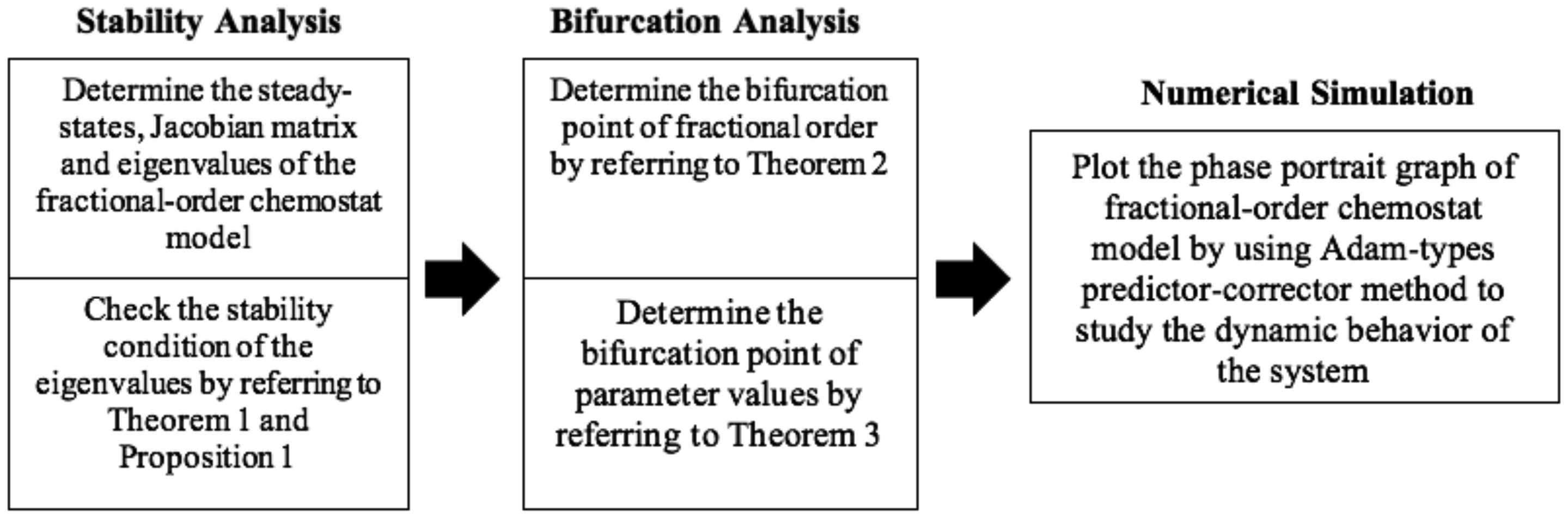
The epigenetic modification of DNA N4-methylcytosine (4mC) is vital for controlling DNA replication and expression. It is crucial to pinpoint 4mC's location to comprehend its role in physiological and pathological processes. However, accurate 4mC detection is difficult to achieve due to technical constraints. In this paper, we propose a deep learning-based approach 4mCPred-GSIMP for predicting 4mC sites in the mouse genome. The approach encodes DNA sequences using four feature encoding methods and combines multi-scale convolution and improved selective kernel convolution to adaptively extract and fuse features from different scales, thereby improving feature representation and optimization effect. In addition, we also use convolutional residual connections, global response normalization and pointwise convolution techniques to optimize the model. On the independent test dataset, 4mCPred-GSIMP shows high sensitivity, specificity, accuracy, Matthews correlation coefficient and area under the curve, which are 0.7812, 0.9312, 0.8562, 0.7207 and 0.9233, respectively. Various experiments demonstrate that 4mCPred-GSIMP outperforms existing prediction tools.
