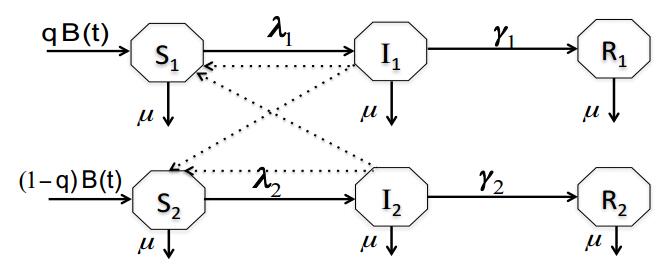
Liquid crystals are a typical type of soft matter that are intermediate between conventional crystalline solids and isotropic fluids. The nematic phase is the simplest liquid crystal phase, and has been studied the most in the mathematical community. There are various continuum models to describe liquid crystals of nematic type, and Q-tensor theory is one among them. The aim of this paper is to give a brief review of recent PDE results regarding the Q-tensor theory in dynamic configurations.
Citation: Xiang Xu. Recent analytic development of the dynamic Q-tensor theory for nematic liquid crystals[J]. Electronic Research Archive, 2022, 30(6): 2220-2246. doi: 10.3934/era.2022113
[1] | Vladimir Rovenski . Willmore-type variational problem for foliated hypersurfaces. Electronic Research Archive, 2024, 32(6): 4025-4042. doi: 10.3934/era.2024181 |
[2] | Yuchen Zhu . Blow-up of solutions for a time fractional biharmonic equation with exponentional nonlinear memory. Electronic Research Archive, 2024, 32(11): 5988-6007. doi: 10.3934/era.2024278 |
[3] | Liu Yang, Yuehuan Zhu . Second main theorem for holomorphic curves on annuli with arbitrary families of hypersurfaces. Electronic Research Archive, 2024, 32(2): 1365-1379. doi: 10.3934/era.2024063 |
[4] | Youngjin Hwang, Ildoo Kim, Soobin Kwak, Seokjun Ham, Sangkwon Kim, Junseok Kim . Unconditionally stable monte carlo simulation for solving the multi-dimensional Allen–Cahn equation. Electronic Research Archive, 2023, 31(8): 5104-5123. doi: 10.3934/era.2023261 |
[5] | Fanqi Zeng . Some almost-Schur type inequalities and applications on sub-static manifolds. Electronic Research Archive, 2022, 30(8): 2860-2870. doi: 10.3934/era.2022145 |
[6] | Fabrizio Catanese, Luca Cesarano . Canonical maps of general hypersurfaces in Abelian varieties. Electronic Research Archive, 2021, 29(6): 4315-4325. doi: 10.3934/era.2021087 |
[7] | Yong Zhou, Jia Wei He, Ahmed Alsaedi, Bashir Ahmad . The well-posedness for semilinear time fractional wave equations on RN. Electronic Research Archive, 2022, 30(8): 2981-3003. doi: 10.3934/era.2022151 |
[8] | Jincheng Shi, Shuman Li, Cuntao Xiao, Yan Liu . Spatial behavior for the quasi-static heat conduction within the second gradient of type Ⅲ. Electronic Research Archive, 2024, 32(11): 6235-6257. doi: 10.3934/era.2024290 |
[9] | Bangna Li, Qingqing Zhang, Xingshi He . Multi-label feature selection via constraint mapping space regularization. Electronic Research Archive, 2024, 32(4): 2598-2620. doi: 10.3934/era.2024118 |
[10] | Qinlong Chen, Wei Cao . The number of rational points on a class of hypersurfaces in quadratic extensions of finite fields. Electronic Research Archive, 2023, 31(7): 4303-4312. doi: 10.3934/era.2023219 |
Liquid crystals are a typical type of soft matter that are intermediate between conventional crystalline solids and isotropic fluids. The nematic phase is the simplest liquid crystal phase, and has been studied the most in the mathematical community. There are various continuum models to describe liquid crystals of nematic type, and Q-tensor theory is one among them. The aim of this paper is to give a brief review of recent PDE results regarding the Q-tensor theory in dynamic configurations.
Influenza A is a highly contagious respiratory viral disease caused by influenza viruses. It is sometimes called "the flu". It can easily spread from person to another by direct contact, where an infected individual can spread it to others up to about 6 feet away. The virus spreads by droplets made by infected individuals when they cough, sneeze or talk. Such droplets may land in the mouth or the nose of nearby susceptible people or they could even be inhaled by them to enter their lungs, which finally lead them to catch the flu [1,2]. Among the three types of influenza (namely, influenza A, influenza B and influenza C), influenza A is the most common one that is known to cause widespread outbreaks. It has many subtypes of which the two subtypes H1N1 and H3N2 are mostly circulating among humans [1,3].
Due to its ability to rapidly evolve and mutate, seasonal flu epidemics and (rarely) pandemics do occur. Such epidemics and pandemics impact significantly on the public health as well as on the economy over the globe. For example, in the united states, seasonal influenza infections are responsible for thousands of deaths and for hundreds of thousands of hospitalisations every year [4]. It is also responsible for the loss of billions of dollars yearly, as a cost of treatment or due to lost days of work and/or education. Consequently, public health communities are always interested in finding not only strategies to mitigate the impact of seasonal influenza but also strategies aiming at reducing morbidity and mortality in the case of pandemics [3,5].
Numerous studies have been done to understand more about the epidemiology of influenza. In particular, mathematical models have been extensively used to extend our understanding about the transmission dynamics of influenza and to help find public health strategies aiming at preventing/containing it [6]. Some models are used to study the epidemic situation of influenza A, where its severity is explored by estimating the (basic) reproduction number (a threshold quantity that is defined to determine the average number of secondary infected individuals who get infected due to successful contacts occurring between susceptible individuals and an infected one, during the infectious period) based on data collected during outbreaks [7,8,9]. Other models study the endemic situation, where the demographic parameters (births and natural deaths) are taken into account [4,10,11].
The general motivation behind these studies is to help find strategies to contain influenza A. Some strategies are individually-based (in the sense that individuals are aware of the way influenza is transmitted and they avoid catching it) and others are based on intervention programmes (e.g., isolation/quarantine or vaccination) directed by the governments and public health decision makers. For example, some control strategies are based on treating infected individuals by either resting in bed, drinking plenty of liquids or taking mild pain killers to relieve the pain. Other strategies may be educational, based on reducing the successful contacts through staying at home while being sick, covering the face when sneezing or coughing, and regularly washing the hands. Isolating or quarantining infected individuals is another strategy [4,12]. Also, applying mass vaccination programmes could be helpful [13]. Influenza vaccine is particularly helpful for those suffering from influenza complications or those being at high risk of getting infected with the flu.
A review paper on influenza epidemics and pandemics [6] discussed the usefulness of mathematical models in understanding the spatial-temporal transmission dynamics of influenza and in helping evaluate the potential effectiveness of public health interventions in controlling pandemics of varying severity. The review deduced that "the current models may not be useful in identifying interventions for epidemics generated by strains" (e.g., influenza A H1N1) and recommends that further studies taking into account more biological complexities are needed. One of such complexities is the sex and gender disparity.
The terms sex and gender are often used interchangeably [14]. However, the literature shows that they refer to different meanings. The term sex refers to the human biology, while the term gender refers to the human behaviour and activities that are determined by the societies or the cultures [15]. The biology literature shows that the gender can affect the health seeking behaviours, while the biological sex affects the exposure to pathogens, vulnerability and immune response to influenza and consequently to vaccination [15,16,17,18,19]. All these effects together result in differences between males and females in response to influenza-vaccination-campaigns as well as the susceptibility, severity and duration of influenza. Moreover, biological studies show that females are less susceptible to influenza than males (as they develop higher innate, humoral and cellular immune responses than males), while the influenza-vaccine efficacy is higher in females than in males [20,21,22,23]. On the other hand, the influenza-vaccine uptake is influenced by the gender, where there is evidence that males are more likely to take the vaccine than females [20]. More details about sex and gender differences and their relation to influenza are shown in the July 2010 WHO report [14]. It is noteworthy that the report strikingly highlights that the role of sex and gender in acute infectious diseases, including influenza, has not been explored extensively and further work that takes these factors in consideration is lacked so that a more effective public health influenza control programme is produced.
To the best of our knowledge, neither the sex nor the gender has been considered while formulating a mathematical model for studying the transmission dynamics of respiratory infections (e.g., influenza) and this is the first work that takes these factors into consideration. Here, the impact of sex and gender on the transmission dynamics and possibility to contain influenza A infection is explored. To this end, we constructed a deterministic SIR influenza model in which it is differentiated between both females and males through two sex-difference factors (namely, the susceptibility and recovery rates), see section 2. In addition to carrying out equilibrium and stability analysis, the impact of disparities in these parameters on the influenza infection outcome has been explored, section 3. Moreover, the possibility to contain/eliminate influenza with a public health strategy based on the application of influenza-mass-vaccination programme solely has been investigated. Therefore, the model has been generalised to take into account disparity in the influenza-vaccine efficacy (that is sex-difference factor) and in the response to influenza-mass vaccination campaigns (i.e, the vaccination rate, which is a gender behavioural difference factor), section 4. The paper closes with a summary and conclusion of our results in section 5.
Consider a closed homogeneously mixing heterosexual population of constant size N, for which both the female and male subpopulations are splitted, according to their epidemiological status, into susceptible S, infected I and recovered with full immunity R. State variables for the female population are denoted by the subscript "1", while those of the male population have the subscript "2". If B(t) is the total number of births at time t, q is the probability that a newborn is female, while μ is the per-capita death rate, then the demographic dynamics of the populations could be described by
dNdt=B(t)−μN,dN1dt=qB(t)−μN1,dN2dt=(1−q)B(t)−μN2 | (2.1) |
where N1(t) and N2(t) denote the total number of females and males, respectively, at time t and N1(t)+N2(t)=N(t). Since the total population size is assumed constant, then B(t)=μN. If we further assume that the male-to-female newborns ratio is 1- to - 1, then q=1/2. This assumption implies that the equilibrium proportion of females as well as males is 1/2.
