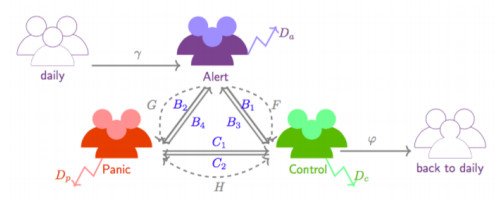
In a world the more and more affected by sudden, unpredictable natural and/or industrial disasters, with few or without warning signs, it is essential to understand, analyze and control population behaviors during such events.
Our objective is to model and investigate the actions that can be deployed by operational staff during catastrophic events in order to optimize risk management, reduce panic and save lives. For this purpose, we propose and solve an optimal control problem by using Pontryagin's Maximum Principle. Finally, we determine the best control strategy in the realistic scenario of a tsunami on the French Riviera.
Citation: Irmand Mikiela, Valentina Lanza, Nathalie Verdière, Damienne Provitolo. Optimal strategies to control human behaviors during a catastrophic event[J]. AIMS Mathematics, 2022, 7(10): 18450-18466. doi: 10.3934/math.20221015
[1] | Meroua Medjoudja, Mohammed El hadi Mezabia, Muhammad Bilal Riaz, Ahmed Boudaoui, Saif Ullah, Fuad A. Awwad . A novel computational fractional modeling approach for the global dynamics and optimal control strategies in mitigating Marburg infection. AIMS Mathematics, 2024, 9(5): 13159-13194. doi: 10.3934/math.2024642 |
[2] | Asma Hanif, Azhar Iqbal Kashif Butt . Atangana-Baleanu fractional dynamics of dengue fever with optimal control strategies. AIMS Mathematics, 2023, 8(7): 15499-15535. doi: 10.3934/math.2023791 |
[3] | Puntipa Pongsumpun, Jiraporn Lamwong, I-Ming Tang, Puntani Pongsumpun . A modified optimal control for the mathematical model of dengue virus with vaccination. AIMS Mathematics, 2023, 8(11): 27460-27487. doi: 10.3934/math.20231405 |
[4] | Houssine Zine, Abderrahim El Adraoui, Delfim F. M. Torres . Mathematical analysis, forecasting and optimal control of HIV/AIDS spatiotemporal transmission with a reaction diffusion SICA model. AIMS Mathematics, 2022, 7(9): 16519-16535. doi: 10.3934/math.2022904 |
[5] | Kai Zhang, Yunpeng Ji, Qiuwei Pan, Yumei Wei, Yong Ye, Hua Liu . Sensitivity analysis and optimal treatment control for a mathematical model of Human Papillomavirus infection. AIMS Mathematics, 2020, 5(3): 2646-2670. doi: 10.3934/math.2020172 |
[6] | Xin Yi, Rong Liu . An age-dependent hybrid system for optimal contraception control of vermin. AIMS Mathematics, 2025, 10(2): 2619-2633. doi: 10.3934/math.2025122 |
[7] | Le You, Chuandong Li, Xiaoyu Zhang, Zhilong He . Edge event-triggered control and state-constraint impulsive consensus for nonlinear multi-agent systems. AIMS Mathematics, 2020, 5(5): 4151-4167. doi: 10.3934/math.2020266 |
[8] | Linni Li, Jin-E Zhang . Input-to-state stability of nonlinear systems with delayed impulse based on event-triggered impulse control. AIMS Mathematics, 2024, 9(10): 26446-26461. doi: 10.3934/math.20241287 |
[9] | Chengbo Yi, Rui Guo, Jiayi Cai, Xiaohu Yan . Pinning synchronization of dynamical neural networks with hybrid delays via event-triggered impulsive control. AIMS Mathematics, 2023, 8(10): 25060-25078. doi: 10.3934/math.20231279 |
[10] | Huiling Li, Jin-E Zhang, Ailong Wu . Finite-time stabilization of nonlinear systems with partially known states via aperiodic intermittent control and event-triggered impulsive control. AIMS Mathematics, 2025, 10(2): 3269-3290. doi: 10.3934/math.2025152 |
In a world the more and more affected by sudden, unpredictable natural and/or industrial disasters, with few or without warning signs, it is essential to understand, analyze and control population behaviors during such events.
Our objective is to model and investigate the actions that can be deployed by operational staff during catastrophic events in order to optimize risk management, reduce panic and save lives. For this purpose, we propose and solve an optimal control problem by using Pontryagin's Maximum Principle. Finally, we determine the best control strategy in the realistic scenario of a tsunami on the French Riviera.
The last decades have been affected by a sharp increase in the number of disasters. Today, studies focusing on disaster management have made possible to put in place many tools that help to better manage crises. Therefore, small but encouraging improvements reducing the damages (human, material and environmental) associated with disasters can be seen. Nevertheless, there is still a significant ignorance about the human behaviors when faced with catastrophic events.
