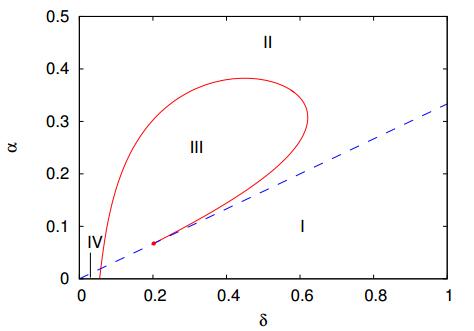
Many tests for comparing survival curves have been proposed over the last decades. There are two branches, one based on weighted log-rank statistics and other based on weighted Kaplan-Meier statistics. If we carefully choose the weight function, a substantial increase in power of tests against non-proportional alternatives can be obtained. However, it is difficult to specify in advance the types of survival differences that may actually exist between two groups. Therefore, a combination test can simultaneously detect equally weighted, early, late or middle departures from the null hypothesis and can robustly handle several non-proportional hazard types with no a priori knowledge of the hazard functions. In this paper, we focus on the most used and the most powerful test statistics related to these two branches which have been studied separately but not compared between them. Through a simulation study, we compare the size and power of thirteen test statistics under proportional hazards and different types of non-proportional hazards patterns. We illustrate the procedures using data from a clinical trial of bone marrow transplant patients with leukemia.
Citation: María del Carmen Pardo, Beatriz Cobo. Comparison of methods to testing for differential treatment effect under non-proportional hazards data[J]. Mathematical Biosciences and Engineering, 2023, 20(10): 17646-17660. doi: 10.3934/mbe.2023784
[1] | Mahvish Samar, Xinzhong Zhu . Structured conditioning theory for the total least squares problem with linear equality constraint and their estimation. AIMS Mathematics, 2023, 8(5): 11350-11372. doi: 10.3934/math.2023575 |
[2] | Salim Bouzebda, Amel Nezzal . Uniform in number of neighbors consistency and weak convergence of kNN empirical conditional processes and kNN conditional U-processes involving functional mixing data. AIMS Mathematics, 2024, 9(2): 4427-4550. doi: 10.3934/math.2024218 |
[3] | Salim Bouzebda, Amel Nezzal, Issam Elhattab . Limit theorems for nonparametric conditional U-statistics smoothed by asymmetric kernels. AIMS Mathematics, 2024, 9(9): 26195-26282. doi: 10.3934/math.20241280 |
[4] | Dayang Dai, Dabuxilatu Wang . A generalized Liu-type estimator for logistic partial linear regression model with multicollinearity. AIMS Mathematics, 2023, 8(5): 11851-11874. doi: 10.3934/math.2023600 |
[5] | Lingsheng Meng, Limin Li . Condition numbers of the minimum norm least squares solution for the least squares problem involving Kronecker products. AIMS Mathematics, 2021, 6(9): 9366-9377. doi: 10.3934/math.2021544 |
[6] | Muhammad Nauman Akram, Muhammad Amin, Ahmed Elhassanein, Muhammad Aman Ullah . A new modified ridge-type estimator for the beta regression model: simulation and application. AIMS Mathematics, 2022, 7(1): 1035-1057. doi: 10.3934/math.2022062 |
[7] | Oussama Bouanani, Salim Bouzebda . Limit theorems for local polynomial estimation of regression for functional dependent data. AIMS Mathematics, 2024, 9(9): 23651-23691. doi: 10.3934/math.20241150 |
[8] | Sizhong Zhou, Jiang Xu, Lan Xu . Component factors and binding number conditions in graphs. AIMS Mathematics, 2021, 6(11): 12460-12470. doi: 10.3934/math.2021719 |
[9] | Jian Yang, Yuefen Chen, Zhiqiang Li . Some sufficient conditions for a tree to have its weak Roman domination number be equal to its domination number plus 1. AIMS Mathematics, 2023, 8(8): 17702-17718. doi: 10.3934/math.2023904 |
[10] | Salim Bouzebda . Weak convergence of the conditional single index U-statistics for locally stationary functional time series. AIMS Mathematics, 2024, 9(6): 14807-14898. doi: 10.3934/math.2024720 |
Many tests for comparing survival curves have been proposed over the last decades. There are two branches, one based on weighted log-rank statistics and other based on weighted Kaplan-Meier statistics. If we carefully choose the weight function, a substantial increase in power of tests against non-proportional alternatives can be obtained. However, it is difficult to specify in advance the types of survival differences that may actually exist between two groups. Therefore, a combination test can simultaneously detect equally weighted, early, late or middle departures from the null hypothesis and can robustly handle several non-proportional hazard types with no a priori knowledge of the hazard functions. In this paper, we focus on the most used and the most powerful test statistics related to these two branches which have been studied separately but not compared between them. Through a simulation study, we compare the size and power of thirteen test statistics under proportional hazards and different types of non-proportional hazards patterns. We illustrate the procedures using data from a clinical trial of bone marrow transplant patients with leukemia.
