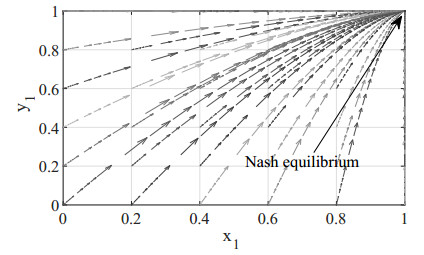
Background: Calcific aortic valve stenosis (CAVS) is a crucial cardiovascular disease facing aging societies. Our research attempts to identify immune-related genes through bioinformatics and machine learning analysis. Two machine learning strategies include Least Absolute Shrinkage Selection Operator (LASSO) and Support Vector Machine Recursive Feature Elimination (SVM-RFE). In addition, we deeply explore the role of immune cell infiltration in CAVS, aiming to study the potential therapeutic targets of CAVS and explore possible drugs. Methods: Download three data sets related to CAVS from the Gene Expression Omnibus. Gene set variation analysis (GSVA) looks for potential mechanisms, determines differentially expressed immune-related genes (DEIRGs) by combining the ImmPort database with CAVS differential genes, and explores the functions and pathways of enrichment. Two machine learning methods, LASSO and SVM-RFE, screen key immune signals and validate them in external data sets. Single-sample GSEA (ssGSEA) and CIBERSORT analyze the subtypes of immune infiltrating cells and integrate the analysis with DEIRGs and key immune signals. Finally, the possible targeted drugs are analyzed through the Connectivity Map (CMap). Results: GSVA analysis of the gene set suggests that it is highly correlated with multiple immune pathways. 266 differential genes (DEGs) integrate with immune genes to obtain 71 DEIRGs. Enrichment analysis found that DEIRGs are related to oxidative stress, synaptic membrane components, receptor activity, and a variety of cardiovascular diseases and immune pathways. Angiotensin II Receptor Type 1(AGTR1), Phospholipid Transfer Protein (PLTP), Secretogranin II (SCG2) are identified as key immune signals of CAVS by machine learning. Immune infiltration found that B cells naï ve and Macrophages M2 are less in CAVS, while Macrophages M0 is more in CAVS. Simultaneously, AGTR1, PLTP, SCG2 are highly correlated with a variety of immune cell subtypes. CMap analysis found that isoliquiritigenin, parthenolide, and pyrrolidine-dithiocarbamate are the top three targeted drugs related to CAVS immunity. Conclusion: The key immune signals, immune infiltration and potential drugs obtained from the research play a vital role in the pathophysiological progress of CAVS.
Citation: Chenyang Jiang, Weidong Jiang. AGTR1, PLTP, and SCG2 associated with immune genes and immune cell infiltration in calcific aortic valve stenosis: analysis from integrated bioinformatics and machine learning[J]. Mathematical Biosciences and Engineering, 2022, 19(4): 3787-3802. doi: 10.3934/mbe.2022174
[1] | Huanhuan Guo, Biao Gao . Game theory analysis of self-awareness and politeness. Mathematical Biosciences and Engineering, 2022, 19(10): 10493-10532. doi: 10.3934/mbe.2022491 |
[2] | Stephen Tully, Monica-Gabriela Cojocaru, Chris T. Bauch . Multiplayer games and HIV transmission via casual encounters. Mathematical Biosciences and Engineering, 2017, 14(2): 359-376. doi: 10.3934/mbe.2017023 |
[3] | Sharon M. Cameron, Ariel Cintrón-Arias . Prisoner's Dilemma on real social networks: Revisited. Mathematical Biosciences and Engineering, 2013, 10(5&6): 1381-1398. doi: 10.3934/mbe.2013.10.1381 |
[4] | Notice Ringa, Chris T. Bauch . Spatially-implicit modelling of disease-behaviour interactions in the context of non-pharmaceutical interventions. Mathematical Biosciences and Engineering, 2018, 15(2): 461-483. doi: 10.3934/mbe.2018021 |
[5] | Zheng Liu, Lingling Lang, Lingling Li, Yuanjun Zhao, Lihua Shi . Evolutionary game analysis on the recycling strategy of household medical device enterprises under government dynamic rewards and punishments. Mathematical Biosciences and Engineering, 2021, 18(5): 6434-6451. doi: 10.3934/mbe.2021320 |
