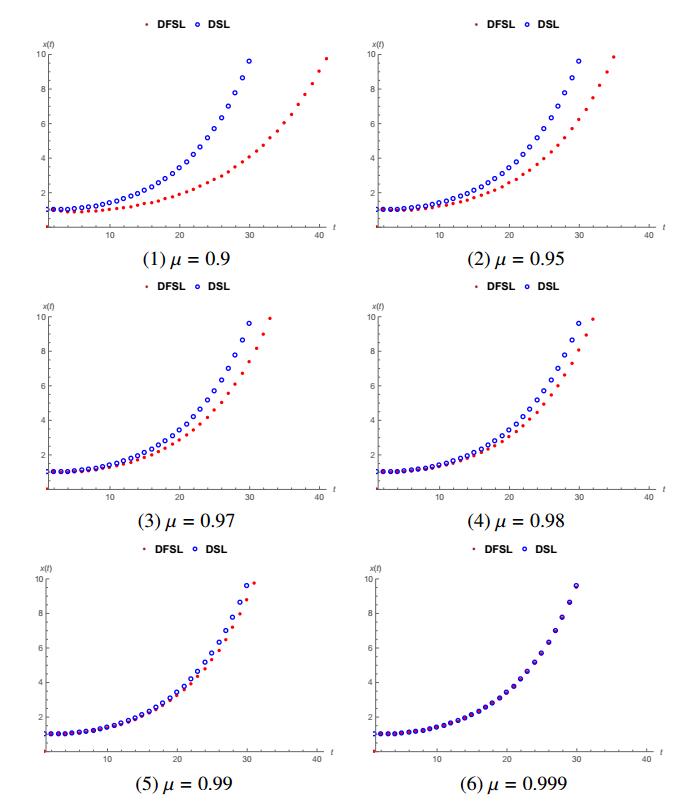
Jaw cysts are mainly caused by abnormal tooth development, chronic oral inflammation, or jaw damage, which may lead to facial swelling, deformity, tooth loss, and other symptoms. Due to the diversity and complexity of cyst images, deep-learning algorithms still face many difficulties and challenges. In response to these problems, we present a horizontal-vertical interaction and multiple side-outputs network for cyst segmentation in jaw images. First, the horizontal-vertical interaction mechanism facilitates complex communication paths in the vertical and horizontal dimensions, and it has the ability to capture a wide range of context dependencies. Second, the feature-fused unit is introduced to adjust the network's receptive field, which enhances the ability of acquiring multi-scale context information. Third, the multiple side-outputs strategy intelligently combines feature maps to generate more accurate and detailed change maps. Finally, experiments were carried out on the self-established jaw cyst dataset and compared with different specialist physicians to evaluate its clinical usability. The research results indicate that the Matthews correlation coefficient (Mcc), Dice, and Jaccard of HIMS-Net were 93.61, 93.66 and 88.10% respectively, which may contribute to rapid and accurate diagnosis in clinical practice.
Citation: Xiaoliang Jiang, Huixia Zheng, Zhenfei Yuan, Kun Lan, Yaoyang Wu. HIMS-Net: Horizontal-vertical interaction and multiple side-outputs network for cyst segmentation in jaw images[J]. Mathematical Biosciences and Engineering, 2024, 21(3): 4036-4055. doi: 10.3934/mbe.2024178
[1] | Ahmed M.A. El-Sayed, Eman M.A. Hamdallah, Hameda M. A. Alama . Multiple solutions of a Sturm-Liouville boundary value problem of nonlinear differential inclusion with nonlocal integral conditions. AIMS Mathematics, 2022, 7(6): 11150-11164. doi: 10.3934/math.2022624 |
[2] | Mukhamed Aleroev, Hedi Aleroeva, Temirkhan Aleroev . Proof of the completeness of the system of eigenfunctions for one boundary-value problem for the fractional differential equation. AIMS Mathematics, 2019, 4(3): 714-720. doi: 10.3934/math.2019.3.714 |
[3] | Tuba Gulsen, Sertac Goktas, Thabet Abdeljawad, Yusuf Gurefe . Sturm-Liouville problem in multiplicative fractional calculus. AIMS Mathematics, 2024, 9(8): 22794-22812. doi: 10.3934/math.20241109 |
[4] | Youyu Wang, Lu Zhang, Yang Zhang . Lyapunov-type inequalities for Hadamard fractional differential equation under Sturm-Liouville boundary conditions. AIMS Mathematics, 2021, 6(3): 2981-2995. doi: 10.3934/math.2021181 |
[5] | Youyu Wang, Xianfei Li, Yue Huang . The Green's function for Caputo fractional boundary value problem with a convection term. AIMS Mathematics, 2022, 7(4): 4887-4897. doi: 10.3934/math.2022272 |
[6] | Erdal Bas, Ramazan Ozarslan, Resat Yilmazer . Spectral structure and solution of fractional hydrogen atom difference equations. AIMS Mathematics, 2020, 5(2): 1359-1371. doi: 10.3934/math.2020093 |
[7] | Haifa Bin Jebreen, Beatriz Hernández-Jiménez . Pseudospectral method for fourth-order fractional Sturm-Liouville problems. AIMS Mathematics, 2024, 9(9): 26077-26091. doi: 10.3934/math.20241274 |
[8] | Zhongqian Wang, Xuejun Zhang, Mingliang Song . Three nonnegative solutions for Sturm-Liouville BVP and application to the complete Sturm-Liouville equations. AIMS Mathematics, 2023, 8(3): 6543-6558. doi: 10.3934/math.2023330 |
[9] | Zeliha Korpinar, Mustafa Inc, Dumitru Baleanu . On the fractional model of Fokker-Planck equations with two different operator. AIMS Mathematics, 2020, 5(1): 236-248. doi: 10.3934/math.2020015 |
[10] | Ndolane Sene . Fractional input stability for electrical circuits described by the Riemann-Liouville and the Caputo fractional derivatives. AIMS Mathematics, 2019, 4(1): 147-165. doi: 10.3934/Math.2019.1.147 |
Jaw cysts are mainly caused by abnormal tooth development, chronic oral inflammation, or jaw damage, which may lead to facial swelling, deformity, tooth loss, and other symptoms. Due to the diversity and complexity of cyst images, deep-learning algorithms still face many difficulties and challenges. In response to these problems, we present a horizontal-vertical interaction and multiple side-outputs network for cyst segmentation in jaw images. First, the horizontal-vertical interaction mechanism facilitates complex communication paths in the vertical and horizontal dimensions, and it has the ability to capture a wide range of context dependencies. Second, the feature-fused unit is introduced to adjust the network's receptive field, which enhances the ability of acquiring multi-scale context information. Third, the multiple side-outputs strategy intelligently combines feature maps to generate more accurate and detailed change maps. Finally, experiments were carried out on the self-established jaw cyst dataset and compared with different specialist physicians to evaluate its clinical usability. The research results indicate that the Matthews correlation coefficient (Mcc), Dice, and Jaccard of HIMS-Net were 93.61, 93.66 and 88.10% respectively, which may contribute to rapid and accurate diagnosis in clinical practice.
Fractional calculus is a notably attractive subject owing to having wide-ranging application areas of theoretical and applied sciences. Despite the fact that there are a large number of worthwhile mathematical works on the fractional differential calculus, there is no noteworthy parallel improvement of fractional difference calculus up to lately. This statement has shown that discrete fractional calculus has certain unforeseen hardship.
Fractional sums and differences were obtained firstly in Diaz-Osler [1], Miller-Ross [2] and Gray and Zhang [3] and they found discrete types of fractional integrals and derivatives. Later, several authors began to touch upon discrete fractional calculus; Goodrich-Peterson [4], Baleanu et al. [5], Ahrendt et al. [6]. Nevertheless, discrete fractional calculus is a rather novel area. The first studies have been done by Atıcı et al. [7,8,9,10,11], Abdeljawad et al. [12,13,14], Mozyrska et al. [15,16,17], Anastassiou [18,19], Hein et al. [20] and Cheng et al. [21] and so forth [22,23,24,25,26].
Self-adjoint operators have an important place in differential operators. Levitan and Sargsian [27] studied self-adjoint Sturm-Liouville differential operators and they obtained spectral properties based on self-adjointness. Also, they found representation of solutions and hence they obtained asymptotic formulas of eigenfunctions and eigenvalues. Similarly, Dehghan and Mingarelli [28,29] obtained for the first time representation of solution of fractional Sturm-Liouville problem and they obtained asymptotic formulas of eigenfunctions and eigenvalues of the problem. In this study, firstly we obtain self-adjointness of DFSL operator within nabla fractional Riemann-Liouville and delta fractional Grünwald-Letnikov operators. From this point of view, we obtain orthogonality of distinct eigenfunctions, reality of eigenvalues. In addition, we open a new gate by obtaining representation of solution of DFSL problem for researchers study in this area.