Citation: Jianhua Jia, Yu Deng, Mengyue Yi, Yuhui Zhu. 4mCPred-GSIMP: Predicting DNA N4-methylcytosine sites in the mouse genome with multi-Scale adaptive features extraction and fusion[J]. Mathematical Biosciences and Engineering, 2024, 21(1): 253-271. doi: 10.3934/mbe.2024012
[1] | Shaimaa A. M. Abdelmohsen, D. Sh. Mohamed, Haifa A. Alyousef, M. R. Gorji, Amr M. S. Mahdy . Mathematical modeling for solving fractional model cancer bosom malignant growth. AIMS Biophysics, 2023, 10(3): 263-280. doi: 10.3934/biophy.2023018 |
[2] | Mati ur Rahman, Mehmet Yavuz, Muhammad Arfan, Adnan Sami . Theoretical and numerical investigation of a modified ABC fractional operator for the spread of polio under the effect of vaccination. AIMS Biophysics, 2024, 11(1): 97-120. doi: 10.3934/biophy.2024007 |
[3] | Yasir Nadeem Anjam, Mehmet Yavuz, Mati ur Rahman, Amna Batool . Analysis of a fractional pollution model in a system of three interconnecting lakes. AIMS Biophysics, 2023, 10(2): 220-240. doi: 10.3934/biophy.2023014 |
[4] | Marco Menale, Bruno Carbonaro . The mathematical analysis towards the dependence on the initial data for a discrete thermostatted kinetic framework for biological systems composed of interacting entities. AIMS Biophysics, 2020, 7(3): 204-218. doi: 10.3934/biophy.2020016 |
[5] | Mehmet Yavuz, Kübra Akyüz, Naime Büşra Bayraktar, Feyza Nur Özdemir . Hepatitis-B disease modelling of fractional order and parameter calibration using real data from the USA. AIMS Biophysics, 2024, 11(3): 378-402. doi: 10.3934/biophy.2024021 |
[6] | Larisa A. Krasnobaeva, Ludmila V. Yakushevich . On the dimensionless model of the transcription bubble dynamics. AIMS Biophysics, 2023, 10(2): 205-219. doi: 10.3934/biophy.2023013 |
[7] | Carlo Bianca . Differential equations frameworks and models for the physics of biological systems. AIMS Biophysics, 2024, 11(2): 234-238. doi: 10.3934/biophy.2024013 |
[8] | Mohammed Alabedalhadi, Mohammed Shqair, Ibrahim Saleh . Analysis and analytical simulation for a biophysical fractional diffusive cancer model with virotherapy using the Caputo operator. AIMS Biophysics, 2023, 10(4): 503-522. doi: 10.3934/biophy.2023028 |
[9] | Bertrand R. Caré, Pierre-Emmanuel Emeriau, Ruggero Cortini, Jean-Marc Victor . Chromatin epigenomic domain folding: size matters. AIMS Biophysics, 2015, 2(4): 517-530. doi: 10.3934/biophy.2015.4.517 |
[10] | David Gosselin, Maxime Huet, Myriam Cubizolles, David Rabaud, Naceur Belgacem, Didier Chaussy, Jean Berthier . Viscoelastic capillary flow: the case of whole blood. AIMS Biophysics, 2016, 3(3): 340-357. doi: 10.3934/biophy.2016.3.340 |
The epigenetic modification of DNA N4-methylcytosine (4mC) is vital for controlling DNA replication and expression. It is crucial to pinpoint 4mC's location to comprehend its role in physiological and pathological processes. However, accurate 4mC detection is difficult to achieve due to technical constraints. In this paper, we propose a deep learning-based approach 4mCPred-GSIMP for predicting 4mC sites in the mouse genome. The approach encodes DNA sequences using four feature encoding methods and combines multi-scale convolution and improved selective kernel convolution to adaptively extract and fuse features from different scales, thereby improving feature representation and optimization effect. In addition, we also use convolutional residual connections, global response normalization and pointwise convolution techniques to optimize the model. On the independent test dataset, 4mCPred-GSIMP shows high sensitivity, specificity, accuracy, Matthews correlation coefficient and area under the curve, which are 0.7812, 0.9312, 0.8562, 0.7207 and 0.9233, respectively. Various experiments demonstrate that 4mCPred-GSIMP outperforms existing prediction tools.
Numerous mathematical models have been established to predict and study the biological system. In the past four decades, there have been far-reaching research on improving cell mass production in chemical reactors [1]. The chemostat model is used to understand the mechanism of cell mass growth in a chemostat. A chemostat is an apparatus for continuous culture that contains bacterial populations. It can be used to investigate the cell mass production under controlled conditions. This reactor provides a dynamic system for population studies and is suitable to be used in a laboratory. A substrate is continuously added into the reactor containing the cell mass, which grows by consuming the substrate that enters through the inflow chamber. Meanwhile, the mixture of cell mass and substrate is continuously harvested from the reactor through the outflow chamber. The dynamics in the chemostat can be investigated by using the chemostat model [2].
Ordinary differential equations (ODEs) are commonly used for modelling biological systems. However, most biological systems behaviour has memory effects, and ODEs usually neglect such effects. The fractional-order differential equations (FDEs) are taken into account when describing the behaviour of the systems' equations. A FDEs is a generalisation of the ODEs to random nonlinear order [3]. This equation is more effective because of its good memory, among other advantages [4]–[8]. The errors occuring from the disregarded parameters when modelling of phenomena in real-life also can be reduced. FDEs are also used to efficiently replicate the real nature of various systems in the field of engineering and sciences [9]. In the past few decades, FDEs have been used in biological systems for various studies [5], [6], [10]–[17].
Since great strides in the study of FDEs have been developed, the dynamics in the chemostat can be investigated using the mathematical model of the chemostat in the form of FDEs. Moreover, there have been few studies on the expansion of the chemostat model with fractional-order theory. Thus, we deepen and complete the analysis on the integer-order chemostat model with fractional-order theory and discuss the stability of the equilibrium points of the fractional-order chemostat model. Next, the bifurcation analysis for the fractional-order chemostat model is conducted to identify the bifurcation point that can change the stability of the system. The analysis identifies the values of the fractional-order and the system parameters to ensure the operation of the chemostat is well-controlled.
