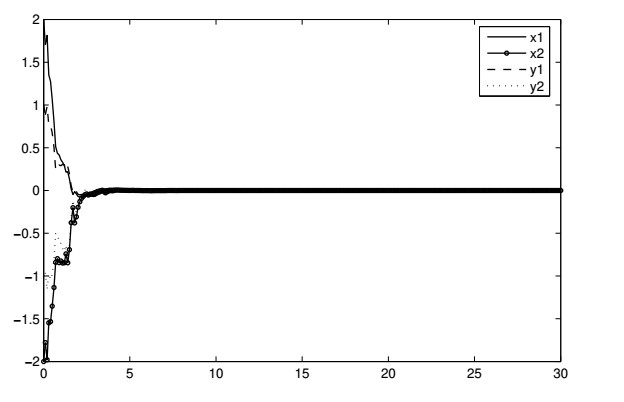
This paper investigates a novel multi-objective optimization framework for the multi-stage missile target allocation (M-MTA) problem, which also widely exists in other real-world complex systems. Specifically, a constrained model of M-MTA is built with the trade-off between minimizing the survivability of targets and minimizing the cost consumption of missiles. Moreover, a multi-objective optimization algorithm (NSGA-MTA) is proposed for M-MTA, where the hybrid encoding mechanism establishes the expression of the model and algorithm. Furthermore, rule-based initialization is developed to enhance the quality and searchability of feasible solutions. An efficient non-dominated sorting method is introduced into the framework as an effective search strategy. Besides, the genetic operators with the greedy mechanism and random repair strategy are involved in handling the constraints with maintaining diversity. The results of numerical experiments demonstrate that NSGA-MTA performs better in diversity and convergence than the excellent current algorithms in metrics and Pareto front obtained in 15 scenarios. Taguchi method is also adopted to verify the contribution of proposed strategies, and the results show that these strategies are practical and promotive to performance improvement.
Citation: Shiqi Zou, Xiaoping Shi, Shenmin Song. A multi-objective optimization framework with rule-based initialization for multi-stage missile target allocation[J]. Mathematical Biosciences and Engineering, 2023, 20(4): 7088-7112. doi: 10.3934/mbe.2023306
[1] | Wenjing Wang, Jingjing Dong, Dong Xu, Zhilian Yan, Jianping Zhou . Synchronization control of time-delay neural networks via event-triggered non-fragile cost-guaranteed control. Mathematical Biosciences and Engineering, 2023, 20(1): 52-75. doi: 10.3934/mbe.2023004 |
[2] | Pan Wang, Xuechen Li, Qianqian Zheng . Synchronization of inertial complex-valued memristor-based neural networks with time-varying delays. Mathematical Biosciences and Engineering, 2024, 21(2): 3319-3334. doi: 10.3934/mbe.2024147 |
[3] | Biwen Li, Xuan Cheng . Synchronization analysis of coupled fractional-order neural networks with time-varying delays. Mathematical Biosciences and Engineering, 2023, 20(8): 14846-14865. doi: 10.3934/mbe.2023665 |
[4] | Ruoyu Wei, Jinde Cao . Prespecified-time bipartite synchronization of coupled reaction-diffusion memristive neural networks with competitive interactions. Mathematical Biosciences and Engineering, 2022, 19(12): 12814-12832. doi: 10.3934/mbe.2022598 |
[5] | Bingrui Zhang, Jin-E Zhang . Fixed-deviation stabilization and synchronization for delayed fractional-order complex-valued neural networks. Mathematical Biosciences and Engineering, 2023, 20(6): 10244-10263. doi: 10.3934/mbe.2023449 |
[6] | Trayan Stamov, Gani Stamov, Ivanka Stamova, Ekaterina Gospodinova . Lyapunov approach to manifolds stability for impulsive Cohen–Grossberg-type conformable neural network models. Mathematical Biosciences and Engineering, 2023, 20(8): 15431-15455. doi: 10.3934/mbe.2023689 |
[7] | Hai Lin, Jingcheng Wang . Pinning control of complex networks with time-varying inner and outer coupling. Mathematical Biosciences and Engineering, 2021, 18(4): 3435-3447. doi: 10.3934/mbe.2021172 |
[8] | Zhen Yang, Zhengqiu Zhang, Xiaoli Wang . New finite-time synchronization conditions of delayed multinonidentical coupled complex dynamical networks. Mathematical Biosciences and Engineering, 2023, 20(2): 3047-3069. doi: 10.3934/mbe.2023144 |
[9] | Karim El Laithy, Martin Bogdan . Synaptic energy drives the information processing mechanisms in spiking neural networks. Mathematical Biosciences and Engineering, 2014, 11(2): 233-256. doi: 10.3934/mbe.2014.11.233 |
[10] | Guowei Wang, Yan Fu . Spatiotemporal patterns and collective dynamics of bi-layer coupled Izhikevich neural networks with multi-area channels. Mathematical Biosciences and Engineering, 2023, 20(2): 3944-3969. doi: 10.3934/mbe.2023184 |
This paper investigates a novel multi-objective optimization framework for the multi-stage missile target allocation (M-MTA) problem, which also widely exists in other real-world complex systems. Specifically, a constrained model of M-MTA is built with the trade-off between minimizing the survivability of targets and minimizing the cost consumption of missiles. Moreover, a multi-objective optimization algorithm (NSGA-MTA) is proposed for M-MTA, where the hybrid encoding mechanism establishes the expression of the model and algorithm. Furthermore, rule-based initialization is developed to enhance the quality and searchability of feasible solutions. An efficient non-dominated sorting method is introduced into the framework as an effective search strategy. Besides, the genetic operators with the greedy mechanism and random repair strategy are involved in handling the constraints with maintaining diversity. The results of numerical experiments demonstrate that NSGA-MTA performs better in diversity and convergence than the excellent current algorithms in metrics and Pareto front obtained in 15 scenarios. Taguchi method is also adopted to verify the contribution of proposed strategies, and the results show that these strategies are practical and promotive to performance improvement.
