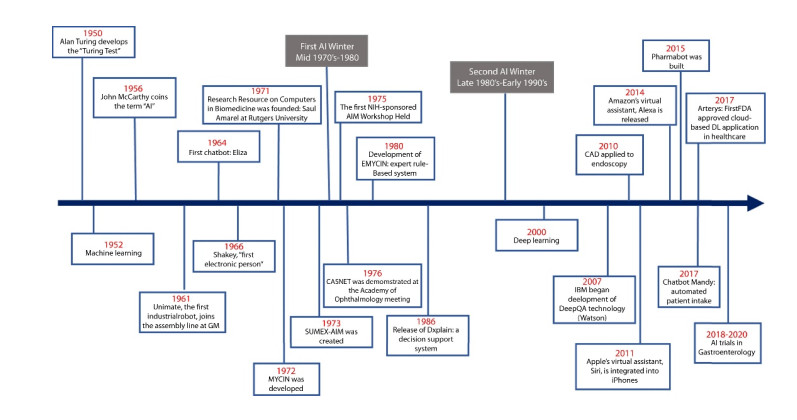
Cerebrovascular disease refers to damage to brain tissue caused by impaired intracranial blood circulation. It usually presents clinically as an acute nonfatal event and is characterized by high morbidity, disability, and mortality. Transcranial Doppler (TCD) ultrasonography is a non-invasive method for the diagnosis of cerebrovascular disease that uses the Doppler effect to detect the hemodynamic and physiological parameters of the major intracranial basilar arteries. It can provide important hemodynamic information that cannot be measured by other diagnostic imaging techniques for cerebrovascular disease. And the result parameters of TCD ultrasonography such as blood flow velocity and beat index can reflect the type of cerebrovascular disease and serve as a basis to assist physicians in the treatment of cerebrovascular diseases. Artificial intelligence (AI) is a branch of computer science which is used in a wide range of applications in agriculture, communications, medicine, finance, and other fields. In recent years, there are much research devoted to the application of AI to TCD. The review and summary of related technologies is an important work to promote the development of this field, which can provide an intuitive technical summary for future researchers. In this paper, we first review the development, principles, and applications of TCD ultrasonography and other related knowledge, and briefly introduce the development of AI in the field of medicine and emergency medicine. Finally, we summarize in detail the applications and advantages of AI technology in TCD ultrasonography including the establishment of an examination system combining brain computer interface (BCI) and TCD ultrasonography, the classification and noise cancellation of TCD ultrasonography signals using AI algorithms, and the use of intelligent robots to assist physicians in TCD ultrasonography and discuss the prospects for the development of AI in TCD ultrasonography.
Citation: Lingli Gan, Xiaoling Yin, Jiating Huang, Bin Jia. Transcranial Doppler analysis based on computer and artificial intelligence for acute cerebrovascular disease[J]. Mathematical Biosciences and Engineering, 2023, 20(2): 1695-1715. doi: 10.3934/mbe.2023077
[1] | Ivan Izonin, Nataliya Shakhovska . Special issue: Informatics & data-driven medicine. Mathematical Biosciences and Engineering, 2021, 18(5): 6430-6433. doi: 10.3934/mbe.2021319 |
[2] | Ivan Izonin, Nataliya Shakhovska . Special issue: informatics & data-driven medicine-2021. Mathematical Biosciences and Engineering, 2022, 19(10): 9769-9772. doi: 10.3934/mbe.2022454 |
[3] | Jingyao Liu, Qinghe Feng, Yu Miao, Wei He, Weili Shi, Zhengang Jiang . COVID-19 disease identification network based on weakly supervised feature selection. Mathematical Biosciences and Engineering, 2023, 20(5): 9327-9348. doi: 10.3934/mbe.2023409 |
[4] | Yanling An, Shaohai Hu, Shuaiqi Liu, Bing Li . BiTCAN: An emotion recognition network based on saliency in brain cognition. Mathematical Biosciences and Engineering, 2023, 20(12): 21537-21562. doi: 10.3934/mbe.2023953 |
[5] | Delong Cui, Hong Huang, Zhiping Peng, Qirui Li, Jieguang He, Jinbo Qiu, Xinlong Luo, Jiangtao Ou, Chengyuan Fan . Next-generation 5G fusion-based intelligent health-monitoring platform for ethylene cracking furnace tube. Mathematical Biosciences and Engineering, 2022, 19(9): 9168-9199. doi: 10.3934/mbe.2022426 |
[6] | Hongyan Xu . Digital media zero watermark copyright protection algorithm based on embedded intelligent edge computing detection. Mathematical Biosciences and Engineering, 2021, 18(5): 6771-6789. doi: 10.3934/mbe.2021336 |
[7] | Bo An . Construction and application of Chinese breast cancer knowledge graph based on multi-source heterogeneous data. Mathematical Biosciences and Engineering, 2023, 20(4): 6776-6799. doi: 10.3934/mbe.2023292 |
[8] | Juanjuan Tian, Li Li . Research on artificial intelligence of accounting information processing based on image processing. Mathematical Biosciences and Engineering, 2022, 19(8): 8411-8425. doi: 10.3934/mbe.2022391 |
[9] | Javad Hassannataj Joloudari, Faezeh Azizi, Issa Nodehi, Mohammad Ali Nematollahi, Fateme Kamrannejhad, Edris Hassannatajjeloudari, Roohallah Alizadehsani, Sheikh Mohammed Shariful Islam . Developing a Deep Neural Network model for COVID-19 diagnosis based on CT scan images. Mathematical Biosciences and Engineering, 2023, 20(9): 16236-16258. doi: 10.3934/mbe.2023725 |
[10] | Shahab Shamshirband, Javad Hassannataj Joloudari, Sahar Khanjani Shirkharkolaie, Sanaz Mojrian, Fatemeh Rahmani, Seyedakbar Mostafavi, Zulkefli Mansor . Game theory and evolutionary optimization approaches applied to resource allocation problems in computing environments: A survey. Mathematical Biosciences and Engineering, 2021, 18(6): 9190-9232. doi: 10.3934/mbe.2021453 |
Cerebrovascular disease refers to damage to brain tissue caused by impaired intracranial blood circulation. It usually presents clinically as an acute nonfatal event and is characterized by high morbidity, disability, and mortality. Transcranial Doppler (TCD) ultrasonography is a non-invasive method for the diagnosis of cerebrovascular disease that uses the Doppler effect to detect the hemodynamic and physiological parameters of the major intracranial basilar arteries. It can provide important hemodynamic information that cannot be measured by other diagnostic imaging techniques for cerebrovascular disease. And the result parameters of TCD ultrasonography such as blood flow velocity and beat index can reflect the type of cerebrovascular disease and serve as a basis to assist physicians in the treatment of cerebrovascular diseases. Artificial intelligence (AI) is a branch of computer science which is used in a wide range of applications in agriculture, communications, medicine, finance, and other fields. In recent years, there are much research devoted to the application of AI to TCD. The review and summary of related technologies is an important work to promote the development of this field, which can provide an intuitive technical summary for future researchers. In this paper, we first review the development, principles, and applications of TCD ultrasonography and other related knowledge, and briefly introduce the development of AI in the field of medicine and emergency medicine. Finally, we summarize in detail the applications and advantages of AI technology in TCD ultrasonography including the establishment of an examination system combining brain computer interface (BCI) and TCD ultrasonography, the classification and noise cancellation of TCD ultrasonography signals using AI algorithms, and the use of intelligent robots to assist physicians in TCD ultrasonography and discuss the prospects for the development of AI in TCD ultrasonography.
Cerebrovascular disease refers to brain tissue damage caused by obstruction of blood circulation in the brain [1]. Cerebrovascular disease is usually manifested in people who experience acute nonfatal events (i.e., emergency department visit, hospitalization) or fatal events [2], including: stroke [3,4,5], subarachnoid hemorrhage (SAH) [6,7,8], arterial dissection [9,10], cerebral autosomal dominant arteriopathy with subcortical infarcts and leukoencephalopathy (CADASIL) [11,12,13], arteriovenous malformation (AVM) [14,15,16], cerebral venous sinus thrombosis (CVST) [17,18,19], and moyamoya disease [20,21,22]. Stroke is the most common type of cerebrovascular disease [23], which is caused by the blockage or rupture of an artery supplying the brain [1]. Cerebrovascular disease is the leading cause of severe long-term disability and the second leading cause of death worldwide [24]. Cerebrovascular disease has a very high mortality and disability rate. If left untreated, it can lead to lifelong disability or death. Therefore, it is very important to study the diagnosis and treatment of cerebrovascular diseases. Nowadays, the main diagnostic modalities for cerebrovascular disease include electroencephalogram (EEG) [25,26], cerebral angiography (CAG) [27,28,29], MRI [30,31] and transcranial Doppler (TCD) ultrasonography [32,33,34]. TCD ultrasonography can penetrate the skull without trauma. It is easy to perform, reproducible, and allows for continuous, long-term dynamic observation of the patient. More importantly, it can provide important hemodynamic information that cannot be measured by MRI, DSA, single photon emission computed tomography (SPECT), and other imaging techniques. Therefore, it has important significance in the evaluation of cerebrovascular disorders and differential diagnosis.
