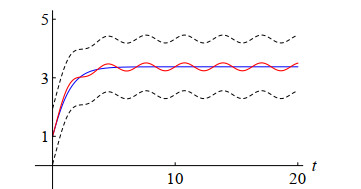
In many studies dealing with mathematical models, the subject is examining the fitting between actual data and the solution of the mathematical model by applying statistical processing. However, if there is a solution that fluctuates greatly due to a small perturbation, it is expected that there will be a large difference between the actual phenomenon and the solution of the mathematical model, even in a short time span. In this study, we address this concern by considering Ulam stability, which is a concept that guarantees that a solution to an unperturbed equation exists near the solution to an equation with bounded perturbations. Although it is known that Ulam stability is guaranteed for the standard von Bertalanffy growth model, it remains unsolved for a model containing the Allee effect. This paper investigates the Ulam stability of a von Bertalanffy growth model with the Allee effect. In a sense, we obtain results that correspond to conditions of the Allee effect being very small or very large. In particular, a more preferable Ulam constant than the existing result for the standard von Bertalanffy growth model, is obtained as the Allee effect approaches zero. In other words, this paper even improves the proof of the result in the absence of the Allee effect. By guaranteeing the Ulam stability of the von Bertalanffy growth model with Allee effect, the stability of the model itself is guaranteed, and, even if a small perturbation is added, it becomes clear that even a small perturbation does not have a large effect on the solutions. Several examples and numerical simulations are presented to illustrate the obtained results.
Citation: Masumi Kondo, Masakazu Onitsuka. Ulam type stability for von Bertalanffy growth model with Allee effect[J]. Mathematical Biosciences and Engineering, 2024, 21(3): 4698-4723. doi: 10.3934/mbe.2024206
[1] | Masakazu Onitsuka . Conditional Ulam stability and its application to von Bertalanffy growth model. Mathematical Biosciences and Engineering, 2022, 19(3): 2819-2834. doi: 10.3934/mbe.2022129 |
[2] | Yongli Cai, Malay Banerjee, Yun Kang, Weiming Wang . Spatiotemporal complexity in a predator--prey model with weak Allee effects. Mathematical Biosciences and Engineering, 2014, 11(6): 1247-1274. doi: 10.3934/mbe.2014.11.1247 |
[3] | Yun Kang, Sourav Kumar Sasmal, Amiya Ranjan Bhowmick, Joydev Chattopadhyay . Dynamics of a predator-prey system with prey subject to Allee effects and disease. Mathematical Biosciences and Engineering, 2014, 11(4): 877-918. doi: 10.3934/mbe.2014.11.877 |
[4] | Minjuan Gao, Lijuan Chen, Fengde Chen . Dynamical analysis of a discrete two-patch model with the Allee effect and nonlinear dispersal. Mathematical Biosciences and Engineering, 2024, 21(4): 5499-5520. doi: 10.3934/mbe.2024242 |
[5] | Juan Ye, Yi Wang, Zhan Jin, Chuanjun Dai, Min Zhao . Dynamics of a predator-prey model with strong Allee effect and nonconstant mortality rate. Mathematical Biosciences and Engineering, 2022, 19(4): 3402-3426. doi: 10.3934/mbe.2022157 |
[6] | Yuhong Huo, Gourav Mandal, Lakshmi Narayan Guin, Santabrata Chakravarty, Renji Han . Allee effect-driven complexity in a spatiotemporal predator-prey system with fear factor. Mathematical Biosciences and Engineering, 2023, 20(10): 18820-18860. doi: 10.3934/mbe.2023834 |
[7] | Sophia R.-J. Jang . Discrete host-parasitoid models with Allee effects and age structure in the host. Mathematical Biosciences and Engineering, 2010, 7(1): 67-81. doi: 10.3934/mbe.2010.7.67 |
[8] | Fang Liu, Yanfei Du . Spatiotemporal dynamics of a diffusive predator-prey model with delay and Allee effect in predator. Mathematical Biosciences and Engineering, 2023, 20(11): 19372-19400. doi: 10.3934/mbe.2023857 |
[9] | Eduardo Liz, Alfonso Ruiz-Herrera . Delayed population models with Allee effects and exploitation. Mathematical Biosciences and Engineering, 2015, 12(1): 83-97. doi: 10.3934/mbe.2015.12.83 |
[10] | Moitri Sen, Malay Banerjee, Yasuhiro Takeuchi . Influence of Allee effect in prey populations on the dynamics of two-prey-one-predator model. Mathematical Biosciences and Engineering, 2018, 15(4): 883-904. doi: 10.3934/mbe.2018040 |
In many studies dealing with mathematical models, the subject is examining the fitting between actual data and the solution of the mathematical model by applying statistical processing. However, if there is a solution that fluctuates greatly due to a small perturbation, it is expected that there will be a large difference between the actual phenomenon and the solution of the mathematical model, even in a short time span. In this study, we address this concern by considering Ulam stability, which is a concept that guarantees that a solution to an unperturbed equation exists near the solution to an equation with bounded perturbations. Although it is known that Ulam stability is guaranteed for the standard von Bertalanffy growth model, it remains unsolved for a model containing the Allee effect. This paper investigates the Ulam stability of a von Bertalanffy growth model with the Allee effect. In a sense, we obtain results that correspond to conditions of the Allee effect being very small or very large. In particular, a more preferable Ulam constant than the existing result for the standard von Bertalanffy growth model, is obtained as the Allee effect approaches zero. In other words, this paper even improves the proof of the result in the absence of the Allee effect. By guaranteeing the Ulam stability of the von Bertalanffy growth model with Allee effect, the stability of the model itself is guaranteed, and, even if a small perturbation is added, it becomes clear that even a small perturbation does not have a large effect on the solutions. Several examples and numerical simulations are presented to illustrate the obtained results.
In this paper, we consider the differential equation
x′=(ax23−bx)(c−dx13) | (1.1) |
for t≥0, where a, b, c, and d are positive constants. If c=1 and d=0, then this model is reduced to the well-known von Bertalanffy growth model:
x′=ax23−bx. | (1.2) |
This model was proposed by von Bertalanffy [1] as a mathematical model of fish growth. Here, x denotes body weight and a and b are parameters representing anabolism and catabolism, respectively. Von Bertalanffy suggested that an exponent of 23 is appropriate. Because the first term on the right side of Eq (1.2) refers to the increase in body weight due to nutrients, it is proportional to the surface area; the second term refers to weight loss due to respiration and waste products, and it is proportional to body weight. This model has been generalized in recent years, and research has been conducted in various fields, including mathematical biology. For example, Calatayud et al. [2] analyzed the randomized nonautonomous von Bertalanffy growth model and obtained a stochastic solution; Edwards and Anderssen [3] derived infinitesimals that allows a certain form of the nonautonomous von Bertalanffy equation to be transformed into autonomous forms in which some new analytic solutions were found; Kim et al. [4] proposed a growth model that includes the effect of water temperature on growth in the von Bertalanffy growth model. Their model was applied to fit growth data for bullhead (Cottus gobio), brown trout (Salmo trutta L.), juvenile salmon (Salmo salar), and Araucanian herring (Strangomera bentincki); Román-Román et al. [5] proposed a stochastic model that is related to the generalized von Bertalanffy growth curve. Their model enables investigation of the time evolution of growth variables related to both individual behaviors and the mean population behavior. In addition, fitting to real data is also performed; Wiff et al. [6] investigated three models with different complexities, depending mostly on what assumptions are made about age structure, and applied them to three fish populations that are targets of commercial fisheries in the Southeast Pacific. As shown in these examples, there are many studies that model the standard von Bertalanffy growth model into nonautonomous systems or more complex nonlinear systems, and then fit the model results to real data. This study treats the model as a classical von Bertalanffy growth model with an Allee effect. We can cite [7] for the study of models with an Allee effect.
