the restriction of the Allee effect.
It must be mentioned that the qualitative properties of the population models are regularly influenced by the seasonal and even violent changes in the environment, such as climate change, food sources, hunting, etc [16]. Thus, it is seen that within the progress of the mathematical biology, the time-varying coefficients are often incorporated with the models. For example, the dynamical models in a view of a periodic environmental change have been investigated by Rosenblat [17] and Legović et al. [18]. More recently, Alharbi [19,20], Alsharidi et al. [21], and Idlango et al. [22,23] considered single species models subjects to variable coefficients. Accordingly, the model (1.2) can be written in terms of variable coefficients as follows
dNdT=P(T)N(T)(1−N(T)K(T))(N(T)S(T)−1)−H(T)N(T),N(0)=N0. | (1.3) |
Clearly, when the time-varying parameters are adopted, as in (1.3), the population models turn to nonautonomous systems and the exact solution is far of achievement, so the numerical approximate method must be resorted to; see, for example, the analysis of those in [17,18] among others [24,25]. However, the numerical method often responds to particular parameter values, which leads to providing a limited information about the population evolution, as in [25]. As a result, it is essential to consider the analytical approximate method in order to obtain a general representation that is capable to describe the influence of the time variation in the model coefficients and its impacts on the population evolution for various general scenarios.
In many cases, it is possible to consider slow fluctuations in the environmental factors, such as climate changes, adequate food, etc. This may lead to proposing a slowly varying population model. Ludwig et al. [26] used such observation in the analysis of the spruce budworm, but leaving their calculation unsolved analytically. Thus, Shepherd et al. [27], Grozdanovski et al. [28], and Alharbi [19,20] considered a multi-scaled perturbation method to investigate population models in a slowly varying environment for various cases. Accordingly, the analytical approximate expressions have been obtained and validated numerically. The comparison between the two methods shows a very good agreement. However, in their analysis, the associated coefficients functions must vary in a slow time scale compared to the intrinsic time scale of the overall population, otherwise the considered hypothesis is not valid in obtaining the analytical expression of the population evolution. In addition, utilizing the multi-scaled perturbation method often provides asymmetric rate of convergence between the leading and higher order differential equations, which must be aligned by eliminating the disturbed terms, arising in the higher order equations.
Therefore, in this paper, we aim to investigate the single species population model (1.3) that exhibits the Allee effect and a slowly periodic fluctuation of the harvesting rate. The assumption of the harvesting periodicity may arise in response to relatively slow fluctuations of the environment. This allows us to utilize the regular perturbation approach based on the small variation to obtain the analytical approximate solutions for the population evolution in the situation of subcritical and the supercritical harvesting with respect to the Allee threshold condition. Such analytical expressions will successfully be able to show the harvesting variation on the same time scale of the overall population. Moreover, the rate of convergence for the leading and higher order population expressions is symmetric without any elimination of the disturbed terms. In addition, due to dealing with the nonlinear system, a numerical computation will be obtained using a fourth-order Runge-Kutta technique in order to validate the analytical approximation. The comparison between the two methods is expected to show that when the time progresses, the analytical expressions will display a very good convergence with the numerical data, while such accuracy falls off when the time approaches the initial density. The finding results are appropriate for a wide range of parameter values, which provides an advantage of avoiding extensive recalculations while displaying the population evolution.
Since we are only interested in the influence of the periodic (or seasonal) fluctuation of the harvesting, which might be caused by a disease, a fishing, or a hunting, on the population behavior, the coefficients P(T),K(T), and S(T) in (1.3) are assumed fixed. So, we arrive at
dNdT=PN(T)(1−N(T)K)(N(T)S−1)−H(T)N(T),N(0)=N0. | (2.1) |
In what follows, we render the system (2.1) dimensionless. By expressing the nondimensional time, population, and harvesting effort scale by t=PT, N(T)=Kn(t), and H(T)=H0η(t), respectively, (2.1) can be written in a dimensionless form as
dndt=n(1−n)(σn−1)−αη(t)n,n(0)=μ, | (2.2) |
where η(t) fluctuates periodically and is given by
η(t)=1+ϵγ(t), | (2.3) |
and σ, α, and μ are given by
σ=KS,α=H0P,μ=N0K, | (2.4) |
respectively, ϵ is a dimensionless, small, and positive parameter, and H0 is the baseline of the harvesting function.