Due to the wide variety of applications in fields including associative memories, signal processing, parallel computation, pattern recognition, neural networks have drawn a lot of interest in recent years. In many real-world systems, artificial neural networks, neural networks, biological systems have inevitable applications. [1,2,3,4,5,6,7]. Due to the obvious transmission frequency of interconnected neurons and the finite switching speeds of the amplifiers, time delays are ineluctably given. Their presence might affect a system's stability by causing oscillation and instability features [8,9,10,11,12,13,14,15,16,17,18]. Delayed dynamical networks and many time-delay system kinds have been studied, and numerous important outcomes have been presented [19,20,21,22,23,24,25].
Kosko was the first person to propose that BAM-NNs could be important to the NNs theory [26]. Furthermore, BAM neural frameworks involving delays attracted significant thought and underwent in-depth research. While there is no connection between neurons in the same layer, neurons in another layer are entirely interconnected to those in the first layer. It performs a two-way affiliated hunt to store bipolar vector combines by cycles of forward and backward propagation information streams between the two layers, and it sums up the single-layer auto-cooperative Hebbian connection to a two-layer design coordinated hetero acquainted circuits. As a result, it has numerous applications in the fields of artificial intelligence and pattern identification [27,28,29,30,31,32,33,34,35]. Appropriately, the BAM neural system has been generally concentrated on both in principle and applications. This makes focusing on the stability of the BAM neural framework, which has largely been studied, fascinating and fundamental [36,37,38,39,40,41,42,43,44].
The synchronization control of chaotic networks plays vital role in applications such as image encryption, secure communication, DC-DC motor and etc. [45,46,47,48]. Satellites play a significant part in the advancement of space technology, civic, military, and scientific endeavors. In particular, feedback control, adaptive control, sampled-data control, and other strategies have been employed to synchronize and regulate the satellite systems [49,50]. Recently, the issue of memristive BAM networks synchronization with stochastic feedback gain variations in [50].
It is inferred that in real-world scenarios, a nearby float framework as noted in practical model controller does not avoid the proceed out of coefficient uncertainty, leading to the murkiness in controller execution caused by the required word length in any updated structures or additional parameter turning in the final controller use. Along the same ideas, it is crucial to design a non-fragile controller such that it is insensitive to uncertainty. Non-fragile control has developed into a fascinating topic in both theory and practical application. There has been extensive research into the use of non-fragile controllers in recent years [51,52]. However, the problem of non-fragile control of a BAM delayed neural network and controller gain fluctuation has not been thoroughly examined.
This study examines the non-fragile synchronization for a BAM delayed neural network and a randomly occurring controller gain fluctuation, which is motivated by the studies. New stability requirements for BAM neural networks with arbitrarily occurring controller gain fluctuation are derived in terms of LMI by building an appropriate Lyapunov-Krasovskii functional (LKF) and using conventional integral inequality techniques. Solving the suggested LMI condition yields the gain matrices for the proposed controller design. Finally, a numerical example is used to illustrate the proposed strategy.
Notations: In this work, Rp represents Euclidean space of p dimension. Rp×q denotes the set of all p×q real matrices. The identity matrix is denoted by I. Here R>0(<0) represents R is a symmetric positive (negative) definite matrices. The elements below the main diagonal of a symmetric matrixl is given by '*'.
In this study, we will investigate the synchronization of BAM delayed neural network with randomly occurring. The BAM NNs with time-varying delay components can be modeled as follows
˙θi(ℵ)=−aiθi(ℵ)+∑nj=1w(1)ij˜fj(λj(ℵ))+∑nj=1w(2)ij˜fj(λj(ℵ−σ(ℵ)))+Ii,˙λj(ℵ)=−bjλj(ℵ)+∑ni=1v(1)ji˜gi(θi(ℵ))+∑ni=1v(2)ji˜gi(θi(ℵ−κ(ℵ)))+Jj,} | (2.1) |
where γi(ℵ) and δj(ℵ) are the state variables of the neuron at time ℵ respectively. The non linear functions ˜fj(⋅), ˜gi(⋅) are neuron activation functions. The positive constants ai,bj denote the time scales of the respective layers of the neurons. w(1)ij, w(2)ij, v(1)ji, v(2)ji are connection weights of the network. Ii and Jj denotes the external inputs. σ(ℵ),κ(ℵ) are time varying delays satisfying
0≤σ(ℵ)≤σ, ˙σ(ℵ)=μ1<1, 0≤κ(ℵ)≤κ, ˙κ(ℵ)=μ2<2, | (2.2) |
and, σ, κ, μ1, and μ2 are constants.
Consequently, the corresponding compact matrix form can be used to describe the master system (2.1) as,
M:{˙θ(ℵ)=−Aθ(ℵ)+W1˜f(λ(ℵ))+W2˜f(λ(ℵ−σ(ℵ))),˙λ(ℵ)=−Bλ(ℵ)+V1˜g(θ(ℵ))+V2˜g(θ(ℵ−κ(ℵ))), | (2.3) |
where, B=diag[b1,b2,...,bn]>0, A=diag[a1,a2,...,an]>0, Wk=(w(k)ij)(n×n), Vk=(v(k)ij)(n×n),k=1,2.
