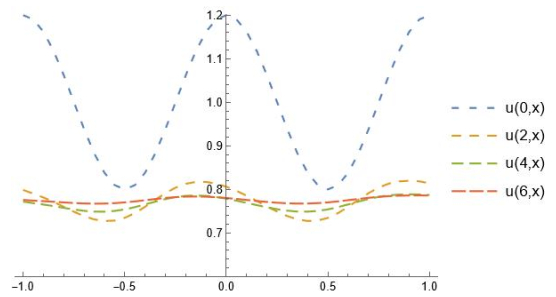
Anthropogenic modification of natural habitats is a growing threat to biodiversity and ecosystem services. The protection of biospecies has become increasingly important. Here, we pay attention to a single species as a conservation target. The species has three processes: reproduction, death and movement. Two different measures of habitat protection are introduced. One is partial protection in a single habitat (patch); the mortality rate of the species is reduced inside a rectangular area. The other is patch protection in a two-patch system, where only the mortality rate in a particular patch is reduced. For the one-patch system, we carry out computer simulations of a stochastic cellular automaton for a "contact process". Individual movements follow random walking. For the two-patch system, we assume an individual migrates into the empty cell in the destination patch. The reaction-diffusion equation (RDE) is derived, whereby the recently developed "swapping migration" is used. It is found that both measures are mostly effective for population persistence. However, comparing the results of the two measures revealed different behaviors. ⅰ) In the case of the one-patch system, the steady-state densities in protected areas are always higher than those in wild areas. However, in the two-patch system, we have found a paradox: the densities in protected areas can be lower than those in wild areas. ⅱ) In the two-patch system, we have found another paradox: the total density in both patches can be lower, even though the proportion of the protected area is larger. Both paradoxes clearly occur for the RDE with swapping migration.
Citation: Nariyuki Nakagiri, Hiroki Yokoi, Yukio Sakisaka, Kei-ichi Tainaka. Population persistence under two conservation measures: Paradox of habitat protection in a patchy environment[J]. Mathematical Biosciences and Engineering, 2022, 19(9): 9244-9257. doi: 10.3934/mbe.2022429
[1] | Wenjing An, Xingdong Zhang . An implicit fully discrete compact finite difference scheme for time fractional diffusion-wave equation. Electronic Research Archive, 2024, 32(1): 354-369. doi: 10.3934/era.2024017 |
[2] | Yunxia Niu, Chaoran Qi, Yao Zhang, Wahidullah Niazi . Numerical analysis and simulation of the compact difference scheme for the pseudo-parabolic Burgers' equation. Electronic Research Archive, 2025, 33(3): 1763-1791. doi: 10.3934/era.2025080 |
[3] | Shasha Bian, Yitong Pei, Boling Guo . Numerical simulation of a generalized nonlinear derivative Schrödinger equation. Electronic Research Archive, 2022, 30(8): 3130-3152. doi: 10.3934/era.2022159 |
[4] | Leilei Wei, Xiaojing Wei, Bo Tang . Numerical analysis of variable-order fractional KdV-Burgers-Kuramoto equation. Electronic Research Archive, 2022, 30(4): 1263-1281. doi: 10.3934/era.2022066 |
[5] | Chang Hou, Hu Chen . Stability and pointwise-in-time convergence analysis of a finite difference scheme for a 2D nonlinear multi-term subdiffusion equation. Electronic Research Archive, 2025, 33(3): 1476-1489. doi: 10.3934/era.2025069 |
[6] | Li Tian, Ziqiang Wang, Junying Cao . A high-order numerical scheme for right Caputo fractional differential equations with uniform accuracy. Electronic Research Archive, 2022, 30(10): 3825-3854. doi: 10.3934/era.2022195 |
[7] | Jun Liu, Yue Liu, Xiaoge Yu, Xiao Ye . An efficient numerical method based on QSC for multi-term variable-order time fractional mobile-immobile diffusion equation with Neumann boundary condition. Electronic Research Archive, 2025, 33(2): 642-666. doi: 10.3934/era.2025030 |
[8] | Yao Yu, Guanyu Xue . A nonlinear correction finite volume scheme preserving maximum principle for diffusion equations with anisotropic and discontinuous coefficient. Electronic Research Archive, 2025, 33(3): 1589-1609. doi: 10.3934/era.2025075 |
[9] | Junseok Kim . Maximum principle preserving the unconditionally stable method for the Allen–Cahn equation with a high-order potential. Electronic Research Archive, 2025, 33(1): 433-446. doi: 10.3934/era.2025021 |
[10] | Mingfa Fei, Wenhao Li, Yulian Yi . Numerical analysis of a fourth-order linearized difference method for nonlinear time-space fractional Ginzburg-Landau equation. Electronic Research Archive, 2022, 30(10): 3635-3659. doi: 10.3934/era.2022186 |
Anthropogenic modification of natural habitats is a growing threat to biodiversity and ecosystem services. The protection of biospecies has become increasingly important. Here, we pay attention to a single species as a conservation target. The species has three processes: reproduction, death and movement. Two different measures of habitat protection are introduced. One is partial protection in a single habitat (patch); the mortality rate of the species is reduced inside a rectangular area. The other is patch protection in a two-patch system, where only the mortality rate in a particular patch is reduced. For the one-patch system, we carry out computer simulations of a stochastic cellular automaton for a "contact process". Individual movements follow random walking. For the two-patch system, we assume an individual migrates into the empty cell in the destination patch. The reaction-diffusion equation (RDE) is derived, whereby the recently developed "swapping migration" is used. It is found that both measures are mostly effective for population persistence. However, comparing the results of the two measures revealed different behaviors. ⅰ) In the case of the one-patch system, the steady-state densities in protected areas are always higher than those in wild areas. However, in the two-patch system, we have found a paradox: the densities in protected areas can be lower than those in wild areas. ⅱ) In the two-patch system, we have found another paradox: the total density in both patches can be lower, even though the proportion of the protected area is larger. Both paradoxes clearly occur for the RDE with swapping migration.