TCD ultrasonography is a non-invasive examination method to evaluate the hemodynamics of the skull base arteries by using the weak parts of the human skull as the detection acoustic windows (such as the temporal bone, the foramen magnum, and the orbit). [35,36,37]. TCD ultrasonography assesses blood flow status mainly by the level of blood flow velocity [38]. It is the only non-invasive and real-time neuroimaging modality for assessing the blood flow characteristics of the cerebral basal vessels, which can add physiological information to structural imaging [39]. TCD ultrasonography is an inexpensive, rapid, real-time, non-invasive method for measuring cerebrovascular blood flow and is the most convenient method to detect vascular changes in response to intervention during the treatment of clinical acute cerebrovascular events [40]. TCD ultrasonography uses pulsed Doppler technology and a 2 MHz emission frequency to allow the ultrasound beam to penetrate into the thin part of the skull. By directly tracing the Doppler signal of the blood flow in the fundic arteries, the hemodynamic parameters of the fundic arteries are obtained to reflect the functional status of the cerebral vessels and to determine possible intracranial problems. Although TCD ultrasonography can sensitively reflect the functional status of cerebral blood vessels, it cannot guarantee the incidence angle of ultrasound. It requires doctors to clearly understand the path of intracranial blood vessels and be able to perform skilled ultrasound diagnosis. The main drawback of TCD ultrasonography is that the operator cannot see the course of the intracranial vessels and the angle between the vessels and the ultrasound beam, reducing the accuracy of repeated blood flow velocity measurements [41].
Artificial intelligence (AI) is a new technical science that studies theories, methods, technologies and application systems for simulating, extending and expanding human intelligence [42]. AI is a branch of computer science that attempts to understand the essence of intelligence and produce a new intelligent machine that responds in a similar way to human intelligence. Research in this field includes robotics, language recognition, image recognition, natural Language processing (NLP) and expert systems, etc. [43,44]. The rapid development of artificial intelligence has brought many changes to the medical field. AI can detect meaningful relationships in datasets. Artificial Intelligence in Medicine (AIM) mainly uses computer technology to make clinical diagnosis and give treatment recommendations and has been widely used in many clinical situations to diagnose, treat and predict outcomes [45]. In recent years, many studies have shown that AI is increasingly applicable to healthcare, and that algorithms have matched or exceeded the performance of doctors on a growing number of tasks [46]. In addition, research related to AI in emergency medicine is rapidly increasing, especially for acute radiological imaging and prediction-based diagnosis [47,48]. AI has shown great advantages in assisting doctors to predict and diagnose. There have been many studies devoted to the application of AI to TCD ultrasonography, including brain computer interface (BCI) [49,50], TCD signal classification [51,52], noise removal [53,54], and other methods to assist diagnosis and treatment [55,56,57]. AI can greatly improve the accuracy of TCD ultrasonography diagnosis and treatment.
In this paper, w we first review the development, principles, and applications of TCD ultrasonography and other related knowledge, and briefly introduce the development of AI in the field of medicine and emergency medicine. Finally, we summarize in detail the applications and advantages of AI technology in TCD ultrasonography including the establishment of an examination system combining brain computer interface (BCI) and TCD ultrasonography, the classification and noise cancellation of TCD ultrasonography signals using AI algorithms, and the use of intelligent robots to assist physicians in TCD ultrasonography and discuss the prospects for the development of AI in TCD ultrasonography.
Under the same conditions, the inner diameter of the cerebral arteries is relatively almost constant. Corresponding changes in regional cerebral blood flow can be inferred from the decrease or increase in cerebral blood flow velocity [58]. The TCD imaging tool assesses flow parameters and cerebrovascular hemodynamics in real time using low-frequency ultrasound (i.e., ≤ 2 MHz) in the basilar cerebral artery. The physiological information provided by these parameters can be used in conjunction with structural information obtained by various existing imaging techniques [59]. TCD is considered to be the most practical technique to observe cerebral blood flow in the diagnosis of cerebrovascular diseases [60], and the four most commonly used acoustic windows for TCD examination are: temporal, suboccipital, transorbital, and submandibular windows [37]. A comprehensive TCD assessment needs to include measurements from each of the four windows and should examine the flow pathways within each major branch of the Willis loop [61]. TCD assesses the physiological health of specific vascular regions by measuring blood flow relative to blood pressure changes (cerebral autoregulation), end-tidal CO2 changes (cerebrovascular reactivity), and response to cognitive and motor activation (neurovascular coupling or functional congestion), which is commonly used in acute ischemic stroke [62,63,64], vasospasm [65,66], SAH [67,68,69], sickle cell disease [70,71,72], and brain death [73,74,75].
In the late 19th century to the early 20th century, after the discovery of the piezoelectric and anti-piezoelectric effects in physics, people found a way to use electronics to generate ultrasound. From then, a chapter in the history of the development and promotion of ultrasound technology was opened. In 1922, the definition of ultrasound was first proposed. Subsequently, ultrasound technology has made great progress in clinical medicine. In the late 1970s, TCD ultrasonography was proposed and used for blood flow detection [76,77,78]. In 1982, Aaslid et al. [79] described a noninvasive method for determining blood flow velocity in the basilar artery of the brain. The middle cerebral artery (MCA) velocity can be determined from the Doppler signal generated by placing the probe of a Doppler ultrasound instrument in the temporal region above the zygomatic arch. The device has been continuously updated and developed in the following decades and has now reached its fourth generation.
TCD ultrasonography is based on the Doppler effect. The Doppler effect refers to the change in the wavelength of an object's radiation due to the relative motion of the source and the observer. The ultrasound beam does not have directional changes when propagating in the same kind of homogeneous medium because of good penetrating ability of ultrasound. When encountering different media surfaces, the ultrasound beam will be partially reflected, and the rest will continue to propagate. When the surface of the media is irregular and the diameter of the obstacle is smaller than the wavelength of the incident wave, scattering of the ultrasound beam occurs. The receiving probe can receive scattered waves at any angle. There are large numbers of red blood cells in the bloodstream, which are seen as scatterers. The scattered wave reflected back is the main component of the Doppler signal. The formula describing the relationship between flow velocity (reflector velocity) and Doppler shift [37] is:
(1) |
where the wave propagation velocity is a constant, and the propagation velocity in various media can be found. θ is the angle of incidence or the angle of the emitted wave relative to the direction of the blood vessel (blood flow). If the angle is zero or if the emitted wave is parallel to the direction of the flow, since the cosine of 0 is 1, we get the most accurate measurement of the flow velocity. The larger the angle and the smaller the cosine value, the larger the error in our velocity measurement. Therefore, it is important to minimize this angle to less than 30 degrees to keep the error below 15% [37].
The detection depth refers to the distance between the examined vessel and the probe. In each group of pulsed ultrasound waves are emitted by the low-frequency pulse probe transmitter, determined by the range gating through the preset interval between transmitting and receiving pulse waves. The detection depth is very important for the identification of intracranial vessels [80].
The blood flow velocity can be calculated from Eq (1) and is an important parameter to determine the pathology of the body. PSV is the initial peak of the TCD waveform for each cardiac cycle. EDV shows the low resistance flow pattern in all major intracranial arteries and is approximately equal to 20-50% of PSV. MFV is equal to EDV plus one third of the difference between PSV and EDV [81,82,83].
PI and RI are descriptive of the spectral pattern. PI equals PSV minus EDV divided by MFV and RI equals PSV minus EDV divided by PSV. PI is one of the most important hemodynamic parameters provided by TCD to assess cerebral hemodynamic changes in patients with acute neurological injury [84]. RI is generally used to measure flow resistance [85].
The spectral feature of blood flow reflects the state of blood flow in blood vessels. The ordinate on the TCD spectrum is the blood flow velocity. The periphery of the spectrum represents the fastest blood flow velocity at a certain point in the cardiac cycle. The baseline represents zero blood flow velocity. The intensity of the spectral signal is represented by color, and the color change of the signal from weak to strong is blue, yellow, and red. That is, where there are more red blood cells, the signal is red, and where there are fewer red blood cells, the signal is blue [86].
Currently, there are two types of transcranial Doppler devices, non-duplex and duplex. Non-duplex devices (non-imaging devices) can "blindly" identify arteries based on audible Doppler shift and spectrum without visual guidance. Specific identification is based on standard criteria, including: cranial window used, probe orientation, sample volume depth, and blood flow direction, etc. [37]. Duplex equipment (imaging equipment) creating images using duplex technology requires ultrasound equipment, phased arrays, 2-3 MHz, sector transducers, and specific post-processing software to display real-time color Doppler images of vessels within the field of view and the spectral waveform of the interrogated vessel [87].
The term "artificial intelligence" (AI) was formally introduced by scholars at a conference at Dartmouth University in 1956 [44]. AI is knowledge engineering that acquires knowledge, analyzes and studies the expressions of knowledge, and uses these expressions to simulate human intellectual activity [88]. AI uses computers to simulate human behavior and train computers to learn human behavior. The birth of AI has greatly promoted the development of society [89]. AI is widely used in medical fields, such as robotics, medical diagnosis, medical statistics, and human biology. There are two main branches of AIM: virtual and physical. The virtual branch includes informatics approaches ranging from deep learning information management to the control of health management systems, including electronic health records, and active guidance of physicians in treatment decisions. The physical branch is represented by robots that help elderly patients or attending surgeons [90]. The development history of AIM is shown in Figure 1 [91]. In the 1970s, scientists began to explore AIM. Stanford University has developed MYCIN system, which can diagnose and prescribe infectious diseases [92], and ONCOCIN system, which can assist diagnosis [93]. Rutgers University has developed the CASNET system for computer-aided medical decision making [94]. Although the early development of AIM was not very successful, scientists have not given up on the search for AIM. So far, the development of AIM has been relatively mature. The best-known is IBM Watson, which can sift through 1.5 million patient records from decades of cancer treatment in seconds and provide doctors with alternative treatments [95]. Emergency medicine is a growing profession that plays a vital role in the event of patients requiring urgent medical care. Emergency medicine is mainly about providing timely medical triage and classifying patients according to urgency and severity [96]. The use of machine learning and deep learning may help the patterns that identify the data collected over the years and provide insights for improving the emergency department admissions process. Machine learning and deep learning can help ongoing medical trials and research and reduce the workload of medical professionals based on their strengths in areas such as data patterns, trend recognition, image analysis and task classification [97,98]. AI in emergency medicine can be used for pre-hospital emergency management, patient triage and disposition, medical disease and condition prediction, emergency department management, etc. [46].