Assumption (1). ˜gj(⋅),˜fi(⋅) are neuron activation functions, which is bounded, then there exist constants H−i, H+i, L−j, L+j such that
L−j≤˜gj(ϕ)−˜gj(ϱ)ϕ−ϱ≤L+j,H−i≤˜fi(ϕ)−˜fi(ϱ)ϕ−ϱ≤H+i, | (2.4) |
where, j=1,..,n,i=1,...m, and ϱ,ϕ∈R with ϕ≠ϱ. We define the subsequent matrices for ease of notation:
H1=diag{H+1H−1,H+2H−2,...,H+mH−m},H2=diag{H−1+H+12,H+2+H−22,...,H+m+H−m2},L1=diag{L+1L−1,L+2L−2,...,L+nL−n},L2=diag{L+1+L−12,L+2+L−22,...,L+n+L−n2}. |
Master system and slave system state variables are represented by θ(ℵ),λ(ℵ) and ˆθ(ℵ) and ˆλ(ℵ), respectively. We take into account the master system's slave system in the follows.
S:{˙ˆθ(ℵ)=−Aˆθ(ℵ)+W1˜f(ˆλ(ℵ))+W2˜f(ˆλ(ℵ−σ(ℵ)))+u(ℵ),˙ˆλ(ℵ)=−Bˆλ(ℵ)+V1˜g(ˆθ(ℵ))+V2˜g(ˆθ(ℵ−κ(ℵ)))+v(ℵ) | (2.5) |
We choose the following non-fragile controller:
u(ℵ)=(K1+ϕ(ℵ)ΔK1(ℵ))ζ(ℵ), v(ℵ)=(K2+ϱ(ℵ)ΔK2(ℵ))ϖ(ℵ), | (2.6) |
Here the controller gain matrices are given by K1,K2. The matrix ΔKi(ℵ)(i=1,2) satisfy
ΔKi(ℵ)=HiΔ(ℵ)Ei (i=1,2) | (2.7) |
and
ΔT(ℵ)Δ(ℵ)≤I, | (2.8) |
where Hi,Ei are known constant matrices. To desribe randomly accuring control gain fluctuation we introduced the stachastic variables ϕ(ℵ),ϱ(ℵ)∈R. It is a sequence of white noise generated by Bernoulli distribution with values of zero or one
Pr{ϱ(ℵ)=0}=1−ϱ, Pr{ϱ(ℵ)=1}=ϱ,Pr{ϕ(ℵ)=0}=1−ϕ, Pr{ϕ(ℵ)=1}=ϕ, | (2.9) |
where 0≤ϱ,ϕ≤1 is constant.
Set the synchronization error signals ϖ(ℵ)=ˆλ(ℵ)−λ(ℵ) and ζ(ℵ)=ˆθ(ℵ)−θ(ℵ). Error dynamics between systems (2.3) and (2.5) can therefore be written as follows:
˙ζ(ℵ)=(−A+(K1+ϕ(ℵ)ΔK1(ℵ)))ζ(ℵ)+W1f(ϖ(ℵ))+W2f(ϖ(ℵ−σ(ℵ))),˙ϖ(ℵ)=(−B+(K2+ϱ(ℵ)ΔK2(ℵ)))ϖ(ℵ)+V1g(ζ(ℵ))+V2g(ζ(ℵ−κ(ℵ)))}. | (2.10) |
where g(ζ(ℵ))=˜g(ˆθ(ℵ))−˜g(θ(ℵ)), and f(ϖ(ℵ))=˜f(ˆλ(ℵ))−˜f(λ(ℵ)).
The following crucial conditions are used to obtain our main findings:
Lemma 2.1. [53] (Schur complement) Let Z, V,O be given matrices such that Z>0, then
[OVTv−Z]<0iffO+VTZ−1V<0. |
Lemma 2.2. [54] Given matrices Z=ZT,R,O and Q=QT>0 with appropriate dimensions
Z+RL(ℵ)O+OTLT(ℵ)RT<0, |
for all L(ℵ) satisfying LT(ℵ)L(ℵ)≤I if and only if there exists a scalar ε>0 such that
Z+ε−1RRT+εOTQO<0. |
Lemma 2.3. [55] For a given matrix Z∈S+n and a function ς:[c,d]→Rn whose derivative ˙ς∈PC([c,d],Rn), the following inequalities hold: ∫dc˙ςT(r)Z˙ς(r)dr≥1d−c ˆφ¯Zˆφ, where ¯Z=diag{Z,3Z,5Z}, ˆφ=[φT1 φT2 φT3]T, φ1=ς(d)−ς(c), φ2=ς(d)+ς(c)−2d−c∫dcς(r)dr, φ3=ς(d)−ς(c)+6d−c∫dcς(r)dr−12(d−c)2∫dc∫dcς(u)duds.
The necessary requirements for guaranteeing the stability of system (2.10) are established in this part.