There are many cases in which Allee effects are considered as a direction for generalizing growth models. The size of a population varies depending on the population density (the number of individuals per unit living space), as well as the growth rate, mortality rate, and individual body weight. This is called the density effect, and the effect of increasing the per-individual reproduction rate when population density increases to a certain degree is called the Allee effect. On the right side of Eq (1.2), the first term is proportional to the surface area and the second term is proportional to body weight, so it may originally be written as aw2−bw3. If we add the Allee effect to this, we get (aw2−bw3)(c−dw). Therefore, if we set x=w3, we get Eq (1.1).
An important problem that always arises when dealing with mathematical models is the gap between the mathematical model and the actual phenomenon. The more we try to create a mathematical model that represents a solution to a real phenomenon, the more its complexity and parameter quantity increase. As a result, parameter determination becomes more difficult. On the other hand, oversimplification increases the possibility that the solution of the mathematical model will not be consistent the actual phenomenon. However, when dealing with phenomena, it seems that we often use somewhat simple mathematical models to analyze them. For example, for the cholera outbreak in Yemen, Nishiura et al. [8] used the Richards model, which is similar to the equation in this study, to analyze weekly data on the number of cases and deaths collected by the World Health Organization to predict the epidemic in real time. In this way, it is often possible to reduce the gap between the mathematical model and the actual phenomenon by statistically processing the actual data, determining the optimal parameters, and deriving a solution to the mathematical model that fits the data. By using this, we can learn about the future circumstances of real phenomena, but the solutions obtained via this statistical processing method do not necessarily fit the data for the real phenomena over a long time span. Therefore, we have to believe that the solution fits the real data up to some short period of time, after which we have to add new data, statistically reprocess it, and modify the parameters. In other words, it is necessary to repeat statistical processing many times in real time.
On the other hand, this study was designed to answer the following question: "Assuming that the difference between the real phenomenon and the mathematical model (von Bertalanffy growth model with Allee effect) is less than a constant ε>0, is there always a solution of the mathematical model that is near the solution of the real phenomenon?" The foundation of this problem can be said to be a kind of perturbation problem, but note that ε>0 is not subject to a low-value condition. The concept related to this has been posed by Ulam, and it is now referred to as Ulam stability. We emphasize here that, if we can estimate ε>0, which refers to the gap between the real phenomenon and the mathematical model, then the solution of the mathematical model will definitely be found near the real phenomenon on interval [0,∞). In other words, it is proven that a certain solution of a mathematical model with parameters obtained by only performing one round of statistical processing, and thus has no need for repeated statistical processing, exists close to the actual phenomenon over an infinite time span. Furthermore, guaranteeing Ulam stability means that the mathematical model is close to the actual phenomenon, and that the stability of the mathematical model itself is guaranteed. If a mathematical model that does not guarantee Ulam stability is processed statistically, a large gap may arise between the actual phenomenon and the solution of the mathematical model in a short time. In other words, the credibility of the results of analysis obtained by using the standard method of statistical processing will be compromised. In this way, the reliability of the mathematical model can be guaranteed by determining the Ulam stability. Thus, we can conclude that Ulam stability in the biological dynamics is an important problem. Until now, much of the research on Ulam stability has been performed with a bias toward mathematical interests. However, in this study, we will explain that the mathematical model (in particular, the von Bertalanffy growth model with Allee effect) is useful from a mathematical perspective by reliably determining the Ulam stability of the mathematical model and clarifying the stability of the model itself. We aim to contribute to ensuring that mathematical models can be handled with confidence. The attempt to consider Ulam stability as the stability of the mathematical model itself is the novelty of this study from both the biological and mathematical perspectives, and it represents a new challenge. Furthermore, the stability of the mathematical model itself cannot be determined no matter how many experiments are conducted. It is an unshakable fact that can only be clarified through mathematical investigation.
Ulam stability of differential equations was started in the 1990s, and it is still actively researched (see [9]). Linear differential equations have been the mainstay of research on Ulam stability, but, recently, the Ulam stability of nonlinear differential equations has also been investigated; see [10,11,12,13,14,15,16,17,18,19,20,21,22,23]. In addition, Ulam stability is known to be closely related to Lipschitz shadowing. For example, see [24,25] and the references cited therein.
In 2018, Popa et al. [26] defined a new concept of Ulam stability, as shown below, in order to carry out particularly sharp investigations into nonlinear analysis.
Let S be a subset of R, and let M>0 or M=∞. Define the class CS by
CS:={x∈C1[0,∞):x(0)∈S⊆R}. |
The nonlinear differential equation
x′=F(x) | (1.3) |
is conditionally Ulam-stable with M and S if there exists K>0 such that, for every ε∈(0,M] and every approximate solution ξ∈CS satisfying
|ξ′−F(ξ)|≤εfort∈[0,∞), |
there exists a solution x∈CS of Eq (1.3) such that
|ξ(t)−x(t)|≤Kεfort∈[0,∞). |
We call such K an Ulam constant for Eq (1.3).
If M=∞ and S=R, then Eq (1.3) can be called Ulam-stable. However, this paper does not deal with mere Ulam stability. Moreover, x is a solution of Eq (1.3) and x∈CS means that the solution x exists on [0,∞) as long as the initial value x(0) is contained in S.
Popa et al. [26] investigated the conditional Ulam stability of a logistic equation in which solutions that diverge in finite time and solutions that exist globally in time coexist. In fact, they gave the following result for the equation x′=x(1−x).
Theorem 1 ([26]). The logistic equation x′=x(1−x) is conditionally Ulam-stable with M=14 and S=[12,∞), and with an Ulam constant K1=2.
Here, M=14 and 12 in S are the threshold values (see [26]). Later, Onitsuka [27] gave a result that extended this theorem. On the other hand, he also obtained a result on stability analysis of the von Bertalanffy growth model described by Eq (1.2). The following result is given in [28].
Theorem 2 ([28]). Let a>0 and b>0. Then, Equation (1.2) is conditionally Ulam-stable with M=a3(2a3b)2 and S=[(2a3b)3,∞), and with an Ulam constant K2=3b(1912)52.
Note that M=a3(2a3b)2 and (2a3b)3 in S are the threshold values (see [28]). This result leads us to ask the following question: "Is conditional Ulam stability guaranteed when the Allee effect is added to Eq (1.2)? Furthermore, if conditional Ulam stability holds, how does the Allee effect affect the above threshold and Ulam constant?" As mentioned earlier, Ulam stability refers to the stability of the given mathematical model itself. In other words, when a real phenomenon is used as a perturbed equation for a mathematical model, it is guaranteed that a solution of the mathematical model that is close to the real phenomenon exists. Theorem 2, which was taken from a previous study, guarantees the conditional Ulam stability of the standard von Bertalanffy growth model given by Eq (1.2), making the stability of the model itself clear. If it is found that the equation including the Allee effect is stable, the stability of the model given by Eq (1.1) itself will be guaranteed, which will be an advantage when proceeding with other research (for example, research that analyzes phenomena by statistically processing mathematical models). Also, if we know the thresholds for the restrictions imposed on the initial value and ε, it becomes clear what initial value to choose to ensure stability. For this reason, we began our study with the aim of answering the above questions. In addition to these questions, we emphasize here that we have derived an Ulam constant that is smaller than that in Theorem 2.
This paper is organized as follows. In the next section, we transform Eq (1.1) into a simpler form of the equation and investigate the detailed behavior of the solutions of its perturbed equations. We will also explain that the conditions imposed on the initial value and ε become the threshold. In Section 3, we give some lemmas that are necessary to prove a theorem given in Section 4. In Section 4, we give the core result of this paper and prove it. In Section 5, we give two main results for Eq (1.1). In Section 6, we present some examples and numerical simulations.
