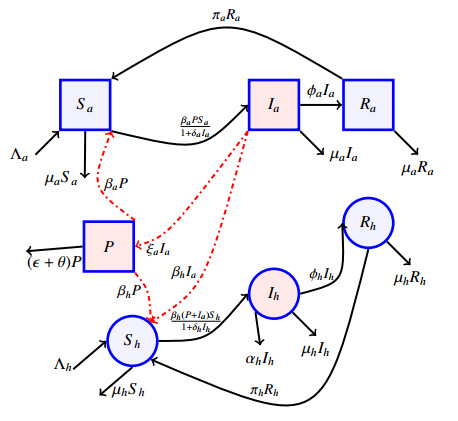
Anthrax is an acute infectious zonootic disease caused by Bacillus anthracis, a gram-positive, rod-shaped non-motile bacterium. It is a disease that mainly affects herbivorous animals of both domestic and wildlife, and causes devastating spillover infections into the human population. Anthrax epidemic results in serious and fatal infections in both animals and humans globally. In this paper, a non-linear differential equation model is proposed to study the transmission dynamics of anthrax in both animal and human populations taking into accounts saturation effect within the animal population and behavioural change of the general public towards the outbreak of the disease. The model is shown to have two unique equilibrium points, namely; the anthrax-free and endemic equilibrium points. The anthrax-free equilibrium point is globally asymptotically stable whenever the reproduction number is less than unity (R0<1) and the endemic equilibrium point is locally asymptotically stable whenever R0>1. Sensitivity analysis suggests that the most influential factors on the spread of anthrax are the infection force βa, pathogen shedding rate ξa, recruitment rate Λa, natural death rate in animals μa and recovery rate in animals ϕa. Numerical simulations demonstrate that the saturation effect and behavioural change of the general public towards the outbreak of the disease increase the size of the susceptible population, reduce the size of the infective population and the pathogen levels in the environment. Findings of this research show that anthrax epidemic can be controlled by reducing the rate of anthrax infection and pathogen shedding rate, while increasing the rate of pathogen decay through proper environmental hygiene as well as increasing treatment to ensure higher recovery rate in infected animals. The results also show that positive behavioural change of the general public through mass awareness interventions can help control the spread of the disease.
Citation: Elijah B. Baloba, Baba Seidu. A mathematical model of anthrax epidemic with behavioural change[J]. Mathematical Modelling and Control, 2022, 2(4): 243-256. doi: 10.3934/mmc.2022023
[1] | Ankur Jyoti Kashyap, Arnab Jyoti Bordoloi, Fanitsha Mohan, Anuradha Devi . Dynamical analysis of an anthrax disease model in animals with nonlinear transmission rate. Mathematical Modelling and Control, 2023, 3(4): 370-386. doi: 10.3934/mmc.2023030 |
[2] | Yaxin Ren, Yakui Xue . Modeling and optimal control of COVID-19 and malaria co-infection based on vaccination. Mathematical Modelling and Control, 2024, 4(3): 316-335. doi: 10.3934/mmc.2024026 |
[3] | Mlyashimbi Helikumi, Paride O. Lolika . Dynamics and analysis of COVID-19 disease transmission: The effect of vaccination and quarantine. Mathematical Modelling and Control, 2023, 3(3): 192-209. doi: 10.3934/mmc.2023017 |
[4] | Paride O. Lolika, Mlyashimbi Helikumi . Global stability analysis of a COVID-19 epidemic model with incubation delay. Mathematical Modelling and Control, 2023, 3(1): 23-38. doi: 10.3934/mmc.2023003 |
[5] | Ihtisham Ul Haq, Nigar Ali, Shabir Ahmad . A fractional mathematical model for COVID-19 outbreak transmission dynamics with the impact of isolation and social distancing. Mathematical Modelling and Control, 2022, 2(4): 228-242. doi: 10.3934/mmc.2022022 |
[6] | Erick M. D. Moya, Diego Samuel Rodrigues . A mathematical model for the study of latent tuberculosis under 3HP and 1HP regimens. Mathematical Modelling and Control, 2024, 4(4): 400-416. doi: 10.3934/mmc.2024032 |
[7] | J. O. Akanni, S. Ajao, S. F. Abimbade, Fatmawati . Dynamical analysis of COVID-19 and tuberculosis co-infection using mathematical modelling approach. Mathematical Modelling and Control, 2024, 4(2): 208-229. doi: 10.3934/mmc.2024018 |
[8] | Abdul-Fatawu O. Ayembillah, Baba Seidu, C. S. Bornaa . Mathematical modeling of the dynamics of maize streak virus disease (MSVD). Mathematical Modelling and Control, 2022, 2(4): 153-164. doi: 10.3934/mmc.2022016 |
[9] | Anil Chavada, Nimisha Pathak, Sagar R. Khirsariya . A fractional mathematical model for assessing cancer risk due to smoking habits. Mathematical Modelling and Control, 2024, 4(3): 246-259. doi: 10.3934/mmc.2024020 |
[10] | Anita T. Kurniawati, Fatmawati, Chidozie W. Chukwu, Windarto, Faishal F. Herdicho . Optimal control of dengue fever model with a logistically growing human population. Mathematical Modelling and Control, 2025, 5(1): 48-60. doi: 10.3934/mmc.2025004 |
Anthrax is an acute infectious zonootic disease caused by Bacillus anthracis, a gram-positive, rod-shaped non-motile bacterium. It is a disease that mainly affects herbivorous animals of both domestic and wildlife, and causes devastating spillover infections into the human population. Anthrax epidemic results in serious and fatal infections in both animals and humans globally. In this paper, a non-linear differential equation model is proposed to study the transmission dynamics of anthrax in both animal and human populations taking into accounts saturation effect within the animal population and behavioural change of the general public towards the outbreak of the disease. The model is shown to have two unique equilibrium points, namely; the anthrax-free and endemic equilibrium points. The anthrax-free equilibrium point is globally asymptotically stable whenever the reproduction number is less than unity (R0<1) and the endemic equilibrium point is locally asymptotically stable whenever R0>1. Sensitivity analysis suggests that the most influential factors on the spread of anthrax are the infection force βa, pathogen shedding rate ξa, recruitment rate Λa, natural death rate in animals μa and recovery rate in animals ϕa. Numerical simulations demonstrate that the saturation effect and behavioural change of the general public towards the outbreak of the disease increase the size of the susceptible population, reduce the size of the infective population and the pathogen levels in the environment. Findings of this research show that anthrax epidemic can be controlled by reducing the rate of anthrax infection and pathogen shedding rate, while increasing the rate of pathogen decay through proper environmental hygiene as well as increasing treatment to ensure higher recovery rate in infected animals. The results also show that positive behavioural change of the general public through mass awareness interventions can help control the spread of the disease.
Anthrax is a zoonotic disease that remains a problem in many countries worldwide [1]. It is caused by the bacterium Bacillus anthracis. The disease primarily affects herbivorous animals, but outbreaks in humans are largely reported. The hosts of Bacillus anthracis include domestic and wild animals such as sheep, goats, cattle, horses, pigs, camels, antelope, deer and buffaloes [2]. Birds are rarely infected with anthrax, but cats and dogs that feed on anthrax-contaminated meat may become infected and often recover from the disease without treatment [5,6]. It is worth noting that, not all infected animals die from anthrax. The survival or otherwise of an anthrax-infected animal probably depends on the dose of pathogens and the natural immunity of the animal [5].