Recently, there are many approaches to define fractional operators such as by Caputo, Riemann-Liouville, Hadamard, and Grunwald-Letnikov [3]. However, Caputo is often used due to its convenience in various applications [18]. Caputo is also useful to encounter an obstacle where the initial condition is done in the differential of integer-order [19]. In this paper, we applied Caputo derivative to define the system of fractional-order. The Caputo derivative for the left-hand side is defined as
where Г denotes the function of gamma, n is an integer, where
The Adams-type predictor-corrector method is one of the technique that have been proposed for fractional-order differential equations [19]–[21]. The Adams-type predictor-corrector method is a analysis of numerical algorithm that involves two basics steps: predictor and corrector. The predictor formula can be described as
meanwhile the corrector formula can be determined by
The predictor-corrector method is also called as the PECE (Predict, Evaluate, Correct, Evaluate) method [22]. The procedure of the predictor-corrector method can be explained as follows
(i) Calculate the predictor step,
(ii) Evaluate
(iii) Calculate the corrector step,
(iv) Evaluate
The procedure repeatedly predicts and corrects the value until the corrected value becomes a converged number [21]. This method is able to maintain the stability of the properties and has good accuracy. Moreover, this method also has lower computational cost than other methods [23]. The algorithm of the Adams-type predictor-corrector method proposed by [22] is shown in Appendix.
The conditions of stability for integer-order differential equations and fractional-order differential equations are different. Both systems could have the same steady-state points but different stability conditions [24], [25]. The stability condition for fractional-order differential equations can be stated by Theorem 1 and the Routh-Hurwitz stability condition as described by Proposition 1.
Theorem 1
Proposition 1
or
The stability theorem on the fractional-order systems and fractional Routh-Hurwitz stability conditions are introduced to analyze the stability of the model. The fractional Routh-Hurwitz stability conditions is specifically introduced for the eigenvalues of the Jacobian matrix that obtained in quadratic form. The proof of this proposition is shown in Appendix.
Bifurcation can be defined as any sudden change that occurs while a parameter value is varied in the differential equation system and it has a significant influence on the solution [27]. An unstable steady-state may becomes stable and vice versa. A slight changes in the parameter value may change the system's stability. Despite the steady-state point and the eigenvalues of the system of fractional-order are similar as the system of integer-order, the discriminant method used for the stability of the steady-state point is different. Accordingly, the Hopf bifurcation condition in the fractional-order system is slightly different as compared with the integer-order system.
Fractional order α can be selected as the bifurcation parameter in a fractional-order system, but this is not allowed in an integer-order system. The existence of Hopf bifurcation can be stated as in Theorem 2.
Theorem 2
(i) 1. The characteristic equation of chemostat system has a pair of complex conjugate roots,
(ii) Critical value
(iii)
Proof. Condition (i) is not easy to obtain due to the selected parameter's value. However, this condition can be managed under some confined conditions. In fact, the washout steady-state solution of the chemostat model has two negative real roots. The remaining two roots depend on the characteristic of the polynomial from the no-washout steady-state solution.
Condition (ii) can be satisfied with the existence of critical value α* and when
The integer system required p = 0 for the bifurcation's operating condition. For the fractional-order system, the operating condition of the system will change into
In studying the dynamic process of chemostat, the parameters such as
Theorem 3
(i) The characteristic equation of chemostat system has a pair of complex conjugate roots,
(ii) Critical value
(iii)
Proof. This theorem can be proved in the same way as Theorem 2. Therefore, condition (i) can be guaranteed. Condition (ii) can be satisfied with the existence of critical value
For condition (iii), the condition of
Firstly, determine the steady-states, Jacobian matrix and eigenvalues of the fractional-order chemostat model. The stability properties of the fractional-order chemostat model were estimated by using the stability and bifurcation analyses with FDEs by referring to Theorem 1 and Proposition 1. Then, determine the bifurcation point of fractional-order by referring to Theorem 2 and determine the bifurcation point of parameter values by referring to Theorem 3. Next, plot the phase portrait of fractional-order chemostat model by using Adam-types predictor-corrector method to study the dynamic behaviour of the system. Figure 1 depicts the flowchart of this research. This flowchart can be applied to all problems with suitable parameter values.