Over the past years, numerous studies have been conducted on crowd dynamics and flows. Several mathematical models characterizing crowd movements have been proposed with different approaches and at different scales: from social force models [4,28], to granular ones [15], from cellular automata models [14,21] to macroscopic ones [9,27]. However, these models do not take into account the different crowd behaviors or at most only panic reactions are considered. Moreover, they do not take into account the fact that a person can change his emotional state and therefore his behavior with time. Starting from 2014 few works exploiting the epidemic models framework to model the propagation of emotions in a population have appeared in literature. First of all, in 2014 and 2015 the Panic-Control-Reflex (PCR) model, based on cognitive sciences and mathematical modeling of infectious diseases, has been proposed to describe the dynamics of the different human behaviors during catastrophic events [3,18,24]. Another example concerns a SIRLS (Susceptible-Infected-Recovered-Latent-Susceptible) model developed for the propagation and control of panic emotions in a human population during emergencies [26]. Both the approaches highlight the importance of taking into account the phenomenon of emotion contagion in the modeling populations in disaster situations. However, the first one [3,18,24] does not focus only on panic, but it presents an accurate analysis and classification of the behaviors depending on the different brain regions that are activated and the different behavioral transitions. In this paper, we consider the Alert-Panic-Control (APC) model proposed and exploited in [11,12,23,25] that categorizes the different behaviors according to the emotional charge and its regulation.
In particular, here we propose an optimal control problem for the APC model. According to [20], several measures can be deployed by institutional staff in order to improve the resilience of populations. Here, we take into account the main three actions that decision-makers can take, that is population training, on site rescuers actions and institutional information. By solving the optimal control problem, we will infer which is the best strategy (or the combination of strategies) in order to control the panic development and the number of deaths during a catastrophic event. This work is one of the results of an interdisciplinary collaboration of mathematicians, geographers, psychologists, computer scientists, operational staff and stakeholders in risk management, within the ANR research project Com2SiCa (https://www.com2sica.cnrs.fr/).
The paper is organized as follows. In section 2, we present the APC model, a model proposed [11,12,25] to describe the dynamics of different human behaviors during a disasters. In section 3, we propose three different controls and we set up an optimal control problem. Then, we prove the existence of an optimal control and we characterize it by using Pontryagin's Maximum Principle [22]. Finally, in section 4, we present the numerical results about the best control strategy in the scenario of a sudden, unexpected, without warning signs event such as a tsunami on the beach on the French riviera.
In this section we briefly recall the Alert-Panic-Control (APC) model proposed in [11,12,25]. The APC model is a compartmental model inspired by the classical epidemic models such as the SIR (Susceptible-Infected-Recovered) one [10]. This model describes the temporal dynamics of several behavioral reactions during catastrophic events. Indeed, during a disaster, the concerned population exhibits many different behaviors [5,6,17,18]. According to the intensity of the emotional charge and the emotional regulation of this charge, the different observed behaviors have been grouped into 3 main classes: Alert, Panic and Control behaviors.
Therefore, the state variables of the APC model are the following:
● the density of individuals in an alert state a(t). This class of behaviors corresponds to all the behaviors such as the search for information, the identification of the danger etc. At the onset of the event all the people pass through a state of alert. The state of alert is characterized by a low emotional charge and a strong emotional regulation.
● the density of individuals in panic behaviors p(t). They correspond to all the behaviors with a high emotional charge that can be barely regulated, such as, for example, panic flight, freeze, trampling, pushing, etc. Collective panic is worried by operational actors since it can lead to potential high-risk situations. It can resolve spontaneously but sometimes an energetic external intervention is needed so that the panicked population regain a state of calm.
● the density of individuals exhibiting control behaviors c(t). Control behaviors, such as evacuation, mutual aid, search for help, etc., correspond to a strong emotional regulation of the emotional load, that can be more or less intense. The duration of the controlled behavior varies from a few minutes to several hours, depending on the intervention of the institutional and emergency actors.
In addition, we note q(t) the density of individuals in the everyday behaviors before the event. and with b(t) the density of population exhibiting behaviors of everyday life after the disaster. Finally, we note d(t) the density of individuals who lose their lives during the disaster.
The APC model scheme is represented in Figure 1 and the mathematical equations are the following:
˙a(t)=γ(t)q(t)−(B1+B2+Da)a(t)−F(a(t),c(t))a(t)c(t)−G(a(t),p(t))a(t)p(t)+B3c(t)+B4p(t),˙p(t)=B2a(t)+C2c(t)−(B4+C1+Dp)p(t)+G(a(t),p(t))a(t)p(t)−H(c(t),p(t))c(t)p(t),˙c(t)=B1a(t)+C1p(t)−(B3+C2+Dc)c(t)+F(a(t),c(t))a(t)c(t)+H(c(t),p(t))c(t)p(t)−φ(t)c(t),˙q(t)=−γ(t)q(t),˙b(t)=φ(t)c(t),˙d(t)=Daa(t)+Dcc(t)+Dpp(t). | (2.1) |
During a catastrophe, human beings do not adopt the same behavior during the all event but sequences of different reactions can be observed [5]. The transitions from one behavior to another in the model are of two types: intrinsic transitions and imitation-induced ones. Each individual experiences a behavioral evolution of his own. Typically, a state of alert is adopted as soon as the event occurs, then people adopt a panic behavior or a controlled one, depending on their past experiences and risk culture. The course of events can induce sometimes a momentary return to the alert state. These intrinsic transitions are modeled in the equations by linear terms and are represented in solid lines in Figure 1. Moreover, imitation or social comparison are important factors in behavioral changes processes, especially in dense populations, except for the alert behaviors that are not imitable [8]. To model the imitation phenomena, the dominant mass principle is used. Indeed, when populations with different behaviors meet, depending on the ratio among the populations, imitation transitions can take place. Therefore, these transitions are modeled by the following nonlinear functions:
F(a,c)=αξ(ca+ε),G(a,p)=βξ(pa+ε),H(c,p)=−Hc→p(c,p)+Hp→c(c,p), |
with
Hc→p(c,p)=γ1ξ(pc+ε),Hp→c(c,p)=γ2ξ(cp+ε), |
and
ξ(w)=w21+w2w∈R |
and ϵ<<1. Function ξ takes into account the fact that people have a tendency to imitate the most numerous behavior.