Brain-computer interface (BCI) refers to create a direct connection between the human or animal brain and an external device to realize the function of information exchange between the brain and the device. It can process brain signals related to mental activity to produce thought-controlled reasoning mechanisms that can be used to control external devices [50]. Changes in TCD signals are related to cognitive tasks. Studies have shown that BCI can be developed based on the classification of cognitive tasks performed by users [99]. TCD has some advantages for the development of noninvasive BCI. Like EEG, TCD is portable. However, it is more robust to non-smoothness from external electrical disturbances and internal background brain activity. In addition, it is cheaper compared to functional MRI and magnetoencephalography [100].
In 2011, Andrew et al. investigated the feasibility of TCD ultrasonography based BCI by classifying the changes in cerebral blood flow velocity due to two thought tasks (word generation task and thought rotation task). The researchers simultaneously asked nine healthy adults to alternate between mental activity (such as word creation and brain rotation) and relaxation and measured cerebral blood flow velocity in their left and right cerebral arteries. Using linear discriminant analysis and a set of temporal features, the word generation task and the thought rotation task were classified according to blood flow velocity with mean accuracies of 82.9% + 10.5 and 85.7% + 10.0, respectively, for all participants. However, since there are few studies on TCD combined with BIC, the authors used a rest and activation time of 45 seconds to obtain high classification accuracy, which is relatively long for BCI applications and will limit the data transfer rate in practical use. In addition, the movement of the body or head of the person during the measurement will change the cerebral blood flow velocity, which is also a factor that cannot be ignored in the study of the combination of TCD and BIC [49]. In 2012, Andrew et al. achieve an average classification accuracy of over 70% of the tertiary problems within 20 seconds of the onset of cognitive activity using bilateral TCD measures, time-domain features, and linear classifiers based on previous research, which corresponds to a maximum data transfer rate of 1.2 bits per minute, as shown in Figure 2(a) [101]. In 2015, Goyal et al. demonstrated the feasibility of a three-class online BCI using a task that requires no visual cues at all. The communication system achieves an average information transfer rate of 1.08 ± 0.69 bits/min. The experimental setup is shown in Figure 2(b) [102]. In 2018, Khalaf et al. used data obtained from bilateral functional TCD ultrasound measurements to investigate the possibility of establishing a level 2 and 3 BCI system. Two different methods for building BCI systems are proposed: Incremental Window (IW) and Moving Window (MW). The main difference between the two methods is windowing and the formulation of eigenvectors. Wavelet transform analysis was performed on the raw data for each method. Statistical features are calculated from the wavelet transform coefficients. Wilcoxon test was performed on these features for feature selection. Then use linear kernel support vector machine (SVC) for classification [103]. The algorithmic flow of the data analysis using the moving window (MW) and incremental window (IW) methods is shown in Figure 2(c). All these results suggest that TCD is a promising measurement for BCI studies. BCI can visually express the changes of brain signals, and the establishment of a reasonable BCI system based on the results of TCD ultrasonography has a crucial role in promoting the development of TCD ultrasonography. The proposed BCI can reduce the high dependence of TCD ultrasonography consultation on human.
Although Doppler spectrum images can reflect the physiological and pathological conditions of each blood vessel to a certain extent, errors will occur when diagnosing diseases only by analyzing the images. TCD signals can be viewed as a series of discrete values that vary over time. Using the relevant knowledge of artificial intelligence to extract features that have never been recognized can reduce the error of diagnosis. In 2002, Guler et al. performed spectral analysis of TCD signals using fast Fourier transform (FFT) and adaptive autoregressive moving average (A-Arma) methods. The results show that the spectral density obtained by the A-Arma method is better than that obtained by the FFT method [52], and the filter structure is shown in Figure 3(a). In 2003, Serhatlioğlu et al. extracted features using FFT and classified these features using back propagation (BP) neural network and self-organizing maps (SOM) algorithm to compare the performance of these classifiers. They used a 16-bit sound card to transmit the TCD signals recorded in the temporal regions of the brains of 110 patients to a computer and processed the recorded signals of each patient using the FFT method. To make a correct and rapid diagnosis, the TCD blood flow signals were statistically sorted and classified by artificial neural network (ANN). Training uses BP neural network and SOM algorithm; learning uses momentum algorithm and delta-bar-delta algorithm. The results of these algorithms are compared in the case of classification and learning [104]. In 2008, Ozturk et al. used two different neuro-fuzzy classifiers to classify chaotic invariant features extracted from TCD signals and compared the performance of the classifiers. They performed a chaotic analysis of TCD signals recorded from the middle artery in the temporal region of the brain in 82 patients and 24 healthy individuals. Among the 82 patients, 20 patients had cerebral aneurysm, 10 patients had cerebral hemorrhage, 22 patients had cerebral edema, and 30 patients had brain tumor. It was found that all TCD signals represent nonlinear dynamics and are potentially low-level deterministic. To compare the classifier performance, two different neuro-fuzzy models, ANFIS and NEFCLASS, are constructed on the training set, and the system structure is shown in Figure 3(b). The rule base for the NEFCLASS model is created by applying a sample of 1000 epochs to the training subset. Furthermore, the ANFIS model was trained for 250 epoch samples until the convergence error dropped to 0.42 × 10−5. For samples in the test set, the ANFIS model achieves better classification accuracy than the NEFCLASS model. The classification accuracy after training of ANFIS model and NEFCLASS model are 94.40 and 88.88%, respectively [105].
From 2008 to 2012, Uğuz et al. conducted a series of studies in the field of TCD signal classification. They first implemented a biomedical system to classify TCD signals recorded from the temporal regions of the brains of 82 patients and 24 healthy individuals. Fuzzy discrete hidden Markov model and Rocchio-based hidden Markov model are used, respectively, to improve the accuracy of TCD signal classification. Subsequently, they combined feature ranking and dimensionality reduction methods when classifying TCD signals to improve classification efficiency and accuracy. To evaluate the effectiveness of the proposed method, experiments were conducted using the SVM classifier. When using the proposed method, higher classification accuracy and minimal number of features are obtained. These results show that the proposed new method can improve the classification efficiency and accuracy compared to the use of each method individually. Its feature extraction process and classification architecture are shown in Figure 3(d), (e) [51,106,107]. In 2017, Seera et al. used recurrent neural network (RNN) to classify TCD signals captured from the brain. They collected a total of 35 real anonymous patient records and conducted a series of narrow diagnostic experiments. Features extracted from TCD signals are classified with an RNN model with much loop feedbacks. The model is shown in Figure 3(f). In addition to the individual RNN results, a majority voting method is used to combine individual RNN predictions into an ensemble prediction. The TCD signals recorded from the arterial segment of the Circle of Willis from ischemic stroke were also analyzed. The results show that the ensemble RNN shows good performance with an average accuracy above 70% and a maximum accuracy of 85% [108]. TCD ultrasonography results are very important for the diagnosis and treatment of cerebrovascular diseases, but simple image analysis cannot make a very accurate judgment of the type of disease. As a result, the classification of TCD signals has greatly improved the accuracy of TCD ultrasonography in the management of acute cerebrovascular disease.
Biomedical signals are often contaminated with noise from sources such as power line interference and interference from recording electrode motion. Noise and interference can affect the original information signal. Karaboga et al. conducted a series of studies on noise cancellation of TCD signals. The artificial bee colony (ABC) algorithm based on the swarm intelligence optimization algorithm is used to design the IIR filter to denoise the TCD noise signal. Noise cancels the patient TCD signal. The SSA method was used for data analysis, and finally the SSA method was used to find new TCD signals' parameters for the determination of healthy subjects and patient subjects. Furthermore, the results are compared with those obtained by methods based on popular and recently introduced evolutionary algorithms and conventional methods. On this basis, the performance of the proposed method is tested to remove the noise on the TCD signal with a signal-to-noise ratio value of 2db. The simulation results show that the IIR filter can be well designed for the noise reduction process by using the ABC algorithm. Therefore, this method can improve the TCD interference signal, making it available for further analysis. Spectrogram indicators for clinical application can be accurately obtained from the maximum frequency curve The structure of the adaptive noise cancellation system is shown in Figure 4(a) [53,109,110]. In 2013, Koza et al. introduced a filter design method to eliminate additive white Gaussian noise through an infinite impulse response digital filter using the Harmony Search (HS) algorithm. To optimize the design, additive white Gaussian noise with different signal-to-noise ratios (-4dB, +4dB, -2dB and +2dB) was added to the mitral TCD signal. Four different infinite impulse response digital filters are designed under different noise levels using the HS algorithm, and their performances are compared. On this basis, a fourth degree IIR filter designed by the ABC algorithm is used to filter the noise-added signal. Using the SSA algorithm to analyze the noise of the TCD signals and discover new TCD parameters for diagnosis [54,111]. TCD signals are easily contaminated by noise in the environment, which can lead to some errors in the diagnosis process. Effective noise removal is necessary to ensure the authenticity of the TCD ultrasonography signals and reduce errors in the diagnosis and treatment of acute cerebrovascular diseases.