Self-adjointness of fractional Sturm-Liouville differential operators have been proven by Bas et al. [30,31], Klimek et al. [32,33]. Variational properties of fractional Sturm-Liouville problem has been studied in [34,35]. However, self-adjointness of conformable Sturm-Liouville and DFSL with Caputo-Fabrizio operator has been proven by [36,37]. Nowadays, several studies related to Atangana-Baleanu fractional derivative and its discrete version are done [38,39,40,41,42,43,44,45].
In this study, we consider DFSL operators within Riemann-Liouville and Grünwald-Letnikov sense, and we prove the self-adjointness, orthogonality of distinct eigenfunctions, reality of eigenvalues of DFSL operator. However, we get sum representation of solutions for DFSL equation by means Laplace transform for nabla fractional difference equations. Finally, we compare the results for the solution of DFSL problem, discrete Sturm-Liouville (DSL) problem with the second order, fractional Sturm-Liouville (FSL) problem and classical Sturm-Liouville (CSL) problem with the second order. The aim of this paper is to contribute to the theory of DFSL operator.
We discuss DFSL equations in three different ways with;
i) Self-adjoint (nabla left and right) Riemann-Liouville (R-L) fractional operator,
L1x(t)=∇μa(p(t)b∇μx(t))+q(t)x(t)=λr(t)x(t), 0<μ<1, |
ii) Self-adjoint (delta left and right) Grünwald-Letnikov (G-L) fractional operator,
L2x(t)=Δμ−(p(t)Δμ+x(t))+q(t)x(t)=λr(t)x(t), 0<μ<1, |
iii)(nabla left) DFSL operator is defined by R-L fractional operator,
L3x(t)=∇μa(∇μax(t))+q(t)x(t)=λx(t), 0<μ<1. |
Definition 2.1. [4] Delta and nabla difference operators are defined by respectively
Δx(t)=x(t+1)−x(t),∇x(t)=x(t)−x(t−1). | (1) |
Definition 2.2. [46] Falling function is defined by, α∈R
tα_=Γ(α+1)Γ(α+1−n), | (2) |
where Γ is Euler gamma function.
Definition 2.3. [46] Rising function is defined by, α∈R,
t¯α=Γ(t+α)Γ(t). | (3) |
Remark 1. Delta and nabla operators have the following properties
Δtα_=αtα−1_, | (4) |
∇t¯α=αt¯α−1. |
Definition 2.4. [2,7] Fractional sum operators are defined by,
(i) The left defined nabla fractional sum with order μ>0 is defined by
∇−μax(t)=1Γ(μ)t∑s=a+1(t−ρ(s))¯μ−1x(s), t∈Na+1, | (5) |
(ii) The right defined nabla fractional sum with order μ>0 is defined by
b∇−μx(t)=1Γ(μ)b−1∑s=t(s−ρ(t))¯μ−1x(s), t∈ b−1N, | (6) |
where ρ(t)=t−1 is called backward jump operators, Na={a,a+1,...}, bN={b,b−1,...}.
Definition 2.5. [47] Fractional difference operators are defined by,
(i) The nabla left fractional difference of order μ>0 is defined
∇μax(t)=∇n∇−(n−μ)ax(t)=∇nΓ(n−μ)t∑s=a+1(t−ρ(s))¯n−μ−1x(s), t∈Na+1, | (7) |
(ii) The nabla right fractional difference of order μ>0 is defined
b∇μx(t)=(−1)nΔn b∇−(n−μ)x(t)=(−1)nΔnΓ(n−μ)b−1∑s=t(s−ρ(t))¯n−μ−1x(s), t∈ b−1N. | (8) |
Fractional differences in (7−8) are called the Riemann-Liouville (R-L) definition of the μ-th order nabla fractional difference.
Definition 2.6. [1,21,48] Fractional difference operators are defined by,
(i) The left defined delta fractional difference of order μ, 0<μ≤1, is defined by
Δμ−x(t)=1hμt∑s=0(−1)sμ(μ−1)...(μ−s+1)s!x(t−s), t=1,...,N. | (9) |
(ii) The right defined delta fractional difference of order μ, 0<μ≤1, is defined by
Δμ+x(t)=1hμN−t∑s=0(−1)sμ(μ−1)...(μ−s+1)s!x(t+s), t=0,..,N−1. | (10) |
Fractional differences in (9−10) are called the Grünwald-Letnikov (G-L) definition of the μ-th order delta fractional difference.
Theorem 2.7. [47] We define the summation by parts formula for R-L fractional nabla difference operator, u is defined on bN and v is defined on Na, then
b−1∑s=a+1u(s)∇μav(s)=b−1∑s=a+1v(s)b∇μu(s). | (11) |
Theorem 2.8. [26,48] We define the summation by parts formula for G-L delta fractional difference operator, u, v is defined on {0,1,...,n}, then
n∑s=0u(s)Δμ−v(s)=n∑s=0v(s)Δμ+u(s). | (12) |
Definition 2.9. [20] f:Na→R, s∈ℜ, Laplace transform is defined as follows,
La{f}(s)=∞∑k=1(1−s)k−1f(a+k), |
where ℜ=C∖{1} and ℜ is called the set of regressive (complex) functions.
Definition 2.10. [20] Let f,g:Na→R, all t∈Na+1, convolution property of f and g is given by
(f∗g)(t)=t∑s=a+1f(t−ρ(s)+a)g(s), |
where ρ(s) is the backward jump function defined in [46] as
ρ(s)=s−1. |
Theorem 2.11. [20] f,g:Na→R, convolution theorem is expressed as follows,
La{f∗g}(s)=La{f}La{g}(s). |
Lemma 2.12. [20] f:Na→R, the following property is valid,
La+1{f}(s)=11−sLa{f}(s)−11−sf(a+1). |
Theorem 2.13. [20] f:Na→R, 0<μ<1, Laplace transform of nabla fractional difference
La+1{∇μaf}(s)=sμLa+1{f}(s)−1−sμ1−sf(a+1),t∈Na+1. |
Definition 2.14. [20] For |p|<1, α>0, β∈R and t∈Na, discrete Mittag-Leffler function is defined by
Ep,α,β(t,a)=∞∑k=0pk(t−a)¯αk+βΓ(αk+β+1), |
where t¯n={t(t+1)⋯(t+n−1),n∈ZΓ(t+n)Γ(t),n∈R is rising factorial function.
Theorem 2.15. [20] For |p|<1, α>0, β∈R, |1−s|<1, and |s|α>p, Laplace transform of discrete Mittag-Leffler function is as follows,
La{Ep,α,β(.,a)}(s)=sα−β−1sα−p. |
Definition 2.16. Laplace transform of f(t)∈R+, t≥0 is defined as follows,
L{f}(s)=∞∫0e−stf(t)dt. |
Theorem 2.17. For z, θ∈C,Re(δ)>0, Mittag-Leffler function with two parameters is defined as follows
Eδ,θ(z)=∞∑k=0zkΓ(δk+θ). |
Theorem 2.18. Laplace transform of Mittag-Leffler function is as follows
L{tθ−1Eδ,θ(λtδ)}(s)=sδ−θsδ−λ. |
Property 2.19. [28] f:Na→R, 0<μ<1, Laplace transform of fractional derivative in Caputo sense is as follows, 0<α<1,
L{CDα0+f}(s)=sαL{f}(s)−sα−1f(0). |
Property 2.20. [28] f:Na→R, 0<μ<1, Laplace transform of left fractional derivative in Riemann-Liouville sense is as follows, 0<α<1,
L{Dα0+f}(s)=sαL{f}(s)−I1−α0+f(t)|t=0, |
here Iα0+ is left fractional integral in Riemann-Liouville sense.
We consider discrete fractional Sturm-Liouville equations in three different ways as follows:
First Case: Self-adjoint L1 DFSL operator is defined by (nabla right and left) R-L fractional operator,
L1x(t)=∇μa(p(t)b∇μx(t))+q(t)x(t)=λr(t)x(t), 0<μ<1, | (13) |
where p(t)>0, r(t)>0, q(t) is a real valued function on [a+1,b−1] and real valued, λ is the spectral parameter, t∈[a+1,b−1], x(t)∈l2[a+1,b−1]. In ℓ2(a+1,b−1), the Hilbert space of sequences of complex numbers u(a+1),...,u(b−1) with the inner product is given by,
⟨u(n),v(n)⟩=b−1∑n=a+1u(n)v(n), |
for every u∈DL1, let's define as follows
DL1={u(n), v(n)∈ℓ2(a+1,b−1):L1u(n), L1v(n)∈ℓ2(a+1,b−1)}. |
Second Case: Self-adjoint L2 DFSL operator is defined by(delta left and right) G-L fractional operator,
L2x(t)=Δμ−(p(t)Δμ+x(t))+q(t)x(t)=λr(t)x(t), 0<μ<1, | (14) |
where p,r,λ is as defined above, q(t) is a real valued function on [0,n], t∈[0,n], x(t)∈l2[0,n]. In ℓ2(0,n), the Hilbert space of sequences of complex numbers u(0),...,u(n) with the inner product is given by, n is a finite integer,
⟨u(i),r(i)⟩=n∑i=0u(i)r(i), |
for every u∈DL2, let's define as follows
DL2={u(i), v(i)∈ℓ2(0,n):L2u(n), L2r(n)∈ℓ2(0,n)}. |
Third Case:L3 DFSL operator is defined by (nabla left) R-L fractional operator,
L3x(t)=∇μa(∇μax(t))+q(t)x(t)=λx(t), 0<μ<1, | (15) |
p,r,λ is as defined above, q(t) is a real valued function on [a+1,b−1], t∈[a+1,b−1].
