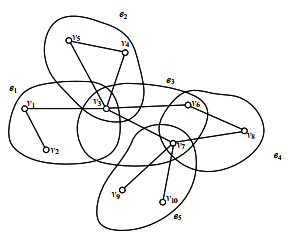
Random hyper-network is an important hyper-network structure. Studying the structure and properties of random hyper-networks, which helps researchers to understand the influence of the hyper-network structure on its properties. Currently, studies related to the influence of the internal structure of the hyper-edge on robustness have not been carried out for research on the robustness of hyper-networks. In this paper, we construct three k-uniform random hyper-networks with different structures inside hyper-edges. The nodes inside hyper-edges are connected in the ways randomly connected, preferentially connected and completely connected. Meanwhile, we propose a capacity-load model that can describe the relationship between the internal structure and the robustness of the hyper-edge, based on the idea of capacity-load model. The robustness of the three hyper-networks was obtained by simulation experiments. The results show the variation of the internal structure of hyper-edge has a large influence on the robustness of the k-uniform random hyper-network. In addition, the larger number of ordinary edges mk inside the hyper-edges and the size of the hyper-network k, the more robust the k-uniform random hyper-network is.
Citation: Bin Zhou, Xiujuan Ma, Fuxiang Ma, Shujie Gao. Robustness analysis of random hyper-networks based on the internal structure of hyper-edges[J]. AIMS Mathematics, 2023, 8(2): 4814-4829. doi: 10.3934/math.2023239
[1] | Shahbaz Ali, Muhammad Khalid Mahmmod, Raúl M. Falcón . A paradigmatic approach to investigate restricted hyper totient graphs. AIMS Mathematics, 2021, 6(4): 3761-3771. doi: 10.3934/math.2021223 |
[2] | Abdelaziz Alsubie, Anas Al-Masarwah . MBJ-neutrosophic hyper $ BCK $-ideals in hyper $ BCK $-algebras. AIMS Mathematics, 2021, 6(6): 6107-6121. doi: 10.3934/math.2021358 |
[3] | Mohammad Hamidi, Irina Cristea . Hyperideal-based zero-divisor graph of the general hyperring $ \mathbb{Z}_{n} $. AIMS Mathematics, 2024, 9(6): 15891-15910. doi: 10.3934/math.2024768 |
[4] | Qi Xiao, Jin Zhong . Characterizations and properties of hyper-dual Moore-Penrose generalized inverse. AIMS Mathematics, 2024, 9(12): 35125-35150. doi: 10.3934/math.20241670 |
[5] | Hashem Bordbar, Sanja Jančič-Rašovič, Irina Cristea . Regular local hyperrings and hyperdomains. AIMS Mathematics, 2022, 7(12): 20767-20780. doi: 10.3934/math.20221138 |
[6] | Yamin Sayyari, Mehdi Dehghanian, Choonkil Park, Jung Rye Lee . Stability of hyper homomorphisms and hyper derivations in complex Banach algebras. AIMS Mathematics, 2022, 7(6): 10700-10710. doi: 10.3934/math.2022597 |
[7] | Faik Babadağ, Ali Atasoy . On hyper-dual vectors and angles with Pell, Pell-Lucas numbers. AIMS Mathematics, 2024, 9(11): 30655-30666. doi: 10.3934/math.20241480 |
[8] | Jia Chen, Renato De Leone . A survival tree for interval-censored failure time data. AIMS Mathematics, 2022, 7(10): 18099-18126. doi: 10.3934/math.2022996 |
[9] | Narjes Alabkary . Hyper-instability of Banach algebras. AIMS Mathematics, 2024, 9(6): 14012-14025. doi: 10.3934/math.2024681 |
[10] | Fei Yu, Hifza Iqbal, Saira Munir, Jia Bao Liu . M-polynomial and topological indices of some transformed networks. AIMS Mathematics, 2021, 6(12): 13887-13906. doi: 10.3934/math.2021804 |
Random hyper-network is an important hyper-network structure. Studying the structure and properties of random hyper-networks, which helps researchers to understand the influence of the hyper-network structure on its properties. Currently, studies related to the influence of the internal structure of the hyper-edge on robustness have not been carried out for research on the robustness of hyper-networks. In this paper, we construct three k-uniform random hyper-networks with different structures inside hyper-edges. The nodes inside hyper-edges are connected in the ways randomly connected, preferentially connected and completely connected. Meanwhile, we propose a capacity-load model that can describe the relationship between the internal structure and the robustness of the hyper-edge, based on the idea of capacity-load model. The robustness of the three hyper-networks was obtained by simulation experiments. The results show the variation of the internal structure of hyper-edge has a large influence on the robustness of the k-uniform random hyper-network. In addition, the larger number of ordinary edges mk inside the hyper-edges and the size of the hyper-network k, the more robust the k-uniform random hyper-network is.
In recent years, hypergraph-based hyper-networks are superior in representing multivariate complex relationships, thus it is widely used to model many real complex systems [1,2,3,4,5,6,7,8,9]. Meanwhile, research on the robustness of hyper-networks has also made great progress. Ma studied the cascading failures of a hyper-network. They found that hyper-networks under attack exhibit similar properties to ordinary networks. And when faced with the same external perturbations, the hyper-network is more robust [10]. Ma proposed a hyper-network cascade failure model based on the structure of the hyper-network. They obtained similar conclusions through the simulation analysis of this model [11]. Chen proposed a capacity-load cascading failure model based on hyper-edge diffusion. The model is applied to random hyper-networks and small-world hyper-networks. The comparison reveals that random hyper-networks have stronger robustness than small-world hyper-networks [12]. Luo found hyper-networks are more suitable than ordinary networks to represent City Bus Network. The node represents bus stops and the hyper-edge represents routes. The authors also found the bus hyper-network is robust to random attacks and vulnerable to deliberate attacks [13]. Wang constructed a hyper-network model for High-speed Rail Logistics in China. They identified the important nodes and routes by analyzing the robustness of the High-speed Rail Logistics hyper-network [14]. Zhang analyzed the differences robustness under different methods of brain function hyper-network construction [15]. Pearcy proposed a bacterial metabolic hyper-network capable of describing general metabolic processes. The concept of percolation process is extended to bacterial metabolic hyper-networks. And the robustness of the bacterial metabolic hyper-network was synthesized [16]. However, in the existing studies on the robustness of hyper-networks, most researchers have ignored the influence of the internal structure of the hyper-edges on the robustness of the hyper-networks. Yet, when the internal substructure of a complex system changes, it can also cause changes in the system performance. For instance, in the QQ group hyper-network [6]. The nodes represent users, and the hyper-edges represent different QQ groups. If the users in the group do not know each other, it will slow down the spread of information. Conversely, it will enhance the spread of information. Similarly, changes in the internal structure of the hyper-edge also have an impact on the robustness of the hyper-network. Researchers can gain more comprehensive understanding of factors, influencing the robustness of hyper-networks. It can also provide a reference for proposing a strategy to improve the robustness of the hyper-networks. But, there are fewer studies on how the internal structure of the hyper-edge affects the robustness of the hyper-network.