A significant limitation of using TCD ultrasound is that it is time-consuming and highly operator dependent. Examination of patients using hand-held technology requires the examiner to have a detailed understanding of cerebral vascular anatomy and its variations, as well as ultrasound technology and various TCD indicators of vasospasm [33]. Computer-aided diagnosis systems have become valuable tools for accurate, reliable, and rapid diagnosis of vascular diseases. The application of AIM has been widely reported in previous studies. Esmaeeli et al. propose that a robot assisted TCD system with AI provides an alternative to manual TCD to assess blood flow velocity within the MCA in acute SAH patients, thereby extending the availability of TCD to settings without specialized clinicians. Recently, a robot-assisted ultrasound system integrating ultrasound, robotics, and machine learning has been proposed, which combines TCD with a square earphone containing a robotic rod that uses machine learning to automatically adjust the ultrasound probe to detect intracranial vasculature. The system can also readjust the probe position after the patient moved to continue sonicating previously acquired positions. In addition, the feasibility and accuracy of robotic-assisted TCD for measuring cerebral blood flow velocities in the anterior and MCA in patients with SAH and vasospasm were examined [57]. In 2022, Clare et al. to test the safety and efficacy of the Nova Guide system in patients with acute SAH in the neurological ICU. The Nova Guide system-five degrees of freedom robotic TCD system is shown in Figure 4(b) [112]. Baig et al. report the use of a robotic TCD system with AI as a novel real-time intraoperative neuromonitoring tool in patients undergoing valve replacement. By reporting the quantity and distribution of high-intensity transient signal (HITS) as a marker of micro embolism during surgery, they propose that the use of sentinel brain protection significantly reduces HITS [56]. In the process of TCD ultrasonography, the operator will be affected by the state of his own body, leading to unnecessary errors in the operation. The intelligent robot will not be affected by these factors, and can accurately complete the TCD ultrasonography, greatly improving the reliability of TCD ultrasonography.
In 2021, Wang et al. designed an AI neighborhood segmentation algorithm based on the characteristics of TCD ultrasound images of patients with traumatic brain injury to detect neuroendocrine changes in the acute phase of patients with severe traumatic brain injury. Their findings showed that the AI neighborhood segmentation algorithm had significantly lower regional segmentation error and runtime for TCD ultrasonography images, and significantly improved concordance between the Glasgow Coma Scale and pituitary hormone abnormalities. However, there are still some shortcomings. For example, the types of neuroendocrine monitoring hormones included in this study are relatively single, mainly focusing on pituitary hormones, and lack of monitoring indicators for other neuroendocrine hormones. The AI neighborhood segmentation algorithm they researched and designed uses relatively few monitoring indicators in image segmentation quality evaluation. In the future, more algorithm evaluation indicators will be included in the further optimization of the algorithm, and then the value of the algorithm will be comprehensively analyzed from multiple perspectives [113]. Mei et al. explored the diagnostic value of convolutional neural network (CNN) in MAC stenosis by analyzing TCD ultrasonography images. According to the results of cerebral angiography, 278 patients who underwent cerebral vascular TCD and cerebral angiography were divided into stenosis group and non-stenosis group. Manual measurements were made on TCD images. The patients were divided into training and test sets, and the TCD images were classified using a CNN architecture. The diagnostic accuracy of manual measurements of MCA stenosis, CNN and TCD parameters were calculated and compared. The diagnostic value of CNN based on TCD images for MCA stenosis was like manual measurements. The results show that CNN can be used as an auxiliary diagnostic tool to improve the diagnosis of MCA stenosis [55]. The training process is shown in Figure 4(c).
TCD ultrasonography has shown great advantages in the treatment of cerebrovascular diseases, but there are still some key issues that need to be addressed to make it more accurate in determining the type of disease. This will certainly lead to further development of TCD ultrasonography. Scientists have not stopped exploring this field, and the future of TCD ultrasonography has great potential for development.
In clinical, cerebrovascular disease usually manifests itself in people who experience acute nonfatal events (i.e., emergency department visit, hospitalization) or fatal events. It is one of the causes of severe human disability and is also the second leading cause of death worldwide. Therefore, timely prediction and diagnosis of cerebrovascular disease can enable timely early treatment [2,114,115]. TCD is a relatively inexpensive, rapid, real-time, noninvasive measure of cerebrovascular blood flow and is the most convenient method for detecting vascular changes during the treatment of acute clinical cerebrovascular events in response to interventions. It has shown great potential in the diagnosis and treatment of cerebrovascular diseases [39,40].AI has demonstrated great advantages in several fields of society since its introduction. It has also brought various important technological tools to the medical field [45,46,47,48]. Meanwhile, in the field of emergency medicine, AI has shown great advantages in pre-hospital emergency management, patient triage and disposal, medical disease and condition prediction, and emergency department management [46,48,97,98]. Until now, there have been many studies on the use of AI for TCD ultrasonography. These include the combination of TCD ultrasonography and BCI [49,50,99,100,101,102], the classification and noise cancellation of TCD signals using AI [51,52,53,54,104,105,106,107,108,109,110,111], and the use of intelligent robots and computerized diagnostic systems to assist physicians in disease diagnosis and treatment [33,55,56,57,112,113]. The development of AI has improved the accuracy of TCD ultrasonography in several ways and greatly promoted the diagnosis of TCD in acute cerebrovascular disease development. The birth of AI has given new impetus to the development of medicine. TCD ultrasonography has more advantages than other diagnostic and treatment methods. Therefore, the combination of AI with it will further promote the application and development of TCD ultrasonography.
TCD ultrasonography has made rapid development after it was proposed, and it has shown great advantages in the diagnosis of acute cerebrovascular diseases. However, the detection process requires the operator to have extremely high proficiency, and the influence of some external factors will reduce the accuracy of the detection results. With the development of AI, people are committed to using AI methods to improve the accuracy of TCD ultrasonography, including AI based TCD signals classification, TCD signals noise removal, and intelligent robots to assist doctors in diagnosis and treatment. With the further development of AI, TCD ultrasonography also has more potential for development.
The authors declare there is no conflict of interest.
[1] |
M. Portegies, P. Koudstaal, M. Ikram, Cerebrovascular disease, Handb. Clin. Neurol., 138 (2016), 239–261. https://doi.org/10.1016/B978-0-12-802973-2.00014-8 doi: 10.1016/B978-0-12-802973-2.00014-8
![]() |
[2] |
X. Tong, Q. Yang, M. D. Ritchey, M. G. George, S. L. Jackson, C. Gillespie, et al., The burden of cerebrovascular disease in the United States, Prev. Chronic. Dis., 16 (2019), 180411. https://doi.org/10.5888/pcd16.180411 doi: 10.5888/pcd16.180411