Bacillus anthracis is a gram-positive, rod-shaped non-motile bacterium, the only obligate aerobic pathogen in the genus Bacillus. The bacterium has both vegetative form and a long-lasting, highly resistant spore form [3,4]. The bacterium exclusively remains in the vegetative form within a low oxygen environment of the host [3]. The anthrax Bacillus forms spores to protect itself when conditions are unfavorable. Spores have a thick outer coating which shields the bacteria from extreme conditions [5]. When an anthrax spore enters into its host, it germinates and results into a vegetative cell and replicates rapidly. The replicated vegetative cells then enter the bloodstream and result in septicemia and eventually lead to the sudden death of the host [4]. Anthrax spores have long life span, capable of persisting in the environment for decades before infecting a new host. The spores are usually present at sites where anthrax-infected carcasses decomposed for many years. The spores have been known to re-infect animals over 70 years after burial sites of anthrax-infected animals were disturbed [7]. Domestic and wild animals can become infected when they breathe in or ingest spores in contaminated soil, plants, or water [8]. Following inhalation or ingestion, the anthrax bacteria spread rapidly throughout the entire body of the animal. Dead animals that are opened and not burned or buried provide an ideal source of new spores to contaminate the soil [6]. The incubation period of Bacillus anthracis in animals after exposure to spores is between 3 to 7 days, but it may range from less than 1 day to 14 days or more. As soon as clinical signs appear, the infected animals usually die within 2 days [9]. Upon or near death, blood oozes from the body openings of the infected animal into the environment. This blood is heavily laden with anthrax organisms. The carcass has a marked bloating and decomposes rapidly [6].
Anthrax infection in human population results from accidental exposure [13], and is based on rural agricultural activities or industrial processing [14]. The risk of humans contracting anthrax is related to many factors including the origin of infection [15]. The source of anthrax infection includes cutaneous, gastrointestinal and inhalation anthrax [16]. The cutaneous or skin infection occurs when anthrax spores invade a cut or abrasion, this happens when a person handles infected animals or contaminated animal products like wool, hides, or hair [6,8]. Symptoms of cutaneous infection of anthrax include itching of the infected part, followed by swelling and discoloration [8]. Cutaneous anthrax has a mortality rate between 5%−20%, if infections are not treated [17]. Cutaneous anthrax accounts for approximately 95% of all reported human anthrax cases [18].
Gastrointestinal anthrax occurs as a result of eating the meat of animals infected with the vegetative Bacillus anthracis, in addition, anthrax spores can also enter the body via mucous membranes that line the mouth and intestines [5,19]. The intestinal tract, mouth, or throat may be infected [19]. Symptoms of gastrointestinal anthrax may include nausea, vomiting, anorexia, mild diarrhoea and fever. The incubation period is commonly 3–7 days [18]. The fatality rate of gastrointestinal anthrax without treatment is ≥50%, but <40% with treatment [9].
Inhalation anthrax occurs as a result of breathing anthrax spores into the lungs [19]. The incubation period reported for inhalation anthrax in humans ranges from 1 to 43 days [9]. Symptoms include fever or chills, sweats, fatigue or malaise, non-productive cough, dyspnoea, changes in mental state including confusion, and nausea or vomiting [18]. The case-fatality rate of inhalation anthrax in humans is >85% [9].
Anthrax is global in its geographical distribution and endemic in agricultural regions of Southern and Eastern Europe, Central and Southwestern Asia, Sub-Saharan Africa, North and South America, and Australia [4,8,9,10]. It is estimated that there are 2,000 to 20,000 human anthrax cases occurring annually worldwide [11]. Apart from its natural occurrence, Bacillus anthracis is currently considered one of the most serious bioterrorism threats [10,19]. Anthrax is still endemic in most African countries, majority of which experience at least one human outbreak per year [12].
The use of mathematical models to explore the spread and control of infectious diseases has proved to be an important tool for scientists and epidemiologists [20]. This research seeks to use mathematical models to study the transmission dynamics of anthrax in animal and human populations. There are quite some number of mathematical models proposed for the study of anthrax dynamics, these include; [21,22,23,24,25,26] among others, but not so much is known about the transmission dynamics of anthrax infection in both animal and human populations using saturation incidence. This work therefore seeks to study the infection dynamics of anthrax using non-linear saturation incidence rate.
Incidence functions form a very important integral part of research in mathematical epidemiology modeling. In [27], incidence rate refers to the frequency with which a disease occurs over a specified period of time, thus it is the number of new cases of a disease within a time period, as a proportion of the number of individuals who are at risk of being infected with the disease. The most widely used incidence functions in epidemic models include the bilinear incidence βSI and standard incidence βSIN [28]. According to [29], the bilinear incidence is based on the law of mass action, which requires a well-mixed population so that each infected individual has equal probability of infecting each susceptible individual. In [30], the bilinear incidence assumes that the disease transmission increases whenever the susceptible population increases which is a limitation of the function because of the intervention of control measures during disease outbreak. [31] defines standard incidence as the number of new cases per unit time due to infectious individuals. The standard incidence is often used, due to the fact that the number of contacts per individual is approximately constant, the standard incidence may be more suitable for human beings, or animals who live in groups [32]. The standard incidence is more suitable than the bilinear form [20].
The concept of saturation incidence was established by Capasso and Serio. The saturation incidence is given by βSI1+αI where βI measures the infection force of the disease and 11+αI measures the inhibition effect from the behavioural change of the susceptible individuals when their number increases. Thus, βI1+αI tends to a saturation level when the infective I gets large [28,33]. Saturation incidence may be mores suitable for our real world situations over bilinear and standard incidence [28]. If a population is crowded or saturated with infectives, then saturated incidence is a better option [29]. The saturation incidence rate is nonmonotone, it has the quality of first increasing and then decreasing when the number of infective individuals reaches a critical value [34].
In this section, a deterministic model is formulated from the work of [35] for the study of anthrax transmission in animal and human populations with much emphasis on saturation and behavioural change within the animal and human populations respectively, at the peak of the disease spread. In formulating the model, it is assumed that anthrax is spread through environmental contamination. Upon infection, an infected animal shows clinical symptoms of the disease and can be cured by treatment. It is further assumed that there is no human to human route of infection.
Figure 1 presents a compartmental model for the transmission of anthrax in animal and human populations. The animal population is subdivided into three compartments of susceptible animals Sa, infective animals Ia, and the class of animals who recovered from the disease Ra. The human population consists of susceptible humans Sh, the infective humans Ih and the population of infective individuals who recovered from the disease Rh due to treatment or natural immunity. Compartment P consists of the environmental reservoir contaminated with anthrax spores or pathogens. Table 1 summarizes the definition of parameters used in the model.
Par. | Description | Value | Ref. |
βa | Infection rate of susceptible animals | 0.02 | [35] |
Λa | The rate of inflow of animals | 0.99 | [24] |
ϕa | The recovery rate of infected animals | 0.0025 | [25] |
μa | Natural death rate in animals | 0.0001 | [24] |
δa | The saturation effect of the animal population | 0.6 | Assumed |
πa | Rate at which recovered animals become susceptibles | 0.5 | [24] |
βh | Infection rate of susceptible humans | 0.0001 | [35] |
Λh | Recruitment rate of humans | 0.92 | [24] |
ϕh | The recovery rate of infected humans | 0.04 | [25] |
μh | Natural death rate in humans | 0.0001 | [24] |
ξa | Pathogen shedding rate | 0.45 | [25] |
δh | Rate of behavioural change | 0.6 | Assumed |
πh | Rate at which recovered humans become susceptible | 0.5 | Assumed |
ϵ | Rate of natural pathogens decay from the environment | 0.8 | [24] |
θ | Rate of decay due to environmental hygiene | 0.5 | [24] |
The susceptible animal population is increased by an inflow rate of Λa. The susceptible animals in Sa become infected when they ingest anthrax contaminated feed or breathe in spores in contaminated environment at a rate of βaPSa which moves the newly infected population into the infected class Ia with a saturation incidence of 11+δaIa. The saturation incidence of the animal population measures the crowding effect of the infected animals. δa is the saturation factor that measures the inhibitory effect due to protection measures taken by farmers and the general public at large. The animals that show clinical symptoms of the disease in the infective class, Ia are treated and undergo recovery at rate of ϕaIa. The treated animals then join a temporal class of Ra for further monitory and other intensive care. Upon full recovery, the recovered population confer temporary immunity and join compartment Sa at a rate of πaRa. The Susceptible, Infected and Recovered animal population reduce in population size by natural death rate of μa. ξa is the rate at which infective animals shed anthrax pathogens into the environment. The anthrax pathogen level in the environment P decays due to the practice of environmental hygiene and by natural phenomenon at rates of θP and ϵP respectively.