[6] | Jianjun Long, Hui Huang . Stability of equilibrium production-price in a dynamic duopoly Cournot-Bertrand game with asymmetric information and cluster spillovers. Mathematical Biosciences and Engineering, 2022, 19(12): 14056-14073. doi: 10.3934/mbe.2022654 |
[7] | Rajanish Kumar Rai, Pankaj Kumar Tiwari, Yun Kang, Arvind Kumar Misra . Modeling the effect of literacy and social media advertisements on the dynamics of infectious diseases. Mathematical Biosciences and Engineering, 2020, 17(5): 5812-5848. doi: 10.3934/mbe.2020311 |
[8] | Alexander S. Bratus, Vladimir P. Posvyanskii, Artem S. Novozhilov . A note on the replicator equation with explicit space and global regulation. Mathematical Biosciences and Engineering, 2011, 8(3): 659-676. doi: 10.3934/mbe.2011.8.659 |
[9] | Dongning Chen, Jianchang Liu, Chengyu Yao, Ziwei Zhang, Xinwei Du . Multi-strategy improved salp swarm algorithm and its application in reliability optimization. Mathematical Biosciences and Engineering, 2022, 19(5): 5269-5292. doi: 10.3934/mbe.2022247 |
[10] | Yueliang Wu, Aolong Yi, Chengcheng Ma, Ling Chen . Artificial intelligence for video game visualization, advancements, benefits and challenges. Mathematical Biosciences and Engineering, 2023, 20(8): 15345-15373. doi: 10.3934/mbe.2023686 |
Background: Calcific aortic valve stenosis (CAVS) is a crucial cardiovascular disease facing aging societies. Our research attempts to identify immune-related genes through bioinformatics and machine learning analysis. Two machine learning strategies include Least Absolute Shrinkage Selection Operator (LASSO) and Support Vector Machine Recursive Feature Elimination (SVM-RFE). In addition, we deeply explore the role of immune cell infiltration in CAVS, aiming to study the potential therapeutic targets of CAVS and explore possible drugs. Methods: Download three data sets related to CAVS from the Gene Expression Omnibus. Gene set variation analysis (GSVA) looks for potential mechanisms, determines differentially expressed immune-related genes (DEIRGs) by combining the ImmPort database with CAVS differential genes, and explores the functions and pathways of enrichment. Two machine learning methods, LASSO and SVM-RFE, screen key immune signals and validate them in external data sets. Single-sample GSEA (ssGSEA) and CIBERSORT analyze the subtypes of immune infiltrating cells and integrate the analysis with DEIRGs and key immune signals. Finally, the possible targeted drugs are analyzed through the Connectivity Map (CMap). Results: GSVA analysis of the gene set suggests that it is highly correlated with multiple immune pathways. 266 differential genes (DEGs) integrate with immune genes to obtain 71 DEIRGs. Enrichment analysis found that DEIRGs are related to oxidative stress, synaptic membrane components, receptor activity, and a variety of cardiovascular diseases and immune pathways. Angiotensin II Receptor Type 1(AGTR1), Phospholipid Transfer Protein (PLTP), Secretogranin II (SCG2) are identified as key immune signals of CAVS by machine learning. Immune infiltration found that B cells naï ve and Macrophages M2 are less in CAVS, while Macrophages M0 is more in CAVS. Simultaneously, AGTR1, PLTP, SCG2 are highly correlated with a variety of immune cell subtypes. CMap analysis found that isoliquiritigenin, parthenolide, and pyrrolidine-dithiocarbamate are the top three targeted drugs related to CAVS immunity. Conclusion: The key immune signals, immune infiltration and potential drugs obtained from the research play a vital role in the pathophysiological progress of CAVS.