An integer-order chemostat model that considered a variable yield coefficient and the Monod growth model from [1] is studied in this section. The chemostat system can be written as
with the initial value of
Let Eq. (3.2) equal to zero in order to find the steady-state solutions
By solving Eq. (3.4), the following solutions are obtained
From
Hence, the solutions of steady-state for the chemostat model are
(i) Washout:
(ii) No Washout:
where
The steady-state solutions are physically meaningful if their components are positive. Therefore,
The solution of steady-state in Equation (3.8) represents the washout situation, where the cell mass is wholly removed from the reactor and where the substrate concentration is at the same stock as in the beginning. This state must always be unstable in order to ensure that the cell mass is able to grow in the chemostat. This is because the cell mass will be continuously removed from the chemostat if the washout steady-state is stable. The Jacobian matrix for the washout steady-state solution can be written as
The eigenvalues of this matrix are
The eigenvalues in
The eigenvalues of the Jacobian matrix in terms of the characteristic polynomial are
where
and
The eigenvalues of the no-washout steady-state solution were evaluated with Routh-Hurwitz condition in Proposition 1. Based on the eigenvalues in Eq. (3.15) and by referring to the study by [5], the eigenvalues' condition can be simplified as the following two cases
(i) If b>0 or equivalent to
(ii) If b<0 or equivalent to
Then, if
The parameter values of the fractional-order chemostat model are provided in Table 1. The initial substrate concentration, S0 and ρ were assumed as non-negative values to ensure that the steady-state solutions were physically meaningful. The stability diagram of the steady-state solutions is plotted in Figure 2.
Parameters | Description | Values | Units |
k | Saturation constant | 1.75 | gl−1 |
Q | Dilution rate | 0.02 | l2gr−1 |
µ | Maximum growth rate | 0.3 | h−1 |
γ | Constant in yield coefficient | 0.01 | – |
β | Constant in yield coefficient | 5.25 | lg−1 |
S0 | Input concentration of substrate | 1 | gl−1 |
The washout steady-state solution is stable if Q > 0 and
The steady-state solutions of the fractional-order chemostat model for the parameter values given in Table 1 are
(i) Washout:
(ii) No Washout:
The eigenvalues obtained from the washout steady-state solution are
and the eigenvalues from the no-washout steady-state solution are
Based on Eq. (3.22) to Eq. (3.25), these satisfied the first condition of Hopf bifurcation in Theorem 2. There exists a pair of complex conjugate roots and the other eigenvalues are negative real roots. The transversality condition as the third condition is also satisfied. The eigenvalues of the washout steady-state solution based on the chemostat system is not imaginary, and so there is no existence of Hopf bifurcation in the washout steady-state solution. According to Theorem 2, the critical value of the fractional-order as stated in the second condition can be obtained as
where
Value of p and q are obtained from
The running state of the fractional-order chemostat system when fractional order α at the Hopf bifurcation point is shown. The fractional-order chemostat system changed its stability once Hopf bifurcation occurred. Therefore, we conjecture that the system of fractional-order chemostat may be lost or gain its stability when the fractional order α is less than the Hopf bifurcation point, or
The initial concentration of the substrate, S0, was chosen as the control parameter, while fractional order α was fixed. The solutions of steady-state of the fractional-order chemostat model with S0 as the control parameter are
(i) Washout:
(ii) No Washout:
The eigenvalues obtained from the washout steady-state solution are
and the eigenvalues from the no-washout steady-state solution are
These satisfied the first condition of Hopf bifurcation in Theorem 3. There exist a pair of complex conjugate roots in terms of S0, and the other eigenvalues were negative real roots in terms of S0. The transversality condition as the third condition is also satisfied. According to Theorem 3, the critical value of the fractional order as stated in the second condition can be obtained as follows
By referring to the study by [18], Eq. (3.37) can also be calculated as
From the calculations, the critical value of the initial concentration of the substrate is
The change in the running state when the value of the initial substrate concentration passes through the Hopf bifurcation point is shown. The stability of the fractional-order chemostat system changed once Hopf bifurcation occurred. In
The Hopf bifurcation points of system of fractional-order chemostat and system of integer-order chemostat are different.
