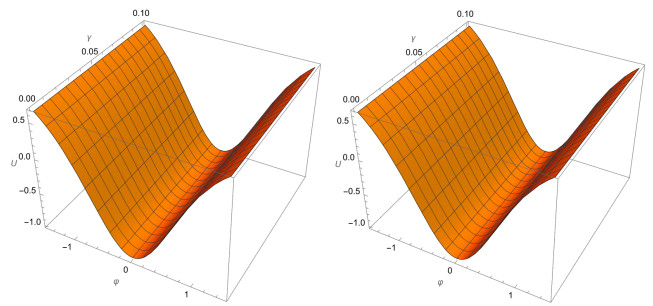
In order to maximize the acquisition of photovoltaic energy when applying photovoltaic systems, the efficiency of photovoltaic system depends on the accuracy of unknown parameters in photovoltaic models. Therefore, it becomes a challenge to extract the unknown parameters in the photovoltaic model. It is well known that the equations of photovoltaic models are nonlinear, and it is very difficult for traditional methods to accurately extract its unknown parameters such as analytical extraction method and key points method. Therefore, with the aim of extracting the parameters of the photovoltaic model more efficiently and accurately, an enhanced hybrid JAYA and Rao-1 algorithm, called EHRJAYA, is proposed in this paper. The evolution strategies of the two algorithms are initially mixed to improve the population diversity and an improved comprehensive learning strategy is proposed. Individuals with different fitness are given different selection probabilities, which are used to select different update formulas to avoid insufficient using of information from the best individual and overusing of information from the worst individual. Therefore, the information of different types of individuals is utilized to the greatest extent. In the improved update strategy, there are two different adaptive coefficient strategies to change the priority of information. Finally, the combination of the linear population reduction strategy and the dynamic lens opposition-based learning strategy, the convergence speed of the algorithm and ability to escape from local optimum can be improved. The results of various experiments prove that the proposed EHRJAYA has superior performance and rank in the leading position among the famous algorithms.
Citation: Yujun Zhang, Yufei Wang, Shuijia Li, Fengjuan Yao, Liuwei Tao, Yuxin Yan, Juan Zhao, Zhengming Gao. An enhanced adaptive comprehensive learning hybrid algorithm of Rao-1 and JAYA algorithm for parameter extraction of photovoltaic models[J]. Mathematical Biosciences and Engineering, 2022, 19(6): 5610-5637. doi: 10.3934/mbe.2022263
[1] | M. Mossa Al-Sawalha, Rasool Shah, Adnan Khan, Osama Y. Ababneh, Thongchai Botmart . Fractional view analysis of Kersten-Krasil'shchik coupled KdV-mKdV systems with non-singular kernel derivatives. AIMS Mathematics, 2022, 7(10): 18334-18359. doi: 10.3934/math.20221010 |
[2] | Ihsan Ullah, Aman Ullah, Shabir Ahmad, Hijaz Ahmad, Taher A. Nofal . A survey of KdV-CDG equations via nonsingular fractional operators. AIMS Mathematics, 2023, 8(8): 18964-18981. doi: 10.3934/math.2023966 |
[3] | Saima Rashid, Rehana Ashraf, Fahd Jarad . Strong interaction of Jafari decomposition method with nonlinear fractional-order partial differential equations arising in plasma via the singular and nonsingular kernels. AIMS Mathematics, 2022, 7(5): 7936-7963. doi: 10.3934/math.2022444 |
[4] | Aslı Alkan, Halil Anaç . The novel numerical solutions for time-fractional Fornberg-Whitham equation by using fractional natural transform decomposition method. AIMS Mathematics, 2024, 9(9): 25333-25359. doi: 10.3934/math.20241237 |
[5] | Rasool Shah, Abd-Allah Hyder, Naveed Iqbal, Thongchai Botmart . Fractional view evaluation system of Schrödinger-KdV equation by a comparative analysis. AIMS Mathematics, 2022, 7(11): 19846-19864. doi: 10.3934/math.20221087 |
[6] | Khalid Khan, Amir Ali, Manuel De la Sen, Muhammad Irfan . Localized modes in time-fractional modified coupled Korteweg-de Vries equation with singular and non-singular kernels. AIMS Mathematics, 2022, 7(2): 1580-1602. doi: 10.3934/math.2022092 |
[7] | Saima Rashid, Sobia Sultana, Bushra Kanwal, Fahd Jarad, Aasma Khalid . Fuzzy fractional estimates of Swift-Hohenberg model obtained using the Atangana-Baleanu fractional derivative operator. AIMS Mathematics, 2022, 7(9): 16067-16101. doi: 10.3934/math.2022880 |
[8] | Muammer Ayata, Ozan Özkan . A new application of conformable Laplace decomposition method for fractional Newell-Whitehead-Segel equation. AIMS Mathematics, 2020, 5(6): 7402-7412. doi: 10.3934/math.2020474 |
[9] | Khalid Khan, Amir Ali, Muhammad Irfan, Zareen A. Khan . Solitary wave solutions in time-fractional Korteweg-de Vries equations with power law kernel. AIMS Mathematics, 2023, 8(1): 792-814. doi: 10.3934/math.2023039 |
[10] | Musawa Yahya Almusawa, Hassan Almusawa . Numerical analysis of the fractional nonlinear waves of fifth-order KdV and Kawahara equations under Caputo operator. AIMS Mathematics, 2024, 9(11): 31898-31925. doi: 10.3934/math.20241533 |
In order to maximize the acquisition of photovoltaic energy when applying photovoltaic systems, the efficiency of photovoltaic system depends on the accuracy of unknown parameters in photovoltaic models. Therefore, it becomes a challenge to extract the unknown parameters in the photovoltaic model. It is well known that the equations of photovoltaic models are nonlinear, and it is very difficult for traditional methods to accurately extract its unknown parameters such as analytical extraction method and key points method. Therefore, with the aim of extracting the parameters of the photovoltaic model more efficiently and accurately, an enhanced hybrid JAYA and Rao-1 algorithm, called EHRJAYA, is proposed in this paper. The evolution strategies of the two algorithms are initially mixed to improve the population diversity and an improved comprehensive learning strategy is proposed. Individuals with different fitness are given different selection probabilities, which are used to select different update formulas to avoid insufficient using of information from the best individual and overusing of information from the worst individual. Therefore, the information of different types of individuals is utilized to the greatest extent. In the improved update strategy, there are two different adaptive coefficient strategies to change the priority of information. Finally, the combination of the linear population reduction strategy and the dynamic lens opposition-based learning strategy, the convergence speed of the algorithm and ability to escape from local optimum can be improved. The results of various experiments prove that the proposed EHRJAYA has superior performance and rank in the leading position among the famous algorithms.
Fractional order Kersten-Krasil'shchik (KK) coupled Korteweg-de Vries (KdV)-modified KdV (mKdV) systems have served as the focus of extensive research in recent years due to their potential use in a variety of disciplines, including fluid dynamics, nonlinear optics and plasma physics [1,2,3,4]. These systems are characterized by the presence of fractional derivatives, which introduce non-local and memory effects into the dynamics of the system [5,6,7,8]. The KK coupled KdV-mKdV system is a system of two coupled nonlinear partial differential equations, which describe the evolution of two waves in a dispersive medium. The first equation is the well-known KdV equation, which describes the propagation of small amplitude, long wavelength waves. The second equation is the mKdV equation, which describes the propagation of larger amplitude, shorter wavelength waves. The KK coupling term, which is a nonlinear and non-local term, describes the interaction between the two waves [3,4,7]. Li et al. delved into an epidemic model's analysis and comparisons with other mechanisms in 2018 [12], while Jin and Wang explored chemotaxis phenomena in 2016 [13]. He et al. focused on fixed-point and variational inequality problems for Hadamard manifolds in 2022 [14]; this was followed by He et al. discussing nonexpansive mapping algorithms in 2023 [15]. Chen et al. contributed to particle physics, discovering hidden-charm pentaquarks in 2021 [16]. Lyu et al. analyzed cavity dynamics in water entries [17], whereas Yang and Kai delved into nonlinear Schr¨odinger equations in 2023.