At the beginning all the population is supposed to be in a daily behavior q. Then, after the triggering of the catastrophe (represented here by a function γ), people pass through an alert state, before adopting, by an intrinsic reaction or an imitation process, a panic or a control behavior. Da, Dp and Dc are the mortality rates of the three main populations, respectively. Finally, a back to daily behavior can be achieved only being in a controlled behavior and only after a certain time. This transition is modeled by the function φ. For example, if we consider the impact zone in the case of unexpected disaster, functions γ and φ could be the following:
γ(t)=ζ(t,1,3) and φ(t)=ζ(t,40,70), | (2.2) |
with ζ defined as:
ζ(t,τ0,τ1)={0si t<τ012−12cos(t−τ0τ1−τ0π)si τ0≤t≤τ11si t>τ1. |
The choice of τ0=1 and τ1=3 for function γ means that we suppose that the population starts to be alerted at t=1 minute and at t=3 minutes the majority of the people has been impacted by the event and quitted their daily behavior. Furthermore, about the parameters in function φ, the choice τ0=40 means that pseudo-daily behaviors can be achieved only after 40 minutes from the onset of the event.
Remark 1. It is easy to notice that one equation is a linear combination of the other equations of the model. Indeed
∀ t≥t0,˙a(t)+˙p(t)+˙c(t)+˙q(t)+˙b(t)+˙d(t)=0, |
so we can consider a reduced model consisting only in five equations in terms of the state variables x=[a,p,c,q,d]T.
Since at the beginning all the population is supposed to be in a daily behavior q, we consider the following initial conditions:
x0=(0,0,0,1,0)T. | (2.3) |
Finally, all the model parameters can be represented by the following vector:
θ=(B1,B2,B3,B4,C1,C2,Da,Dc,Dp,α,β,γ1,γ2) ∈Θ=(R∗+)6×(R+)7 |
The definition of the parameters and the functions of the APC model are summarized in Tables 2 and 3 in the Appendix, respectively.
In this section we are interested in controls that could reduce the phenomenon of panic and limit the number of deaths during a catastrophic events. The following three controls are taken into account:
1. u1 represents the effect of the training and the experience of the population. This control acts on the intrinsic transition between alert and controlled behaviors, i.e. on the parameter B1. Therefore, we consider B1+u1 instead of B1 in system (2.1). Since B1+u1 is a transition rate, u1 is supposed to vary from 0 to 1.
2. u2 represents the effects of the actions of operational staff who are present and who intervene in the disaster impact zone. They have a calming effect throughout the event. This control favours imitation in the alert to control direction and in the panic to control direction. Therefore, this control acts in the same way on both transitions, that is on the imitation functions F and H. In particular, it acts on the parameter α to force the passage from alert to control. Thus, one will have α+u2 in the controlled system. Moreover, u2 also acts on the parameter γ2 to force the passage from panic to control. Thus, γ2 is substituted by γ2+u2 in the controlled system.
3. u3 reflects the effect of institutional information transmitted during the event, such as the transmission of a message or the triggering of a siren. This control can be taken into account in the mathematical model in the following manner. People will not act in the same way to the information transmitted during the event according to their experience. For example, people who have been trained on how to react to the arrival of a tsunami will adopt appropriate reactions when they hear the specific sound of the siren for this type of event. Thus, control u3 partially acts on the intrinsic transition between Alert-Control and we have B1+u1+ρu3∈[0,1] where ρ∈]0;1[. Moreover, u3 favors imitation in the alert to control and panic to control directions, acting on parameters α and γ2.
Thus, the controlled system reads as:
˙a(t)=γ(t)q−(B1+u1+ρu3+B2+Da)a(t)+B3c(t)+B4p(t)−˜F(a(t),c(t),u2,u3)a(t)c(t)−G(a(t),p(t))a(t)p(t),˙p(t)=B2a(t)+C2c(t)−(B4+C1+Dp)p(t)+G(a(t),p(t))a(t)p(t)−˜H(c(t),p(t),u2,u3)c(t)p(t), | (3.1) |
[2ex]˙c(t)=(B1+u1+ρu3)a(t)+C1p(t)−(B3+C2+Dc)c(t)+˜F(a(t),c(t),u2,u3)a(t)c(t)+˜H(c(t),p(t),u2,u3)c(t)p(t)−φ(t)c(t),˙q(t)=−γ(t)q(t),˙d(t)=Daa(t)+Dcc(t)+Dpp(t). | (3.2) |
Functions F and H defined in system (2.1) become
{˜F(a,c)=(α+u2+u3)×ξ(ca+ε)˜H(c,p)=−γ1×ξ(pc+ε)+(γ2+u2+u3)×ξ(cp+ε). | (3.3) |
In this section, we show that the Cauchy problem associated with system (3.1) is well posed. Hereinafter, we exploit the following notations:
UT={(u1,u2,u3)|uipiecewice continuous function,ai≤ui(t)≤bi,i=1,2,3,∀t∈[0,T]}, | (3.4) |
is the set of admissible controls, where ai and bi are constants in [0,1], I=[0,T], V=[0,1]5, x=(a,p,c,q,d)T∈V, u=(u1, u2, u3)∈UT. Therefore, system (3.1) can be rewritten as:
˙x(t)=f(t,x(t),u(t)), x(t0)=x0,t∈I, x∈V and u∈UT. | (3.5) |
Remark 2. UT⊂L∞loc(I;R3+), since the controls we have chosen are piecewise continuous functions on I.