Theorem 3.1. From Assumption (A), ϕ,ϱ, and ϵi are positive scalars, if there exist symmetric matrices Pi>0, Qi>0, Ri>0, Zi>0, any matrices Ji and Gi, diagonal matrices Si>0 (i=1,2) satisfying
Ξ=[ΠΩ∗Λ]<0, | (3.1) |
where
Π=[Π113Z1κS2L20−24Z1κ260Z1κ3Π17∗Π220036Z1κ2−60Z1κ30∗∗Π330000∗∗∗Π44000∗∗∗∗−192Z1κ3360Z1κ40∗∗∗∗∗−720Z1κ50∗∗∗∗∗∗Π77],Ω=[00J1W1J1W20000000000VT1JT200000VT1JT2VT2JT200000VT2JT20000000000000000J1W1J1W2000],Λ=[Λ113Z2σS1H20−24Z2σ260Z2σ3Λ17∗Λ220036Z2σ2−60Z2σ30∗∗Λ330000∗∗∗Λ44000∗∗∗∗−192Z2σ3360Z2σ40∗∗∗∗∗−720Z2σ50∗∗∗∗∗∗Λ77], |
Π11=R1−S2L1−9Z1κ−J1A+G1+ϕJ1ΔK1(ℵ)+GT1−ATJT1+ϕΔKT1(ℵ)JT1,Π17=−J1−ϵ1ATJT1+ϵ1GT1+ϵ1ϕΔKT1(ℵ)JT1+P1,Π22=−R1−9Z1κ,Π33=Q2−S2,Π44=−(1−μ2)Q2,Π77=−ϵ1J1+κZ1−ϵ1JT1,Λ11=R2−S1H1−J2B+G2+ϱJ2ΔK2(ℵ)−9Z2σ−BTJT+GT2+ϱΔKT2(ℵ)JT2,Λ17=−J2−ϵ2BTJT2+ϵ2GT2+ϵ2ϱΔKT2(ℵ)JT2+P2,Λ22=−R2−9Z2σ,Λ33=Q1−S1,Λ44=−(1−μ1)Q1,Λ77=σZ2−ϵ2J2−ϵ2JT2, |
When this happens, the system (2.10) is asymptotically stable and Ki=J−1iGi. are control gain matrices.
Proof. Take into account the LKF candidate below:
V(ζℵ,ϖℵ,ℵ)=4∑i=1Vi(ζℵ,ϖℵ,ℵ), | (3.2) |
where
V1(ζℵ,ϖℵ,ℵ)=ζT(ℵ)P1ζ(ℵ)+ϖT(ℵ)P2ϖ(ℵ),V2(ζℵ,ϖℵ,ℵ)=∫ℵℵ−σ(ℵ)fT(ϖ(s))Q1f(ϖ(s))ds+∫ℵℵ−κ(ℵ)gT(ζ(s))Q2g(ζ(s))ds,V3(ζℵ,ϖℵ,ℵ)=∫ℵℵ−κζT(s)R1ζ(s)ds+∫ℵℵ−σϖT(s)R2ϖ(s)ds,V4(ζℵ,ϖℵ,ℵ)=∫0−κ∫ℵℵ+θ˙ζT(s)Z1˙ζ(s)dsdθ+∫0−σ∫ℵℵ+θ˙ϖT(s)Z2˙ϖ(s)dsdθ. |
The infinitesimal operator L ofV(ζℵ,ϖℵ,ℵ) is:
LV(ζℵ,ϖℵ,ℵ)=limΔ→01Δ{E{V(ζℵ+Δ,yℵ+Δ,ℵ)|(ζℵ,ϖℵ,ℵ)}−V(ζℵ,ϖℵ,ℵ)},E{LV(ζℵ,ϖℵ,ℵ)}=4∑i=1E{LVi(ζℵ,ϖℵ,ℵ)}. | (3.3) |
Using the stochastic derivative of V(ζℵ,ϖℵ,ℵ), we can determine:
E{LV1(ζℵ,ϖℵ,ℵ)}=2ζT(ℵ)P1˙ζ(ℵ)+2ϖT(ℵ)P2˙ϖ(ℵ), | (3.4) |
E{LV2(ζℵ,ϖℵ,ℵ)}≤fT(ϖ(ℵ))Q1f(ϖ(ℵ))−(1−μ1)fT(ϖ(ℵ−σ(ℵ)))Q1f(ϖ(ℵ−σ(ℵ)))+gT(ζ(ℵ))Q2g(ζ(ℵ))−(1−μ2)gT(ζ(ℵ−κ(ℵ)))Q2g(ζ(ℵ−κ(ℵ))), | (3.5) |
E{LV3(ζℵ,ϖℵ,ℵ)}=ζT(ℵ)R1ζ(ℵ)−ζT(ℵ−κ)R1ζ(ℵ−κ)+ϖT(ℵ)R2ϖ(ℵ)−ϖT(ℵ−σ)R2ϖ(ℵ−σ), | (3.6) |
E{LV4(ζℵ,ϖℵ,ℵ)}=κ˙ζT(ℵ)Z1˙ζ(ℵ)−∫ℵℵ−κ˙ζT(s)Z1˙ζ(s)ds+σ˙ϖT(ℵ)Z2˙ϖ(ℵ)−∫ℵℵ−σ˙ϖT(s)Z2˙ϖ(s)ds, | (3.7) |
By applying Lemma 2.3 in (2.17), we can obtain
−∫ℵℵ−κ˙ζT(s)Z1˙ζ(s)ds≤−1κηT1(ℵ)[Z10003Z10005Z1]η1(ℵ), | (3.8) |
−∫ℵℵ−σ˙ϖT(s)Z2˙ϖ(s)ds≤−1σηT2(ℵ)[Z20003Z20005Z2]η2(ℵ), | (3.9) |
where
η1(ℵ)=[ζT(ℵ)−ζT(ℵ−κ)ζT(ℵ)+ζT(ℵ−κ)−2κ∫ℵℵ−κζT(s)dsζT(ℵ)−ζT(ℵ−κ)+6κ∫ℵℵ−κζT(s)ds−12κ2∫ℵℵ−κ∫tsζT(u)duds]T,η2(ℵ)=[ϖT(ℵ)−ϖT(ℵ−σ)ϖT(ℵ)+ϖT(ℵ−σ)−2σ∫ℵℵ−σϖT(s)dsϖT(ℵ)−ϖT(ℵ−σ)+6σ∫ℵℵ−σϖT(s)ds−12σ2∫ℵℵ−κ∫tsϖT(u)duds]T. |