Time fractional Kersten-Krasil'shchik coupled KdV-mKdV nonlinear system and homogeneous two component time fractional coupled third order KdV systems are very important fractional nonlinear systems for describing the behaviour of waves in multi-component plasma and elaborate various nonlinear phenomena in plasma physics. Other studies have focused on the stability, existence and uniqueness of solutions for KK coupled KdV-mKdV systems of fractional order. In addition to these studies, there have been many other works that have explored the properties of fractional order KK coupled KdV-mKdV systems, such as their integrability, conservation laws and soliton interactions. Overall, the literature on fractional order KK coupled KdV-mKdV systems is rich and diverse, and it continues to grow as researchers explore new properties and applications of these systems [18,19,20].
There are several methods that have been proposed to solve the fractional KdV (fKdV) equation and the mKdV equation. One of these methods is the homotopy perturbation method; this method uses a perturbation series and a homotopy approach to solve nonlinear differential equations. It has been used to find approximate solutions to the fKdV equation [21]. The variational iteration method uses a variation of a trial solution to find approximate solutions to nonlinear differential equations. The homotopy analysis method uses a homotopy approach and a perturbation series to solve nonlinear differential equations. It has been used to find approximate solutions to the fKdV equation [22] and the mKdV equation [23]. The Adomian decomposition method uses a decomposition of the nonlinear term of a differential equation into a series of simpler functions. It has been used to find approximate solutions to the fKdV equation and the mKdV equation [24,25]. Yang and Kai, dynamical properties, modulation instability analysis and chaotic behaviors to the nonlinear coupled Schrodinger equation in fiber Bragg gratings [26]. Chen et al. presented a linear free energy relationship in chemistry in 2020 [27]. Luo et al. proposed a new gradient method for force identification in vehicle-bridge systems in 2022 [28]. Additionally, Chen et al. focused on adaptive control of underwater vehicles in 2022 [29]. Lastly, Gu, Li and Liao developed an evolutionary multitasking approach for solving nonlinear equation systems in 2024 [30]. These studies collectively offer significant insights and advancements across a broad spectrum of scientific research areas, enriching our understanding and methodologies in their respective domains.
The ADM is a powerful technique for solving nonlinear differential equations. Developed by George Adomian in the late 1980s, the ADM is based on the idea of decomposing the solution of a nonlinear equation into a series of simpler functions, known as Adomian polynomials. These polynomials are obtained by iteratively applying the nonlinear operator to a constant function [31,32]. The ADM has been applied to a wide range of nonlinear problems, including partial differential equations, integral equations and stochastic differential equations. One of the key advantages of the ADM is its ability to handle equations with singularities, such as those that arise in physics and engineering. In recent years, researchers have been exploring the use of the ADM in combination with other techniques, such as the ZZ transform. The ZZ transform is a mathematical tool that can be used to transform a nonlinear equation into a linear equation, making it easier to solve. By combining the ADM with the ZZ transform, researchers have been able to solve a wide range of nonlinear problems with greater efficiency and accuracy. Many researchers have used the ADM together with the ZZ transform to solve, for example, the nonlinear fractional partial differential equations in fluid dynamics, nonlinear integral equations in quantum mechanics and nonlinear fractional stochastic differential equations in finance. Overall, the ADM with the ZZ transform has been shown to be an efficient and flexible strategy for addressing nonlinear problems, with numerous potential applications in various fields [33,34].
The current work is organized as follows. In Section 2, some fundamental definitions of fractional calculus are provided. The basic ideas of the Aboodh transform and the ADM are described in Section 3. In Section 4, we build approximate solutions to fractional Kersten-Krasil'shchik coupled KdV-mKdV systems of partial differential equations. Section 5 contains the conclusions.
Definition 2.1. For functions, the Aboodh transformation is achieved as follow:
B={U(ϱ):∃M,n1,n2>0,|U(ϱ)|<Me−εϱ}, |
which is described as follows [33,34]:
A{U(ϱ)}=1ε∫∞0U(ϱ)e−εϱdϱ, ϱ>0 and n1≤ε≤n2. |
Theorem 2.2. Consider G and F as the Aboodh and Laplace transformations, respectively, of U(ϱ) over the set B [35,36]. Then
G(ε)=F(ε)ε. | (2.1) |
Generalizing the Laplace and Aboodh integral transformations, Zain Ul Abadin Zafar created the ZZ transformation [37]. The ZZ transform is described as follows.
Definition 2.3. For all values of ϱ≥0, the Z-transform for the function U(ϱ) is Z(κ,ε), which can be expressed as follows [37]:
ZZ(U(ϱ))=Z(κ,ε)=ε∫∞0U(κϱ)e−εϱdϱ. |
The Z-transform is linear in nature, just as the Laplace and Aboodh transforms. On the other hand, the Mittag-Leffler function (MLF) is an expansion of the exponential function:
Eδ(z)=∞∑m=0zmΓ(1+mδ),Re(δ)>0. |
Definition 2.4. The Atangana-Baleanu-Caputo (ABC) derivative of a function U(φ,ϱ) in the space H1(a,b) for β∈(0,1) has the following definition [38]:
ABCaDβϱU(φ,ϱ)=B(β)−β+1∫ϱaU′(φ,ϱ)Eβ(−β(ϱ−η)β−β+1)dη. |
Definition 2.5. The Atangana-Baleanu Riemann-Liouville (ABR) derivative is a part of the space H1(a,b). The derivative can be represented for any value of β∈(0,1) as follows [38]:
ABRaDβϱU(φ,η)=B(β)−β+1ddϱ∫ϱaU(φ,η)Eβ(−β(ϱ−η)β−β+1)dη. |
The property of the function B(β) is that it tends to 1 for both 0 and 1. Additionally, ∀β>0, B(β)>a.
Theorem 2.6. The Laplace transformation for the ABR derivative and ABC derivative are given by [38]:
L{ABCaDβϱU(φ,ϱ)}(ε)=B(β)−β+1εβL{U(φ,ϱ)}−εβ−1U(φ,0)εβ+β−β+1 | (2.2) |
and
L{ABRaDβϱU(φ,ϱ)}(ε)=B(β)−β+1εβL{U(φ,ϱ)}εβ+β−β+1. | (2.3) |
In the theorems below we assume that U(ϱ)∈H1(a,b), where b>a and β∈(0,1).