In mathematical biology, predicting the time evolution of a biomass or a population over a spatial domain is a very important problem. Often, Lotka-Voterra-type equations are used to describe population dynamics, whether studying competition or predator-prey models. Such systems have been studied in many settings. Conditions under which two species can coexist have been treated theoretically. Numerical models have been developed that hope to mimic behavior of the system represented in the models. In the case that dispersion is occurring to each species, biomass or population studies over a domain ΩT=(0,T)×Ω for some Ω⊂Rd, generally with d=1,2, or 3 may take the general form
{ut=a1Δu+b1Lu+f1(u,v) in ΩTvt=a2Δv+b2Lv+f2(u,v) in ΩTu(0,x)=u0(x), v(0,x)=v0(x) in Ω | (1.1) |
where L is a linear operator on Ω, distinct from the Laplacian, that can take various forms. If a1,a2>0 in system (1.1), then some degree of local diffusion of each species is modeled by the equations. The b1=b2=0 case has been considered by multiple authors, such as in [1,2,3,4] and in their accompanying references.
In real world settings, populations in competition may demonstrate diffusion, dispersion, or some degree of both local and nonlocal behaviors. Competition for common resources may not only follow in their immediate neighborhood, but also in the entire spatial domain. In addition, this competition is not necessarily occurring only between individuals at the same location, but also between individuals at different locations; see [5] and references therein for an excellent motivation for and summary of models of nonlocal dispersion operators. Following similar reasoning, when b1=b2=1, the operator L given by
Lu(t,x)=∫ΩJ(x−y)u(t,y)dy−∫ΩJ(x−y) dy⋅u(t,x) | (1.2) |
has been motivated as an accurate reflection of dispersion between species for suitable J, and will be used here. The authors in [6] show the derivation of the nonlinear dispersion operator as J∗u−(J∗1)u, but then point out that it is unrealistic to use a convolution term to model biological species in bounded domains. Further, the restriction L in (1.2) of J∗u−(J∗1)u arises naturally in cases of hostile surroundings, a periodic environment, or under reflected boundary conditions. In [7], these assumptions are shown to be unnecessary, where the dispersion operator L in (1.2) is used to model nonlocal interaction. There, the authors show that for L, total internal energy is conserved and free energy decreases along trajectories so that L a suitable choice to reflect dispersion as a replacement for local dispersion modeled by the Laplacian for a symmetric interaction kernel J. Thus, operator L is also used both in nonlocal Allen-Cahn-type equations (c.f. [8]) or when describing population changes with nonlocal dispersion in biological systems (as in [9] and references therein).
Indeed, when restricted to ΩT⊂(0,T)×Rd, kernel function J in (1.2) becomes a measure of the probability that population members at all positions x affect those at y∈Rd, and vice-versa. Hence,
−ut(t,x)=−Lu(t,x) |
may be used to describe the rate at which members of a species are leaving x∈Ω at time t to travel to all other sites y∈Rd. Thus, for b1=b2=1, various forms of system (1.1) using L in (1.2) have also been studied in depth, including traveling wave solutions, spreading speed, stability of traveling waves, and of entire solutions as in [10,11,12], and references therein.
There has been a good deal of work on Lotka-Volterra models that include local diffusion, and more recently, nonlocal dispersion has been treated in such systems (see [1,2,3,4,13,14], along with references therein). In [15], an implicit approach to the numerical analysis of the system is introduced that mimics the dynamical properties of the true solution. In addition, it is proven that the scheme introduced there is uniquely solvable and unconditionally stable. The asymptotic behavior of the difference scheme is studied by constructing upper and lower solutions for the difference scheme. The convergence rate of the numerical solution to the true solution of the system is also given.
Following notation in (1.1) and (1.2) is
{ut=Lu+u(K1−u−av) in ΩTvt=Lu+v(K2−v−bu) in ΩTu(0,x)=u0(x), v(0,x)=v0(x) in Ω | (1.3) |
where Ω, ΩT, and L are defined previously. Here, u(t,x)≥0 and v(t,x)≥0 denote the population densities of the competing species for time t≥0 and x∈Ω⊂Rd for d=1,2. In addition, we assume that the system whose solutions describe the density of each species that began with (1.1) has been nondimensionalized, so that in (1.3), a,b,K1,K2>0 depend on the initial choice of constants in (1.1). To our knowledge, this system has not been analyzed with an unconditionally stable, nonstandard numerical method whose convergence rate can be given. This is the goal of this contribution.