Using τ:=bct and y:=(ba)3x, Equation (1.1) is transformed into the differential equation
dydτ=(y23−y)(1−adbcy13) |
for τ≥0. Note that we only need to consider the case in which the condition
0<adbc≤1 |
is satisfied, because, by using τ:=adt and z:=(dc)3x, Equation (1.1) is transformed into the differential equation
dzdτ=(z23−bcadz)(1−z13)=z23(1−bcadz13)(1−z13)=(z23−z)(1−bcadz13) |
for τ≥0. This equation is equivalent to the previous transformed equation with adbc replaced by bcad. If adbc≥1, then
0<bcad≤1, |
then, it will be the same as the previous situation. In this paper, we are interested in cases in which the Allee effect d>0 is very small or very large. From the above discussion, we can see that we only need to focus on cases in which the Allee effect d>0 is very small; that is, we assume that
0<adbc≪1. |
Now, we consider the differential equation
x′=(x23−x)(1−γx13), | (2.1) |
where
0<γ<1. |
Consider the perturbed equations
p′=(p23−p)(1−γp13)+f(t),|f(t)|≤ε, | (2.2) |
q′=(q23−q)(1−γq13)−ε, | (2.3) |
and
r′=(r23−r)(1−γr13)+ε | (2.4) |
for t≥0, where f is a continuous function. Since the right-hand sides of the differential equations above are all continuously differentiable in the positive domain, the uniqueness of the solution is guaranteed in the positive domain.
Lemma 3. Let 0<γ<1, and let
f(x):=(x23−x)(1−γx13) | (2.5) |
for x≥0; also, let
α1:=[3(γ+1)−3√(γ+1)2−329γ8γ]3,α2:=[3(γ+1)+3√(γ+1)2−329γ8γ]3. | (2.6) |
Then, f(0)=f(1)=f(γ−3)=0, and
0<α1<1<α2<γ−3, | (2.7) |
dfdx(α1)=dfdx(α2)=0 | (2.8) |
hold. Moreover, dfdx(x)>0 if x∈(0,α1)∪(α2,∞); dfdx(x)<0 if x∈(α1,α2). Therefore, f(x) takes the local maximum value at x=α1 and the local minimum value at x=α2; also, f(x)>0 if x∈(0,1)∪(γ−3,∞); f(x)<0 if x∈(1,γ−3).
Proof. Clearly, f(0)=f(1)=f(γ−3)=0 holds. We have
dfdx(x)=23x−13+43γx13−γ−1=x−13[43γx23−(γ+1)x13+23]. |
Now, we consider the function
f1(z)=43γz2−(γ+1)z+23,z>0. |
Then, we can solve f1(z)=0, and we get
z=3(γ+1)±3√(γ+1)2−329γ8γ. |
Let α1 and α2 be given by Eq (2.6). Then, we obtain Eq (2.8). Since the graph of f1(z) is a parabola, 0<γ<1, and
limz→+0f1(z)=23>0,f1(1)=−1−γ3<0,f1(γ−1)=1−γ3γ>0, |
hold, we obtain Eq (2.7). These facts imply that dfdx(x)>0 if x∈(0,α1)∪(α2,∞); dfdx(x)<0 if x∈(α1,α2). This completes the proof.
Lemma 3 states that equilibrium points x=0 and x=γ−3 of Eq (2.1) are unstable, and that the equilibrium point x=1 of Eq (2.1) is asymptotically stable. Therefore, we obtain the following lemma.
Lemma 4. Let 0<γ<1. Then, the solution x of Eq (2.1) with
x(0)∈[0,γ−3] |
exists on [0,∞). Moreover, x(t)∈[0,γ−3] for all t∈[0,∞).
In addition, the following lemmas also hold.
Lemma 5. Let 0<γ<1. Let f and α1 be given as in Eqs (2.5) and (2.6). If 0<ε≤f(α1), then the solution q of Eq (2.3) with
q(0)∈[α1,γ−3] |
exists on [0,∞). Moreover, q(t)∈[α1,γ−3] for all t∈[0,∞).
Lemma 6. Let 0<γ<1. Let f and α2 be given as in Eqs (2.5) and (2.6). If 0<ε≤|f(α2)|, then the solution r of Eq (2.4) with
r(0)∈[0,α2] |
exists on [0,∞). Moreover, r(t)∈[0,α2] for all t∈[0,∞).
Using Lemmas 4–6, we get the following result.
Proposition 7. Let 0<γ<1, and let x, p, q, and r be the solutions of Eqs (2.1)–(2.4) with
x(0)=p(0)=q(0)=r(0)=p0, | (2.9) |
respectively. Let f, α1, and α2 be given as in Eqs (2.5) and (2.6). If
0<ε≤min{f(α1),|f(α2)|}andα1≤p0≤α2, |
then x, p, q, and r exist on [0,∞), and
α1≤q(t)≤p(t)≤r(t)≤α2andq(t)<x(t)<r(t) |
for t∈(0,∞).
Proof. Let 0<γ<1. Let f, α1, and α2 be given as in Eqs (2.5) and (2.6). Suppose that
0<ε≤min{f(α1),|f(α2)|}andα1≤p0≤α2. |
Now, we consider the solutions x, p, q, and r of Eqs (2.1)–(2.4) with Eq (2.9), respectively. From Lemmas 4–6, we see that x, q, and r exist on [0,∞).
First, we prove that q(t)≤p(t) for t≥0. Define g(t):=p(t)−q(t) for t≥0. By Eq (2.9), we have that g(0)=0. Suppose that there exists τ1≥0 such that g(τ1)<0. Because g is a C1-function with g(0)=0, we see that there exists 0≤t1≤τ1 such that g(t1)=0 and
g(t)<0 |
for t1<t≤τ1. Using this, we get
g′=p′−q′=(p23−q23)+γ(p43−q43)−(1+γ)(p−q)+f(t)+ε=(p23−q23p−q+γp43−q43p−q−1−γ)(p−q)+f(t)+ε≥(p23−q23p−q+γp43−q43p−q−1−γ)g=:ψ(t)g, |
thus,
(g(t)exp(−∫tt1ψ(s)ds))′≥0 |
for t1<t≤τ1. Therefore,
g(t)≥g(t1)exp(∫tt1ψ(s)ds)=0 |
for t1<t≤τ1. This contradicts the fact that g(t) is negative for t1<t≤τ1. Hence, we can conclude that q(t)≤p(t) for t≥0. Using the same method, we see that the inequality p(t)≤r(t) holds for t≥0. Thus, we obtain that q(t)≤p(t)≤r(t) for t≥0. In addition, the solution p of Eq (2.2) exists on [0,∞).
Next, we prove that q(t)<x(t) for t>0. Let h(t):=x(t)−q(t) for t≥0. By Eq (2.9), we have that h(0)=0. Note that Eq (2.1) is a special case of Eq (2.2); that is, when f(t)≡0, p(t) becomes x(t). Since q(t)≤p(t)=x(t) holds for t≥0, we see that h(t)≥0 for t≥0. Applying h(0)=0, we get that h′(0)=ε>0. This shows that there is an interval on the right side of t=0 where h takes a positive value.
Now, we suppose that there exists τ2>0 such that h(τ2)=0 and h(t)>0 for 0<t<τ2. Then, we have
h′(t)=(x23−q23x−q+γx43−q43x−q−1−γ)(x−q)+ε>ψ(t)h. |
This implies that
(h(t)exp(−∫t0ψ(s)ds))′>0 |
for 0<t<τ2. Integrating this inequality from τ22 to τ2, we obtain
h(τ2)>h(τ22)exp(∫τ2τ22ψ(s)ds)>0. |
But, we assumed that h(τ2)=0. This is a contradiction. Therefore, q(t)<x(t) for t>0. Using the same method, we can get that x(t)<r(t) for t>0. This completes the proof.
Remark 8. Let f, α1, and α2 be given as in Eqs (2.5) and (2.6). Suppose that ε>f(α1). For δ>0, let ε=f(α1)+δ. From Eq (2.3) and Lemma 3, we have
q′=f(q)−ε=f(q)−f(α1)−δ≤−δ<0 |
for q∈(0,α2]. Integrating this inequality from 0 to t≥0, we have
q(t)≤q(0)−δt |
for t≥0. This implies that
limt→∞q(t)=−∞. |
However, since q(t) is a solution to Eq (2.3), it is not negative. This means that the solution q(t) vanishes in finite time. Thus, we note that we cannot discuss conditional Ulam stability. For this reason, ε=f(α1) is the threshold.