As influenza is not vertically transmitted, it is assumed that all newbirths are susceptible. Female newborns (whose number at time t is μN/2) enter the class of susceptible females S1(t). They either die naturally (at per-capita rate μ) or acquire influenza infection due to successful contacts with infected males and females at rate λ1(t) and transit to the infected females class. Infected females either die at per-capita rate μ or recover and acquire full immunity against the attacking influenza strain at a per-capita rate γ1. Once they recover, females acquire full immunity against the strain and die naturally at rate μ. Therefore, the dynamics of the female population is governed by the system
dS1dt=12μN−(λ1+μ)S1,dI1dt=λ1S1−(γ1+μ)I1,dR1dt=γ1I1−μR1. | (2.2) |
Similarly, susceptible males increase due to male newbirths (of size μN/2) and decrease due to either natural death (at rate μ) or acquiring influenza infection at rate λ2. Infected males either die at rate μ or they recover from the attacking strain at rate γ2. Recovered males acquire full immunity against the attacking strain and die naturally at rate μ. A flowchart for the transition between the model states is shown in Figure 1, while model states and parameters' physical meaning are shown in Tables 1 and 2, respectively. Hence, the male population's dynamics is described through
dS2dt=12μN−(λ2+μ)S2,dI2dt=λ2S2−(γ2+μ)I2,dR2dt=γ2I2−μR2. | (2.3) |
State variable | Description |
x1=S1/N | Proportion of susceptible females at time t. |
y1=I1/N | Proportion of infected females at time t. |
z1=R1/N | Proportion of recovered females at time t. |
x2=S2/N | Proportion of susceptible males at time t. |
y2=I2/N | Proportion of infected males at time t. |
z2=R2/N | Proportion of recovered males at time t. |
N1(t) | Total female population size at time t. |
N2(t) | Total male population size at time t. |
N=N1+N2 | Total population size at time t, assumed to be constant. |
Parameters | Description | Value | Dim. | Ref. |
B(t) | Total number of newborns. | – | Time−1 | – |
μ | Per-capita death rate. | 1/70 | Year−1 | [4] |
ˉβ | Per-capita contact rate between individuals | – | Year−1 | – |
β | Per-capita effective contact rate at which susceptible males acquire influenza. | 181.173 | Year−1 | Est. |
γ | Per-capita recovery rate for infected males. | 365/3.38 | Year−1 | [24] |
R0 | The basic reproduction number for model (2.6). | 1.525 | – | [24] |
ψ | Per-capita vaccination rate for susceptible males. | 0.57 | Year−1 | Arbit. |
r1 | The susceptibility of females. | – | – | – |
r2 | The susceptibility of males. | – | – | – |
g=r1/r2 | Relative susceptibility of females with respect to males. | 0.9<1 | – | Arbit.[13] |
a | A rescaling parameter representing the relative recovery rate of infected females with respect to infected males. | 1.1 | – | Arbit. |
e0 | Relative response to vaccination campaigns of females with respect to males. | 0.9 | – | Arbit.[20] |
e1(>e2) | Influenza vaccine efficacy in females. | 0.9 | – | Arbit.[20] |
e2 | Influenza vaccine efficacy in males. | 0.8 | – | Arbit.[20] |
Assume now that ˜β denotes the average number of contacts that an individual has with other individuals in the total population. Also, r1 is the susceptibility of females (which is proportional to the probability of success that a susceptible female gets infected due to contacts with infected males and females [25]), while r2 is the susceptibility of males (which is proportional to the probability that a susceptible male gets successfully infected as a result of contacts with infected individuals [25]). Assume also that rij accounts for the transmissibility of influenza from infected individuals in the population i to susceptible individuals in the population j, for all i,j∈{1,2}. Hence, the rate at which susceptible females acquire infection λ1(t) and that at which susceptible males acquire infection read
λ1(t)=r1˜β(r11I1N+r21I2N)andλ2(t)=r2˜β(r12I1N+r22I2N). | (2.4) |
Assume further that infected males and females are equally likely to transmit influenza, in the sense that the effective rate at which infected females infect susceptible males/females equals that at which infected males infect susceptible males/females (i.e., r11=r12=r21=r22:=r). Hence, β=r2r˜β:=r2ˉβ accounts for the effective contact rate at which susceptible males acquire influenza and r1r˜β=(r1/r2)r2ˉβ:=gβ is the effective contact rate at which susceptible females acquire influenza virus. Here, g=r1/r2 accounts for the relative susceptibility [26,27] of females with respect to males. Hence, (2.4) reads
λ1(t)=gβ(I1N+I2N):=gλ(t)andλ2(t)=β(I1N+I2N):=λ(t). | (2.5) |
The recovery rates could also be rescaled by assuming that γ2=γ and γ1=aγ. Hence, a is a dimensionless parameter accounting for the relative recoverability of females with respect to males. Thus, the populations' overall dynamics is described by the following system of ordinary differential equations
dS1dt = 12μN−(gλ+μ)S1,dS2dt = 12μN−(λ+μ)S2,dI1dt = gλS1−(aγ+μ)I1,dI2dt = λS2−(γ+μ)I2,dR1dt = aγI1−μR1,dR2dt = γI2−μR2. | (2.6) |
It is noteworthy that this modelling approach could be applied to several other infectious diseases, including but not limited to tuberculosis (TB), pertussis (whooping cough) and human immunodeficiency virus (HIV) infection, where the literature shows evidence that sex and gender have significant impact on their spread. For example, in most countries, TB notification is twice as high in men as in women (with a male/female ratio of 1.96±0.6 for the worldwide case notification rate) [28,29]. However, in case of pertussis, females have been shown to be more affected than males with male-to-female ratio of 0.8:1 [30]. On the other hand, although the proportion of females living with HIV is about 51 of the global total, it varies from region to another. For example, in the United States, one in four people having HIV is a woman. However, women account for a proportion of 56 of those having HIV in Western and Central Africa. Moreover, in Eastern and Southern Africa, females account for more than 59 of the total number of people having HIV in that region [31,32,33].
Let
x1=S1N,y1=I1N,z1=R1N,x2=S2N,y2=I2N,z2=R2N. |
Hence,
dx1dt = 12μ−(gλ+μ)x1,dx2dt = 12μ−(λ+μ)x2,dy1dt = gλx1−(aγ+μ)y1,dy2dt = λx2−(γ+μ)y2,dz1dt = aγy1−μz1,dz2dt = γy2−μz2, | (3.1) |
where the rescaled force of infection λ is a function of both variables y1 and y2 with
λ(y1,y2)=β(y1+y2) | (3.2) |
and x1+y1+z1+x2+y2+z2=1.
If we assume that p1=N1/N=x1+y1+z1 and p2=N2/N=x2+y2+z2, then the rate of change in the proportion of females and males at time t is given by
dp1dt=12μ−μp1,dp2dt=12μ−μp2. | (3.3) |
Its solution is given by
p1(t)=12[1−(1−2p1(0))e−μt],p2(t)=12[1−(1−2p2(0))e−μt], | (3.4) |
where p1(0) and p2(0) are the initial proportions of females and males, respectively, and p1(0)+p2(0)=1. It is easy to check that the fact that p1(t)+p2(t)=1 implies that p1(0)=p2(0)=1/2. Thus, p1(t)=p2(t)=1/2. I.e., the female-to-male ratio at any time t is 1:1. Hence, x1(t)+y1(t)+z1(t)=x2(t)+y2(t)+z2(t)=1/2.
Simple computations show that model (3.1) has the influenza-free equilibrium E0=(x1,0,y1,0,z1,0,x2,0,y2,0,z2,0)T=(1/2,0,0,1/2,0,0)T, where the prime T here means vector transpose.
The basic reproduction number is computed by following the approach shown in Diekmann et al [34]. The linearised infection subsystem, at the influenza-free equilibrium, is
dy1dt=12gβ(y1+y2)−(aγ+μ)y1,dy2dt=12β(y1+y2)−(γ+μ)y2, |
which is two-dimensional. Hence, the corresponding non-negative matrix for the new-infection terms T and the non-singular matrix for the remaining transfer terms Σ are two-dimensional too, with
T=(gβ/2gβ/2β/2β/2)andΣ=(−(aγ+μ)00−(γ+μ)). |
Therefore, the next-generation matrix with large domain KL=−TΣ−1 reads
KL=(gβ/2(aγ+μ)gβ/2(γ+μ)β/2(aγ+μ)β/2(γ+μ)). |
Thus, the basic reproduction number R0 is the dominant eigenvalue of KL and is given by
R0=β2(1μ+γ+gμ+aγ). | (3.5) |
The local stability analysis of the influenza-free equilibrium E0 is investigated by considering the eigenvalues of the corresponding Jacobian matrix J0 evaluated at E0. The computations show that J0 has four multiple eigenvalues −μ, −μ, −μ, −μ in addition to those of the matrix
Jsub=(gβ/2−(aγ+μ)gβ/2β/2β/2−(γ+μ)). |
Hence, the local stability of E0 depends on the determinant and trace of the matrix Jsub. Now,
det(Jsub)=(γ+μ)(aγ+μ)(1−R0). |
Hence, det(Jsub)>0 if and only if R0<1. Moreover, if R0<1, then β/2<γ+μ and gβ/2<aγ+μ. Hence, tr(Jsub)=[β/2−(γ+μ)]+[gβ/2−(aγ+μ)]<0. Thus, E0 is locally asymptotically stable for R0<1 and we show the following proposition.
Proposition 1. Model (3.1) has an influenza-free equilibrium E0=(1/2,0,0,1/2,0,0)T that is locally asymptotically stable if and only if the basic reproduction number R0 is less than unity.
To find the endemic equilibrium of system (3.1), we put the derivatives in the left hand side of its equations equal zero and solve to get
x1=μ2(gˉλ+μ),y1=μ2(aγ+μ)gˉλ(gˉλ+μ),z1=aγ2(aγ+μ)gˉλ(gˉλ+μ), | (3.6) |
x2=μ2(ˉλ+μ),y2=μ2(γ+μ)ˉλ(ˉλ+μ),z2=γ2(γ+μ)ˉλ(ˉλ+μ) | (3.7) |
where ˉλ∈[0,∞) is the equilibrium force of infection and is the unique feasible solution of the equation
1=βμ2(1(γ+μ)(ˉλ+μ)+g(aγ+μ)(gˉλ+μ)). | (3.8) |
It is clear that the equilibrium proportion of the subpopulations depend on the equilibrium force of infection ˉλ which is determined by the solution of equation (3.8). Once a solution of equation (3.8) is obtained, we get the endemic equilibrium of the model (3.1). The uniqueness of the feasible solution of equation (3.8) could be proven by rewriting it as
H1(ˉλ)=:1−βμ2(γ+μ)(ˉλ+μ)=gβμ2(aγ+μ)(gˉλ+μ):=H2(ˉλ). | (3.9) |
It is easy to check the following
H1(0)=1−β2(γ+μ),H2(0)=gβ2(aγ+μ) |
and
dH1dˉλ=βμ2(γ+μ)(ˉλ+μ)2>0,dH2dˉλ=−g2βμ2(aγ+μ)(gˉλ+μ)2<0. |
Hence, equation (3.9) has a unique nonnegative solution if and only if H1(0)≤H2(0), while otherwise it has no feasible solution, see Figure 2. The condition H1(0)≤H2(0) is equivalent to R0≥1 and we show the following proposition.
Proposition 2. Model (3.1) has a unique endemic equilibrium if and only if the basic reproduction number R0>1, while otherwise it has no endemic steady state.
To find the unique feasible solution of equation (3.8), we rewrite it in the quadratic form
A2ˉλ2+A1ˉλ+A0=0 | (3.10) |
where
A2=2g(γ+μ)(aγ+μ),A1=μ[2(1+g)(γ+μ)(aγ+μ)−gβ((aγ+μ)+(γ+μ))],A0=μ2[2(γ+μ)(aγ+μ)−β(aγ+μ+g(γ+μ))]. |
Hence,
ˉλ=μ2{R0(1+(1−g)(γ+μ)aγ+μ+g(γ+μ))−(1+1g)+√(R0(1+(1−g)(γ+μ)aγ+μ+g(γ+μ))−(1+1g))2+4g(R0−1)}. | (3.11) |
Since x1+y1+z1=x2+y2+z2=1/2 and both z1 and z2 do not appear in the other equations, we consider the four equations of x1,y1,x2 and y2 only. Hence, the Jacobian matrix evaluated at the endemic equilibrium, for these four equations, reads
J=(−(gˉλ+μ)−gβμ2(gˉλ+μ)0−gβμ2(gˉλ+μ)gˉλgβμ2(gˉλ+μ)−(aγ+μ)0gβμ2(gˉλ+μ)0−βμ2(ˉλ+μ)−(ˉλ+μ)−βμ2(ˉλ+μ)0βμ2(ˉλ+μ)ˉλβμ2(ˉλ+μ)−(γ+μ)). |
If ρ is the eigenvalue of J, then the characteristic polynomial of J is of degree four and reads
ρ4+C1ρ3+C2ρ2+C3ρ+C4=0 | (3.12) |
where
● the coefficient of ρ3 is
C1=(gˉλ+μ)+(aγ+μ)−gβμ2(gˉλ+μ)+(ˉλ+μ)+(γ+μ)−βμ2(ˉλ+μ). |
Using (3.9), we get
C1=(ˉλ+μ)+(gˉλ+μ)+βμ(aγ+μ)2(ˉλ+μ)(γ+μ)+gβμ(γ+μ)2(gˉλ+μ)(aγ+μ). | (3.13) |
● the coefficient of ρ2 is
C2=(ˉλ+μ)(γ+μ)+(gˉλ+μ)(aγ+μ)−βμ22(ˉλ+μ)−gβμ22(gˉλ+μ)+((gˉλ+μ)+(aγ+μ)−gβμ2(gˉλ+μ))((ˉλ+μ)+(γ+μ)−βμ2(ˉλ+μ))−gβ2μ24(ˉλ+μ)(gˉλ+μ). |
Using (3.9), we obtain
C2=(gˉλ+2μ)(γ+μ)gβμ2(gˉλ+μ)(aγ+μ)+(ˉλ+2μ)(aγ+μ)βμ2(ˉλ+μ)(γ+μ)+ˉλ(γ+μ)+gˉλ(aγ+μ)+(ˉλ+μ)(gˉλ+μ). | (3.14) |
● the coefficient of ρ is
C3=((ˉλ+μ)+(γ+μ)−βμ2(ˉλ+μ))((gˉλ+μ)(aγ+μ)−gβμ22(gˉλ+μ))+((gˉλ+μ)+(aγ+μ)−gβμ2(gˉλ+μ))((ˉλ+μ)(γ+μ)−βμ22(ˉλ+μ))−gβ2μ32(ˉλ+μ)(gˉλ+μ). |
Using (3.9), it simplifies to
C3=ˉλ(γ+μ)(gˉλ+μ)+gˉλ(aγ+μ)(ˉλ+μ)+gˉλ(γ+μ)gβμ2(gˉλ+μ)+ˉλ(aγ+μ)βμ2(ˉλ+μ)+βμ2(aγ+μ)2(γ+μ)+gβμ2(γ+μ)2(aγ+μ). | (3.15) |
● the term-free of ρ reads
C4=((ˉλ+μ)(γ+μ)−βμ22(ˉλ+μ))((gˉλ+μ)(aγ+μ)−gβμ22(gˉλ+μ))−gβ2μ44(ˉλ+μ)(gˉλ+μ) |
which, using (3.9), simplifies to
C4=βμˉλ(aγ+μ)(gˉλ+μ)2(ˉλ+μ)+g2βμˉλ(γ+μ)(ˉλ+μ)2(gˉλ+μ). | (3.16) |
Since, all model parameters are non-negative and since ˉλ is positive for R0>1, then the relations (3.13), (3.14), (3.15), and (3.16) imply that the coefficients C1,C2,C3 and C4 are all positive, for R0>1. Hence, based on the Routh-Hurwitz criterion (see appendix A.5 of [35]), the roots of the characteristic polynomial (3.12) have strictly negative real parts if and only if the determinant Δ=C1C2C3−C23−C21C4 is strictly positive. To this end, we compute Δ. On performing some tedious calculations and with the use of the relation (3.9), it is shown in appendix A that Δ>0. We show the following proposition.