The stability analysis of the fractional-order chemostat model was conducted based on the stability theory of FDEs. The integer-order chemostat model was extended to the FDEs. There are two steady-state solutions obtained, which are washout and no-washout steady-state solutions. The Hopf bifurcation of the order of α occured at the solutions of steady-state when the Hopf bifurcation conditions is fulfilled. The results show that the increasing or decreasing the value of α may stabilise the unstable state of the chemostat system. Therefore, the running state of the fractional-order chemostat system is affected by the value of α. The Hopf bifurcation of the initial concentration of the substrate, S0, also occurred when the Hopf bifurcation condition is fulfilled. As the evidence from the phase portrait plots, increase the value of the initial substrate concentration may destabilise the stable state of the chemostat system. The value of the initial substrate should remain at
[1] |
L. Zhao, J. Song, Y. Liu, C. Song, C. Yi, Mapping the epigenetic modifications of DNA and RNA, Protein Cell, 11 (2020), 792–808. https://doi.org/10.1007/s13238-020-00733-7 doi: 10.1007/s13238-020-00733-7
![]() |
[2] |
L. D. Moore, T. Le, G. Fan, DNA methylation and its basic function, Neuropsychopharmacology, 38 (2013), 23–38. https://doi.org/10.1038/npp.2012.112 doi: 10.1038/npp.2012.112
![]() |
[3] |
N. Zhang, C. Lin, X. Huang, A. Kolbanovskiy, B. E. Hingerty, S. Amin, et al., Methylation of cytosine at C5 in a CpG sequence context causes a conformational switch of a benzo[a]pyrene diol epoxide-N2-guanine adduct in DNA from a minor groove alignment to intercalation with base displacement, J. Mol. Biol., 346 (2005), 951–965. https://doi.org/10.1016/j.jmb.2004.12.027 doi: 10.1016/j.jmb.2004.12.027
![]() |
[4] |
A. Breiling, F. Lyko, Epigenetic regulatory functions of DNA modifications: 5-methylcytosine and beyond, Epigenet. Chromatin, 8 (2015), 24. https://doi.org/10.1186/s13072-015-0016-6 doi: 10.1186/s13072-015-0016-6
![]() |
[5] |
A. Jeltsch, R. Z. Jurkowska, New concepts in DNA methylation, Trends Biochem. Sci., 39 (2014), 310–318. https://doi.org/10.1016/j.tibs.2014.05.002 doi: 10.1016/j.tibs.2014.05.002
![]() |
[6] |
D. Schübeler, Function and information content of DNA methylation, Nature, 517 (2015), 321–326. https://doi.org/10.1038/nature14192 doi: 10.1038/nature14192
![]() |
[7] |
N. P. Blackledge, R. Klose, CpG island chromatin, Epigenetics, 6 (2011), 147–152. https://doi.org/10.4161/epi.6.2.13640 doi: 10.4161/epi.6.2.13640
![]() |
[8] |
F. J. Clasen, R. E. Pierneef, B. Slippers, O. Reva, EuGI: a novel resource for studying genomic islands to facilitate horizontal gene transfer detection in eukaryotes, BMC Genomics, 19 (2018). https://doi.org/10.1186/s12864-018-4724-8 doi: 10.1186/s12864-018-4724-8
![]() |
[9] |
X. Guo, Y. Guo, H. Chen, X. Liu, P. He, W. Li, et al., Systematic comparison of genome information processing and boundary recognition tools used for genomic island detection, Comput. Biol. Med., 166 (2023), 107550. https://doi.org/10.1016/j.compbiomed.2023.107550 doi: 10.1016/j.compbiomed.2023.107550
![]() |
[10] |
Q. Dai, C. Bao, Y. Hai, S. Ma, T. Zhou, C. Wang, et al., MTGIpick allows robust identification of genomic islands from a single genome, Briefings Bioinf., 19 (2018), 361–373. https://doi.org/10.1093/bib/bbw118 doi: 10.1093/bib/bbw118
![]() |
[11] | A. Chialastri, S. Sarkar, E. E. Schauer, S. Lamba, S. S. Dey, Combinatorial quantification of 5mC and 5hmC at individual CpG dyads and the transcriptome in single cells reveals modulators of DNA methylation maintenance fidelity, preprint. |
[12] |
G. Luo, M. A. Blanco, E. L. Greer, C. He, Y. Shi, DNA N6-methyladenine: a new epigenetic mark in eukaryotes?, Nat. Rev. Mol. Cell Biol., 16 (2015), 705–710. https://doi.org/10.1038/nrm4076 doi: 10.1038/nrm4076
![]() |
[13] |