As described previously, the system is used to model the two species competing with each other for the same prey, where both species are continuously distributed in time t throughout a region Ω, with each exhibiting free movement in the form of nonlocal dispersion. The method introduced to discretize (1.3) and the study of its properties will follow a similar development to the one used in [16], where properties of a single integro-differential equation that models an Ising spin system, with a convolution term that involves the Kac potential, are discussed in detail (see [17,18,19,20]).
In Section 2, we introduce the difference scheme used for the approximation of (1.3) over ΩT⊂(0,T)×R. We prove existence of the numerical solution to the scheme and that this solution is stable, independent of the choice of Δt and Δx. We give the convergence rate of the numerical scheme to the true solution. In Section 3, we present some results of numerical experiments that confirm the stability and convergence of the proposed difference scheme in one- and two-dimensional spatial domains Ω. In Section 4, we provide a summary of the results.
Analysis will be carried out over a domain Ω in one-dimensional space. All results carry over naturally to higher-dimensional space. For t>0 we introduce time step tk=kΔt for k=0,1,2,…, where Δt is of fixed size to be determined later. On the interval Ω=(−L,L)⊂R we define the partition
Ωx={xi|xi=−L+i△x,i=1,2,…,N−1}, where Δx=2L/N. |
Using uki and vki to represent the numerical approximation to the true solutions u and v to (1.3) at (tk,xi), our choice of difference scheme for n=1 in (1.3) is nonstandard to invoke desirable properties that will be established later, namely
{uk+1i−uki△t=(J∗uk)i−(J∗1)iuk+1i+K1uki−ukiuk+1i−auk+1ivkivk+1i−vki△t=(J∗vk)i−(J∗1)ivk+1i+K2vki−vkivk+1i−bukivk+1i | (2.1) |
for k=0,1,2,… and for 0≤i≤N. Throughout, for convenience, the discretization of Lu as given in (1.2) for (2.1) will be denoted by
(J∗uk)i=Δx[12J(x0−xi)uk0+N−1∑m=1J(xm−xi)ukm+12J(xN−xi)ukN]; |
a similar expression is used for Lv. We also introduce the initial conditions in (1.3) as
u0i=u0(xi) and v0i=v0(xi) |
for i=0,1,2,…,N, where for all i, u0(xi),v0(xi)≥0.
Solving (2.1) for uk+1i and vk+1i gives the iteration scheme for n=1 as
{uk+1i=(J∗uk)iΔt+(1+K1Δt)uki1+(J∗1)iΔt+ukiΔt+avkiΔtvk+1i=(J∗vk)iΔt+(1+K2Δt)vki1+(J∗1)iΔt+vkiΔt+bukiΔt | (2.2) |
for k=0,1,2,… and i=0,1,2,…,N.
Although we present and prove theorems for n=1, it is useful for programming numerical models to show the method of approximating (1.3) for n=2 as well. In this case, we choose Ω=(−L,L)×(−W,W)⊂R2, and partitions
Ωxy={(xi,yj)|xi=−L+i△x,yj=−W+j△y,0≤i≤M,0≤j≤N} |
and
Ωt={tk|tk=k△t,0≤t≤K}, |
where △x=2L/M and △y=2W/N.
The difference scheme for (1.3) includes
u0i,j=u0(xi,yj) and v0i,j=v0(xi,yj), | (2.3) |
together with
{uk+1i,j−uki,j△t=(J∗uk)i,j−(J∗1)i,juk+1i,j+K1uki,j−uki,juk+1i,j−auk+1i,jvki,jvk+1i,j−vki,j△t=(J∗vk)i,j−(J∗1)i,jvk+1i,j+K2vki,j−vki,jvk+1i,j−bvk+1i,juki,j | (2.4) |
all for i=0,1,…,M and j=0,1,…,N, where in (2.4),
(J∗uk)i,j=△x△y[M−1∑m=1N−1∑n=1J(xm−xi,yn−yj)ukm,n+12M−1∑m=1(J(xm−xi,y0−yj)ukm,0+J(xm−xi,yN−yj)ukm,N)+12N−1∑n=1(J(x0−xi,yn−yj)uk0,n+J(xM−xi,yn−yj)ukM,n)+14(J(x0−xi,y0−yj)uk0,0+J(xM−xi,y0−yj)ukM,0+J(x0−xi,yN−yj)uk0,N+J(xM−xi,yN−yj)ukM,N)]. |
From (2.3) and (2.4), we arrive at the explicit finite difference scheme
{[1+(uki,j+avki,j+(J∗1)i,j)Δt]uk+1i,j=[(J∗uk)i,j+K1uki,j]Δt+uki,j[1+(vki,j+buki,j+(J∗1)i,j)Δt]vk+1i,j=[(J∗vk)i,j+K2vki,j]Δt+vki,j | (2.5) |
for i=0,1,…,M, j=0,1,…,N, and k=0,1,2,….
Theorem 2.1. For n=1 and for the initial conditions in (1.3), let m1=maxu0(x), m2=maxv0(x), M1=max{K1,m1}, and M2=max{K2,m2}. Then for all k=0,1,2,… and for i=0,1,2,…,N,
0≤uki≤M1and0≤vki≤M2. | (2.6) |
Hence, the numerical scheme (2.2) is unconditionally nonnegative and unconditionally stable.