Proposition 3. The unique endemic equilibrium of model (3.1) is locally asymptotically stable whenever it exists.
Global stability analysis of the disease-free equilibrium (for R0<1) is established by following the approach shown in section 3 of Castillo-Chavez et al [36]. Model (3.1) can be rewritten as
dxdt=F(x,y),dydt=G(x,y) | (3.17) |
where
x=(x1z1x2z2),y=(y1y2) |
and
F(x,y)=(μ/2−(gλ+μ)x1aγy1−μz1μ/2−(λ+μ)x2γy2−μz2),G(x,y)=(gλx1−(aγ+μ)y1λx2−(γ+μ)y2). |
The components of x∈R4 denote the uninfected (susceptible) subpopulations, while those of y∈R2 denote the infected subpopulations. The disease-free equilibrium (DFE) of model (3.17) is U0=(x∗,0)T which corresponds to the DFE of the original model (3.1). Thus, the global stability of U0 is equivalent to that of E0 and vice-versa. Castillo-Chavez et al [36] showed that the global stability of U0 (provided that R0<1) is guaranteed if the following two conditions are held
(H1) For dxdt=F(x,0), x∗ is globally asymptotically stable (g. a. s.),
(H2) G(x,y)=By−ˆG(x,y),ˆG(x,y)≥0 for (x,ˉy)∈Ω,
where B=DyG(x∗,0) is an M-matrix and Ω={(x1,z1,x2,z2,y1,y2):x1+z1+x2+z2+y1+y2=1,x1>0,x2>0,z1≥0,z2≥0,y1≥0,y2≥0} is the region where model (3.17) makes biological sense. To this end, we prove each condition separately. Condition (H1) is proven by considering the following Lyapunov function
V(x1,z1,x2,z2)=x1−x∗1−x∗1lnx1x∗1+x2−x∗2−x∗2lnx2x∗2+z1+z2. | (3.18) |
Clearly, V(x1,z1,x2,z2)≥0 along the solution of the system
ddt(x1z1x2z2)=(μ/2−μx1−μz1μ/2−μx2−μz2) | (3.19) |
and is zero if and only if
(x1z1x2z2)=(x∗1z∗1x∗2z∗2)=(1/201/20). |
The time derivative of the Lyapunov function V(x1,z1,x2,z2), computed along the solutions of system (3.19), is
˙V=−μ{x1(1−12x1)2+(1−12x2)2+z1+z2}. | (3.20) |
Thus, ˙V<0 so that x∗ is globally asymptotically stable for the system (3.19).
Condition (H2) is proven by rewriting the vector matrix G(x,y) in the form By−ˆG(x,y), where
B=DyG(x∗,0)=(gβ/2−(aγ+μ)gβ/2β/2β/2−(γ+μ)) |
is an M-matrix (as the off-diagonal elements are non-negative) and
ˆG(x,y)=(g(1/2−x1)1/2−x2)λ≥0. |
Thus, the disease-free equilibrium U0=(x∗,0)T for model (3.17) is globally asymptotically stable for R0<1 and we have shown the following proposition.
Proposition 4. The influenza-free equilibrium E0 is globally asymptotically stable if and only if the basic reproduction number R0<1.
In this subsection we study the impact of sex disparity on the overall outcome of influenza. More specifically, we study how the basic reproduction number R0 and the equilibrium force of infection ˉλ depend on the variance in susceptibilities r1,r2 (of females and males, respectively) and in recovery rates if their means are kept constant. Before going into details, we study the qualitative behaviour of R0,ˉλ,¯y1 and ¯y2 on the relative susceptibility g. Formula (3.5) shows clearly that the basic reproduction number R0 increases monotonically with the increase of the relative susceptibility g, if all other parameters are kept constant. However, numerical simulations have been done to show the dependence of ¯y1 and ¯y2 on g and R0. Figure 3 shows the qualitative behaviour of the endemic prevalence of both infected females ¯y1 (see part(a)) and infected males ¯y2 (see part (b)). For fixed g, both the endemic prevalence of infected females and males increase monotonically with the increase of R0. The behaviour of ¯y2 looks similar to that of ˉλ, while that of ¯y1 looks different. There is a slow increase in the prevalence of infected males followed by a slow decrease in it, if g is allowed to change from 0 to 1. A different behaviour is noticed for the case of females, especially for high values of R0, where the endemic prevalence of infected females increases quickly, with the increase of the relative susceptibility g, till reaching a maximum at g=1.
Motivated by the work shown in [37], we assume that μr and νr are, respectively, the weighted mean and variance in susceptibilities of females and males. Since the proportion of females likewise that of males is 1/2, then
μr=r1+r22andνr=12(r1−μr)2+12(r2−μr)2=(r1−r22)2 | (3.21) |
where 0<r1≤r2. Hence,
r1=μr−√νr,r2=μr+√νrandg=r1r2=μr−√νrμr+√νr | (3.22) |
where 0≤νr≤μ2r:=νmaxr. It is clear that g=1 when νr=0. Moreover, g decreases with the increase of νr. On substituting from (3.22) into (3.5), we get
R0=ˉβ2((1γ+μ+1aγ+μ)μr+(1γ+μ−1aγ+μ)√νr). | (3.23) |
It is clear that
R0|νr=0=ˉβ2(1γ+μ+1aγ+μ)μr>0,∂R0∂νr|νr=0=ˉβ4√νr(1γ+μ−1aγ+μ){>0ifa>1,<0ifa<1.R0|νr=μ2r=ˉβμrγ+μwhich does not depend on a. |
If a<1, then 1/(aγ+μ)>1/(γ+μ). Therefore, R0(νr=0)>R0(νr=νmaxr) and we get monotonically decreasing curves, see Figure 4. However, if a>1, we get the opposite behaviour. Hence, if the mean of females and males susceptibilities is kept fixed, then the basic reproduction number R0 increases with the increase of the variance in susceptibilities between females and males if infected females recover faster than infected males (i.e., if the duration of influenza in females is less than it in males). Conversely, if infected males recover quicker than infected females (i.e., a<1), then any increase in the variance of susceptibility of females and males increases the value of the basic reproduction number R0. Therefore, we show the following proposition.
Proposition 5. If influenza duration in females is shorter than its duration in males, then (for constant mean of females and males susceptibilities) the basic reproduction number R0 increases with the increase of the variance in females and males susceptibilities and vice versa.
Similarly, we substitute from (3.22) into (3.11) to get
ˉλ=μ2{ˉβ2(1γ+μ+1aγ+μ)(μr+√νr)−2μrμr−√νr+√(ˉβ2(1γ+μ+1aγ+μ)(μr+√νr)−2μrμr−√νr)2+4μr+√νrμr−√νr(R0−1)} | (3.24) |
where R0 in (3.24) is given by (3.23). It is easy to check that
ˉλ|νr=0=ˉβμμr2(1γ+μ+1aγ+μ),ˉλ|νr=μ2r=ˉβμμrγ+μ. |
Moreover, if a<1, then ˉλ|νr=0>ˉλ|νr=μ2r and vice versa.
Simulations have been done to study the impact of disparity in females and males susceptibilities on the equilibrium force of infection ˉλ (Figure 5(a)) as well as on the endemic prevalence of infected females and males, Figure 5(b). The simulations show (for different values of the recovery rescaling parameter a) that ˉλ increases initially with the increase of the variance in susceptibilities νr till reaching a maximum and then it decreases again to reach its final value. It is clear that the value of ˉλ,¯y1 and ¯y2 at the maximum possible variance νmaxr does not depend on the value of a. Part (b) of the figure shows that the infected male population ¯y2 has a similar behaviour to the equilibrium force of infection ˉλ, while the endemic prevalence of infected females is always decreasing with the increase of the variance νr.
To study the impact of disparity in the recovery rates on the disease outcome, we assume that μγ,νγ are, respectively, the weighted mean and variance [37] of recovery rates aγ and γ of infected females and males. Thus,
μγ=(1+a)γ2andνγ=12(aγ−μγ)2+12(γ−μγ)2=((1−a)γ2)2. | (3.25) |
Hence,
μγ=(1+a)γ2and√νγ=γ2|1−a|. |
Thus, there are two cases: either a<1 or a>1.
● Let a<1. Then
γ=μγ+√νγ,a=μγ−√νγμγ+√νγ | (3.26) |
where 0≤νγ<μ2γ.
● If a>1, then
γ=μγ−√νγ,a=μγ+√νγμγ−√νγ | (3.27) |
where 0≤νγ<μ2γ.
It is clear that a=1 when νγ=0. On using (3.26) and (3.27) in (3.5), we get
R0|a<1=β2(1μ+μγ+√νγ+gμ+μγ−√νγ), | (3.28) |
R0|a>1=β2(1μ+μγ−√νγ+gμ+μγ+√νγ). | (3.29) |
Hence,
∂R0∂νγ|a<1=β4√νγ(−1(μ+μγ+√νγ)2+g(μ+μγ−√νγ)2),∂R0∂νγ|a>1=β4√νγ(1(μ+μγ−√νγ)2−g(μ+μγ+√νγ)2). |
It is easy to check that
∂R0∂νγ|a<1>0if and only ifνγ>(1−√g1+√g(μ+μγ))2:=ν⋆γ | (3.30) |
while
∂R0∂νγ|a>1,νγ>0>0holds under no condition. |
Moreover, ∂R0∂νγ|a<1,νγ=0<0. It is noteworthy that ν⋆γ<νmaxγ=μ2γ if and only if
μγ>μ2(√1g−1):=μ⋆γ. |
It is worth noting that formula (3.28) could be rewritten as
R0|a<1=R01+R02 |
where
R01=gβ2(μ+μγ−√νγ)andR02=β2(μ+μγ+√νγ). | (3.31) |
The first expression R01 represents the basic reproduction number for female population, while the second expression R02 denotes the basic reproduction number for male population, if males recover faster than females (i.e., a<1). As it is assumed that females are less susceptible than males (i.e., g<1), it holds that R01<R02 for small enough disparity in recovery rates between both sexes, precisely, for
0≤νγ<(1−g1+g(μ+μγ))2. |
If νγ increases, then R01 increases, while R02 decreases. It is easy to check that |dR01dνγ|>|dR02dνγ|, which means that the rate of increase in R01 is higher than the rate of decrease of R02 with respect to νγ. This interprets the initial reduction in the value of R0|a<1, see the dashed-dotted curve in Figure 6. In epidemiological terms, and motivated by formulae (3.31), any increase in the recovery rates disparity enlarges (shrinks) the infectious period of infected females (males) and therefore extends (shortens) the time window during which infected individuals are capable of transmitting the infection, which in turn increases (decreases) the female (male) reproduction number.