Proposition 3. For any θ∈Θ and for any control u∈UT, the Cauchy problem associated to (3.1) admits a unique solution.
Proof. The application of Cauchy-Lipschitz Theorem [16] gives directly the existence and uniqueness of a solution of (3.1) with the initial conditions (2.3).
Proposition 4. For any control u∈UT, for any θ∈Θ and for any initial condition with positive or zero components, the components of the solution of system (3.1) are non negative.
Proposition 5. Let us consider the compact set
K={(a, p, c, q, d)∈(R+)5| a+p+c+q+d≤1}. |
Then, for any control u∈UT and for any parameter set θ∈Θ, the compact set K is positively invariant for the flow induced by system (3.1) with the initial conditions x0 = ( 0, 0, 0, 1, 0).
Proof. Let θ∈Θ and u∈UT. Let us note xu the solution of system (3.1) with the initial condition x0. Then, for t≥0, the components of xu satisfy:
˙a(t)+˙p(t)+˙c(t)+˙q(t)+˙d(t)=−φ(t)c(t)≤ 0. |
It yields that
a(t)+p(t)+c(t)+q(t)+d(t)≤ a0+p0+c0+q0+d0, |
that is
a(t)+p(t)+c(t)+q(t)+d(t)≤ 1. |
Our aim is to study an optimal control problem for system (3.1), by exploiting Pontryagin's maximum principle [22]. For this purpose, we define the following objective (cost) functional
J(u1,u2,u3)=∫T0Φ(u1,u2,u3)dt, | (3.6) |
with
Φ(u1,u2,u3)=Cpp+Cdd+A1u21+A2u22+A3u23. | (3.7) |
The two first terms in (3.6) represent the benefit of panic and deceased behaviors. Moreover, A1, A2 and A3 are positive constants that correspond to the weights that permit to regulate the costs of the controls for the formation, the actions of the rescuers and the external information, respectively.
Our objective is to reduce the panic phenomenon and limit the number of deaths, minimizing at the same time the cost of the controls, therefore we aim to find an optimal control u∗=(u∗1,u∗2,u∗3)∈UT such that
min(u1,u2,u3)∈UTJ(u1,u2,u3)=J(u∗1,u∗2,u∗3). | (3.8) |
The existence of this optimal control can be obtained by using a theorem by Fleming and Rishel [7]:
Theorem 6. Let us consider the control problem (3.1). There exists an optimal control u∗=(u∗1,u∗2,u∗3)∈UT such that
min(u1,u2,u3)∈UTJ(u1,u2,u3)=J(u∗1,u∗2,u∗3). | (3.9) |
Proof. In order to obtain the existence of an optimal control, the following conditions (Corollary 4.1, [7]) must be fulfilled:
1. the set of controls and the corresponding state variables is non empty.
In our case, the existence of solutions of system (3.1) with bounded coefficients given by Theorem 9.2.1 in [13] assure us that this condition is checked.
2. UT is convex and closed.
Our set of admissible controls UT given in (3.4) is convex and closed by definition, so this second condition is fulfilled.
3. the right hand side of system (3.1) is bounded by a linear function in the state and control.
In our case, the right hand side of (3.1) satisfies this condition since, as we have seen before, the state solutions are bounded.
4. Φ(u1,u2,u3) is convex on UT.
In our case, it is simple to notice that the integrand (3.7) of our objective functional is convex on UT.
5. Φ(u1,u2,u3)≥c1(|u1|2+|u2|2+|u3|2)β2−c2, for some c1>0 and β>1.
For u∈UT, we have:
|Φ(u1,u2,u3)|=|Cpp+Cdd+A1u21+A2u22+A3u23|≤|Cpp+Cdd|+|A1u21|+|A2u22|+|A3u23|≤|Cpp+Cdd|+max(A1,A2,A3)(|u21|+|u22|+|u23|)≤M+M(|u21|+|u22|+|u23|), |
where M=max{Cp+Cd,max(A1,A2,A3)} Since the solution of (3.1) is bounded and
|u21|+|u22|+|u23|≤3, |
then
|Φ(u)|≤ 4M≤ 4M+3−(|u1|2+|u2|2+|u3|2)≤c2−c1(|u1|2+|u2|2+|u3|2)β2, |
where c1=1, c2=4M+3 and β=2. Thus, this fifth condition is satisfied.
Once established that an optimal control exists, for minimizing the functional (3.6) subject to equations (3.1), Pontryagin's maximum principle [22] is exploited to derive necessary conditions for the determination of such optimal control.
Theorem 7. Given an optimal control u∗ and solutions of the corresponding state system (3.1), there exist adjoint variables λ=(λ1,λ2,λ3,λ4,λ5) satisfying:
˙λ1=(λ1−λ3)[B1+u1+ρu3]+(λ1−λ2)B2+(λ1−λ5)Da+(λ1−λ3)[∂˜F∂aac+˜Fc]+(λ1−λ2)[∂G∂aap+Gp],˙λ2=(λ2−λ1)B4+(λ2−λ5)Dp+(λ2−λ3)C1−Cp+(λ1−λ2)[∂G∂ppa+Ga]+(λ2−λ3)[∂˜H∂ppc+˜Hc],˙λ3=(λ3−λ1)B3+(λ3−λ2)C2+(λ3−λ5)Dc+λ3φ+(λ1−λ3)[∂˜F∂cac+˜Fa]+(λ2−λ3)[∂˜H∂cpc+˜Hp],˙λ4=(λ4−λ1)γ,˙λ5=−Cd | (3.10) |
with the terminal condition λi(T)=0 for i=1,…,5 and with the dependencies of the different variables and functions being omitted to lighten notations.
