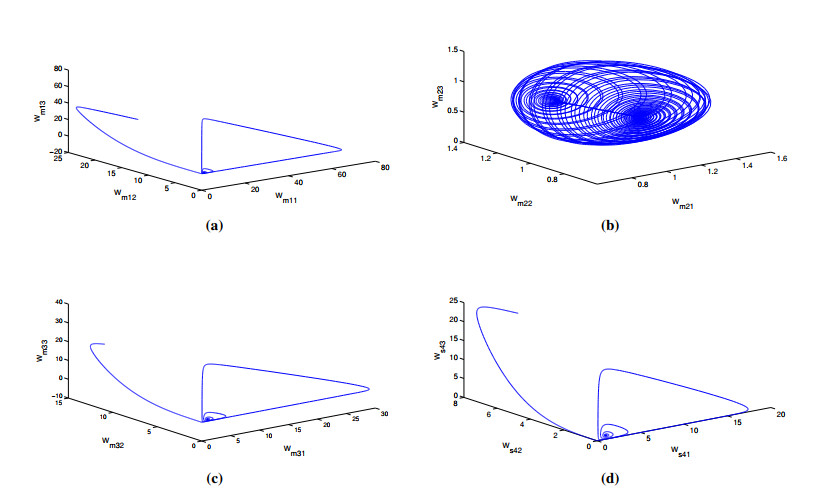
Avian influenza is an infectious viral disease caused by type A virus, which occurs frequently around the world and causes serious economic losses. Therefore, the adaptive control problem is explored in this paper for an avian influenza model in consideration of slaughtering to poultry, educational campaigns to the susceptible human and treatment to the infected human. First, by analyzing the transmission mechanism of avian influenza, a nonlinear adaptive control problem of avian influenza model is formulated, where some errors between model parameters and real values are allowed. Then, the parameters are estimated by constructing adaptive laws, which can be effectively used to design the applicative controllers to achieve the control goals. Besides, the stability of controlled model is analyzed with the aid of Lyapunov stability theory. Finally, numerical examples are proposed to verify the effectiveness and robustness of the designed controllers.
Citation: Ting Kang, Qimin Zhang, Qingyun Wang. Nonlinear adaptive control of avian influenza model with slaughter, educational campaigns and treatment[J]. Electronic Research Archive, 2023, 31(8): 4346-4361. doi: 10.3934/era.2023222
[1] | Honglei Wang, Wenliang Zeng, Xiaoling Huang, Zhaoyang Liu, Yanjing Sun, Lin Zhang . MTTLm6A: A multi-task transfer learning approach for base-resolution mRNA m6A site prediction based on an improved transformer. Mathematical Biosciences and Engineering, 2024, 21(1): 272-299. doi: 10.3934/mbe.2024013 |
[2] | Shanzheng Wang, Xinhui Xie, Chao Li, Jun Jia, Changhong Chen . Integrative network analysis of N6 methylation-related genes reveal potential therapeutic targets for spinal cord injury. Mathematical Biosciences and Engineering, 2021, 18(6): 8174-8187. doi: 10.3934/mbe.2021405 |
[3] | Pingping Sun, Yongbing Chen, Bo Liu, Yanxin Gao, Ye Han, Fei He, Jinchao Ji . DeepMRMP: A new predictor for multiple types of RNA modification sites using deep learning. Mathematical Biosciences and Engineering, 2019, 16(6): 6231-6241. doi: 10.3934/mbe.2019310 |
[4] | Yong Zhu, Zhipeng Jiang, Xiaohui Mo, Bo Zhang, Abdullah Al-Dhelaan, Fahad Al-Dhelaan . A study on the design methodology of TAC3 for edge computing. Mathematical Biosciences and Engineering, 2020, 17(5): 4406-4421. doi: 10.3934/mbe.2020243 |
[5] | Atefeh Afsar, Filipe Martins, Bruno M. P. M. Oliveira, Alberto A. Pinto . A fit of CD4+ T cell immune response to an infection by lymphocytic choriomeningitis virus. Mathematical Biosciences and Engineering, 2019, 16(6): 7009-7021. doi: 10.3934/mbe.2019352 |
[6] | Tamás Tekeli, Attila Dénes, Gergely Röst . Adaptive group testing in a compartmental model of COVID-19*. Mathematical Biosciences and Engineering, 2022, 19(11): 11018-11033. doi: 10.3934/mbe.2022513 |
[7] | Wenli Cheng, Jiajia Jiao . An adversarially consensus model of augmented unlabeled data for cardiac image segmentation (CAU+). Mathematical Biosciences and Engineering, 2023, 20(8): 13521-13541. doi: 10.3934/mbe.2023603 |
[8] | Tongmeng Jiang, Pan Jin, Guoxiu Huang, Shi-Cheng Li . The function of guanylate binding protein 3 (GBP3) in human cancers by pan-cancer bioinformatics. Mathematical Biosciences and Engineering, 2023, 20(5): 9511-9529. doi: 10.3934/mbe.2023418 |
[9] | Xin Yu, Jun Liu, Ruiwen Xie, Mengling Chang, Bichun Xu, Yangqing Zhu, Yuancai Xie, Shengli Yang . Construction of a prognostic model for lung squamous cell carcinoma based on seven N6-methylandenosine-related autophagy genes. Mathematical Biosciences and Engineering, 2021, 18(5): 6709-6723. doi: 10.3934/mbe.2021333 |
[10] | Tahir Rasheed, Faran Nabeel, Muhammad Bilal, Yuping Zhao, Muhammad Adeel, Hafiz. M. N. Iqbal . Aqueous monitoring of toxic mercury through a rhodamine-based fluorescent sensor. Mathematical Biosciences and Engineering, 2019, 16(4): 1861-1873. doi: 10.3934/mbe.2019090 |