![]() |
[3] |
S. Yoshimura, N. Sakai, H. Yamagami, K. Uchida, M. Beppu, K. Toyoda, et al., Endovascular therapy for acute stroke with a large ischemic region, N. Engl. J. Med., 386 (2022), 1303–1313. https://doi.org/10.1056/NEJMoa2118191 doi: 10.1056/NEJMoa2118191
![]() |
[4] |
Q. Z. Tuo, S. T. Zhang, P. Lei, Mechanisms of neuronal cell death in ischemic stroke and their therapeutic implications, Med. Res. Rev., 42 (2022), 259–305. https://doi.org/10.1002/med.21817 doi: 10.1002/med.21817
![]() |
[5] |
K. N. Kadyrovich, S. K. Erkinovich, K. M. Ilhomovna, Microscopic examination of postcapillary cerebral venues in hemorrhagic stroke, Am. J. Med. Sci. Pharm. Res., 3 (2021), 69–73. https://doi.org/10.37547/TAJMSPR/Volume03Issue08-11 doi: 10.37547/TAJMSPR/Volume03Issue08-11
![]() |
[6] |
M. L. Osgood, Aneurysmal subarachnoid hemorrhage: Review of the pathophysiology and management strategies, Curr. Neurol. Neurosci. Rep., 21 (2021), 1–11. https://doi.org/10.1007/s11910-021-01136-9 doi: 10.1007/s11910-021-01136-9
![]() |
[7] |
S. N. Neifert, E. K. Chapman, M. L. Martini, W. H. Shuman, A. J. Schupper, E. K. Oermann, et al., Aneurysmal subarachnoid hemorrhage: The last decade, Transl. Stroke Res., 12 (2021), 428–446. https://doi.org/10.1007/s12975-020-00867-0 doi: 10.1007/s12975-020-00867-0
![]() |
[8] |
W. S. Dodd, D. Laurent, A. S. Dumont, D. M. Hasan, P. M. Jabbour, R. M. Starke, et al., Pathophysiology of delayed cerebral ischemia after subarachnoid hemorrhage: A review, J. Am. Heart Assoc., 10 (2021), e021845. https://doi.org/10.1161/jaha.121.021845 doi: 10.1161/jaha.121.021845
![]() |
[9] |
I. Daghals, M. Sargurupremraj, R. Danning, P. Gormley, R. Malik, P. Amouyel, et al., Migraine, stroke, and cervical arterial dissection: Shared genetics for a triad of brain disorders with vascular involvement, Neurol. Genet., 8 (2022), e653. https://doi.org/10.1212/nxg.0000000000000653 doi: 10.1212/nxg.0000000000000653
![]() |
[10] |
M. Marciniec, K. Sapko, M. Kulczyński, S. Popek-Marciniec, A. Szczepańska-Szerej, K. Rejdak, Non-traumatic cervical artery dissection and ischemic stroke: A narrative review of recent research, Clin. Neurol. Neurosurg., 187 (2019), 105561. https://doi.org/10.1016/j.clineuro.2019.105561 doi: 10.1016/j.clineuro.2019.105561
![]() |
[11] |
M. M. Ruchoux, C. A. Maurage, CADASIL: Cerebral autosomal dominant arteriopathy with subcortical infarcts and leukoencephalopathy, J. Neuropathol. Exp. Neurol., 56 (1997), 947–964. https://doi.org/10.1097/00005072-199709000-00001 doi: 10.1097/00005072-199709000-00001
![]() |
[12] |
M. Bousser, E. Tournier-Lasserve, Cerebral autosomal dominant arteriopathy with subcortical infarcts and leukoencephalopathy: From stroke to vessel wall physiology, J. Neurol. Neurosurg. Psychiatry, 70 (2001), 285–287. https://doi.org/10.1136/jnnp.70.3.285 doi: 10.1136/jnnp.70.3.285
![]() |
[13] | E. Navarro, F. Díaz, L. Muñoz, S. Giménez-Roldán, Dominant autosomal cerebral arteriopathy with subcortical infarcts and leukoencephalopathy (CADASIL): A review, Neurologia, 17 (2002), 410–417. |
[14] |
L. Prado, C. Han, S. P. Oh, H. Su, Recent advances in basic research for brain arteriovenous malformation, Int. J. Mol. Sci., 20 (2019), 5324. https://doi.org/10.3390/ijms20215324 doi: 10.3390/ijms20215324
![]() |
[15] |
P. Pan, S. Weinsheimer, D. Cooke, E. Winkler, A. Abla, H. Kim, et al., Review of treatment and therapeutic targets in brain arteriovenous malformation, J. Cereb. Blood Flow Metab., 41 (2021), 3141–3156. https://doi.org/10.1177/0271678x211026771 doi: 10.1177/0271678x211026771
![]() |
[16] |
S. S. Shaligram, E. Winkler, D. Cooke, H. Su, Risk factors for hemorrhage of brain arteriovenous malformation, CNS Neurosci. Ther., 25 (2019), 1085–1095. https://doi.org/10.1111/cns.13200 doi: 10.1111/cns.13200
![]() |
[17] |
M. Capecchi, M. Abbattista, I. Martinelli, Cerebral venous sinus thrombosis, J. Thromb. Haemostasis, 76 (2018), 12–15. https://doi.org/10.1111/jth.14210 doi: 10.1111/jth.14210
![]() |
[18] |
G. Sébire, B. Tabarki, D. E. Saunders, I. Leroy, R. Liesner, C. Saint-Martin, et al., Cerebral venous sinus thrombosis in children: Risk factors, presentation, diagnosis and outcome, Brain, 128 (2005), 477–489. https://doi.org/10.1093/brain/awh412 doi: 10.1093/brain/awh412
![]() |
[19] |
J. M. Ferro, P. Canhão, Cerebral venous sinus thrombosis: Update on diagnosis and management, Curr. Cardiol. Rep., 16 (2014), 523. https://doi.org/10.1007/s11886-014-0523-2 doi: 10.1007/s11886-014-0523-2
![]() |
[20] |
H. Zhang, L. Zheng, L. Feng, Epidemiology, diagnosis and treatment of moyamoya disease, Exp. Ther. Med., 17 (2019), 1977–1984. https://doi.org/10.3892/etm.2019.7198 doi: 10.3892/etm.2019.7198
![]() |
[21] |
J. Li, M. Jin, X. Sun, J. Li, Y. Liu, Y. Xi, et al., Imaging of moyamoya disease and moyamoya syndrome: Current status, J. Comput. Assist. Tomogr., 43 (2019), 257–263. https://doi.org/10.1097/rct.0000000000000834 doi: 10.1097/rct.0000000000000834
![]() |
[22] |
S. Shang, D. Zhou, J. Ya, S. Li, Q. Yang, Y. Ding, et al., Progress in moyamoya disease, Neurosurg. Rev., 43 (2020), 371–382. https://doi.org/10.1007/s10143-018-0994-5 doi: 10.1007/s10143-018-0994-5
![]() |
[23] |
C. Esenwa, J. Gutierrez, Secondary stroke prevention: Challenges and solutions, Vasc. Health Risk Manag., 11 (2015), 437–450. https://doi.org/10.2147/vhrm.S63791 doi: 10.2147/vhrm.S63791
![]() |
[24] |
V. L. Feigin, M. H. Forouzanfar, R. Krishnamurthi, G. A. Mensah, M. Connor, D. A. Bennett, et al., Global and regional burden of stroke during 1990–2010: Findings from the global burden of disease study 2010, Lancet, 383 (2014), 245–254. https://doi.org/10.1016/s0140-6736(13)61953-4 doi: 10.1016/s0140-6736(13)61953-4
![]() |
[25] |
I. Nagy, D. Fabó, Clinical neurophysiological methods in diagnosis and treatment of cerebrovascular diseases, Ideggyogy. Sz., 71 (2018), 7–14. https://doi.org/10.18071/isz.71.0007 doi: 10.18071/isz.71.0007
![]() |
[26] |
J. A. Barios, S. Ezquerro, A. Bertomeu-Motos, J. M. Catalan, J. M. Sanchez-Aparicio, L. Donis-Barber, et al., Movement-related EEG oscillations of contralesional hemisphere discloses compensation mechanisms of severely affected motor chronic stroke patients, Int. J. Neural. Syst., 31 (2021), 2150053. https://doi.org/10.1142/s0129065721500532 doi: 10.1142/s0129065721500532
![]() |
[27] |
S. Shaban, B. Huasen, A. Haridas, M. Killingsworth, J. Worthington, P. Jabbour, et al., Digital subtraction angiography in cerebrovascular disease: Current practice and perspectives on diagnosis, acute treatment and prognosis, Acta Neurol. Belg., 122 (2022), 763–780. https://doi.org/10.1007/s13760-021-01805-z doi: 10.1007/s13760-021-01805-z
![]() |
[28] |
C. C. Young, R. H. Bonow, G. Barros, M. Mossa-Basha, L. J. Kim, M. R. Levitt, Magnetic resonance vessel wall imaging in cerebrovascular diseases, Neurosurg. Focus, 47 (2019), E4. https://doi.org/10.3171/2019.9.Focus19599 doi: 10.3171/2019.9.Focus19599
![]() |
[29] |
S. F. Xiang, J. T. Li, S. J. Yang, F. F. Ding, W. W. Wang, S. Huo, et al., Whole-brain volume CT angiography can effectively detect early ischemic cerebrovascular diseases, Curr. Med. Imaging, 18 (2022), 731–738. https://doi.org/10.2174/1573405617666211206091831 doi: 10.2174/1573405617666211206091831
![]() |
[30] |
K. Taneja, H. Lu, B. G. Welch, B. P. Thomas, M. Pinho, D. Lin, et al., Evaluation of cerebrovascular reserve in patients with cerebrovascular diseases using resting-state MRI: A feasibility study, Magn. Reson. Imaging, 59 (2019), 46–52. https://doi.org/10.1016/j.mri.2019.03.003 doi: 10.1016/j.mri.2019.03.003
![]() |
[31] |
L. Carnevale, G. Lembo, Innovative MRI techniques in neuroimaging approaches for cerebrovascular diseases and vascular cognitive impairment, Int. J. Mol. Sci., 20 (2019), 2656. https://doi.org/10.3390/ijms20112656 doi: 10.3390/ijms20112656
![]() |
[32] |