Furthermore, the optimal controls u1,u2 and u3 are represented by:
u1=max{0,min{b1θ,(λ1−λ32A1)a}},withb1θ=1−B1,u2=max{0,min{b2θ,(λ1−λ32A2)∂˜F∂u2×ac+(λ2−λ32A2)∂˜H∂u2×pc}},withb2θ=1−max(α,γ2),u3=max{0,min{b3θ,(λ1−λ32A3)×ρa+(λ1−λ32A3)∂˜F∂u3×ac+(λ2−λ32A3)∂˜H∂u3×pc}},withb3θ=min{b2θ−u2,b1θ−u1ρ} | (3.11) |
Proof. The form of the adjoint system and the terminal conditions follow from the application of Pontryagin's maximum principle [22].
In our case the Lagrangian L has the following form:
L(t,x,u,λ)=λ1(t)˙a(t)+λ2(t)˙p(t)+λ3(t)˙c(t)+λ4(t)˙q(t)+λ5(t)˙d(t)+Cpp(t)+Cdd(t)+A1u1(t)2+A2u2(t)2+A3u3(t)2 |
Therefore, the adjoint system (3.10) is deduced by differentiating the Lagrangian L with respect to states:
˙λ1=−∂L∂a,˙λ2=−∂L∂c,λ3=−∂L∂p,˙λ4=−∂L∂q,˙λ5=−∂L∂d. |
To obtain the optimal control, we also differentiate the Lagrangian L with respect to u=(u1,u2,u3) and set it equal to zero. Then we project the solution into UT, taking into account that the system parameters are transfer rates so they vary between 0 and 1.
In order to determine u∗, we start from a null control and we solve the state system (3.1). With the obtained solution, the adjoint system (3.10) is solved. Then, by exploiting the solutions of the state and adjoint systems, the control (3.11) is updated and compared with its previous value. We reiterate the algorithm until the norm of the difference of the two controls is as small as desired and convergence is achieved.
In the following, we will simulate a population concerned by a tsunami-type disaster on the beach of the city of Nice (France) [19]. A tsunami is supposed to be a sudden and unexpected event, without warning signs [1]. We model these hazard properties by choosing functions γ and φ as in (2.2). Therefore, the population starts to be alerted at t=1 minute and at t=3 minutes the majority of the people has been impacted by the event and left the compartment of daily behavior.
We consider two cases of population density: a dense or a sparse population, in order to take into account the different tourist pressures on mediterranean littoral (the beach is mainly populated in summer). According to [11,12], in the case of a sparse population, the intrinsic transitions are the most important ones and the coefficients of the imitation functions are chosen less than 0.5. On the contrary, for a dense population the coefficients of the imitation functions are greater than 0.5, since imitation processes are the most important ones. In both cases, we suppose a low risk culture population, that is, the most significant intrinsic transitions are the ones towards panic (B2>B1 and C2>C1).
To each scenario we can relate a set of parameters of the APC system of the form:
θ=(B1,B2,B3,B4,C1,C2,Da,Dc,Dp,α,β,γ1,γ2,ρ) ∈Θ=(R∗+)6×(R+)7×]0,1]. |
In this paper, we answer the following question: For a given scenario, which is the best strategy (management and/or prevention) to reduce panic and limit deaths?
As we have seen in the definition of the cost function
J=∫T0[Cpp(t)+Cdd(t)+A1u21+A2u22+A3u23]dt, | (4.1) |
to each control (u1,u2 and u3) corresponds a weight that we have noted A1, A2, and A3, respectively. For each scenario, a combination of these weights (ex: A1=0.01,A2=0.01,A3=1) defines a strategy, that is which are the most important factors in the cost function J. For modeling the different strategies, the following binary system is adopted: for i=1,2,3, we set
● Ai=0.01 if the control ui is supposed cheap (thus, the strategy mainly aims to increase efforts on control ui),
● Ai=1 if the control ui is supposed expensive. In this case, the strategy would aim to make the least possible effort on control ui.
We recall that u1 is the control concerning the formation of the population, u2 the one corresponding to the actions of operational staff on the scene of the disaster, and u3 the control taking into account communications. Thus, a management strategy mainly involving on site rescuers corresponds to the following triplet (A1=1, A2=0.01, A3=1), a prevention strategy mainly based on the education of the population corresponds to the triplet (A1=0.01, A2=1, A3=1) and a prevention strategy mainly based on communication corresponds to the triplet (A1=1, A2=1, A3=0.01). For both the scenarios (sparse or dense population), we test the eight strategies in Table 1.
strategy | A1 | A2 | A3 |
1 | 10−2 | 10−2 | 10−2 |
2 | 10−2 | 10−2 | 1 |
3 | 10−2 | 1 | 10−2 |
4 | 10−2 | 1 | 1 |
5 | 1 | 10−2 | 10−2 |
6 | 1 | 10−2 | 1 |
7 | 1 | 1 | 10−2 |
8 | 1 | 1 | 1 |
In the series of strategies, the strategy 1 will not be taken into account in determining the best strategy. It consists in a test strategy that allows us to observe the controls in the unrealistic situation where all the controls are equally cheap.