Remark 9. Let f, α1, and α2 be given as in Eqs (2.5) and (2.6). Suppose that ε=f(α1) and q(0)<α1. From Eq (2.3) and Lemma 3, we have
q′=f(q)−ε=f(q)−f(α1)<0 |
for q∈(0,α1). Integrating this inequality from 0 to t≥0, and using q(0)<α1, we have
q(t)≤q(0)<α1 |
for t≥0. From Lemma 3, we know that dfdx(x)>0 for x∈(0,α1). Using the above facts, we obtain
q′(t)=f(q(t))−ε≤f(q(0))−ε<f(α1)−ε=0 |
for t≥0. Integrating this inequality, we get
q(t)≤q(0)+(f(q(0))−ε)t |
for t≥0. Since f(q(0))−ε is negative, we get
limt→∞q(t)=−∞. |
For the same reason as Remark 8, we see that q(0)=α1 is the threshold.
Lemma 10. Let 0<γ<1 and L≥1. Let α2 be given as in Eq (2.6). Then, the solution x of Eq (2.1) with
x(0)∈[0,min{L,α2}] |
satisfies that x(t)∈[0,min{L,α2}] for all t∈[0,∞).
Proof. Lemma 3 implies that equilibrium points x=0 and x=γ−3 of Eq (2.1) are unstable, and that the equilibrium point x=1 of Eq (2.1) is asymptotically stable. In addition, the solution x(t) of Eq (2.1) is a strictly monotonic function, except when x=0, 1, γ−3. Thus, x(0)∈[0,min{L,α2}] implies that x(t)∈[0,min{L,α2}] for all t∈[0,∞).
Lemma 11. Let 0<γ<1, and let f, α1, and α2 be given as in Eqs (2.5) and (2.6). Then, the following relationships hold:
limγ→0α1=(23)3=827,limγ→0f(α1)=13(23)2=427,limγ→0α2=∞,limγ→0f(α2)=−∞. |
In addition, if 0<γ≪1, then f(α1)=min{f(α1),|f(α2)|} holds and there exists 1<r∗<2 such that f(r∗)+f(α1)=0.
Proof. By Eq (2.6) and l'Hopital's rule, we have
limγ→0α131=limγ→03−3⋅12[(γ+1)2−329γ]−12[2(γ+1)−329]8=3−32(2−329)8=23, |
thus,
limγ→0α1=(23)3,limγ→0f(α1)=(23)2−(23)3=13(23)2. |
By Eq (2.6), we have that limγ→0α2=∞. Since
f(α2)=[3(γ+1)+3√(γ+1)2−329γ8γ]2[1−3(γ+1)+3√(γ+1)2−329γ8γ]×[1−3(γ+1)+3√(γ+1)2−329γ8] |
and
limγ→03(γ+1)+3√(γ+1)2−329γ8=34 |
hold, we obtain that limγ→0f(α2)=−∞. Because limγ→0f(α1)<∞ and limγ→0|f(α2)|=∞ are satisfied, if 0<γ≪1, then f(α1)=min{f(α1),|f(α2)|}. Suppose that 0<γ≪1. Let
g(x)=f(x)+f(α1)=(x23−x)(1−γx13)+(α231−α1)(1−γα131). |
Then,
limγ→0g(1)=limγ→0f(α1)=13(23)2>0, |
and
limγ→0g(2)=223−2+(23)2−(23)3=223−2+13(23)2≈−0.26445<0. |
This means that, if 0<γ≪1, then there exists 1<r∗<2 such that g(r∗)=0.
Lemma 12. Let 0<γ≪1, and let f, α1, and α2 be given as in Eqs (2.5) and (2.6). Let 1<r∗<2 satisfy that f(r∗)+f(α1)=0, and let L≥r∗. Suppose that 0<ε≤f(α1). Then, the solution r of Eq (2.4) with
r(0)∈[α1,min{L,α2}] |
satisfies that r(t)∈[α1,min{L,α2}] for all t∈[0,∞).
Proof. Let 0<γ≪1. From Lemma 11, we see that f(α1)=min{f(α1),|f(α2)|}, and that there exists 1<r∗<2 such that f(r∗)+f(α1)=0. Let L≥r∗ and 1<rε<α2 satisfy that f(rε)+ε=0. Then, by Lemma 3, r=rε is a equilibrium point of Eq (2.4) and is asymptotically stable; also, rε≤r∗ holds. In addition, the solution r(t) of Eq (2.4) is a strictly monotonic function on [α1,min{L,α2}], except when r=rε. Thus, r(0)∈[α1,min{L,α2}] implies that r(t)∈[α1,min{L,α2}] for all t∈[0,∞).
Before discussing conditional Ulam stability, we give some lemmas.
Lemma 13. The inequality
1−y21−y3≤431+y |
holds for y>0 and y≠1.
Proof. For 0<y<1, we have
0≤13(1−y)3=43(1−y3)−(1+y)(1−y2). |
This implies that the desired inequality is satisfied. For y>1, we have
0≥13(1−y)3=43(1−y3)−(1+y)(1−y2). |
Since 1−y3 is negative, we get
(1+y)1−y21−y3≤43, |
thus, we obtain the desired inequality. This completes the proof.
Lemma 14. Let 0<γ<1, and let α1 be given as in Eq (2.6). Define
X(t,w):=1−γw−(1−w)e−13(1−γ)t1−γw−γ(1−w)e−13(1−γ)t | (3.1) |
for (t,w)∈[0,∞)×[0,∞). Then, the solution to Eq (2.1) with x(0)=x0≥0 is given by
x(t)=X3(t,x130) |
for t∈[0,∞). If x0≥α1, then
x(t)=X3(t,x130)≥X3(t,α131)>α1 |
for all t∈(0,∞).
Proof. Clearly, x(t)≡1, γ−3 constitutes trivial solutions. Assume that x(t)≠1, γ−3 for all t≥0. Let y:=x13. Then,
y′=13x−23x′=13x−23(x23−x)(1−γx13)=13(1−y)(1−γy), |
thus,
11−γ(−γ1−γy−−11−y)=y′(1−y)(1−γy)=13 |
for all t≥0. Integrating this inequality, we get
1−y(t)1−γy(t)=1−y(0)1−γy(0)e−13(1−γ)t |
Thus,
y(t)=1−γy(0)−(1−y(0))e−13(1−γ)t1−γy(0)−γ(1−y(0))e−13(1−γ)t=X(t,y(0))=X(t,x130). |
This equality gives the solution to Eq (2.1) with x(0)=x0≥0.
Since
X(t,w)=1−γw−(1−w)e−13(1−γ)t1−γw−γ(1−w)e−13(1−γ)t=1−e−13(1−γ)t+(e−13(1−γ)t−γ)w1−γe−13(1−γ)t+(γe−13(1−γ)t−γ)w |
holds, we have
∂∂wX(t,w)=(1−γ)2e−13(1−γ)t[1−γe−13(1−γ)t+(γe−13(1−γ)t−γ)w]2>0 |
and
(X(t,α131))′=13(1−γ)2(1−α131)(1−γα131)e−13(1−γ)t[1−γα131−γ(1−α131)e−13(1−γ)t]2>0 |
Using these facts, we get
y(t)=X(t,x130)≥X(t,α131)>X(0,α131)=α131 |
for all t>0. This completes the proof.