J. S. Minhas, R. B. Panerai, G. Ghaly, P. Divall, T. G. Robinson, Cerebral autoregulation in hemorrhagic stroke: A systematic review and meta-analysis of transcranial Doppler ultrasonography studies, J. Clin. Ultrasound, 47 (2019), 14–21. https://doi.org/10.1002/jcu.22645 doi: 10.1002/jcu.22645
![]() |
[33] |
S. Sharma, R. J. Lubrica, M. Song, R. Vandse, W. Boling, P. Pillai, The role of transcranial Doppler in cerebral vasospasm: A literature review, Subarachnoid Hemorrh., 127 (2020), 201–205. https://doi.org/10.1007/978-3-030-04615-6_32 doi: 10.1007/978-3-030-04615-6_32
![]() |
[34] |
N. Fatima, A. Shuaib, T. S. Chughtai, A. Ayyad, M. Saqqur, The role of transcranial Doppler in traumatic brain injury: A Systemic review and Meta-analysis, Asian J. Neurosurg., 14 (2019), 626–633. https://doi.org/10.4103/ajns.AJNS_42_19 doi: 10.4103/ajns.AJNS_42_19
![]() |
[35] |
V. K. Sharma, K. S. Wong, A. V. Alexandrov, Transcranial Doppler, Front. Neurol. Neurosci., 40 (2016), 124–140. https://doi.org/10.1159/000448309 doi: 10.1159/000448309
![]() |
[36] |
C. C. Bishop, S. Powell, D. Rutt, N. L. Browse, Transcranial Doppler measurement of middle cerebral artery blood flow velocity: A validation study, Stroke, 17 (1986), 913–915. https://doi.org/10.1161/01.str.17.5.913 doi: 10.1161/01.str.17.5.913
![]() |
[37] |
S. Purkayastha, F. Sorond, Transcranial Doppler ultrasound: Technique and application, Semin. Neurol., 32 (2012), 411–420. https://doi.org/10.1055/s-0032-1331812 doi: 10.1055/s-0032-1331812
![]() |
[38] |
C. K. Willie, F. L. Colino, D. M. Bailey, Y. C. Tzeng, G. Binsted, L. W. Jones, et al., Utility of transcranial Doppler ultrasound for the integrative assessment of cerebrovascular function, J. Neurosci. Methods., 196 (2011), 221–237. https://doi.org/10.1016/j.jneumeth.2011.01.011 doi: 10.1016/j.jneumeth.2011.01.011
![]() |
[39] |
G. Tsivgoulis, A. V. Alexandrov, M. A. Sloan, Advances in transcranial Doppler ultrasonography, Curr. Neurol. Neurosci. Rep., 9 (2009), 46–54. https://doi.org/10.1007/s11910-009-0008-7 doi: 10.1007/s11910-009-0008-7
![]() |
[40] |
F. A. Rasulo, E. De Peri, A. Lavinio, Transcranial Doppler ultrasonography in intensive care, Eur. J. Anaesthesiol. Suppl., 42 (2008), 167–173. https://doi.org/10.1017/s0265021507003341 doi: 10.1017/s0265021507003341
![]() |
[41] |
T. Montrief, S. Alerhand, C. Jewell, J. Scott, Incorporation of transcranial Doppler into the ED for the neurocritical care patient, Am. J. Emerg. Med., 37 (2019), 1144–1152. https://doi.org/10.1016/j.ajem.2019.03.003 doi: 10.1016/j.ajem.2019.03.003
![]() |
[42] | J. H. Fetzer, What is artificial intelligence, in Artificial Intelligence: Its Scope and Limits (eds J. H. Fetzer), (1990), 3–27. https://doi.org/10.1007/978-94-009-1900-6_1 |
[43] |
M. Haenlein, A. Kaplan, A brief history of artificial intelligence: On the past, present, and future of artificial intelligence, Calif. Manage. Rev., 61 (2019), 5–14. https://doi.org/10.1177/0008125619864925 doi: 10.1177/0008125619864925
![]() |
[44] |
C. Zhang, Y. Lu, Study on artificial intelligence: The state of the art and future prospects, J. Ind. Inf. Integr., 23 (2021), 100224. https://doi.org/10.1016/j.jii.2021.100224 doi: 10.1016/j.jii.2021.100224
![]() |
[45] |
Y. K. Chan, Y. F. Chen, T. Pham, W. Chang, M. Y. Hsieh, Artificial intelligence in medical applications, J. Healthcare Eng., 2018 (2018), 1–2. https://doi.org/10.1155/2018/4827875 doi: 10.1155/2018/4827875
![]() |
[46] |
J. Stewart, P. Sprivulis, G. Dwivedi, Artificial intelligence and machine learning in emergency medicine, Emerg. Med. Australas., 30 (2018), 870–874. https://doi.org/10.1111/1742-6723.13145 doi: 10.1111/1742-6723.13145
![]() |
[47] |
A. Kirubarajan, A. Taher, S. Khan, S. Masood, Artificial intelligence in emergency medicine: A scoping review, J. Am. Coll. Emerg. Phys. Open., 1 (2020), 1691–1702. https://doi.org/10.1002/emp2.12277 doi: 10.1002/emp2.12277
![]() |
[48] |
Y. Berlyand, A. S. Raja, S. C. Dorner, A. M. Prabhakar, J. D. Sonis, R. V. Gottumukkala, et al., How artificial intelligence could transform emergency department operations, Am. Jo. Emerg. Med., 36 (2018), 1515–1517. https://doi.org/10.1016/j.ajem.2018.01.017 doi: 10.1016/j.ajem.2018.01.017
![]() |
[49] |
A. J. Myrden, A. Kushki, E. Sejdić, A. M. Guerguerian, T. Chau, A brain-computer interface based on bilateral transcranial Doppler ultrasound, PLoS One, 6 (2011), e24170. https://doi.org/10.1371/journal.pone.0024170 doi: 10.1371/journal.pone.0024170
![]() |
[50] |
N. Birbaumer, Breaking the silence: Brain-computer interfaces (BCI) for communication and motor control, Psychophysiology, 43 (2006), 517–532. https://doi.org/10.1111/j.1469-8986.2006.00456.x doi: 10.1111/j.1469-8986.2006.00456.x
![]() |
[51] |
H. Uğuz, A. Öztürk, R. Saraçoğlu, A. Arslan, A biomedical system based on fuzzy discrete hidden Markov model for the diagnosis of the brain diseases, Expert Syst. Appl., 35 (2008), 1104–1114. https://doi.org/10.1016/j.eswa.2007.08.006 doi: 10.1016/j.eswa.2007.08.006
![]() |
[52] |
I. Güler, F. Hardalaç, M. Kaymaz, Comparison of FFT and adaptive ARMA methods in transcranial Doppler signals recorded from the cerebral vessels, Comput. Biol. Med., 32 (2002), 445–453. https://doi.org/10.1016/s0010-4825(02)00036-7 doi: 10.1016/s0010-4825(02)00036-7
![]() |
[53] |
N. Karaboga, F. Latifoglu, Elimination of noise on transcranial Doppler signal using ⅡR filters designed with artificial bee colony—ABC-algorithm, Digital Signal Process., 23 (2013), 1051–1058. https://doi.org/10.1016/j.dsp.2012.09.015 doi: 10.1016/j.dsp.2012.09.015
![]() |
[54] | T. Koza, S. Koçkana, N. Karaboğa, F. Latifoğlu, SSA analysis of noise eliminated transcranial doppler signals with iIR filters, in 2015 23nd Signal Processing and Communications Applications Conference (SIU), (2015), 2021–2024. https://doi.org/10.1109/SIU.2015.7130262 |
[55] |
Y. J. Mei, R. T. Hu, J. Lin, H. Y. Xu, L. Y. Wu, H. P. Li, et al., Diagnosis of middle cerebral artery stenosis using transcranial Doppler images based on convolutional neural network, World Neurosurg., 161 (2022), e118–e125. https://doi.org/10.1016/j.wneu.2022.01.068 doi: 10.1016/j.wneu.2022.01.068
![]() |
[56] |
A. Baig, C. Manion, V. Iyer, W. Khawar, B. Donnelly, A. Monteiro, et al., E-142 robotic transcranial doppler with artificial intelligence to identify cerebral emboli during transcatheter aortic valve replacement - a novel neuromonitoring tool, J. NeuroInterventional Surg., 14 (2022), A152. https://doi.org/10.1136/neurintsurg-2022-SNIS.253 doi: 10.1136/neurintsurg-2022-SNIS.253
![]() |
[57] |
S. Esmaeeli, C. M. Hrdlicka, A. Brenes Bastos, J. Wang, S. Gomez-Paz, K. A. Hanafy, et al., Robotically assisted transcranial Doppler with artificial intelligence for assessment of cerebral vasospasm after subarachnoid hemorrhage, J. Neurocrit. Care, 13 (2020), 32–40. https://doi.org/10.18700/jnc.200002 doi: 10.18700/jnc.200002
![]() |
[58] |
H. White, B. Venkatesh, Applications of transcranial Doppler in the ICU: A review, Intensive Care Med., 32 (2006), 981–994. https://doi.org/10.1007/s00134-006-0173-y doi: 10.1007/s00134-006-0173-y
![]() |
[59] |
Y. Pan, W. Wan, M. Xiang, Y. Guan, Transcranial Doppler ultrasonography as a diagnostic tool for cerebrovascular disorders, Front. Hum. Neurosci., 16 (2022), 841809. https://doi.org/10.3389/fnhum.2022.841809 doi: 10.3389/fnhum.2022.841809
![]() |
[60] |
J. C. Granry, Transcranial Doppler in anesthesia and intensive care, Ann. Fr. Anesth. Reanim., 10 (1991), 127–136. https://doi.org/10.1016/s0750-7658(05)80453-8 doi: 10.1016/s0750-7658(05)80453-8
![]() |
[61] |
M. P. Spencer, D. Whisler, Transorbital Doppler diagnosis of intracranial arterial stenosis, Stroke, 17 (1986), 916–921. https://doi.org/10.1161/01.str.17.5.916 doi: 10.1161/01.str.17.5.916
![]() |
[62] |
L. Thomassen, U. Waje-Andreassen, H. Naess, J. Aarseth, D. Russell, Doppler ultrasound and clinical findings in patients with acute ischemic stroke treated with intravenous thrombolysis, Eur. J. Neurol., 12 (2005), 462–465. https://doi.org/10.1111/j.1468-1331.2005.01008.x doi: 10.1111/j.1468-1331.2005.01008.x