As the model (2.2) stands, two situations that depend on the harvesting have been identified. One is the subcritical harvesting, where the population evolves to equilibrium, or is extinguished due to the Allee effect. The other is supercritical harvesting, where the population is extinct for any initial density μ.
It is important to note that, for the general variation, assuming at least one of the model coefficients varies with time, the system (2.2) can only be solved using numerical techniques. However, applying (2.3) in (2.2) and assuming ϵ is sufficiently small, this permits obtaining the analytical approximate expressions for the population evolution model using the perturbation approach. Accordingly, we propose the perturbation expansion based on ϵ as follows
n(t)∼n0(t)+ϵn1(t)+O(ϵ2), | (3.1) |
and substituting (3.1) into (2.2) obtains, on equating like powers of ϵ, a leading order term
dn0dt=n0(δ−σ(n0−ψ)2), | (3.2) |
a higher order term
dn1dt+((3n0σ−2(σ+1))n0+1+α))n1=−αγ(t)n0, | (3.3) |
and the initial conditions
n0(0)=μ,and,n1(0)=0, | (3.4) |
where ψ and δ are defined by
ψ=σ+12σ,andδ=σψ2−(1+α), | (3.5) |
respectively. Therefore, the leading order term (3.2) is an autonomous differential equation, whereas the higher order term (3.3) describes a slowly varying population. Since α measures the ratio of the baseline of the harvesting effort, H0, to the growth rate, we conclude from the second of (3.5) and (3.2) that when α<σψ2−1, δ>0, so the harvesting is subcritical. In contrast, when α>σψ2−1, δ<0, so the harvesting becomes supercritical. Consequently, we consider these two cases.
In the following, we consider δ>0, so the harvesting is subcritical. Note that sometimes the initial density, μ, is driven below the critical threshold, so it will, in this section, be expected that the population either survives to a positive limiting state or is extinguished, depending on the μ value.
With the application of the initial condition, given in the first of (3.4), the differential equation (3.2) may be solved implicitly in the form
F(n0)−e−ϕt=0, | (4.1) |
where
F(u)=(uμ)β√σδ(σ(u−ψ)2−δσ(μ−ψ)2−δ)(σ(μ−ψ)+√σδσ(u−ψ)+√σδ)βψ,ϕ=2√σδσ(σψ+√σδ),β=2(σψ+√σδ)1+α. |
Straightforward, applying the stability analysis to the leading order term (3.2) displays the behavior of the solution (4.1), which is governed by the initial density, μ. In order to discuss that, we first introduce the critical points as follows
(1) cp1=0, which is stable.
(2) cp2=σψ−√σδσ, which is unstable and acts as breakpoints [29].
(3) cp3=σψ+√σδσ, which is stable.
Thus, when the initial population obeys cp2<μ<cp3, n0(t) increases to the surviving limiting state, cp3, as t→∞, whereas it decreases to the limit when the μ value is beyond cp3. In contrast, when the μ value lies below cp2, the population tends to extinguish, i.e., n(t)→0 as t→∞; see Figure 1.
Note that n0(t) appears implicitly in (4.1), so our approach is apparently not able to obtain the expression of the higher order term, n1(t). However, it can be observed that as t→∞, the term e−ϕt→0. Such an observation shows a possibility of obtaining the asymptotic expression of n0(t) by defining the solution of (4.1) as a power series in terms of a small e−ϕt. Once this achieved, the linear differential Eq (3.3) can, in principle, be solved utilizing an integrating factor together with the application of the initial condition, given in the second of (3.4). Accordingly, we express n0(t) as a Poincarˊe expansion in e−ϕt
n0s(t)∼f0+f1e−ϕt+O(e−2ϕt), | (4.2) |
where the subscript s denotes association with the survival case. Substituting (4.2) in (4.1), expanding in power of e−ϕt, and equating like powers of e−ϕt yields the coefficients f0,f1,⋯. Thus, with the choice f0=σψ+√σδσ, the expansion for n0s(t) when n0s(t)→cp3 as t→∞ becomes
n0s(t)=σψ+√σδσ+χe−ϕt+⋯, | (4.3) |
where χ is given by
χ=σ(μ−ψ)2−δ2√σδ(σψ+√σδσμ)2√σδσψ−√σδ(σ(μ−ψ)+√σδ2√σδ)2σψ√σψ−σψ, | (4.4) |
determining the leading order term when μ>cp2.