The gloomy consequences of rapid population growth and environmental degradation have been a major public concern, probably first expressed explicitly in the work of Thomas Malthus [1], who believed that informed public policies could mitigate or even avoid altogether the happening of those circumstances. Following this line, in the 1970s the Club of Rome commissioned a major enterprise aiming at modeling the interactions between the earth and human systems using massive computer simulations capable of producing detailed near-future scenarios [2,3]. Although efforts in that direction are still ongoing [4], this approach is somewhat abstruse as the complexity of the simulated models rivals that of the real-world, thus making it difficult to untangle the effects of the model parameters. An alternative approach to address such concerns, which we adopt here, is the study of minimal models that link the population dynamics and the resource dynamics. Works in this line typically build on Lotka-Volterra predator-prey models [5], where man is seen as the predator and the resource base as the prey [6,7].
Yet the predator-prey approach to human-nature dynamics misses the important fact that humans do not primarily prey on nature but modify it. In fact, humans are the ultimate ecosystem engineers, who have shaped the world into a more hospitable place for themselves, most often with unlooked-for long term effects [8]. One such effect is the collapse of human societies likely caused by habitat destruction and overpopulation [9,10], hence the public concern on this matter. Accordingly, here we argue that the baseline population dynamics model that is more suitable to address human-nature interactions is the ecosystem engineers model [11,12], rather than the predator-prey model. The feature of the population dynamics of ecosystem engineers that makes this approach well suited to model those interactions is that the growth of the population is determined by the availability of usable habitats, which in turn are created by the engineers through the modification, and consequent destruction, of virgin habitats.
The usefulness of the concept of ecosystem engineers as organisms that change their environment [11,12] is somewhat controversial since all species modify their environments one way or another and, in that sense, ubiquity may be equated to nonutility (see [13] for a lucid discussion on the criticisms to the concept of organisms as ecosystem engineers). From a mathematical perspective, however, the explicit incorporation of abiotic interactions between organisms and environment [14] alters their population dynamics resulting in a density-dependent carrying capacity that produces a very rich dynamic behavior both in the continuous-time [12] and the discrete-time formulations [15].
More pointedly, the Gurney and Lawton model for the population dynamics of ecosystem engineers describes the time evolution of the density of engineers and of the habitats, which can exist in three different forms: virgin, usable and degraded. The transition of a habitat from the virgin form to the usable form occurs due to the work of the engineers. The usable habitats then degrade and eventually recover to become virgin habitats again. Virgin and degraded habitats are unsuitable for the growth of the population. To expedite the numerical analysis, here we use a discrete-time approach where the logistic growth equation of the original model is replaced by the Beverton-Holt equation [16]. The weak nonlinearity of this equation prevents the large fluctuations on the population size produced by the Ricker equation [17], which may result in a spurious chaotic dynamics [15].
We find that the survival of the population depends on two independent factors, viz., the competence of the engineers to transform natural resource base (e.g., native forests) into useful goods (e.g., farms) and the population growth rate per generation. Survival is guaranteed provided that the product of these two factors is greater than the decay rate per generation of the usable habitats. However, survival is not without adversities in the case the regeneration rate per generation of the degraded habitats is too slow. In this case, the dynamics exhibits rounds of prosperity and collapse, in which the population reaches vanishing small densities. Extinction does not occur because the fixed-point associated to it is unstable. The oscillatory regime is favored by large population growth rates.
Most interestingly, we find that increase of the efficiency of the engineers to explore the resource base, thus modeling a high-tech society scenario where a few individuals can extract and produce the goods needed for survival, eliminates the dangerous oscillatory patterns of feast and famine and leads to a smooth adjustment between population and resources. This finding supports the viewpoint of growth optimists that technological progress may avoid collapse.
In the case the resources are non-renewable, the long-term outcome is a doomsday scenario in which the ecosystem is reduced to degraded habitats only. Since the population is not immediately affected by the disappearance of the virgin habitats, we can interpret the usable habitats as a kind of wealth, i.e., surpluses that humans accumulate and that may extend their survival well beyond the point where the natural resources are exhausted. This observation offers a connection between ecosystem engineers models and economic models of human-nature interactions [7].
The rest of the paper is organized as follows. In Section 2, we introduce the discrete time version of Gurney and Lawton model of ecosystem engineers and derive the recursion equations for the density of engineers as well as for the fractions of the three forms of habitats. In that section we also derive and begin the analysis of the local stability of the fixed point solutions of those equations. Section 3 presents the results of the numerical study of the eigenvalues of the Jacobian matrices that determine the local stability of the fixed points as well as the results of the numerical iteration of the recursion equations. Finally, Section 4 is reserved for our concluding remarks.