Firstly, we consider the first case and give the following theorems and proofs;
Theorem 3.1. DFSL operator L1 is self-adjoint.
Proof.
u(t)L1v(t)=u(t)∇μa(p(t)b∇μv(t))+u(t)q(t)v(t), | (16) |
v(t)L1u(t)=v(t)∇μa(p(t)b∇μu(t))+v(t)q(t)u(t). | (17) |
If (16−17) is subtracted from each other
u(t)L1v(t)−v(t)L1u(t)=u(t)∇μa(p(t)b∇μv(t))−v(t)∇μa(p(t)b∇μu(t)) |
and sum operator from a+1 to b−1 to both side of the last equality is applied, we get
b−1∑s=a+1(u(s)L1v(s)−v(s)L1u(s))=b−1∑s=a+1u(s)∇μa(p(s)b∇μv(s)) | (18) |
−b−1∑s=a+1v(s)∇μa(p(s)b∇μu(s)). |
If we apply the summation by parts formula in (11) to right hand side of (18), we have
b−1∑s=a+1(u(s)L1v(s)−v(s)L1u(s))=b−1∑s=a+1p(s)b∇μv(s)b∇μu(s)−b−1∑s=a+1p(s)b∇μu(s)b∇μv(s)=0, |
⟨L1u,v⟩=⟨u,L1v⟩. |
Hence, the proof completes.
Theorem 3.2. Two eigenfunctions, u(t,λα) and v(t,λβ), of the equation (13) are orthogonal as λα≠λβ.
Proof. Let λα and λβ are two different eigenvalues corresponds to eigenfunctions u(t) and v(t) respectively for the the equation (13),
∇μa(p(t)b∇μu(t))+q(t)u(t)−λαr(t)u(t)=0,∇μa(p(t)b∇μv(t))+q(t)v(t)−λβr(t)v(t)=0. |
If we multiply last two equations by v(t) and u(t) respectively, subtract from each other and apply definite sum operator, owing to the self-adjointness of the operator L1, we have
(λα−λβ)b−1∑s=a+1r(s)u(s)v(s)=0, |
since λα≠λβ,
b−1∑s=a+1r(s)u(s)v(s)=0,⟨u(t),v(t)⟩=0. |
Hence, the proof completes.
Theorem 3.3. All eigenvalues of the equation (13) are real.
Proof. Let λ=α+iβ, owing to the self-adjointness of the operator L1, we can write
⟨L1u(t),u(t)⟩=⟨u(t),L1u(t)⟩,⟨λru(t),u(t)⟩=⟨u(t),λr(t)u(t)⟩, |
(λ−¯λ)⟨u(t),u(t)⟩r=0. |
Since ⟨u(t),u(t)⟩r≠0,
λ=¯λ |
and hence β=0. The proof completes.
Secondly, we consider the second case and give the following theorems and proofs;
Theorem 3.4. DFSL operator L2 is self-adjoint.
Proof.
u(t)L2v(t)=u(t)Δμ−(p(t)Δμ+v(t))+u(t)q(t)v(t), | (19) |
v(t)L2u(t)=v(t)Δμ−(p(t)Δμ+u(t))+v(t)q(t)u(t). | (20) |
If (19−20) is subtracted from each other
u(t)L2v(t)−v(t)L2u(t)=u(t)Δμ−(p(t)Δμ+v(t))−v(t)Δμ−(p(t)Δμ+u(t)) |
and definite sum operator from 0 to t to both side of the last equality is applied, we have
t∑s=0(u(s)L1v(s)−v(s)L2u(s))=t∑s=0u(s)Δμ−(p(s)Δμ+v(s))−t∑s=0v(s)Δμ−(p(s)Δμ+u(s)). | (21) |
If we apply the summation by parts formula in (12) to r.h.s. of (21), we get
t∑s=0(u(s)L2v(s)−v(s)L2u(s))=t∑s=0p(s)Δμ+v(s)Δμ+u(s)−t∑s=0p(s)Δμ+u(s)Δμ+v(s)=0, |
⟨L2u,v⟩=⟨u,L2v⟩. |
Hence, the proof completes.
Theorem 3.5. Two eigenfunctions, u(t,λα) and v(t,λβ), of the equation (14) are orthogonal as λα≠λβ. orthogonal.
Proof. Let λα and λβ are two different eigenvalues corresponds to eigenfunctions u(t) and v(t) respectively for the the equation (14),
Δμ−(p(t)Δμ+u(t))+q(t)u(t)−λαr(t)u(t)=0,Δμ−(p(t)Δμ+v(t))+q(t)v(t)−λβr(t)v(t)=0. |
If we multiply last two equations to v(t) and u(t) respectively, subtract from each other and apply definite sum operator, owing to the self-adjointness of the operator L2, we get
(λα−λβ)t∑s=0r(s)u(s)v(s)=0, |
since λα≠λβ,
t∑s=0r(s)u(s)v(s)=0⟨u(t),v(t)⟩=0. |
So, the eigenfunctions are orthogonal. The proof completes.
Theorem 3.6. All eigenvalues of the equation (14) are real.
Proof. Let λ=α+iβ, owing to the self-adjointness of the operator L2
⟨L2u(t),u(t)⟩=⟨u(t),L2u(t)⟩,⟨λr(t)u(t),u(t)⟩=⟨u(t),λr(t)u(t)⟩, |
(λ−¯λ)⟨u,u⟩r=0. |
Since ⟨u,u⟩r≠0,
λ=¯λ, |
and hence β=0. The proof completes.
Now, we consider the third case and give the following theorem and proof;
Theorem 3.7.
L3x(t)=∇μa(∇μax(t))+q(t)x(t)=λx(t),0<μ<1, | (22) |
x(a+1)=c1,∇μax(a+1)=c2, | (23) |
where p(t)>0, r(t)>0, q(t) is defined and real valued, λ is the spectral parameter. The sum representation of solution of the problem (22)−(23) is found as follows,
x(t)=c1[(1+q(a+1))Eλ,2μ,μ−1(t,a)−λEλ,2μ,2μ−1(t,a)] | (24) |
+c2[Eλ,2μ,2μ−1(t,a)−Eλ,2μ,μ−1(t,a)]−t∑s=a+1Eλ,2μ,2μ−1(t−ρ(s)+a)q(s)x(s), |
where |λ|<1, |1−s|<1, and |s|α>λ from Theorem 2.15.
Proof. Let's use the Laplace transform of both side of the equation (22) by Theorem 2.13, and let q(t)x(t)=g(t),
La+1{∇μa(∇μax)}(s)+La+1{g}(s)=λLa+1{x}(s),=sμLa+1{∇μax}(s)−1−sμ1−sc2=λLa+1{x}(s)−La+1{g}(s),=sμ(sμLa+1{x}(s)−1−sμ1−sc1)−1−sμ1−sc2=λLa+1{x}(s)−La+1{g}(s), |
=La+1{x}(s)=1−sμ1−s1s2μ−λ(sμc1+c2)−1s2μ−λLa+1{g}(s), |
from Lemma 2.12, we get
La{x}(s)=c1(sμ−λs2μ−λ)−1−ss2μ−λ(11−sLa{g}(s)−11−sg(a+1))+c2(1−sμs2μ−λ). | (25) |
Applying inverse Laplace transform to the equation (25), then we get representation of solution of the problem (22)−(23),
x(t)=c1((1+q(a+1))Eλ,2μ,μ−1(t,a)−λEλ,2μ,2μ−1(t,a))+c2(Eλ,2μ,2μ−1(t,a)−Eλ,2μ,μ−1(t,a))−t∑s=a+1Eλ,2μ,2μ−1(t−ρ(s)+a)q(s)x(s). |
Now, let us consider comparatively discrete fractional Sturm-Liouville (DFSL) problem, discrete Sturm-Liouville (DSL) problem, fractional Sturm-Liouville (FSL) problem and classical Sturm-Liouville (CSL) problem respectively as follows by taking q(t)=0,
DFSL problem:
∇μ0(∇μ0x(t))=λx(t), | (26) |
x(1)=1, ∇μax(1)=0, | (27) |
and its analytic solution is as follows by the help of Laplace transform in Lemma 2.12
x(t)=Eλ,2μ,μ−1(t,0)−λEλ,2μ,2μ−1(t,0), | (28) |
DSL problem:
∇2x(t)=λx(t), | (29) |
x(1)=1, ∇x(1)=0, | (30) |
and its analytic solution is as follows
x(t)=12(1−λ)−t[(1−√λ)t(1+√λ)−(−1+√λ)(1+√λ)t], | (31) |
FSL problem:
CDμ0+(Dμ0+x(t))=λx(t), | (32) |
I1−μ0+x(t)|t=0=1, Dμ0+x(t)|t=0=0, | (33) |
and its analytic solution is as follows by the help of Laplace transform in Property 2.19 and 2.20
x(t)=tμ−1E2μ,μ(λt2μ), | (34) |
CSL problem:
x′′(t)=λx(t), | (35) |
x(0)=1, x′(0)=0, | (36) |
and its analytic solution is as follows
x(t)=cosht√λ, | (37) |
where the domain and range of function x(t) and Mittag-Leffler functions must be well defined. Note that we may show the solution of CSL problem can be obtained by taking μ→1 in the solution of FSL problem and similarly, the solution of DSL problem can be obtained by taking μ→1 in the solution of DFSL problem.