J. Beaulaurier, E. E. Schadt, G. Fang, Deciphering bacterial epigenomes using modern sequencing technologies, Nat. Rev. Genet., 20 (2019), 157–172. https://doi.org/10.1038/s41576-018-0081-3 doi: 10.1038/s41576-018-0081-3
![]() |
[14] |
M. Ehrlich, G. G. Wilson, K. C. Kuo, C. W. Gehrke, N4-methylcytosine as a minor base in bacterial DNA, Journal of Bacteriology, 169 (1987), 939–943. https://doi.org/10.1128/jb.169.3.939-943.1987 doi: 10.1128/jb.169.3.939-943.1987
![]() |
[15] |
F. Rodriguez, I. A. Yushenova, D. DiCorpo, I. R. Arkhipova, Bacterial N4-methylcytosine as an epigenetic mark in eukaryotic DNA, Nat. Commun., 13 (2022), 1072. https://doi.org/10.1038/s41467-022-28471-w doi: 10.1038/s41467-022-28471-w
![]() |
[16] |
M. Yu, L. Ji, D. A. Neumann, D. Chung, J. Groom, J. Westpheling, et al., Base-resolution detection of N 4-methylcytosine in genomic DNA using 4mC-Tet-assisted-bisulfite- sequencing, Nucleic Acids Res., 43 (2015), 148. https://doi.org/10.1093/nar/gkv738 doi: 10.1093/nar/gkv738
![]() |
[17] |
S. Ardui, A. Ameur, J. R. Vermeesch, M. S. Hestand, Single molecule real-time (SMRT) sequencing comes of age: applications and utilities for medical diagnostics, Nucleic Acids Res., 46 (2018), 2159–2168. https://doi.org/10.1093/nar/gky066 doi: 10.1093/nar/gky066
![]() |
[18] |
B. Manavalan, S. Basith, T. H. Shin, D. Y. Lee, L. Wei, G. Lee, 4mCpred-EL: An ensemble learning framework for identification of DNA N4-methylcytosine sites in the mouse genome, Cells, 8 (2019), 1332. https://doi.org/10.3390/cells8111332 doi: 10.3390/cells8111332
![]() |
[19] |
W. He, C. Jia, Q. Zou, 4mCPred: machine learning methods for DNA N4-methylcytosine sites prediction, Bioinformatics, 35 (2019), 593–601. https://doi.org/10.1093/bioinformatics/bty668 doi: 10.1093/bioinformatics/bty668
![]() |
[20] |
M. M. Hasan, B. Manavalan, W. Shoombuatong, M. S. Khatun, H. Kurata, i4mC-Mouse: Improved identification of DNA N4-methylcytosine sites in the mouse genome using multiple encoding schemes, Comput. Struct. Biotechnol. J., 18 (2020), 906–912. https://doi.org/10.1016/j.csbj.2020.04.001 doi: 10.1016/j.csbj.2020.04.001
![]() |
[21] |
W. Chen, H. Yang, P. Feng, H. Ding, H. Lin, iDNA4mC: identifying DNA N4-methylcytosine sites based on nucleotide chemical properties, Bioinformatics, 33 (2017), 3518–3523. https://doi.org/10.1093/bioinformatics/btx479 doi: 10.1093/bioinformatics/btx479
![]() |
[22] |
B. Manavalan, S. Basith, T. H. Shin, L. Wei, G. Lee, Meta-4mCpred: A sequence-based meta-predictor for accurate DNA 4mC site prediction using effective feature representation, Mol. Ther.-Nucleic Acids, 16 (2019), 733–744. https://doi.org/10.1016/j.omtn.2019.04.019 doi: 10.1016/j.omtn.2019.04.019
![]() |
[23] |
H. Xu, P. Jia, Z. Zhao, Deep4mC: systematic assessment and computational prediction for DNA N4-methylcytosine sites by deep learning, Briefings Bioinf., 22 (2021). https://doi.org/10.1093/bib/bbaa099 doi: 10.1093/bib/bbaa099
![]() |
[24] |
Q. Liu, J. Chen, Y. Wang, S. Li, C. Jia, J. Song, et al., DeepTorrent: a deep learning-based approach for predicting DNA N4-methylcytosine sites, Briefings Bioinf., 22 (2021). https://doi.org/10.1093/bib/bbaa124 doi: 10.1093/bib/bbaa124
![]() |
[25] |
X. Yu, J. Ren, Y. Cui, R. Zeng, H. Long, C. Ma, DRSN4mCPred: accurately predicting sites of DNA N4-methylcytosine using deep residual shrinkage network for diagnosis and treatment of gastrointestinal cancer in the precision medicine era, Front. Med., 10 (2023). https://doi.org/10.3389/fmed.2023.1187430 doi: 10.3389/fmed.2023.1187430
![]() |
[26] |
Y. Yu, W. He, J. Jin, G. Xiao, L. Cui, R. Zeng, et al., iDNA-ABT: advanced deep learning model for detecting DNA methylation with adaptive features and transductive information maximization, Bioinformatics, 37 (2021), 4603–4610. https://doi.org/10.1093/bioinformatics/btab677 doi: 10.1093/bioinformatics/btab677
![]() |
[27] |
J. Jin, Y. Yu, R. Wang, X. Zeng, C. Pang, Y. Jiang, et al., iDNA-ABF: multi-scale deep biological language learning model for the interpretable prediction of DNA methylations, Genome Biol., 23 (2022). https://doi.org/10.1186/s13059-022-02780-1 doi: 10.1186/s13059-022-02780-1