Although most complex systems in reality are not always random. But, random networks still hold an important network structure of interest to researchers [17,18,19,20,21,22,23,24]. Studying the properties of random networks can provide a reference for the research of other network properties. This enables a clear understanding of impact different network structures on system performance. In previous studies, scholars compared structural properties of some networks with random networks [23,24], an objective and accurate evaluation of the performance of the network structure is made. We carried out a study on the influence of the internal structure of the hyper-edges on the robustness of k-uniform random hyper-networks.
In this paper, we construct three random hyper-networks with nodes connected in different ways inside the hyper-edges. We propose a new load assignment method based on the capacity-load model [25]. This model can better reflect the relationship between the hyper-edges and the nodes of the hyper-network. And we obtained the influence of different structures inside the hyper-edge on the robustness of the random hyper-network by simulation analysis.
Berge [26,27] first gave the concept of hypergraph, definition is as follows: If the binary relation H=(V,E) satisfies the condition: Ø=ei∈P(V),i∈{1,2,⋯,m};∪mi=1=V.
Where V={v1,v2,⋯,vn},E={e1,e2,⋯,em}, and P(V) denotes the power set of the set V; then H is a hypergraph. The elements in the set V are the nodes of the hypergraph. The hyper-degree of node vi is denoted by dH(vi) [28] is defined as the number of hyper-edges containing node vi. The node degree of node vi is similar to that of the ordinary network. It is still defined as the number of ordinary edges associated with node vi, denoted as the d(vi) [28]. The ordinary degree of a node vi within a separate hyper-edge ei is denoted by kei(vi). Figure 1 is a hypergraph containing 10 nodes and 5 hyper-edges. Take the example of node v3. The hyper-degree dH(v3) of v3 is 3. The node degree d(v3) is 5. The ordinary degree ke2(v3) inside the hyper-edge e2 is 2.
A hypergraph is said to be k-uniform hypergraph if the number of nodes in each hyper-edge is k. A hyper-network with k-uniform hypergraph as topology is a k-uniform hyper-network. In this paper, we study the influence of the internal structure of the hyper-edges on the robustness of k-uniform random hyper-networks. The procedure for the construction of the k-uniform random hyper-network is given below, as described in Algorithm 1.
Algorithm 1 Modeling the k-uniform random hyper-network. |
Input: Uniform variable, k; Initial number of isolated hyper-edges, M0; Number of nodes, N=M0×k; Hyper-edge connection probability, p∈[0,1]; |
Output: hyper-network-matrix |
1: do Time step T=0, hyper-network-matrix; |
2: for T≤(M02); |
3: do Choose two e1, e2 randomly among M0 isolated hyper-edges; |
4: do Generate a random numbers, s∈(0,1); |
5: if s≤p; |
6: do Randomly select k nodes from e1, e2 (select at least 1 node in each hyper-edge); |
7: do Connecting them into a new hyper-edge; |
8: end if |
9: do index ← the number of k nodes; |
10: for i in index; |
11: do hyper-network-matrix (M0+T,i)=1; |
12: do T=T+1; |
13: end for |
14: end for |
To better discover the influence of the internal structure of the hyper-edges in random hyper-networks on the robustness, we did further research. Based on the k-uniform random hyper-network, we construct three random hyper-network models with different connection methods of nodes inside the hyper-edges. Shown in Table 1.
Nodes connection method inside the hyper-edge | |||
Hyper-network type | Randomly connected | Preferentially connected | Completely connected |
ER-R hyper-network | ER-P hyper-network | ER-C hyper-network |
The construction process of the three hyper-network models is described in Algorithm 2.
Algorithm 2 Modeling three random hyper-networks |
Input: Uniform variable, k; Total hyper-edges, M; Total nodes, N; Node reconnection edges probability, p∈[0,1]; |
Output: inner-hyper-network-matrix |
1: do Disconnect the original connections between nodes inside the hyper-edges; |
2: switch (selecting the hyper-edge internal connection method); |
3: case1 Hyper-edge internal nodes are randomly connected; |
4: do Make k nodes inside the hyper-edge ei become isolated nodes; ordinary edge mk=0; inner-hyper-network-matrix |
5: for mk≤p×k(k−1)/2; |
6: do Generate a random number r; |
7: do Randomly select a pair of nodes vi,vj inside the same hyper-edge; |
8: if r<p |
9: if inner-hyper-network-matrix (vi,vj)!=1 |
10: do inner-hyper-network-matrix (vi,vj)=1 |
11: do inner-hyper-network-matrix (vj,vi)=1 |
12: end if |
13: end if |
14: end for |
15: end |
16: case2 Hyper-edge internal nodes are preferentially connected; |
17: do Make k nodes inside the hyper-edge ei become isolated nodes; time step t=0; inner-hyper-network-matrix |
18: do Select 3 nodes to be completely connected. Make them the initial connected branches inside the hyper-edges C0; |
19: do Γt (The set of nodes contained in the connected branch Ct at time t) |
20: for Γt≤k |
21: do t=t+1 |
22: do Randomly select a node vj outside Ct at time t+1, vj(vj∈Vei,vj∉Ct) |
23: do Ct+1=Ct+vj |
24: do Πi=kei(vi)∑|Γt|j=1kei(vj),vi,vj∈Ct |
25: do vj selects a node vi inside Ct with a preferential connection probability of Π(vi∈Vei,vi∈Ct) |
26: if inner-hyper-network-matrix (vi,vj)!=1 |
27: do inner-hyper-network-matrix (vi,vj)=1 |
28: do inner-hyper-network-matrix (vj,vi)=1 |
29: end if |
30: end for |
31: end |
32: case3 Hyper-edge internal nodes are completely connected; |
33: do Make k nodes inside the hyper-edge ei become isolated nodes; ordinary edge mk=0; inner-hyper-network-matrix |
34: do Each node is connected to k−1 nodes with edges |
35: if inner-hyper-network-matrix (vi,vj)!=1 |
36: do inner-hyper-network-matrix (vi,vj)=1 |
37: do inner-hyper-network-matrix (vj,vi)=1 |
38: do diag-inner-hyper-network-matrix=0; |
39: end if |
40: end |
41: end |
Previous researchers have proposed some hyper-network cascade failure models. But, there is the problem of considering too single metrics. As an example, Chen proposed a hyper-network cascading failure model based on hyper-edge diffusion [12]. The authors converted the hyper-networks into line graphs for research. They default hyper-edge internal nodes are completely connected. When a hyper-edge is attacked and fails. Failure hyper-edge's load is spread to its neighboring unfailed hyper-edges. But it does not consider the case where the load is spread through the nodes inside the hyper-edges. To better characterize the influence of the internal structure of the hyper-edge on the robustness of the hyper-network. We propose a new load distribution method based on the capacity-load model.