The human population suffers a spillover transmission of anthrax from outbreaks of the disease within the animal population.
The susceptible human population Sh increases through recruitment at a rate of Λh. Susceptible humans become infected with anthrax through effective contact with pathogen sources (P,Ia) at a constant rate of βh.
In the infective human class Ih, infected population recover from the disease due to treatment or natural recovery at a rate of ϕhIh and joins the recovery compartment Rh. Individuals in Rh become susceptible at a rate of πhRh due to waning of infection-acquired immunity. The Susceptible, Infected and Recovered humans die naturally at rates μhSh, μhIh and μhRh respectively. The infective human population also suffer from anthrax related deaths at rate αhIh.
During an anthrax epidemic, the general public especially farmers react behaviourally, psychologically, and socially towards the disease spread. These attitudes may have an impact on the overall infection rate or number of infectious persons as well as the susceptible human population through the incidence rate. As the number of infective individuals increases, the susceptible human population may tend to reduce the number of contacts with infective animal population per unit time due to the behavioural effect. The incidence rate which describes the behavioural change towards the outbreak of the disease is given as βhSh(P+Ia)1+δhIh, where δh>0 is the behavioural parameter. This incidence rate describes the transmission of the anthrax pathogen from infective animals to susceptible humans taking into accounts the behavioural effect of the general public towards the spread of the disease.
The corresponding model is presented as a system of differential equations indicated in equation (2.1).
{dSadt=Λa+πaRa−βaPSa1+δaIa−μaSa,dIadt=βaPSa1+δaIa−(μa+ϕa)Ia,dRadt=ϕaIa−(μa+πa)Ra,dPdt=ξaIa−(ϵ+θ)P,dShdt=Λh+πhRh−βh(P+Ia)Sh1+δhIh−μhSh,dIhdt=βh(P+Ia)Sh1+δhIh−(μh+αh+ϕh)Ih,dRhdt=ϕhIh−(πh+μh)Rh. | (2.1) |
with non-negative initial conditions;
Sa(0)>0,Ia(0)≥0,Ra(0)≥0,Sh(0)>0,
Ih(0)≥0,Rh(0)≥0,P(0)≥0.
For convenience, the following variables will be substituted where appropriate;
ca1=(μa+ϕa),ca2=(μa+πa),ca4=(ϵ+θ),ch1=(μh+αh+ϕh),ch2=(μh+πh),ga=1+δaIa,gh=1+δhIh,ka=11+δaIa, and kh=11+δhIh. |
The total population size of the system (2.1) is given by:
{Na(t)=Λa−Naμa,Nh(t)=Λh−Nhμh−αhIh,dPdt=ξaIa−(ϵ+θ)P(t). | (2.2) |
where Na(t)=Sa+Ia+Ra and Nh(t)=Sh+Ih+Rh represent the total population size of animals and humans respectively. The animal and human populations can be simplified as follows:
{dNadt≤Λa−Naμa,dNhdt≤Λh−Nhμh. | (2.3) |
In the next section, basic properties of the model system (2.1) are discussed. These properties are useful for stability analysis.
The study of the invariant region is carried-out to determine whether the solutions of the model system (2.1) make biological sense.
Recall that,
dNadt≤Λa−Naμa. | (3.1) |
dNhdt≤Λh−Nhμh. | (3.2) |
The closed set
Ω={X∈R7+|Na≤Λaμa,,Nh≤Λhμh,P≤ξaΛaca4μa} |
is a feasible region to the model (2.1), where X=(Sa,Ia,Ra,P,Sh,Ih,Rh).
Lemma 3.1. Let (Sa,Ia,Ra,P,Sh,Ih,Rh) be the solution of the model system (2.1) with non-negative initial conditions. The closed set Ω is bounded and positively invariant.
Proof. We construct the proof by applying the theorem on the differential inequality [36] to equation (3.1). Solving the first equation of (3.1) gives:
Na≤1μa[Λa(1−e−μat)+Na0μae−μat]. | (3.3) |
Thus, the size of the animal population Na approaches Λaμa as t→∞.
Similarly, as t→∞, then Nh→Λhμh.
Hence, all solutions of the system (2.1) are contained in the region Ω. Thus, Ω is bounded and positively invariant region for the model.
This section presents a proof that all state variables of the model system (2.1) remain non-negative for all non-negative initial conditions for t≥0 and that the model equations are continuous and locally Lipschitz in (Sa,Ia,Ra,P,Sh,Ih,Rh)∈R7+, hence solutions of the system exist for all positive time. This is necessary since the model is a biological system representing populations of animals and humans.
The model system (2.1) can be written in the form
x′=F(t,x)=F(x), |
with x=(Sa,Ia,Ra,P,Sh,Ih,Rh) and F(x)=(F1(x),F2(x),...,F7(x)). Thus,
F(x)=(Λa+πaRa−βaPSa1+δaIa−μaSaβaPSa1+δaIa−(μa+ϕa)IaϕaIa−(μa+πa)RaξaIa−(ϵ+θ)PΛh+πhRh−βh(P+Ia)Sh1+δhIh−μhShβh(P+Ia)Sh1+δhIh−(μh+αh+ϕh)IhϕhIh−(πh+μh)Rh.). |
Proposition 3.2. For any Sa(0),Ia(0),Ra(0),P(0),Sh(0),Ih(0),Rh(0)≥0 there exists a unique solution Sa0,Ia0,Ra0,P0,Sh0,Ih0,Rh0 respectively, defined on R7+=[0,∞)7. Also, Fj(x,t)≥0 for every j=1,2,...,7 if x∈[0,∞)7 and xj=0 for t≥0. In addition x(0)∈[0,∞).
Theorem 3.3. ([39]). Let Rn+=[0,∞)n be the cone of nonnegative vectors in Rn+. Let F:Rn+1+→Rn be locally Lipschitz and
Fj(x,t)≥0 whenever t≥0, x∈R7+, xj=0 for j=1,2,...,7.
Then, for every x0∈R7+ there exist a unique solution of x′=F(x,t), x(0)=x0, with values in R7+ which is defined on some interval [0,b), b>0. If b<∞. Then lim supt↗b7∑j=1xj=∞.
Proof. From the first equation of the model system (2.1) if Sa=0 then
dSadt=Λa+πaRa≥0. |
It follows that dIadt≥0 if Ia=0, dRadt≥0 if Ra=0, dPdt≥0 if P=0, dShdt≥0 if Sh=0, dIhdt≥0 if Ih=0, dRhdt≥0 if Rh=0 where Sa,Ia,Ra,P,Sh,Ih,Rh∈R7+.