Avian influenza is an infectious viral disease caused by type A virus, which occurs frequently around the world and causes serious economic losses. Therefore, the adaptive control problem is explored in this paper for an avian influenza model in consideration of slaughtering to poultry, educational campaigns to the susceptible human and treatment to the infected human. First, by analyzing the transmission mechanism of avian influenza, a nonlinear adaptive control problem of avian influenza model is formulated, where some errors between model parameters and real values are allowed. Then, the parameters are estimated by constructing adaptive laws, which can be effectively used to design the applicative controllers to achieve the control goals. Besides, the stability of controlled model is analyzed with the aid of Lyapunov stability theory. Finally, numerical examples are proposed to verify the effectiveness and robustness of the designed controllers.
The constituent members in a system mainly found in nature can be interacting with each other through cooperation and competition. Demonstrations for such systems involve biological species, countries, businesses, and many more. It's very much intriguing to investigate in a comprehensive manner numerous social as well as biological interactions existent in dissimilar species/entities utilizing mathematical modeling. The predation and the competition species are the most famous interactions among all such types of interactions. Importantly, Lotka [1] and Volterra [2] in the 1920s have announced individually the classic equations portraying population dynamics. Such illustrious equations are notably described as predator-prey (PP) equations or Lotka-Volterra (LV) equations. In this structure, PP/LV model represents the most influential model for interacting populations. The interplay between prey and predator together with additional factors has been a prominent topic in mathematical ecology for a long period. Arneodo et al. [3] have established in 1980 that a generalized Lotka-Volterra biological system (GLVBS) would depict chaos phenomena in an ecosystem for some explicitly selected system parameters and initial conditions. Additionally, Samardzija and Greller [4] demonstrated in 1988 that GLVBS would procure chaotic reign from the stabled state via rising fractal torus. LV model was initially developed as a biological concept, yet it is utilized in enormous diversified branches for research [5,6,7,8]. Synchronization essentially is a methodology of having different chaotic systems (non-identical or identical) following exactly a similar trajectory, i.e., the dynamical attributes of the slave system are locked finally into the master system. Specifically, synchronization and control have a wide spectrum for applications in engineering and science, namely, secure communication [9], encryption [10,11], ecological model [12], robotics [13], neural network [14], etc. Recently, numerous types of secure communication approaches have been explored [15,16,17,18] such as chaos modulation [18,19,20,21], chaos shift keying [22,23] and chaos masking [9,17,20,24]. In chaos communication schemes, the typical key idea for transmitting a message through chaotic/hyperchaotic models is that a message signal is nested in the transmitter system/model which originates a chaotic/ disturbed signal. Afterwards, this disturbed signal has been emitted to the receiver through a universal channel. The message signal would finally be recovered by the receiver. A chaotic model has been intrinsically employed both as receiver and transmitter. Consequently, this area of chaotic synchronization & control has sought remarkable considerations among differential research fields.