Theorem 2.7. The Aboodh transform gives rise to a new ABR derivative, which is known as the Aboodh transformed ABR derivative [36]
G(ε)=A{ABRaDβϱU(φ,ϱ)}(ε)=1ε[B(β)−β+1εβL{U(φ,ϱ)}εβ+β−β+1]. | (2.4) |
Theorem 2.8. The Aboodh transformation of ABC derivative is defined as follows [36]:
G(ε)=A{ABCaDβϱU(φ,ϱ)}(ε)=1ε[B(β)−β+1εβL{U(φ,ϱ)}−εβ−1U(φ,0)εβ+β−β+1]. | (2.5) |
Theorem 2.9. The ZZ transformation of U(ϱ)=ϱβ−1 is defined as
Z(κ,ε)=Γ(β)(κε)β−1. | (2.6) |
Proof. The Aboodh transformation of U(ϱ)=ϱβ, β≥0 is given by
G(ε)=Γ(β)εβ+1. |
Now, G(εκ)=Γ(β)κβ+1εβ+1. |
Applying Eq (2.6), we obtain
Z(κ,ε)=ε2κ2G(εκ)=ε2κ2Γ(β)κβ+1εβ+1=Γ(β)(κε)β−1. |
Theorem 2.10. Let β and ω be complex numbers and assume that the real part of β is greater than 0. The ZZ transformation of Eβ(ωϱβ) can be defined as follows [36]:
ZZ{(Eβ(ωϱβ))}=Z(κ,ε)=(1−ω(κε)β)−1. | (2.7) |
Proof. The Aboodh transformation of Eβ(ωϱβ) is defined as follows:
G(ε)=F(ε)ε=εβ−1ε(εβ−ω). | (2.8) |
So,
G(εκ)=(εκ)β−1(εκ)((εκ)β−ω); | (2.9) |
we obtain
Z(κ,ε)=(εκ)2G(εκ)=(εκ)2(εκ)β−1(εκ)((εκ)β−ω)=(εκ)β(εκ)β−ω=(1−ω(κε)β)−1. |
Theorem 2.11. The ZZ transform of the ABC derivative can be defined as follows: If G(ε) and Z(κ,ε) are the ZZ and Aboodh transformations of U(ϱ), respectively [36], they we have
ZZ{ABC0DβϱU(ϱ)}=[B(β)−β+1εa+2κβ+2G(εκ)−εβκβf(0)εβκβ+β−β+1]. | (2.10) |
Proof. Applying this Eqs (2.1) and (2.5), we get
G(εκ)=κε[B(β)−β+1(εκ)β+1G(εκ)−(εκ)β−1f(0)(εκ)β+β−β+1]. | (2.11) |
The ABC Z transformation is represented as follows:
Z(κ,ε)=(εκ)2G(εκ)=(εκ)2κε[B(β)−β+1(εκ)β+1G(εκ)−(εκ)β−1f(0)(εκ)β+β−β+1]=[B(β)−β+1(εκ)β+2G(εκ)−(εκ)βf(0)(εκ)β+β−β+1]. |
Theorem 2.12. Let us assume that the ZZ transformation of U(ϱ) is represented by G(ε) and the Aboodh transformation of U(ϱ) is represented by Z(κ,ε). Then, the ZZ transformation of the ABR derivative is defined as [36]
ZZ{ABR0Dβϱf(ϱ)}=[B(β)−β+1εβ+2κβ+2G(εκ)εμκμ+β−β+1]. | (2.12) |
Proof. Applying Eqs (2.1) and (2.4), we get
G(εκ)=κε[B(β)−β+1(εκ)β+1G(εκ)(εκ)β+β−β+1]. | (2.13) |
Z(κ,ε)=(εκ)2G(εκ)=(εκ)2(κε)[B(β)−β+1(εκ)β+1G(εκ)(εκ)β+β−β+1]=[B(β)−β+1(εκ)β+2G(εκ)(εκ)β+β−β+1]. |
In this section, we will examine the equation known as the fractional partial differential equation:
Dβ℘U(φ,℘)=L(U(φ,℘))+N(U(φ,℘))+h(φ,℘)=M(φ,℘), | (3.1) |
with the initial condition
U(φ,0)=ϕ(φ), | (3.2) |
where L(φ,℘) represents linear terms, N(φ,℘) represents nonlinear terms and h(φ,℘) represents the source term.
Using the ZZ transform and ABC fractional derivatives, Eq (3.1) can be re-expressed as follows:
1q(β,κ,ε)(Z[U(φ,℘)]−ϕ(φ)ε)=Z[M(φ,℘)], | (3.3) |
with
q(β,κ,ε)=1−β+β(κε)βB(β). | (3.4) |
By taking the inverse ZZ transform, we get
U(φ,℘)=Z−1(ϕ(φ)ε+q(β,κ,ε)Z[M(φ,℘)]). | (3.5) |
In terms of Adomain decomposition, we have
∞∑i=0Ui(φ,℘)=Z−1(ϕ(φ)ε+q(β,κ,ε)Z[h(φ,℘)])+Z−1(q(β,κ,ε)Z[∞∑i=0L(Ui(φ,℘))+A℘]), | (3.6) |
UABC0(φ,℘)=Z−1(ϕ(φ)ε+q(β,κ,ε)Z[h(φ,℘)]),UABC1(φ,℘)=Z−1(q(β,κ,ε)Z[L(U0(φ,℘))+A0]),⋮UABCl+1(φ,℘)=Z−1(q(β,κ,ε)Z[L(Ul(φ,℘))+Al]), l=1,2,3,⋯. | (3.7) |
The solution to Eq (3.1) can be expressed by using ADMABC.
UABC(φ,℘)=UABC0(φ,℘)+UABC1(φ,℘)+UABC2(φ,℘)+⋯. | (3.8) |
Example 4.1. Let us examine the following fractional KK joined KdV-mKdV nonlinear system:
Dβ℘U+U3φ−6UUφ+3VV3φ+3VφV2φ−3UφV2+6UVVφ=0, ℘>0, φ∈R, 0<β≤1,Dβ℘V+V3φ−3V2Vφ−3UVφ+3UφV=0, | (4.1) |
with the initial conditions given by
U(φ,0)=c−2c sech2(√cφ), c>0,V(φ,0)=2√c sech(√cφ). | (4.2) |
By taking the ZZ transform, we get
Z[Dβ℘U(φ,℘)]=−Z[U3φ−6UUφ+3VV3φ+3VφV2φ−3UφV2+6UVVφ],Z[Dβ℘V(φ,℘)]=−Z[V3φ−3V2Vφ−3UVφ+3UφV]. | (4.3) |
Thus we have
1εβZ[U(φ,℘)]−ε2−βU(φ,0)=−Z[U3φ−6UUφ+3VV3φ+3VφV2φ−3UφV2+6UVVφ],1εβZ[V(φ,℘)]−ε2−βU(φ,0)=−Z[V3φ−3V2Vφ−3UVφ+3UφV]. | (4.4) |
By simplification we get
Z[U(φ,℘)]=ε2[c−2c sech2(√cφ)]−(1−β+β(κε)β)B(β)Z[U3φ−6UUφ+3VV3φ+3VφV2φ−3UφV2+6UVVφ],Z[V(φ,℘)]=ε2[2√c sech(√cφ)]−(1−β+β(κε)β)B(β)Z[V3φ−3V2Vφ−3UVφ+3UφV]. | (4.5) |
By taking the inverse ZZ transformation, we have
U(φ,℘)=[c−2c sech2(√cφ)]−Z−1[(1−β+β(κε)β)B(β)Z{U3φ−6UUφ+3VV3φ+3VφV2φ−3UφV2+6UVVφ}],V(φ,℘)=[2√c sech(√cφ)]−Z−1[(1−β+β(κε)β)B(β)Z{V3φ−3V2Vφ−3UVφ+3UφV}]. | (4.6) |
Assume that for the unknown functions U(φ,℘) and V(φ,℘), the series form solution is given as
U(φ,℘)=∞∑l=0Ul(φ,℘),V(φ,℘)=∞∑l=0Ul(φ,℘), | (4.7) |
The nonlinear components of the Adomian polynomials can be represented as follows: −6UUφ+3VV3φ=∑∞m=0Am, 3VφV2φ−3UφV2=∑∞m=0Bm, 6UVVφ=∑∞m=0Cm and −3V2Vφ−3UVφ+3UφV=∑∞m=0Dm. With the help of these terms, Eq (4.6) can be expressed as follows:
∞∑l=0Ul+1(φ,℘)=c−2c sech2(√cφ)−Z−1[(1−β+β(κε)β)B(β)Z{U3φ+∞∑l=0Al+∞∑l=0Bl+∞∑l=0Cl}],∞∑l=0Vl+1(φ,℘)=2√c sech(√cφ)−Z−1[(1−β+β(κε)β)B(β)Z{U3φ+∞∑l=0Dl}]. | (4.8) |
On comparing both sides of Eq (4.8), we have
U0(φ,℘)=c−2c sech2(√cφ),V0(φ,℘)=2√c sech(√cφ), |
U1(φ,℘)=8c52sinh(√cφ) sech3(√cφ)(1−β+β℘βΓ(β+1)),V1(φ,℘)=−4c2sinh(√cφ) sech2(√cφ)(1−β+β℘βΓ(β+1)), | (4.9) |
U2(φ,℘)=−16c4[2cosh2(√cφ)−3] sech4(√cφ)[β2℘2βΓ(2β+1)+2β(1−β)℘βΓ(β+1)+(1−β)2],V2(φ,℘)=8c72[cosh2(√cφ)−2] sech3(√cφ)[β2℘2βΓ(2β+1)+2β(1−β)℘βΓ(β+1)+(1−β)2]. | (4.10) |
In this manner, the terms Ul and Vl for (l≥3) can be easily obtained. As a result, the series solution can be expressed as follows:
U(φ,℘)=∞∑l=0Ul(φ,℘)=U0(φ,℘)+U1(φ,℘)+U2(φ,℘)+⋯,U(φ,℘)=c−2c sech2(√cφ)+8c52sinh(√cφ) sech3(√cφ)(1−β+β℘βΓ(β+1))−16c4[2cosh2(√cφ)−3] sech4(√cφ)[β2℘2βΓ(2β+1)+2β(1−β)℘βΓ(β+1)+(1−β)2]+⋯V(φ,℘)=∞∑l=0Vl(φ,℘)=V0(φ,℘)+V1(φ,℘)+V2(φ,℘)+⋯,V(φ,℘)=2√c sech(√cφ)−4c2sinh(√cφ) sech2(√cφ)(1−β+β℘βΓ(β+1))+8c72[cosh2(√cφ)−2] sech3(√cφ)[β2℘2βΓ(2β+1)+2β(1−β)℘βΓ(β+1)+(1−β)2]+⋯ | (4.11) |
When β=1, we get the exact solution as
U(φ,℘)=c−2c sech2(√c(φ+2c℘)),V(φ,℘)=2√c sech(√c(φ+2c℘)). | (4.12) |
The graphical discussion involves several key figures illustrate the solutions for U(φ,℘) and V(φ,℘) in Example 4.1 at different parameter values. Figure 1 showcases the analytical and exact solutions at β=1 for U(φ,℘). In Figure 2, the approximate solutions are depicted at β=0.8,0.6.
Figure 3 extends the analysis by presenting analytical solutions at various values of β for U(φ,℘). Moving on to V(φ,℘), Figure 4 exhibits the analytical and exact solutions at β=1, while Figure 5 displays the analytical results at β=0.8,0.6.
Lastly, Figure 6 provides a comprehensive overview, presenting analytical results at various values of β, including β=1,0.8,0.6,0.4, for V(φ,℘). These figures collectively offer a detailed visual representation of the solutions under different conditions, facilitating a thorough understanding of the system's behavior.
Example 4.2. Let us examine a homogeneous two-component KdV system of third order with a time-fractional component, as follows:
Dβ℘U−U3φ−UUφ−VVφ=0, ℘>0, φ∈R, 0<β≤1,Dβ℘V+2V3φ−UVφ=0, | (4.13) |
with the initial conditions given by
U(φ,0)=3−6tanh2(φ2),V(φ,0)=−3c√2tanh(φ2). | (4.14) |
By taking the ZZ transform, we get
Z[Dβ℘U(φ,℘)]=−Z[−U3φ−UUφ−VVφ],Z[Dβ℘V(φ,℘)]=−Z[2V3φ−UVφ]. | (4.15) |
Thus we have
1εβZ[U(φ,℘)]−ε2−βU(φ,0)=−Z[−U3φ−UUφ−VVφ],1εβZ[V(φ,℘)]−ε2−βU(φ,0)=−Z[2V3φ−UVφ]. | (4.16) |
By simplification we get
Z[U(φ,℘)]=ε2[3−6tanh2(φ2)]−(1−β+β(κε)β)B(β)Z[−U3φ−UUφ−VVφ],Z[V(φ,℘)]=ε2[−3c√2tanh(φ2)]−(1−β+β(κε)β)B(β)Z[2V3φ−UVφ]. | (4.17) |
By taking the inverse ZZ transform, we have
U(φ,℘)=3−6tanh2(φ2)−Z−1[(1−β+β(κε)β)B(β)Z{−U3φ−UUφ−VVφ}],V(φ,℘)=[−3c√2tanh(φ2)]−Z−1[(1−β+β(κε)β)B(β)Z{2V3φ−UVφ}]. | (4.18) |
Assume that for the unknown functions U(φ,℘) and V(φ,℘), the series form solution is given as
U(φ,℘)=∞∑l=0Ul(φ,℘),V(φ,℘)=∞∑l=0Ul(φ,℘). | (4.19) |
The representation of nonlinear components using Adomian polynomials is shown as follows: −UUφ−VVφ=∑∞m=0Am and UVφ=∑∞m=0Bm. With these terms, Eq (4.18) can be expressed as follows:
∞∑l=0Ul+1(φ,℘)=3−6tanh2(φ2)+Z−1[(1−β+β(κε)β)B(β)Z{−U3φ+∞∑l=0Al}],∞∑l=0Vl+1(φ,℘)=−3c√2tanh(φ2)+Z−1[(1−β+β(κε)β)B(β)Z{2V3φ−∞∑l=0Bl}]. | (4.20) |
On comparing both sides of Eq (4.20), we have
U0(φ,℘)=3−6tanh2(φ2),V0(φ,℘)=−3c√2tanh(φ2), |
U1(φ,℘)=6 sech2(φ2)tanh(φ2)(1−β+β℘βΓ(β+1)),V1(φ,℘)=3c√2 sech2(φ2)tanh(φ2)(1−β+β℘βΓ(β+1)), | (4.21) |
U2(φ,℘)=3[2+7 sech2(φ2)−15 sech4(φ2)] sech2(φ2)[β2℘2βΓ(2β+1)+2β(1−β)℘βΓ(β+1)+(1−β)2],V2(φ,℘)=3c√22[2+21 sech2(φ2)−24 sech4(φ2)] sech2(φ2)[β2℘2βΓ(2β+1)+2β(1−β)℘βΓ(β+1)+(1−β)2]. | (4.22) |
By using this method, the terms Ul and Vl can be easily obtained for l≥3. Therefore, the solution in the form of a series is as follows:
U(φ,℘)=∞∑l=0Ul(φ,℘)=U0(φ,℘)+U1(φ,℘)+U2(φ,℘)+⋯,U(φ,℘)=3−6tanh2(φ2)+6 sech2(φ2)tanh(φ2)(1−β+β℘βΓ(β+1))+3[2+7 sech2(φ2)−15 sech4(φ2)] sech2(φ2)[β2℘2βΓ(2β+1)+2β(1−β)℘βΓ(β+1)+(1−β)2]+⋯.V(φ,℘)=∞∑l=0Vl(φ,℘)=V0(φ,℘)+V1(φ,℘)+V2(φ,℘)+⋯,V(φ,℘)=−3c√2tanh(φ2)+3c√2 sech2(φ2)tanh(φ2)(1−β+β℘βΓ(β+1))+3c√22[2+21 sech2(φ2)−24 sech4(φ2)] sech2(φ2)[β2℘2βΓ(2β+1)+2β(1−β)℘βΓ(β+1)+(1−β)2]+⋯. | (4.23) |
When β=1, we get the exact solution as
U(φ,℘)=3−6tanh2(φ+℘2),V(φ,℘)=−3c√2tanh(φ+℘2). | (4.24) |
The graphical discussion involves several key figures that illustrate the solutions for U(φ,℘) and V(φ,℘) in Example 4.2 at different parameter values. Figure 7 showcases the analytical and exact solutions at β=1 for U(φ,℘). In Figure 8, the approximate solutions are depicted at β=0.8,0.6.