On the other hand, formula (3.29) could be rewritten as
R0|a>1=˜R01+˜R02 |
where
˜R01=gβ2(μ+μγ+√νγ)and˜R02=β2(μ+μγ−√νγ). | (3.32) |
At νγ=0, we have ˜R01<˜R02. Moreover, ˜R01 decreases while ˜R02 increases, with the increase of νγ. Also, the rate of increase in ˜R02 is higher than the rate of decrease in ˜R01, with respect to νγ. In other words, it holds that |d˜R01dνγ|<|d˜R02dνγ|. This clarifies the monotone increase in the basic reproduction number R0|a>1, see the solid curve in Figure 6. Epidemiologically, formulae (3.32) say that increasing the disparity in recovery rates between both sexes enlarges (shrinks) the infectious period of infected males (females) and therefore extends (shortens) the time window during which infected individuals are capable of transmitting the infection, which in turn increases (decreases) the male (female) reproduction number. Hence, the above result is summarised in the following proposition.
Proposition 6. In analysing the impact of recovery rate disparity, two cases arise.
1. If the duration of influenza in females is shorter than in males (i.e., a>1), then the basic reproduction number increases with the increase of the variance in recovery rate between infected females and males, see the solid curve in Figure 6.
2. If the duration of influenza in females is longer than in males (i.e., a<1), then we have the following:
● if μγ<μ⋆γ, then the basic reproduction number decreases with the increase of the variance in recovery rates between infected females and males.
● if μγ>μ⋆γ, then the basic reproduction number decreases initially till reaching a minimum at νγ=ν⋆γ and then it increases again, with the increase of the variance in recovery rates between infected females and males, see the dashed-dotted curve in Figure 6.
On substituting from (3.26) and (3.27) into (3.11) we get
ˉλ|a<1=μ2{β2(1μ+μγ+√νγ+1μ+μγ−√νγ)−(1+1g)+√(β2(1μ+μγ+√νγ+1μ+μγ−√νγ)−(1+1g))2+4g(R0|a<1−1)} |
and
ˉλ|a>1=μ2{β2(1μ+μγ−√νγ+1μ+μγ+√νγ)−(1+1g)+√(β2(1μ+μγ−√νγ+1μ+μγ+√νγ)−(1+1g))2+4g(R0|a>1−1)}. |
The impact of increasing the variance νγ in recovery rates of infected females and males, while keeping their mean constant, on the equilibrium force of infection ˉλ as well as on the endemic prevalence of infected females ¯y1 and infected males ¯y2 is numerically investigated in Figure 7. Part(a) shows that ˉλ and therefore the total proportion of infected individuals in the whole population at the endemic situation (¯y1+¯y2) behave similar to the basic reproduction number R0 if the variance νγ is allowed to increase, while the mean of recovery rates μγ is kept fixed. However, the proportion of infected females and males at the endemic situation behave differently, see Figure 7(b). If females recover faster than males (a>1), then the endemic prevalence of infected females ¯y1 decreases with the increase of the variance νγ, while the endemic prevalence of infected males increases ¯y2. Such behaviour could be because the increase in disparity in recovery rates νγ reduces (extends) the infected female (male) infectious period and, consequently, speeds up (slows down) the process of infected females (males) recovery, which in turn lowers (raises) the endemic prevalence of infected females (males) in the total population, see Figure 7(b).
Conversely, if males recover faster than females (i.e., a<1), then the prevalence of infected females increases while that of males decreases, with the increase of the variance νγ in recovery rates, while keeping their mean μγ constant. This dynamical behaviour is due to the fact that the increase in recovery rates' disparity extends (reduces) the length of infectious period in females (males) and in consequence decelerates (accelerates) the recovery process of females (males), which in turn increases (reduces) the endemic prevalence of infected females (males), see Figure 7(b).
The implication of the above results is summarised in the following items, giving that the mean recovery rate in males and females is constant.
● The faster the recovery of females than males is, the higher the value of the basic reproduction number is and the higher the prevalence of infection in the total population is, see figures 6, 7(a).
● If the duration of infection is shorter in females than in males, then the greater the disparity in recovery rates between females and males is, the higher the prevalence of infection in males is, the lower the endemic prevalence of infection in females is, but the higher the overall prevalence of infection in the total population is, see Figure 7. The converse is also true.
● If males recover quicker than females, then, as the disparity in recovery rates between both sexes is allowed to increase, an initial decrease followed by a rapid increase in the levels of the basic reproduction number as well as the total endemic prevalence of infection is remarkable, see figures 6, 7(a).
● In both male and female subpopulations, the higher the recovery rate in either subpopulations, compared to that of the other, is (i.e., the shorter the infectious period is), the lower the endemic prevalence of infection in that subpopulation is, see Figure 7(b).
Vaccines can prevent or ameliorate morbidity from infections. They are the most effective and cost-saving tools for disease prevention, preventing untold suffering and saving tens of thousands of lives and billions of dollars in healthcare costs each year [38]. There are two ways to vaccinate people. Either through the application of routine immunisation programmes or through the use of mass vaccination where susceptible people receive the vaccine to protect them from a potential infectious disease outbreak.
As influenza viruses mutate regularly, influenza vaccines are modified every year based on the prevalent influenza virus strains. The modified vaccines are always ready before the beginning of the seasons during which influenza display. However, due to the high mutation rates and frequent genetic reassortments of influenza viruses that contribute to the appearance of new subtypes, influenza vaccines are not included in routine immunisation programmes. Therefore, mass vaccination against influenza is applied, where populations at risk are rapidly vaccinated before the epidemic season for influenza begins. However, the efficacy of influenza vaccines depends on the degree of antigenic match to circulating viruses [39].
The WHO report [14] shows differences between the rate of vaccination against seasonal influenza between females and males in some countries. For example, males are more likely to receive the seasonal influenza vaccine in some european countries like the Czech Republic, France, Italy, Spain, the Netherlands, Poland and Portugal. However, the report shows that males and females are equally likely to get vaccinated against seasonal influenza in other countries like Austria, Finland, Germany and Ireland. According to the report, the situation in the United States is different. The analysis of vaccination data revealed that receiving seasonal influenza vaccine is age-dependent, where in some age groups females are more likely to receive the vaccine than males, while the converse happens in other age groups. A compartmental model for the transition between population states is shown in Figure 8, where these disparities are taken into account. The mathematical model with population proportions reads
dx1dt=12μ−(gλv+e0ψ+μ)x1,dw1dt=e0ψx1−((1−e1)gλv+μ)w1,dy1dt=gλv(x1+(1−e1)w1)−(aγ+μ)y1,dz1dt=aγy1−μz1,dx2dt=12μ−(λv+ψ+μ)x2,dw2dt=ψx2−((1−e2)λv+μ)w2,dy2dt=λv(x2+(1−e2)w2)−(γ+μ)y2,dz2dt=γy2−μz2, | (4.1) |
where w1 and w2 represent the proportion of vaccinated females and males, respectively, and
1=x1+y1+w1+z1+x2+y2+w2+z2. |
Similarly and as argued above, it holds that x1(t)+y1(t)+w1(t)+z1(t)=x2(t)+y2(t)+w2(t)+z2(t)=1/2.
It is easy to check that model (4.1) has the influenza-free (trivial) equilibrium
E0v=(x1,0vw1,0vy1,0vz1,0vx2,0vw2,0vy2,0vz2,0v)=(μ/(2(μ+e0ψ))e0ψ/(2(μ+e0ψ))00μ/(2(μ+ψ))ψ/(2(μ+ψ))00). |
Again, following the approach of Diekmann et al. [34], we compute the transmission matrix Tv and the transition matrix Σv as
Tv=(gβ(μ+(1−e1)e0ψ)/2(μ+e0ψ)gβ(μ+(1−e1)e0ψ)/2(μ+e0ψ)β(μ+(1−e2)ψ)/2(μ+ψ)β(μ+(1−e2)ψ)/2(μ+ψ)) |
and
Σv=(−(aγ+μ)00−(γ+μ)). |
Thus, the effective reproduction number Rψ is the dominant eigenvalue of the next generation matrix with large domain KLv=−TvΣ−1v, where
Rψ=β2(μ+(1−e2)ψ(γ+μ)(μ+ψ)+g[μ+(1−e1)e0ψ](aγ+μ)(μ+e0ψ)). | (4.2) |
Local stability:
The stability analysis of the influenza-free equilibrium in the presence of vaccination E0v shows that E0v is locally asymptotically stable if and only if the effective reproduction number Rψ<1. Therefore, we state the following proposition, whose proof is shown in appendix B.
Proposition 7. Model (4.1) has an influenza-free equilibrium E0v that is locally asymptotically stable if and only if the effective reproduction number Rψ<1.
Global stability:
The global stability of the influenza-free equilibrium in the presence of vaccination E0v is established using the fluctuation lemma [40], which we state below. To this end, we introduce some notations and state the a proposition about the positivity of the solutions, whose proof is deferred to appendix C. Assume that f is a real-valued function defined on the interval [0,∞). Then, we define
f∞=limt→∞inff(t)andf∞=limt→∞supf(t). | (4.3) |
Proposition 8. Model (4.1) has a unique solution with components x1(t), w1(t), y1(t), z1(t), x2(t), w2(t), y2(t) and z2(t) that are non-negative for all forward times t≥0, if the initial values of the components are non-negative.
The proof of this proposition is deferred to appendix C.
Remark 1. Since all solutions x1(t), w1(t), y1(t), z1(t), x2(t), w2(t), y2(t) and z2(t) are non-negative and their sum equals 1, then they are all bounded from above.
Now, we state (without proof) the fluctuation lemma [40] that is needed in the global stability analysis of E0v.
Lemma 1. Let f:[0,∞)→R be bounded and differentiable and have no limit as t→∞. Then, there exist sequences {sn}∞n=1 and {tn}∞n=1, such that, for all n
dfdt(sn)=dfdt(tn)=0 |
and
limn→∞f(sn)=f∞,limn→∞f(tn)=f∞. |
It is noteworthy that f∞=limn→∞f(sn)=limt→∞supf(t), f∞=limn→∞f(tn)=limt→∞inff(t) and that f∞≤f∞. In the following, we introduce a proposition showing our result on the global stability of the influenza-free equilibrium in the presence of vaccination E0v.
Proposition 9. The influenza-free equilibrium in the presence of vaccination E0v is globally stable if and only if the effective reproduction number Rψ<1.
Proof. Before going into details, we recall that all state variables are non-negative and bounded from above. From the x1 equation in (4.1), it is clear that x1 satisfies the differential inequality
dx1dt<12μ−(e0ψ+μ)x1. | (4.4) |
On applying the above fluctuation lemma, there exists a sequence {sn}∞n=1 such that sn→∞,x1(sn)→x∞1,˙x1(sn)→0, as n→∞. Hence, from the differential inequality (4.4) we get
x∞1≤μ/2μ+e0ψ. |
Similarly, we apply the fluctuation lemma to the differential equations of x2,w1,w2,y1 and y2 in (4.1). Accordingly,
● we can find a sequence {sn}∞n=1 such that sn→∞, x2(sn)→x∞2,˙x2(sn)→0, as n→∞. Hence,
x∞2≤μ/2μ+ψ. |
● we can find another sequence {sn}∞n=1 such that sn→∞, w1(sn)→w∞1, ˙w1(sn)→0, as n→∞. Hence,
w∞1≤e0ψμx∞1≤e0ψ/2μ+e0ψ |
● there can exist a different sequence {sn}∞n=1 such that sn→∞, w2(sn)→w∞2, ˙w2(sn)→0, as n→∞. Hence,
w∞2≤ψμx∞2≤ψ/2μ+ψ |
● there can also exist another sequence {sn}∞n=1 such that sn→∞, y1(sn)→y∞1, ˙y1(sn)→0, as n→∞. Hence,
0≤gλ∞v(x∞1+(1−e1)w∞1)−(aγ+μ)y∞1. |
Hence,
y∞1≤gλ∞v(μ+(1−e1)e0ψ)2(aγ+μ)(μ+e0ψ). |
● there exists also another different sequence {sn}∞n=1 such that sn→∞, y2(sn)→y∞2, ˙y2(sn)→0, as n→∞. Hence,
0≤λ∞v(x∞2+(1−e2)w∞2)−(γ+μ)y∞2. |
Hence,
y∞2≤λ∞v(μ+(1−e2)ψ)2(γ+μ)(μ+ψ). |
Now,
λ∞v=β(y∞1+y∞2)≤βλ∞v2(g[μ+(1−e1)e0ψ](aγ+μ)(μ+e0ψ)+μ+(1−e2)ψ(γ+μ)(ψ+μ)) |
Hence,
λ∞v[β2(g[μ+(1−e1)e0ψ](aγ+μ)(μ+e0ψ)+μ+(1−e2)ψ(γ+μ)(ψ+μ))−1]≥0 |
I.e.,
λ∞v(Rψ−1)≥0. |
Since Rψ<1, it should be held that λ∞v≤0. On the other hand, it is shown in proposition 8 that y1(t)≥0 and y2(t)≥0 for all forward times t≥0, which implies that y1∞≥0 and y2∞≥0. Therefore, λv∞≥0. Since λv∞≤λ∞v, then it should hold that λv∞=λ∞v=0. Hence, y∞1=y∞2=0. Thus, the proof is complete.