Most prominently, synchronization theory has been in existence for over 30 years due to the phenomenal research of Pecora and Carroll [25] established in 1990 using drive-response/master-slave/leader-follower configuration. Consequently, many authors and researchers have started introducing and studying numerous control and synchronization methods [9,26,27,28,29,30,31,32,33,34,35,36] etc. to achieve stabilized chaotic systems for possessing stability. In [37], researchers discussed optimal synchronization issues in similar GLVBSs via optimal control methodology. In [38,39], the researchers studied the adaptive control method (ACM) to synchronize chaotic GLVBSs. Also, researchers [40] introduced a combination difference anti-synchronization scheme in similar chaotic GLVBSs via ACM. In addition, authors [41] investigated a combination synchronization scheme to control chaos existing in GLVBSs using active control strategy (ACS). Bai and Lonngren [42] first proposed ACS in 1997 for synchronizing and controlling chaos found in nonlinear dynamical systems. Furthermore, compound synchronization using ACS was first advocated by Sun et al. [43] in 2013. In [44], authors discussed compound difference anti-synchronization scheme in four chaotic systems out of which two chaotic systems are considered as GLVBSs using ACS and ACM along with applications in secure communications of chaos masking type in 2019. Some further research works [45,46] based on ACS have been reported in this direction. The considered chaotic GLVBS offers a generalization that allows higher-order biological terms. As a result, it may be of interest in cases where biological systems experience cataclysmic changes. Unfortunately, some species will be under competitive pressure in the coming years and decades. This work may be comprised as a step toward preserving as many currently living species as possible by using the proposed synchronization approach which is based on master-slave configuration and Lyapunov stability analysis.
In consideration of the aforementioned discussions and observations, our primary focus here is to develop a systematic approach for investigating compound difference anti-synchronization (CDAS) approach in 4 similar chaotic GLVBSs via ACS. The considered ACS is a very efficient yet theoretically rigorous approach for controlling chaos found in GLVBSs. Additionally, in view of widely known Lyapunov stability analysis (LSA) [47], we discuss actively designed biological control law & convergence for synchronization errors to attain CDAS synchronized states.
The major attributes for our proposed research in the present manuscript are:
● The proposed CDAS methodology considers four chaotic GLVBSs.
● It outlines a robust CDAS approach based active controller to achieve compound difference anti-synchronization in discussed GLVBSs & conducts oscillation in synchronization errors along with extremely fast convergence.
● The construction of the active control inputs has been executed in a much simplified fashion utilizing LSA & master-salve/ drive-response configuration.
● The proposed CDAS approach in four identical chaotic GLVBSs of integer order utilizing ACS has not yet been analyzed up to now. This depicts the novelty of our proposed research work.
This manuscript is outlined as follows: Section 2 presents the problem formulation of the CDAS scheme. Section 3 designs comprehensively the CDAS scheme using ACS. Section 4 consists of a few structural characteristics of considered GLVBS on which CDAS is investigated. Furthermore, the proper active controllers having nonlinear terms are designed to achieve the proposed CDAS strategy. Moreover, in view of Lyapunov's stability analysis (LSA), we have examined comprehensively the biological controlling laws for achieving global asymptotical stability of the error dynamics for the discussed model. In Section 5, numerical simulations through MATLAB are performed for the illustration of the efficacy and superiority of the given scheme. Lastly, we also have presented some conclusions and the future prospects of the discussed research work in Section 6.
We here formulate a methodology to examine compound difference anti-synchronization (CDAS) scheme viewing master-slave framework in four chaotic systems which would be utilized in the coming up sections.
Let the scaling master system be
˙wm1= f1(wm1), | (2.1) |
and the base second master systems be
˙wm2= f2(wm2), | (2.2) |
˙wm3= f3(wm3). | (2.3) |
Corresponding to the aforementioned master systems, let the slave system be
˙ws4= f4(ws4)+U(wm1,wm2,wm3,ws4), | (2.4) |
where wm1=(wm11,wm12,...,wm1n)T∈Rn, wm2=(wm21,wm22,...,wm2n)T∈Rn, wm3=(wm31,wm32,...,wm3n)T∈Rn, ws4=(ws41,ws42,...,ws4n)T∈Rn are the state variables of the respective chaotic systems (2.1)–(2.4), f1,f2,f3,f4:Rn→Rn are four continuous vector functions, U=(U1,U2,...,Un)T:Rn×Rn×Rn×Rn→Rn are appropriately constructed active controllers.