Similar to the above but more precise calculation, when μ<cp2, n0(t)→0 as t→∞. Thus, the expansion of n0(t) is given by
n0d(t)=ωe−(1+α)t−2ω2σψ1+αe−2(1+α)t+⋯, | (4.5) |
where ω is given by
ω=μ(1+ασ(μ−ψ)2−δ)√σδ−σψ2√σδ(σ(μ−ψ)+√σδ√σδ−σψ)−σψ√σδ | (4.6) |
and the subscript d denotes association with the extinction case. Note that in the expansion (4.3), the convergence rate to cp3 (survival) depends on ϕ, while in the expansion (4.5), the convergence rate to cp1 (extinction) depends on (1+α).
Therefore, the solution of the leading order term n0(t) has split into two parts: n0s(t), given in (4.3), is to describe the surviving population, and n0d(t), given in (4.5), is to represent the extinct population due to driven μ below the critical threshold. Such division will be maintained while solving the higher order terms.
Substituting the leading terms (4.3) into (3.3) yields
dn1sdt+(ϕ+⋯)n1s=−αγ(t)(σψ+√σδσ+χe−ϕt+⋯). | (4.7) |
Considering the leading terms indicated in (4.7) and solving, we obtain, on applying the initial condition, given in the second of (3.4),
n1s(t)=−αe−ϕt∫t0γ(s)(σψ+√σδσeϕs+χ+⋯)ds, | (4.8) |
determining the higher order expression of the surviving population in terms of the periodic function γ(t).
In a like manner, substituting the leading terms (4.5) into (3.3), we arrive at
dn1ddt+(1+α+⋯)n1d=−αγ(t)(ωe−(1+α)t−2ω2σψ1+αe−2(1+α)t+⋯). | (4.9) |
As earlier, preserving the leading terms mentioned in (4.9) and solving, we obtain, on applying the initial condition, given in the second of (3.4),
n1d(t)=−αe−(1+α)∫t0γ(s)(ω−2ω2σψ1+αe−(1+α)s+⋯)ds, | (4.10) |
determining the higher order expression of the extinct population.
To recapitulate, considering the subdivisions of the leading and higher order terms, the perturbation expansion (3.1) for the surviving population at the positive limiting state becomes
ns(t)=n0s(t)+ϵn1s(t)+⋯, | (4.11) |
whereas the expansion for the extinct population due to the Allee effect becomes
nd(t)=n0d(t)+ϵn1d(t)+⋯, | (4.12) |
where n0s(t),n1s(t),n0d(t) and n1d(t) are given by (4.3), (4.5), (4.8), and (4.10), respectively.
Considering α>σψ2−1, δ is always negative, the harvesting is supercritical. Accordingly, we redefine δ, given in the second of (3.5), in such a way that δ=−δe, where δe is given by
δe=1+α−σψ2, | (5.1) |
and the subscript e denotes association with supercritical harvesting.
Thus, considering (5.1) together with the first of (3.4), the solution of (3.2) yields
G(n0e)−e−(1+α)t=0, | (5.2) |
where
G(u)=uμ(σ(μ−ψ)2+δeσ(u−ψ)2+δe)12eσψ√σδe(arctan(σ(u−ψ)√σδe)−arctan(σ(μ−ψ)√σδe)). | (5.3) |
Again, n0e(t) only appears implicitly. So, applying analogous reasonings of that in Section 4 but when n(t)→0 as t→∞, we define the asymptotic expansion of n0e(t) as a power series in terms of a small e−(1+α)t as follows
n0e=τe−(1+α)−2τ2σψ1+αe−2(1+α)+⋯, | (5.4) |
where τ is given by
τ=μ√σ(μ−ψ)2+δe1+αeσψ√σδe(arctan(σψ√σδe)+arctan(σ(μ−ψ)√σδe)), | (5.5) |
determining the leading order term of the supercritical harvesting.