Also,
lim supt↗b(Sa(t)+Ia(t)+Ra(t)+P(t)+Sh(t)+Ih(t)+Rh(t))=∞. |
Lemma 3.4 (Lipschitz condition). The system equations Fj(x) for j=1,2,...,7 satisfy Lipschitz's conditions and are contraction mappings, if the following condition holds,
0<b=max(b1,b2,b3,b4,b5,b6,b7)<∞. |
Applying the Lipschitz condition in Lemma (3.2) on the first equation of system (2.1) where Sa,S′a∈R7+ gives
‖F1(Sa)−F1(S′a)‖=‖[Λa+πaRa−βaPSa1+δaIa−μaSa]−[Λa+πaRa−βaPS′a1+δaIa−μaS′a]‖,≤||(−βaPSa1+δaIa−μaSa)−(−βaPS′a1+δaIa−μaS′a)||,≤|−1|‖(βaP1+δaIa+μa)Sa−(βaP1+δaIa+μa)S′a‖,≤(βaP1+δaIa+μa)||Sa−S′a||,≤b1||Sa−S′a||. |
where b1=βaP1+δaIa+μa thus, F1(t,Sa) satisfies the Lipschitz condition. Similarly, it can be shown that Fj(x) for j=2,...,7 also satisfy the Lipschitz condition for
0<max{b2,b3,b4,b5,b6,b7}<∞. |
Next, it is important to show that Fj(x),∀j are continuously differentiable and bounded in R7+, by first determining the norm of the partial derivatives of the system equations in (2.1). From the first equation of system (2.1) gives
|∂F1∂Sa|=|−(βaP1+δaIa+μa)|<∞,|∂F1∂Ia|=|βaPSaδa(1+δaIa)2|<∞,|∂F1∂Ra|=|πa|<∞,|∂F1∂P|=|−βaSa1+δaIa|<∞,|∂F1∂Sh|=|∂F1∂Ih|=|∂F1∂Rh|=0<∞. |
By extending the same analogy to the remaining equations in system (2.1), it can be established that ∂Fj(t,x)∂xi<∞ for i,j=2,...,7. Therefore, all the partial derivatives are continuous and bounded. Hence, there exist a unique solution for the model system (2.1) for all positive time. Thus the model system is well posed.
Two types of equilibrium points are studied in this section, the anthrax-free equilibrium point and the endemic equilibrium point. The equilibrium points of the model system are obtained by first setting the differential equations in (2.1) to zero as given in (3.4).
{0=Λa+πaRa−βakaPSa−μaSa,0=βakaPSa−ca1Ia,0=ϕaIa−ca2Ra,0=ξaIa−ca4P,0=Λh+πhRh−βhkh(P+Ia)Sh−μhSh,0=βhkh(P+Ia)Sh−ch1Ih,0=ϕhIh−ch2Rh. | (3.4) |
The anthrax-free equilibrium point is a steady state solution where there is no disease spreading in the populations. Thus, disease-free equilibrium point of the system is attained when all the variables and parameters related to anthrax infection are zero. Thus, Ia=0,P=0 and Ih=0.
From the first equation of (3.4), Sa=1μa(Λa+πaRa), plugging in Ra=0 gives Sa=1μaΛa. Also, from the fifth equation of (3.4), Sh=1μh(Λh+πhRh) and substituting Rh=0 gives Sh=1μhΛh. Therefore, the anthrax-free equilibrium point is given as E0={Sa0,0,0,0,Sh0,0,0}, where Sa0=Λaμa and Sh0=Λhμh.
Next the basic reproduction number is determined using the method described in [37]. The basic reproduction number is defined as the average number of secondary anthrax infections a single infected animal causes during its infectious life, if it is introduced into an initially anthrax-free human-animal system [35]. The basic reproduction number R0 is the dominant eigenvalue of ρ(FV−1) corresponding to the spectral radius of the next generation matrix FV−1, where F and V−1 are the transmission and the inverse of transition matrices respectively. For this model, the F and V−1 matrices are defined as follows
F=[−βaPSaδa(Iaδa+1)2βaSaIaδa+10000βhShIhδh+1βhShIhδh+1−βh(P+Ia)Shδh(Ihδh+1)2], |
V−1=[1ca100ξaca1ca41ca40001ch1]. |
Evaluating FV−1 at the anthrax-free equilibrium point E0 and solving give
the reproduction number of the model system as
R0=βaΛaξaμa(μa+ϕa)(ϵ+θ) |
.
It is noticed that the infection force of the human population βh does not contribute to the reproduction number R0, this shows that the stability of anthrax epidemic within the animal-human system is strongly influenced by the spread of the disease within the animal population. Thus, controlling the anthrax transmission within the animal population will lead to a reduction in the spread of the disease within the human population.
The endemic equilibrium point is a non-disease free state of the model, it is the point at which the anthrax persist in the populations. It is obtained by setting the derivatives in the model system (2.1) equal to zero and solving for the state variables. The endemic equilibrium point is given by E∗={S∗a,I∗a,R∗a,P∗,S∗h,I∗h,R∗h}, where the elements of E∗ are defined by equation (3.5).
{S∗a=R0(πaϕa(R0−1)+Λaδaca2)ca4(βaξa(R0−1)+R0μaδaca4)ca2,I∗a=R0−1δa,R∗a=(R0−1)ϕaδaca2,P∗=(R0−1)ξaδaca4,S∗h=ch1I∗h(1+δhI∗h)βh(P∗+I∗a),R∗h=ϕhI∗hch2. | (3.5) |
where Ih satisfies the function H(Ih)=0, defined by
H(Ih)=μhch1ch2δhI2h+Ψ1Ih−Λhβhch2(P∗+I∗a) |
where Ψ1=[ch2(βhch1(P∗+I∗a)+μhch1)−πhβh(P∗+I∗a)ϕh]. It can be verified that H(0)<0 and also that H(Ih)→+∞ as Ih→+∞.
This section presents a study that investigates the local stability of the anthrax free equilibrium point using the indirect Lyapunov method. By this method, the local asymptotically stability of a model system is proofed by showing that all eigenvalues of the Jacobian matrix J, evaluated at anthrax-free equilibrium have negative real parts, otherwise the anthrax-free equilibrium is unstable.
Theorem 3.5 (Local stability of anthrax-free equilibrium). The anthrax-free equilibrium, E0 is locally asymptotically stable whenever R0<1 and unstable otherwise.
Proof. The Jacobian matrix of the model system (2.1) is given as,
JE0=[−μa0πa−βaΛaμa0000−ca10βaΛaμa0000ϕa−ca200000ξa0−ca40000−Λhβhμh0−Λhβhμh−μh0πh0Λhβhμh0Λhβhμh0−ch1000000ϕh−ch2]. |
From the Jacobian matrix JE0, it is easy to show that μh,μa,ch1 and ch2 are the negative eigenvalues of the Jacobian of the model evaluated at E0 and the remaining two eigenvalues can be shown to be negative if R0<1. Therefore, the anthrax-free equilibrium is locally asymptotically stable when ever R0<1, concluding the proof.
The local stability of the endemic equilibrium is determined by linearizing the system evaluated at the endemic equilibrium points using the Jacobian matrix approach. The characteristic equation of the Jacobian matrix of the system is obtained by the equation |JE∗−λI|=0.
Theorem 3.6 (Local stability of the endemic equilibrium). The endemic equilibrium, E∗ is locally asymptotically stable whenever R0>1 and unstable otherwise.
Proof. Using the Jacobian matrix of the system evaluated at endemic equilibrium points the characteristic equation is given in (3.6).