Proof. We proceed by induction. For k=0,
0≤u0i≤m1≤M1 and 0≤v0i≤m2≤M2 |
for i=0,1,2,…,N, so that (2.6) holds.
Assume now that (2.6) holds for some k∈N. Then for k+1,
uk+1i=(J∗uk)iΔt+(1+K1Δt)uki1+(J∗1)iΔt+ukiΔt+avkiΔt≤(J∗1)iM1Δt+(uki+K1ukiΔt)1+(J∗1)iΔt+ukiΔt+avkiΔt≤(J∗1)iM1Δt+(M1+M1ukiΔt)1+(J∗1)iΔt+ukiΔt+avkiΔt≤M1[(J∗1)iΔt+1+ukiΔt]1+(J∗1)iΔt+ukiΔt+avkiΔt≤ M1. |
Similarly, vk+1i≤M2, so the result holds for k+1 if it holds for k. Therefore, by mathematical induction, (2.6) is valid for all k=0,1,2… and for i=0,1,2,…,N.
We now turn to the question of convergence of the difference equations (2.2) to the true solution of (1.3).
Theorem 2.2. If u,v∈C1,2([0,T]ׯΩ) are solutions to (1.3), then the solution of (2.2) converges to u and v as Δt,Δx→0, uniformly on [0,T], with rate O(Δt+Δx2).
Proof. Let (u(t,x),v(t,x)) represent the solution pair to (1.3), where u,v∈C1,2([0,T]ׯΩ). Set
U0i=u0(xi), V0i=v0(xi),Uki=u(tk,xi), and Vki=v(tk,xi). |
We will prove the convergence claim based on u, then the same will follow for v by the symmetry of equations in (1.3). Let Δt=T/K, so that tk=kΔt for k=0,1,2,…,K. From (1.3) and (2.1) we have
Uk+1i−UkiΔt=(J∗Uk)i−(J∗1)iUki+K1Uki−(Uik)2−aUkiVki+Ru(Δt,Δx), | (2.7) |
where Ru is a function with Ru(Δt,Δx)=O(Δt+Δx2). Let
Xki=Uki−uki, Yki=Vki−vki |
for k=0,1,2,…,K and i=0,1,2,…,N. Then X0i=0, Y0i=0, for i=0,1,2,…,N. Using (2.1) in conjunction with (2.7),
Xk+1i=Uki−uki+Δt[(J∗Uk)i−(J∗uk)i−(J∗1)i(Uki−uki)−(J∗1)i(uk+1i−uki)]+Δt[K1(Uki−uki)−((Uki)2−ukiuk+1i)−a(UkiVki−uk+1ivki)]+ΔtRu(Δt,Δx), | (2.8) |
so that from (2.8),
|Xk+1i|≤|Uki−uki|+Δt|(J∗Uk)i−(J∗uk)i|+Δt|(J∗1)i(Uki−uki)|+Δt|(J∗1)i(uk+1i−uki)|+K1Δt|Uki−uki|+Δt|(Uki)2−ukiuk+1i|+aΔt|UkiVki−uk+1ivki|+ΔtRu(Δt,Δx). | (2.9) |
We turn to upper bounds on each of the terms in (2.9). To accomplish this, for each k, k=0,1,2,…,K, we will use Wku=maxi|Uki−uki| and Wkv=maxi|Vki−vki|. Setting C1=maxi(J∗1)i,
|(J∗Uk)i−(J∗uk)i)|≤Δx2J(x0−xi)|Uk0−uk0|+ΔxN−1∑m=1J(xm−xi)|Ukm−ukm|+Δx2J(xN−xi)|UkN−ukN|≤Δx[12J(x0−xi)+N−1∑m=1J(xm−xi)+12J(xN−xi)]Wku≤C1Wku, | (2.10) |
|(J∗1)i(Uki−uki)|=(J∗1)i|Uki−uki|≤C1Wku, |
and
|(J∗1)i(uk+1i−uki)|≤C1|uk+1i−uki|. |
Now, since |uki| and |vki| are uniformly bounded by M1 and M2, from (2.1),
|uk+1i−uki|≤C(M1,M2,C1)△t, |
where C(M1,M2,C1) is a constant that depends only on M1, M2, and C1. Hence there exists C2 such that
|(J∗1)i(uk+1i−uki)|≤C1|uk+1i−uki|≤C1C(M1,M2,C1)△t=C2△t |
and
K1|Uki−uki|≤K1Wku, |
where K1 is that constant related to carrying capacity in (1.3), so that for some constants C3, C4, and C5,
|(Uki)2−ukiuk+1i|=|UkiUki−Ukiuki+Ukiuki−uk+1iUki+uk+1iUki−ukiuk+1i|≤|UkiUki−Ukiuki|+|Ukiuki−uk+1iUki|+|uk+1iUki−ukiuk+1i|≤|Uki||Uki−uki|+|Uki||uki−uk+1i|+|uk+1i||Uki−uki|≤C3Wku+C3C(M1,M2,C1)△t+C(M1,M2,C1)Wku= C4△t+C5Wku, |
and thus there exist constants C6, C7, and C8 with
a|UkiVki−uk+1ivki|= a|UkiVki−Vkiuki+Vkiuki−uk+1iVki+uk+1iVki−uk+1ivki|≤ a(|Vki||Uki−uki|+|Vki||uki−uk+1i|+|uk+1i||Vki−vki|)≤ C6Wku+C7△t+C8Wkv. | (2.11) |
Substituting (2.10) and (2.11) into (2.9), we obtain
|Xk+1i|≤(1+C8△t)Wku+C9△tWkv+ΔtRu(Δt,Δx) | (2.12) |
for i=0,1,…,N, where C8 and C9 are constants independent of i and k, and where △t2-terms are absorbed into ΔtRu(Δt,Δx). Therefore, for each k, k=0,1,2,…,K,
Wk+1u≤(1+C8△t)Wku+C9△tWkv+ΔtRu(Δt,Δx). | (2.13) |
Similarly, there exist C10 and C11 with
Wk+1v≤(1+C10△t)Wkv+C11△tWku+ΔtRv(Δt,Δx), | (2.14) |