Being difficult to define the "costs" of a panic situation and of deaths, in all the simulations we set the weights Cp and Cd in (4.1) as Cp=Cd=1, respectively.
In order to determine which is the best strategy that reduces panic, we look at the strategy whose controls give us fewer people in a state of panic and whose objective function value is the smallest. We note:
IΔp=∫T0(p(t)−pc(t))dt | (4.2) |
where pc and p correspond to densities of individuals in a state of panic with and without control, respectively. This index IΔp allows us to judge the effectiveness of the control: the higher the value of IΔp is, the more effective the controls will be in reducing panic.
In a similar way we introduce the index that provides the measure of the effectiveness of death controls:
IΔd=∫T0(d(t)−dc(t))dt | (4.3) |
Therefore, for each scenario, the best strategy to reduce panic will correspond to the strategy with the highest IΔp index and the lowest value of the cost function J defined in (4.1).
Analogously, the best strategy to reduce deaths will correspond to the strategy with the highest IΔd index and the lowest value of the cost function J.
For the scenario of a sparse population with low risk culture, we choose the following set of parameters:
B1=0.1,B2=0.11,B3=10−3,B4=0.001,C1=0.1,C2=0.2,Da=Dc=10−4,Dp=1.5×10−5,α=β=γ1=γ2=0.2,ρ=0.5. | (4.4) |
According to [11,12], in the case of a sparse population, since the intrinsic transitions processes are the most important ones, the coefficients of the imitation functions are chosen between less than 0.5. Moreover, in order to model low risk culture, that is, most significant intrinsic transitions towards panic, we suppose B2>B1 and C2>C1.
The numerical simulations of the series of strategies in Table 1 and their results represented in Figure 2 show that the best strategy for reducing panic and deaths during the disaster is the number 2: (A1=10−2, A2=10−2, A3=1). Indeed, this strategy is the one with the highest values of IΔp and IΔd, combined with the lowest value of J. It is interesting to notice that this strategy consists in a mixed strategy combining management and prevention based on population training. However, if one prefers a strategy whose efforts are only on a single control at a time (according to Table 1, strategies n. 4, 6 and 7), the best strategy is the number 6 corresponding to the action of on site rescuers. In Figure 2, in order to have all the indices on the same graph, we had to multiply IΔd by one hundred.
In Figure 3 the optimal controls and the time evolution of the density of panic and deaths, with and without control, are represented. The controlled system exhibits a significant decrease of the density of panic and deaths with respect to the system without control. Here, only u1 and u2 are different to zero. Indeed, for strategy number 2, the control u3 is supposed more expensive than the others two (see Table 1). Moreover, control u1 is active mainly at the beginning of the event, while control u2 lasts till the population can go back to a daily behavior (see parameters of function φ in (2.2)).
For the scenario of a dense population with low risk culture, we choose the following set of parameters:
B1=0.05,B2=0.051,B3=B4=10−3,C1=0.05,C2=0.01,Da=Dc=10−4,Dp=1.5×10−5,α=β=γ1=γ2=0.7,ρ=0.5. | (4.5) |
In the case of a dense population, the imitation processes are the most important ones [11,12]. Therefore, here the coefficients of the imitation functions are greater than 0.5. It is worth noting that we have still the hypotheses B2>B1 and C2>C1, since we suppose a low risk culture level.
By testing all the strategies of Table 1, we find that in case of a dense population the best strategy to reduce panic and death (see Figure 4) corresponds once again to the strategy number 2: A1=10−2, A2=10−2, A3=1 combining the action of on site rescuers and population training. Figure 5 shows the optimal controls and the density of panic and deaths with and without control for such optimal strategy.
Moreover, also in this case the best strategy involving one control at a time is the strategy number 6 corresponding to the action of operational staff.
In conclusion, in both cases of sparse and dense populations, the best strategy is the one who combines population training and on site rescuers actions.
In this paper, an optimal control problem for a mathematical model describing the human behavior in case of a catastrophe is formulated. In particular, three different controls, modeling population training, on site rescuers actions and institutional information, have been proposed. The existence and the characterization of an optimal control have been carried out by exploiting the Pontryagin's maximum principle. Finally, several numerical simulations have been presented, taking into account different population scenarios in the case test of a tsunami on the French Riviera. Our results can be fruitful to improve strategies and methods to manage sudden and unexpected catastrophic events.
With the collaboration of the experts of human behavior and crisis management, we have identified the main controls that can have an effect on behavioral dynamics, we have thematically and mathematically defined them, and we have considered on which parameters and transitions they act. Our global approach (the integration of multidisciplinary and operational knowledge, the new mathematical modeling of behaviors during a disaster and the detailed and accurate discussion of the results) goes further with respect to previous works [26]. Our future purpose is to implement an optimal control problem on a spatial APC model, as [2] did for a network of PCR (Panic-Control-Reflex) models. This would permit to more deeply investigate the interactions between the spatial context and decision-makers actions. Moreover, a study of the effect of institutional measures in evacuation scenarios could be considered.
This work has been supported by the French government, through the National Research Agency (ANR) under the Societal Challenge 9 "Freedom and security of Europe, its citizens and residents" with the reference number ANR- 17-CE39-0008, co-financed by French Defence Procurement Agency (DGA) and The General Secretariat for Defence and National Security (SGDSN)
The authors declare that there is no conflicts of interest.
Tables 2 and 3 sum up the parameters and the functions of the APC model.