![]() |
[28] |
W. Zeng, A. Gautam, D. H. Huson, MuLan-Methyl-multiple transformer-based language models for accurate DNA methylation prediction, GigaScience, 12 (2023). https://doi.org/10.1093/gigascience/giad054 doi: 10.1093/gigascience/giad054
![]() |
[29] |
M. U. Rehman, H. Tayara, K. T. Chong, DCNN-4mC: Densely connected neural network based N4-methylcytosine site prediction in multiple species, Comput. Struct. Biotechnol. J., 19 (2021), 6009–6019. https://doi.org/10.1016/j.csbj.2021.10.034 doi: 10.1016/j.csbj.2021.10.034
![]() |
[30] |
S. Park, M. U. Rehman, F. Ullah, H. Tayara, K. T. Chong, I. Birol, iCpG-Pos: an accurate computational approach for identification of CpG sites using positional features on single-cell whole genome sequence data, Bioinformatics, 39 (2023). https://doi.org/10.1093/bioinformatics/btad474 doi: 10.1093/bioinformatics/btad474
![]() |
[31] |
L. Zhang, X. Xiao, Z. Xu, iPromoter-5mC: A novel fusion decision predictor for the identification of 5-Methylcytosine sites in genome-Wide DNA promoters, Front. Cell Dev. Biol., 8 (2020). https://doi.org/10.3389/fcell.2020.00614 doi: 10.3389/fcell.2020.00614
![]() |
[32] |
D. Y. Lim, M. U. Rehman, K. T. Chong, iRG-4mC: Neural network based tool for identification of DNA 4mC sites in rosaceae genome, Symmetry, 13 (2021), 899. https://doi.org/10.3390/sym13050899 doi: 10.3390/sym13050899
![]() |
[33] |
M. U. Rehman, K. J. Hong, H. Tayara, K. T. Chong, m6A-NeuralTool: Convolution neural tool for RNA N6-Methyladenosine site identification in different species, IEEE Access, 9 (2021), 17779–17786. https://doi.org/10.1109/access.2021.3054361 doi: 10.1109/access.2021.3054361
![]() |
[34] |
Q. H. Nguyen, H. V. Tran, B. P. Nguyen, T. T. T. Do, Identifying transcription factors that prefer binding to methylated DNA using reduced G-Gap dipeptide composition, ACS Omega, 7 (2022), 32322–32330. https://doi.org/10.1021/acsomega.2c03696 doi: 10.1021/acsomega.2c03696
![]() |
[35] |
Z. Li, H. Jiang, L. Kong, Y. Chen, K. Lang, X. Fan, et al., Deep6mA: A deep learning framework for exploring similar patterns in DNA N6-methyladenine sites across different species, PLOS Comput. Biol., 17 (2021), 1008767. https://doi.org/10.1371/journal.pcbi.1008767 doi: 10.1371/journal.pcbi.1008767
![]() |
[36] |
X. Cheng, J. Wang, Q. Li, T. Liu, BiLSTM-5mC: A bidirectional long short-term memory-based approach for predicting 5-Methylcytosine sites in genome-wide DNA promoters, Molecules, 26 (2021), 7414. https://doi.org/10.3390/molecules26247414 doi: 10.3390/molecules26247414
![]() |
[37] |
Z. Abbas, H. Tayara, K. T. Chong, 4mCPred-CNN-Prediction of DNA N4-Methylcytosine in the mouse genome using a convolutional neural network, Genes, 12 (2021), 296. https://doi.org/10.3390/genes12020296 doi: 10.3390/genes12020296
![]() |
[38] |
J. Jin, Y. Yu, L. Wei, Mouse4mC-BGRU: Deep learning for predicting DNA N4-methylcytosine sites in mouse genome, Methods, 204 (2022), 258–262. https://doi.org/10.1016/j.ymeth.2022.01.009 doi: 10.1016/j.ymeth.2022.01.009
![]() |
[39] |
P. Zheng, G. Zhang, Y. Liu, G. Huang, MultiScale-CNN-4mCPred: a multi-scale CNN and adaptive embedding-based method for mouse genome DNA N4-methylcytosine prediction, BMC Bioinformatics, 24 (2023). https://doi.org/10.1186/s12859-023-05135-0 doi: 10.1186/s12859-023-05135-0
![]() |
[40] |
T. Nguyen-Vo, Q. H. Trinh, L. Nguyen, P. Nguyen-Hoang, S. Rahardja, B. P. Nguyen, i4mC-GRU: Identifying DNA N4-Methylcytosine sites in mouse genomes using bidirectional gated recurrent unit and sequence-embedded features, Comput. Struct. Biotechnol. J., 21 (2023), 3045–3053. https://doi.org/10.1016/j.csbj.2023.05.014 doi: 10.1016/j.csbj.2023.05.014
![]() |
[41] | C. Szegedy, W. Liu, Y. Jia, P. Sermanet, S. Reed, D. Anguelov, et al., Going deeper with convolutions, in 2015 IEEE Conference on Computer Vision and Pattern Recognition (CVPR), IEEE, (2015), 1–9. https://doi.org/10.1109/CVPR.2015.7298594 |