where, as with Ru, Rv is a function with Rv(Δt,Δx)=O(Δt+Δx2). Now setting Zk=Wku+Wkv, from (2.13) and (2.14), there exists a constant C0 with
Zk+1≤(1+C0△t)Zk+ΔtRu(Δt,Δx) | (2.15) |
for k=0,1,2,…,K. Set
D=1+C0Δt≥1. | (2.16) |
Then since Z0=0, using (2.15) and (2.16) and iterating,
Zk+1≤Dk+1Z0+[1+D+D2+…+Dk]ΔtRu(Δt,Δx)≤Dk+1−1C0△tΔtRu(Δt,Δx) |
for k=0,1,2,…,K−1. Since ex≥1+x, it follows that eKx≥(1+x)K, so that for all k, k=0,1,2,…,K−1, and again using D from (2.16),
Dk+1−1≤DK−1≤eC0K△t−1=eC0T−1. |
Thus, for k=0,1,2,…,K−1,
Zk+1≤(eC0T−1)Ru(Δt,Δx), |
so that Zk→0 for k=0,1,2,…,K as Δt→0, Δx→0. This completes the proof.
Remark. Similar results hold for n=2 in Theorems 2.1 and 2.2, and their proofs.
In this section we finish by presenting some results of computational experiments that verify the stability and convergence of the proposed difference scheme, confirming that the numerical solutions preserve the properties of the theoretical solution as well as those guaranteed by Theorem 2.2. Since there is no exact solution to compare with the approximation generated by the difference scheme, we use fix △x and compute for various △t values, then vice-versa. We compare the results in tables. We also present graphical results for dimensions n=1 in (2.2) and n=2 in (2.5).
CASEI. For n=1, we test method (2.1) for Ω=(−1,1), ϵ=0.1, a=0.4, b=0.6, K1=K2=1, u0(x)=0.2cos(2πx)+1, and v0(x)=0.3sin(2πx)+1, where J(x)=(ϵ√π)−1exp(−x2/ϵ2). We call these approximations (u(t,x),v(t,x)). Their convergence to steady state solutions is demonstrated in Figures 1 and 2 for Δt=Δx=0.05, as an example, since convergence is independent of time and space steps and graphs look much the same for any reasonable choices of small Δt and Δx.
CASEIA: Hold △x=0.05. Let (u(t,x),v(t,x)) denote the numerical solution under the parameters as chosen above corresponding to △t, while (u1(t,x),v1(t,x)) corresponds to △t1. Table 1 shows the maximum absolute errors, max|u(t,x)−u1(t,x)| and max|v(t,x)−v1(t,x)|, at t=5 across Ω.
△t | △t1 | max|u(5,x)−u1(5,x)| | max|v(5,x)−v1(5,x)| |
0.1 | 0.05 | 0.0040 | 0.00670 |
0.01 | 0.005 | 0.0004 | 0.00067 |
We note that the reduction of Δt by a factor of 0.5 reduces the error by O(Δt), as predicted by Theorem 2.2.
CASEIB: We fix △t=0.1 and vary △x. As before, we let (u(t,x),v(t,x)) represent the numerical solutions corresponding to △x, and let (u1(t,x),v1(t,x)) represent the numerical solutions corresponding to △x1. We compare the error differences max|u(t,x)−u1(t,x)| and max|v(t,x)−v1(t,x)| at t=5 across Ω.
△x | △x1 | max|u(5,x)−u1(5,x)| | max|v(5,x)−v1(5,x)| |
0.05 | 0.025 | 0.000181 | 0.000277 |
0.025 | 0.0125 | 0.000044 | 0.000068 |
We note that the reduction of Δx by a factor of 0.5 reduces the error by a factor of O(Δx2), or about 0.25, as stated in Theorem 2.2.
CASEII. For n=2, let Ω=(−1,1)×(−1,1), and let ϵ=0.1, a=0.4, b=0.6, u0(x,y)=0.4+0.2cos(2πx)cos(2πy), and v0(x,y)=0.5+0.3sin(2πx)sin(2πy), where Jϵ(x,y)=1ϵ2πexp(−x2+y2ϵ2).
We first show graphs of some approximate solutions generated by the two-dimensional method (2.5) for Δt=0.25, and Δx=Δy=0.2 in Figures 3 and 4. As in the one-dimensional case, since convergence is independent of time and space steps and graphs look much the same for any reasonable choices of small Δt and Δx, we have chosen these values as a representative of any such reasonable choice.