In a like manner, substituting (5.4) into (3.3), we arrive at
dn1edt+(1+α+⋯)n1e=−αγ(t)(τe−(1+α)t−2τ2σψ1+αe−2(1+α)t+⋯). | (5.6) |
Retaining the leading terms mentioned in (5.6) and solving, we obtain, on considering the initial condition given in the second of (3.4),
n1e=−αe−(1+α)∫t0γ(t)(τ−2τ2σψ1+αe−(1+α)s+⋯)ds, | (5.7) |
determining the higher order term of the supercritical harvesting.
Therefore, the perturbation expansion (3.1) regarding the supercritical harvesting becomes
ne(t)=n0e(t)+ϵn1e(t)+⋯, | (5.8) |
where n0e(t) and n1e(t) are given by (5.4) and (5.7), respectively.
The analytical approximate expressions for the logistic population model that exhibits the Allee effect together with the slowly periodic harvesting term are readily obtained and the expansions (4.11), (4.12), and (5.8) represent the solutions. These expansions have the advantage of not requiring specific parameters values to be provided, which leads to avoiding the extensive recalculations while displaying the population behavior. It is important to note that our expansions are limited in the first two terms due to displaying a very good convergence with the numerical solution and avoiding the swell in the expression. In addition, since the asymptotic expansions of the leading order terms, given in (4.3), (4.5) and (5.4), are constructed in terms of small exponential terms, we expect that when t is close to the initial density, the perturbation expansions (4.11), (4.12), and (5.8) diverge from the numerical solutions, whereas these expansions provide remarkable agreement as t→∞.
For α<σψ2−1, the harvesting is subcritical and the population survives at the equilibrium state when μ>cp2 and the expansion (4.11) displays the evolution. On the other hand, when the initial density is below the Allee threshold (i.e., μ<cp2), the population is extinguished and the expansion (4.12) exhibits the population behavior.
When α is increased beyond the limit σψ2−1, the harvesting is turned to supercritical, which leads to declining the population to zero in a finite time, as shown in the expansion (5.8).
Note that ϵ=0 corresponds to the exact solution (no slowly varying term), and the leading order terms (4.1) and (5.2) describe the population evolution in the situations of subcritical and supercritical harvesting, respectively, including the Allee threshold condition. However, when ϵ>0, (4.11), (4.12), and (5.8) describe the slowly varying population behavior.
As mentioned earlier, the implicit representation of the leading order terms of the subcritical and supercritical harvesting make the explicit expressions of the higher order terms unattainable. However, for a particular case, setting the periodic function γ(t)=sint, the solution of (4.8), (4.10), and (5.7) yields
n1s(t)=−αe−ϕt(σψ+√σδσ(ϕ2+1)e−ϕt(ϕsin(t)−cos(t)+e−ϕt)+χ(1−cos(t))+⋯), | (6.1) |
n1d(t)=−αe−(1+α)t(ω(1−cos(t))+2ω2σψe−(1+α)t(1+α)3+(1+α)(cos(t)+(1+α)sin(t)−e(1+α)t)), | (6.2) |
n1e=−αe−(1+α)t(τ(1−cos(t))+2τ2σψe−(1+α)t(1+α)(1+(1+α)2)(cos(t)+(1+α)sin(t)−e(1+α)t)), | (6.3) |
determining slowly varying expressions for subcritical harvesting (survival and extinct case due to the Allee threshold) and supercritical harvesting, respectively
From (6.1)–(6.3), note that for a large class of time, we arrive at
n1s→α(σψ+√σδ)σ(cos(t)−ϕsin(t)),n1d→0,n1e→0 |
and, hence, the perturbation expansions (4.11), (4.12), and (5.8) become as t→∞
ns→σψ+√σδσ+ϵα(σψ+√σδ)σ(cos(t)−ϕsin(t))+⋯, | (6.4) |
nd→0,ne→0, | (6.5) |
describing the population behavior at the limiting states.