[42] | X. Li, W. Wang, X. Hu, J. Yang, Selective kernel networks, in 2019 IEEE/CVF Conference on Computer Vision and Pattern Recognition (CVPR), (2019), 510–519. https://doi.org/10.1109/CVPR.2019.00060 |
[43] | S. Woo, S. Debnath, R. Hu, X. Chen, Z. Liu, I. S. Kweon, et al., ConvNeXt V2: Co-Designing and scaling ConvNets with masked autoencoders, in 2023 IEEE/CVF Conference on Computer Vision and Pattern Recognition (CVPR), (2023), 16133–16142. https://doi.org/10.1109/CVPR52729.2023.01548 |
[44] | A. G. Howard, M. Zhu, B. Chen, D. Kalenichenko, W. Wang, T. Weyand, et al., MobileNets: efficient convolutional neural networks for mobile vision applications, preprint, arXiv: 1704.04861. |
[45] |
P. Ye, Y. Luan, K. Chen, Y. Liu, C. Xiao, Z. Xie, MethSMRT: an integrative database for DNA N6-methyladenine and N4-methylcytosine generated by single-molecular real-time sequencing, Nucleic Acids Res., 45 (2017), 85–89. https://doi.org/10.1093/nar/gkw950 doi: 10.1093/nar/gkw950
![]() |
[46] |
L. Fu, B. Niu, Z. Zhu, S. Wu, W. Li, CD-HIT: accelerated for clustering the next-generation sequencing data, Bioinformatics, 28 (2012), 3150–3152. https://doi.org/10.1093/bioinformatics/bts565 doi: 10.1093/bioinformatics/bts565
![]() |
[47] | W. Chen, H. Tang, J. Ye, H. Lin, K. Chou, iRNA-PseU: Identifying RNA pseudouridine sites, Mol. Ther.-Nucleic Acids, 5 (2016), 332. |
[48] |
T. Nguyen-Vo, Q. H. Nguyen, T. T. T. Do, T. Nguyen, S. Rahardja, B. P. Nguyen, iPseU-NCP: Identifying RNA pseudouridine sites using random forest and NCP-encoded features, BMC Genomics, 20 (2019). https://doi.org/10.1186/s12864-019-6357-y doi: 10.1186/s12864-019-6357-y
![]() |
[49] | A. S. Nair, S. P. Sreenadhan, A coding measure scheme employing electron-ion interaction pseudopotential (EⅡP), Bioinformation, 1 (2006), 197–202. |
[50] | R. Avenash, P. Viswanath, Semantic segmentation of satellite images using a modified CNN with hard-swish activation function, in Proceedings of the 14th International Joint Conference on Computer Vision, Imaging and Computer Graphics Theory and Applications, (2019), 413–420. |
[51] | K. He, X. Zhang, S. Ren, J. Sun, Deep residual learning for image recognition, in 2016 IEEE Conference on Computer Vision and Pattern Recognition (CVPR), (2016), 770–778. https://doi.org/10.1109/CVPR.2016.90 |
[52] |
A. Krizhevsky, I. Sutskever, G. E. Hinton, ImageNet classification with deep convolutional neural networks, Commun. ACM, 60 (2017), 84–90. https://doi.org/10.1145/3065386 doi: 10.1145/3065386
![]() |
[53] | S. Ioffe, C. Szegedy, Batch normalization: Accelerating deep network training by reducing internal covariate shift, preprint, arXiv: 1502.03167. |
[54] | J. L. Ba, J. R. Kiros, G. E. Hinton, Layer normalization, preprint, arXiv: 1607.06450. |
[55] |
M. Sokolova, G. Lapalme, A systematic analysis of performance measures for classification tasks, Inf. Process. Manage., 45 (2009), 427–437. https://doi.org/10.1016/j.ipm.2009.03.002 doi: 10.1016/j.ipm.2009.03.002
![]() |
[56] |
H. Lv, F. Dao, D. Zhang, Z. Guan, H. Yang, W. Su, et al., iDNA-MS: An integrated computational tool for detecting DNA modification sites in multiple genomes, iScience, 23 (2020), 100991. https://doi.org/10.1016/j.isci.2020.100991 doi: 10.1016/j.isci.2020.100991
![]() |
[57] | A. Vaswani, N. Shazeer, N. Parmar, J. Uszkoreit, L. Jones, A. N. Gomez, et al., Attention is all you need, preprint, arXiv: 1706.03762v5. |
1. | Xiaomeng Ma, Zhanbing Bai, Sujing Sun, Stability and bifurcation control for a fractional-order chemostat model with time delays and incommensurate orders, 2022, 20, 1551-0018, 437, 10.3934/mbe.2023020 |
Parameters | Description | Values | Units |
k | Saturation constant | 1.75 | gl−1 |
Q | Dilution rate | 0.02 | l2gr−1 |
µ | Maximum growth rate | 0.3 | h−1 |
γ | Constant in yield coefficient | 0.01 | – |
β | Constant in yield coefficient | 5.25 | lg−1 |
S0 | Input concentration of substrate | 1 | gl−1 |