CASEIIA: We hold △x=△y=0.1 and compare accuracy for various △t-values in (2.5). Denote the numerical solution (u(t,x,y),v(t,x,y)) as the one generated by (2.5) corresponding to △t and (u1(t,x,y),v1(t,x,y)) corresponding to △t1. We compare the differences max|u(t,x,y)−u1(t,x,y)| and max|v(t,x,y)−v1(t,x,y)| at t=5 across Ω in Table 3.
△t | △t1 | max|u(5,x,y)−u1(5,x,y)| | max|v(5,x,y)−v1(5,x,y)| |
0.1 | 0.05 | 0.011975 | 0.009556 |
0.05 | 0.025 | 0.005879 | 0.004991 |
CASEIIB: Finally, we carry out the same accuracy test for fixed Δt=0.25 and various Δx=Δy and Δx1=Δy1 values at time t=5. The results are displayed in Table 4.
△x | △x1 | max|u(5,x,y)−u1(5,x,y)| | max|v(5,x,y)−v1(5,x,y)| |
0.1 | 0.05 | 0.0022898 | 0.0027063 |
0.05 | 0.025 | 0.0005250 | 0.0006352 |
All approximations in the tables show convergence at the rates predicted by Theorem 2.2.
The foregoing results have motivated the use of a Lotka-Volterra-type equation with operator L that reflects intra-species dispersion, or nonlocal interaction, with competition between species whose populations are given by u and v. A nonstandard numerical scheme was introduced that is stable, independent of the choice of time step, and that yields biologically sensible (nonnegative) numerical approximations to populations u and v of this system. Moreover, this nonstandard scheme was shown to be convergent to the solution of the proposed system and the order of convergence given. Because its convergence was established, it is possible to state with confidence that accurate solutions to the system are shown in the numerical experiments that were offered to confirm the results.
The authors declare they have not used Artificial Intelligence (AI) tools in the creation of this article.
The authors would like to thank the reviewers for their help and insightful suggestions for improvement of this paper.
The authors declare there are no conflicts of interest.
[1] | O. H. Frankel, M. E. Soule, Conservation and Evolution, Cambridge Univ. Press, Cambridge, 1981. |
[2] | E. O. Wilson, The Diversity of Life, Harvard Univ. Press, Cambridge, 1992. |
[3] |
K. L. Ryall, L. Fahrig, Habitat loss decreases predator-prey ratios in a pine-bark beetle system, Oikos, 110 (2005), 265–270. https://doi.org/10.1111/j.0030-1299.2005.13691.x doi: 10.1111/j.0030-1299.2005.13691.x
![]() |
[4] |
N. Nakagiri, K. Tainaka, J. Yoshimura, Bond and site percolation and habitat destruction in model ecosystems, J. Phys. Soc. Jpn., 74 (2005), 3163–3166. https://doi.org/10.1143/JPSJ.74.3163 doi: 10.1143/JPSJ.74.3163
![]() |
[5] |
N. Nakagiri, Y. Sakisaka, T. Togashi, S. Morita, K. Tainaka, Effects of habitat destruction in model ecosystems: Parity law depending on species richness, Ecol. Inform., 5 (2010), 241–247. https://doi.org/10.1016/j.ecoinf.2010.05.003 doi: 10.1016/j.ecoinf.2010.05.003
![]() |
[6] |
M. E. Gilpin, M. L. Rosenzweig, Enriched predator–prey systems: Theoretical stability, Science, 177 (1972), 902–904. https://doi.org/10.1126/science.177.4052.902 doi: 10.1126/science.177.4052.902
![]() |
[7] |
N. Nakagiri, K. Tainaka, T. Tao, Indirect relation between species extinction and habitat destruction, Ecol. Model., 137 (2001), 109–118. https://doi.org/10.1016/S0304-3800(00)00417-8 doi: 10.1016/S0304-3800(00)00417-8
![]() |
[8] |
P. P. Avelino, B. F. Oliveira, R. S. Trintin, Predominance of the weakest species in Lotka-Volterra and May-Leonard formulations of the rock-paper-scissors model, Phys. Rev. E, 100 (2019), 042209. https://link.aps.org/doi/10.1103/PhysRevE.100.042209 doi: 10.1103/PhysRevE.100.042209
![]() |
[9] |
K. Tainaka, N. Nakagiri, H. Yokoi, K. Sato, Multi-layered model for rock-paper-scissors game: A swarm intelligence sustains biodiversity, Ecol. Inform., 66 (2021), 101477. https://doi.org/10.1016/j.ecoinf.2021.101477 doi: 10.1016/j.ecoinf.2021.101477
![]() |
[10] |