From the assumption (A), there exists a diagonal matrices S1>0, S2>0 and Hi, Li(i=1,2), the following inequalities hold:
0≤[ϖ(ℵ)f(ϖ(ℵ))]T[−S1H1S1H2∗−S1][ϖ(ℵ)f(ϖ(ℵ))], | (3.10) |
0≤[ζ(ℵ)g(ζ(ℵ))]T[−S2L1S2L2∗−S2][ζ(ℵ)g(ζ(ℵ))]. | (3.11) |
The following equations satisfy for any matrices J1,J2 and scalar ϵ1,ϵ2:
0=2[ζT(ℵ)+ϵ1˙ζ(ℵ)]J1[−˙ζ(ℵ)+(−A+(K1+ϕ(ℵ)ΔK1(ℵ)))ζ(ℵ)+W1f(ϖ(ℵ))+W2f(ϖ(ℵ−σ(ℵ)))], | (3.12) |
0=2[ϖT(ℵ)+ϵ2˙ϖ(ℵ)]J2[−˙ϖ(ℵ)+(−B+(K2+ϱ(ℵ)ΔK2(ℵ)))ϖ(ℵ)+V1g(ζ(ℵ))+V2g(ζ(ℵ−κ(ℵ)))], | (3.13) |
substituting (3.4)–(3.6), and (3.7)–(3.23) in (3.3), we get
E{LV(ζℵ,ϖℵ,ℵ)}≤ξT(ℵ) Ξ ξ(ℵ), | (3.14) |
where ξ(ℵ)=[ζT(ℵ) ζT(ℵ−κ) gT(ζ(ℵ)) gT(ζ(ℵ−κ(ℵ))) ∫ℵℵ−κζT(s)ds ∫ℵℵ−κ∫ℵsζT(s)duds ˙ζT(ℵ) ϖT(ℵ)ϖT(ℵ−σ) fT(ϖ(ℵ)) fT(ϖ(ℵ−κ(ℵ))) ∫ℵℵ−σϖT(s)ds ∫ℵℵ−σ∫ℵsϖT(s)duds ˙ϖT(ℵ)]T.
It gives that Ξ <0. This demonstrates that the system (2.10) is asymptotically stable using the Lyapunov stability theory. The proof is now complete.
Theorem 3.2. Under Assumption (A), ϕ,ϱ and ϵi are positive scalars, then there exist symmetric matrices Pi>0, Qi>0, Ri>0, Zi>0, diagonal matrices Si>0, for any matrices Ji, Gi, and scalars ρi>0 (i=1,2) such that
˜Ξ=[ˆΞΥ1ρ1Υ2Ψ1Ψ2∗−ρ1I000∗∗−ρ1I00∗∗∗−ρ2I0∗∗∗∗−ρ2I]<0, | (3.15) |
where
ˆΞ=[ˆΠΩ∗ˆΛ],ˆΠ=[ˆΠ113Z1κS2L20−24Z1κ260Z1κ3ˆΠ17∗Π220036Z1κ2−60Z1κ30∗∗Π330000∗∗∗Π44000∗∗∗∗−192Z1κ3360Z1κ40∗∗∗∗∗−720Z1κ50∗∗∗∗∗∗Π77],ˆΛ=[ˆΛ113Z2σS1H20−24Z2σ260Z2σ3ˆΛ17∗Λ220036Z2σ2−60Z2σ30∗∗Λ330000∗∗∗Λ44000∗∗∗∗−192Z2σ3360Z2σ40∗∗∗∗∗−720Z2σ50∗∗∗∗∗∗Λ77], |
ˆΠ11=R1−S2L1−9Z1κ−J1A+G1+GT1−ATJT1,ˆΠ17=−J1−ϵ1ATJT1+ϵ1GT1+P1,ˆΛ11=R2−S1H1−J2B+G2−9Z2σ−BTJT+GT2,ˆΛ17=−J2−ϵ2BTJT2+ϵ2GT2+P2,Υ1=[ϕHT1JT1 0,...,0⏟5elements ϕϵ1HT1JT1 0,...,0⏟7elements]T,Υ2=[E1 0,...,0⏟13elements]T,Ψ1=[0,...,0⏟7elements ϱHT2JT2 0,...,0⏟5elements ϱϵ2HT2JT2]T,Ψ2=[0,...,0⏟7elements ρ2E2 0,...,0⏟6elements]T |
The controller gain matrices are also provided by in equation (2.6) are given by Ki=J−1iGi.
Proof. By using Schur complement,
[ˆΞ]+Υ1ΔK1(ℵ)ΥT2+Υ2ΔKT1(ℵ)ΥT1+Ψ1ΔK2(ℵ)ΨT2+Ψ2ΔKT2(ℵ)ΨT1<0. |
From Lemma 2, we get
˜Ξ=[ˆΞΥ1ρ1Υ2Ψ1Ψ2∗−ρ1I000∗∗−ρ1I00∗∗∗−ρ2I0∗∗∗∗−ρ2I]<0 |
It is clear that the disparities in (3.15) still exist. The evidence is now complete.