Proposition 15. Let 0<γ≪1, and let f, α1, and α2 be given as in Eqs (2.5) and (2.6). Let 1<r∗<2 satisfy that f(r∗)+f(α1)=0, and let L≥r∗. Suppose that ε∈(0,f(α1)] and p0∈[α1,min{L,α2}]. Let x, p, q, and r be the solutions of Eqs (2.1)–(2.4) with Eq (2.9), respectively. Then,
x23(t)−q23(t)x(t)−q(t)−1+γ(x43(t)−q43(t)x(t)−q(t)−1)<4(1+α131)(1+γα131)g′(t)g(t)−1+γ(43L13−1) |
and
r23(t)−x23(t)r(t)−x(t)−1+γ(r43(t)−x43(t)r(t)−x(t)−1)<4(1+α131)(1+γα131)g′(t)g(t)−1+γ(43L13−1) |
hold for t∈(0,∞), where
g(t):=(1+α131)(1−γα131)γ(1−α131)e13(1+γα131)t−1γ(1+γα131)e13γ(1+α131)t>0. | (3.2) |
Proof. Let 0<γ≪1, and let f, α1, and α2 be given as in Eqs (2.5) and (2.6). By Lemma 11, we see that f(α1)=min{f(α1),|f(α2)|}, and that there exists 1<r∗<2 such that f(r∗)+f(α1)=0. Let L≥r∗. Assume that ε∈(0,f(α1)] and p0∈[α1,min{L,α2}]. From Proposition 7, we see that
α1≤q(t)<x(t)<r(t)≤α2 |
for t>0. Let X be given by Eq (3.1).
We will prove the first inequality. Since 0<q(t)x(t)<1 is satisfied, using Lemma 13, we have
x23(t)−q23(t)x(t)−q(t)−1+γ(x43(t)−q43(t)x(t)−q(t)−1)=x23(t)x(t)1−(q(t)x(t))231−(q(t)x(t))33−1+γ[x43(t)x(t)1−(q(t)x(t))431−(q(t)x(t))33−1]≤x23(t)x(t)431+(q(t)x(t))13−1+γ{x43(t)x(t)43[1+(q(t)x(t))23]1+(q(t)x(t))13−1}=43x13(t)+q13(t)−1+γ{x13(t)43[1+(q(t)x(t))23]1+(q(t)x(t))13−1}<43x13(t)+q13(t)−1+γ(43x13(t)−1) |
for t>0. Moreover, by Lemmas 10 and 14, we obtain
x23(t)−q23(t)x(t)−q(t)−1+γ(x43(t)−q43(t)x(t)−q(t)−1)<43X(t,α131)+α131−1+γ(43L13−1) |
for t>0. Notice that
43X(t,α131)+α131=431−γα131−γ(1−α131)e−13(1−γ)t(1+α131)(1−γα131)−(1+γα131)(1−α131)e−13(1−γ)t=431−γα131γ(1−α131)−e−13(1−γ)t(1+α131)(1−γα131)γ(1−α131)−1γ(1+γα131)e−13(1−γ)t×e13(1+γα131)te13(1+γα131)t=4(1+α131)(1+γα131)g′(t)g(t). |
Hence, we get the first inequality. The second inequality can also be shown in the same way, but we need to pay attention to the calculation of the following inequalities. Since 0<x(t)r(t)<1 holds, we have
r43(t)−x43(t)r(t)−x(t)≤r43(t)r(t)1−(x(t)r(t))431−(x(t)r(t))33≤r43(t)r(t)43[1+(x(t)r(t))23]1+(x(t)r(t))13<43r13(t). |
Moreover, by Lemma 12, we obtain
r43(t)−x43(t)r(t)−x(t)<43L13 |
for t>0. This completes the proof of this proposition.
Lemma 16. Let g be given by Eq (3.2). Then,
g(t)g(s)<(1+α131)(1−γα131)e13(1+γα131)(t−s)2(1−γ)α131 |
for all t, s∈(0,∞).
Proof. Since g can be rewritten as
g(t)=[(1+α131)(1−γα131)γ(1−α131)−1γ(1+γα131)e−13(1−γ)t]e13(1+γα131)t, |
we obtain the inequalities
g(t)<(1+α131)(1−γα131)γ(1−α131)e13(1+γα131)t, |
and
g(t)>[(1+α131)(1−γα131)γ(1−α131)−1γ(1+γα131)]e13(1+γα131)t |
for all t>0. Thus, we have
g(t)g(s)<(1+α131)(1−γα131)γ(1−α131)e13(1+γα131)(t−s)(1+α131)(1−γα131)γ(1−α131)−1γ(1+γα131)=(1+α131)(1−γα131)e13(1+γα131)(t−s)2(1−γ)α131 |
for t, s>0.
In this section, we prove the following theorem, which plays an important role in proving the main theorems.
Theorem 17. Let 0<γ≪1, and let f, α1, and α2 be given as in Eqs (2.5) and (2.6). Let 1<r∗<2 satisfy that f(r∗)+f(α1)=0, and let L≥r∗. Suppose that ε∈(0,f(α1)] and p0∈[α1,min{L,α2}]. Let x and p be the solutions of Eqs (2.1) and (2.2) with Eq (2.9), respectively. Then,
|p(t)−x(t)|<˜Kε |
for t∈[0,∞), where
˜K:=[(1+α131)(1−γα131)2(1−γ)α131]4(1+α131)(1+γα131)[3α131−13(1+α131)−γ(43L13−1)]−1. | (4.1) |
Proof. Let 0<γ≪1, and let f, α1, and α2 be given as in Eqs (2.5) and (2.6). By Lemma 11, we see that f(α1)=min{f(α1),|f(α2)|}, and that there exists 1<r∗<2 such that f(r∗)+f(α1)=0. Let L≥r∗. Consider the solutions x, p, q, and r of Eqs (2.1)–(2.4) with Eq (2.9), respectively. Assume that
0<ε≤f(α1)andα1≤p0≤min{L,α2}. |
Then, by Proposition 7, we obtain
α1≤q(t)≤p(t)≤r(t)≤α2andq(t)<x(t)<r(t) |
for t>0; thus,
−(x(t)−q(t))=q(t)−x(t)≤p(t)−x(t)≤r(t)−x(t) |
for t>0. This implies that
|p(t)−x(t)|≤max{r(t)−x(t),x(t)−q(t)} | (4.2) |
for t>0. Let w(t):=r(t)−x(t) and z(t):=x(t)−q(t) for t≥0. Then, we obtain
w′=(r23−x23r−x+γr43−x43r−x−1−γ)w+ε |
and
z′=(x23−q23x−q+γx43−q43x−q−1−γ)z+ε |
for t>0. Note here that w(t) and z(t) are positive functions for t>0. Define
ψ(t):=4(1+α131)(1+γα131)g′(t)g(t)−1+γ(43L13−1), |
where g is given by Eq (3.2). Using Proposition 15, we get the inequality
h′<ψ(t)h+ε |
for t>0, where h is either w or z. From this, we have
(h(t)e−∫t0ψ(s)ds)′<εe−∫t0ψ(s)ds. |
This, together with h(0)=0, yields that
h(t)<h(0)+ε∫t0e∫tsψ(τ)dτds=ε∫t0e∫tsψ(τ)dτds | (4.3) |
for t>0. Using the fact that
∫tsψ(τ)dτ=log(g(t)g(s))4(1+α131)(1+γα131)+[−1+γ(43L13−1)](t−s) |
for t≥s, and Lemma 16, we obtain
∫t0e∫tsψ(τ)dτds=∫t0(g(t)g(s))4(1+α131)(1+γα131)e[−1+γ(43L13−1)](t−s)ds<∫t0[(1+α131)(1−γα131)e13(1+γα131)(t−s)2(1−γ)α131]4(1+α131)(1+γα131)e[−1+γ(43L13−1)](t−s)ds=[(1+α131)(1−γα131)2(1−γ)α131]4(1+α131)(1+γα131)∫t0e[1−3α1313(1+α131)+γ(43L13−1)](t−s)ds |
for t>0. Note that, by Lemma 11, we have
limγ→0α131=23. |
This says that, for 0<γ≪1,
3α131−13(1+α131)−γ(43L13−1)>0. |
Hence, we obtain
∫t0e∫tsψ(τ)dτds<[(1+α131)(1−γα131)2(1−γ)α131]4(1+α131)(1+γα131)[3α131−13(1+α131)−γ(43L13−1)]−1=˜K |
for t>0. From Eq (4.2), we see that
|p(t)−x(t)|≤max{w(t),z(t)}<˜Kε |
for t∈(0,∞). When t=0, this inequality is true. This ends the proof.