Firstly, we compare the solutions of DFSL and DSL problems and from here we show that the solutions of DFSL problem converge to the solutions of DSL problem as μ→1 in Figure 1 for discrete Mittag-Leffler function Ep,α,β(t,a)=1000∑k=0pk(t−a)¯αk+βΓ(αk+β+1); let λ=0.01,
Secondly, we compare the solutions of DFSL, DSL, FSL and CSL problems for discrete Mittag-Leffler function Ep,α,β(t,a)=1000∑k=0pk(t−a)¯αk+βΓ(αk+β+1). At first view, we observe the solution of DSL and CSL problems almost coincide in any order μ, and we observe the solutions of DFSL and FSL problem almost coincide in any order μ. However, we observe that all of the solutions of DFSL, DSL, FSL and CSL problems almost coincide to each other as μ→1 in Figure 2. Let λ=0.01,
Thirdly, we compare the solutions of DFSL problem (22−23) with different orders, different potential functions and different eigenvalues for discrete Mittag-Leffler function Ep,α,β(t,a)=1000∑k=0pk(t−a)¯αk+βΓ(αk+β+1) in the Figure 3;
Eigenvalues of DFSL problem (22−23), correspond to some specific eigenfunctions for numerical values of discrete Mittag-Leffler function Ep,α,β(t,a)=i∑k=0pk(t−a)¯αk+βΓ(αk+β+1), is given with different orders while q(t)=0 in Table 1;
i | λ1,i | λ2,i | λ3,i | λ1,i | λ2,i | λ3,i | λ1,i | λ2,i | λ3,i |
750 | −0.992 | −0.982 | −0.057 | −0.986 | −0.941 | −0.027 | −0.483 | −0.483 | 0 |
1000 | −0.989 | −0.977 | −0.057 | −0.990 | −0.954 | −0.027 | −0.559 | −0.435 | 0 |
2000 | −0.996 | −0.990 | −0.057 | −0.995 | −0.978 | −0.027 | −0.654 | −0.435 | 0 |
x(5),μ=0.5 | x(10),μ=0.9 | x(2000),μ=0.1 | |||||||
i | λ1,i | λ2,i | λ3,i | λ1,i | λ2,i | λ3,i | λ1,i | λ2,i | λ3,i |
750 | −0.951 | −0.004 | 0 | −0.868 | −0.793 | −0.0003 | −0.190 | −3.290×10−6 | 0 |
1000 | −0.963 | −0.004 | 0 | −0.898 | −0.828 | −0.0003 | −0.394 | −3.290×10−6 | 0 |
2000 | −0.981 | −0.004 | 0 | −0.947 | −0.828 | −0.0003 | −0.548 | −3.290×10−6 | 0 |
x(20),μ=0.5 | x(100),μ=0.9 | x(1000),μ=0.7 | |||||||
i | λ1,i | λ2,i | λ3,i | λ1,i | λ2,i | λ3,i | λ1,i | λ2,i | λ3,i |
750 | −0.414 | −9.59×10−7 | 0 | −0.853 | −0.0003 | 0 | −0.330 | −4.140×10−6 | 0 |
1000 | −0.478 | −9.59×10−7 | 0 | −0.887 | −0.0003 | 0 | −0.375 | −4.140×10−6 | 0 |
2000 | −0.544 | −9.59×10−7 | 0 | −0.940 | −0.0003 | 0 | −0.361 | −4.140×10−6 | 0 |
x(1000),μ=0.3 | x(100),μ=0.8 | x(1000),μ=0.9 | |||||||
i | λ1,i | λ2,i | λ3,i | λ1,i | λ2,i | λ3,i | λ1,i | λ2,i | λ3,i |
750 | −0.303 | −3.894×10−6 | 0 | −0.192 | −0.066 | 0 | −0.985 | −0.955 | −0.026 |
1000 | −0.335 | −3.894×10−6 | 0 | −0.197 | −0.066 | 0 | −0.989 | −0.941 | −0.026 |
2000 | −0.399 | −3.894×10−6 | 0 | −0.289 | −0.066 | 0 | −0.994 | −0.918 | −0.026 |
x(1000),μ=0.8 | x(2000),μ=0.6 | x(10),μ=0.83 |
Finally, we give the solutions of DFSL problem (22−23) with different orders, different potential functions and different eigenvalues for discrete Mittag-Leffler function Ep,α,β(t,a)=100∑k=0pk(t−a)¯αk+βΓ(αk+β+1) in Tables 2–4;
x(t) | μ=0.1 | μ=0.2 | μ=0.5 | μ=0.7 | μ=0.9 |
x(1) | 1 | 1 | 1 | 1 | 1 |
x(2) | 0.125 | 0.25 | 0.625 | 0.875 | 1.125 |
x(3) | 0.075 | 0.174 | 0.624 | 1.050 | 1.575 |
x(5) | 0.045 | 0.128 | 0.830 | 1.968 | 4.000 |
x(7) | 0.0336 | 0.111 | 1.228 | 4.079 | 11.203 |
x(9) | 0.0274 | 0.103 | 1.878 | 8.657 | 31.941 |
x(12) | 0.022 | 0.098 | 3.622 | 27.05 | 154.56 |
x(15) | 0.0187 | 0.0962 | 7.045 | 84.75 | 748.56 |
x(16) | 0.0178 | 0.0961 | 8.800 | 124.04 | 1266.5 |
x(18) | 0.0164 | 0.0964 | 13.737 | 265.70 | 3625.6 |
x(20) | 0.0152 | 0.0972 | 21.455 | 569.16 | 10378.8 |
x(t) | q(t)=1 | q(t)=t | q(t)=√t |
x(1) | 1 | 1 | 1 |
x(2) | 0.2261 | 0.1505 | 0.1871 |
x(3) | 0.1138 | 0.0481 | 0.0767 |
x(5) | 0.0518 | 0.0110 | 0.0252 |
x(7) | 0.0318 | 0.0043 | 0.0123 |
x(9) | 0.0223 | 0.0021 | 0.0072 |
x(12) | 0.0150 | 0.0010 | 0.0039 |
x(15) | 0.0110 | 0.0005 | 0.0025 |
x(16) | 0.0101 | 0.0004 | 0.0022 |
x(18) | 0.0086 | 0.0003 | 0.0017 |
x(20) | 0.0075 | 0.0002 | 0.0014 |
x(t) | q(t)=1 | q(t)=t | q(t)=√t |
x(1) | 1 | 1 | 1 |
x(2) | 0.2261 | 0.1505 | 0.1871 |
x(3) | 0.1138 | 0.0481 | 0.0767 |
x(5) | 0.0518 | 0.0110 | 0.0252 |
x(7) | 0.0318 | 0.0043 | 0.0123 |
x(9) | 0.0223 | 0.0021 | 0.0072 |
x(12) | 0.0150 | 0.0010 | 0.0039 |
x(15) | 0.0110 | 0.0005 | 0.0025 |
x(16) | 0.0101 | 0.0004 | 0.0022 |
x(18) | 0.0086 | 0.0003 | 0.0017 |
x(20) | 0.0075 | 0.0002 | 0.0014 |
Now, let's consider the problems together DFSL (26)−(27), DSL (29)−(30), FSL (32)−(33) and CSL (35)−(36). Eigenvalues of these problems are the roots of the following equation
x(35)=0. |
Thus, if we apply the solutions (28), (31), (34) and (37) of these four problems to the equation above respectively, we can find the eigenvalues of these problems for the orders μ=0.9 and μ=0.99 respectively in Table 5, and Table 6,
λ1 | λ2 | λ3 | λ4 | λ5 | λ6 | λ7 | λ8 | λ9 | λ10 | |
DFSL | −0.904 | −0.859 | −0.811 | −0.262 | −0.157 | −0.079 | −0.029 | −0.003 | 0.982 | |
FSL | −0.497 | −0.383 | −0.283 | −0.196 | −0.124 | −0.066 | −0.026 | −0.003 | 0 | ... |
DSL | −1.450 | −0.689 | −0.469 | −0.310 | −0.194 | −0.112 | −0.055 | −0.019 | −0.002 | |
CSL | −0.163 | −0.128 | −0.098 | −0.072 | −0.050 | −0.032 | −0.008 | −0.002 | 0 |
λ1 | λ2 | λ3 | λ4 | λ5 | λ6 | λ7 | λ8 | λ9 | λ10 | |
DFSL | −0.866 | −0.813 | −0.200 | −0.115 | −0.057 | −0.020 | −0.002 | 0 | 0.982 | |
FSL | −0.456 | −0.343 | −0.246 | −0.165 | −0.100 | −0.051 | −0.018 | −0.002 | 0 | ... |
DSL | −1.450 | −0.689 | −0.469 | −0.310 | −0.194 | −0.112 | −0.055 | −0.019 | −0.002 | ... |
CSL | −0.163 | −0.128 | −0.098 | −0.072 | −0.050 | −0.032 | −0.008 | −0.002 | 0 |
In here, we observe that these four problems have real eigenvalues under different orders μ=0.9 and μ=0.99, hence we can find eigenfunctions putting these eigenvalues into the four solutions. Furthermore, as the order changes, we can see that eigenvalues change for DFSL problems.