In a hyper-network with N nodes. The initial load of node vi is related to the hyper-degree dH(vi) and the node degree d(vi) of this node. The initial load Lvi(0) is defined as:
Lvi(0)=α(dH(vi)+d(vi))β,i=1,2,⋯,N,α≥1,β≥1. | (1) |
To control the initial load of node vi, let α is a load parameter and β is an adjustable parameter. From (1), it shows that the initial load of the node is proportional to its hyper-degree and node degree.
Let node vi and node vj connected by ordinary edges inside a certain hyper-edge. At time t, node vi failed. Firstly, the load Lvi of the failed node vi will be equally divided by the hyper-degree dH(vi) of vi. And assigns the equalized load to all the unfailed hyper-edges associated with the failed node. Then the load received by all hyper-edges associated with the failed node vi is Lvi/dH(vi). Then, the load received by the hyper-edge ei is equalized again according to the vi's node degree kei(vi) in the hyper-edge ei. The node vj receives the load as shown in (2).
ΔLvi→vj(t)=Lvi(t)×1dθH(vi)×1kθei(vi). | (2) |
The θ is the perturbation parameter, and 0≤θ≤1. From (2), a node vj inside the hyper-edge ei at time t receives a load ΔLvi→vj(t) that is related to three factors: The hyper-degree of the failed node vi at time t; The ordinary node degree of node vi inside the hyper-edge ei; and the initial load of node vi. If node vj has not failed at time t, its load is the sum of the load of node vj at time t−1 and the load redistributed from the failed node vi. That is:
Lvj(t)=Lvj(t−1)+ΔLvi→vj(t). | (3) |
The capacity is the largest amount of load can be handled by the node or hyper-edge. It is proportional to the initial load of the node. Let the capacity of node vj be Cvj, which is expressed by (4):
Cvj=(1+T)Lvj,T≥0. | (4) |
T is the capacity parameter. When the value of T is larger, the capacity of the node is larger. With a larger node capacity, the node is more resilient to failures. But the cost of resilience increases. The critical threshold TC is the smallest value of the capacity parameter to avoid a global crash of the hyper-network. When T>TC, there is no global crash in the whole hyper-network. When T<TC, a global crash occurs in the whole hyper-network. So, the critical threshold TC of T is an important measure of the robustness of the hyper-network. Obviously, the smaller TC the more robust hyper-network is.
If node vj fails after obtaining extra load, it will meet (5):
Lvj(t−1)+ΔLvi→vj(t)>Cvj. | (5) |
If (5) is true, then node vj will be overloaded and fail. When the load of vj is redistributed, it may cause other nodes to fail. Combining with (2), (5) can be expressed as:
Lvj(t−1)+Lvi(t)×1dθH(vi)×1kθei(vi)>Cvj. | (6) |
To measure the robustness of the hyper-network. We initially attack the node vi and defeat it, and then redistribute its load. For other nodes after load redistribution, if (6) is fulfilled, the node fails. When all nodes inside a hyper-edge failed, the failure of this hyper-edge occurs. After the number of failed nodes in the hyper-network attains steady state or all nodes failed, we count the number of failed hyper-edges FM(0≤FM≤M) in the hyper-network. The hyper-edge failure ratio fM is shown in (7):
fM=FMM,0≤fM≤1. | (7) |
M is the total number of hyper-edges in the hyper-network. From (7), it can be seen that the larger the fM, the higher the number of failed hyper-edges in the hyper-network. It means the less robustness of the hyper-network.
To analyze the influence of the node connection method inside the hyper-edge on the robustness of the k-uniform random hyper-network. We designed a simulation experiment as in Algorithm 3. The cascading failure process of k-uniform random hyper-network is simulated under both deliberate and random attack strategies. And record the relevant experimental data. Table 2 shows the experimental parameters in the simulation experiments. Each datum is averaged over 50 independent realizations.