As the rate of change in model states vanishes at equilibrium, we put the left hand side of (4.1) equal zero and solve the resulting system with respect to the model's state variables, we get (if ˉλv≠0) the endemic equilibrium
E1v=(x1v,y1v,w1v,z1v,x2v,y2v,w2v,z2v)T | (4.5) |
where
x1v=μ2(μ+e0ψ+gˉλv),w1v=e0ψμ2(μ+(1−e1)gˉλv)(μ+e0ψ+gˉλv)y1v=gˉλvμ[μ+e(1−e1)ψ+(1−e1)gˉλv]2(μ+aγ)(μ+(1−e1)gˉλv)(μ+e0ψ+gˉλv),z1v=12−x1v−w1v−y1v,x2v=μ2(μ+ψ+ˉλv),w2v=ψμ2(μ+(1−e2)ˉλv)(μ+ψ+ˉλv)y2v=ˉλvμ[μ+(1−e2)ψ+(1−e2)ˉλv]2(μ+γ)(μ+(1−e2)ˉλv)(μ+ψ+ˉλv),z2v=12−x2v−w2v−y2v | (4.6) |
and ˉλv∈(0,∞) is the equilibrium force of infection for model (4.1) and is the unique positive solution of
1=βμ2(μ+(1−e2)ˉλv+(1−e2)ψ(γ+μ)(μ+ψ+ˉλv)(μ+(1−e2)ˉλv)+g[μ+(1−e1)gˉλv+(1−e1)e0ψ](aγ+μ)(μ+e0ψ+gˉλv)(μ+(1−e1)gˉλv)). | (4.7) |
The analysis shows that (4.7) has a unique positive solution if and only if Rψ>1. It is clear that there is a one-to-one correspondence between the solution of (4.7) and the states in (4.6). Once the feasible solution of (4.7) is found, we substitute in (4.6) to get the unique endemic equilibrium of model (4.1).
To prove the existence of a unique positive solution for (4.7), we rewrite it as
G1(ˉλv)=G2(ˉλv) |
where
G1(ˉλv)=2βμ−1(γ+μ)(μ+ψ+ˉλv)−g(aγ+μ)(μ+e0ψ+gˉλv),G2(ˉλv)=(1−e2)ψ(γ+μ)(μ+ψ+ˉλv)(μ+(1−e2)ˉλv)+g(1−e1)e0ψ(aγ+μ)(μ+e0ψ+gˉλv)(μ+(1−e1)gˉλv). |
It is clear that
G1(0)=2βμ−1(γ+μ)(μ+ψ)−g(aγ+μ)(μ+e0ψ),G2(0)=(1−e2)ψμ(γ+μ)(μ+ψ)+g(1−e1)e0ψμ(aγ+μ)(μ+e0ψ), |
and
dG1(ˉλv)dˉλv=1(γ+μ)(μ+ψ+ˉλv)2+g(aγ+μ)(μ+e0ψ+gˉλv)2,dG2(ˉλv)dˉλv=−(1−e2)ψ[μ+(1−e2)(2ˉλv+μ+ψ)](γ+μ)(μ+ψ+ˉλv)2(μ+(1−e2)ˉλv)2−g2(1−e1)e0ψ[μ+(1−e1)(2gˉλv+μ+e0ψ)](aγ+μ)(μ+e0ψ+gˉλv)2(μ+(1−e1)gˉλv)2. |
Hence, G1 is monotonically increasing, while G2 is monotonically decreasing in ˉλv. Thus, equation (4.7) has a unique positive solution if and only if G1(0)<G2(0), while otherwise it has no positive solution. Simple computations show that the existence condition G1(0)<G2(0) is equivalent to Rψ>1.Hence, we show the following proposition.
Proposition 10. Model (4.1) has a unique endemic equilibrium that exists if and only if the effective reproduction number Rψ>1.
The direct effect of vaccination is the number of vaccinated individuals who caught the infection due to imperfection of the vaccine, but would have been completely protected if the vaccine was totally perfect (i.e., it is the infection incidences in vaccinees). However, the indirect effect of vaccination is the difference between its total effect and its direct effect [41]. The total effect of vaccination is the reduction in the number of incidences due to vaccination. To quantify the total effect of influenza mass vaccination in our models, we assume that Ia is the influenza incidences in the absence of vaccination and Ip is the influenza incidences in the presence of vaccination. Hence, models (2.6) and (3.1) imply that
Ia=gλS1+λS2=Nλ(gx1+x2). |
Similarly, model (4.1) implies that
Ip=N(gλv(x1+(1−e1)w1)+λv(x2+(1−e2)w2)). |
Thus, the total effect of vaccination (i.e., the reduction in the total number of incidences due to vaccination) is
IT=Ia−Ip=N{λ(gx1+x2)−gλv(x1+(1−e1)w1)−λv(x2+(1−e2)w2)}. |
The infection incidences in vaccinee (due to the imperfection of vaccination) is denoted by ID and is given by
ID=N(gλv(1−e1)w1+λv(1−e2)w2) |
which accounts also for the direct effect. Thus, the indirect effect of vaccination is
IIND=IT−ID. |
If we look at the equilibrium state, then
Ia=μNˉλ2(ggˉλ+μ+1ˉλ+μ),Ip=μNˉλv2(g(μ+(1−e1)(e0ψ+gˉλv))(μ+(1−e1)gˉλv)(μ+e0ψ+gˉλv)+μ+(1−e2)(ψ+ˉλv)(μ+(1−e2)ˉλv)(μ+ψ+ˉλv)),ID=μψNˉλv2(e(1−e1)g(μ+(1−e1)gˉλv)(μ+e0ψ+gˉλv)+(1−e2)(μ+(1−e2)ˉλv)(μ+ψ+ˉλv)) |
where ˉλ is given by (3.11), while ˉλv is the solution of (4.7).
Figure 9(a) shows the total effect IT, direct effect ID and indirect effect IIND of vaccination as functions of the effective reproduction number Rψ. Both the indirect effect and total effect are monotonically decreasing in Rψ, while the direct effect is monotonically increasing in Rψ. Part (b) of Figure 9 shows the ratio of the indirect to direct protection IIND/ID as a function of Rψ. Since Rψ decreases with the increase of the vaccination rate ψ, we then deduce that the higher the vaccination rate is, the higher the total effect IT, the indirect effect IIND and the protection ratio IIND/ID are, the lower the direct effect ID is.
Simulations have also been done for various levels of vaccination efficacies e1 and e2. Figure 10 shows that (for fixed e2 and other model parameters) increasing the vaccine efficacy in females e1 increases the total effect, the indirect effect and the ratio of indirect to direct effects, but decreases the direct effect ID of vaccination. Hence, the higher the efficacy of females vaccine is, the more reduction in the total number of new incidences is. However, if the vaccine efficacy in females e1 is kept fixed with the other parameters, while the vaccine efficacy in males e2 is allowed to change, simulations in Figure 11 show that the behaviour of these effects depends on the value of the effective reproduction number Rψ. For low levels of Rψ (slightly above one, but less than certain level), increasing the efficacy e2 increases the protection ratio of indirect to direct effect as well as the direct, indirect, and total effect. Conversely, for high enough values of Rψ, these effects as well as that ratio decrease with the increase of the vaccine efficacy in males e2.
Mathematical models have been extensively used to explore the evolutionary dynamics of influenza viruses. However, to the best of our knowledge, the role of sex and/or gender in respiratory infections (e.g., influenza) transmission dynamics has not been explored mathematically and a greater understanding of the influence of sex and gender disparity on the overall outcome of influenza is lacking. Therefore, a mathematical model to describe the transmission dynamics of influenza A viruses, taking into account sex and gender disparity, has been formulated and thoroughly analysed. The model we considered is of type SIR (Susceptible - Infected - Recovered) in which it is differentiated between females and males through their susceptibility, duration of infection (based on different recovery rates), response to vaccination campaigns and their immune response to the vaccine.
In the absence of vaccination, the model has the influenza-free equilibrium E0 that is shown to be globally asymptotically stable if and only if the basic reproduction number R0 is less than one. However, it has a unique endemic equilibrium that exists if and only if R0>1. This endemic equilibrium is proved to be locally asymptotically stable whenever it exists. Moreover, the impact of sex disparity (represented by differential susceptibility and differential recovery rates of infected females and males) on the influenza virus disease outcome has been studied. The analysis shows that, irrespective of the value of the rescaling recovery rate parameter a which represents the ratio of the recovery rate of infected females to that of infected males, the basic reproduction number R0 increases with the increase of the relative susceptibility g of females with respect to males. Moreover, simulations show that the endemic prevalence of infected males ¯y2 as well as the total proportion of infected individuals (¯y1+¯y2) in the whole population increase initially with the increase of g till reaching a maximum (approximately at around g=0.5) and then they decrease again. However, for slightly high values of R0, the endemic prevalence of infected females ¯y1 grows monotonically as g increases.
On the other hand, the disease outcomes have been analysed in terms of the mean and variance of susceptibilities as well as of recovery rates of females and males. The analysis shows that if the duration of influenza A in females is shorter than in males a>1, then the basic reproduction number R0 increases as the variance νr in susceptibilities of both sexes increases, given that their mean μr is kept constant. However, if males recover faster than females (i.e., a<1), then R0 decreases with the increase of νr. The analysis shows further that the endemic prevalence of infected males increases initially with the increase of the variance νr and then it decreases again to reach its final value (at νr=μ2r=νmaxr) that does not depend on a, while the endemic prevalence of infected females decreases monotonically to vanish at νr=μ2r.
In analysing the disease outcomes in terms of mean and variance of recovery rates of females and males, it is shown that if females recover faster than males, then R0 increases with the increase of the variance νγ in recovery rates of females and males (given that their mean μγ is kept constant). However, if males recover faster than females, then R0 decreases initially till reaching a minimum and then it increases again with the increase of the variance νγ. The equilibrium force of infection ˉλ and therefore the total proportion of infected population at equilibrium ¯y1+¯y2 behave similar to R0, although ¯y1 and ¯y2 have opposite behaviours.
The possibility to apply mass vaccination as a prevention strategy to contain influenza A has been studied, where it is differentiated between vaccine efficacy in both sexes. Also, the gender disparity (represented by disparity in individuals' response to vaccination campaigns) has been taken into account. A thorough equilibrium analysis of the model has been performed and the local and global stability analysis of the influenza-free equilibrium (in the presence of vaccination) have been established, when the effective reproduction number Rψ is less than one.
The direct effect (i.e., the reduction in the incidences of vaccinated individuals if the vaccine was totally perfect) and indirect effect (i.e., the rest of the total incidences) of influenza mass vaccination have been studied. The analysis revealed that the efficacy of the vaccine for both sexes (e1&e2) and the response of the gender to mass-vaccination campaigns ψ play a crucial role in influenza A containment and elimination process. High response to vaccination campaigns and high efficacy of the vaccine in females imply to high effect of vaccination on the burden of the infection, which implies to a more reduction in the total number of new incidences. However, high efficacy of the vaccine in males would cause much reduction in the number of new incidences if the effective reproduction number Rψ is small (slightly above one, but less than certain level), while it reduces the effect of vaccination (i.e., it increases the occurrence of new incidences) for high enough values of Rψ.