λ7+λ6A6+λ5A5+λ4A4+λ3A3+λ2A2+λA1+A0=0 | (3.6) |
where,
A6=βhR0(R0−1)ξa+ca4βhR0(R0−1)+βagh(R0−1)ξa+k1δaca4δaca4,A5=R0ghδa2ca42k2+[βaβh(R0−1)ξa2+R0ca4](R0−1)R0ghδa2ca42+[(δa(βagh+βh)ca4+(βaghk5+βhk6)δa+βaβh(R0−1))ca4ξa](R0−1)R0ghδa2ca42,A4=R0δaca42(k15δagh+βhk13)+(R0−1)2βhβak8ξa(1+ca4)R0ghδa2ca42+[(k10βagh+k14R0βh)δaξaca4+R0k9δaβh]ca4(R0−1)R0ghδa2ca42,A3=(R0−1)(βhβaξak23ca4(R0−1)+1+k17+ca4k29+δaca4k19)+δa2ca42k26R0R0ghδa2ca42,A2=βa(βh(R0−1))2k40+(R0−1)(βhR0k39+βak31ξa+βhk30ξa)δaca4+δa2ca42k38R0ghδa2ca42,A1=βa(βh(ca1ca4+ca2ca4)(R0−1))2ξa2k43+(R0−1)k51R0gh(δaca4)2,A0=(R0−1)2k52+(R0−1)βaca43ch1ch2ghμhk43δaR0ghδa2ca42 |
It can be noticed that Ai>0∀i, if R0>1. By Descartes' rule of signs, the characteristic equation in (3.6) has all its roots in the left half of the real plane. Thus, the eigenvalues of the Jacobian matrix of the model evaluated at the endemic equilibrium point have all it's real parts negative whenever R0>1. Hence, theorem (3.6) is satisfied.
[48] outlined two conditions for determining the global asymptotic stability of anthrax-free equilibrium of a system which is rewritten in the form:
dQdt=F(Q,W),dWdt=G(Q,W),G(Q,0)=0, | (3.7) |
where Q∈Rm represents the number of uninfected individuals W∈Rn denotes the number of infected population and E0=(Q∗,0) represents the anthrax-free equilibrium. The following assumptions must be satisfied for anthrax-free equilibrium of a system to be globally asymptotically stable:
H1=dQdt=F(Q,0), Q∗ is globally asymptotically stable. H2=G(Q,W)=AW−G∗(Q,W), where G∗(Q,W)≥0 for (Q,W)∈Ω, and A=DWG(Q∗,0) is an M–matrix (the off diagonal elements are nonnegative).
Theorem 3.7. The equilibrium point E0 of the system (2.1) is globally asymptotically stable if R0<1(locally asymptotically stable) and the assumptions H1 and H2 are satisfied.
Proof.
Let Q(Sa,Ra,Sh,Rh)∈R4 and Q(Ia,P,Ih,)∈R3
F(Q,W)=(Λa+πaRa−βaPSa1+δaIa−μaSaϕaIa−ca2RaΛh+πhRh−βh(P+Ia)Sh1+δhIh−μhShϕhIh−ch2Rh), |
G(Q,W)=(βaPSa1+δaIa−ca1IaξaIa−ca4Pβh(P+Ia)Sh1+δhIh−ch1Ih). |
The reduced system is given as dQdt=F(Q,0)
dQdt={dSadt=Λa−μaSa,dShdt=Λh−μhSh. | (3.8) |
The reduced system dQdt=F(Q,0) is globally asymptotically stable at the anthrax-free equilibrium point E0=(Λaμa,0,Λhμh). It can be shown from the first equation of (3.8) that Sa(t)=Λaμa+(Sa(0)−Λaμa)e−μat, this implies that Sa(t)→Λaμa as t→∞. Similarly, from the second equation of (3.8), Sh(t)→Λhμh as t→∞. It can be observed that this particular dynamics does not depend on the initial conditions. Hence, the convergence of the solutions of the reduced system in (3.8) is global in Ω.
DWG=(−βaPSaδa(Iaδa+1)2−ca1βaSa(Iaδa+1)0ξa−ca40βhSh(Ihδh+1)βhSh(Ihδh+1)−βh(P+Ia)Shδh(Ihδh+1)2−ch1). |
From the second assumption H2, G(Q,W) can be expressed as G(Q,W)=DWG(Q∗,0)W−G∗(Q,W).
G∗(Q,W)=(βa(Λaμa−Sa)P1+δaIa0βh(P+Ia)(Λhμh−Sh)1+δhIh). |
Clearly, since Sa and Sh are bounded above by Λaμa and Λhμh respectively, it implies that Λaμa≥Sa and Λhμh≥Sh. Therefore, G∗(Q,W)≥0,∀Q,W∈Ω. This conclude the proof that anthrax-free equilibrium is globally asymptotically stable when R0<1.
In this section, sensitivity analysis is performed to study the impact of individual parameters on the basic reproduction number (R0). Here, the Normalized Forward Sensitivity Index is used for this study. The normalized forward sensitivity index of model output y relative to parameter x is given by Υxy=∂y∂x×xy. Thus, the sensitivity indexes in Table 2 were evaluated using the base line parameter values in Table 1 with respect to the reproduction number expressed in the form ΥpiR0=∂R0∂pi×piR0, where pi are the parameters in R0.
R0 | |||||||
Par. | Λa | βa | ξa | μa | θ | ϕa | ϵ |
Indexes | 1.0000 | 1.0000 | 1.0000 | −1.0000 | −0.9996 | −0.6000 | −0.4000 |
Similarly, the sensitivity indexes of E∗ of the animal sub-population were computed and the results presented in Table 3. The impact of each parameter on both E∗ and R0 are presented in Table 4. The partial rank correction coefficient(PRCC) is presented in Figure 2 which further highlighted the contributions of each parameter value towards the overall reproduction number. The length of each bar suggests the statistical influence of each parameter on R0.
Sensitivity Indexes | ||||
Par. | S∗a | I∗a | R∗a | P∗ |
Λa | 1.0000 | 1.0000 | 1.0000 | 1.0000 |
βa | −1.0879e−05 | 1.000 | 1.0000 | 1.0000 |
μa | −1.0386 | −1.0385 | −1.0386 | −1.0385 |
θ | 1.0701e−05 | 0.0000 | 0.0000 | 0.0000 |
ϵ | 1.7835e−07 | −0.8000 | −0.8000 | −1.6000 |
ϕa | 0.0385 | −1.1615 | −01615 | −1.3615 |
πa | 1.4280e−04 | 0.0000 | −0.9999 | 0.0000 |
δa | 0.0467 | −1.0000 | −1.0000 | −1.0000 |
ξa | −1.0879e−05 | 1.0000 | 1.0000 | 2.0000 |
R0 | RankR0 | S∗a | RankS∗a | I∗a | RankI∗a | R∗a | RankR∗a | P∗ | RankP∗ |
μa | 1 | μa | 1 | ϕa | 1 | μa | 1 | ξa | 1 |
βa | 1 | Λa | 2 | μa | 2 | Λa | 1 | ϵ | 2 |
Λa | 1 | ϕa | 3 | Λa | 3 | βa | 2 | ϕa | 3 |
ξa | 1 | πa | 4 | βa | 3 | δa | 2 | μa | 4 |
ϕa | 2 | βa | 5 | δ | 3 | ξa | 2 | Λa | 5 |
θ | 3 | δa | 5 | ξa | 3 | π | 3 | βa | 5 |
ϵ | 4 | ξa | 5 | ϵ | 4 | ϵ | 4 | δa | 5 |
πa | 5 | θ | 6 | θ | 5 | ϕa | 5 | θ | 7 |
δa | 5 | ϵ | 6 | πa | 5 | θ | 6 | πa | 7 |
The numerical simulation of the model system (2.1) was performed using the fourth order Runge-Kutta scheme. The simulation shows the impact of parameters on the entire model as well as demonstrate the influence of parameters on individual state variables. The parameter values in Table 1 were used for running the simulations.
The following are the findings of the study:
(i) The anthrax-free equilibrium point is globally asymptotically stable anytime the basic reproduction number R0 is less than unity.
(ii) The most sensitive negative parameters on the reproduction number are the natural death rate and recovery rate of infected animals, whereas recruitment rate, pathogen shedding rate and the rate of infection of susceptible animals are the most positive sensitive parameters.
(iii) Increase in behavioural change within the human population causes an increase in the susceptible human population.
(iv) Increase in saturation effect causes an increase in the susceptible animal population.
(v) The saturation effect of the animal population and behavioural change within the human population has the tendency to reduce the number of infective population.