The population dynamics of ecosystem engineering was modeled originally by Gurney and Lawton in the 1990s using a continuous-time model [12], while discrete-time versions were considered only much more recently [15,18]. We recall that the main advantage of discrete-time formulations is that they can easily be extended to incorporate a spatial dependence of the dynamic variables [19], as well as of the model parameters, as neatly demonstrated in the classic studies of the spatial dynamics of host-parasitoid systems [20,21] (see [22,23] for more recent contributions). However, discrete-time nonlinear systems usually exhibit chaotic behavior, which is somewhat problematic from a biological perspective [24] and are not observed in their continuous-time counterparts [25]. Here we show that this drawback can be circumvented by a suitable choice of the equation that determines the growth of the population. In particular, whereas in previous studies the logistic equation of the continuous-time Gurney and Lawton model was replaced by the highly nonlinear Ricker equation [17], here we use the growth equation of Beverton and Holt [16], whose much weaker nonlinearity greatly limits the between-generations fluctuations and produces a stauncher representation of the continuous-time model.
We assume that the population of engineers at generation t is composed of Et individuals and that the carrying capacity of the environment depends on the number of units of usable habitats available at generation t, which we denote by Ht. Thus, using the Beverton-Holt equation we write the expected number of engineers at generation t+1 as
Et+1=rEt/(1+Et/Ht) | (2.1) |
where r>1 is the intrinsic growth rate of the population of engineers and the time-dependent carrying capacity is (r−1)Ht. This model describes a system of ecosystem engineers due to the assumption that the usable habitats are produced by engineers working on virgin habitats [12]. More pointedly, assuming that there are Vt units of virgin habitats at generation t, a fraction C(Et)Vt of them will be transformed in usable habitats by the engineers at the next generation t+1. Here C(Et) is any function that satisfies 0≤C(Et)≤1 for all Et and C(0)=0. In other words, if there are no engineers at generation t, then virgin habitats cannot be transformed in usable ones. Hence C(Et) measures the efficiency of the engineer population to build usable habitats from the raw materials provided by the virgin habitats.
Usable habitats decay into degraded habitats that are useless to the engineers, in the sense that they are inhabitable and lack the raw materials needed to build usable habitats. Denoting by δ∈[0,1] the probability that a unit of usable habitat decays and becomes a unit of degraded habitat in one generation, we write the expected number of units of usable habitats at generation t+1 as
Ht+1=(1−δ)Ht+C(Et)Vt. | (2.2) |
In this paper we assume that the decay probability δ is a density independent parameter. We recall that in the Gurney and Lawton model the resources are represented by the virgin habitats, whose probability of change into usable habitats is density dependent, i.e. C=C(Et). For example, in an island scenario, the virgin habitats can be thought of as the native forests whereas the usable habitats are the lands cleared for crops, whose degradation, due mainly to erosion and soil depletion of nutrients, is more suitably modeled by a constant decay probability δ, rather than by a density-dependent one (i.e., δ=δ(Et)). However, in an economic context where the habitats represent, for instance, oil wells, the decay probability should be modeled by a density-dependent parameter, of course.
To take into account the possibility that the degraded habitats will eventually recover and become virgin habitats again, we introduce the parameter ρ∈[0,1] that gives the probability that one unit of degraded habitat becomes one unit of virgin habitat in one generation. Thus, denoting the units of degraded habitats at generation t by Dt we write
Dt+1=(1−ρ)Dt+δHt. | (2.3) |
Thus ρ is the regeneration rate per generation that measures the renewability of the resource base. Finally, recalling that virgin habitats are destroyed by the action of the engineers and created by the restoration of the degraded habitats, we write the recursion equation for the expected number of units of virgin habitats as
Vt+1=[1−C(Et)]Vt+ρDt, | (2.4) |
which completes the description of the feedback interactions between habitats and engineers.
Since habitats can only be transformed from one form to another, the total number of units of habitats Vt+1+Ht+1+Dt+1=Vt+Ht+Dt=T is conserved. This fact allows us to introduce the habitat fractions vt≡Vt/T, ht≡Ht/T and dt≡Dt/T that satisfy vt+ht+dt=1 for all t. Accordingly, we define also the density of engineers et=Et/T that, differently from the habitat fractions, may take on values greater than 1. In terms of these intensive quantities, the above recursion equations are rewritten as
et+1=ret/(1+et/ht) | (2.5) |
ht+1=(1−δ)ht+c(et)vt | (2.6) |
vt+1=ρ(1−vt−ht)+[1−c(et)]vt, | (2.7) |
where we have used dt=1−vt−ht and c(et)≡C(Tet).