Compound difference anti-synchronization error (CDAS) is defined as
E=Sws4+Pwm1(Rwm3−Qwm2), |
where P=diag(p1,p2,.....,pn),Q=diag(q1,q2,.....,qn),R=diag(r1,r2,.....,rn),S=diag(s1,s2,.....,sn) and S≠0.
Definition: The master chaotic systems (2.1)–(2.3) are said to achieve CDAS with slave chaotic system (2.4) if
limt→∞‖E(t)‖=limt→∞‖Sws4(t)+Pwm1(t)(Rwm3(t)−Qwm2(t))‖=0. |
We now present our proposed CDAS approach in three master systems (2.1)–(2.3) and one slave system (2.4). We next construct the controllers based on CDAS approach by
Ui= ηisi−(f4)i−KiEisi, | (3.1) |
where ηi=pi(f1)i(riwm3i−qiwm2i)+piwm1i(ri(f3)i−qi(f2)i), for i=1,2,...,n.
Theorem: The systems (2.1)–(2.4) will attain the investigated CDAS approach globally and asymptotically if the active control functions are constructed in accordance with (3.1).
Proof. Considering the error as
Ei= siws4i+piwm1i(riwm3i−qiwm2i),fori=1,2,3,.....,n. |
Error dynamical system takes the form
˙Ei= si˙ws4i+pi˙wm1i(riwm3i−qiwm2i)+piwm1i(ri˙wm3i−qi˙wm2i)= si((f4)i+Ui)+pi(f1)i(riwm3i−qiwm2i)+piwm1i(ri(f3)i−qi(f2)i)= si((f4)i+Ui)+ηi, |
where ηi=pi(f1)i(riwm3i−qiwm2i)+piwm1i(ri(f3)i−qi(f2)i), i=1,2,3,....,n. This implies that
˙Ei= si((f4)i−ηisi−(f4)i−KiEisi)+ηi= −KiEi | (3.2) |
The classic Lyapunov function V(E(t)) is described by
V(E(t))= 12ETE= 12ΣE2i |
Differentiation of V(E(t)) gives
˙V(E(t))=ΣEi˙Ei |
Using Eq (3.2), one finds that
˙V(E(t))=ΣEi(−KiEi)= −ΣKiE2i). | (3.3) |
An appropriate selection of (K1,K1,.......,Kn) makes ˙V(E(t)) of eq (3.3), a negative definite. Consequently, by LSA [47], we obtain
limt→∞Ei(t)=0,(i=1,2,3). |
Hence, the master systems (2.1)–(2.3) and slave system (2.4) have attained desired CDAS strategy.
We now describe GLVBS as the scaling master system:
{˙wm11=wm11−wm11wm12+b3w2m11−b1w2m11wm13,˙wm12=−wm12+wm11wm12,˙wm13=b2wm13+b1w2m11wm13, | (4.1) |
where (wm11,wm12,wm13)T∈R3 is state vector of (4.1). Also, wm11 represents the prey population and wm12, wm13 denote the predator populations. For parameters b1=2.9851, b2=3, b3=2 and initial conditions (27.5,23.1,11.4), scaling master GLVBS displays chaotic/disturbed behaviour as depicted in Figure 1(a).
The base master systems are the identical chaotic GLVBSs prescribed respectively as:
{˙wm21=wm21−wm21wm22+b3w2m21−b1w2m21wm23,˙wm22=−wm22+wm21wm22,˙wm23=b2wm23+b1w2m21wm23, | (4.2) |
where (wm21,wm22,wm23)T∈R3 is state vector of (4.2). For parameter values b1=2.9851, b2=3, b3=2, this base master GLVBS shows chaotic/disturbed behaviour for initial conditions (1.2,1.2,1.2) as displayed in Figure 1(b).
{˙wm31=wm31−wm31wm32+b3w2m31−b1w2m31wm33,˙wm32=−wm32+wm31wm32,˙wm33=b2wm33+b1w2m31wm33, | (4.3) |
where (wm31,wm32,wm33)T∈R3 is state vector of (4.3). For parameters b1=2.9851, b2=3, b3=2, this second base master GLVBS displays chaotic/disturbed behaviour for initial conditions (2.9,12.8,20.3) as shown in Figure 1(c).