Game theory is an important branch of operations research, in which population games are a classical game model. Because population games not only can reveal the essential features of collaboration and competition but also can provide profound insights and revelations among populations, it has been widely used in social sciences, biology, economics, and other fields. Therefore, population games have been a hot topic of academic research. In the game theory, Nash equilibrium [1,2] is an important concept. However, the traditional Nash equilibrium requires that players are perfectly rational and have complete information. Fudenberg and Levine [3] propose an alternative interpretation of equilibrium, "The equilibrium is the long-term outcome of the process by which imperfectly rational players seek to optimize over time". Influenced by Fudenberg's interpretation of equilibrium, we consider how to find the path to Nash equilibrium under conditions of imperfect rationality and incomplete information. In reality, players aim to maximize their benefits, and equilibrium emerges after repeated games. Nash equilibrium is an integral component of this equilibrium, and due to its challenging establishment, investigating the process of Nash equilibrium formation holds intrinsic value. These players are not smart enough, and their ability is limited. To depict the strategic interactions among these players, we develop an algorithm to simulate their gaming processes. Among these algorithms, the particle swarm optimization (PSO) algorithm [4,5] is based on the feeding behavior of a bird flock. Both the PSO algorithm and the realization of Nash equilibrium are based on the concept of optimization, albeit with distinct approaches. The PSO algorithm emphasizes collective optimization, whereas Nash equilibrium realization centers around individual optimization. Therefore, we can glean insights from the PSO algorithm to develop an algorithm suitable for achieving Nash equilibrium. The algorithmic realization of Nash equilibrium is rooted in the decisions made by imperfectly rational players, developing algorithms to simulate equilibrium evolution. However, limited research exists regarding achieving Nash equilibrium in population games using swarm intelligence algorithms.
In the field of game theory, Nash equilibrium theory holds significant importance. Learning rules provide a perspective for studying Nash equilibrium from the players' viewpoint. Currently, three primary types of learning models exist. The first type is the virtual action learning theory [6,7,8,9,10,11,12,13], which was first proposed by Fudenberg and Levine [3]. It is believed that the opponent's strategy remains uncertain in each game, requiring the anticipation of the opponent's moves. The theory of virtual action learning considers the opponent's prior strategy choices, assigning weight to these choices and using the weighted outcome to determine the opponent's subsequent strategy. The second type is the social learning model [14,15,16,17,18] for population games, which is also proposed by Fudenberg and Levine [19]. Within this model, players can glean information about fellow players who achieve superior benefits within the population. This collective learning process eventually converges the system to a stable state. The third type is the reinforcement learning model [20,21,22,23,24], Littman [25] proposed a two-player model in zero-sum games. The model assumes that players can retain the memory of strategies and their associated benefits from previous games. Through continuous reflective learning, players strive to achieve Nash equilibrium. In addition, Borgers and Sarin [26] proposed the stimulus-response learning model based on the reinforcement learning model. In this model, players can solely recall their past strategy selections and the associated benefits. Consequently, they are inclined to employ their previous actions to guide future strategic decisions. The model posits that well-performing actions are positively reinforced, while poorly performing actions are negatively reinforced. Jordan [27] proposed the Bayesian learning model, and Camerer and Hua [28] proposed the experience-adding weight affinity (EWA) model. These two models are also important learning models based on virtual, social, and reinforcement learning models.
Previous research on realizing Nash equilibrium has been mainly based on reinforcement learning theory. In 2000, Singh et al. [29] first proposed the infinitesimal gradient algorithm (IGA), which enables each player to adjust its strategy based on the gradient of its expected benefit. This algorithm converges to a particular Nash equilibrium. After that, Zinkevich [30] proposed the generalized infinitesimal gradient algorithm (GIGA), which extends the applicability of the IGA algorithm from just two strategies to encompass multi-strategy scenarios. Wang et al. [31] expanded their investigation to meta-games, achieving a path towards meta-equilibrium using Q-learning. However, the existing realizations of Nash equilibrium mainly focus on inter-player games, and further exploration and refinement are necessary for the realization of Nash equilibrium in population games.
This paper develops the population game particle swarm optimization (PGPSO) algorithm, which uses social learning and population imitation as theoretical sources. We theoretically prove the convergence of the PGPSO algorithm, and the Nash equilibrium of a single mixed strategy is proved to be the center of a stable limit ring in the algorithm. Using the PGPSO algorithm, we simulate the evolution of three two-population games and search their Nash equilibriums' realization paths. The experimental outcomes validate the efficacy of the PGPSO algorithm in uncovering Nash equilibria. Additionally, the effect of introspection rate and initial state on the PGPSO algorithm to realize Nash equilibrium is further explored.