![]() |
[63] |
J. Allendoerfer, M. Goertler, G. M. von Reutern, Prognostic relevance of ultra-early doppler sonography in acute ischaemic stroke: A prospective multicentre study, Lancet Neurol., 5 (2006), 835–840. https://doi.org/10.1016/s1474-4422(06)70551-8 doi: 10.1016/s1474-4422(06)70551-8
![]() |
[64] |
A. Mattioni, S. Cenciarelli, P. Eusebi, M. Brazzelli, T. Mazzoli, M. Del Sette, et al., Transcranial Doppler sonography for detecting stenosis or occlusion of intracranial arteries in people with acute ischaemic stroke, Cochrane Database Syst. Rev., 2 (2020), Cd010722. https://doi.org/10.1002/14651858.CD010722.pub2 doi: 10.1002/14651858.CD010722.pub2
![]() |
[65] |
N. Samagh, H. Bhagat, K. Jangra, Monitoring cerebral vasospasm: How much can we rely on transcranial Doppler, J. Anaesthesiol. Clin. Pharmacol., 35 (2019), 12–18. https://doi.org/10.4103/joacp.JOACP_192_17 doi: 10.4103/joacp.JOACP_192_17
![]() |
[66] |
R. Aaslid, Transcranial Doppler assessment of cerebral vasospasm, Eur. J. Ultrasound, 16 (2002), 3–10. https://doi.org/10.1016/s0929-8266(02)00045-9 doi: 10.1016/s0929-8266(02)00045-9
![]() |
[67] |
D. D. Li, J. Y. Chang, C. X. Zhou, J. B. Cui, Clinical diagnosis of cerebral vasospasm after subarachnoid hemorrhage by using transcranial Doppler sonography, Eur. Rev. Med. Pharmacol. Sci., 22 (2018), 2029–2035. https://doi.org/10.26355/eurrev_201804_14732 doi: 10.26355/eurrev_201804_14732
![]() |
[68] | C. E. Scherle Matamoros, E. A. Samaniego, K. Sam, J. A. Roa, J. P. Nellar, D. R. Rodríguez, Prediction of symptomatic vasospasm in patients with aneurysmal subarachnoid hemorrhage using early transcranial Doppler, J. Vasc. Interv. Neurol., 11 (2020), 19–26. |
[69] |
R. Behrouz, The rise and fall of transcranial Doppler ultrasonography for the diagnosis of vasospasm in aneurysmal subarachnoid hemorrhage, J. Neurosurg. Anesthesiol., 31 (2019), 79–80. https://doi.org/10.1097/ana.0000000000000490 doi: 10.1097/ana.0000000000000490
![]() |
[70] |
B. P. D. Inusa, L. Sainati, C. MacMahon, R. Colombatti, M. Casale, S. Perrotta, et al., An educational study promoting the delivery of transcranial Doppler ultrasound screening in paediatric sickle cell disease: A european multi-centre perspective, J. Clin. Med., 9 (2019), 44. https://doi.org/10.3390/jcm9010044 doi: 10.3390/jcm9010044
![]() |
[71] |
J. Kanter, S. Phillips, A. M. Schlenz, M. Mueller, M. Dooley, L. Sirline, et al., Transcranial doppler screening in a current cohort of children with sickle cell anemia: Results from the displace study, J. Pediatr. Hematol. Oncol., 43 (2021), e1062–e1068. https://doi.org/10.1097/mph.0000000000002103 doi: 10.1097/mph.0000000000002103
![]() |
[72] |
S. Thurn, K. Kleinschmidt, I. Kovacic, C. Wendl, R. A. Linker, S. Corbacioglu, et al., Transcranial Doppler sonography and the effect of haematopoietic stem cell transplantation in sickle cell disease, Neurol. Res. Pract., 4 (2022), 12. https://doi.org/10.1186/s42466-022-00175-y doi: 10.1186/s42466-022-00175-y
![]() |
[73] |
A. Pedicelli, M. Bartocci, E. Lozupone, F. D'Argento, A. Alexandre, G. Garignano, et al., The role of cervical color Doppler ultrasound in the diagnosis of brain death, Neuroradiology, 61 (2019), 137–145. https://doi.org/10.1007/s00234-018-2111-4 doi: 10.1007/s00234-018-2111-4
![]() |
[74] |
Y. Zurynski, N. Dorsch, I. Pearson, R. Choong, Transcranial Doppler ultrasound in brain death: Experience in 140 patients, Neurol. Res., 13 (1991), 248–252. https://doi.org/10.1080/01616412.1991.11740000 doi: 10.1080/01616412.1991.11740000
![]() |
[75] |
D. Escudero, J. Otero, B. Quindós, L. Viña, Transcranial Doppler ultrasound in the diagnosis of brain death. Is it useful or does it delay the diagnosis, Med. Intensiva, 39 (2015), 244–250. https://doi.org/10.1016/j.medin.2014.11.005 doi: 10.1016/j.medin.2014.11.005
![]() |
[76] |
D. Grosset, Aneurysm re-rupture: Doppler evidence of first phase vasospasm, J. Neurol. Neurosurg. Psychiatry, 53 (1990), 361. https://doi.org/10.1136/jnnp.53.4.361-b doi: 10.1136/jnnp.53.4.361-b
![]() |
[77] | J. De Léan, N. Thomas, Neurological Complications of Pregnancy Advances in Neurology, VOLUME 64, 1993, Edited by O. Devinsky, E. Feldman and B. Hainline, Published by Raven Press, 286 pages, $C117.00, Can. J. Neurol. Sci., 24 (1977), 88. https://doi.org/10.1017/S031716710002120X |
[78] | R. A. Purdy, Neurology in Clinical Practice, VOLUME 1 & 2, 1995, Edited by W. G. Bradley, R. B. Daroff, G. M. Fenichel, C. D. Marsden, Published by Butterworth-Heinemann, 3264 pages, $C439.00, Can. J. Neurol. Sci., 24 (1977), 88–89. https://doi.org/10.1017/S0317167100021223 |
[79] |
R. Aaslid, T. M. Markwalder, H. Nornes, Noninvasive transcranial Doppler ultrasound recording of flow velocity in basal cerebral arteries, J. Neurosurg., 57 (1982), 769–774. https://doi.org/10.3171/jns.1982.57.6.0769 doi: 10.3171/jns.1982.57.6.0769
![]() |
[80] |
A. H. Ropper, S. M. Kehne, L. Wechsler, Transcranial Doppler in brain death, Neurology, 37 (1987), 1733–1733. https://doi.org/10.1212/wnl.37.11.1733 doi: 10.1212/wnl.37.11.1733
![]() |
[81] |
N. K. Altinbas, E. Ustuner, H. Ozcan, S. Bilgic, T. Sancak, E. Dusunceli, Effect of carotid artery stenting on ophthalmic artery flow patterns, J. Ultrasound Med., 33 (2014), 629–638. https://doi.org/10.7863/ultra.33.4.629 doi: 10.7863/ultra.33.4.629
![]() |
[82] |
J. Sun, Y. Liu, J. Zhang, X. Chen, Z. Lin, S. Nie, et al., Electroacupuncture improves cerebral vasospasm and functional outcome of patients with aneurysmal subarachnoid hemorrhage, Front. Neurosci., 12 (2018), 724. https://doi.org/10.3389/fnins.2018.00724 doi: 10.3389/fnins.2018.00724
![]() |
[83] |
D. B. Berry, E. K. Englund, S. Chen, L. R. Frank, S. R. Ward, Medical imaging of tissue engineering and regenerative medicine constructs, Biomater. Sci., 9 (2021), 301–314. https://doi.org/10.1039/d0bm00705f doi: 10.1039/d0bm00705f
![]() |
[84] | O. M. Pinillos, C. N. Rodríguez, R. Hakimi, Transcranial Doppler ultrasound pulsatility index: Utility and clinical interpretation, in Neurosonology in Critical Care: Monitoring the Neurological Impact of the Critical Pathology (eds C. N. Rodríguez and C. Baracchini), (2022), 357–376. https://doi.org/10.1007/978-3-030-81419-9_21 |
[85] |
N. G. Rainov, J. B. Weise, W. Burkert, Transcranial Doppler sonography in adult hydrocephalic patients, Neurosurg. Rev., 23 (2000), 34–38. https://doi.org/10.1007/s101430050029 doi: 10.1007/s101430050029
![]() |
[86] |
L. Wang, Y. Xing, Y. Li, K. Han, J. Chen, Evaluation of flow velocity in unilateral middle cerebral artery stenosis by Transcranial Doppler, Cell Biochem. Biophys., 70 (2014), 823–830. https://doi.org/10.1007/s12013-014-9986-4 doi: 10.1007/s12013-014-9986-4
![]() |
[87] |
M. B. McCarville, Comparison of duplex and nonduplex transcranial Doppler ultrasonography, Ultrasound Q, 24 (2008), 167–171. https://doi.org/10.1097/RUQ.0b013e3181862b67 doi: 10.1097/RUQ.0b013e3181862b67
![]() |
[88] |
L. Duan, L. D. Xu, Business Intelligence for Enterprise Systems: A Survey, IEEE Trans. Ind. Inf., 8 (2012), 679–687. https://doi.org/10.1109/tii.2012.2188804 doi: 10.1109/tii.2012.2188804
![]() |
[89] |
Y. Lu, L. D. Xu, Internet of things (IoT) cybersecurity research: A review of current research topics, IEEE Int. Things J., 6 (2019), 2103–2115. https://doi.org/10.1109/jiot.2018.2869847 doi: 10.1109/jiot.2018.2869847
![]() |
[90] |
P. Hamet, J. Tremblay, Artificial intelligence in medicine, Metabolism, 69 (2017), S36–S40. https://doi.org/10.1016/j.metabol.2017.01.011 doi: 10.1016/j.metabol.2017.01.011
![]() |
[91] |
V. Kaul, S. Enslin, S. A. Gross, History of artificial intelligence in medicine, Gastrointest. Endosc., 92 (2020), 807–812. https://doi.org/10.1016/j.gie.2020.06.040 doi: 10.1016/j.gie.2020.06.040
![]() |
[92] |
E. H. Shortliffe, R. Davis, S. G. Axline, B. G. Buchanan, C. C. Green, S. N. Cohen, Computer-based consultations in clinical therapeutics: Explanation and rule acquisition capabilities of the MYCIN system, Comput. Biomed. Res., 8 (1975), 303–320. https://doi.org/10.1016/0010-4809(75)90009-9 doi: 10.1016/0010-4809(75)90009-9