The slave system, represented by similar GLVBS, is presented by
{˙ws41=ws41−ws41ws42+b3w2s41−b1w2s41ws43+U1,˙ws42=−ws42+ws41ws42+U2,˙ws43=b2ws43+b1w2s41ws43+U3, | (4.4) |
where (ws41,ws42,ws43)T∈R3 is state vector of (4.4). For parameter values, b1=2.9851, b2=3, b3=2 and initial conditions (5.1,7.4,20.8), the slave GLVBS exhibits chaotic/disturbed behaviour as mentioned in Figure 1(d).
Moreover, the detailed theoretical study for (4.1)–(4.4) can be found in [4]. Further, U1, U2 and U3 are controllers to be determined.
Next, the CDAS technique has been discussed for synchronizing the states of chaotic GLVBS. Also, LSA-based ACS is explored & the necessary stability criterion is established.
Here, we assume P=diag(p1,p2,p3), Q=diag(q1,q2,q3), R=diag(r1,r2,r3), S=diag(s1,s2,s3). The scaling factors pi,qi,ri,si for i=1,2,3 are selected as required and can assume the same or different values.
The error functions (E1,E2,E3) are defined as:
{E1=s1ws41+p1wm11(r1wm31−q1wm21),E2=s2ws42+p2wm12(r2wm32−q2wm22),E3=s3ws43+p3wm13(r3wm33−q3wm23). | (4.5) |
The major objective of the given work is the designing of active control functions Ui,(i=1,2,3) ensuring that the error functions represented in (4.5) must satisfy
limt→∞Ei(t)=0for(i=1,2,3). |
Therefore, subsequent error dynamics become
{˙E1=s1˙ws41+p1˙wm11(r1wm31−q1wm21)+p1wm11(r1˙wm31−q1˙wm21),˙E2=s2˙ws42+p2˙wm12(r2wm32−q2wm22)+p2wm12(r2˙wm32−q2˙wm22),˙E3=s3˙ws43+p3˙wm13(r3wm33−q3wm23)+p3wm13(r3˙wm33−q3˙wm23). | (4.6) |
Using (4.1), (4.2), (4.3), and (4.5) in (4.6), the error dynamics simplifies to
{˙E1=s1(ws41−ws41ws42+b3w2s41−b1w2s41ws43+U1)+p1(wm11−wm11wm12+b3w2m11−b1w2m11wm13)(r1wm31−q1wm21)+p1wm11(r1(wm31−wm31wm32+b3w2m31−b1w2m31wm33)−q1(wm21−wm21wm22+b3w2m21−b1w2m21wm23),˙E2=s2(−ws42+ws41ws42+U2)+p2(−wm12+wm11wm12)(r2wm32−q2wm22)+p2wm12(r2(−wm32+wm31wm32)−q2(−wm22+wm21wm22)),˙E3=s3(b2ws43+b1w2s41ws43+U3)+p3(b2wm13+b1w2m11wm13)(r3wm33−q3wm23)+p3wm13(r3(b2wm33+b1w2m31wm33)−q3(b2wm23+b1w2m21wm23)). | (4.7) |
Let us now choose the active controllers:
U1= η1s1−(f4)1−K1E1s1, | (4.8) |
where η1=p1(f1)1(r1wm31−q1wm21)+p1wm11(r1(f3)1−q1(f2)1), as described in (3.1).
U2= η2s2−(f4)2−K2E2s2, | (4.9) |
where η2=p2(f1)2(r2wm32−q2wm22)+p2wm12(r2(f3)2−q2(f2)2).
U3= η3s3−(f4)3−K3E3s3, | (4.10) |
where η3=p3(f1)3(r3wm33−q3wm23)+p3wm13(r3(f3)3−q3(f2)3) and K1>0,K2>0,K3>0 are gaining constants.
By substituting the controllers (4.8), (4.9) and (4.10) in (4.7), we obtain
{˙E1=−K1E1,˙E2=−K2E2,˙E3=−K3E3. | (4.11) |
Lyapunov function V(E(t)) is now described by
V(E(t))= 12[E21+E22+E23]. | (4.12) |
Obviously, the Lyapunov function V(E(t)) is +ve definite in R3. Therefore, the derivative of V(E(t)) as given in (4.12) can be formulated as:
˙V(E(t))= E1˙E1+E2˙E2+E3˙E3. | (4.13) |
Using (4.11) in (4.13), one finds that
˙V(E(t))= −K1E21−K2E22−K3E23<0, |
which displays that ˙V(E(t)) is -ve definite.