It must be pointed out that the leading and higher order terms of our perturbation expansions have a symmetric convergence rate, whereas in the analysis of Alharbi [19,20] and Idlango et al. [22,23], they needed to be aligned by eliminating the disturbed terms, arising in the higher order terms.
Figures 3 and 4 were generated using the expansions (4.11), (4.12), and (5.8), respectively, together with the numerical data obtained using a fourth-order Runge-Kutta technique. It is clearly seen that the convergence between the two solutions is very good for t→∞, but such convergence falls off at t close to zero as expected from the asymptotic expressions above. In addition, Table 1 is obtained by calculating the absolute difference between the approximate expressions and the numerical data as t→∞. This shows a remarkable discrepancy between the analytical approximate and numerical solution when t is small, but when t progresses, the two solutions match each other. Graphically, Figure 2(a), (b) confirms the result of Table 1 by showing a monotonically decreasing discrepancy curve for subcritical and supercritical harvesting as the time increases.
∣Analytical−numerical∣ | |||
Time | Subcritical | Subcritical with extinction | Supercritical |
t=0 | 0.8377 | 0.0683 | 0.4812 |
t=1 | 7×10−3 | 2×10−3 | 1.7×10−5 |
t=2 | 6.74×10−4 | 1.81×10−4 | 2.14×10−7 |
t=3 | 1.36×10−4 | 9.6×10−5 | 3.72×10−10 |
Figure 3(a), (b) regards the population evolution in the case of subcritical harvesting. They show that when μ>cp2, the population survives to the equilibrium state, cp3, from below when cp2<μ<cp3 (Figure 3(a)) and from above when μ>cp3 (Figure 3(b)).
In contrast, Figure 4(a) displays that when μ locates below the critical Allee threshold, μ<cp2, the population is instantly extinct in finite time. Figure 4(b) relates to supercritical harvesting. It can be seen that for any initial population, μ, the population dies out as t→∞.
Figure 5(a), (b), which was generated using the expression of the equilibrium given in (6.4), shows the influence of the associated parameters σ and α on the population behavior. Figure 5(a) shows that fixing the value of α while increasing σ leads to increasing the variation of the population at the equilibrium. In contrast, in Figure 5(b), when fixing the value of σ, the population density reduces as α increases.
In this paper, we investigate the single species population model that exhibits the Allee effect and the slowly varying periodic harvesting. The slow periodicity of the harvesting term may arise in response to relatively slow fluctuations of the environment. This assumption allows us to utilize the perturbation approach based on the small variation to obtain the analytical approximate solutions for the population evolution in the situation of subcritical and the supercritical harvesting with respect to the Allee threshold condition. In addition, due to the absence of the exact solution of such a system, we obtain the numerical computation using a fourth-order Runge-Kutta technique to validate the analytical results. The comparison between the two methods shows a remarkable agreement as t→∞, while such an agreement falls off when the time is close to the initial density. In addition, we show that setting ϵ=0 extinguishes the slowly varying term and the exact solution will be available in an implicit form. The finding results are appropriate for a wide range of parameter values, which leads to avoiding the extensive recalculations while displaying the population behavior.
The author has not used Artificial Intelligence (AI) tools in the creation of this article.
The author declares that he has no known competing financial interests or personal relationships that could have appeared to influence the work reported in this paper.