We consider firstly discrete fractional Sturm-Liouville (DFSL) operators with nabla Riemann-Liouville and delta Grünwald-Letnikov fractional operators and we prove self-adjointness of the DFSL operator and fundamental spectral properties. However, we analyze DFSL problem, discrete Sturm-Liouville (DSL) problem, fractional Sturm-Liouville (FSL) problem and classical Sturm-Liouville (CSL) problem by taking q(t)=0 in applications. Firstly, we compare the solutions of DFSL and DSL problems and we observe that the solutions of DFSL problem converge to the solutions of DSL problem when μ→1 in Fig. 1. Secondly, we compare the solutions of DFSL, DSL, FSL and CSL problems in Fig. 2. At first view, we observe the solutions of DSL and CSL problems almost coincide with any order μ, and we observe the solutions of DFSL and FSL problem almost coincide with any order μ. However, we observe that all of solutions of DFSL, DSL, FSL and CSL problems almost coincide with each other as μ→1. Thirdly, we compare the solutions of DFSL problem (22−23) with different orders, different potential functions and different eigenvalues in Fig. 3.
Eigenvalues of DFSL problem (22−23) corresponded to some specific eigenfunctions is given with different orders in Table 1. We give the eigenfunctions of DFSL problem (22−23) with different orders, different potential functions and different eigenvalues in Table 2, Table 3 and Table 4.
In Section 4.1, we consider DFSL, DSL, FSL and CSL problems together and thus, we can compare the eigenvalues of these four problems in Table 5 and Table 6 for different values of μ. We observe that these four problems have real eigenvalues under different values of μ, from here we can find eigenfunctions corresponding eigenvalues. Moreover, when the order change, eigenvalues change for DFSL problems.
Consequently, important results in spectral theory are given for discrete Sturm-Liouville problems. These results will lead to open gates for the researchers studied in this area. Especially, representation of solution will be practicable for future studies. It worths noting that visual results both will enable to be understood clearly by readers and verify the results to the integer order discrete case while the order approaches to one.
This paper includes a part of Ph.D. thesis data of Ramazan OZARSLAN.
The authors declare no conflict of interest.
[1] |
P. Wang, J. Z. Peng, M. Pedersoli, Y. F. Zhou, C. M. Zhang, C. Desrosiers, CAT: Constrained adversarial training for anatomically-plausible semi-supervised segmentation, IEEE Trans. Med. Imaging, 42 (2023), 2146–2161. https://doi.org/10.1109/TMI.2023.3243069 doi: 10.1109/TMI.2023.3243069
![]() |
[2] |
L. Zhang, K. J. Zhang, H. W. Pan, SUNet plus plus: A deep network with channel attention for small-scale object segmentation on 3D medical images, Tsinghua Sci. Technol., 28 (2023), 628–638. https://doi.org/10.26599/TST.2022.9010023 doi: 10.26599/TST.2022.9010023
![]() |
[3] |
D. D. Meng, S. Li, B. Sheng, H. Wu, S. Q. Tian, W. J. Ma, et al., 3D reconstruction-oriented fully automatic multi-modal tumor segmentation by dual attention-guided VNet, Visual Comput., 39 (2023), 3183–3196. https://doi.org/10.1007/s00371-023-02965-0 doi: 10.1007/s00371-023-02965-0
![]() |
[4] |
Y. Feng, Y. H. Wang, H. H. Li, M. J. Qu, J. Z. Yang, Learning what and where to segment: A new perspective on medical image few-shot segmentation, Med. Image Anal., 87 (2023), 102834. https://doi.org/10.1016/j.media.2023.102834 doi: 10.1016/j.media.2023.102834
![]() |
[5] |
Y. X. Ma, S. Wang, Y. Hua, R. H. Ma, T. Song, Z. G. Xue, et al. Perceptual data augmentation for biomedical coronary vessel segmentation, IEEE/ACM Trans. Comput. Biol. Bioinf., 20 (2023), 2494–2505. https://doi.org/10.1109/TCBB.2022.3188148 doi: 10.1109/TCBB.2022.3188148
![]() |
[6] | O. Ronneberger, P. Fischer, T. Brox, U-net: Convolutional networks for biomedical image segmentation, in International Conference on Medical Image Computing and Computer-assisted Intervention, Munich, Germany, (2015), 234–241. https://doi.org/10.1007/978-3-319-24574-4_28 |
[7] |
A. Sharma, P. K. Mishra, DRI-UNet: dense residual-inception UNet for nuclei identification in microscopy cell images, Neural Comput. Appl., 35 (2023), 19187–19220. https://doi.org/10.1007/s00521-023-08729-0 doi: 10.1007/s00521-023-08729-0
![]() |
[8] |
B. Sarica, D. Z. Seker, B. Bayram, A dense residual U-net for multiple sclerosis lesions segmentation from multi-sequence 3D MR images, Int. J. Med. Inf., 170 (2023), 104965. https://doi.org/10.1016/j.ijmedinf.2022.104965 doi: 10.1016/j.ijmedinf.2022.104965
![]() |
[9] |
Q. Xu, Z. Ma, H. E. Na, W. Duan, DCSAU-Net: A deeper and more compact split-attention U-Net for medical image segmentation, Comput. Biol. Med., 154 (2023), 106626. https://doi.org/10.1016/j.compbiomed.2023.106626 doi: 10.1016/j.compbiomed.2023.106626
![]() |
[10] |
H. Wang, G. Xu, X. Pan, Z. Liu, N. Tang, R. Lan, et al., Attention-inception-based U-Net for retinal vessel segmentation with advanced residual, Comput. Electr. Eng., 98 (2022), 107670. https://doi.org/10.1016/j.compeleceng.2021.107670 doi: 10.1016/j.compeleceng.2021.107670
![]() |
[11] |
J. Zhang, Y. Zhang, Y. Jin, J. Xu, X. Xu, MDU-Net: multi-scale densely connected U-Net for biomedical image segmentation, Health Inf. Sci. Syst., 11 (2023), 13. https://doi.org/10.1007/s13755-022-00204-9 doi: 10.1007/s13755-022-00204-9
![]() |
[12] |
S. Banerjee, J. Lyu, Z. Huang, F. H. Leung, T. Lee, D. Yang, et al., Ultrasound spine image segmentation using multi-scale feature fusion skip-inception U-Net (SIU-Net), Biocybern. Biomed. Eng., 42 (2022), 341–361. https://doi.org/10.1016/j.bbe.2022.02.011 doi: 10.1016/j.bbe.2022.02.011
![]() |
[13] |
S. Wang, V. K. Singh, E. Cheah, X. Wang, Q. Li, S. H. Chou, et al., Stacked dilated convolutions and asymmetric architecture for U-Net-based medical image segmentation, Comput. Biol. Med., 148 (2022), 105891. https://doi.org/10.1016/j.compbiomed.2022.105891 doi: 10.1016/j.compbiomed.2022.105891
![]() |
[14] |