It is worth noting that influenza could pose a significant mortality burden to vulnerable populations and lead to changes in the demographic makeup of the population. In other words, influenza dynamics and the severity of epidemics is not only affected by the underlying population characteristics, but yearly epidemics also induce changes in population demographics. One more important component for studying epidemic effects on demographic characteristics of populations is the inclusion of age-structure factor, as the transmission rates, susceptibility, risk of death, and vaccination coverage rates vary across age groups. These factors could probably be taken into account in a future work.
The authors would like to thank the Associate editor as well as the anonymous referees very much for their invaluable and comprehensive comments which helped in improving the paper.
The author declares no conflict of interest.
Before going into details, we assume that
X=βμ2(γ+μ)(ˉλ+μ)andY=gβμ2(aγ+μ)(gˉλ+μ) |
where, according to (3.9), X+Y=1. Using the formulae of C1,C2 and C3, we have
C1C2−C3=(ˉλ+μ)(gˉλ+μ)((1+g)ˉλ+2μ)+(γ+μ)[(ˉλ+μ)(ˉλ+(gˉλ+2μ)Y)+Y(gˉλ+μ)((1+g)ˉλ+2μ)]+(aγ+μ)[(gˉλ+μ)(gˉλ+(ˉλ+2μ)X)+X(ˉλ+μ)((1+g)ˉλ+2μ)]+(γ+μ)2Y[ˉλ+Y(gˉλ+2μ)]+(aγ+μ)2X[gˉλ+X(ˉλ+2μ)]+(γ+μ)(aγ+μ)XY((1+g)ˉλ+4μ)>0. |
Thus, after some tedious calculations, we get
Δ=(C1C2−C3)C3−C4C21=[(γ+μ)ˉλ(ˉλ+μ)(gˉλ+μ)2+(aγ+μ)gˉλ(gˉλ+μ)(ˉλ+μ)2]((1+g)ˉλ+2μ)+(γ+μ)2ˉλ(gˉλ+μ)[ˉλ(ˉλ+μ)+Y(ˉλ+μ)(gˉλ+2μ)+Y(gˉλ+μ)((1+g)ˉλ+2μ)]+(aγ+μ)2gˉλ(ˉλ+μ)[gˉλ(gˉλ+μ)+X(gˉλ+μ)(ˉλ+2μ)+X(ˉλ+μ)((1+g)ˉλ+2μ)]+(γ+μ)(aγ+μ)[gˉλ2(X(ˉλ+μ)2+Y(gˉλ+μ)2)+(ˉλ+μ)(gˉλ+μ)(ˉλX+gˉλY)((1+g)ˉλ+2μ)]+(γ+μ)3ˉλY(gˉλ+μ)[ˉλ+Y(gˉλ+2μ)]+(aγ+μ)3gˉλX(ˉλ+μ)[gˉλ+X(ˉλ+2μ)]+(γ+μ)2(aγ+μ)[gˉλY2(gˉλ+μ)((1+g)ˉλ+2μ)+Xˉλ2(ˉλ+μ)+ˉλXY(2(gˉλ+μ)(ˉλ+2μ)+gˉλ(ˉλ+μ))]+(γ+μ)(aγ+μ)2[ˉλX2(ˉλ+μ)((1+g)ˉλ+2μ)+Yg2ˉλ2(gˉλ+μ)+gˉλXY(2(ˉλ+μ)(gˉλ+2μ)+ˉλ(gˉλ+μ))]+μ[(aγ+μ)(ˉλ+μ)X+(γ+μ)(gˉλ+μ)Y](C1C2−C3)+(γ+μ)(aγ+μ)Δsub |
where
Δsub=(γ+μ)2[XYˉλ(ˉλ+μY)+gˉλY2(ˉλX+(gˉλ+μ)Y)]+(aγ+μ)2[XYgˉλ(gˉλ+μX)+ˉλX2(gˉλY+(ˉλ+μ)X)]+(γ+μ)(aγ+μ)[X2Yˉλ(2μ+(1−g)ˉλ)+Y2Xgˉλ(2μ+gˉλ−ˉλ)]. |
Here, we have two cases, either g≤1 or g>1, and in each case we have two sub-cases: a≤1 and a>1. Therefore, we study the sign of Δsub in the following four cases.
● Case (1): g≤1 and a≤1. Since we assume that a≤1, then −gˉλ2XY2(γ+μ)(aγ+μ)≥−gˉλ2XY2(γ+μ)2. Hence,
Δsub|(g≤1&a≤1)≥(γ+μ)2[XYˉλ(ˉλ+μY)+gˉλ(gˉλ+μ)Y3]+(aγ+μ)2[XYgˉλ(gˉλ+μX)+ˉλX2(gˉλY+(ˉλ+μ)X)]+(γ+μ)(aγ+μ)[X2Yˉλ(2μ+(1−g)ˉλ)+Y2Xgˉλ(2μ+gˉλ)]>0. |
● Case (2): g≤1 and a>1. If we assume that a≥1, then (γ+μ)(ˉλ+μ)≤(aγ+μ)(ˉλ+μ/g). Hence, X≥Y. Also, a≥1 implies that (γ+μ)(aγ+μ)≤(aγ+μ)2. Hence,
−gˉλ2XY2(γ+μ)(aγ+μ)≥−gˉλ2XY2(aγ+μ)2≥−gˉλ2X2Y(aγ+μ)2. |
Thus,
Δsub|(g≤1&a≥1)≥(γ+μ)2[XYˉλ(ˉλ+μY)+gˉλY2(ˉλX+(gˉλ+μ)Y)]+(aγ+μ)2[XYgˉλ(gˉλ+μX)+ˉλ(ˉλ+μ)X3]+(γ+μ)(aγ+μ)[X2Yˉλ(2μ+(1−g)ˉλ)+Y2Xgˉλ(2μ+gˉλ)]>0. |
● Case (3): g>1 and a≤1. In this case, we have (γ+μ)(ˉλ+μ)≥(aγ+μ)(ˉλ+μ/g). Hence, X≤Y. Also, a≤1 implies that (γ+μ)(aγ+μ)≤(γ+μ)2. Hence,
−gˉλ2X2Y(γ+μ)(aγ+μ)≥−gˉλ2X2Y(γ+μ)2≥−gˉλ2XY2(γ+μ)2. |
Thus,
Δsub|(g>1&a≤1)≥(γ+μ)2[XYˉλ(ˉλ+μY)+gˉλ(gˉλ+μ)Y3]+(aγ+μ)2[XYgˉλ(gˉλ+μX)+ˉλX2(gˉλY+(ˉλ+μ)X)]+(γ+μ)(aγ+μ)[X2Yˉλ(2μ+ˉλ)+Y2Xgˉλ(2μ+(g−1)ˉλ)]>0. |
● Case (4): g>1 and a>1. In this case, we have −gˉλ2X2Y(γ+μ)(aγ+μ)≥−gˉλ2X2Y(aγ+μ)2. Hence,
Δsub|(g>1&a>1)≥(γ+μ)2[XYˉλ(ˉλ+μY)+gˉλY2(ˉλX+(gˉλ+μ)Y)]+(aγ+μ)2[XYgˉλ(gˉλ+μX)+ˉλ(ˉλ+μ)X3]+(γ+μ)(aγ+μ)[X2Yˉλ(2μ+ˉλ)+Y2Xgˉλ(2μ+(g−1)ˉλ)]>0. |
Hence, Δsub>0. Thus, Δ>0.
Proof. The local stability of the influenza-free equilibrium E0v of model (4.1) is investigated by considering the eigenvalues of the Jacobian matrix J0v of the model evaluated at the influenza-free equilibrium E0v, where
J0v=(−Dx10−J1,3000−J1,70e0ψ−μ−J2,3000−J2,7000J3,3000J3,7000aγ−μ000000−J5,30−Dx20−J5,7000−J6,30ψ−μ−J6,7000J7,3000J7,70000000γ−μ) |
where Dx1=e0ψ+μ, J1,3=gβx1,0v, J1,7=gβx1,0v, J2,3=g(1−e1)βw1,0v, J2,7=g(1−e1)βw1,0v, J3,3=gβ[x1,0v+(1−e1)w1,0v]−(aγ+μ), J3,7=gβ(x1,0v+(1−e1)w1,0v), J5,3=βx2,0v, J5,7=βx2,0v, Dx2=μ+ψ, J6,3=(1−e2)βw2,0v, J6,7=(1−e2)βw2,0v, J7,3=β[x2,0v+(1−e2)w2,0v], J7,7=β[x2,0v+(1−e2)w2,0v]−(γ+μ). Its eigenvalues are −μ, −μ, −μ, −μ, −(ψ+μ), −(e0ψ+μ) and those of the matrix
Jvsub=(J3,3J3,7J7,3J7,7). |
Hence, the local stability of E0v depends on the determinant and trace of the matrix Jvsub. Now,
det(Jvsub)=J3,3J7,7−J3,7J7,3=(γ+μ)(aγ+μ)(1−Rψ) |
Hence, det(Jvsub)>0 if and only if Rψ<1. Thus, to complete proving the local stability of E0v for Rψ<1, it remains to show that tr(Jvsub)<0. The inequality Rψ<1 is equivalent to
β[x2,0v+(1−e2)w2,0v]γ+μ+gβ[x1,0v+(1−e1)w1,0v]aγ+μ<1 |
which implies that
β[x2,0v+(1−e2)w2,0v]γ+μ<1andgβ[x1,0v+(1−e1)w1,0v]aγ+μ<1. |
Therefore,
β[x2,0v+(1−e2)w2,0v]−(γ+μ)<0andgβ[x1,0v+(1−e1)w1,0v]−(aγ+μ)<0. |
I.e.,
J3,3<0andJ7,7<0. |
Thus,
tr(Jvsub)=J3,3+J7,7<0. |
Proof. As the right hand side of (4.1) is continuously differentiable functions, it is locally Lipschitz. Therefore, model (4.1) has a unique local solution with components x1(t), w1(t), y1(t), z1(t), x2(t), w2(t), y2(t) and z2(t) and with initial values x1(0), w1(0), y1(0), z1(0), x2(0), w2(0), y2(0), z2(0)≥0, where x1(t)+w1(t)+y1(t)+z1(t)+x2(t)+w2(t)+y2(t)+z2(t)=1 for all t≥0. This solution is defined on the forward invariant set
Ω={(x1(t),w1(t),y1(t),z1(t),x2(t),w2(t),y2(t),z2(t))∈R8:x1,w1,y1,z1,x2,w2,y2,z2∈[0,1/2] and x1+w1+y1+z1=x2+w2+y2+z2=1/2} |
To show that the state variables are non-negative for all t≥0, we assume the converse. I.e., we assume that x1(0)>0, w1(0)>0, y1(0)>0, z1(0)>0, x2(0)>0, w2(0)>0, y2(0)>0, z2(0)>0 and that at least one of the state variables x1(t), w1(t), y1(t), z1(t), x2(t), w2(t), y2(t) and z2(t) become(s) negative for some t>0. Assume further that t=tx1 is the first time at which x1(t)=0. Then
dx1dt(tx1)=12μ>0. |
Since x1 is continuous in time, it implies that x1(t)<0 for t<tx1, which contradicts our assumption that tx1 is the first time at which x1 vanishes. Thus, x1(t) is non-negative for all t≥0. Similarly, if we assume that tw1,ty1,tz1,tx2,tw2,ty2 and tz2 represent the first times at which w1(t), y1(t), z1(t), x2(t), w2(t), y2(t) and z2(t), respectively, become zero, then we compute
dw1dt(tw1)=e0ψx1,dy1dt(ty1)=gβy2(x1+(1−e1)w1),dz1dt(tz1)=aγy1,dx2dt(tx2)=12μ, |
dw2dt(tw2)=ψx2,dy2dt(ty2)=rβy1(x2+(1−e2)w2),dz2dt(tz2)=γy2 |
which are all positive. Thus, by continuity of these state variables, it implies that w1(t)<0 for t<tw1, y1(t)<0 for t<ty1, z1(t)<0 for t<tz1, x2(t)<0 for t<tx2, w2(t)<0 for t<tw2, y2(t)<0 for t<ty2 and z2(t)<0 for t<tz2 which contradict the above assumptions. Thus, all state variables are non-negative for all forward times t≥0.