The contour plots in Figures 3 and 4 show the relationship between the reproduction number and two of its parameters (μa,βa), (ϕa,βa), (θ,βa), (μa,Λa), (ξa,βa) and (Λa,βa). Figure 3 suggests that βa increases R0 while μa,ϕa and θ reduce the R0. Figure 4 shows that βa, ξa and Λa increase the reproduction number. These results show that it is essential to reduce the infection force while increasing both environmental hygiene and recovery of infected population in other to control the spread of anthrax.
Figure 5 shows the impact of saturation effect of the animal population and behavioural change of humans towards the spread of anthrax on susceptible human population. Increase in saturation effect or behavioural change leads to an increase in the susceptible human population, in addition, increasing the saturation effect also causes an increase in the susceptible animal population as shown in Figure 6.
In Figure 7, increase in saturation effect of the animal population leads to a decrease in the infective populations of both animals and humans in a time period. It can be noticed in Figure 8 that behavioural change towards the spread of the disease and infected human population are inversely related. A decrease or negative behavioural change leads to an increase in the infective population whereas an increase, in other words, a positive behaviour towards the spread of the disease as infective population gets higher leads to a decrease in the infected population within a time period. Similarly, an increase in saturation factor trigger a reduction in the pathogen level within the environment in a long run as shown in Figure 10.
Figure 9 shows the impact of behavioural change and saturation effect on recovered humans and animals respectively. Implementing attitudinal change towards disease spread and saturation effect lead to a general decrease in recovered population, but increasing these rates further result in slightly increase in the recovered population and finally declines in a short run. The slightly increment may be as a result of many infective population recovering faster at that point in time due to stringent treatment measures resulting from the behavioural change.
It is noticed in Figure 10 and 11 that an increase in the force of infection leads to a corresponding increase in the level of pathogens in the environment as well as an increase in the number of infective population.
In this paper, we proposed an Ordinary differential equation (ODE) model to describe the transmission dynamics of anthrax disease in both animal and human populations taking into consideration the saturation effect in the animal population as infective population gets higher. The model also takes account of the behavioural changes of the general public as the human infective increases. The impact on the susceptible population as well as other state variables are studied.
Qualitative analyses revealed that the model system has two unique equilibrium points the anthrax-free and endemic equilibrium points. It is proofed that the anthrax-free equilibrium point is locally asymptotically stable if the reproduction number is less than unity (R0<1) and the endemic equilibrium point is locally asymptotically stable whenever R0>1. A study of global stability shows that the anthrax-free equilibrium point is globally asymptotically stable anytime R0<1.
In other to reduce the spread of anthrax, it is essential to reduce the levels of anthrax infection rate in animals and pathogen shedding rate while increasing the rate of environmental hygiene, recovery of infective animals and decay rate of pathogens.
The numerical simulations demonstrate the importance of saturation effect and behavioural change towards the outbreak of the disease. These parameters have the potential to control disease spread since an increase in their values increase the number of susceptible population, reduce the number of infective population and also reduce the pathogen level in the environment. The behavioural change of the general public influences the recovery rate of infective individuals, since health care personnel can change their attitude towards the disease and improve in quality health care in other to reduce anthrax related fatality.
The stability of anthrax epidemic within the animal-human system is strongly influenced by the spread of the disease within the animal population. It is worth noting that, controlling the spread of anthrax within the animal population will reduce the transmission of the disease in human population.
The numerical data used to support the findings of this research are included within the article.
The authors declare that there are no conflicts of interest regarding the publication of this research.
[1] |
J. K. Blackburn, K. M. Mcnyset, A. Curtis, M. E. Hugh-Jones, Modeling the geographic distribution of Bacillus anthracis, the causative agent of anthrax disease, for the contiguous United States using Predictive Ecologic Niche Modeling, The American Journal of Tropical Medicine and Hygiene, 77 (2007), 1103–1110. https://doi.org/10.4269/ajtmh.2007.77.1103 doi: 10.4269/ajtmh.2007.77.1103
![]() |
[2] | World Health Organisation, Emerging infectious diseases and zoonoses, WHO, India, 2014. |
[3] | B. Ahmed, Y. Sultana, D. S. M. Fatema, K. Ara, N. Begum, S. M. Mostanzid, et al., Anthrax : An emerging zoonotic disease in Bangladesh, Bangladesh Journal of Medical Microbiology, 4 (2010), 46–50. |
[4] | D. C. Dragon, R. P. Rennie, The ecology of anthrax spores: Tough but not invincible, The Canadian Veterinary Journal, 36 (1995), 295–301. |
[5] | J. Decker, Deadly diseases and epidemics - Anthrax. (E. I. Alcamo, Ed.). USA : Chelsea House Publishers, 2003. |
[6] | C. L. Stoltenow, Anthrax, NDSU Extension, USA, 2021. |
[7] | J. K. Awoonor-Williams, P. A. Apanga, M. Anyawie, T. Abachie, S. Boidoitsiah, J. L. Opare, et al., Anthrax outbreak investigation among humans and animals in Northern Ghana: Case Report, (2016). |
[8] | National Center for Emerging and Zoontic Infectious Diseases, Guide to understanding anthrax, Center for Disease Control and Prevention, USA, 2016. |
[9] |
S. V. Shadomy, T. L. Smith, Zoonosis update, anthrax, Journal of the American Veterinary Medical Association, 233 (2008), 63–72. https://doi.org/10.2460/javma.233.1.63 doi: 10.2460/javma.233.1.63
![]() |
[10] | A. P. Suma, K. P. Suresh, M. R. Gajendragad, B. A. Kavya, Forecasting anthrax in livestock in Karnataka State using remote sensing and climatic variables, International Journal of Science and Research, 6 (2017), 1891–1897. |