J. Mutaguchi, K. I. Morooka, S. Kobayashi, A. Umehara, S. Miyauchi, F. Kinoshita, et al., Artificial intelligence for segmentation of bladder tumor cystoscopic images performed by U-Net with dilated convolution, J. Endourol., 36 (2022), 827–834. https://doi.org/10.1089/end.2021.0483 doi: 10.1089/end.2021.0483
![]() |
[15] |
J. Vidal, J. C. Vilanova, R. Martí, A U-Net ensemble for breast lesion segmentation in DCE MRI, Comput. Biol. Med., 140 (2022), 105093. https://doi.org/10.1016/j.compbiomed.2021.105093 doi: 10.1016/j.compbiomed.2021.105093
![]() |
[16] |
K. Sun, Y. Xin, Y. Ma, M. Lou, Y. Qi, J. Zhu, ASU-Net: U-shape adaptive scale network for mass segmentation in mammograms, J. Intell. Fuzzy Syst., 42 (2022), 4205–4220. https://doi.org/10.3233/JIFS-210393 doi: 10.3233/JIFS-210393
![]() |
[17] |
F. Abdolali, R. A. Zoroofi, Y. Otake, Y. Sato, Automatic segmentation of maxillofacial cysts in cone beam CT images, Comput. Biol. Med., 72 (2016), 108–119. https://doi.org/10.1016/j.compbiomed.2016.03.014 doi: 10.1016/j.compbiomed.2016.03.014
![]() |
[18] |
M. K. Alsmadi, A hybrid Fuzzy C-means and Neutrosophic for jaw lesions segmentation, Ain Shams Eng. J., 9 (2018), 697–706. https://doi.org/10.1016/j.asej.2016.03.016 doi: 10.1016/j.asej.2016.03.016
![]() |
[19] | J. Hu, Z. Feng, Y. Mao, J. Lei, D. Yu, M. Song, A location constrained dual-branch network for reliable diagnosis of jaw tumors and cysts, in International Conference of Medical Image Computing and Computer Assisted Intervention, Strasbourg, France, (2021), 723–732. https://doi.org/10.1007/978-3-030-87234-2_68 |
[20] |
S. Sivasundaram, C. Pandian, Performance analysis of classification and segmentation of cysts in panoramic dental images using convolutional neural network architecture, Int. J. Imaging Syst. Technol., 31 (2021), 2214–2225. https://doi.org/10.1002/ima.22625 doi: 10.1002/ima.22625
![]() |
[21] |
D. K. Veena, A. Jatti, M. J. Vidya, R. Joshi, S. Gade, A novel approach towards automatic contour identification of jaw cysts from digital panoramic radiographs to improvise the treatment planning, Int. J. Biol. Biomed. Eng., 16 (2022), 1–8. https://doi.org/10.46300/91011.2022.16.1 doi: 10.46300/91011.2022.16.1
![]() |
[22] | Z. W. Zhou, M. M. R. Siddiquee, N. Tajbakhsh, J. M. Liang, UNet++: A nested U-Net architecture for medical image segmentation, in Deep Learning in Medical Image Analysis and Multimodal Learning for Clinical Decision Support, Granada, Spain, (2018), 3–11. https://doi.org/10.1007/978-3-030-00889-5_1 |
[23] |
Z. Zhou, M. M. R. Siddiquee, N. Tajbakhsh, J. Liang, UNet++: Redesigning Skip Connections to Exploit Multiscale Features in Image Segmentation, IEEE Trans. Med. Imaging, 39 (2020), 1856–1867. https://doi.org/10.1109/TMI.2019.2959609 doi: 10.1109/TMI.2019.2959609
![]() |
[24] | K. Sun, B. Xiao, D. Liu, J. Wang, Deep high-resolution representation learning for human pose estimation, in IEEE International Conference on Computer Vision & Pattern Recognition, Long Beach, CA, USA, (2019), 5686–5696. https://doi.org/10.1109/CVPR.2019.00584 |
[25] |
M. Zhao, Y. Wei, Y. Lu, K. K. L. Wong, A novel U-Net approach to segment the cardiac chamber in magnetic resonance images with ghost artifacts, Comput. Methods Programs Biomed., 196 (2020), 105623. https://doi.org/10.1016/j.cmpb.2020.105623 doi: 10.1016/j.cmpb.2020.105623
![]() |
[26] |
A. S. Mahmoud, S. A. Mohamed, R. A. El-Khoriby, H. M. AbdelSalam, I. A. El-Khodary, Oil spill identification based on dual attention UNet model using synthetic aperture radar images, J. Indian Soc. Remote Sens., 51 (2023), 121–133. https://doi.org/10.1007/s12524-022-01624-6 doi: 10.1007/s12524-022-01624-6
![]() |
[27] |
X. Xie, X. Pan, W. Zhang, J. An, A context hierarchical integrated network for medical image segmentation, Comput. Electr. Eng., 101 (2022), 108029. https://doi.org/10.1016/j.compeleceng.2022.108029 doi: 10.1016/j.compeleceng.2022.108029
![]() |
[28] |
L. Zhu, L. Zhang, W. Hu, H. Chen, H. Li, S. Wei, et al., A multi-task two-path deep learning system for predicting the invasiveness of craniopharyngioma, Comput. Meth. Prog. Bio., 216 (2022), 106651. https://doi.org/10.1016/j.cmpb.2022.106651 doi: 10.1016/j.cmpb.2022.106651
![]() |
[29] |
L. Zhang, Y. Liao, G. Wang, J. Chen, H. Wang, A multi-scale contextual information enhancement network for crack segmentation, Appl. Sci., 12 (2022), 11135. https://doi.org/10.3390/app122111135 doi: 10.3390/app122111135
![]() |
[30] |
M. Jiang, X. Zhang, Y. Sun, W. Feng, Q. Gan, Y. Ruan, AFSNet: Attention-guided full-scale feature aggregation network for highresolution remote sensing image change detection, GISci. Remote Sens., 59 (2022), 1882–1900. https://doi.org/10.1080/15481603.2022.2142626 doi: 10.1080/15481603.2022.2142626
![]() |
[31] |
C. Xu, Y. Qi, Y. Wang, M. Lou, J. Pi, Y. Ma, ARF-Net: An adaptive receptive field network for breast masssegmentation in whole mammograms and ultrasound images, Biomed. Signal Process. Control, 71 (2022), 103178. https://doi.org/10.1016/j.bspc.2021.103178 doi: 10.1016/j.bspc.2021.103178
![]() |
[32] |
D. Maji, P. Sigedar, M. Singh, Attention Res-UNet with guided decoder for semantic segmentation of brain tumors, Biomed. Signal Process. Control, 71 (2022), 103077. https://doi.org/10.1016/j.bspc.2021.103077 doi: 10.1016/j.bspc.2021.103077
![]() |
[33] | H. Huang, L. Lin, R. Tong, H. Hu, J. Wu, UNet 3+: A full-scale connected UNet for medical image segmentation, in IEEE International Conference on Acoustics, Speech and Signal Processing, Barcelona, Spain, (2020), 1055–1059. https://doi.org/10.1109/ICASSP40776.2020.9053405 |
[34] |
K. Wang, S. Liang, S. Zhong, Q. Feng, Y. Zhang, Breast ultrasound image segmentation: A coarse-to-fine fusion convolutional neural network, Med. Phys., 48 (2021), 4262–4278. https://doi.org/10.1002/mp.15006 doi: 10.1002/mp.15006
![]() |
[35] |
J. Wang, P. Lv, H. Wang, C. Shi, SAR-U-Net: Squeeze-and-excitation block and atrous spatial pyramid pooling based residual U-Net for automatic liver ct segmentation, Comput. Methods Programs Biomed., 208 (2021), 106268. https://doi.org/10.1016/j.cmpb.2021.106268 doi: 10.1016/j.cmpb.2021.106268