N. Nakagiri, K. Tainaka, Indirect effects of habitat destruction in model ecosystems, Ecol. Model., 174 (2004), 103–114. https://doi.org/10.1016/j.ecolmodel.2003.12.047 doi: 10.1016/j.ecolmodel.2003.12.047
![]() |
[11] |
Y. Chen, S. J. Wright, H. C. Muller-Landau, S. P. Hubbell, Y. Wang, S. Yu, Positive effects of neighborhood complementarity on tree growth in a Neotropical forest, Ecology, 97 (2016), 776–785. https://doi.org/10.1890/15-0625.1 doi: 10.1890/15-0625.1
![]() |
[12] |
M. Perc, Does strong heterogeneity promote cooperation by group interactions?, New J. Phys., 13 (2011), 123027. https://doi.org/10.1088/1367-2630/13/12/123027 doi: 10.1088/1367-2630/13/12/123027
![]() |
[13] |
G. Szabó, G. Fáth, Evolutionary games on graphs, Phys. Rep., 446 (2007), 97–216. https://doi.org/10.1016/j.physrep.2007.04.004 doi: 10.1016/j.physrep.2007.04.004
![]() |
[14] |
A. Szolnoki, M. Perc, Evolutionary dynamics of cooperation in neutral populations, New J. Phy., 20 (2018), 013031. https://doi.org/10.1088/1367-2630/aa9fd2 doi: 10.1088/1367-2630/aa9fd2
![]() |
[15] |
K. Tainaka, Lattice model for the Lotka-Volterra system, J. Phys. Soc. Jpn., 57 (1988), 2588–2590. https://doi.org/10.1143/JPSJ.57.2588 doi: 10.1143/JPSJ.57.2588
![]() |
[16] |
K. Tainaka, Stationary pattern of vortices or strings in biological systems: lattice version of the Lotka-Volterra model, Phys. Rev. Lett., 63 (1989), 2688–2691. https://doi.org/10.1103/PhysRevLett.63.2688 doi: 10.1103/PhysRevLett.63.2688
![]() |
[17] |
T. E. Harris, Contact interaction on a lattice, Ann. Prob., 2 (1974), 969–988. https://doi.org/10.1214/aop/1176996493 doi: 10.1214/aop/1176996493
![]() |
[18] |
J. Tubay, H. Ito, T. Uehara, S. Kakishima, S. Morita, T. Togashi, et al., The paradox of enrichment in phytoplankton by induced competitive interactions, Sci. Rep., 3 (2013), 2835. https://doi.org/10.1038/srep02835 doi: 10.1038/srep02835
![]() |
[19] |
N. Nakagiri, K. Sato, Y. Sakisaka, K. Tainaka, Serious role of non-quarantined COVID-19 patients for random walk simulations, Sci. Rep., 12 (2022), 738. https://doi.org/10.1038/s41598-021-04629-2 doi: 10.1038/s41598-021-04629-2
![]() |
[20] |
T. Tahara, M. K. A. Gavina, T. Kawano, J. M. Tubay, J. F. Rabajante, H. Ito, et al., Asymptotic stability of a modified Lotka-Volterra model with small immigrations, Sci. Rep., 8 (2018), 7029. https://doi.org/10.1038/s41598-018-25436-2 doi: 10.1038/s41598-018-25436-2
![]() |
[21] | I. Hanski, Metapopulation Ecology, Oxford University Press, New York, 1999. |
[22] | I. Hanski, M. E. Gilpin, Metapopulation Biology: Ecology, Genetics, and Evolution, Academic Press, San Diego, 1997. |
[23] |
S. A. Levin, Dispersion and population interactions, Am. Nat., 108 (1974), 207–228. https://doi.org/10.1086/282900 doi: 10.1086/282900
![]() |
[24] |
K. M. A. Kabir, J. Tanimoto, Analysis of epidemic outbreaks in two-layer networks with different structures for information spreading and disease diffusion, Commun. Nonlinear Sci. Numer. Simul., 72 (2019), 565–574. https://doi.org/10.1016/j.cnsns.2019.01.020 doi: 10.1016/j.cnsns.2019.01.020
![]() |
[25] |
K. M. A. Kabir, J. Tanimoto, Evolutionary vaccination game approach in metapopulation migration model with information spreading on different graphs, Chaos Solitons Fractals, 120 (2019), 41–55. https://doi.org/10.1016/j.chaos.2019.01.013 doi: 10.1016/j.chaos.2019.01.013
![]() |
[26] |
T. Nagatani, G. Ichinose, K. Tainaka, Heterogeneous network promotes species coexistence: metapopulation model for rock-paper-scissors game, Sci. Rep., 8 (2018), 7094. https://doi.org/10.1038/s41598-018-25353-4 doi: 10.1038/s41598-018-25353-4
![]() |
[27] |
A. Sadykov, K. D. Farnsworth, Model of two competing populations in two habitats with migration: Application to optimal marine protected area size, Theor. Popul. Biol., 142 (2021), 114–122. https://doi.org/10.1016/j.tpb.2021.10.002 doi: 10.1016/j.tpb.2021.10.002
![]() |
[28] |