[1] | R. B. Banks, Growth and diffusion phenomena: Mathematical frameworks and applications, Springer Science & Business Media, 14 (1993). |
[2] | M. Braun, M. Golubitsky, Differential equations and their applications, Springer, 1 (1983). https://doi.org/10.1007/978-1-4684-0164-6-1 |
[3] |
F. Courchamp, T. C. Brock, B. Grenfell, Inverse density dependence and the Allee effect, Trends Ecol. Evol., 14 (1999), 405–410. https://doi.org/10.1016/S0169-5347(99)01683-3 doi: 10.1016/S0169-5347(99)01683-3
![]() |
[4] |
P. A. Stephens, W. J. Sutherland, R. P. Freckleton, What is the Allee effect? Oikos, 1999,185–190. https://doi.org/10.2307/3547011 doi: 10.2307/3547011
![]() |
[5] | M. J. Panik, Stochastic differential equations: An introduction with applications in population dynamics modeling, John Wiley & Sons, 2017. https://doi.org/10.1002/9781119377399 |
[6] |
M. Krstić, M. Jovanović, On stochastic population model with the Allee effect, Math. Comput. Model., 52 (2010), 370–379. https://doi.org/10.1016/j.mcm.2010.02.051 doi: 10.1016/j.mcm.2010.02.051
![]() |
[7] |
Q. Yang, D. Jiang, A note on asymptotic behaviors of stochastic population model with Allee effect, Appl. Math. Model., 35 (2011), 4611–4619. https://doi.org/10.1016/j.apm.2011.03.034 doi: 10.1016/j.apm.2011.03.034
![]() |
[8] |
J. R. Graef, S. Padhi, S. Pati, Periodic solutions of some models with strong Allee effects, Nonlinear Anal.-Real, 13 (2012), 569–581. https://doi.org/10.1016/j.nonrwa.2011.07.044 doi: 10.1016/j.nonrwa.2011.07.044
![]() |
[9] |
B. F. Brockett, M. Hassall, The existence of an Allee effect in populations of porcellio scaber (isopoda: Oniscidea), Eur. J. Soil Biol., 41 (2005), 123–127. https://doi.org/10.1016/j.ejsobi.2005.09.004 doi: 10.1016/j.ejsobi.2005.09.004
![]() |
[10] |
F. Courchamp, T. C. Brock, B. Grenfell, Inverse density dependence and the Allee effect, Trends Ecol. Evol., 14 (1999), 405–410. https://doi.org/10.1016/S0169-5347(99)01683-3 doi: 10.1016/S0169-5347(99)01683-3
![]() |
[11] |
F. M. Hilker, M. Langlais, S. V. Petrovskii, H. Malchow, A diffusive si model with Allee effect and application to FIV, Math. Biol., 206 (2007), 61–80. https://doi.org/10.1016/j.mbs.2005.10.003 doi: 10.1016/j.mbs.2005.10.003
![]() |
[12] |
A. Hurford, M. Hebblewhite, M. A. Lewis, A spatially explicit model for an Allee effect: Why wolves recolonize so slowly in greater yellowstone, Theor. Popul. Biol., 70 (2006), 244–254. https://doi.org/10.1016/j.tpb.2006.06.009 doi: 10.1016/j.tpb.2006.06.009
![]() |
[13] |
P. Amarasekare, Allee effects in metapopulation dynamics, Am. Nat., 152 (1998), 298–302. https://doi.org/10.1086/286169 doi: 10.1086/286169
![]() |
[14] |
M. A. Idlango, J. J. Shepherd, J. A. Gear, Multiscaling analysis of a slowly varying single species population model displaying an Allee effect, Math. Method. Appl. Sci., 37 (2014), 1561–1569. https://doi.org/10.1002/mma.2911 doi: 10.1002/mma.2911
![]() |
[15] |
A. Tesfay, D. Tesfay, J. Brannan, J. Duan, A logistic-harvest model with Allee effect under multiplicative noise, Stoch. Dynam., 21 (2021), 2150044. https://doi.org/10.1142/S0219493721500441 doi: 10.1142/S0219493721500441
![]() |
[16] |
F. B. Rizaner, S. P. Rogovchenko, Dynamics of a single species under periodic habitat fluctuations and Allee effect, Nonlinear Anal.-Real, 13 (2012), 141–157. https://doi.org/10.1016/j.nonrwa.2011.07.021 doi: 10.1016/j.nonrwa.2011.07.021