Figure 9 extends the analysis by presenting analytical solutions at various values of β for U(φ,℘). We will moving on to V(φ,℘).
Figure 10 exhibits analytical and exact solutions at β=1, while Figure 11 displays the analytical results at β=0.8,0.6. Lastly, Figure 12 provides a comprehensive overview, presenting the analytical results at various values of β, including β=1,0.8,0.6,0.4, for V(φ,℘). These figures collectively offer a detailed visual representation of the solutions under different conditions, facilitating a thorough understanding of the system's behavior.
In summary, the combination of the ADM and the ZZ transform has demonstrated its effectiveness in the analysis of the fractional KK coupled KdV-mKdV system encountered in multi-component plasmas. The utilization of this method has yielded accurate and efficient solutions, offering valuable insights into the intricate behavior of these complex systems. Additionally, the incorporation of the ZZ transform has enabled this frequency domain analysis, contributing supplementary information regarding the system's dynamics. This integrated approach stands as a valuable tool for comprehending multi-component plasma behaviors, and it holds the potential for application to analogous systems in future investigations. Future work may explore extending this methodology to different plasma models or investigating the impact of additional physical parameters, thereby broadening the scope of its applicability.
The authors declare they have not used Artificial Intelligence (AI) tools in the creation of this article.
This work was supported by the Deanship of Scientific Research, the Vice Presidency for Graduate Studies and Scientific Research, King Faisal University, Saudi Arabia (Grant No. 5081).
This work was supported by the Deanship of Scientific Research, the Vice Presidency for Graduate Studies and Scientific Research, King Faisal University, Saudi Arabia (Grant No. 5081).
The authors declare that they have no competing interests.
[1] |
S. Li, W. Gong, X. Yan, C. Hu, D. Bai, L. Wang, Parameter estimation of photovoltaic models with memetic adaptive differential evolution, Sol. Energy, 190 (2019), 465–474. https://doi.org/10.1016/j.solener.2019.08.022 doi: 10.1016/j.solener.2019.08.022
![]() |
[2] |
Z. Liao, Q. Gu, S. Li, Z. Hu, B. Ning, An improved differential evolution to extract photovoltaic cell parameters, IEEE Access, 8 (2020), 177838–177850. https://doi.org/10.1109/ACCESS.2020.3024975 doi: 10.1109/ACCESS.2020.3024975
![]() |
[3] |
S. Li, Q. Gu, W. Gong, B. Ning, An enhanced adaptive differential evolution algorithm for parameter extraction of photovoltaic models, Energy Convers. Manage., 205 (2020), 112443. https://doi.org/10.1016/j.enconman.2019.112443 doi: 10.1016/j.enconman.2019.112443
![]() |
[4] |
Z. Liao, Z. Chen, S. Li, Parameters Extraction of Photovoltaic Models Using Triple-Phase Teaching-Learning-Based Optimization, IEEE Access, 8 (2020), 69937-69952. https://doi.org/10.1109/ACCESS.2020.2984728 doi: 10.1109/ACCESS.2020.2984728
![]() |
[5] |
H. M. Ridha, H. Hizam, C. Gomes, A. A. Heidari, H. Chen, M. Ahmadipour, et al., Parameters extraction of three diode photovoltaic models using boosted LSHADE algorithm and Newton Raphson method, Energy, 224 (2021), 120136. https://doi.org/10.1016/j.energy.2021.120136 doi: 10.1016/j.energy.2021.120136
![]() |
[6] |
S. Li, W. Gong, Q. Gu, A comprehensive survey on meta-heuristic algorithms for parameter extraction of photovoltaic models, Renewable Sustainable Energy Rev., 141 (2021), 110828. https://doi.org/10.1016/j.rser.2021.110828 doi: 10.1016/j.rser.2021.110828
![]() |
[7] |
D. Chan, J. Phang, Analytical methods for the extraction of solar-cell single- and double-diode model parameters from Ⅰ-Ⅴ characteristics, IEEE Trans. Electron Devices, 34 (1987), 286-293. https://doi.org/10.1109/T-ED.1987.22920 doi: 10.1109/T-ED.1987.22920
![]() |
[8] |
H. Saleem, S. Karmalkar, An Analytical Method to Extract the Physical Parameters of a Solar Cell From Four Points on the Illuminated, IEEE Electron Device Lett.s, 30 (2009), 349–352. https://doi.org/10.1109/LED.2009.2013882 doi: 10.1109/LED.2009.2013882
![]() |
[9] |
T. Easwarakhanthan, J. Bottin, I. Bouhouch, C. Boutrit, Nonlinear minimization algorithm for determining the solar cell parameters with microcomputers, Int. J. Sol. Energy, 4 (1986), 1–12. https://doi.org/10.1080/01425918608909835 doi: 10.1080/01425918608909835
![]() |
[10] |
A. Ortiz-Conde, F. J. García Sánchez, J. Muci, New method to extract the model parameters of solar cells from the explicit analytic solutions of their illuminated Ⅰ–Ⅴ characteristics, Sol. Energy Mate. Sol. Cells, 90 (2006), 352–361. https://doi.org/10.1016/j.solmat.2005.04.023 doi: 10.1016/j.solmat.2005.04.023
![]() |
[11] |
R. Messaoud, Extraction of uncertain parameters of single-diode model of a photovoltaic panel using simulated annealing optimization, Energy Rep., 6 (2020), 350–357. https://doi.org/10.1016/j.egyr.2020.01.016 doi: 10.1016/j.egyr.2020.01.016
![]() |
[12] |
M. Abd Elaziz, D. Oliva, Parameter estimation of solar cells diode models by an improved opposition-based whale optimization algorithm, Energy Convers. Manage., 171 (2018), 1843–1859. https://doi.org/10.1016/j.enconman.2018.05.062. doi: 10.1016/j.enconman.2018.05.062
![]() |
[13] |
J. Liang, K. Qiao, M. Yuan, K. Yu, B. Qu, S. Ge, et al., Evolutionary multi-task optimization for parameters extraction of photovoltaic models, Energy Convers. Manage., 207 (2020), 112509. https://doi.org/10.1016/j.enconman.2020.112509 doi: 10.1016/j.enconman.2020.112509
![]() |
[14] |
A. Askarzadeh, A. Rezazadeh, Parameter identification for solar cell models using harmony search-based algorithms, Sol. Energy, 86 (2012), 3241–3249. https://doi.org/10.1016/j.solener.2012.08.018 doi: 10.1016/j.solener.2012.08.018
![]() |
[15] |