Algorithm 3 Simulation steps |
1: do Construct a k-uniform random hyper-network with k of 20, 40, 60 |
2: do Construct ER-R, ER-P and ER-C hyper-networks |
3: for i=1:N |
4: do Set node vi initial capacity and load |
5: end for |
6: switch (Choose an attack strategy) |
7: case1: Random attack strategy: Randomly select a node |
8: case2: Deliberate attack strategy: Select a node with the largest sum of hyper-degree and node degree in the hyper-network |
9: end |
10: while Hyper-network not in stable state |
11: do Attacking a node disables it |
12: do The load of the failed node is distributed to the non-failed nodes adjacent to it |
13: if All nodes in a hyper-edge are invalid |
14: do This hyper-edge fails and counts the number of failed hyper-edges |
15: end if |
16: end |
17: do Calculate and output the hyper-edge failure ratio |
Parameter Name | Parameter Meaning | Value range |
N | Total number of hyper-network nodes | [80, 1600] |
M | Total number of hyper-edges | 50 |
α | Load parameters | 10 |
β | Adjustable parameters | 1 |
θ | Perturbation parameters | 1 |
L | Node Load | – |
d | Node-degree | – |
dH | Hyper-degree | – |
k | Uniform number | 20, 40, 60 |
kei | Node-degree of the node inside a hyper-edge ei | – |
C | Node Capacity | – |
T | Capacity Parameters | [0, 1] |
FM | Failure hyper-edge number | [0, M] |
fM | Hyper-edge failure ratio | [0, 1] |
TC | Critical Threshold | – |
In this section, we make three k-uniform random hypernetworks under attack at different k. Subsequently, we analyze the variation of hyper-edge failure ratio fM with capacity parameter T for three k-uniform random hypernetworks. The results show that: Under deliberate and random attacks, hyper-edge failure ratio tends to decrease with increasing value of capacity parameter. The hyper-network attains a critical point of global collapse under certain capacity parameters. When T≤TC, all three types of k-uniform random hyper-networks are in a state of global collapse. When T>TC, the failure size starts to decrease and eventually attains the state of global non-failure.
Figure 2 shows the variation of the hyper-edge failure ratio fM with the capacity parameter T, when the ER-R hyper-network is attacked with different sizes k. From Figures 2a and 2b it can be seen that the critical threshold TC of the ER-R hyper-network gradually reduces with the increase of the size k. Since the smaller the TC, the more robust the hyper-network is, i. e., the robustness of the ER-R hyper-network increases with the size k of the hyper-network.
The diagram cannot clearly distinguish the difference between the critical threshold TC of the ER-R hyper-network when it is subjected to random attacks and deliberate attacks. So, we give the ER-R hyper-network specific critical thresholds when it is under attack, shown in Table 3. From the data in Table 3, it can be seen that ER-R hyper-network's TC under deliberate attack is always smaller than TC under random attack. Again, the smaller TC is, the more robust the hyper-network is, which gives: The ER-R hyper-network is robust to deliberate attacks and vulnerable to random attacks.
Hyper-network type | Attack strategy | Critical threshold TC | ||
k=20 | k=40 | k=60 | ||
ER-R hyper-network | Random attack | 0.0668 | 0.03 | 0.02 |
Deliberate attack | 0.0652 | 0.0292 | 0.0192 |
It can be seen that the ER-R hyper-network exhibits the same robustness as the random network when subjected to external attacks. That is, ER-R hyper-network is robust to deliberate attacks and vulnerable to random attacks. Next, we analyze the reasons for this robustness from the perspective of the ER-R hyper-network structure.
The connection between each hyper-edge of the k-uniform random hyper-network is a random connection. The distribution of the hyper-degree of the k-uniform random hyper-network at k=60 is shown in Figure 3. When the connection between the nodes inside the hyper-edge is randomly connected, the node degree distribution and the hyper-degree distribution inside each hyper-edge are Poisson distributions. As shown in Figures 3 and 4. This connection enhances the random property of k-uniform random hyper-networks. Thus, k-uniform random hyper-networks exhibit properties consistent with random networks when attacked. That is, it is robust to deliberate attacks and vulnerable to random attacks.
Figure 5 shows the variation of the hyper-edge failure ratio fM with the capacity parameter T, when the ER-P hyper-network is attacked with different sizes k. From Figures 5(a) and 5(b), it can be seen that the robustness of the ER-P hyper-network increases with the increase of the hyper-network size k.
Next, we give the ER-P hyper-network specific critical thresholds when it is under attack, shown in Table 4. From the data in Table 4, it can be seen that ER-P hyper-network's TC under deliberate attack is always bigger than TC under random attack. That is, the ER-P hyper-network is robust to random attacks and vulnerable to deliberate attacks.
Hyper-network type | Attack strategy | Critical threshold TC | ||
k=20 | k=40 | k=60 | ||
ER-P hyper-network | Random attack | 0.114 | 0.068 | 0.0516 |
Deliberate attack | 0.1524 | 0.1464 | 0.13 |
It can be seen that the ER-P hyper-network exhibits the contrary robustness as the random network when subjected to external attacks. That is, ER-P hyper-network is robust to random attacks and vulnerable to deliberate attacks. Why does this kinetic phenomenon occur? Next, we analyze the reasons for this robustness from the perspective of the ER-P hyper-network structure. When the connection between nodes inside the hyper-edges is preferentially connected, the node degree distribution inside each hyper-edge is a power-law distribution. Since the hyper-degree distribution is still Poisson, it makes the entire node degree distribution of the ER-P hyper-network like a power-law distribution. As shown in Figure 6. This hyper-edge internal structure reduces the randomness of the k-uniform random hyper-network, so that ER-P hyper-network exhibits the consistent properties of a scale-free network when it is under attack. That is, it is robust to random attacks and vulnerable to deliberate attacks.
Figure 7 shows the variation of the hyper-edge failure ratio fM with the capacity parameter T, when the ER-C hyper-network is attacked with different sizes k. From Figures 7(a) and 7(b), it can be seen that the robustness of the ER-C hyper-network increases with the increase of the hyper-network size k.
Again, we give the ER-C hyper-network specific critical thresholds when it is under attack, shown in Table 5. From the data in Table 5, it can be seen that ER-C hyper-network's TC under deliberate attack is always bigger than TC under random attack. That is, the ER-C hyper-network is robust to random attacks and vulnerable to deliberate attacks.
Hyper-network type | Attack strategy | Critical threshold TC | ||
k=20 | k=40 | k=60 | ||
ER-C hyper-network | Random attack | 0.0392 | 0.0204 | 0.0138 |
Deliberate attack | 0.0472 | 0.0206 | 0.015 |
The ER-C hyper-network, like the ER-P hyper-network, both exhibit the opposite robustness to the random network when subjected to attacks. That is, the ER-C hyper-network is robust to random attacks and vulnerable to deliberate attacks. Here we analyze the reasons in detail.