H. Yokoi, K. Tainaka, K. Sato, Metapopulation model for a prey-predator system: Nonlinear migration due to the finite capacities of patches, J. Theor. Biol., 477 (2019), 24–35. https://doi.org/10.1016/j.jtbi.2019.05.021 doi: 10.1016/j.jtbi.2019.05.021
![]() |
[29] |
H. Yokoi, K. Tainaka, N. Nakagiri, K. Sato, Self-organized habitat segregation in an ambush-predator system: Nonlinear migration of prey between two patches with finite capacities, Ecol. Inform., 55 (2020), 101022. https://doi.org/10.1016/j.ecoinf.2019.101022 doi: 10.1016/j.ecoinf.2019.101022
![]() |
[30] | T. M. Liggett, Interacting Particle Systems, Springer, New York, 1985. https://doi.org/10.1007/978-1-4613-8542-4 |
[31] | J. Marro, R. Dickman, Nonequilibrium Phase Transition in Lattice Models, Cambridge University Press, Cambridge, 1999. https://doi.org/10.1017/CBO9780511524288 |
[32] | N. Konno, Phase Transition of Interacting Particle Systems, World Scientific, Singapore, 1991. |
[33] |
M. Katori, N. Konno, Upper bounds for survival probability of the contact process, J. Stat. Phys., 63 (1991), 115–130. https://doi.org/10.1007/BF01026595 doi: 10.1007/BF01026595
![]() |
[34] |
W. Cota, A. S. Mata, S. C. Ferreira, Robustness and fragility of the susceptible-infected-susceptible epidemic models on complex networks, Phys. Rev. E, 98 (2018), 012310. https://doi.org/10.1103/PhysRevE.98.012310 doi: 10.1103/PhysRevE.98.012310
![]() |
[35] |
T. Gross, C. J. D'Lima, B. Blasius, Epidemic dynamics on an adaptive network, Phys. Rev. Lett., 96 (2006), 208701. https://doi.org/10.1103/PhysRevLett.96.208701 doi: 10.1103/PhysRevLett.96.208701
![]() |
[36] |
J. Peterson, The contact process on the complete graph with random vertex-dependent infection rates, Stoch. Proc. Their Appl., 121 (2011), 609–629. https://doi.org/10.1016/j.spa.2010.11.003 doi: 10.1016/j.spa.2010.11.003
![]() |
[37] |
Y. Harada, H. Ezoe, Y. Iwasa, H. Matsuda, K. Sato, Population persistence and spatially limited social interaction, Theor. Popul. Biol., 48 (1995), 65–91. https://doi.org/10.1006/tpbi.1995.1022 doi: 10.1006/tpbi.1995.1022
![]() |
[38] |
K. Tainaka, N. Nakagiri, Segregation in an interacting particle system, Phys. Lett. A, 271 (2000), 92–99. https://doi.org/10.1016/S0375-9601(00)00302-9 doi: 10.1016/S0375-9601(00)00302-9
![]() |
[39] |
J. M. Tubay, J. Yoshimura, Resistance of a terrestrial plant community to local microhabitat changes, Ecol. Evol., 8 (2018), 5101–5110. https://doi.org/10.1002/ece3.4093 doi: 10.1002/ece3.4093
![]() |
[40] |
X. Zou, K. Wang, A robustness analysis of biological population models with protection zone, Appl. Math. Model., 35 (2011), 5553–5563. https://doi.org/10.1016/j.apm.2011.05.020 doi: 10.1016/j.apm.2011.05.020
![]() |
[41] |
F. Wei, C. Wang, Survival analysis of a single-species population model with fluctuations and migrations between patches, Appl. Math. Model., 81 (2020), 113–127. https://doi.org/10.1016/j.apm.2019.12.023 doi: 10.1016/j.apm.2019.12.023
![]() |
△t | △t1 | max|u(5,x)−u1(5,x)| | max|v(5,x)−v1(5,x)| |
0.1 | 0.05 | 0.0040 | 0.00670 |
0.01 | 0.005 | 0.0004 | 0.00067 |
△x | △x1 | max|u(5,x)−u1(5,x)| | max|v(5,x)−v1(5,x)| |
0.05 | 0.025 | 0.000181 | 0.000277 |
0.025 | 0.0125 | 0.000044 | 0.000068 |
△t | △t1 | max|u(5,x,y)−u1(5,x,y)| | max|v(5,x,y)−v1(5,x,y)| |
0.1 | 0.05 | 0.011975 | 0.009556 |
0.05 | 0.025 | 0.005879 | 0.004991 |
△x | △x1 | max|u(5,x,y)−u1(5,x,y)| | max|v(5,x,y)−v1(5,x,y)| |
0.1 | 0.05 | 0.0022898 | 0.0027063 |
0.05 | 0.025 | 0.0005250 | 0.0006352 |
△t | △t1 | max|u(5,x)−u1(5,x)| | max|v(5,x)−v1(5,x)| |
0.1 | 0.05 | 0.0040 | 0.00670 |
0.01 | 0.005 | 0.0004 | 0.00067 |
△x | △x1 | max|u(5,x)−u1(5,x)| | max|v(5,x)−v1(5,x)| |
0.05 | 0.025 | 0.000181 | 0.000277 |
0.025 | 0.0125 | 0.000044 | 0.000068 |
△t | △t1 | max|u(5,x,y)−u1(5,x,y)| | max|v(5,x,y)−v1(5,x,y)| |
0.1 | 0.05 | 0.011975 | 0.009556 |
0.05 | 0.025 | 0.005879 | 0.004991 |
△x | △x1 | max|u(5,x,y)−u1(5,x,y)| | max|v(5,x,y)−v1(5,x,y)| |
0.1 | 0.05 | 0.0022898 | 0.0027063 |
0.05 | 0.025 | 0.0005250 | 0.0006352 |