T. Kang, J. Yao, M. Jin, S. Yang, T. Duong, A Novel Improved Cuckoo Search Algorithm for Parameter Estimation of Photovoltaic (PV) Models, Energies, 11 (2018), 1–31. https://doi.org/10.3390/en11051060 doi: 10.3390/en11051060
![]() |
[16] |
M. R. AlRashidi, M. F. AlHajri, K. M. El-Naggar, A. K. Al-Othman, A new estimation approach for determining the Ⅰ–Ⅴ characteristics of solar cells, Sol. Energy, 85 (2011), 1543–1550. https://doi.org/10.1016/j.solener.2011.04.013 doi: 10.1016/j.solener.2011.04.013
![]() |
[17] |
A. Askarzadeh, A. Rezazadeh, Artificial bee swarm optimization algorithm for parameters identification of solar cell models, Appl. Energy, 102 (2013), 943–949. https://doi.org/10.1016/j.apenergy.2012.09.052 doi: 10.1016/j.apenergy.2012.09.052
![]() |
[18] |
S. Li, W. Gong, L. Wang, X. Yan, C. Hu, A hybrid adaptive teaching–learning-based optimization and differential evolution for parameter identification of photovoltaic models, Energy Convers. Manage., 225 (2020), 113474. https://doi.org/10.1016/j.enconman.2020.113474 doi: 10.1016/j.enconman.2020.113474
![]() |
[19] |
G. Kanimozhi, K. Harish, Modeling of solar cell under different conditions by Ant Lion Optimizer with LambertW function, Appl. Soft Comput., 71 (2018), 141–151. https://doi.org/10.1016/j.asoc.2018.06.025. doi: 10.1016/j.asoc.2018.06.025
![]() |
[20] |
H. M. Ridha, H. Hizam, S. Mirjalili, M. L. Othman, M. E. Ya'acob, L. Abualigah, A novel theoretical and practical methodology for extracting the parameters of the single and double diode photovoltaic models, IEEE Access, 10 (2022), 11110–11137. https://doi.org/10.1109/ACCESS.2022.3142779 doi: 10.1109/ACCESS.2022.3142779
![]() |
[21] |
W. Zhou, P. Wang, A. A. Heidari, X. Zhao, H. Turabieh, M. Mafarja, et al., Metaphor-free dynamic spherical evolution for parameter estimation of photovoltaic modules, Energy Rep., 7 (2021), 5175–5202. https://doi.org/10.1016/j.egyr.2021.07.041 doi: 10.1016/j.egyr.2021.07.041
![]() |
[22] |
A. Farah, A. Belazi, F. Benabdallah, A. Almalaq, M. Chtourou, M.A. Abido, Parameter extraction of photovoltaic models using a comprehensive learning Rao-1 algorithm, Energy Convers. Manage., 252 (2022), 115057. https://doi.org/10.1016/j.enconman.2021.115057 doi: 10.1016/j.enconman.2021.115057
![]() |
[23] |
J. Luo, J. Zhou, X. Jiang, A Modification of the Imperialist Competitive Algorithm With Hybrid Methods for Constrained Optimization Problems, IEEE Access, 9 (2021), 161745–161760. https://doi.org/10.1109/ACCESS.2021.3133579 doi: 10.1109/ACCESS.2021.3133579
![]() |
[24] |
M. Sattar, A. Al Sumaiti, H. Ali, A. Diab, Marine predators algorithm for parameters estimation of photovoltaic modules considering various weather conditions, Neural Comput. Appl., 33 (2021), 11799–11819. https://doi.org/10.1007/s00521-021-05822-0 doi: 10.1007/s00521-021-05822-0
![]() |
[25] |
S. Jiao, G. Chong, C. Huang, H. Hu, M. Wang, A. A. Heidari, et al., Orthogonally adapted Harris hawks optimization for parameter estimation of photovoltaic models, Energy, 203 (2020), 117804. https://doi.org/10.1016/j.energy.2020.117804 doi: 10.1016/j.energy.2020.117804
![]() |
[26] |
H. Shaban, E. H. Houssein, M. Pérez-Cisneros, D. Oliva, A. Y. Hassan, A. Ismaeel, et al., Identification of parameters in photovoltaic models through a runge kutta optimizer, Mathematics, 9 (2021). https://doi.org/10.3390/math9182313 doi: 10.3390/math9182313
![]() |
[27] |
A. Ramadan, S. Kamel, M. H. Hassan, E. M. Ahmed, H. M. Hasanien, Accurate photovoltaic models based on an adaptive opposition artificial hummingbird algorithm, Electronics, 11 (2022). https://doi.org/10.3390/electronics11030318 doi: 10.3390/electronics11030318
![]() |
[28] |
A. Al-Shamma'a, H. Omotoso, F. Alturki, H. Farh, A. Alkuhayli, K. Alsharabi, et al., Parameter estimation of photovoltaic cell/modules using bonobo optimizer, Energies, 15 (2022), 140. https://doi.org/10.3390/en15010140 doi: 10.3390/en15010140
![]() |
[29] |
Y. Chen, D. Pi, B. Wang, J. Chen, Y. Xu, Bi-subgroup optimization algorithm for parameter estimation of a PEMFC model, Expert Syst. Appl., 196 (2022), 116646. https://doi.org/10.1016/j.eswa.2022.116646 doi: 10.1016/j.eswa.2022.116646
![]() |
[30] |
H. Rezk, S. Ferahtia, A. Djeroui, A. Chouder, A. Houari, M. Machmoum, et al., Optimal parameter estimation strategy of PEM fuel cell using gradient-based optimizer, Energy, 239 (2022), 122096. https://doi.org/10.1016/j.energy.2021.122096 doi: 10.1016/j.energy.2021.122096
![]() |
[31] | H. Rezk, T. S. Babu, M. Al-Dhaifallah, H.A. Ziedan, A robust parameter estimation approach based on stochastic fractal search optimization algorithm applied to solar PV parameters, Energy Rep., 7 (2021), 620–640. doi: DOI:10.1016/j.egyr.2021.01.024 |
[32] |
R. V. Rao, Jaya: A simple and new optimization algorithm for solving constrained and unconstrained optimization problems, Int. J. Ind. Eng. Comput., 7 (2016), 19–34. https://doi.org/10.5267/j.ijiec.2015.8.004 doi: 10.5267/j.ijiec.2015.8.004
![]() |
[33] |
R. V. Rao, Rao algorithms: three metaphor-less simple algorithms for solving optimization problems, Int. J. Ind. Eng. Comput., 11 (2020), 107–130. https://doi.org/10.5267/j.ijiec.2019.6.002 doi: 10.5267/j.ijiec.2019.6.002
![]() |
[34] | Y. Zhang, Z. Jin, Comprehensive learning Jaya algorithm for engineering design optimization problems, J. Intell. Manuf., https://doi.org/10.1007/s10845-020-01723-6 |
[35] |
Y. Zhang, A. Chi, S. Mirjalili, Enhanced Jaya algorithm: A simple but efficient optimization method for constrained engineering design problems, Knowl. Based Syst., 233 (2021), 107555. https://doi.org/10.1016/j.knosys.2021.107555 doi: 10.1016/j.knosys.2021.107555
![]() |
[36] |
M. Afifi, H. Rezk, M. Ibrahim, M. El-Nemr, Multi-objective optimization of switched reluctance machine design using Jaya algorithm (MO-Jaya), Mathematics, 9 (2021), 1107. https://doi.org/10.3390/math9101107 doi: 10.3390/math9101107