![]() |
[36] |
G. Xiao, B. Zhu, Y. Zhang, H. Gao, FCSNet: A quantitative explanation method for surface scratch defects during belt grinding based on deep learning, Comput. Ind., 144 (2023), 103793. https://doi.org/10.1016/j.compind.2022.103793 doi: 10.1016/j.compind.2022.103793
![]() |
[37] |
A. Iqbal, M. Sharif, M. A. Khan, W. Nisar, M. Alhaisoni, FF-UNet: A u-shaped deep convolutional neural network for multimodal biomedical image segmentation, Cognit. Comput., 14 (2022), 1287–1302. https://doi.org/10.1007/s12559-022-10038-y doi: 10.1007/s12559-022-10038-y
![]() |
[38] |
F. Xie, Z. Huang, Z. Shi, T. Wang, G. Song, B. Wang, et al., DUDA-Net: A double u-shaped dilated attention network for automatic infection area segmentation in COVID-19 lung CT images, Int. J. Comput. Assisted Radiol. Surg., 16 (2021), 1425–1434. https://doi.org/10.1007/s11548-021-02418-w doi: 10.1007/s11548-021-02418-w
![]() |
[39] | R. Azad, M. Asadi-Aghbolaghi, M. Fathy, S. Escalera, Bi-directional ConvLSTM U-Net with densley connected convolutions, in Proceedings of the IEEE/CVF International Conference on Computer Vision workshops, Seoul, Korea, (2019), 406–415. https://doi.org/10.1109/ICCVW.2019.00052 |
[40] |
D. Peng, Y. Zhang, H. Guan, End-to-end change detection for high resolution satellite images using improved UNet++, Remote Sens., 11 (2019), 1382. https://doi.org/10.3390/rs11111382 doi: 10.3390/rs11111382
![]() |
[41] |
H. Wu, Z. Zhao, Z. Wang, META-Unet: Multi-scale efficient transformer attention Unet for fast and high-accuracy polyp segmentation, IEEE Trans. Autom. Sci. Eng., (2023), 1–12. https://doi.org/10.1109/TASE.2023.3292373 doi: 10.1109/TASE.2023.3292373
![]() |
[42] |
R. Su, D. Zhang, J. Liu, C. Cheng, MSU-Net: Multi-scale U-Net for 2D medical image segmentation, Front. Genet., 12 (2021), 639930. https://doi.org/10.3389/fgene.2021.639930 doi: 10.3389/fgene.2021.639930
![]() |
[43] |
Z. Gu, J. Cheng, H. Fu, K. Zhou, H. Hao, Y. Zhao, et al., CE-Net: Context encoder network for 2d medical image segmentation, IEEE Trans. Med. Imaging, 38 (2019), 2281–2292. https://doi.org/10.1109/TMI.2019.2903562 doi: 10.1109/TMI.2019.2903562
![]() |
[44] |
M. M. Ji, Z. B. Wu, Automatic detection and severity analysis of grape black measles disease based on deep learning and fuzzy logic, Comput. Electron. Agric., 193 (2022), 106718. https://doi.org/10.1016/j.compag.2022.106718 doi: 10.1016/j.compag.2022.106718
![]() |
[45] |
M. Jiang, F. Zhai, J. Kong, A novel deep learning model DDU-net using edge features to enhance brain tumor segmentation on MR images, Artif. Intell. Med., 121 (2021), 102180. https://doi.org/10.1016/j.artmed.2021.102180 doi: 10.1016/j.artmed.2021.102180
![]() |
[46] |
Y. Y. Yang, C. Feng, R. F. Wang, Automatic segmentation model combining U-Net and level set method for medical images, Expert Syst. Appl., 153 (2020), 113419. https://doi.org/10.1016/j.eswa.2020.113419 doi: 10.1016/j.eswa.2020.113419
![]() |
[47] |
C. Zhao, R. J. Shuai, L. Ma, W. J. Liu, M. L. Wu, Segmentation of dermoscopy images based on deformable 3D convolution and ResU-NeXt++, Med. Biol. Eng. Comput., 59 (2021), 1815–1832. https://doi.org/10.1007/s11517-021-02397-9 doi: 10.1007/s11517-021-02397-9
![]() |
1. | Jing Li, Jiangang Qi, On a nonlocal Sturm–Liouville problem with composite fractional derivatives, 2021, 44, 0170-4214, 1931, 10.1002/mma.6893 | |
2. | Ramazan Ozarslan, Erdal Bas, Reassessments of gross domestic product model for fractional derivatives with non-singular and singular kernels, 2021, 25, 1432-7643, 1535, 10.1007/s00500-020-05237-4 | |
3. | Ahu Ercan, Ramazan Ozarslan, Erdal Bas, Existence and uniqueness analysis of solutions for Hilfer fractional spectral problems with applications, 2021, 40, 2238-3603, 10.1007/s40314-020-01382-6 | |
4. | Erdal Bas, Funda Metin Turk, Ramazan Ozarslan, Ahu Ercan, Spectral data of conformable Sturm–Liouville direct problems, 2021, 11, 1664-2368, 10.1007/s13324-020-00428-6 | |
5. | Alberto Almech, Eugenio Roanes-Lozano, A 3D proposal for the visualization of speed in railway networks, 2020, 5, 2473-6988, 7480, 10.3934/math.2020479 | |
6. | Churong Chen, Martin Bohner, Baoguo Jia, Ulam‐Hyers stability of Caputo fractional difference equations, 2019, 42, 0170-4214, 7461, 10.1002/mma.5869 | |
7. | Muath Awadalla, Nazim I. Mahmudov, Jihan Alahmadi, A novel delayed discrete fractional Mittag-Leffler function: representation and stability of delayed fractional difference system, 2024, 70, 1598-5865, 1571, 10.1007/s12190-024-02012-8 | |
8. | B. Shiri, Y. Guang, D. Baleanu, Inverse problems for discrete Hermite nabla difference equation, 2025, 33, 2769-0911, 10.1080/27690911.2024.2431000 | |
9. | Ahu Ercan, Erdal Bas, Ramazan Ozarslan, Solving Hilfer fractional dirac systems: a spectral approach, 2025, 95, 0939-1533, 10.1007/s00419-025-02767-x |
i | λ1,i | λ2,i | λ3,i | λ1,i | λ2,i | λ3,i | λ1,i | λ2,i | λ3,i |
750 | −0.992 | −0.982 | −0.057 | −0.986 | −0.941 | −0.027 | −0.483 | −0.483 | 0 |
1000 | −0.989 | −0.977 | −0.057 | −0.990 | −0.954 | −0.027 | −0.559 | −0.435 | 0 |
2000 | −0.996 | −0.990 | −0.057 | −0.995 | −0.978 | −0.027 | −0.654 | −0.435 | 0 |
x(5),μ=0.5 | x(10),μ=0.9 | x(2000),μ=0.1 | |||||||
i | λ1,i | λ2,i | λ3,i | λ1,i | λ2,i | λ3,i | λ1,i | λ2,i | λ3,i |
750 | −0.951 | −0.004 | 0 | −0.868 | −0.793 | −0.0003 | −0.190 | −3.290×10−6 | 0 |
1000 | −0.963 | −0.004 | 0 | −0.898 | −0.828 | −0.0003 | −0.394 | −3.290×10−6 | 0 |
2000 | −0.981 | −0.004 | 0 | −0.947 | −0.828 | −0.0003 | −0.548 | −3.290×10−6 | 0 |
x(20),μ=0.5 | x(100),μ=0.9 | x(1000),μ=0.7 | |||||||
i | λ1,i | λ2,i | λ3,i | λ1,i | λ2,i | λ3,i | λ1,i | λ2,i | λ3,i |
750 | −0.414 | −9.59×10−7 | 0 | −0.853 | −0.0003 | 0 | −0.330 | −4.140×10−6 | 0 |
1000 | −0.478 | −9.59×10−7 | 0 | −0.887 | −0.0003 | 0 | −0.375 | −4.140×10−6 | 0 |
2000 | −0.544 | −9.59×10−7 | 0 | −0.940 | −0.0003 | 0 | −0.361 | −4.140×10−6 | 0 |
x(1000),μ=0.3 | x(100),μ=0.8 | x(1000),μ=0.9 | |||||||
i | λ1,i | λ2,i | λ3,i | λ1,i | λ2,i | λ3,i | λ1,i | λ2,i | λ3,i |