To complete the model we must specify the density-dependent probability c(et), which measures the engineers' efficiency to transform virgin habitats into usable ones. The function c(et) incorporates the cooperation strategies used to build structures (e.g., beaver dams, termite mounds and anthills) to respond to external and internal threats to the population survival [26,27]. For humans, this function incorporates the beneficial effects (from their perspective) of the technological advancements that enable a more efficient harvesting of natural resources [28]. Here we consider the continuous function
c(et)={αet+(1−α)e2tif et<1.1if et≥1. | (2.8) |
where α∈[0,1] is the efficiency parameter that measures the competence of the engineers in transforming natural resources into useful goods. The range of variation of α guarantees that c(et)≤1 regardless of the value of et. For α≪1 we have a scenario where the efficient exploration of the virgin habitats requires a high density of engineers. In this low-tech scenario, we expect to observe an Allee effect, in which the population quickly goes extinct below a critical density [29]. We note that when et>1 all the available virgin habitats are transformed into usable ones in just a single generation. This arbitrary threshold value does not produce any significant effect on our results since we find that et<1 at all times in the parameter regions of interest, i.e., in the regions close to the transitions between the fixed points and the invariant curves attractors.
In this section we study the fixed-point solutions of the system of recursion equations (2.5)-(2.7) that are obtained by setting et+1=et=e∗, ht+1=ht=h∗ and vt+1=vt=v∗. Next we consider separately the two cases, e∗=0 (zero-engineers fixed point) and e∗>0 (finite-engineers fixed point). The local stability of the fixed point solutions are determined by the eigenvalues of the Jacobian matrix of the system of recursion equations (2.5), (2.6), (2.7),
Jet=[r/(1+xt)2r[xt/(1+xt)]20vtc′(et)1−δc(et)−vtc′(et)−ρ1−ρ−c(et)], | (2.9) |
where xt=et/ht and c′(et)=dc(et)/det.
In the absence of the engineers (i.e., e∗=0) all usable habitats will degrade and then recover to virgin habitats (provided that ρ>0), so we have h∗=0 and v∗=1. To circumvent the indetermination of the ratio e∗/h∗, we write an equation for xt dividing Eq (2.5) by Eq (2.6),
xt+1=rxt(1+xt)[1−δ+xtvtˆc(et)], | (2.10) |
where ˆc(et)=c(et)/et. Thus the fixed point xt+1=xt=x∗>0 is given by the positive solution of the quadratic equation
(1+x∗)[1−δ+αx∗]=r, | (2.11) |
where we have used ˆc(0)=lime∗→0c(e∗)/e∗=c′(0)=α. The quadratic equation (2.11) has two real roots, one positive and the other negative, for all values of the model parameters. In particular, for δ=α(r−1) the solution is x∗=r−1 and we can easily show that x∗>r−1 for δ>α(r−1) and x∗<r−1 for δ<α(r−1), a result that will proven helpful to verify the local stability of this fixed point.
In fact, the local stability of the zero-engineers fixed point is determined by requiring that the three eigenvalues of the Jacobian matrix
Je∗=0=[r/(1+x∗)2r[x∗/(1+x∗)]20α1−δ0−α−ρ1−ρ] | (2.12) |
have norms less than 1. The eigenvalues of the Jacobian matrix (2.12) are λ1=r/(1+x∗), λ2=r/(1+x∗)2−αx∗ and λ3=1−ρ. The condition ∣λ1∣<1 is satisfied provided that x∗>r−1, which is guaranteed for δ>α(r−1), whereas the condition ∣λ3∣<1 is always satisfied since ρ<1. The proof that the condition ∣λ2∣<1 is always satisfied whenever λ1<1 is very simple. Since α>0 and x∗>0 we can write λ2<r/(1+x∗)2=λ1/(1+x∗)<1/(1+x∗)<1. Next we need to show that λ2>−1. To do so we use Eq (2.11) to write αx∗=λ1−1+δ so that λ2=1−δ−x∗λ1/(1+x∗)>1−δ−1>−δ>−1.
We note that this stability analysis describes the convergence (or divergence) of the system of recursion equations for et, ht and vt, Eqs (2.5), (2.6) and (2.7), respectively, to the fixed point e∗=h∗=0 and v∗=1 and that Eqs (2.10) and (2.11) were used only to specify the ratio limt→∞et/ht that is required to evaluate the Jacobian (2.9). We mention this because use of recursion equations for et, xt and vt instead, yields a different Jacobian for the zero-engineers fixed point, which exhibits the same eigenvalues λ1 and λ3 as the Jacobian (2.12), but a different eigenvalue λ2. However, the local stability of the fixed point is again determined solely by the norm of the common eigenvalue λ1 so this issue has no effect in our findings regarding the zero-engineers fixed point.
In sum, the condition for the stability of the zero-engineers fixed point is δ<α(r−1), which, as expected, does not depend on the regeneration rate ρ since there is no shortage of virgin habitats (i.e., vt≈1) in the vicinity of this fixed point.
Since e∗>0 we have h∗=e∗/(r−1) and v∗=1−e∗γ/(r−1) with e∗ given by the solution of the equation
δe∗=c(e∗)[r−1−e∗γ], | (2.13) |
where we have introduced the notation γ=(1+δ/ρ).