![]() |
[37] |
S. Basak, B. Bhattacharyya, B. Dey, Combined economic emission dispatch on dynamic systems using hybrid CSA-JAYA Algorithm, Int. J. Syst. Assur. Eng. Manage., (2022). https://doi.org/10.1007/s13198-022-01635-z doi: 10.1007/s13198-022-01635-z
![]() |
[38] |
D. Saadaoui, M. Elyaqouti, K. Assalaou, D. B. hmamou, S. Lidaighbi, Multiple learning JAYA algorithm for parameters identifying of photovoltaic models, Mater. Today Proc., (2021). https://doi.org/10.1016/j.matpr.2021.11.106 doi: 10.1016/j.matpr.2021.11.106
![]() |
[39] |
L. Wang, Z. Wang, H. Liang, C. Huang, Parameter estimation of photovoltaic cell model with Rao-1 algorithm, Optik, 210 (2020), 163846. https://doi.org/10.1016/j.ijleo.2019.163846 doi: 10.1016/j.ijleo.2019.163846
![]() |
[40] |
K. Junhua, L. Shuijia, G. Wenyin, Photovoltaic models parameter estimation via an enhanced Rao-1 algorithm, Math. Biosci. Eng., 19 (2022), 1128–1153. https://doi.org/10.3934/mbe.2022052 doi: 10.3934/mbe.2022052
![]() |
[41] |
L. Bhukya, A. Annamraju, S. Nandiraju, A novel maximum power point tracking technique based on Rao-1 algorithm for solar PV system under partial shading conditions, Int. Trans. Electr. Energy Syst., 31 (2021), e13028. https://doi.org/10.1002/2050-7038.13028 doi: 10.1002/2050-7038.13028
![]() |
[42] |
X. Yu, X. Wu, W. Luo, Parameter Identification of Photovoltaic Models by Hybrid Adaptive JAYA Algorithm, Mathematics, 10 (2022), 183. https://doi.org/10.3390/math10020183 doi: 10.3390/math10020183
![]() |
[43] |
X. Yang, W. Gong, Opposition-based JAYA with population reduction for parameter estimation of photovoltaic solar cells and modules, Appl. Soft Comput., 104 (2021), 107218. https://doi.org/10.1016/j.asoc.2021.107218 doi: 10.1016/j.asoc.2021.107218
![]() |
[44] |
E. H. Houssein, E. Çelik, M. A. Mahdy, R. M. Ghoniem, Self-adaptive Equilibrium Optimizer for solving global, combinatorial, engineering, and Multi-Objective problems, Expert Syst. Appl., 195 (2022), 116552. https://doi.org/10.1016/j.eswa.2022.116552 doi: 10.1016/j.eswa.2022.116552
![]() |
[45] |
Y.Xiao, X. Sun, Y. Guo, S. Li, Y. Zhang, Y. Wang, An improved gorilla troops optimizer based on Lens opposition-based learning and adaptive β-Hill climbing for global optimization, Comput. Model. Eng. Sci. 131 (2022). https://doi.org/10.32604/cmes.2022.019198 doi: 10.32604/cmes.2022.019198
![]() |
[46] |
A. W. Mohamed, A. K. Mohamed, Adaptive guided differential evolution algorithm with novel mutation for numerical optimization, Int. J. Mach. Learn. Cybern., 10 (2019), 253–277. https://doi.org/10.1007/s13042-017-0711-7 doi: 10.1007/s13042-017-0711-7
![]() |
[47] |
K. Yu, J. J. Liang, B. Y. Qu, Z. Cheng, H. Wang, Multiple learning backtracking search algorithm for estimating parameters of photovoltaic models, Appl. Energy, 226 (2018), 408–422. https://doi.org/10.1016/j.apenergy.2018.06.010 doi: 10.1016/j.apenergy.2018.06.010
![]() |
[48] |
X. Xia, L. Gui, F. Yu, H. Wu, B. Wei, Y.L. Zhang, et al., Triple Archives Particle Swarm Optimization, IEEE Trans. Cybern., 50 (2020), 4862–4875. https://doi.org/10.1109/TCYB.2019.2943928 doi: 10.1109/TCYB.2019.2943928
![]() |
[49] |
X. Chen, K. Yu, W. Du, W. Zhao, G. Liu, Parameters identification of solar cell models using generalized oppositional teaching learning based optimization, Energy, 99 (2016), 170–180. https://doi.org/10.1016/j.energy.2016.01.052 doi: 10.1016/j.energy.2016.01.052
![]() |
[50] |
K. Yu, J. J. Liang, B. Y. Qu, X. Chen, H. Wang, Parameters identification of photovoltaic models using an improved JAYA optimization algorithm, Energy Convers.Manage., 150 (2017), 742–753. https://doi.org/10.1016/j.enconman.2017.08.063. doi: 10.1016/j.enconman.2017.08.063
![]() |
[51] |
S. Li, W. Gong, X. Yan, C. Hu, D. Bai, L. Wang, et al., Parameter extraction of photovoltaic models using an improved teaching-learning-based optimization, Energy Convers.Manage., 186 (2019), 293–305. https://doi.org/10.1016/j.enconman.2019.02.048 doi: 10.1016/j.enconman.2019.02.048
![]() |
[52] |
K. Yu, B. Qu, C. Yue, S. Ge, X. Chen, J. Liang, A performance-guided JAYA algorithm for parameters identification of photovoltaic cell and module, Appl. Energy, 237 (2019), 241–257. https://doi.org/10.1016/j.apenergy.2019.01.008 doi: 10.1016/j.apenergy.2019.01.008
![]() |
[53] |
X. Chen, B. Xu, C. Mei, Y. Ding, K. Li, Teaching–learning–based artificial bee colony for solar photovoltaic parameter estimation, Appl. Energy, 212 (2018), 1578–1588. https://doi.org/10.1016/j.apenergy.2017.12.115 doi: 10.1016/j.apenergy.2017.12.115
![]() |
[54] |
D. H. Wolpert, W. G. Macready, No free lunch theorems for optimization, IEEE Trans. Evol. Comput., 1 (1997), 67–82. https://doi.org/10.1109/4235.585893 doi: 10.1109/4235.585893
![]() |
[55] |
K. Yu, X. Chen, X. Wang, Z. Wang, Parameters identification of photovoltaic models using self-adaptive teaching-learning-based optimization, Energy Convers. Manage. 145 (2017), 233–246. https://doi.org/10.1016/j.enconman.2017.04.054 doi: 10.1016/j.enconman.2017.04.054
![]() |
[56] |
D. Oliva, M. Abd El Aziz, A. Ella Hassanien, Parameter estimation of photovoltaic cells using an improved chaotic whale optimization algorithm, Appl. Energy, 200 (2017), 141–154. https://doi.org/10.1016/j.apenergy.2017.05.029 doi: 10.1016/j.apenergy.2017.05.029
![]() |
[57] |
G. Xiong, J. Zhang, X. Yuan, D. Shi, Y. He, G. Yao, Parameter extraction of solar photovoltaic models by means of a hybrid differential evolution with whale optimization algorithm, Sol. Energy, 176 (2018), 742–761. https://doi.org/10.1016/j.solener.2018.10.050 doi: 10.1016/j.solener.2018.10.050
![]() |
[58] |
X. Chen, K. Yu, Hybridizing cuckoo search algorithm with biogeography-based optimization for estimating photovoltaic model parameters, Sol. Energy, 180 (2019), 192–206. https://doi.org/10.1016/j.solener.2019.01.025. doi: 10.1016/j.solener.2019.01.025