When the nodes inside the hyper-edge are completely connected to each other, the inside of each hyper-edge is a regular network. Due to the load assignment method defined in this paper, there will be some nodes with large hyper-degree in the load assignment with overlapping load assignment. Because the random hyper-network is a uniform hyper-network, the difference in hyper-degree from node to node is small. Attacking the node whose hyper-degree is large, the neighboring nodes of the failed node will receive more load. When a neighboring node receives the load assigned by the failed node, it is more likely to crash. So, the ER-C hyper-network is robust to random attacks and vulnerable to deliberate attacks. The difference between the critical threshold TC of random and deliberate attacks is particularly small influenced by the hyper-network structure.
In addition, Chen studied random hyper-network that also used full connections within the hyper-edge [12]. However, it uses the method of converting the hyper-network into a line graph network to study. In other words, the effect of the internal structure of the hyperedges on the robustness of the hypernetwork is ignored. Moreover, Chen proposed a capacity-load model with equal load distribution that does not consider the effect brought by the structure. i.e., the effect of the hyper-edge inside and outside together on the hyper-network robustness. We reproduced the simulation of Chen and compared it with the method in this paper, as shown in Figure 8. To ensure the validity of the comparison, the two methods are compared at the same hyper-network size k=20. When the k-uniform random hyper-network under random attack, in Chen's method the critical threshold TC=0.075 and in this papers' method the critical threshold TC=0.039. When the k-uniform random hyper-network under deliberate attack, in Chen's method the critical threshold TC=0.07 and in this papers' method the critical threshold TC=0.047. Thus, it can be seen that the robustness of this paper's method is better than Chen's method under attack.
This shows that, it can be seen that the robustness of the three k-uniform random hyper-networks is closely related to the connection method of nodes inside the hyper-edges. When the nodes inside the hyper-edges are randomly connected, the k-uniform random hyper-network exhibits robustness consistent with that of a random network. When the nodes inside the hyper-edges are preferentially connected and completely connected, the k-uniform random hyper-network exhibits robustness in contrast to the random network.
Analyzing again the critical threshold TC for the three k-uniform random hyper-networks under attack reveals that: For the same hyper-network size k, the ER-C hyper-network has the best robustness, followed by the ER-R hyper-network, and the ER-P hyper-network has the worst robustness. Analysis of its causes: The robustness of k-uniform random hyper-networks under attack is related to the number of ordinary edges inside the hyper-edges. The size of the ordinary edges with different connection methods of nodes inside the hyper-edge is shown in Figure 9.
The innovations of this paper are mainly 2 points. First point is: Studying the robustness of k-uniform random hyper-networks from perspective of the internal structure of hyper-edges. Second point is: Based on the capacity-load model, we propose a capacity-load model that is more suitable for describing the load distribution inside and outside the hyper-edge. Applying this model to k-uniform random hyper-networks, its robustness under attack is obtained.
Based on the innovations of this paper, we have obtained conclusions as follows. When the connection between nodes inside a hyper-edge is same as the connection between hyper-edges, the k-uniform random hyper-network is robust to deliberate attacks and vulnerable to random attacks. When the connection between nodes inside a hyper-edge is not consistent with the connection between hyper-edges, the k-uniform random hyper-network is robust to random attacks and vulnerable to deliberate attacks. The ER-C hyper-network has the best robustness, followed by the ER-R hyper-network, and the ER-P hyper-network has the worst robustness. In other words, the larger number mk of ordinary edges inside the hyper-edge, the better robustness of the k-uniform random hyper-network. The larger size k of the k-uniform random hyper-network, the more robust it is. To summarize, the internal structure of hyper-edges has an important impact on the robustness of hyper-networks.
This work is supported by Natural Science Foundation of Qinghai Province in China (NO. 2019-ZJ-7012) and the National Natural Science Foundation of China (NOS. 11801296, 61603206).
All authors have no conflicts of interest to declare.
[1] |
G. J. Wang, Z. L. Ye, H. X. Zhao, Y. Zhu, L. Meng, Analysis of hyper-network characteristics in Tang poems and Song lyrics, J. Comput. Appl., 41 (2021), 2432–2439. https://doi.org/10.11772/j.issn.1001-9081.2020101569 doi: 10.11772/j.issn.1001-9081.2020101569
![]() |
[2] |
F. Hu, H. X. Zhao, J. B. He, F. X. Li, S. L. Li, Z. K. Zhang, An evolving model for hypergraph-structure-based scientific collaboration networks, Acta Phys. Sin., 62 (2013), 547–554. https://doi.org/10.7498/aps.62.198901 doi: 10.7498/aps.62.198901
![]() |
[3] |