Using Theorem 17, we obtain the following result immediately.
Theorem 18. Let 0<γ≪1, and let f, α1, and α2 be given as in Eqs (2.5) and (2.6). Let 1<r∗<2 satisfy that f(r∗)+f(α1)=0, and let L≥r∗. Then, Equation (2.1) is conditionally Ulam-stable with M=f(α1) and S=[α1,min{L,α2}], and with an Ulam constant ˜K, where ˜K is given in Theorem 17.
The first main theorem of this paper is given below.
Theorem 19. Let 0<adbc≪1, and let f, α1, and α2 be given as in Eqs (2.5) and (2.6). Let 1<r∗<2 satisfy that f(r∗)+f(α1)=0, and let L≥r∗. Suppose that ε∈(0,bc(ab)3f(α1)] and ξ0∈[(ab)3α1,(ab)3min{L,α2}]. Let x and ξ be the solutions of Eq (1.1) and
ξ′=(aξ23−bξ)(c−dξ13)+f(t),|f(t)|≤ε | (5.1) |
with x(0)=ξ(0)=ξ0, respectively. Then,
|ξ(t)−x(t)|<˜Kbcε |
for t∈[0,∞), where ˜K is given by Eq (4.1).
Proof. Assume that 0<adbc≪1. Let f, α1, and α2 be given as in Eqs (2.5) and (2.6), let 1<r∗<2 satisfy that f(r∗)+f(α1)=0, and let L≥r∗. Suppose that
0<ε≤bc(ab)3f(α1)and(ab)3α1≤ξ0≤(ab)3min{L,α2}. | (5.2) |
Now, we consider the solution ξ(t) to Eq (5.1) with ξ(0)=ξ0. Let s:=bct and z(s):=(ba)3ξ(t). Then,
ε≥|ξ′(t)−(aξ23(t)−bξ(t))(c−dξ13(t))|=bc(ab)3|dzds(s)−(z23(s)−z(s))(1−adbcz13(s))|. |
That is, we have
|dzds(s)−(z23(s)−z(s))(1−adbcz13(s))|≤1bc(ba)3ε=:˜ε |
for s≥0. Moreover, from Eq (5.2), we see that
0<˜ε≤f(α1)andα1≤z(0)≤min{L,α2}. |
Now, we consider the solution y(s) to the equation
dyds=(y23−y)(1−adbcy13) |
with y(0)=z(0). Then, using Theorem 17, we can conclude that
|z(s)−y(s)|≤˜K˜ε |
for s≥0, where ˜K is given by Eq (4.1).
Let t:=sbc and x(t):=(ab)3y(s). Then,
x′(t)=dxdt(t)=(ab)3dsdtdyds(s)=bc(ab)3(y23(s)−y(s))(1−adbcy13(s))=(ax23(t)−bx(t))(c−dx13(t)) |
for t≥0. This means that x(t) is a solution to Eq (1.1) with
x(0)=(ab)3y(0)=(ab)3z(0)=ξ(0)=ξ0. |
In additon,
|ξ(t)−x(t)|=(ab)3|z(s)−y(s)|≤(ab)3˜K˜ε=(ab)3˜K1bc(ba)3ε=˜Kbcε |
for t≥0. This ends the proof.
Using Theorem 19, we obtain the following result immediately.
Theorem 20. Let 0<adbc≪1, and let f, α1, and α2 be given as in Eqs (2.5) and (2.6). Let 1<r∗<2 satisfy that f(r∗)+f(α1)=0, and let L≥r∗. Then, Equation (1.1) is conditionally Ulam-stable with M=bc(ab)3f(α1) and S=[(ab)3α1,(ab)3min{L,α2}], and with an Ulam constant ˜Kbc, where ˜K is given in Theorem 17.
Remark 21. When c=1 and d→+0, we see that
M=bc(ab)3f(α1)→b(ab)313(23)2=a3(2a3b)2and(ab)3α1→(2a3b)3 |
hold by Lemma 11. Here, when comparing the above results with Theorem 2, we find that the values of M are the same and the values on the left side of the set S are also the same. In addition, c=1 and d→+0 imply that L=min{L,∞}; thus, we can choose L≥r∗ to be arbitrary. Moreover, by Lemma 11, we obtain
˜Kbc→1b(1+23223)41+23[323−13(1+23)]−1=5b(1+23223)41+23=5b(54)125=:K0. |
Comparing this Ulam constant with the Ulam constant K2 presented in Theorem 2, we see that
K2=3b(1912)52≈9.46351b>8.54189b≈5b(54)125=K0. |
This indicates that our main result yields a smaller and more precise Ulam constant than in the previous study.
The second main theorem of this paper is given below.
Theorem 22. Let 0<bcad≪1, and let f, α1, and α2 be given as in Eqs (2.5) and (2.6). Let 1<r∗<2 satisfy that f(r∗)+f(α1)=0, and let L≥r∗. Suppose that ε∈(0,ad(cd)3f(α1)] and ξ0∈[(cd)3α1,(cd)3min{L,α2}]. Let x and ξ be the solutions of Eqs (1.1) and (5.1) with x(0)=ξ(0)=ξ0, respectively. Then,
|ξ(t)−x(t)|<˜Kadε |
for t∈[0,∞), where ˜K is given by Eq (4.1).
Proof. Assume that 0<bcad≪1. Let f, α1, and α2 be given as in Eqs (2.5) and (2.6), let 1<r∗<2 satisfy that f(r∗)+f(α1)=0, and let L≥r∗. Suppose that
0<ε≤ad(cd)3f(α1)and(cd)3α1≤ξ0≤(cd)3min{L,α2}. | (5.3) |
Now, we consider the solution ξ(t) to Eq (5.1) with ξ(0)=ξ0. Let s:=adt and z(s):=(dc)3ξ(t). Then,
ε≥|ξ′(t)−(aξ23(t)−bξ(t))(c−dξ13(t))|=ad(cd)3|dzds(s)−(1−bcadz13(s))(z23(s)−z(s))|. |
That is, we have
|dzds(s)−(1−bcadz13(s))(z23(s)−z(s))|≤1ad(dc)3ε=:˜ε |
for s≥0. Moreover, from Eq (5.3), we see that
0<˜ε≤f(α1)andα1≤z(0)≤min{L,α2}. |
Now, we consider the solution y(s) to the equation
dyds=(y23−y)(1−bcady13) |
with y(0)=z(0). Then, using Theorem 17, we can conclude that
|z(s)−y(s)|≤˜K˜ε |
for s≥0, where ˜K is given by Eq (4.1).
Let t:=sad and x(t):=(cd)3y(s). Then,
x′(t)=dxdt(t)=(cd)3dsdtdyds(s)=ad(cd)3(y23(s)−y(s))(1−bcady13(s))=(c−dx13(t))(ax23(t)−bx(t)) |
for t≥0. This means that x(t) is a solution to Eq (1.1) with
x(0)=(cd)3y(0)=(cd)3z(0)=ξ(0)=ξ0. |
In addition,
|ξ(t)−x(t)|=(cd)3|z(s)−y(s)|≤(cd)3˜K˜ε=(cd)3˜K1ad(dc)3ε=˜Kadε |
for t≥0. This ends the proof.
Using Theorem 22, we obtain the following result immediately.
Theorem 23. Let 0<bcad≪1, and let f, α1, and α2 be given as in Eqs (2.5) and (2.6). Let 1<r∗<2 satisfy that f(r∗)+f(α1)=0, and let L≥r∗. Then, Equation (1.1) is conditionally Ulam-stable with M=ad(cd)3f(α1) and S=[(cd)3α1,(cd)3min{L,α2}], and with an Ulam constant ˜Kad, where ˜K is given in Theorem 17.