Two examples are provided in this part to show the applicability of our findings.
Example 4.1. The following BAM neural network with time varying delays:
˙ζ(ℵ)=(−A+(K1+ϕ(ℵ)ΔK1(ℵ)))ζ(ℵ)+W1f(ϖ(ℵ))+W2f(ϖ(ℵ−σ(ℵ))),˙ϖ(ℵ)=(−B+(K2+ϱ(ℵ)ΔK2(ℵ)))ϖ(ℵ)+V1g(ζ(ℵ))+V2g(ζ(ℵ−κ(ℵ))),} | (4.1) |
with the following parameters:
A=[1001], W1=[2−0.11−53.2],W2=[−1.6−0.8−0.18−2.5], H1=[0.45000.45],E1=[0.35000.35], B=[3002], V1=[1−1.11−21.2],V2=[−0.6−1.8−0.68−0.5],H2=[0.25000.25], E2=[0.15000.15], H1=L1=0, H2=L2=I. |
Additionally, we take κ=3.2, σ=3.2, μ1=0.9, μ2=0.9, ϕ=0.9, ϱ=0.9, ϵ1=0.5, and ϵ2=0.5. By solving the LMIs in Theorem 3.2, we get
P1=[48.3823−0.5707−0.570749.5117], P2=[46.62800.25890.258950.0702],Q1=[19.28354.76024.760228.1068], Q2=[19.03795.74755.747525.7761],R1=[35.0318−1.4108−1.410831.6352],R2=[35.2798−1.5288−1.528833.4075],Z1=[0.01670.00090.00090.0116], Z2=[0.0332−0.0196−0.01960.0971],G1=[−96.51481.09481.0948−99.3356],G2=[−91.4745−1.3821−1.3821−96.5435],J1=[0.15710.03320.03320.1827], J2=[0.3903−0.1807−0.18070.9874]ρ1=42.3781, ρ2=44.9762. |
As well as the following non-fragile controller gains matrices:
K1=[−639.9952126.6829122.2775−566.6860], K2=[−256.7506−53.3312−48.3949−107.5330]. |
In this scenario is proved the given system is asymptotically stable. In Figure 1, state response are depicted.
The issue of BAM delayed neural networks with non-fragile control and controller gain fluctuation has been examined in this article. To ensure the stability of the aforementioned systems, delay-dependent conditions are established. In terms of linear matrix inequalities (LMIs), necessary conditions are obtained by building an L-K functional and utilizing the traditional integral inequality technique. This technique ensures asymptotically stability of addressed to the concerned neural networks. Lastly, a numerical example is provided to demonstrate the viability of the findings in this study. Stochastic differential equations and complex networks both respond well to this approach in future research.
[1] |
R. K. Ahuja, A. Kumar, K. C. Jha, J. B. Orlin, Exact and heuristic algorithms for the weapon-target assignment problem, Oper. Res., 55 (2007), 1136–1146. https://doi.org/10.1287/opre.1070.0440 doi: 10.1287/opre.1070.0440
![]() |
[2] |
W. Wei, R. Yang, H. Gu, W. Zhao, C. Chen, S. Wan, Multi-objective optimization for resource allocation in vehicular cloud computing networks, IEEE Trans. Intell. Transp. Syst., 23 (2021), 25536–25545. https://doi.org/10.1109/TITS.2021.3091321 doi: 10.1109/TITS.2021.3091321
![]() |
[3] |
X. Hao, N. Yao, L. Wang, J. Wang, Joint resource allocation algorithm based on multi-objective optimization for wireless sensor networks, Appl. Soft Comput., 94 (2020), 106470. https://doi.org/10.1016/j.asoc.2020.106470 doi: 10.1016/j.asoc.2020.106470
![]() |
[4] |
O. A. Ogbolumani, N. I. Nwulu, Multi-objective optimisation of constrained food-energy-water-nexus systems for sustainable resource allocation, Sustainable Energy Technol. Assess., 44 (2021), 100967. https://doi.org/10.1016/j.seta.2020.100967 doi: 10.1016/j.seta.2020.100967
![]() |
[5] | S. P. Lloyd, H. S. Witsenhausen, Weapons allocation is np-complete, in 1986 Summer Computer Simulation Conference, (1986), 1054–1058. |
[6] |
J. Li, B. Xin, P. M. Pardalos, J. Chen, Solving bi-objective uncertain stochastic resource allocation problems by the CVaR-based risk measure and decomposition-based multi-objective evolutionary algorithms, Ann. Oper. Res., 296 (2021), 639–666. https://doi.org/10.1007/s10479-019-03435-4 doi: 10.1007/s10479-019-03435-4
![]() |
[7] | P. A. Hosein, M. Athans, Preferential Defense Strategies, 1990. |
[8] |
B. Xin, J. Chen, J. Zhang, L. Dou, Z. Peng, Efficient decision makings for dynamic weapon-target assignment by virtual permutation and tabu search heuristics, IEEE Trans. Syst. Man Cyber. C, 40 (2010), 649–662. https://doi.org/10.1109/TSMCC.2010.2049261 doi: 10.1109/TSMCC.2010.2049261
![]() |
[9] |
X. Shi, S. Zou, S. Song, R. Guo, A multi-objective sparse evolutionary framework for large-scale weapon target assignment based on a reward strategy, J. Intell. Fuzzy Syst., 40 (2021), 10043–10061. https://doi.org/10.3233/JIFS-202679 doi: 10.3233/JIFS-202679
![]() |
[10] |
O. Karasakal, Air defense missile-target allocation models for a naval task group, Comput. Oper. Res., 35 (2008), 1759–1770. https://doi.org/10.1016/j.cor.2006.09.011 doi: 10.1016/j.cor.2006.09.011