Let us assume that e∗<1 so we can use Eq (2.8) to rewrite Eq (2.13) as the quadratic equation
(1−α)γ(e∗)2−e∗[(1−α)(r−1)−αγ]+δ−α(r−1)=0. | (2.14) |
The local stability of the two solutions of this equation is determined by the the Jacobian matrix (2.9) that is rewritten as
Je∗<1=[1/r(r−1)2/r0v∗c′(e∗)1−δc(e∗)−v∗c′(e∗)−ρ1−ρ−c(e∗)]. | (2.15) |
The main difficulty of the analysis of the eigenvalues of this Jacobian matrix is that two eigenvalues are complex, leading to the enthralling dynamic behavior exhibited by the recursion equations (2.5), (2.6), (2.7) with the prescription (2.8). However, this problem can easily be solved numerically as follows. First we determine the critical points of the characteristic cubic polynomial, which allows us to obtain one real root of the characteristic equation with high numerical accuracy using the bisection method. (We recall that the critical points of a function are those values of the variable where the slope of the function is zero.) Then this root is factored out and the remaining roots of the characteristic equation are found by solving a quadratic equation. Actually, in the case of complex roots, we do no need to solve this quadratic equation since the local stability is determined by the norm of those roots that is given by the square root of the ratio between the constant and the quadratic coefficient of the quadratic equation. The fixed point solution is locally stable provided that the norms of all three roots of the characteristic polynomial are less than 1. We find that the smaller root of the fixed-point equation (2.14) is always locally unstable.
Now we consider the case e∗>1. Equation (2.13) yields
e∗=r−11+δ(1+1/ρ) | (2.16) |
that holds for δ<(r−2)/(1+1/ρ). In this case, the Jacobian matrix (2.9) becomes
Je∗>1=[1/r(r−1)2/r001−δ10−ρ−ρ]. | (2.17) |
Hence λ1=1/r<1 is always a root of the characteristic polynomial and the other two roots are given by the quadratic equation g(λ)=λ2−λ(1−ρ−δ)+ρδ=0. In the case of complex roots, we have ∣λ∣2=ρδ<1. In the case of real roots, we use that g(1)>0 and g(−1)>0 and that the critical point of g(λ) is between −1 and 1 to guarantee that the absolute value of those roots are less than 1. Hence the fixed point (2.16) is always locally stable.
The local stability of the fixed points e∗=0 and 0<e∗<1 is determined by the requirement that the norms of the eigenvalues of the Jacobian matrices (2.12) and (2.15), respectively, are less than 1. Accordingly, Figure 1 shows the regions of stability of those fixed points. There is a large region in the space of parameters (δ,α) where those fixed points are unstable and the discrete-time dynamics is attracted to invariant curves, so the solutions exhibit oscillatory behavior (region Ⅲ). The drop-shaped region delimits the regions of stability of the finite-engineers fixed point and since this fixed point changes stability via a pair of complex eigenvalues with unit modulus, the delimiting curve of region Ⅲ signals the onset of Neimark-Sacker bifurcations [30].
To better understand the results shown in Figure 1, we first examine whether Eq (2.14) admits a physical solution with a vanishingly small density of engineers. In fact, for e∗≪1 we have
e∗≈α(r−1)−δαγ−(1−α)(r−1), | (3.1) |
so that the density of engineers vanishes continuously as the line α=δ/(r−1) is approached from the region of large α, provided the denominator in Eq (3.1) is positive. i.e., αγ−(1−α)(r−1)>0. In Figure 1 we indicate with a red bullet the point δ=δc≈0.202 and α=αc≈0.067 at which both the numerator and the denominator in Eq (3.1) vanish, meaning that e∗=0 is a double root of the quadratic equation (2.14). Thus, there is a continuous transition between the finite and the zero-engineers fixed point at α=δ/(r−1) for δ>δc. We note that since the fixed point e∗=0 exists for all values of the parameters and is never destroyed, the dashed line in Figure 1 signals de onset of transcritical bifurcations [31].
Next, we focus on the fixed-point solutions at the transition line α=δ/(r−1). In this case, Eq (2.14) has the solution e∗=0 and
e∗=(1−α)(r−1)−αγ(1−α)γ, | (3.2) |
provided that α>(r−1)/((r−1)−γ), which guarantees that e∗<1. Otherwise, we have e∗=(r−1)/(δ+γ)>1 (see Section 2.1.2). Figure 2 shows the finite-engineers fixed points at the transition, where the stable and unstable segments are flagged with different colors. The figure reveals that the fixed point e∗>0 is stable for small δ, as expected, and that it is also stable close to the point (δc,αc). This indicates that this point does not belong to the drop-shaped curve shown in Figure 1, which delimits the regions of stability of the finite-engineers fixed point. This facet will become much more evident when we consider larger values of the recovery probability ρ.