F. Hu, M. Liu, J. Zhao, L. Lei, Analysisand application of the topological properties of protein complex hyper-networks, Complex Syst. Complexity Sci., 15 (2018), 31–38. https://doi.org/10.13306/j.1672-3813.2018.04.005 doi: 10.13306/j.1672-3813.2018.04.005
![]() |
[4] | T. Ma, J. L. Guo, Industry-university-research cooperative hyper-network model for applying patent based on weighted hypergraph: Case of electronic information industry from Shanghai, J. Technol. Econom., 38 (2019), 109. |
[5] | T. Ma, J. L. Guo, Industry-university-research cooperative hyper-network for applying patent based on weighted hypergraph: A case of ICT industry from Shanghai, Syst. Eng., 36 (2018), 140–152. |
[6] |
M. Liu, F. Hu, Analysis of characteristics of QQ group hyper-network, Appl. Res. Comput., 35 (2018), 3259–3262. https://doi.org/10.3969/j.issn.1001-3695.2018.11.014 doi: 10.3969/j.issn.1001-3695.2018.11.014
![]() |
[7] |
Z. P. Wang, J. Wang, Dynamic model of public opinion evolution based on hyper-network, Complex Syst. Complexity Sci., 18 (2021), 29–38. https://doi.org/10.13306/j.1672-3813.2021.02.004 doi: 10.13306/j.1672-3813.2021.02.004
![]() |
[8] |
W. Wang, S. F. Liu, B. Li, A Hyper-network based model for emergency response system, Chin. J. Electron., 31 (2022), 129–136. https://doi.org/10.1049/cje.2020.00.335 doi: 10.1049/cje.2020.00.335
![]() |
[9] |
Q. Suo, J. L. Guo, The evolutionary mechanism of high-speed railway system based on hyper-network theory, Int. J. Mod. Phys. B, 32 (2018), 1850182. https://doi.org/10.1142/S0217979218501825 doi: 10.1142/S0217979218501825
![]() |
[10] |
X. J. Ma, H. X. Zhao, F. Hu, Cascading failure analysis in hyper-network based on the hypergraph, Acta Phys. Sin., 65 (2016), 374–383. https://doi.org/10.7498/aps.65.088901 doi: 10.7498/aps.65.088901
![]() |
[11] |
X. J. Ma, F. X. Ma, J. Yin, H. X. Zhao, Cascading failures of k uniform hyper-network based on the hyper adjacent matrix, Physica A, 510 (2018), 281–289. https://doi.org/10.1016/j.physa.2018.06.122 doi: 10.1016/j.physa.2018.06.122
![]() |
[12] |
Y. Chen, X. J. Ma, F. X. Ma, Q. Liu, W. X. Cheng, The capacity load model of K-Uniform hyper-network based on equal load distribution, J. Phys. Conf. Ser., 1828 (2021), 012060. https://doi.org/10.1088/1742-6596/1828/1/012060 doi: 10.1088/1742-6596/1828/1/012060
![]() |
[13] |
H. X. Luo, H. X. Zhao, Y. Z. Xiao, Z. L. Ye, H. Y. Ma, F. X. Li, A hypergraph-based analysis of the topology and robustness of bus hyper-networks, J. Southwest Univ., 43 (2021), 181–191. https://doi.org/10.13718/j.cnki.xdzk.2021.10.022 doi: 10.13718/j.cnki.xdzk.2021.10.022
![]() |
[14] | F. H. Wang, N. Wan, L. Wang, J. L. Guo, Study on location and robustness of freight high-railway hyper-network, J. Tech. Econ. Manage., 10 (2017), 17–23. |
[15] |
C. R. Zhang, J. J. Chen, H. Guo, Comparative analysis of robustness of resting human brain functional hyper-network model, Comput. Sci., 49 (2022), 241–247. https://doi.org/10.11896/jsjkx.201200067 doi: 10.11896/jsjkx.201200067
![]() |
[16] |
N. Pearcy, N. Chuzhanova, J. J. Crofts, Complexity and robustness in hyper-network models of metabolism, J. Theor. Biol., 406 (2016), 99–104. https://doi.org/10.1016/j.jtbi.2016.06.032 doi: 10.1016/j.jtbi.2016.06.032
![]() |
[17] | P. Erdős, A. Rényi, On the evolution of random graphs, Publ. Math. Inst. Hung. Acad. Sci., 5 (1960). |
[18] |
X. P. Xu, F. Liu, Continuous-time quantum walks on Erdös-Rényi networks, Phys. Lett. A, 372 (2008), 6727–6732. https://doi.org/10.1016/j.physleta.2008.09.042 doi: 10.1016/j.physleta.2008.09.042
![]() |
[19] |
X. F. Xue, Law of large numbers for the SIR model with random vertex weights on Erdős-Rényi graph, Physica A, 486 (2017), 434–445. https://doi.org/10.1016/j.physa.2017.04.096 doi: 10.1016/j.physa.2017.04.096
![]() |
[20] |
F. W. S. Lima, A. O. Sousa, M. A. Sumuor, Majority-vote on directed Erdős-Rényi random graphs, Physica A, 387 (2008), 3503–3510. https://doi.org/10.1016/j.physa.2008.01.120 doi: 10.1016/j.physa.2008.01.120
![]() |
[21] |
A. N. Zehmakan, Opinion forming in Erdős-Rényi random graph and expanders, Discrete. Appl. Math., 277 (2020), 280–290. https://doi.org/10.4230/LIPIcs.ISAAC.2018.168 doi: 10.4230/LIPIcs.ISAAC.2018.168
![]() |
[22] |
Y. Li, G. Tang, L. J. Song, Z. P. Xun, H. Xia, D. P. Hao, Numerical simulations of the phase transition property of the explosive percolation model on Erdős-Rényi random network, Acta Phys. Sin., 62 (2013), 398–406. https://doi.org/10.7498/aps.62.046401 doi: 10.7498/aps.62.046401
![]() |
[23] |
Y. L. Shang, Percolation on random networks with proliferation, Int. J. Mod. Phys. B, 32 (2018), 1850359. https://doi.org/10.1142/S0217979218503599 doi: 10.1142/S0217979218503599
![]() |
[24] |
P. L. Juhász, Information propagation in stochastic networks, Physica A, 577 (2021), 126070. https://doi.org/10.1016/J.PHYSA.2021.126070 doi: 10.1016/J.PHYSA.2021.126070
![]() |
[25] |
A. E. Motter, L. Y. Cheng, Cascade-based attacks on complex networks, Phys. Rev. E, 66 (2002), 065102. https://doi.org/10.1103/physreve.66.065102 doi: 10.1103/physreve.66.065102
![]() |
[26] | C. Berge, E. Minieka, Graphs and hpergraphs, North Holland: North-Holland Publishing Company Amster-dams, 1973. |
[27] |
C. Berge, F. Sterboul, Equipartite colorings in graphs and hypergraphs, J. Comb. Theory, Ser. B, 22 (1977), 97–113. https://doi.org/10.1016/0095-8956(77)90002-8 doi: 10.1016/0095-8956(77)90002-8