[11] | Food and Agriculture Organisation of the United Nations, Anthrax outbreaks: A warning for improved prevention, control and heightened awareness, Empres Watch, 2016. |
[12] |
R. Makurumidze, N. T. Gombe, T. Magure, M. Tshimanga, Investigation of an anthrax outbreak in Makoni District, Zimbabwe, BMC Public Health, 298 (2021), 1–10. https://doi.org/10.1186/s12889-021-10275-0 doi: 10.1186/s12889-021-10275-0
![]() |
[13] |
A. E. Frankel, S. R. Kuo, D. Dostal, L. Watson, N. S. Duesbery, C. P. Cheng, et al., Pathophysiology of anthrax, Frontiers in Bioscience-Landmark, 14 (2009), 4516–4524. https://doi.org/10.2741/3544 doi: 10.2741/3544
![]() |
[14] |
I. Kracalik, L. Malania, P. Imnadze, J. K. Blackburn, Human anthrax transmission at the urban rural interface, Georgia, The American Journal of Tropical Medicine and Hygiene, 93 (2015), 1156–1159. https://doi.org/10.4269/ajtmh.15-0242 doi: 10.4269/ajtmh.15-0242
![]() |
[15] |
A. Fasanella, R. Adone, M. Hugh-Jones, Classification and management of animal anthrax outbreaks based on the source of infection, Ann 1st Super Sanita, 50 (2014), 192–195. https://doi.org/10.4415/ANN_14_02_14 doi: 10.4415/ANN_14_02_14
![]() |
[16] | J. G. Wright, P. C. Quinn, S. Shadomy, N. Messonnier, Use of anthrax vaccine in the United States recommendations of the Advisory Committee on Immunization Practices(ACIP), MMWR Recomm Rep, 59 (2009). |
[17] | A. D. Sweeney, W. C. Hicks, X. Cui, Y. Li, Q. P. Eichacker, Anthrax infection, concise clinical review, American Journal of Respiratory and Critical Care Medicine, 184 (2011), 1333–1341. |
[18] | World Health Organisation, Anthrax in humans and animals (4th ed.). (P. Turnbull, Ed.). WHO Press, 2008. |
[19] | Fact Sheet, Bacillus anthracis (Anthrax), UPMC Center for Health Security, (2014), 1–4. Available from: www.UPMCHealth Security.org |
[20] |
H. W. Hethcote, The Mathematics of infectious diseases, SIAM Rev., 42 (2000), 599–653. https://doi.org/10.1137/S0036144500371907 doi: 10.1137/S0036144500371907
![]() |
[21] |
B. D. Hahn, P. R. Furniss, A deterministic model of an anthrax epizootic: Threshold Results, Ecol. Model., 20 (1983), 233–241. https://doi.org/10.1016/0304-3800(83)90009-1 doi: 10.1016/0304-3800(83)90009-1
![]() |
[22] |
S. Mushayabasa, Global stability of an anthrax model with environmental decontamination and time delay, Discrete Dynamics in Nature and Society, 2015 (2015). https://doi.org/10.1155/2015/573146 doi: 10.1155/2015/573146
![]() |
[23] |
A. Friedman, A. Yakubu, Anthrax epizootic and migration: Persistence or extinction, Math. Biosci, 241 (2012), 137–144. https://doi.org/10.1016/j.mbs.2012.10.004 doi: 10.1016/j.mbs.2012.10.004
![]() |
[24] | Z. M. Sinkie, N. S. Murthy, Modeling and simulation study of anthrax attack on environment, Journal of Multidisciplinary Engineering Science and Technology, 3 (2016), 4574–4578. |
[25] | S. Osman, O. D. Makinde, M. D. Theuri, Mathematical modelling of the transmission dynamics of anthrax in human and animal population, Mathematical Theory and Modeling, 8 (2018), 47–67. |
[26] | R. Kumar, C. C. Chow, J. D. Bartels, G. Clermont, A mathematical simulation of the inflammatory response to anthrax infection, Shock Society, 29 (2008), 104–111. |
[27] |
A. Kashkynbayev, F. A. Rihan, Dynamics of fractional-order epidemic models with general nonlinear incidence rate and time-delay, Mathematics, 9 (2021), 1–17. https://doi.org/10.3390/math9151829 doi: 10.3390/math9151829
![]() |
[28] | Y. Du, R. Xu, A delayed SIR epidemic model with nonlinear incidence rate and pulse vaccination, J. Appl. Math. Inform., 28 (2010), 1089–1099. |
[29] |
S. N. Chong, J. M. Tchuenche, R. J. Smith, A mathematical model of avian in uenza with half-saturated incidence, Theory in Biosciences, 133 (2014), 23–38. https://doi.org/10.1007/s12064-013-0183-6 doi: 10.1007/s12064-013-0183-6
![]() |
[30] |
M. Chinyoka, T. B. Gashirai, S. Mushayabasa, On the dynamics of a fractional-order ebola epidemic model with nonlinear incidence rates, Discrete Dynamics in Nature and Society, 2021 (2021). https://doi.org/10.1155/2021/2125061 doi: 10.1155/2021/2125061
![]() |
[31] |
L. Han, Z. Ma, H. W. Hethcote, Four predator prey models with infectious diseases, Math. Comput. Model., 34 (2001), 849–858. https://doi.org/10.1016/S0895-7177(01)00104-2 doi: 10.1016/S0895-7177(01)00104-2
![]() |
[32] | Z. Ma, J. Li, Dynamical modeling and analysis of epidemics, Singapore: World Scientic Publishing Co. Pte. Ltd., 2009. |
[33] |
V. Capasso, G. Serio, A generalization of the Kermack-Mackendrick deterministic epidemic models, Math. Biosci., 42 (1978), 43–61. https://doi.org/10.1016/0025-5564(78)90006-8 doi: 10.1016/0025-5564(78)90006-8
![]() |
[34] |
S. Liu, L. Pang, S. Ruan, X. Zhang, Global dynamics of avian influenza epidemic models with psychological effect, Computational and Mathematical Methods in Medicine, 2015 (2015), 913726. https://doi.org/10.1155/2015/913726 doi: 10.1155/2015/913726
![]() |
[35] |
E. B. Baloba, B. Seidu, C. S. Bornaa, Mathematical analysis of the effects of controls on the transmission dynamics of anthrax in both animal and human populations, Computational and Mathematical Methods in Medicine, 2020 (2020), 1581358. https://doi.org/10.1155/2020/1581358 doi: 10.1155/2020/1581358
![]() |
[36] | G. Birkhoff, G. Rota, Ordinary differential equations, John Wiley and Sons Inc., New York, 1989. |
[37] |
P. Van den Driessche, J. Watmough, Reproduction numbers and sub-threshold endemic equilibria for compartmental models of disease transmission, Math. Biosci., 180 (2002), 29–48. https://doi.org/10.1016/S0025-5564(02)00108-6 doi: 10.1016/S0025-5564(02)00108-6
![]() |
[38] |
C. Castillo-Chavez, B. Song, Dynamical models of tuberculosis and their applications, Math. Biosci. Eng., 1 (2004), 361–404. https://doi.org/10.3934/mbe.2004.1.361 doi: 10.3934/mbe.2004.1.361
![]() |
[39] | H. R. Thieme, Mathematics in Population Biology, Princeton University Press, 2003. |
[40] |
C. S. Bornaa, Y. I. Seini, B. Seidu, Modeling zoonotic diseases with treatment in both human and animal populations, Commun. Math. Biol. Neurosci., 2017 (2017). https://doi.org/10.28919/cmbn/3236 doi: 10.28919/cmbn/3236
![]() |
[41] |
J. K. K. Asamoah, Z. Jin, G. Sun, M. Y. Li, A Deterministic model for Q fever transmission dynamics within dairy cattle herds : Using Sensitivity Analysis and Optimal Controls, Computational and Mathematical Methods in Medicine, 2020 (2020), 6820608. https://doi.org/10.1155/2020/6820608 doi: 10.1155/2020/6820608