In this section, we present some examples. We consider the perturbed von Bertalanffy growth model with Allee effect of the form
ξ′=(aξ23−bξ)(c−dξ13)+f(t) | (6.1) |
for t≥0, where a, b, c, and d are positive constants and f(t) is a continuous function. Note that the parameters used in all examples presented in this section are not determined based on data that describe real phenomena, and that the parameters are given by the authors for an example calculation.
Example 24. Consider Eq (6.1) with
a=6,b=2,c=1,d=19,f(t)=6.265sin2t. | (6.2) |
In this case, we have that r∗≈1.49485 satisfies the equation f(r∗)+f(α1)=0, where f and α1 are given as in Eqs (2.5) and (2.6). Let L=32=1.5 (>r∗). Then, we see that
adbc=13,M=bc(ab)3f(α1)=−812+27√3≈6.26537, |
and
(ab)3α1=814(9−5√3)≈6.87986,(ab)3min{L,α2}=812, |
where these values appear in Theorems 19 and 20, and α2 is given as in Eq (2.6). Then,
˜Kbc≈136.877, |
where ˜K is given by Eq (4.1).
Let x and ξ be the solutions of Eqs (1.1) and (6.1) with Eq (6.2) and x(0)=ξ(0)=ξ0, respectively. Assume that
ξ0∈[814(9−5√3),812]. |
Let ε=6.265 (<M). Then, by Theorem 19, we can conclude that
|ξ(t)−x(t)|<˜Kbcε≈136.877×6.265=857.534 |
for t∈[0,∞).
In this case, the amplitude of f(t) is large, and this has a strong effect on the error between the approximate solution ξ(t) and the true solution x(t). However, if f(t) is changed to a function with a small amplitude, the error can be suppressed to a small value.
Example 25. Consider Eq (6.1) with
a=3,b=2,c=32,d=1100,f(t)=0.3sin2t. | (6.3) |
In this case, we have that r∗≈1.40072 satisfies the equation f(r∗)+f(α1)=0, where f and α1 are given as in Eqs (2.5) and (2.6). Let L=10 (>r∗). Then, we see that
adbc=1100,M=bc(ab)3f(α1)≈1.49001,(ab)3α1≈0.996644, |
and
(ab)3min{L,α2}≈33.75, |
where these values appear in Theorems 19 and 20, and α2 is given as in Eq (2.6). Then,
˜Kbc≈3.16765, |
where ˜K is given by Eq (4.1).
Let x and ξ be the solutions of Eqs (1.1) and (6.1) with Eq (6.3) and x(0)=ξ(0)=ξ0, respectively. Assume that
ξ0∈[0.996644,33.75]. |
Let ε=0.3 (<M). Then, by Theorem 19, we can conclude that
|ξ(t)−x(t)|<˜Kbcε≈3.16765×0.3=0.950295 |
for t∈[0,∞).
The blue and red curves represent the solution curves for Eqs (1.1) and (6.1) with Eq (6.3) and x(0)=ξ(0)=1, respectively. See Figure 1. The dashed lines represent the ˜Kbcε neighborhood of the solution curve for Eq (6.1) with Eq (6.3).
Example 26. Consider Eq (6.1) with
a=3,b=2,c=32,d=1100,f(t)=−1.5. | (6.4) |
Let L=10. Note that the values r∗, adbc, M, (ab)3α1, (ab)3min{L,α2}, and ˜Kbc appearing in Theorem 19 are the same as in the previous example. However, we must note that the perturbation term f(t) in our equation is −1.5. Then, we have
M=bc(ab)3f(α1)≈1.49001<1.5=ε. |
Therefore, our Theorem 19 cannot be applied.
Actually, if the initial value is selected as x(0)=ξ(0)=1 and a numerical simulation is performed in this case, the result will be as shown in Figure 2. The blue and red curves represent the solution curves for Eqs (1.1) and (6.1) with Eq (6.4) and x(0)=ξ(0)=1, respectively. Figure 2 shows that the solution curve for Eq (6.1) with Eq (6.4) hits the t axis at a finite time and then disappears.
In many studies dealing with mathematical models, the challenge is to examine the fit between actual data and the solution of the mathematical model by performing statistical processing. However, many previous reports do not mention the possibility that the solution of the mathematical model may not be consistent with the actual phenomenon due to small perturbations. For example, depending on the balance between the initial value and the amplitude of the perturbation, that is, ε>0, the behavior of the solutions of the unperturbed and perturbed equations can be very different, even if the initial values are the same, as shown in Figure 2. Therefore, we need a concept that guarantees that the behavior of the solutions of the unperturbed and perturbed equations is similar. In this study, we adopted Ulam stability, a concept that guarantees that the solution of an unperturbed equation exists close to the solution of an equation with a bounded perturbation. Furthermore, we aimed to identify appropriate initial values and perturbation amplitudes that would guarantee the concept.
Especially, we considered the conditional Ulam stability of a von Bertalanffy growth model with Allee effect. This study proceeded as follows. First, we transformed the original equation into a simple equation:
x′=(x23−x)(1−γx13). |
Next, the behavior of solutions to the perturbed equations has been investigated. This yields thresholds related to ε and the initial value, which are important for conditional Ulam stability. After presenting some sharp inequalities, the core theorem (Theorem 17) was proven. We then established the main theorems (Theorems 19, 20, 22, and 23) for the original equation. In a sense, it can be said that the results obtained correspond to the case in which the Allee effect is either very small or very large. In addition, we must emphasize that we succeeded in deriving an Ulam constant that is better than the previous result for the standard von Bertalanffy growth model when the Allee effect approaches 0. It becomes clear that the Ulam constant K0 actually obtained in this study satisfies the inequality
K2=3b(1912)52≈9.46351b>8.54189b≈5b(54)125=K0, |
and is smaller than the previous result K2 (see Remark 21). In other words, we can conclude that this paper improves on the proof of Theorem 2, which is given in [28] in the absence of the Allee effect. Finally, some examples and numerical simulations have been attached to illustrate the obtained results.
The above results show that Ulam stability can be guaranteed in the von Bertalanffy growth model with Allee effect by selecting an appropriate initial value and perturbation amplitude. In other words, the solutions of the perturbed equation and the unperturbed equation remain close to each other in the infinite interval [0,∞); thus, the stability of the equation itself is guaranteed. This study showed that slight variations have no effect on solutions with initial values within the appropriate range.
As mentioned at the beginning of Section 2, Equation (1.1) is transformed into the equation
dydτ=(y23−y)(1−adbcy13) |
for τ≥0 by applying τ:=bct and y:=(ba)3x. From Lemma 14, we can see that the solution y(τ) of this equation with the initial condition y(0)=y0≥0 is given as y(τ)=X3(τ,y130), where X is given by Eq (3.1). Hence, we were able to obtain the solution of Eq (1.1) with x(0)=x0≥0 by using
x(t)=(ab)3y(bct)=(abX(bct,bax130))3, |
that is, we can obtain an explicit solution of Eq (1.1). If actual data of some phenomenon are given, we may be able to determine parameters a, b, c, and d by fitting the solution curve x(t)=(abX(bct,bax130))3. However, we note that the problem of how to determine f(t) in Eq (6.1) still remains. In mathematical models, f(t) often means inflow or outflow, so if we can identify its amplitude, that is, ε>0, we may be able to apply our results to actual phenomena.
The authors declare they have not used Artificial Intelligence (AI) tools in the creation of this article.
The authors would like to thank the referees for reading carefully and giving valuable comments to improve the quality of the paper. The second author was supported by JSPS KAKENHI grant number JP20K03668.
The authors declare that there is no conflict of interest.