[1] | S. Chandrasekhar, Liquid Crystals, Cambridge U. Press, Cambridge, 1977. |
[2] | P. G. de Gennes, J. Prost, The Physics of Liquid Crystals, Oxford Science Publications, Oxford, 1993. |
[3] |
J. Ericksen, Liquid crystals with variable degree of orientation, Arch Rational Mech. Anal., 113 (1990), 97–120. https://doi.org/10.1007/BF00380413 doi: 10.1007/BF00380413
![]() |
[4] | E. Virga, Variational Theories for Liquid Crystals, Applied Mathematics and Mathematical Computation, 8, Chapman & Hall, London, 1994. https://doi.org/10.1007/978-1-4899-2867-2 |
[5] | F. C. Frank, On the theory of liquid crystals, Discuss. Faraday Soc., 25 (1958), 19–28. |
[6] | N. J. Mottram, J. P. Newton, Introduction to Q-tensor theory, arXiv preprint, arXiv: 1409.3542, 2014. |
[7] |
R. Hardt, D. Kinderlehrer, F. H. Lin, Existence and partial regularity of static liquid crystal configurations, Comm. Math. Phys., 105 (1986), 547–570. https://doi.org/10.1007/BF01238933 doi: 10.1007/BF01238933
![]() |
[8] |
O. Alper, R. Hardt, F. H. Lin, Defects of liquid crystals with variable degree of orientation, Calc. Var. Partial Dif., 56 (2017), Paper No. 128. https://doi.org/10.1007/s00526-017-1218-5 doi: 10.1007/s00526-017-1218-5
![]() |
[9] |
F. H. Lin, On nematic liquid crystals with variable degree of orientation, Comm. Pure Appl. Math., 44 (1991), 453–468. https://doi.org/10.1002/cpa.3160440404 doi: 10.1002/cpa.3160440404
![]() |
[10] | J. Ball, Mathematics of liquid crystals, Cambridge Centre for Analysis short course, (2012), 13–17. |
[11] | A. Zarnescu, Topics in the Q-tensor theory of liquid crystals, Topics in mathematical modeling and analysis, 187–252, Jindrich Necas Cent. Math. Model. Lect. Notes, 7, Matfyzpress, Prague, 2012. |
[12] |
M. Paicu, A. Zarnescu, Global existence and regularity for the full coupled Navier-Stokes and Q-tensor system, SIAM J. Math. Anal., 43 (2011), 2009–2049. https://doi.org/10.1137/10079224X doi: 10.1137/10079224X
![]() |
[13] |
M. Paicu, A. Zarnescu, Energy dissipation and regularity for a coupled Navier-Stokes and Q-tensor system, Arch. Ration. Mech. Anal., 203 (2012), 45–67. https://doi.org/10.1007/s00205-011-0443-x doi: 10.1007/s00205-011-0443-x
![]() |
[14] | A. N. Beris, B. J. Edwards, Thermodynamics of Flowing Systems with Internal Microstructure, Oxford Engineerin Science Series, No. 36, Oxford university Press, Oxford, New York, 1994. |
[15] |
F. De Anna, A global 2D well-posedness result on the order tensor liquid crystal theory, J. Differ. Equ., 262 (2017), 3932–3979. https://doi.org/10.1016/j.jde.2016.12.006 doi: 10.1016/j.jde.2016.12.006
![]() |
[16] |
M. M. Dai, E. Feireisl, E. Rocca, G. Schimperna, M. Schonbek, On asymptotic isotropy for a hydrodynamic model of liquid crystals, Asymptot. Anal., 97 (2016), 189–210. https://doi.org/10.3233/ASY-151348 doi: 10.3233/ASY-151348
![]() |
[17] |
H. R. Du, X. P. Hu, C. Y. Wang, Suitable weak solutions for the co-rotational Beris-Edwards system in dimension three, Arch. Ration. Mech. Anal., 238 (2020), 749–803. https://doi.org/10.1007/s00205-020-01554-y doi: 10.1007/s00205-020-01554-y
![]() |
[18] |
F. Guillén-González, M. A. Rodríguez-Bellido, Weak solutions for an initial-boundary Q-tensor problem related to liquid crystals, Nonlinear Anal., 112 (2015), 84–104. https://doi.org/10.1016/j.na.2014.09.011 doi: 10.1016/j.na.2014.09.011
![]() |
[19] | H. Abels, G. Dolzmann, Y. N. Liu, Strong solutions for the Beris–Edwards model for nematic liquid crystals with homogeneous Dirichlet boundary conditions, Adv. Differ. Equ., 21 (2016), 109–152. |
[20] |
F. Guillén-González, M. A. Rodríguez-Bellido, Weak time regularity and uniqueness for a Q-tensor model, SIAM J. Math. Anal., 46 (2014), 3540–3567. https://doi.org/10.1137/13095015X doi: 10.1137/13095015X
![]() |
[21] |
F. De Anna, A. Zarnescu, Uniqueness of weak solutions of the full coupled Navier–Stokes and Q-tensor system in 2D, Commun. Math. Sci., 14 (2016), 2127–2178. https://doi.org/10.4310/CMS.2016.v14.n8.a3 doi: 10.4310/CMS.2016.v14.n8.a3
![]() |
[22] |
C. Cavaterra, E. Rocca, H. Wu, X. Xu, Global strong solutions of the full Navier–Stokes and Q-tensor system for nematic liquid crystal flows in two dimensions, SIAM J. Math. Anal., 48 (2016), 1368–1399. https://doi.org/10.1137/15M1048550 doi: 10.1137/15M1048550
![]() |
[23] |
H. Abels, G. Dolzmann, Y. N. Liu, Well-posedness of a fully coupled Navier–Stokes/Q-tensor system with inhomogeneous boundary data, SIAM J. Math. Anal., 46 (2014), 3050–3077. https://doi.org/10.1137/130945405 doi: 10.1137/130945405
![]() |
[24] |
J. Ericksen, Conservation laws for liquid crystals, Trans. Soc. Rheol., 5 (1961), 22–34. https://doi.org/10.1122/1.548883 doi: 10.1122/1.548883
![]() |
[25] |
F. Leslie, Some constitutive equations for liquid crystals, Arch. Ration. Mech. Anal., 28 (1968), 265–283. https://doi.org/10.1007/BF00251810 doi: 10.1007/BF00251810
![]() |
[26] |
W. Wang, P. W. Zhang, Z. F. Zhang, Rigorous derivation from Landau–de Gennes theory to Ericksen–Leslie theory, SIAM J. Math. Anal., 47 (2015), 127–158. https://doi.org/10.1137/13093529X doi: 10.1137/13093529X
![]() |
[27] |
F. H. Lin, C. Y. Wang, Recent developments of analysis for hydrodynamic flow of nematic liquid crystals, Philos. Trans. R. Soc. Lond. Ser. A Math. Phys. Eng. Sci., 372 (2014), 20130361. https://doi.org/10.1098/rsta.2013.0361 doi: 10.1098/rsta.2013.0361
![]() |
[28] |
G. Iyer, X. Xu, A. Zarnescu, Dynamic cubic instability in a 2D Q-tensor model for liquid crystals, Math. Mod. Methods Appl. Sci., 25 (2015), 1477–1517. https://doi.org/10.1142/S0218202515500396 doi: 10.1142/S0218202515500396
![]() |
[29] |
H. Wu, X. Xu, A. Zarnescu, Dynamics and flow effects in the Beris-Edwards system modelling nematic liquid crystals, Arch. Rational Mech. Anal., 231 (2019), 1217–1267. https://doi.org/10.1007/s00205-018-1297-2 doi: 10.1007/s00205-018-1297-2
![]() |
[30] |
A. Contreras, X. Xu, W. J. Zhang, An elementary proof of eigenvalue preservation for the co-rotational Beris-Edwards system, J. Nonlinear Sci., 29 (2019), 789–801. https://doi.org/10.1007/s00332-018-9503-9 doi: 10.1007/s00332-018-9503-9
![]() |
[31] |
A. C. Murza, A. E. Teruel, A. Zarnescu, Shear flow dynamics in the Beris-Edwards model of nematic liquid crystals, Proc. R. Soc. A, 474 (2018), 20170673. https://doi.org/10.1098/rspa.2017.0673 doi: 10.1098/rspa.2017.0673
![]() |
[32] |
T. Qian, P. Sheng, Generalized hydrodynamic equations for nematic liquid crystals, Phys. Rev. E, 58 (1998), 7475. https://doi.org/10.1103/PhysRevE.58.7475 doi: 10.1103/PhysRevE.58.7475
![]() |
[33] |
F. Leslie, Theory of flow phenomena in liquid crystals, Adv. Liquid Crystals, 4 (1979), 1–81. https://doi.org/10.1016/B978-0-12-025004-2.50008-9 doi: 10.1016/B978-0-12-025004-2.50008-9
![]() |
[34] |
S. R. Li, W. Wang, Rigorous justification of the uniaxial limit from the Qian-Sheng inertial Q-tensor theory to the Ericksen-Leslie theory, SIAM J. Math. Anal., 52 (2020), 4421–4468. https://doi.org/10.1137/19M129200X doi: 10.1137/19M129200X
![]() |
[35] | F. De Anna, A. Zarnescu, Global well-posedness and twist-wave solutions for the inertial Qian-Sheng model of liquid crystals, J. Differ. Equ., 264 (2018), 1080–1118. |
[36] |
J. Ericksen, Twisted waves in liquid crystals, Q. J. Mech. Appl. Math., 21 (1968), 463–465. https://doi.org/10.1093/qjmam/21.4.463 doi: 10.1093/qjmam/21.4.463
![]() |
[37] |
N. Jiang, Y. L. Luo, Y. Ma, S. J. Tang, Entropy inequality and energy dissipation of inertial Qian-Sheng model for nematic liquid crystals, J. Hyperbolic Differ. Equ., 18 (2021), 221–256. https://doi.org/10.1142/S0219891621500065 doi: 10.1142/S0219891621500065
![]() |
[38] |
E. Feireisl, E. Rocca, G. Schimperna, A. Zarnescu, On a hyperbolic system arising in liquid crystals modeling, J. Hyperbolic Differ. Equ., 15 (2018), 15–35. https://doi.org/10.1142/S0219891618500029 doi: 10.1142/S0219891618500029
![]() |
[39] |
G. Chen, T. Huang, W. S. Liu, Poiseuille flow of nematic liquid crystals via the full Ericksen-Leslie model, Arch. Ration. Mech. Anal., 236 (2020), 839–891. https://doi.org/10.1007/s00205-019-01484-4 doi: 10.1007/s00205-019-01484-4
![]() |
[40] |
M. W. Fei, W. Wang, P. W. Zhang, Z. F. Zhang, On the isotropic-nematic phase transition for the liquid crystal, Peking Math. J., 1 (2018), 141–219. https://doi.org/10.1007/s42543-018-0005-3 doi: 10.1007/s42543-018-0005-3
![]() |
[41] |
T. Laux, Y. N. Liu, Nematic-Isotropic Phase Transition in Liquid Crystals: A Variational Derivation of Effective Geometric Motions, Arch. Ration. Mech. Anal., 241 (2021), 1785–1814. https://doi.org/10.1007/s00205-021-01681-0 doi: 10.1007/s00205-021-01681-0
![]() |
[42] |
Y. Y. Cai, J. Shen, X. Xu, A stable scheme and its convergence analysis for a 2D dynamic Q-tensor model of nematic liquid crystals, Math. Mod. Methods Appl. Sci., 27 (2017), 1459–1488. https://doi.org/10.1142/S0218202517500245 doi: 10.1142/S0218202517500245
![]() |
[43] | J. Ball, Analysis of liquid crystals and their defects, Lecture notes of the Scuola Estiva GNFM, Ravello 17–22 Sep. 2018. |
[44] |
J. Ball, A. Majumdar, Nematic liquid crystals: from Maier-Saupe to a continuum theory, Mol. Cryst. Liq. Cryst., 525 (2010), 1–11. https://doi.org/10.1080/15421401003795555 doi: 10.1080/15421401003795555
![]() |
[45] | J. Ball, A. Majumdar, Passage from the mean-field Maier-Saupe to the continuum Landau-de Gennes theory for nematic liquid crystals, work in progress. |
[46] |
J. Ball, A. Zarnescu, Orientability and energy minimization in liquid crystal models, Arch. Ration. Mech. Anal., 202 (2011), 493–535. https://doi.org/10.1007/s00205-011-0421-3 doi: 10.1007/s00205-011-0421-3
![]() |
[47] |