![]() |
[42] |
J. K. K. Asamoah, C. S. Bornaa, B. Seidu, Z. Jin, Mathematical analysis of the effects of controls on transmission dynamics of SARS-CoV-2, Alex. Eng. J., 59 (2020), 5069–5078. https://doi.org/10.1016/j.aej.2020.09.033 doi: 10.1016/j.aej.2020.09.033
![]() |
[43] |
C. S. Bornaa, B. Seidu, M. I. Daabo, Mathematical analysis of rabies infection, J. Appl. Math., 2020 (2020), 1804270. https://doi.org/10.1155/2020/1804270 doi: 10.1155/2020/1804270
![]() |
[44] |
B. Seidu, O. D. Makinde, I. Y. Seini, Mathematical analysis of the effects of HIV-Malaria co-infection on workplace productivity, Acta Biotheor., 63 (2015), 151–182. https://doi.org/10.1007/s10441-015-9255-y doi: 10.1007/s10441-015-9255-y
![]() |
[45] |
B. Seidu, . D. Makinde, I. Y. Seini, Mathematical analysis of an industrial HIV/AID model that incorporates carefree attitude towards sex, Acta Biother., 69 (2021), 257–276. https://doi.org/10.1007/s10441-020-09407-7 doi: 10.1007/s10441-020-09407-7
![]() |
[46] |
B. Seidu, C. S. Bornaa, O. D. Makinde, An ebola model with hyper-susceptibility, Chaos, Solitons & Fractals, 138 (2020), 109938. https://doi.org/10.1016/j.chaos.2020.109938 doi: 10.1016/j.chaos.2020.109938
![]() |
[47] |
S. Abagna, B. Seidu, C. S. Bornaa, A mathematical model of the transmission dynamics and control of bovine brucellosis in cattle, Abstr. Appl. Anal., 2022 (2022), 9658567. https://doi.org/10.1155/2022/9658567 doi: 10.1155/2022/9658567
![]() |
[48] | C. Castillo-Chavez, H. Wenzhang, On the computation R0 and its role on global stability, IMA J. Appl. Math., 125 (1992), 229–250. |
1. | Elijah B. Baloba, Baba Seidu, Christopher Saaha Bornaa, Eric Okyere, Optimal control and cost-effectiveness analysis of anthrax epidemic model, 2023, 42, 23529148, 101355, 10.1016/j.imu.2023.101355 | |
2. | Kazeem Babatunde Akande, Samuel Tosin Akinyemi, Nneka O. Iheonu, Alogla Monday Audu, Folashade Mistura Jimoh, Atede Anne Ojoma, Victoria Iyabode Okeowo, Abdulrahaman Lawal Suleiman, Kayode Oshinubi, A Risk-Structured Model for the Transmission Dynamics of Anthrax Disease, 2024, 12, 2227-7390, 1014, 10.3390/math12071014 | |
3. | Eric Abaa Baba Sandow, Baba Seidu, Stephen Abagna, A non-standard numerical scheme for an alcohol-abuse model with induced-complications, 2023, 9, 24058440, e22263, 10.1016/j.heliyon.2023.e22263 | |
4. | Anita Triska, Mona Zevika, A Study of the anthrax transmission model in herbivorous animals involving vaccination and harvesting, 2025, 26668181, 101095, 10.1016/j.padiff.2025.101095 |
Par. | Description | Value | Ref. |
βa | Infection rate of susceptible animals | 0.02 | [35] |
Λa | The rate of inflow of animals | 0.99 | [24] |
ϕa | The recovery rate of infected animals | 0.0025 | [25] |
μa | Natural death rate in animals | 0.0001 | [24] |
δa | The saturation effect of the animal population | 0.6 | Assumed |
πa | Rate at which recovered animals become susceptibles | 0.5 | [24] |
βh | Infection rate of susceptible humans | 0.0001 | [35] |
Λh | Recruitment rate of humans | 0.92 | [24] |
ϕh | The recovery rate of infected humans | 0.04 | [25] |
μh | Natural death rate in humans | 0.0001 | [24] |
ξa | Pathogen shedding rate | 0.45 | [25] |
δh | Rate of behavioural change | 0.6 | Assumed |
πh | Rate at which recovered humans become susceptible | 0.5 | Assumed |
ϵ | Rate of natural pathogens decay from the environment | 0.8 | [24] |
θ | Rate of decay due to environmental hygiene | 0.5 | [24] |
R0 | |||||||
Par. | Λa | βa | ξa | μa | θ | ϕa | ϵ |
Indexes | 1.0000 | 1.0000 | 1.0000 | −1.0000 | −0.9996 | −0.6000 | −0.4000 |
Sensitivity Indexes | ||||
Par. | S∗a | I∗a | R∗a | P∗ |
Λa | 1.0000 | 1.0000 | 1.0000 | 1.0000 |
βa | −1.0879e−05 | 1.000 | 1.0000 | 1.0000 |
μa | −1.0386 | −1.0385 | −1.0386 | −1.0385 |
θ | 1.0701e−05 | 0.0000 | 0.0000 | 0.0000 |
ϵ | 1.7835e−07 | −0.8000 | −0.8000 | −1.6000 |
ϕa | 0.0385 | −1.1615 | −01615 | −1.3615 |
πa | 1.4280e−04 | 0.0000 | −0.9999 | 0.0000 |
δa | 0.0467 | −1.0000 | −1.0000 | −1.0000 |
ξa | −1.0879e−05 | 1.0000 | 1.0000 | 2.0000 |
R0 | RankR0 | S∗a | RankS∗a | I∗a | RankI∗a | R∗a | RankR∗a | P∗ | RankP∗ |
μa | 1 | μa | 1 | ϕa | 1 | μa | 1 | ξa | 1 |
βa | 1 | Λa | 2 | μa | 2 | Λa | 1 | ϵ | 2 |
Λa | 1 | ϕa | 3 | Λa | 3 | βa | 2 | ϕa | 3 |
ξa | 1 | πa | 4 | βa | 3 | δa | 2 | μa | 4 |
ϕa | 2 | βa | 5 | δ | 3 | ξa | 2 | Λa | 5 |
θ | 3 | δa | 5 | ξa | 3 | π | 3 | βa | 5 |
ϵ | 4 | ξa | 5 | ϵ | 4 | ϵ | 4 | δa | 5 |
πa | 5 | θ | 6 | θ | 5 | ϕa | 5 | θ | 7 |
δa | 5 | ϵ | 6 | πa | 5 | θ | 6 | πa | 7 |
Par. | Description | Value | Ref. |
βa | Infection rate of susceptible animals | 0.02 | [35] |
Λa | The rate of inflow of animals | 0.99 | [24] |
ϕa | The recovery rate of infected animals | 0.0025 | [25] |
μa | Natural death rate in animals | 0.0001 | [24] |
δa | The saturation effect of the animal population | 0.6 | Assumed |
πa | Rate at which recovered animals become susceptibles | 0.5 | [24] |
βh | Infection rate of susceptible humans | 0.0001 | [35] |
Λh | Recruitment rate of humans | 0.92 | [24] |
ϕh | The recovery rate of infected humans | 0.04 | [25] |
μh | Natural death rate in humans | 0.0001 | [24] |
ξa | Pathogen shedding rate | 0.45 | [25] |
δh | Rate of behavioural change | 0.6 | Assumed |
πh | Rate at which recovered humans become susceptible | 0.5 | Assumed |
ϵ | Rate of natural pathogens decay from the environment | 0.8 | [24] |
θ | Rate of decay due to environmental hygiene | 0.5 | [24] |
R0 | |||||||
Par. | Λa | βa | ξa | μa | θ | ϕa | ϵ |
Indexes | 1.0000 | 1.0000 | 1.0000 | −1.0000 | −0.9996 | −0.6000 | −0.4000 |
Sensitivity Indexes | ||||
Par. | S∗a | I∗a | R∗a | P∗ |
Λa | 1.0000 | 1.0000 | 1.0000 | 1.0000 |
βa | −1.0879e−05 | 1.000 | 1.0000 | 1.0000 |
μa | −1.0386 | −1.0385 | −1.0386 | −1.0385 |
θ | 1.0701e−05 | 0.0000 | 0.0000 | 0.0000 |
ϵ | 1.7835e−07 | −0.8000 | −0.8000 | −1.6000 |
ϕa | 0.0385 | −1.1615 | −01615 | −1.3615 |
πa | 1.4280e−04 | 0.0000 | −0.9999 | 0.0000 |
δa | 0.0467 | −1.0000 | −1.0000 | −1.0000 |
ξa | −1.0879e−05 | 1.0000 | 1.0000 | 2.0000 |
R0 | RankR0 | S∗a | RankS∗a | I∗a | RankI∗a | R∗a | RankR∗a | P∗ | RankP∗ |
μa | 1 | μa | 1 | ϕa | 1 | μa | 1 | ξa | 1 |
βa | 1 | Λa | 2 | μa | 2 | Λa | 1 | ϵ | 2 |
Λa | 1 | ϕa | 3 | Λa | 3 | βa | 2 | ϕa | 3 |
ξa | 1 | πa | 4 | βa | 3 | δa | 2 | μa | 4 |
ϕa | 2 | βa | 5 | δ | 3 | ξa | 2 | Λa | 5 |
θ | 3 | δa | 5 | ξa | 3 | π | 3 | βa | 5 |
ϵ | 4 | ξa | 5 | ϵ | 4 | ϵ | 4 | δa | 5 |
πa | 5 | θ | 6 | θ | 5 | ϕa | 5 | θ | 7 |
δa | 5 | ϵ | 6 | πa | 5 | θ | 6 | πa | 7 |