750 | −0.303 | −3.894×10−6 | 0 | −0.192 | −0.066 | 0 | −0.985 | −0.955 | −0.026 |
1000 | −0.335 | −3.894×10−6 | 0 | −0.197 | −0.066 | 0 | −0.989 | −0.941 | −0.026 |
2000 | −0.399 | −3.894×10−6 | 0 | −0.289 | −0.066 | 0 | −0.994 | −0.918 | −0.026 |
x(1000),μ=0.8 | x(2000),μ=0.6 | x(10),μ=0.83 |
x(t) | μ=0.1 | μ=0.2 | μ=0.5 | μ=0.7 | μ=0.9 |
x(1) | 1 | 1 | 1 | 1 | 1 |
x(2) | 0.125 | 0.25 | 0.625 | 0.875 | 1.125 |
x(3) | 0.075 | 0.174 | 0.624 | 1.050 | 1.575 |
x(5) | 0.045 | 0.128 | 0.830 | 1.968 | 4.000 |
x(7) | 0.0336 | 0.111 | 1.228 | 4.079 | 11.203 |
x(9) | 0.0274 | 0.103 | 1.878 | 8.657 | 31.941 |
x(12) | 0.022 | 0.098 | 3.622 | 27.05 | 154.56 |
x(15) | 0.0187 | 0.0962 | 7.045 | 84.75 | 748.56 |
x(16) | 0.0178 | 0.0961 | 8.800 | 124.04 | 1266.5 |
x(18) | 0.0164 | 0.0964 | 13.737 | 265.70 | 3625.6 |
x(20) | 0.0152 | 0.0972 | 21.455 | 569.16 | 10378.8 |
x(t) | q(t)=1 | q(t)=t | q(t)=√t |
x(1) | 1 | 1 | 1 |
x(2) | 0.2261 | 0.1505 | 0.1871 |
x(3) | 0.1138 | 0.0481 | 0.0767 |
x(5) | 0.0518 | 0.0110 | 0.0252 |
x(7) | 0.0318 | 0.0043 | 0.0123 |
x(9) | 0.0223 | 0.0021 | 0.0072 |
x(12) | 0.0150 | 0.0010 | 0.0039 |
x(15) | 0.0110 | 0.0005 | 0.0025 |
x(16) | 0.0101 | 0.0004 | 0.0022 |
x(18) | 0.0086 | 0.0003 | 0.0017 |
x(20) | 0.0075 | 0.0002 | 0.0014 |
x(t) | q(t)=1 | q(t)=t | q(t)=√t |
x(1) | 1 | 1 | 1 |
x(2) | 0.2261 | 0.1505 | 0.1871 |
x(3) | 0.1138 | 0.0481 | 0.0767 |
x(5) | 0.0518 | 0.0110 | 0.0252 |
x(7) | 0.0318 | 0.0043 | 0.0123 |
x(9) | 0.0223 | 0.0021 | 0.0072 |
x(12) | 0.0150 | 0.0010 | 0.0039 |
x(15) | 0.0110 | 0.0005 | 0.0025 |
x(16) | 0.0101 | 0.0004 | 0.0022 |
x(18) | 0.0086 | 0.0003 | 0.0017 |
x(20) | 0.0075 | 0.0002 | 0.0014 |
λ1 | λ2 | λ3 | λ4 | λ5 | λ6 | λ7 | λ8 | λ9 | λ10 | |
DFSL | −0.904 | −0.859 | −0.811 | −0.262 | −0.157 | −0.079 | −0.029 | −0.003 | 0.982 | |
FSL | −0.497 | −0.383 | −0.283 | −0.196 | −0.124 | −0.066 | −0.026 | −0.003 | 0 | ... |
DSL | −1.450 | −0.689 | −0.469 | −0.310 | −0.194 | −0.112 | −0.055 | −0.019 | −0.002 | |
CSL | −0.163 | −0.128 | −0.098 | −0.072 | −0.050 | −0.032 | −0.008 | −0.002 | 0 |
λ1 | λ2 | λ3 | λ4 | λ5 | λ6 | λ7 | λ8 | λ9 | λ10 | |
DFSL | −0.866 | −0.813 | −0.200 | −0.115 | −0.057 | −0.020 | −0.002 | 0 | 0.982 | |
FSL | −0.456 | −0.343 | −0.246 | −0.165 | −0.100 | −0.051 | −0.018 | −0.002 | 0 | ... |
DSL | −1.450 | −0.689 | −0.469 | −0.310 | −0.194 | −0.112 | −0.055 | −0.019 | −0.002 | ... |
CSL | −0.163 | −0.128 | −0.098 | −0.072 | −0.050 | −0.032 | −0.008 | −0.002 | 0 |
i | λ1,i | λ2,i | λ3,i | λ1,i | λ2,i | λ3,i | λ1,i | λ2,i | λ3,i |
750 | −0.992 | −0.982 | −0.057 | −0.986 | −0.941 | −0.027 | −0.483 | −0.483 | 0 |
1000 | −0.989 | −0.977 | −0.057 | −0.990 | −0.954 | −0.027 | −0.559 | −0.435 | 0 |
2000 | −0.996 | −0.990 | −0.057 | −0.995 | −0.978 | −0.027 | −0.654 | −0.435 | 0 |
x(5),μ=0.5 | x(10),μ=0.9 | x(2000),μ=0.1 | |||||||
i | λ1,i | λ2,i | λ3,i | λ1,i | λ2,i | λ3,i | λ1,i | λ2,i | λ3,i |
750 | −0.951 | −0.004 | 0 | −0.868 | −0.793 | −0.0003 | −0.190 | −3.290×10−6 | 0 |
1000 | −0.963 | −0.004 | 0 | −0.898 | −0.828 | −0.0003 | −0.394 | −3.290×10−6 | 0 |
2000 | −0.981 | −0.004 | 0 | −0.947 | −0.828 | −0.0003 | −0.548 | −3.290×10−6 | 0 |
x(20),μ=0.5 | x(100),μ=0.9 | x(1000),μ=0.7 | |||||||
i | λ1,i | λ2,i | λ3,i | λ1,i | λ2,i | λ3,i | λ1,i | λ2,i | λ3,i |
750 | −0.414 | −9.59×10−7 | 0 | −0.853 | −0.0003 | 0 | −0.330 | −4.140×10−6 | 0 |
1000 | −0.478 | −9.59×10−7 | 0 | −0.887 | −0.0003 | 0 | −0.375 | −4.140×10−6 | 0 |
2000 | −0.544 | −9.59×10−7 | 0 | −0.940 | −0.0003 | 0 | −0.361 | −4.140×10−6 | 0 |
x(1000),μ=0.3 | x(100),μ=0.8 | x(1000),μ=0.9 | |||||||
i | λ1,i | λ2,i | λ3,i | λ1,i | λ2,i | λ3,i | λ1,i | λ2,i | λ3,i |
750 | −0.303 | −3.894×10−6 | 0 | −0.192 | −0.066 | 0 | −0.985 | −0.955 | −0.026 |
1000 | −0.335 | −3.894×10−6 | 0 | −0.197 | −0.066 | 0 | −0.989 | −0.941 | −0.026 |
2000 | −0.399 | −3.894×10−6 | 0 | −0.289 | −0.066 | 0 | −0.994 | −0.918 | −0.026 |
x(1000),μ=0.8 | x(2000),μ=0.6 | x(10),μ=0.83 |
x(t) | μ=0.1 | μ=0.2 | μ=0.5 | μ=0.7 | μ=0.9 |
x(1) | 1 | 1 | 1 | 1 | 1 |
x(2) | 0.125 | 0.25 | 0.625 | 0.875 | 1.125 |
x(3) | 0.075 | 0.174 | 0.624 | 1.050 | 1.575 |
x(5) | 0.045 | 0.128 | 0.830 | 1.968 | 4.000 |
x(7) | 0.0336 | 0.111 | 1.228 | 4.079 | 11.203 |
x(9) | 0.0274 | 0.103 | 1.878 | 8.657 | 31.941 |
x(12) | 0.022 | 0.098 | 3.622 | 27.05 | 154.56 |
x(15) | 0.0187 | 0.0962 | 7.045 | 84.75 | 748.56 |
x(16) | 0.0178 | 0.0961 | 8.800 | 124.04 | 1266.5 |
x(18) | 0.0164 | 0.0964 | 13.737 | 265.70 | 3625.6 |
x(20) | 0.0152 | 0.0972 | 21.455 | 569.16 | 10378.8 |
x(t) | q(t)=1 | q(t)=t | q(t)=√t |
x(1) | 1 | 1 | 1 |
x(2) | 0.2261 | 0.1505 | 0.1871 |
x(3) | 0.1138 | 0.0481 | 0.0767 |
x(5) | 0.0518 | 0.0110 | 0.0252 |
x(7) | 0.0318 | 0.0043 | 0.0123 |
x(9) | 0.0223 | 0.0021 | 0.0072 |
x(12) | 0.0150 | 0.0010 | 0.0039 |
x(15) | 0.0110 | 0.0005 | 0.0025 |
x(16) | 0.0101 | 0.0004 | 0.0022 |
x(18) | 0.0086 | 0.0003 | 0.0017 |
x(20) | 0.0075 | 0.0002 | 0.0014 |
x(t) | q(t)=1 | q(t)=t | q(t)=√t |
x(1) | 1 | 1 | 1 |
x(2) | 0.2261 | 0.1505 | 0.1871 |
x(3) | 0.1138 | 0.0481 | 0.0767 |
x(5) | 0.0518 | 0.0110 | 0.0252 |
x(7) | 0.0318 | 0.0043 | 0.0123 |
x(9) | 0.0223 | 0.0021 | 0.0072 |
x(12) | 0.0150 | 0.0010 | 0.0039 |
x(15) | 0.0110 | 0.0005 | 0.0025 |
x(16) | 0.0101 | 0.0004 | 0.0022 |
x(18) | 0.0086 | 0.0003 | 0.0017 |
x(20) | 0.0075 | 0.0002 | 0.0014 |
λ1 | λ2 | λ3 | λ4 | λ5 | λ6 | λ7 | λ8 | λ9 | λ10 | |
DFSL | −0.904 | −0.859 | −0.811 | −0.262 | −0.157 | −0.079 | −0.029 | −0.003 | 0.982 | |
FSL | −0.497 | −0.383 | −0.283 | −0.196 | −0.124 | −0.066 | −0.026 | −0.003 | 0 | ... |
DSL | −1.450 | −0.689 | −0.469 | −0.310 | −0.194 | −0.112 | −0.055 | −0.019 | −0.002 | |
CSL | −0.163 | −0.128 | −0.098 | −0.072 | −0.050 | −0.032 | −0.008 | −0.002 | 0 |
λ1 | λ2 | λ3 | λ4 | λ5 | λ6 | λ7 | λ8 | λ9 | λ10 | |
DFSL | −0.866 | −0.813 | −0.200 | −0.115 | −0.057 | −0.020 | −0.002 | 0 | 0.982 | |
FSL | −0.456 | −0.343 | −0.246 | −0.165 | −0.100 | −0.051 | −0.018 | −0.002 | 0 | ... |
DSL | −1.450 | −0.689 | −0.469 | −0.310 | −0.194 | −0.112 | −0.055 | −0.019 | −0.002 | ... |
CSL | −0.163 | −0.128 | −0.098 | −0.072 | −0.050 | −0.032 | −0.008 | −0.002 | 0 |