![]() |
[59] |
S. M. Ebrahimi, E. Salahshour, M. Malekzadeh, G. Francisco, Parameters identification of PV solar cells and modules using flexible particle swarm optimization algorithm, Energy, 179 (2019), 358–372. https://doi.org/10.1016/j.energy.2019.04.218 doi: 10.1016/j.energy.2019.04.218
![]() |
[60] |
N. Pourmousa, S. M. Ebrahimi, M. Malekzadeh, M. Alizadeh, Parameter estimation of photovoltaic cells using improved Lozi map based chaotic optimization Algorithm, Sol. Energy, 180 (2019), 180–191. https://doi.org/10.1016/j.solener.2019.01.026 doi: 10.1016/j.solener.2019.01.026
![]() |
[61] |
Y. Zhang, C. Huang, Z. Jin, Backtracking search algorithm with reusing differential vectors for parameter identification of photovoltaic models, Energy Convers. Manage., 223 (2020), 113266. https://doi.org/10.1016/j.enconman.2020.113266 doi: 10.1016/j.enconman.2020.113266
![]() |
[62] |
J. Liang, S. Ge, B. Qu, K. Yu, F. Liu, H. Yang, et al., Classified perturbation mutation based particle swarm optimization algorithm for parameters extraction of photovoltaic models, Energy Convers. Manage., 203 (2020), 112138. https://doi.org/10.1016/j.enconman.2019.112138 doi: 10.1016/j.enconman.2019.112138
![]() |
[63] | X. Lin, Y. Wu, Parameters identification of photovoltaic models using niche-based particle swarm optimization in parallel computing architecture, Energy, 196 (2020), 117054. doi: DOI:10.1016/j.energy.2020.117054 |
[64] |
Y. Zhang, M. Ma, Z. Jin, Backtracking search algorithm with competitive learning for identification of unknown parameters of photovoltaic systems, Expert Syst. Appl., 160 (2020), 113750. https://doi.org/10.1016/j.eswa.2020.113750 doi: 10.1016/j.eswa.2020.113750
![]() |
[65] |
W. Long, T. Wu, M. Xu, M. Tang, S. Cai, Parameters identification of photovoltaic models by using an enhanced adaptive butterfly optimization algorithm, Energy, 229 (2021), 120750. https://doi.org/10.1016/j.energy.2021.120750 doi: 10.1016/j.energy.2021.120750
![]() |
[66] |
Y. Liu, A. A. Heidari, X. Ye, C. Chi, X. Zhao, C. Ma, et al., Evolutionary shuffled frog leaping with memory pool for parameter optimization, Energy Rep., 7 (2021), 584–606. https://doi.org/10.1016/j.egyr.2021.01.001 doi: 10.1016/j.egyr.2021.01.001
![]() |
[67] |
Z. Hu, W. Gong, S. Li, Reinforcement learning-based differential evolution for parameters extraction of photovoltaic models, Energy Rep., 7 (2021), 916–928. https://doi.org/10.1016/j.egyr.2021.01.096 doi: 10.1016/j.egyr.2021.01.096
![]() |
[68] |
Y. Zhang, M. Ma, Z. Jin, Comprehensive learning Jaya algorithm for parameter extraction of photovoltaic models, Energy, 211 (2020), 118644. https://doi.org/10.1016/j.energy.2020.118644 doi: 10.1016/j.energy.2020.118644
![]() |
[69] |
M. Abdel-Basset, R. Mohamed, S. Mirjalili, R. K. Chakrabortty, M. J. Ryan, Solar photovoltaic parameter estimation using an improved equilibrium optimizer, Sol. Energy, 209 (2020), 694–708. https://doi.org/10.1016/j.solener.2020.09.032 doi: 10.1016/j.solener.2020.09.032
![]() |
[70] |
M. Abdel-Basset, R. Mohamed, R. K. Chakrabortty, K. Sallam, M. J. Ryan, An efficient teaching-learning-based optimization algorithm for parameters identification of photovoltaic models: Analysis and validations, Energy Convers. Manage., 227 (2021), 113614. https://doi.org/10.1016/j.enconman.2020.113614 doi: 10.1016/j.enconman.2020.113614
![]() |
[71] |
X. Jian, Y. Zhu, Parameters identification of photovoltaic models using modified Rao-1 optimization algorithm, Optik, 231 (2021), 166439. https://doi.org/10.1016/j.ijleo.2021.166439 doi: 10.1016/j.ijleo.2021.166439
![]() |
[72] |
A. M. Beigi, A. Maroosi, Parameter identification for solar cells and module using a Hybrid Firefly and Pattern Search Algorithms, Sol. Energy, 171 (2018), 435–446. https://doi.org/10.1016/j.solener.2018.06.092 doi: 10.1016/j.solener.2018.06.092
![]() |
[73] |
L. Deotti, J. Pereira, I. d. Silva Júnior, Parameter extraction of photovoltaic models using an enhanced Lévy flight bat algorithm, Energy Convers. Manage., 221 (2020), 113114. https://doi.org/10.1016/j.enconman.2020.113114 doi: 10.1016/j.enconman.2020.113114
![]() |
1. | M. Sivakumar, M. Mallikarjuna, R. Senthamarai, A kinetic non-steady state analysis of immobilized enzyme systems with external mass transfer resistance, 2024, 9, 2473-6988, 18083, 10.3934/math.2024882 | |
2. | Sajad Iqbal, Francisco Martínez, An innovative approach to approximating solutions of fractional partial differential equations, 2024, 99, 0031-8949, 065259, 10.1088/1402-4896/ad4928 | |
3. | Musawa Yahya Almusawa, Hassan Almusawa, Dark and bright soliton phenomena of the generalized time-space fractional equation with gas bubbles, 2024, 9, 2473-6988, 30043, 10.3934/math.20241451 | |
4. | Nazik J. Ahmed, Abdulghafor M. Al-Rozbayani, 2024, Chapter 43, 978-3-031-70923-4, 571, 10.1007/978-3-031-70924-1_43 | |
5. | Panpan Wang, Xiufang Feng, Shangqin He, Lie Symmetry Analysis of Fractional Kersten–Krasil’shchik Coupled KdV–mKdV System, 2025, 24, 1575-5460, 10.1007/s12346-024-01152-3 | |
6. | Khudhayr A. Rashedi, Musawa Yahya Almusawa, Hassan Almusawa, Tariq S. Alshammari, Adel Almarashi, Lump-type kink wave phenomena of the space-time fractional phi-four equation, 2024, 9, 2473-6988, 34372, 10.3934/math.20241637 | |
7. | Manohar R. Gombi, B. J. Gireesha, P. Venkatesh, M. L. Keerthi, G. K. Ramesh, Fractional-order energy equation of a fully wet longitudinal fin with convective–radiative heat exchange through Sumudu transform analysis, 2025, 29, 1385-2000, 10.1007/s11043-025-09773-0 | |
8. | Galal M. Moatimid, Mona A. A. Mohamed, Khaled Elagamy, Ahmed A. Gaber, Analysis of Eyring–Powell couple stress nanofluid flow with motile microorganisms over a rough sphere: Modified Adomian decomposition, 2025, 105, 0044-2267, 10.1002/zamm.202400981 |