Another interesting feature of Figure 1 is the appearance of a region of bistability of the two fixed points for small values of δ and α<δ/3 (region Ⅳ). The dependence on the initial density of engineers, as well as on the initial habitats fractions, that characterizes the bistability regime results in an Allee effect where the environment cannot sustain a low density of engineers, as the change of the virgin habitats into usable ones can only be achieved by high-density populations.
Figure 3 illustrates the time dependence of the density of engineers et and of the fraction of virgins habitats vt for three representative regions of Figure 1, where the zero-engineers fixed point (e∗=0) is unstable, i.e, for δ<α(r−1), so the results do not depend on the initial conditions. The time dependence of the fraction of usable habitats ht is similar to that of et. The recursion equations (2.5)–(2.7) were iterated using quadruple precision. The oscillatory convergence to the finite-engineers fixed point (e∗>0) is the effect of the complex eigenvalues of the Jacobian matrix (2.15). These oscillations may lead to extremely low values of the density of engineers (e.g., et on the order of 10−10), hence the need of a high precision numerical scheme to iterate the recursion equations. Since e∗=0 is unstable, the population eventually recovers from these near-extinction events as illustrated in the middle and right panels of Figure 3.
The nature of the transition between the oscillatory solutions and the zero-engineers fixed point is clarified in Figure 4 where we fix the decay rate per generation to δ=0.15 and show et and vt for different values of α in distinct panels. The left and middle panels, which show results for values of α very close to the stability boundary α=0.05, reveal what happens: the period of the oscillations increases as that boundary is approached and diverges when the zero-engineers fixed point becomes stable, so that e∗=0 and v∗=1 for all times. The right panel in Figure 4 reminds us that the oscillatory solutions are not necessarily associated to near-extinction events and that they can reproduce smooth oscillations with the usual phase shift of one-quarter of a cycle between engineers and virgin habitats, which characterizes the predator-prey models [32].
Next we consider the effects of varying the parameters r and ρ that were kept fixed in the above analysis. Figure 5 shows the regions of stability of the fixed points for three values of the intrinsic growth rate r. To keep the size of the region where the zero-engineers fixed point is stable we use the scaled variable δ/(r−1) as the independent variable. The results indicate that increase of r increases the size of the regions where the oscillatory solutions appear and decreases the size of the regions of bistability, so variation of r produces quantitative effects only. This is in stark contrast with the effects of varying the regeneration rate per generation ρ shown in Figure 6. In fact, increase of ρ decreases the size of the region of oscillatory solutions, which eventually disappears altogether for large ρ, since there is no region left in the space of parameters where the fixed points e∗>0 and e∗=0 are simultaneously unstable. For instance, the curve for ρ=0.3 shown in the right panel of Figure 6 is given by the condition that the discriminant of the quadratic equation (2.14) is zero, so for large ρ the finite-engineers fixed point is locally stable wherever it is real. The piecewise curve for ρ=0.1 is given by the composition of the conditions that e∗ is real and that the norms of the eigenvalues of the Jacobian (2.15) are less than 1. In addition, increase of ρ greatly increases the region of bistability as well as the region where e∗>1.
To conclude, we consider now a simple but instructive scenario where the natural resources are not renewable, i.e., ρ=0. Figure 7 shows the time evolution of the population towards the doomsday fixed point v∗=e∗=h∗=0 and d∗=1 where only the degraded habitats are left. We have not considered this fixed point in Section 2.1.1 because it exists and is stable solely for ρ=0 and δ>0, regardless of the values the other model parameters. The interesting aspect this figure highlights is that the population survives for a long time after the irreversible disappearance of the virgin habitats, thus revealing that the usable habitats introduce a delay on the influence of the virgin habitats on the population that is absent in the usual predator-prey models. However, this delay appears in more sophisticated models of human-nature interactions in which humans accumulate surpluses (i.e., wealth) and use them when natural resources are scarce or unavailable [7].
The derivation of the discrete-time recursion equations (2.5)–(2.8) assumes non-overlapping generations, which is clearly not the case for human populations. Our justification to use this approach is purely pragmatic since it is much easier and much less error-prone to iterate those recursions than to solve numerically the differential equations of a continuous-time model, particularly in the region of reversible collapses where the density of engineers is very close to zero for a very long time. However, as the solutions do not exhibit short-time fluctuations and are all smooth in the scale of a few generations we do not expect any significant differences between the discrete and the continuous time approaches. In addition, the discrete-time approach can easily be extended to describe space-dependent problems, resulting in coupled map lattices that can be thoroughly studied numerically. In particular, in this context a different type of population collapse can be observed when the expanding colonies of engineers reach the boundaries of their territories, thus ending the exploration of new virgin habitats and resulting in a sharp drop on the population density [18].