[1] |
L. von Bertalanffy, Quantitative laws in metabolism and growth, Q. Rev. Biol., 32 (1957), 217–231. https://doi.org/10.1086/401873 doi: 10.1086/401873
![]() |
[2] |
J. Calatayud, T. Caraballo, J. C. Cortés, M. Jornet, Mathematical methods for the randomized non-autonomous Bertalanffy model, Electron. J. Differ. Equations, 2020 (2020), 19. https://doi.org/10.58997/ejde.2020.50 doi: 10.58997/ejde.2020.50
![]() |
[3] |
M. P. Edwards, R. S. Anderssen, Symmetries and solutions of the non-autonomous von Bertalanffy equation, Commun. Nonlinear Sci. Numer. Simul., 22 (2015), 1062–1067. https://doi.org/10.1016/j.cnsns.2014.08.033 doi: 10.1016/j.cnsns.2014.08.033
![]() |
[4] |
H. Kim, R. Lim, Y. I. Seo, D. Sheen, A modified von Bertalanffy growth model dependent on temperature and body size, Math. Biosci., 294 (2017), 57–61. https://doi.org/10.1016/j.mbs.2017.10.006 doi: 10.1016/j.mbs.2017.10.006
![]() |
[5] |
P. Román-Román, D. Romero, F. Torres-Ruiz, A diffusion process to model generalized von Bertalanffy growth patterns: fitting to real data, J. Theor. Biol., 263 (2010), 59–69. https://doi.org/10.1016/j.jtbi.2009.12.009 doi: 10.1016/j.jtbi.2009.12.009
![]() |
[6] |
R. Wiff, M. A. Barrientos, A. C. Milessi, J. C. Quiroz, J. Harwood, Modelling production per unit of food consumed in fish populations, J. Theor. Biol., 365 (2015), 67–75. https://doi.org/10.1016/j.jtbi.2014.10.004 doi: 10.1016/j.jtbi.2014.10.004
![]() |
[7] |
J. L. Rocha, A. K. Taha, D. Fournier-Prunaret, Big bang bifurcations in von Bertalanffy's dynamics with strong and weak Allee effects, Nonlinear Dyn., 84 (2016), 607–626. https://doi.org/10.1007/s11071-015-2510-6 doi: 10.1007/s11071-015-2510-6
![]() |
[8] |
H. Nishiura, S. Tsuzuki, B. Yuan, T. Yamaguchi, Y. Asai, Transmission dynamics of cholera in Yemen, 2017: a real time forecasting, Theor. Biol. Med. Model., 14 (2017), 14. https://doi.org/10.1186/s12976-017-0061-x doi: 10.1186/s12976-017-0061-x
![]() |
[9] | J. Brzdęk, D. Popa, I. Raşa, B. Xu, Ulam Stability of Operators, Academic Press, London, 2018. |
[10] |
A. Buicǎ, Ulam–Hyers stability and exponentially dichotomic evolution equations in Banach spaces, Electron. J. Qual. Theory Differ. Equ., 2023 (2023), 1–10. https://doi.org/10.14232/ejqtde.2023.1.8 doi: 10.14232/ejqtde.2023.1.8
![]() |
[11] |
C. Chen, L. Liu, Q. Dong, Existence and Hyers-Ulam stability for boundary value problems of multi-term Caputo fractional differential equations, Filomat, 37 (2023), 9679–9692. https://doi.org/10.1002/mma.3928 doi: 10.1002/mma.3928
![]() |
[12] |
M. Choubin, H. Javanshiri, A new approach to the Hyers–Ulam–Rassias stability of differential equations, Results Math., 76 (2021), 1–14. https://doi.org/10.1007/s00025-020-01318-w doi: 10.1007/s00025-020-01318-w
![]() |
[13] |
J. Huang, S. M. Jung, Y. Li, On Hyers–Ulam stability of nonlinear differential equations, Bull. Korean Math. Soc., 52 (2015), 685–697. https://doi.org/10.4134/BKMS.2015.52.2.685 doi: 10.4134/BKMS.2015.52.2.685
![]() |
[14] | K. Hyasat, M. Qarawani, Hyers–Ulam–Rassias instability for Bernoulli's and nonlinear differential equations, Jordan J. Math. Stat., 15 (2022), 857–870. |
[15] | S. M. Jung, A fixed point approach to the stability of differential equations y′=F(x,y), Bull. Malays. Math. Sci. Soc., 33 (2010), 47–56. |
[16] |
R. Murali, C. Park, A. Ponmana Selvan, Hyers–Ulam stability for an nth order differential equation using fixed point approach, J. Appl. Anal. Comput., 11 (2021), 614–631. https://doi.org/10.11948/20190093 doi: 10.11948/20190093
![]() |
[17] |
S. Öğrekçi, Y. Başcı, A. Mısır, A fixed point method for stability of nonlinear Volterra integral equations in the sense of Ulam, Math. Methods Appl. Sci., 46 (2023), 8437–8444. https://doi.org/10.1002/mma.8988 doi: 10.1002/mma.8988
![]() |
[18] |
A. Reinfelds, D. Šteinberga, Bounded solutions and Hyers–Ulam stability of quasilinear dynamic equations on time scales, Nonlinear Anal. Model. Control, 28 (2023), 377–391. https://doi.org/10.15388/namc.2023.28.31603 doi: 10.15388/namc.2023.28.31603
![]() |
[19] | I. A. Rus, Ulam stability of ordinary differential equations, Stud. Univ. Babeş-Bolyai Math., 54 (2009), 125–133. |
[20] | I. A. Rus, Ulam stabilities of ordinary differential equations in a Banach space, Carpathian J. Math., 26 (2010), 103–107. |
[21] |
P. Scindia, S. Tikare, A. A. El-Deeb, Ulam stability of first-order nonlinear impulsive dynamic equations, Boundary Value Probl., 2023 (2023), 86. https://doi.org/10.1186/s13661-023-01752-z doi: 10.1186/s13661-023-01752-z
![]() |
[22] |
H. M. Srivastava, A. K. Nain, R. K. Vats, P. Das, A theoretical study of the fractional-order p-Laplacian nonlinear Hadamard type turbulent flow models having the Ulam-Hyers stability, Rev. R. Acad. Cienc. Exactas, Fís. Nat. Ser. A Mat., 117 (2023), 160. https://doi.org/10.1007/s13398-023-01488-6 doi: 10.1007/s13398-023-01488-6
![]() |
[23] |
O. Tunç, C. Tunç, Ulam stabilities of nonlinear iterative integro-differential equations, Rev. R. Acad. Cienc. Exactas, Fís. Nat. Ser. A Mat., 117 (2023), 118. https://doi.org/10.1007/s13398-023-01450-6 doi: 10.1007/s13398-023-01450-6
![]() |
[24] |
L. Backes, D. Dragičević, A general approach to nonautonomous shadowing for nonlinear dynamics, Bull. Sci. Math., 170 (2021), 102996. https://doi.org/10.1016/j.bulsci.2021.102996 doi: 10.1016/j.bulsci.2021.102996
![]() |
[25] |
L. Backes, D. Dragičević, M. Onitsuka, M. Pituk, Conditional Lipschitz shadowing for ordinary differential equations, J. Dyn. Differ. Equations, 2023 (2023), 1–18. https://doi.org/10.1007/s10884-023-10246-6 doi: 10.1007/s10884-023-10246-6
![]() |
[26] |
D. Popa, I. Raşa, A. Viorel, Approximate solutions of the logistic equation and Ulam stability, Appl. Math. Lett., 85 (2018), 64–69. https://doi.org/10.1016/j.aml.2018.05.018 doi: 10.1016/j.aml.2018.05.018
![]() |
[27] |
M. Onitsuka, Conditional Ulam stability and its application to the logistic model, Appl. Math. Lett., 122 (2021), 107565. https://doi.org/10.1016/j.aml.2021.107565 doi: 10.1016/j.aml.2021.107565
![]() |
[28] |
M. Onitsuka, Conditional Ulam stability and its application to von Bertalanffy growth model, Math. Biosci. Eng., 19 (2022), 2819–2834. https://doi.org/10.3934/mbe.2022129 doi: 10.3934/mbe.2022129
![]() |
1. | Krzysztof Ciepliński, On Ulam Stability of Functional Equations in Non-Archimedean Spaces, 2025, 17, 2073-8994, 112, 10.3390/sym17010112 |