In view of LSA [47], we, therefore, understand that CDAS error dynamics is globally as well as asymptotically stable, i.e., CDAS error E(t)→0 asymptotically for t→∞ to each initial value E(0)∈R3.
This section conducts a few simulation results for illustrating the efficacy of the investigated CDAS scheme in identical chaotic GLVBSs using ACS. We use 4th order Runge-Kutta algorithm for solving the considered ordinary differential equations. Initial conditions for three master systems (4.1)–(4.3) and slave system (4.4) are (27.5,23.1,11.4), (1.2,1.2,1.2), (2.9,12.8,20.3) and (14.5,3.4,10.1) respectively. We attain the CDAS technique among three masters (4.1)–(4.3) and corresponding one slave system (4.4) by taking pi=qi=ri=si=1, which implies that the slave system would be entirely anti-synchronized with the compound of three master models for i=1,2,3. In addition, the control gains (K1,K2,K3) are taken as 2. Also, Figure 2(a)–(c) indicates the CDAS synchronized trajectories of three master (4.1)–(4.3) & one slave system (4.4) respectively. Moreover, synchronization error functions (E1,E2,E3)=(51.85,275.36,238.54) approach 0 as t tends to infinity which is exhibited via Figure 2(d). Hence, the proposed CDAS strategy in three masters and one slave models/systems has been demonstrated computationally.
In this work, the investigated CDAS approach in similar four chaotic GLVBSs using ACS has been analyzed. Lyapunov's stability analysis has been used to construct proper active nonlinear controllers. The considered error system, on the evolution of time, converges to zero globally & asymptotically via our appropriately designed simple active controllers. Additionally, numerical simulations via MATLAB suggest that the newly described nonlinear control functions are immensely efficient in synchronizing the chaotic regime found in GLVBSs to fitting set points which exhibit the efficacy and supremacy of our proposed CDAS strategy. Exceptionally, both analytic theory and computational results are in complete agreement. Our proposed approach is simple yet analytically precise. The control and synchronization among the complex GLVBSs with the complex dynamical network would be an open research problem. Also, in this direction, we may extend the considered CDAS technique on chaotic systems that interfered with model uncertainties as well as external disturbances.
The authors gratefully acknowledge Qassim University, represented by the Deanship of Scientific Research, on the financial support for this research under the number 10163-qec-2020-1-3-I during the academic year 1441 AH/2020 AD.
The authors declare there is no conflict of interest.
[1] | Centers for Disease Control and Prevention, Seasonal Influenza (flu), Technical report, 2012. Available from: https://www.cdc.gov/flu/. |
[2] | Public Health Agency of Canada, Human Health Issues Related to Avian Influenza in Canada, Technical report, 2006. Available from: https://www.canada.ca/en/public-health/services/reports-publications/human-health-issues-related-avian-influenza.html. |
[3] |
P. Chen, J. Xie, Q. Lin, L. Zhao, Y. Zhang, H. Chen, et al., A study of the relationship between human infection with avian influenza a (H5N6) and environmental avian influenza viruses in Fujian, China, BMC Infect. Dis., 19 (2019), 762. https://doi.org/10.1186/s12879-019-4145-6 doi: 10.1186/s12879-019-4145-6
![]() |
[4] |
H. Gao, H. Lu, B. Cao, B. Du, H. Shang, J. Gan, et al., Clinical findings in 111 cases of influenza A (H7N9) virus infection, N. Engl. J. Med., 368 (2013), 2277–2285. https://doi.org/10.1056/NEJMoa1305584 doi: 10.1056/NEJMoa1305584