Parameters | Notation |
Intrinsic evolution from alert to control | B1 |
Intrinsic evolution from alert to panic | B2 |
Intrinsic evolution from control to alert | B3 |
Intrinsic evolution from panic to alert | B4 |
Intrinsic evolution from panic to control | C1 |
Intrinsic evolution from control to panic | C2 |
Mortality rates | Da, Dp, Dc |
Imitation from alert to control | α |
Imitation from alert to panic | β |
Imitation from control to panic | γ1 |
Imitation from panic to control | γ2 |
Functions | Notation |
Beginning of the catastrophe | γ(t) |
Leaving from the impact zone | φ(t) |
Imitation functions | F,G,H |
[1] | L. Boschetti, D. Provitolo, E. Tric, A method to analyze territory resilience to natural hazards, the example of the French Riviera against tsunami, EGU General Assembly Conference Abstracts, 19 (2017), 12935. |
[2] |
G. Cantin, N. Verdière, V. Lanza, Synchronization under control in complex networks for a panic model, International Conference on Computational Science, (2019), 262–275. https://doi.org/10.1007/978-3-030-22741-8_19 doi: 10.1007/978-3-030-22741-8_19
![]() |
[3] |
G. Cantin, N. Verdière, V. Lanza, M. Aziz-Alaoui, R. Charrier, C. Bertelle, et al., Mathematical modeling of human behaviors during catastrophic events: stability and bifurcations, Int. J. Bifurcat. Chaos, 26 (2016), 1630025. https://doi.org/10.1142/S0218127416300251 doi: 10.1142/S0218127416300251
![]() |
[4] |
F. E. Cornes, G. A. Frank, C. O. Dorso, Fear propagation and the evacuation dynamics, Simul. Model. Pract. Th., 95 (2019), 112–133. https://doi.org/10.1016/j.simpat.2019.04.012 doi: 10.1016/j.simpat.2019.04.012
![]() |
[5] | L. Crocq, Paniques Collectives (Les), Odile Jacob, 2013. |
[6] | E. Dubos-Paillard, A. Berred, D. Provitolo, Classification des catastrophes fondée sur l'analyse des relations entre les propriétés de l'événement et les comportements humains, Technical report, ANR, 2021. |
[7] | W. H. Fleming, R. W. Rishel, Deterministic and stochastic optimal control, vol. 1, Springer Science & Business Media, 2012. |
[8] |
D. T. Gilbert, R. B. Giesler, K. A. Morris, When comparisons arise., J. Pers. Soc. Psychol., 69 (1995), 227. https://doi.org/10.1037/0022-3514.69.2.227 doi: 10.1037/0022-3514.69.2.227
![]() |
[9] | D. Helbing, A. Johansson, Pedestrian, crowd, and evacuation dynamics, arXiv preprint arXiv: 1309.1609. |
[10] |
H. W. Hethcote, The mathematics of infectious diseases, SIAM review, 42 (2000), 599–653. https://doi.org/10.1137/S0036144500371907 doi: 10.1137/S0036144500371907
![]() |
[11] |
V. Lanza, E. Dubos-Paillard, R. Charrier, N. Verdière, D. Provitolo, O. Navarro, et al., Spatio-temporal dynamics of human behaviors during disasters: A mathematical and geographical approach, Complex Systems, Smart Territories and Mobility, (2021), 201–218. https://doi.org/10.1007/978-3-030-59302-5_11 doi: 10.1007/978-3-030-59302-5_11
![]() |
[12] | V. Lanza, D. Provitolo, N. Verdière, C. Bertelle, E. Dubos-Paillard, R. Charrier, et al., Modeling and analyse of the impact of risk culture on the human behavior during a catastrophic event, Submitted. |
[13] | D. L. Lukes, Differential equations: classical to controlled, Elsevier, 1982. |
[14] |
F. Martinez-Gil, M. Lozano, I. García-Fernández, F. Fernández, Modeling, evaluation and scale on artificial pedestrians: A literature review, ACM Comput. Surv., 50 (2017), 1–35. https://doi.org/10.1145/3117808 doi: 10.1145/3117808
![]() |
[15] |
B. Maury, J. Venel, A discrete contact model for crowd motion, ESAIM: Math. Model. Num., 45 (2011), 145–168. https://doi.org/10.1051/m2an/2010035 doi: 10.1051/m2an/2010035
![]() |
[16] | L. Perko, Differential equations and dynamical systems, Springer, New York, 3rd edn., 2001. https://doi.org/10.1007/978-1-4613-0003-8 |
[17] | D. Provitolo, E. Dubos-Paillard, J.-P. Müller, Emergent human behaviour during a disaster: Thematic versus complex systems approaches, European Conference on Complex System, (2011), 1–11. |
[18] |
D. Provitolo, E. Dubos-Paillard, N. Verdière, V. Lanza, R. Charrier, C. Bertelle, M. Aziz-Alaoui, Les comportements humains en situation de catastrophe: de l'observation à la modélisation conceptuelle et mathématique, Cybergeo: European Journal of Geography, (2015), 735. https://doi.org/10.4000/cybergeo.27150 doi: 10.4000/cybergeo.27150
![]() |