![]() |
[11] |
A. G. Kline, D. K. Ahner, B. J. Lunday, Real-time heuristic algorithms for the static weapon target assignment problem, J. Heuristics, 25 (2019), 377–397. https://doi.org/10.1007/s10732-018-9401-1 doi: 10.1007/s10732-018-9401-1
![]() |
[12] |
A. G. Kline, D. K. Ahner, B. J. Lunday, A heuristic and metaheuristic approach to the static weapon target assignment problem, J. Global Optim., 78 (2020), 791–812. https://doi.org/10.1007/s10898-020-00938-4 doi: 10.1007/s10898-020-00938-4
![]() |
[13] |
G. denBroeder Jr, R. Ellison, L. Emerling, On optimum target assignments, Oper. Res., 7 (1959), 322–326. https://doi.org/10.1287/opre.7.3.322 doi: 10.1287/opre.7.3.322
![]() |
[14] |
Z. J. Lee, C. Y. Lee, S. F. Su, An immunity-based ant colony optimization algorithm for solving weapon–target assignment problem, Appl. Soft Comput., 2 (2002), 39–47. https://doi.org/10.1016/S1568-4946(02)00027-3 doi: 10.1016/S1568-4946(02)00027-3
![]() |
[15] |
C. M. Lai, T. H. Wu, Simplified swarm optimization with initialization scheme for dynamic weapon–target assignment problem, Appl. Soft Comput., 82 (2019), 105542. https://doi.org/10.1016/j.asoc.2019.105542 doi: 10.1016/j.asoc.2019.105542
![]() |
[16] |
Z. J. Lee, S. F. Su, C. Y. Lee, Efficiently solving general weapon-target assignment problem by genetic algorithms with greedy eugenics, IEEE Trans. Syst. Man Cyber. B, 33 (2003), 113–121. https://doi.org/10.1109/TSMCB.2003.808174 doi: 10.1109/TSMCB.2003.808174
![]() |
[17] |
T. Chang, D. Kong, N. Hao, K. Xu, G. Yang, Solving the dynamic weapon target assignment problem by an improved artificial bee colony algorithm with heuristic factor initialization, Appl. Soft Comput., 70 (2018), 845–863. https://doi.org/10.1016/j.asoc.2018.06.014 doi: 10.1016/j.asoc.2018.06.014
![]() |
[18] | L. Juan, C. Jie, X. Bin, Efficiently solving multi-objective dynamic weapon-target assignment problems by NSGA-II, in 2015 34th Chinese Control Conference (CCC), (2015), 2556–2561. https://doi.org/10.1109/ChiCC.2015.7260033 |
[19] | J. Li, J. Chen, B. Xin, L. Dou, Solving multi-objective multi-stage weapon target assignment problem via adaptive NSGA-II and adaptive MOEA/D: A comparison study, in 2015 IEEE Congress on Evolutionary Computation (CEC), (2015), 3132–3139. https://doi.org/10.1109/CEC.2015.7257280 |
[20] |
K. Deb, A. Pratap, S. Agarwal, T. Meyarivan, A fast and elitist multiobjective genetic algorithm: NSGA-II, IEEE Trans. Evol. Comput., 6 (2002), 182–197. https://doi.org/10.1109/4235.996017 doi: 10.1109/4235.996017
![]() |
[21] |
Q. Zhang, H. Li, MOEA/D: A multiobjective evolutionary algorithm based on decomposition, IEEE Trans. Evol. Comput., 11 (2007), 712–731. https://doi.org/10.1109/TEVC.2007.892759 doi: 10.1109/TEVC.2007.892759
![]() |
[22] |
N. Srinivas, K. Deb, Muiltiobjective optimization using nondominated sorting in genetic algorithms, Evol. Comput., 2 (1994), 221–248. https://doi.org/10.1162/evco.1994.2.3.221 doi: 10.1162/evco.1994.2.3.221
![]() |
[23] |
F. Sarro, F. Ferrucci, M. Harman, A. Manna, J. Ren, Adaptive multi-objective evolutionary algorithms for overtime planning in software projects, IEEE Trans. Software Eng., 43 (2017), 898–917. https://doi.org/10.1109/TSE.2017.2650914 doi: 10.1109/TSE.2017.2650914
![]() |
[24] |
P. Arumugam, E. Amankwah, A. Walker, C. Gerada, Design optimization of a short-term duty electrical machine for extreme environment, IEEE Trans. Ind. Electron., 64 (2017), 9784–9794. https://doi.org/10.1109/TIE.2017.2711555 doi: 10.1109/TIE.2017.2711555
![]() |
[25] |
J. Zhou, J. Sun, X. Zhou, T. Wei, M. Chen, S. Hu, et al., Resource management for improving soft-error and lifetime reliability of real-time mpsocs, IEEE Trans. Comput. Aided Design Integr. Circuits Syst., 38 (2019), 2215–2228. https://doi.org/10.1109/TCAD.2018.2883993 doi: 10.1109/TCAD.2018.2883993
![]() |
[26] |
X. Zhang, Y. Tian, R. Cheng, Y. Jin, An efficient approach to nondominated sorting for evolutionary multiobjective optimization, IEEE Trans. Evol. Comput., 19 (2014), 201–213. https://doi.org/10.1109/TEVC.2014.2308305 doi: 10.1109/TEVC.2014.2308305
![]() |
[27] |