![]() |
[93] |
B. G. Buchanan, E. A. Feigenbaum, The stanford heuristic programming project: Goals and activities, AI Mag., 1 (2017), 25. https://doi.org/10.1609/aimag.v1i1.89 doi: 10.1609/aimag.v1i1.89
![]() |
[94] |
S. M. Weiss, C. A. Kulikowski, S. Amarel, A. Safir, A model-based method for computer-aided medical decision-making, Artif. Intell., 11 (1978), 145–172. https://doi.org/10.1016/0004-3702(78)90015-2 doi: 10.1016/0004-3702(78)90015-2
![]() |
[95] |
E. Strickland, IBM Watson, heal thyself: How IBM overpromised and underdelivered on AI health care, IEEE Spectr., 56 (2019), 24–31. https://doi.org/10.1109/mspec.2019.8678513 doi: 10.1109/mspec.2019.8678513
![]() |
[96] |
M. Christ, F. Grossmann, D. Winter, R. Bingisser, E. Platz, Modern triage in the emergency department, Dtsch. Arztebl. Int., 107 (2010), 892–898. https://doi.org/10.3238/arztebl.2010.0892 doi: 10.3238/arztebl.2010.0892
![]() |
[97] |
C. I. Price, L. Shaw, S. Islam, M. Javanbakht, A. Watkins, P. McMeekin, et al., Effect of an enhanced paramedic acute stroke treatment assessment on thrombolysis delivery during emergency stroke care: A cluster randomized clinical trial, JAMA Neurol., 77 (2020), 840–848. https://doi.org/10.1001/jamaneurol.2020.0611 doi: 10.1001/jamaneurol.2020.0611
![]() |
[98] |
M. Kanglie, S. Bipat, I. A. H. van den Berk, T. S. R. van Engelen, M. G. W. Dijkgraaf, J. M. Prins, et al., OPTimal IMAging strategy in patients suspected of non-traumatic pulmonary disease at the emergency department: Chest X-ray or ultra-low-dose chest CT (OPTIMACT) trial-statistical analysis plan, Trials, 21 (2020), 407. https://doi.org/10.1186/s13063-020-04343-w doi: 10.1186/s13063-020-04343-w
![]() |
[99] |
G. Vingerhoets, N. Stroobant, Lateralization of cerebral blood flow velocity changes during cognitive tasks. A simultaneous bilateral transcranial Doppler study, Stroke, 30 (1999), 2152–2158. https://doi.org/10.1161/01.str.30.10.2152 doi: 10.1161/01.str.30.10.2152
![]() |
[100] |
T. Wessels, J. U. Harrer, C. Jacke, U. Janssens, C. Klö tzsch, The prognostic value of early transcranial Doppler ultrasound following cardiopulmonary resuscitation, Ultrasound Med. Biol., 32 (2006), 1845–1851. https://doi.org/10.1016/j.ultrasmedbio.2006.06.023 doi: 10.1016/j.ultrasmedbio.2006.06.023
![]() |
[101] |
A. Myrden, A. Kushki, E. Sejdić, T. Chau, Towards increased data transmission rate for a three-class metabolic brain-computer interface based on transcranial Doppler ultrasound, Neurosci. Lett., 528 (2012), 99–103. https://doi.org/10.1016/j.neulet.2012.09.030 doi: 10.1016/j.neulet.2012.09.030
![]() |
[102] |
A. Goyal, A. A. Samadani, A. M. Guerguerian, T. Chau, An online three-class Transcranial Doppler ultrasound brain computer interface, Neurosci. Res., 107 (2016), 47–56. https://doi.org/10.1016/j.neures.2015.12.013 doi: 10.1016/j.neures.2015.12.013
![]() |
[103] |
A. Khalaf, M. Sybeldon, E. Sejdic, M. Akcakaya, A brain-computer interface based on functional transcranial doppler ultrasound using wavelet transform and support vector machines, J. Neurosci. Methods, 293 (2018), 174–182. https://doi.org/10.1016/j.jneumeth.2017.10.003 doi: 10.1016/j.jneumeth.2017.10.003
![]() |
[104] |
S. Serhatlioğlu, F. Hardalaç, I. Güler, Classification of transcranial Doppler signals using artificial neural network, J. Med. Syst., 27 (2003), 205–214. https://doi.org/10.1023/a:1021821229512 doi: 10.1023/a:1021821229512
![]() |
[105] |
A. Ozturk, A. Arslan, F. Hardalac, Comparison of neuro-fuzzy systems for classification of transcranial Doppler signals with their chaotic invariant measures, Expert Syst. Appl., 34 (2008), 1044–1055. https://doi.org/10.1016/j.eswa.2006.12.006 doi: 10.1016/j.eswa.2006.12.006
![]() |
[106] |
H. Uğuz, A hybrid system based on information gain and principal component analysis for the classification of transcranial Doppler signals, Comput. Methods Prog. Biomed., 107 (2011), 598–609. https://doi.org/10.1016/j.cmpb.2011.03.013 doi: 10.1016/j.cmpb.2011.03.013
![]() |
[107] |
H. Uğuz, A. Arslan, A new approach based on discrete hidden Markov model using Rocchio algorithm for the diagnosis of the brain diseases, Digital Signal Process., 20 (2010), 923–934. https://doi.org/10.1016/j.dsp.2009.11.001 doi: 10.1016/j.dsp.2009.11.001
![]() |
[108] |
M. Seera, C. P. Lim, K. S. Tan, W. S. Liew, Classification of transcranial Doppler signals using individual and ensemble recurrent neural networks, Neurocomputing, 249 (2017), 337–344. https://doi.org/10.1016/j.neucom.2016.05.11 doi: 10.1016/j.neucom.2016.05.11
![]() |
[109] |
N. Karaboga, F. Latifoglu, Adaptive filtering noisy transcranial Doppler signal by using artificial bee colony algorithm, Eng. Appl. Artif. Intell., 26 (2013), 677–684. https://doi.org/10.1016/j.engappai.2012.10.011 doi: 10.1016/j.engappai.2012.10.011
![]() |
[110] |
N. Karaboga, F. Latifoglu, T. Koza, SSA analysis of transcranial Doppler signal using ⅡR filters designed with ABC algorithm, Curr. Opin. Biotechnol., 22 (2011), 5. https://doi.org/10.1016/j.copbio.2011.05.159 doi: 10.1016/j.copbio.2011.05.159
![]() |
[111] | B. Kamişlıoğlu, T. Koza, S. Koçkanat, N. Karaboğa, Noise cancellation on mitral valve Doppler signal with ⅡR digital filter using harmony search algorithm, in 2013 IEEE INISTA, (2013), 1–5. https://doi.org/10.1109/INISTA.2013.6577620 |
[112] |
K. Clare, A. Stein, N. Damodara, E. Feldstein, H. Alshammari, S. Ali, et al., Safety and efficacy of a novel robotic transcranial doppler system in subarachnoid hemorrhage, Sci. Rep., 12 (2022), 1–6. https://doi.org/10.1038/s41598-021-04751-1 doi: 10.1038/s41598-021-04751-1
![]() |
[113] |
T. Wang, Y. Chen, H. Du, Y. Liu, L. Zhang, M. Meng, Monitoring of neuroendocrine changes in acute stage of severe craniocerebral injury by transcranial Doppler ultrasound image features based on artificial intelligence algorithm, Comput. Math. Methods Med., 2021 (2021), 3584034. https://doi.org/10.1155/2021/3584034 doi: 10.1155/2021/3584034
![]() |
[114] | M. L. P. Portegies, P. J. Koudstaal, M. A. Ikram, Cerebrovascular disease, in Handbook of Clinical Neurology (eds M. J. Aminoff and F. Boller), (2016), 239–261. https://doi.org/10.1016/B978-0-12-802973-2.00014-8 |
[115] |
R. Capildeo, S. Haberman, F. C. Rose, The definition and classification of stroke: A new approach, QJM Int. J. Med., 47 (1978), 177–196. https://doi.org/10.1093/oxfordjournals.qjmed.a067535 doi: 10.1093/oxfordjournals.qjmed.a067535
![]() |
1. | Pavuluri Jaswanth, Pavuluri Yaswanth chowdary, M.V.S. Ramprasad, Deep learning based intelligent system for robust face spoofing detection using texture feature measurement, 2023, 29, 26659174, 100868, 10.1016/j.measen.2023.100868 | |
2. | Meetali Kalani, Ashish Anjankar, Revolutionizing Neurology: The Role of Artificial Intelligence in Advancing Diagnosis and Treatment, 2024, 2168-8184, 10.7759/cureus.61706 | |
3. | Na Yang, Qinghong Wang, Hongmei Qi, Zhen Song, Changjiang Zhou, Shengqiang Zhang, Bai Zhang, TCD-Guided management in carotid endarterectomy: a retrospective study, 2024, 19, 1749-8090, 10.1186/s13019-024-03069-z | |
4. | Wen-Juan Liu, The diagnosis of intracranial artery stenosis in patients with stroke by transcranial Doppler ultrasound: A meta-analysis, 2024, 32, 09287329, 639, 10.3233/THC-220844 | |
5. | Ho Tae JEONG, Soo Na JEON, Sol HAN, Manual of Transcranial Doppler Ultrasonography, 2024, 56, 1738-3544, 277, 10.15324/kjcls.2024.56.3.277 | |
6. | Yiqian Wang, Yang Zou, Zhou Li, Emerging intelligent wearable devices for cardiovascular health monitoring, 2024, 59, 17480132, 102544, 10.1016/j.nantod.2024.102544 | |
7. | Wangping Xiong, Yimin Zhu, Qingxia Zeng, Jianqiang Du, Kaiqi Wang, Jigen Luo, Ming Yang, Xian Zhou, Dose-effect relationship analysis of TCM based on deep Boltzmann machine and partial least squares, 2023, 20, 1551-0018, 14395, 10.3934/mbe.2023644 |