![]() |
[17] |
S. Rosenblat, Population models in a periodically fluctuating environment, J. Math. Biol., 9 (1980) 23–36. https://doi.org/10.1007/BF00276033 doi: 10.1007/BF00276033
![]() |
[18] |
T. Legović, G. Perić, Harvesting population in a periodic environment, Ecol. Model., 24 (1984), 221–229. https://doi.org/10.1016/0304-3800(84)90042-5 doi: 10.1016/0304-3800(84)90042-5
![]() |
[19] |
F. M. Alharbi, A slow single-species model with non-symmetric variation of the coefficients, Fractal Fract., 6 (2022), 72. https://doi.org/10.3390/fractalfract6020072 doi: 10.3390/fractalfract6020072
![]() |
[20] |
F. M. Alharbi, The general analytic expression of a harvested logistic model with slowly varying coefficients, Axioms, 11 (2022), 585. https://doi.org/10.3390/axioms11110585 doi: 10.3390/axioms11110585
![]() |
[21] |
A. K. Alsharidi, A. A. Khan, J. J. Shepherd, A. J. Stacey, Multiscaling analysis of a slowly varying anaerobic digestion model, Math. Method. Appl. Sci., 43 (2020), 5729–5743. https://doi.org/10.1002/mma.6315 doi: 10.1002/mma.6315
![]() |
[22] |
M. A. Idlango, J. J. Shepherd, J. A. Gear, Multiscaling analysis of a slowly varying single species population model displaying an Allee effect, Math. Method. Appl. Sci., 37 (2014), 1561–1569. https://doi.org/10.1002/mma.2911 doi: 10.1002/mma.2911
![]() |
[23] |
M. A. Idlango, J. J. Shepherd, J. A. Gear, Logistic growth with a slowly varying holling type Ⅱ harvesting term, Commun. Nonlinear Sci., 49 (2017), 81–92. https://doi.org/10.1016/j.cnsns.2017.02.005 doi: 10.1016/j.cnsns.2017.02.005
![]() |
[24] |
T. Cromer, Harvesting in a seasonal environment, Math. Comput. Model., 10 (1988), 445–450. https://doi.org/10.1016/0895-7177(88)90034-9 doi: 10.1016/0895-7177(88)90034-9
![]() |
[25] |
P. S. Meyer, J. H. Ausubel, Carrying capacity: A model with logistically varying limits, Technol. Forecast. Soc., 61 (1999), 209–214. https://doi.org/10.1016/S0040-1625(99)00022-0 doi: 10.1016/S0040-1625(99)00022-0
![]() |
[26] |
D. Ludwig, D. D. Jones, C. S. Holling, Qualitative analysis of insect outbreak systems: The spruce budworm and forest, J. Anim. Ecol., 47 (1978), 315–332. https://doi.org/10.2307/3939 doi: 10.2307/3939
![]() |
[27] |
J. J. Shepherd, L. Stojkov, The logistic population model with slowly varying carrying capacity, Anziam J., 47 (2005), C492–C506. https://doi.org/10.21914/anziamj.v47i0.1058 doi: 10.21914/anziamj.v47i0.1058
![]() |
[28] |
T. Grozdanovski, J. J. Shepherd, A. Stacey, Multi-scaling analysis of a logistic model with slowly varying coefficients, Appl. Math. Lett., 22 (2009), 1091–1095. https://doi.org/10.1016/j.aml.2008.10.002 doi: 10.1016/j.aml.2008.10.002
![]() |
[29] |
R. M. May, Thresholds and breakpoints in ecosystems with a multiplicity of stable states, Nature, 269 (1977), 471–477. https://doi.org/10.1038/269471a0 doi: 10.1038/269471a0
![]() |
1. | Dongpo Hu, Rongxue Du, Biao Liu, Ningning Zhu, Ming Liu, Single-time and multi-time scales dynamic analysis in a predator–prey system with Michaelis-Menten type predator harvesting, 2024, 0924-090X, 10.1007/s11071-024-10770-0 |
∣Analytical−numerical∣ | |||
Time | Subcritical | Subcritical with extinction | Supercritical |
t=0 | 0.8377 | 0.0683 | 0.4812 |
t=1 | 7×10−3 | 2×10−3 | 1.7×10−5 |
t=2 | 6.74×10−4 | 1.81×10−4 | 2.14×10−7 |
t=3 | 1.36×10−4 | 9.6×10−5 | 3.72×10−10 |