J. Chen, B. Xin, Z. Peng, L. Dou, J. Zhang, Evolutionary decision-makings for the dynamic weapon-target assignment problem, Sci. China Ser. F Inf. Sci., 52 (2009), 2006. https://doi.org/10.1007/s11432-009-0190-x doi: 10.1007/s11432-009-0190-x
![]() |
[28] |
Y. Wang, B. Xin, J. Chen, An adaptive memetic algorithm for the joint allocation of heterogeneous stochastic resources, IEEE Trans. Cybern., 52 (2021), 11526–11538. https://doi.org/10.1109/TCYB.2021.3087363 doi: 10.1109/TCYB.2021.3087363
![]() |
[29] |
K. Deb, H. Jain, An evolutionary many-objective optimization algorithm using reference-point-based nondominated sorting approach, part i: Solving problems with box constraints, IEEE Trans. Evol. Comput., 18 (2013), 577–601. https://doi.org/10.1109/TEVC.2013.2281535 doi: 10.1109/TEVC.2013.2281535
![]() |
[30] |
K. Li, R. Chen, G. Fu, X. Yao, Two-archive evolutionary algorithm for constrained multiobjective optimization, IEEE Trans. Evol. Comput., 23 (2018), 303–315. https://doi.org/10.1109/TEVC.2018.2855411 doi: 10.1109/TEVC.2018.2855411
![]() |
[31] |
H. Jain, K. Deb, An evolutionary many-objective optimization algorithm using reference-point based nondominated sorting approach, part ii: Handling constraints and extending to an adaptive approach, IEEE Trans. Evol. Comput., 18 (2013), 602–622. https://doi.org/10.1109/TEVC.2013.2281534 doi: 10.1109/TEVC.2013.2281534
![]() |
[32] |
A. A. Ghanbari, H. Alaei, Meta-heuristic algorithms for resource management in crisis based on OWA approach, Appl. Intell., 51 (2021), 646–657. https://doi.org/10.1007/s10489-020-01808-y doi: 10.1007/s10489-020-01808-y
![]() |
[33] |
X. Wu, C. Chen, S. Ding, A modified moea/d algorithm for solving bi-objective multi-stage weapon-target assignment problem, IEEE Access, 9 (2021), 71832–71848. https://doi.org/10.1109/ACCESS.2021.3079152 doi: 10.1109/ACCESS.2021.3079152
![]() |
[34] |
C. A. C. Coello, N. C. Cortés, Solving multiobjective optimization problems using an artificial immune system, Genet. Program. Evol. Mach., 6 (2005), 163–190. https://doi.org/10.1007/s10710-005-6164-x doi: 10.1007/s10710-005-6164-x
![]() |
[35] | H. Ishibuchi, H. Masuda, Y. Nojima, Sensitivity of performance evaluation results by inverted generational distance to reference points, in 2016 IEEE Congress on Evolutionary Computation (CEC), (2016), 1107–1114. https://doi.org/10.1109/CEC.2016.7743912 |
[36] |
E. Zitzler, L. Thiele, Multiobjective evolutionary algorithms: A comparative case study and the strength pareto approach, IEEE Trans. Evol. Comput., 3 (1999), 257–271. https://doi.org/10.1109/4235.797969 doi: 10.1109/4235.797969
![]() |
[37] | K. Deb, S. Jain, Running performance metrics for evolutionary multi-objective optimization, in Proceedings of the Fourth Asia-Pacific Conference on Simulated Evolution and Learning (SEAL'02), (Singapore), (2002), 13–20. https://doi.org/10.1016/S1350-4789(02)80143-X |
[38] |
M. Srinivas, L. M. Patnaik, Adaptive probabilities of crossover and mutation in genetic algorithms, IEEE Trans. Syst. Man Cybern., 24 (1994), 656–667. https://doi.org/10.1109/21.286385 doi: 10.1109/21.286385
![]() |
[39] |
W. Xu, C. Chen, S. Ding, P. M. Pardalos, A bi-objective dynamic collaborative task assignment under uncertainty using modified MOEA/D with heuristic initialization, Expert Syst. Appl., 140 (2020), 112844. https://doi.org/10.1016/j.eswa.2019.112844 doi: 10.1016/j.eswa.2019.112844
![]() |
[40] |
I. Voutchkov, A. Keane, A. Bhaskar, T. M. Olsen, Weld sequence optimization: The use of surrogate models for solving sequential combinatorial problems, Comput. Methods Appl. Mech. Eng., 194 (2005), 3535–3551. https://doi.org/10.1155/IMRN.2005.3551 doi: 10.1155/IMRN.2005.3551
![]() |
[41] |
W. Luo, J. Lü, K. Liu, L. Chen, Learning-based policy optimization for adversarial missile-target assignment, IEEE Trans. Syst. Man Cybern. Syst., 52 (2021), 4426–4437. https://doi.org/10.1109/TSMC.2021.3096997 doi: 10.1109/TSMC.2021.3096997
![]() |
1. | Chengqiang Wang, Xiangqing Zhao, Can Wang, Zhiwei Lv, Synchronization of Takagi–Sugeno Fuzzy Time-Delayed Stochastic Bidirectional Associative Memory Neural Networks Driven by Brownian Motion in Pre-Assigned Settling Time, 2023, 11, 2227-7390, 3697, 10.3390/math11173697 | |
2. | A. Karnan, G. Nagamani, Event-triggered extended dissipative synchronization for delayed neural networks with random uncertainties, 2023, 175, 09600779, 113982, 10.1016/j.chaos.2023.113982 |