[19] | D. Provitolo, A. Tricot, A. Schleyer-Lindenmann, A.-H. Boudoukha, N. Verdière, S. Haule, et al., Saisir les comportements humains en situation de catastrophes : proposition d'une démarche méthodologique immersive, Cybergeo : European Journal of Geography, in press. |
[20] | M. Reghezza-Zitt, S. Rufat, Resilience imperative: uncertainty, risks and disasters, Elsevier, 2015. |
[21] |
W. Tong, L. Cheng, Simulation of pedestrian flow based on multi-agent, Procedia-Social and Behavioral Sciences, 96 (2013), 17–24. https://doi.org/10.1016/j.sbspro.2013.08.005 doi: 10.1016/j.sbspro.2013.08.005
![]() |
[22] |
E. Trélat, Optimal control and applications to aerospace: some results and challenges, J. Optimiz. Theory App., 154 (2012), 713–758. https://doi.org/10.1007/s10957-012-0050-5 doi: 10.1007/s10957-012-0050-5
![]() |
[23] | A. Tricot, D. Provitolo, E. Dubos-Paillard, Typologie synthétique des comportements humains lors de catastrophes, Technical report, ANR, 2020. |
[24] | N. Verdière, V. Lanza, R. Charrier, D. Provitolo, E. Dubos-Paillard, C. Bertelle, et al., Mathematical modeling of human behaviors during catastrophic events, 4th International Conference on Complex Systems and Applications (ICCSA2014), (2014), 67–74. |
[25] |
N. Verdière, O. Navarro, A. Naud, A. Berred, D. Provitolo, Towards parameter identification of a behavioral model from a virtual reality experiment, Mathematics, 9 (2021), 3175. https://doi.org/10.3390/math9243175 doi: 10.3390/math9243175
![]() |
[26] |
X. Wang, L. Zhang, Y. Lin, Y. Zhao, X. Hu, Computational models and optimal control strategies for emotion contagion in the human population in emergencies, Knowledge-Based Systems, 109 (2016), 35–47. https://doi.org/10.1016/j.knosys.2016.06.022 doi: 10.1016/j.knosys.2016.06.022
![]() |
[27] | V. Zachariadis, J. Amos, B. Kohn, Simulating pedestrian route-choice behavior under transient traffic conditions, in Pedestrian Behavior, Emerald Group Publishing Limited, 2009. https://doi.org/10.1108/9781848557512-006 |
[28] |
W. Zeng, H. Nakamura, P. Chen, A modified social force model for pedestrian behavior simulation at signalized crosswalks, Procedia-Social and Behavioral Sciences, 138 (2014), 521–530. https://doi.org/10.1016/j.sbspro.2014.07.233 doi: 10.1016/j.sbspro.2014.07.233
![]() |
1. | Marcello Pompa, Antonio Cerasa, Simona Panunzi, Andrea De Gaetano, HAPC Model of Crowd Behavior during Crises, 2023, 11, 2227-7390, 2711, 10.3390/math11122711 | |
2. | Valentina Lanza, Damienne Provitolo, Nathalie Verdière, Cyrille Bertelle, Edwige Dubos-Paillard, Oscar Navarro, Rodolphe Charrier, Irmand Mikiela, Moulay Aziz-Alaoui, Abdel Halim Boudoukha, Anne Tricot, Alexandra Schleyer-Lindenmann, Alexandre Berred, Sébastien Haule, Emmanuel Tric, Modeling and Analysis of the Impact of Risk Culture on Human Behavior during a Catastrophic Event, 2023, 15, 2071-1050, 11063, 10.3390/su151411063 |
strategy | A1 | A2 | A3 |
1 | 10−2 | 10−2 | 10−2 |
2 | 10−2 | 10−2 | 1 |
3 | 10−2 | 1 | 10−2 |
4 | 10−2 | 1 | 1 |
5 | 1 | 10−2 | 10−2 |
6 | 1 | 10−2 | 1 |
7 | 1 | 1 | 10−2 |
8 | 1 | 1 | 1 |
Parameters | Notation |
Intrinsic evolution from alert to control | B1 |
Intrinsic evolution from alert to panic | B2 |
Intrinsic evolution from control to alert | B3 |
Intrinsic evolution from panic to alert | B4 |
Intrinsic evolution from panic to control | C1 |
Intrinsic evolution from control to panic | C2 |
Mortality rates | Da, Dp, Dc |
Imitation from alert to control | α |
Imitation from alert to panic | β |
Imitation from control to panic | γ1 |
Imitation from panic to control | γ2 |
Functions | Notation |
Beginning of the catastrophe | γ(t) |
Leaving from the impact zone | φ(t) |
Imitation functions | F,G,H |
strategy | A1 | A2 | A3 |
1 | 10−2 | 10−2 | 10−2 |
2 | 10−2 | 10−2 | 1 |
3 | 10−2 | 1 | 10−2 |
4 | 10−2 | 1 | 1 |
5 | 1 | 10−2 | 10−2 |
6 | 1 | 10−2 | 1 |
7 | 1 | 1 | 10−2 |
8 | 1 | 1 | 1 |
Parameters | Notation |
Intrinsic evolution from alert to control | B1 |
Intrinsic evolution from alert to panic | B2 |
Intrinsic evolution from control to alert | B3 |
Intrinsic evolution from panic to alert | B4 |
Intrinsic evolution from panic to control | C1 |
Intrinsic evolution from control to panic | C2 |
Mortality rates | Da, Dp, Dc |
Imitation from alert to control | α |
Imitation from alert to panic | β |
Imitation from control to panic | γ1 |
Imitation from panic to control | γ2 |
Functions | Notation |
Beginning of the catastrophe | γ(t) |
Leaving from the impact zone | φ(t) |
Imitation functions | F,G,H |