![]() |
[5] |
R. Gao, B. Cao, Y. Hu, Z. Feng, D. Wang, W. Hu, et al., Human infection with a novel avian-origin influenza A (H7N9) virus, N. Engl. J. Med., 368 (2013), 1888–1897. https://doi.org/ 10.1056/NEJMoa1304459 doi: 10.1056/NEJMoa1304459
![]() |
[6] |
D. Liu, W. Shi, Y. Shi, D. Wang, H. Xiao, W. Li, et al., Origin and diversity of novel avian influenza A H7N9 viruses causing human infection: phylogenetic, structural, and coalescent analyses, Lancet, 381 (2013), 1926–1932. https://doi.org/10.1016/S0140-6736(13)60938-1 doi: 10.1016/S0140-6736(13)60938-1
![]() |
[7] |
E. Claas, A. Osterhaus, R. Van-Beek, J. Jong, G. F. Rimmelzwaan, D. A. Senne, et al., Human influenza A H5N1 virus related to a highly pathogenic avian influenza virus, Lancet, 351 (1998), 472–477. https://doi.org/10.1016/S0140-6736(97)11212-0 doi: 10.1016/S0140-6736(97)11212-0
![]() |
[8] |
T. Kang, Q. Zhang, L. Rong, A delayed avian influenza model with avian slaughter: stability analysis and optimal control, Physica A, 529 (2019), 121544. https://doi.org/10.1016/j.physa.2019.121544 doi: 10.1016/j.physa.2019.121544
![]() |
[9] |
K. A. Pawelek, A. Oeldorf-Hirsch, L. Rong, Modeling the impact of twitter on influenza epidemics, Math. Biosci. Eng., 11 (2014), 1337–1356. https://doi.org/10.3934/mbe.2014.11.1337 doi: 10.3934/mbe.2014.11.1337
![]() |
[10] | World Health Organization, Human infection with avian influenza A (H7N9) virus–update, 2014. Available from: https://www.who.int/emergencies/disease-outbreak-news/item/2014_09_04_avian_influenza-en. |
[11] |
L. Chen, X. Lin, W. Guo, J. Tian, W. Wang, X. Ying, et al., Diversity and evolution of avian influenza viruses in live poultry markets, free-range poultry and wild wetland birds in China, J. Gen. Virol., 97 (2016), 844–854. https://doi.org/10.1099/jgv.0.000399 doi: 10.1099/jgv.0.000399
![]() |
[12] |
K. Shimizu, L. Wulandari, E. D. Poetranto, R. A. Setyoningrum, R. Yudhawati, A. Sholikhah, et al., Seroevidence for a high prevalence of subclinical infection with avian influenza A(H5N1) virus among workers in a livepoultry market in Indonesia, J. Infect. Dis., 214 (2016), 1929–1936. https://doi.org/10.1093/infdis/jiw478 doi: 10.1093/infdis/jiw478
![]() |
[13] |
C. Bao, L. Cui, M. Zhou, L. Hong, G. F. Gao, H. Wang, Live-animal markets and influenza A (H7N9) virus infection, N. Engl. J. Med., 368 (2013), 2337–2339. https://doi.org/10.1056/NEJMc1306100 doi: 10.1056/NEJMc1306100
![]() |
[14] |
Y. Xiao, X. Sun, S. Tang, J. Wu, Transmission potential of the novel avian influenza A(H7N9) infection in mainland China, J. Theor. Biol., 352 (2014), 1–5. https://doi.org/10.1016/j.jtbi.2014.02.038 doi: 10.1016/j.jtbi.2014.02.038
![]() |
[15] |
X. Wang, W. Cheng, Z. Yu, S. Liu, H. Mao, E. Chen, Risk factors for avian influenza virus in backyard poultry flocks and environments in Zhejiang province, China: a cross-sectional study, Infect. Dis. Poverty, 7 (2018), 65. https://doi.org/10.1186/s40249-018-0445-0 doi: 10.1186/s40249-018-0445-0
![]() |
[16] |
E. Jung, S. Iwami, Y. Takeuchi, T. Jo, Optimal control strategy for prevention of avian influenza pandemic, J. Theor. Biol., 260 (2009), 220–229. https://doi.org/10.1016/j.jtbi.2009.05.031 doi: 10.1016/j.jtbi.2009.05.031
![]() |
[17] |
T. Kang, Q. Zhang, H. Wang, Optimal control of an avian influenza model with multiple time delays in state and control variables, Discrete Contin. Dyn. Syst. - Ser. B, 26 (2021), 4147–4171. https://doi.org/10.3934/dcdsb.2020278 doi: 10.3934/dcdsb.2020278
![]() |
[18] |