![]() |
[28] | A. Bretto, Hypergraph theory: An introduction, Berlin: Springer Science Business Media, 2013. |
1. | Shuaijie Li, Chaojie Zhang, Chengli Zhao, Chengyi Xia, Robustness of LEO satellite hypernetworks under different network topologies and attack strategies, 2024, 526, 03759601, 129952, 10.1016/j.physleta.2024.129952 | |
2. | Juan Du, Xiujuan Ma, Fuxiang Ma, Bin Zhou, Wenqian Yu, 2023, Chapter 25, 978-981-99-5970-9, 344, 10.1007/978-981-99-5971-6_25 | |
3. | Juan Du, Xiujuan Ma, Fuxiang Ma, Wenqian Yu, Synchronization analyze of k-uniform hyper-networks, 2024, 14, 2045-2322, 10.1038/s41598-024-56198-9 |
Algorithm 1 Modeling the k-uniform random hyper-network. |
Input: Uniform variable, k; Initial number of isolated hyper-edges, M0; Number of nodes, N=M0×k; Hyper-edge connection probability, p∈[0,1]; |
Output: hyper-network-matrix |
1: do Time step T=0, hyper-network-matrix; |
2: for T≤(M02); |
3: do Choose two e1, e2 randomly among M0 isolated hyper-edges; |
4: do Generate a random numbers, s∈(0,1); |
5: if s≤p; |
6: do Randomly select k nodes from e1, e2 (select at least 1 node in each hyper-edge); |
7: do Connecting them into a new hyper-edge; |
8: end if |
9: do index ← the number of k nodes; |
10: for i in index; |
11: do hyper-network-matrix (M0+T,i)=1; |
12: do T=T+1; |
13: end for |
14: end for |
Nodes connection method inside the hyper-edge | |||
Hyper-network type | Randomly connected | Preferentially connected | Completely connected |
ER-R hyper-network | ER-P hyper-network | ER-C hyper-network |
Algorithm 2 Modeling three random hyper-networks |
Input: Uniform variable, k; Total hyper-edges, M; Total nodes, N; Node reconnection edges probability, p∈[0,1]; |
Output: inner-hyper-network-matrix |
1: do Disconnect the original connections between nodes inside the hyper-edges; |
2: switch (selecting the hyper-edge internal connection method); |
3: case1 Hyper-edge internal nodes are randomly connected; |
4: do Make k nodes inside the hyper-edge ei become isolated nodes; ordinary edge mk=0; inner-hyper-network-matrix |
5: for mk≤p×k(k−1)/2; |
6: do Generate a random number r; |
7: do Randomly select a pair of nodes vi,vj inside the same hyper-edge; |
8: if r<p |
9: if inner-hyper-network-matrix (vi,vj)!=1 |
10: do inner-hyper-network-matrix (vi,vj)=1 |
11: do inner-hyper-network-matrix (vj,vi)=1 |
12: end if |
13: end if |
14: end for |
15: end |
16: case2 Hyper-edge internal nodes are preferentially connected; |
17: do Make k nodes inside the hyper-edge ei become isolated nodes; time step t=0; inner-hyper-network-matrix |
18: do Select 3 nodes to be completely connected. Make them the initial connected branches inside the hyper-edges C0; |
19: do Γt (The set of nodes contained in the connected branch Ct at time t) |
20: for Γt≤k |
21: do t=t+1 |
22: do Randomly select a node vj outside Ct at time t+1, vj(vj∈Vei,vj∉Ct) |
23: do Ct+1=Ct+vj |
24: do Πi=kei(vi)∑|Γt|j=1kei(vj),vi,vj∈Ct |
25: do vj selects a node vi inside Ct with a preferential connection probability of Π(vi∈Vei,vi∈Ct) |
26: if inner-hyper-network-matrix (vi,vj)!=1 |
27: do inner-hyper-network-matrix (vi,vj)=1 |
28: do inner-hyper-network-matrix (vj,vi)=1 |
29: end if |
30: end for |
31: end |
32: case3 Hyper-edge internal nodes are completely connected; |
33: do Make k nodes inside the hyper-edge ei become isolated nodes; ordinary edge mk=0; inner-hyper-network-matrix |
34: do Each node is connected to k−1 nodes with edges |
35: if inner-hyper-network-matrix (vi,vj)!=1 |
36: do inner-hyper-network-matrix (vi,vj)=1 |
37: do inner-hyper-network-matrix (vj,vi)=1 |
38: do diag-inner-hyper-network-matrix=0; |
39: end if |
40: end |
41: end |
Algorithm 3 Simulation steps |
1: do Construct a k-uniform random hyper-network with k of 20, 40, 60 |
2: do Construct ER-R, ER-P and ER-C hyper-networks |
3: for i=1:N |
4: do Set node vi initial capacity and load |
5: end for |
6: switch (Choose an attack strategy) |
7: case1: Random attack strategy: Randomly select a node |
8: case2: Deliberate attack strategy: Select a node with the largest sum of hyper-degree and node degree in the hyper-network |
9: end |
10: while Hyper-network not in stable state |
11: do Attacking a node disables it |
12: do The load of the failed node is distributed to the non-failed nodes adjacent to it |
13: if All nodes in a hyper-edge are invalid |
14: do This hyper-edge fails and counts the number of failed hyper-edges |
15: end if |
16: end |
17: do Calculate and output the hyper-edge failure ratio |
Parameter Name | Parameter Meaning | Value range |
N | Total number of hyper-network nodes | [80, 1600] |
M | Total number of hyper-edges | 50 |
α | Load parameters | 10 |
β | Adjustable parameters | 1 |
θ | Perturbation parameters | 1 |
L | Node Load | – |
d | Node-degree | – |
dH | Hyper-degree | – |
k | Uniform number | 20, 40, 60 |
kei | Node-degree of the node inside a hyper-edge ei | – |
C | Node Capacity | – |
T | Capacity Parameters | [0, 1] |
FM | Failure hyper-edge number | [0, M] |
fM | Hyper-edge failure ratio | [0, 1] |
TC | Critical Threshold | – |
Hyper-network type | Attack strategy | Critical threshold TC | ||
k=20 | k=40 | k=60 | ||
ER-R hyper-network | Random attack | 0.0668 | 0.03 | 0.02 |
Deliberate attack | 0.0652 | 0.0292 | 0.0192 |
Hyper-network type | Attack strategy | Critical threshold TC | ||