In agreement with the findings for the predator-prey type models [6,7], we find that a key parameter to determine the long-term outcome of the population dynamics of ecosystem engineers is the regeneration rate per generation ρ, which determines the renewability of the resource base (see Figure 6). Rounds of prosperity, collapse and revival occur in the case the degraded habitats have a slow regeneration rate. In the extreme case of non-renewable resources (i.e., ρ=0), the outcome of the dynamics is the irreversible collapse of the entire ecosystem (see Figure 7). In the other extreme, when the degraded habitats rapidly recover into virgin habitats, the outcome of the dynamics is determined by the basins of attraction of the finite and zero-engineers fixed points, which are never simultaneously unstable in this extreme.
The local stability analysis of the zero-engineers fixed point yields a simple condition for the survival of the population, viz., the product of the parameter that measures the effective population growth rate (i.e., r−1) and the parameter that measures the efficiency of the transformation of virgin into usable habitats (i.e., α) must be greater than the decay rate per generation of the usable habitats, i.e., α(r−1)>δ. Hence increase of the efficacy of the engineers to explore the virgin habitats or increase of their intrinsic effective growth rate will both guarantee the survival of the population, regardless of the regeneration rate ρ>0 of the degraded habitats. The characteristics of the steady-states for large r and large α, however, are completely distinct.
On the one hand, increase of r favors oscillatory solutions characterized by long periods of time when et≪1, which we refer to as reversible collapses. These collapses are reversible because they occur in regions of the model parameters where the zero-engineers fixed point is unstable. This scenario is expected since a large value of r produces a large density of engineers, which, according to the prescription (2.8), can transform all virgin habitats in a single generation, thus producing the oscillatory pattern of feast and famine illustrated in the left and middle panels of Figure 4.
On the other hand, increase of α favors the finite-engineers fixed points and leads to a stable balance between population and resources. In our setup, a small α corresponds to a low-tech society where the exploration of the virgin habitats requires a high density of individuals. Interestingly, our model predicts that technology improvements that allow a small population to explore efficiently the available natural resources, a situation that is modeled by increasing α while keeping all the other parameters fixed, can eliminate the dangerous rounds of prosperity, collapse and revival.
This result is especially notable because it neatly expresses the argument put forward by the growth optimists, namely, that technological progress can reduce the impact of resource depletion and avoid environmental and economic collapse [33]. This finding highlights the advantage of the ecosystem engineers approach over the traditional predator-prey approach to model the ecological and economic aspects of human-nature dynamics.
J.F.F. is supported in part by Grant No. 2017/23288-0, Fundação de Amparo à Pesquisa do Estado de São Paulo (FAPESP) and by Grant No. 305058/2017-7, Conselho Nacional de Desenvolvimento Científico e Tecnológico (CNPq). GML is supported by a CAPES scholarship.
All authors declare no conflicts of interest in this paper.
[1] |
R. S. Herbst, P. Baas, D. W. Kim, E. Felip, J. L. Pérez-Gracia, J. Y. Han, et al., Pembrolizumab Versus Docetaxel for Previously Treated, PDL1-Positive, Advanced Non-Small-Cell Lung Cancer (KEYNOTE-010): A Randomised Controlled Trial, The Lancet, 387 (2016), 1540–-1550. https://doi.org/10.1016/S0140-6736(15)01281-7 doi: 10.1016/S0140-6736(15)01281-7
![]() |
[2] |
M. S. Pepe, T. R. Fleming, Weighted Kaplan-Meier statistics: A class of distance tests for censored survival data, Biometrics, 45 (1989), 497–507. http://dx.doi.org/10.2307/2531492 doi: 10.2307/2531492
![]() |
[3] |
B. Huang, P. F. Kuan, Comparison of the restricted mean survival time with the hazard ratio in superiority trials with a time-to-event end point, Pharmaceut. Statist., 17 (2018), 202–213. http://dx.doi.org/10.1002/pst.1846 doi: 10.1002/pst.1846
![]() |
[4] |
S. G. Self, An adaptive weighted log-rank test with application to cancer prevention and screening trials, Biometrics, 47 (1991), 975–986. http://dx.doi.org/10.2307/2532653 doi: 10.2307/2532653