S. Sharma, A. Mondal, A. Pal, G. P. Samanta, Stability analysis and optimal control of avian influenza virus A with time delays, Int. J. Dyn. Control, 6 (2018), 1351–1366. https://doi.org/10.1007/s40435-017-0379-6 doi: 10.1007/s40435-017-0379-6
![]() |
[19] |
B. Cao, X. Nie, Event-triggered adaptive neural networks control for fractional-order nonstrict-feedback nonlinear systems with unmodeled dynamics and input saturation, Neural Networks, 142 (2021), 288–302. https://doi.org/10.1016/j.neunet.2021.05.014 doi: 10.1016/j.neunet.2021.05.014
![]() |
[20] |
B. Cao, X. Nie, Z. Wu, C. Xue, J. Cao, Adaptive neural network control for nonstrict-feedback uncertain nonlinear systems with input delay and asymmetric time-varying state constraints, J. Franklin Inst., 358 (2021), 7073–7095. https://doi.org/10.1016/j.jfranklin.2021.07.020 doi: 10.1016/j.jfranklin.2021.07.020
![]() |
[21] |
B. Cao, T. Kang, Nonlinear adaptive control of COVID-19 with media campaigns and treatment, Biochem. Biophys. Res. Commun., 555 (2021), 202–209. https://doi.org/10.1016/j.bbrc.2020.12.105 doi: 10.1016/j.bbrc.2020.12.105
![]() |
[22] |
H. Moradi, M. Sharifi, G. Vossoughi, Adaptive robust control of cancer chemotherapy in the presence of parametric uncertainties: a comparison between three hypotheses, Comput. Biol. Med., 56 (2015), 145–157. https://doi.org/10.1016/j.compbiomed.2014.11.002 doi: 10.1016/j.compbiomed.2014.11.002
![]() |
[23] |
M. H. Nematollahi, R. Vatankhah, M. Sharifi, Nonlinear adaptive control of tuberculosis with consideration of the risk of endogenous reactivation and exogenous reinfection, J. Theor. Biol., 486 (2020), 110081. https://doi.org/10.1016/j.jtbi.2019.110081 doi: 10.1016/j.jtbi.2019.110081
![]() |
[24] |
Y. Hayama, Y. Kimura, T. Yamamoto, S. Kobayashi, T, Tsutsui, Potential risk associated with animal culling and disposal during the foot-and-mouth disease epidemic in Japan in 2010, Res. Vet. Sci., 102 (2015), 228–230. https://doi.org/10.1016/j.rvsc.2015.08.017 doi: 10.1016/j.rvsc.2015.08.017
![]() |
[25] |
B. Barnes, A. Scott, M. Hernandez-Jover, J. A. Toribio, B. Moloney, K. Glass, Modelling high pathogenic avian influenza outbreaks in the commercial poultry industry, Theor. Popul Biol., 126 (2019), 59–71. https://doi.org/10.1016/j.tpb.2019.02.004 doi: 10.1016/j.tpb.2019.02.004
![]() |
[26] |
A. Wang, Y. Xiao, A Filippov system describing media effects on the spread of infectious diseases, Nonlinear Anal. Hybrid Syst., 11 (2014), 84–97. https://doi.org/10.1016/j.nahs.2013.06.005 doi: 10.1016/j.nahs.2013.06.005
![]() |
[27] |
X. Zhang, X. Liu, Backward bifurcation of an epidemic model with saturated treatment function, J. Math. Anal. Appl., 348 (2008), 433–443. https://doi.org/10.1016/j.jmaa.2008.07.042 doi: 10.1016/j.jmaa.2008.07.042
![]() |
[28] | J. J. E. Slotine, W. Li, Applied Nonlinear Control, Prentice Hall, Englewood Cliffs, New Jersey, 1991. |
[29] | F. Chen, J. Cui, Cross-species epidemic dynamic model of influenza, in 2016 9th International Congress on Image and Signal Processing, BioMedical Engineering and Informatics (CISP-BMEI), (2016), 1567–1572. https://doi.org/10.1109/CISP-BMEI.2016.7852965 |
[30] |
S. Liu, S. Ruan, X. Zhang, Nonlinear dynamics of avian influenza epidemic models, Math. Biosci., 283 (2017), 118–135. https://doi.org/10.1016/j.mbs.2016.11.014 doi: 10.1016/j.mbs.2016.11.014
![]() |
1. | Muhammad Zubair Mehboob, Arslan Hamid, Jeevotham Senthil Kumar, Xia Lei, Comprehensive characterization of pathogenic missense CTRP6 variants and their association with cancer, 2025, 25, 1471-2407, 10.1186/s12885-025-13685-0 |