P. Bauman, D. Phillips, Regularity and the behavior of eigenvalues for minimizers of a constrained Q-tensor energy for liquid crystals, Calc. Var. Partial Dif., 55 (2016), 55–81. https://doi.org/10.1007/s00526-016-1009-4 doi: 10.1007/s00526-016-1009-4
![]() |
[48] |
X. F. Chen, X. Xu, Existence and uniqueness of global classical solutions of a gradient flow of the Landau–de Gennes energy, Proc. Amer. Math. Soc., 144 (2016), 1251–1263. https://doi.org/10.1090/proc/12803 doi: 10.1090/proc/12803
![]() |
[49] |
T. A. Davis, E. C. Gartland, Finite element analysis of the Landau-de Gennes minimization problem for liquid crystals, SIAM J. Numer. Anal., 35 (1998), 336–362. https://doi.org/10.1137/S0036142996297448 doi: 10.1137/S0036142996297448
![]() |
[50] | L. C. Evans, O. Kneuss, H. Tran, Partial regularity for minimizers of singular energy functionals, with application to liquid crystal models, Trans. Amer. Math. Soc., 368 (2016), 3389–3413. |
[51] |
E. Feireisl, E. Rocca, G. Schimperna, A. Zarnescu, Evolution of non-isothermal Landau-de Gennes nematic liquid crystals flows with singular potential, Commun. Math. Sci., 12 (2014), 317–343. https://doi.org/10.4310/CMS.2014.v12.n2.a6 doi: 10.4310/CMS.2014.v12.n2.a6
![]() |
[52] |
E. Feireisl, E. Rocca, G. Schimperna, A. Zarnescu, Nonisothermal nematic liquid crystal flows with the Ball-Majumdar free energy, Ann. di Mat. Pura ed Appl., 194 (2015), 1269–1299. https://doi.org/10.1007/s10231-014-0419-1 doi: 10.1007/s10231-014-0419-1
![]() |
[53] |
Z. Y. Geng, J. J. Tong, Regularity of minimizers of a tensor-valued variational obstacle problem in three dimensions, Calc. Var., 59 (2020), Paper No. 57. https://doi.org/10.1007/s00526-020-1717-7 doi: 10.1007/s00526-020-1717-7
![]() |
[54] |
J. R. Huang, S. J. Ding, Global well-posedness for the dynamical Q-tensor model of liquid crystals, Sci. China Math., 58 (2015), 1349–1366. https://doi.org/10.1007/s11425-015-4990-8 doi: 10.1007/s11425-015-4990-8
![]() |
[55] |
T. Huang, N. Zhao, On the regularity of weak small solution of a gradient flow of the Landau–de Gennes energy, Proc. Amer. Math. Soc., 147 (2019), 1687–1698. https://doi.org/10.1090/proc/14337 doi: 10.1090/proc/14337
![]() |
[56] |
J. Katriel, G. Kventsel, G. Luckhurst, T. Sluckin, Free energies in the Landau and molecular field approaches, Liq. Cryst., 1 (1986), 337–355. https://doi.org/10.1080/02678298608086667 doi: 10.1080/02678298608086667
![]() |
[57] |
G. Kitavtsev, J. M. Robbins, V. Slastikov, A. Zarnescu, Liquid crystal defects in the Landau-de Gennes theory in two dimensions-beyond the one-constant approximation, Math. Mod. Methods Appl. Sci., 26 (2016), 2769–2808. https://doi.org/10.1142/S0218202516500664 doi: 10.1142/S0218202516500664
![]() |
[58] |
F. H. Lin, C. Poon, On Ericksen's model for liquid crystals, J. Geom. Anal., 4 (1994), 379–392. https://doi.org/10.1007/BF02921587 doi: 10.1007/BF02921587
![]() |
[59] |
J. Y. Lin, C. Zhou, Existence of solutions to the biaxial nematic liquid crystals with two order parameter tensors, Math. Methods Appl. Sci., 43 (2020), 6430–6453. https://doi.org/10.1002/mma.6387 doi: 10.1002/mma.6387
![]() |
[60] |
Y. N. Liu, X. Y. Lu, X. Xu, Regularity of a gradient flow generated by the anisotropic Landau-de Gennes energy with a singular potential, SIAM J. Math. Anal., 53 (2021), 3338–3365. https://doi.org/10.1137/20M1386499 doi: 10.1137/20M1386499
![]() |
[61] |
Y. N. Liu, W. Wang, On the initial boundary value problem of a Navier-Stokes/Q-tensor model for liquid crystals, Discrete Contin. Dyn. Syst. Ser. B, 23 (2018), 3879–3899. https://doi.org/10.3934/dcdsb.2018115 doi: 10.3934/dcdsb.2018115
![]() |
[62] |
Y. N. Liu, H. Wu, X. Xu, Global well-posedness of the two dimensional Beris-Edwards system with general Laudau-de Gennes free energy, J. Differ. Equ., 267 (2019), 6958–7001. https://doi.org/10.1016/j.jde.2019.07.010 doi: 10.1016/j.jde.2019.07.010
![]() |
[63] |
X. Y. Lu, X. Xu, W. J. Zhang, Blowup rate estimates of a singular potential and its gradient in the Landau-de Gennes theory, Journal of Nonlinear Sci., 32 (2022), 1–30. https://doi.org/10.1007/s00332-021-09761-x doi: 10.1007/s00332-021-09761-x
![]() |
[64] | W. Maier, A. Saupe, A simple molecular statistical theory of the nematic crystalline-liquid phase, I Z Naturf. a, 14 (1959), 882–889. |
[65] |
A. Majumdar, A. Zarnescu, Landau-De Gennes theory of nematic liquid crystals: the Oseen-Frank limit and beyond, Arch. Ration. Mech. Anal., 196 (2010), 227–280. https://doi.org/10.1007/s00205-009-0249-2 doi: 10.1007/s00205-009-0249-2
![]() |
[66] |
C. D. Schimming, J. Vinals, S. W. Walker, Numerical method for the equilibrium configurations of a Maier-Saupe bulk potential in a Q-tensor model of an anisotropic nematic liquid crystal, J. Comput. Phys., 441 (2021), Paper No. 110441. https://doi.org/10.1016/j.jcp.2021.110441 doi: 10.1016/j.jcp.2021.110441
![]() |
[67] |
M. Wilkinson, Strict physicality of global weak solutions of a Navier-Stokes Q-tensor system with singular potential, Arch. Ration. Mech. Anal., 218 (2015), 487–526. https://doi.org/10.1007/s00205-015-0864-z doi: 10.1007/s00205-015-0864-z
![]() |
[68] |
A. Zarnescu, Mathematical problems of nematic liquid crystals: between dynamical and stationary problems, Trans. R. Soc. Lond. Ser. A., 379 (2021), Paper No. 20200432. https://doi.org/10.1098/rsta.2020.0432 doi: 10.1098/rsta.2020.0432
![]() |
1. | Muntaser Safan, Hybrid models for helminth infections with special reference to onchocerciasis, 2020, 178, 03784754, 345, 10.1016/j.matcom.2020.06.023 | |
2. | Muntaser Safan, Impact of reduction in contact time activity of infected individuals on the dynamics and control of directly transmitted respiratory infections in SIR models, 2020, 2020, 1687-1847, 10.1186/s13662-020-02708-8 | |
3. | Muntaser Safan, Kamal Barley, Mohamed M. Elhaddad, Mohamed A. Darwish, Samir H. Saker, Mathematical Analysis of an SIVRWS Model for Pertussis with Waning and Naturally Boosted Immunity, 2022, 14, 2073-8994, 2288, 10.3390/sym14112288 | |
4. | Muntaser Safan, Bayan Humadi, An SIS sex-structured influenza A model with positive case fatality in an open population with varying size, 2024, 21, 1551-0018, 6975, 10.3934/mbe.2024306 | |
5. | Muntaser Safan, Alhanouf Altheyabi, Mathematical Analysis of an Anthroponotic Cutaneous Leishmaniasis Model with Asymptomatic Infection, 2023, 11, 2227-7390, 2388, 10.3390/math11102388 |
State variable | Description |
x1=S1/N | Proportion of susceptible females at time t. |
y1=I1/N | Proportion of infected females at time t. |
z1=R1/N | Proportion of recovered females at time t. |
x2=S2/N | Proportion of susceptible males at time t. |
y2=I2/N | Proportion of infected males at time t. |
z2=R2/N | Proportion of recovered males at time t. |
N1(t) | Total female population size at time t. |
N2(t) | Total male population size at time t. |
N=N1+N2 | Total population size at time t, assumed to be constant. |
Parameters | Description | Value | Dim. | Ref. |
B(t) | Total number of newborns. | – | Time−1 | – |
μ | Per-capita death rate. | 1/70 | Year−1 | [4] |
ˉβ | Per-capita contact rate between individuals | – | Year−1 | – |
β | Per-capita effective contact rate at which susceptible males acquire influenza. | 181.173 | Year−1 | Est. |
γ | Per-capita recovery rate for infected males. | 365/3.38 | Year−1 | [24] |
R0 | The basic reproduction number for model (2.6). | 1.525 | – | [24] |
ψ | Per-capita vaccination rate for susceptible males. | 0.57 | Year−1 | Arbit. |
r1 | The susceptibility of females. | – | – | – |
r2 | The susceptibility of males. | – | – | – |
g=r1/r2 | Relative susceptibility of females with respect to males. | 0.9<1 | – | Arbit.[13] |
a | A rescaling parameter representing the relative recovery rate of infected females with respect to infected males. | 1.1 | – | Arbit. |
e0 | Relative response to vaccination campaigns of females with respect to males. | 0.9 | – | Arbit.[20] |
e1(>e2) | Influenza vaccine efficacy in females. | 0.9 | – | Arbit.[20] |
e2 | Influenza vaccine efficacy in males. | 0.8 | – | Arbit.[20] |
State variable | Description |
x1=S1/N | Proportion of susceptible females at time t. |
y1=I1/N | Proportion of infected females at time t. |
z1=R1/N | Proportion of recovered females at time t. |
x2=S2/N | Proportion of susceptible males at time t. |
y2=I2/N | Proportion of infected males at time t. |
z2=R2/N | Proportion of recovered males at time t. |
N1(t) | Total female population size at time t. |
N2(t) | Total male population size at time t. |
N=N1+N2 | Total population size at time t, assumed to be constant. |
Parameters | Description | Value | Dim. | Ref. |
B(t) | Total number of newborns. | – | Time−1 | – |
μ | Per-capita death rate. | 1/70 | Year−1 | [4] |
ˉβ | Per-capita contact rate between individuals | – | Year−1 | – |
β | Per-capita effective contact rate at which susceptible males acquire influenza. | 181.173 | Year−1 | Est. |
γ | Per-capita recovery rate for infected males. | 365/3.38 | Year−1 | [24] |
R0 | The basic reproduction number for model (2.6). | 1.525 | – | [24] |
ψ | Per-capita vaccination rate for susceptible males. | 0.57 | Year−1 | Arbit. |
r1 | The susceptibility of females. | – | – | – |
r2 | The susceptibility of males. | – | – | – |
g=r1/r2 | Relative susceptibility of females with respect to males. | 0.9<1 | – | Arbit.[13] |
a | A rescaling parameter representing the relative recovery rate of infected females with respect to infected males. | 1.1 | – | Arbit. |
e0 | Relative response to vaccination campaigns of females with respect to males. | 0.9 | – | Arbit.[20] |
e1(>e2) | Influenza vaccine efficacy in females. | 0.9 | – | Arbit.[20] |
e2 | Influenza vaccine efficacy in males. | 0.8 | – | Arbit.[20] |