![]() |
[5] |
T. R. Fleming, D. P. Harrington, A class of hypothesis tests for one and two samples of censored survival data, Commun. Statist. Theory Methods, 13 (1981), 2469–2486. http://dx.doi.org/10.1080/03610928108828073 doi: 10.1080/03610928108828073
![]() |
[6] |
D. P. Harrington, T. R. Fleming, A class of rank test procedures for censored survival data, Biometrika, 69 (1982), 553–566. http://dx.doi.org/10.1093/biomet/69.3.553 doi: 10.1093/biomet/69.3.553
![]() |
[7] | T. R. Fleming, D. P. Harrington, Counting Processes and Survival Analysis, New York: Wiley, (1991). http://dx.doi.org/10.1002/9781118150672 |
[8] |
J. W. Lee, Some versatile tests based on the simultaneous use of weighted log-rank statistics, Biometrics, 52 (1996), 721–725. http://dx.doi.org/10.2307/2532911 doi: 10.2307/2532911
![]() |
[9] |
S. H. Lee, On the versatility of the combination of the weighted log-rank statistics, Comput. Statist. Data Anal., 51 (2007), 6557–6564. http://dx.doi.org/10.1016/j.csda.2007.03.006 doi: 10.1016/j.csda.2007.03.006
![]() |
[10] |
T. G. Karrison, Versatile tests for comparing survival curves based on weighted log-rank statistics, Stat. J., 16 (2016), 678–690. http://dx.doi.org/10.1177/1536867X1601600308 doi: 10.1177/1536867X1601600308
![]() |
[11] |
Y. Shen, J. Cai, Maximum of the Weighted Kaplan-Meier tests with application to cancer prevention and screening trials, Biometrics, 57 (2001), 837–843. http://dx.doi.org/10.1111/j.0006-341X.2001.00837.x doi: 10.1111/j.0006-341X.2001.00837.x
![]() |
[12] |
S. H. Lee, Maximum of the weighted Kaplan-Meier tests for the two-sample censored data, J. Statist. Comput. Simul., 81 (2011), 1017–1026. http://dx.doi.org/10.1080/00949651003627753 doi: 10.1080/00949651003627753
![]() |
[13] |
P. Royston, M. K. B. Parmar, A simulation study comparing the power of nine tests of the treatment effect in randomized controlled trials with a time-to-event outcome, Trials, 21 (2020), 315. http://dx.doi.org/10.1186/s13063-020-4153-2 doi: 10.1186/s13063-020-4153-2
![]() |
[14] |
R. S. Lin, J. Lin, S. Roychoudhury, K. M. Anderson, T. Hu, B. Huang, et al., Alternative Analysis Methods for Time to Event Endpoints under Non-proportional Hazards: A Comparative Analysis, Statist. Biopharm. Res., 12 (2020), 187–198. https://doi.org/10.1080/19466315.2019.1697738 doi: 10.1080/19466315.2019.1697738
![]() |
[15] |
S. Roychoudhury, K. M. Anderson, J. Ye, P. Mukhopadhyay, Robust Design and Analysis of Clinical Trials with Nonproportional Hazards: A Straw Man Guidance From a Cross- Pharma Working Group, Statist. Biopharm. Res., 15 (2021), 280–294. http://dx.doi.org/10.1080/19466315.2021.1874507 doi: 10.1080/19466315.2021.1874507
![]() |
[16] |
E. Kaplan, P. Meier, Nonparametric estimation from incomplete observations, J. Amer. Statist. Assoc., 53 (1958), 457–481. http://dx.doi.org/10.1080/01621459.1958.10501452 doi: 10.1080/01621459.1958.10501452
![]() |
[17] |
T. R. Fleming, D. P. Harrington, M. O'Sullivan, Supremum versions of the log-rank and generalized Wilcoxon statistics, J. Am. Stat. Assoc., 82 (1987), 312–320. http://dx.doi.org/10.1080/01621459.1987.10478435 doi: 10.1080/01621459.1987.10478435
![]() |
[18] | W. Yang, W. Haiyan, A. Keaven, R. Satrajit, H. Tianle, L. Hongliu, R package nphsim: Non proportional hazards sample size and simulation, (2017). Available from: https://github.com/keaven/nphsim |
[19] | R. Ristl, N. Ballarini, R package nph: Planning and Analysing Survival Studies under Non-Proportional Hazards, (2020). Available from: https://CRAN.R-project.org/package=nph |
[20] | M. Bofill, G. Gómez, R package SurvBin: Two-sample statistics for binary and time-to-event outcomes, (2020). Available from: https://github.com/MartaBofillRoig/SurvBin |
[21] | H. Uno, L. Tian, M. Horiguchi, A. Cronin, C. Battioui, J. Bell, R package survRM2: Comparing Restricted Mean Survival Time, (2020). Available from: https://CRAN.R-project.org/package=survRM2 |
[22] | J. P Klein, M. L. Moeschberger, J. Yan, R package KMsurv: Data sets from Klein and Moeschberger (1997), Survival Analysis, (2012). Available from: https://CRAN.R-project.org/package=KMsurv |
[23] |
R. S. Lin, L. F. León, Estimation of treatment effects in weighted log-rank tests, Contempor. Clin. Trials Commun., 8 (2017), 147–155. http://dx.doi.org/10.1016/j.conctc.2017.09.004 doi: 10.1016/j.conctc.2017.09.004
![]() |
1. | Balázs Csutak, Gábor Szederkényi, Robust control and data reconstruction for nonlinear epidemiological models using feedback linearization and state estimation, 2025, 22, 1551-0018, 109, 10.3934/mbe.2025006 |