k=20 | k=40 | k=60 | ||
ER-P hyper-network | Random attack | 0.114 | 0.068 | 0.0516 |
Deliberate attack | 0.1524 | 0.1464 | 0.13 |
Hyper-network type | Attack strategy | Critical threshold TC | ||
k=20 | k=40 | k=60 | ||
ER-C hyper-network | Random attack | 0.0392 | 0.0204 | 0.0138 |
Deliberate attack | 0.0472 | 0.0206 | 0.015 |
Algorithm 1 Modeling the k-uniform random hyper-network. |
Input: Uniform variable, k; Initial number of isolated hyper-edges, M0; Number of nodes, N=M0×k; Hyper-edge connection probability, p∈[0,1]; |
Output: hyper-network-matrix |
1: do Time step T=0, hyper-network-matrix; |
2: for T≤(M02); |
3: do Choose two e1, e2 randomly among M0 isolated hyper-edges; |
4: do Generate a random numbers, s∈(0,1); |
5: if s≤p; |
6: do Randomly select k nodes from e1, e2 (select at least 1 node in each hyper-edge); |
7: do Connecting them into a new hyper-edge; |
8: end if |
9: do index ← the number of k nodes; |
10: for i in index; |
11: do hyper-network-matrix (M0+T,i)=1; |
12: do T=T+1; |
13: end for |
14: end for |
Nodes connection method inside the hyper-edge | |||
Hyper-network type | Randomly connected | Preferentially connected | Completely connected |
ER-R hyper-network | ER-P hyper-network | ER-C hyper-network |
Algorithm 2 Modeling three random hyper-networks |
Input: Uniform variable, k; Total hyper-edges, M; Total nodes, N; Node reconnection edges probability, p∈[0,1]; |
Output: inner-hyper-network-matrix |
1: do Disconnect the original connections between nodes inside the hyper-edges; |
2: switch (selecting the hyper-edge internal connection method); |
3: case1 Hyper-edge internal nodes are randomly connected; |
4: do Make k nodes inside the hyper-edge ei become isolated nodes; ordinary edge mk=0; inner-hyper-network-matrix |
5: for mk≤p×k(k−1)/2; |
6: do Generate a random number r; |
7: do Randomly select a pair of nodes vi,vj inside the same hyper-edge; |
8: if r<p |
9: if inner-hyper-network-matrix (vi,vj)!=1 |
10: do inner-hyper-network-matrix (vi,vj)=1 |
11: do inner-hyper-network-matrix (vj,vi)=1 |
12: end if |
13: end if |
14: end for |
15: end |
16: case2 Hyper-edge internal nodes are preferentially connected; |
17: do Make k nodes inside the hyper-edge ei become isolated nodes; time step t=0; inner-hyper-network-matrix |
18: do Select 3 nodes to be completely connected. Make them the initial connected branches inside the hyper-edges C0; |
19: do Γt (The set of nodes contained in the connected branch Ct at time t) |
20: for Γt≤k |
21: do t=t+1 |
22: do Randomly select a node vj outside Ct at time t+1, vj(vj∈Vei,vj∉Ct) |
23: do Ct+1=Ct+vj |
24: do Πi=kei(vi)∑|Γt|j=1kei(vj),vi,vj∈Ct |
25: do vj selects a node vi inside Ct with a preferential connection probability of Π(vi∈Vei,vi∈Ct) |
26: if inner-hyper-network-matrix (vi,vj)!=1 |
27: do inner-hyper-network-matrix (vi,vj)=1 |
28: do inner-hyper-network-matrix (vj,vi)=1 |
29: end if |
30: end for |
31: end |
32: case3 Hyper-edge internal nodes are completely connected; |
33: do Make k nodes inside the hyper-edge ei become isolated nodes; ordinary edge mk=0; inner-hyper-network-matrix |
34: do Each node is connected to k−1 nodes with edges |
35: if inner-hyper-network-matrix (vi,vj)!=1 |
36: do inner-hyper-network-matrix (vi,vj)=1 |
37: do inner-hyper-network-matrix (vj,vi)=1 |
38: do diag-inner-hyper-network-matrix=0; |
39: end if |
40: end |
41: end |
Algorithm 3 Simulation steps |
1: do Construct a k-uniform random hyper-network with k of 20, 40, 60 |
2: do Construct ER-R, ER-P and ER-C hyper-networks |
3: for i=1:N |
4: do Set node vi initial capacity and load |
5: end for |
6: switch (Choose an attack strategy) |
7: case1: Random attack strategy: Randomly select a node |
8: case2: Deliberate attack strategy: Select a node with the largest sum of hyper-degree and node degree in the hyper-network |
9: end |
10: while Hyper-network not in stable state |
11: do Attacking a node disables it |
12: do The load of the failed node is distributed to the non-failed nodes adjacent to it |
13: if All nodes in a hyper-edge are invalid |
14: do This hyper-edge fails and counts the number of failed hyper-edges |
15: end if |
16: end |
17: do Calculate and output the hyper-edge failure ratio |
Parameter Name | Parameter Meaning | Value range |
N | Total number of hyper-network nodes | [80, 1600] |
M | Total number of hyper-edges | 50 |
α | Load parameters | 10 |
β | Adjustable parameters | 1 |
θ | Perturbation parameters | 1 |
L | Node Load | – |
d | Node-degree | – |
dH | Hyper-degree | – |
k | Uniform number | 20, 40, 60 |
kei | Node-degree of the node inside a hyper-edge ei | – |
C | Node Capacity | – |
T | Capacity Parameters | [0, 1] |
FM | Failure hyper-edge number | [0, M] |
fM | Hyper-edge failure ratio | [0, 1] |
TC | Critical Threshold | – |
Hyper-network type | Attack strategy | Critical threshold TC | ||
k=20 | k=40 | k=60 | ||
ER-R hyper-network | Random attack | 0.0668 | 0.03 | 0.02 |
Deliberate attack | 0.0652 | 0.0292 | 0.0192 |
Hyper-network type | Attack strategy | Critical threshold TC | ||
k=20 | k=40 | k=60 | ||
ER-P hyper-network | Random attack | 0.114 | 0.068 | 0.0516 |
Deliberate attack | 0.1524 | 0.1464 | 0.13 |
Hyper-network type | Attack strategy | Critical threshold TC | ||
k=20 | k=40 | k=60 | ||
ER-C hyper-network | Random attack | 0.0392 | 0.0204 | 0.0138 |
Deliberate attack | 0.0472 | 0.0206 | 0.015 |