This section will mainly introduce the fundamental concepts of population games, social learning theory, and population imitation theory.
The two-population game is denoted by {Γ,X,F} [32].
1) Γ={1,2} denotes the two populations. For each population, p∈Γ, Sp={1,2} denotes the set of pure strategies available to population p.
2) For population 1, x1 denotes the proportion of players who choose strategy 1; for population 2, y1 denotes the proportion of players who choose strategy 1. Further, x=(x1,x2) denotes the pure strategy distribution state of population 1, y=(y1,y2) denotes the pure strategy distribution state of population 2. The strategy choice of the i-th player in population 1 is denoted as xi, and likewise, the strategy choice of the j-th player in population 2 is denoted as yj. Given that players can only select pure strategies, xi,yj∈{0,1}. If xi (yj) = 1, it means that the i-th (j-th) player has chosen strategy 1; If xi (yj) = 0, it means that the i-th (j-th) player has chosen strategy 2. The combined set X=(x,y) represents the social state of the two populations Γ.
3) For any given population p, Fps:X→R denotes the expected benefit associated with pure strategy s, s∈Sp. Therefore, the corresponding set of pure strategies for population p is denoted as Sp, and Fp:X→R2 represents the expected benefit of population p. The overall expected benefit function of the entire society Γ is denoted as F=(F1;F2):X→R4.
The following definition is the Nash equilibrium definition of the two populations game {Γ,X,F} [32].
Definition 1. Let {Γ,X,F} be a two-population game. If the social state ˉz=(ˉx,ˉy)∈X satisfies ∀p∈Γ,ˉxs>0,ˉys>0⇒Fps(ˉz)=maxr∈SpFpr(ˉz), ∀s∈Sp, then we define ˉz=(ˉx,ˉy) as the Nash equilibrium of the population game {Γ,X,F}, and denote the set containing all Nash equilibria as E(F).
According to the above definition of Nash equilibrium for the two-population game, the Nash equilibrium of the prisoner's dilemma game is (ˉx,ˉy)=((1,0),(1,0)). The Nash equilibrium of the coin-flip game is (ˉx,ˉy)=((12,12),(12,12)). The Nash equilibrium set of the coordination game is E(F)={((1,0),(1,0)),((0,1),(0,1)),((13,23),(13,23))}. The benefit matrices for the three games are presented in Examples 4.1–4.3 later on.
Fudenberg and Levine [3] proposed the social learning theory to explain the formation of the Nash equilibrium of the population game. In a single iteration, the initial population is called the "parent", denoted as q(t). The population that completes strategy adjustment is called the "offspring", denoted as q(t+1). There is an excessive generation from the parent to the offspring, called the pending generation, denoted as q(t′). It is crucial that the overall strategy distribution of the pending generation is the same as that of the parent, i.e., xs(t′)=xs(t), with pending players corresponding one-to-one with their parent players.
During each iteration, for one of the populations p, a proportion α of pending players chooses to adjust their strategies, while the remaining pending players will keep their original strategy. Björnerstedt and Weibull [15] interpret the phenomenon as an introspective phenomenon, where certain players in the population actively imitate and learn from others. Players who adjust their strategies are called "introspective players", whereas those who keep their original strategies are called "non-introspective players". In the context of a game, following the principle of random matching, all players choose the pure strategy. This process can be illustrated using the game model presented in [3] as an example.
LRUD[9,00,02,02,0] |
The model is based on a game framework featuring a virtual population 2, as proposed by Fudenberg and Levine [3]. The social learning theory for strategy updating is as follows: x1(t) denotes the proportion of parents in population 1 who choose strategy U, x2(t) denotes the proportion of parents in population 1 who choose strategy D, y1(t) denotes the proportion of parents in population 2 who choose strategy L, and y2(t) denotes the proportion of parents who choose strategy R. For population 1, the proportion of direct choice strategy U without introspection is (1−α)x1(t). According to the social learning theory, a player's strategy remains unchanged if their strategy is consistent with their parent's, and the proportion is αx1(t)2.
When a player's strategy does not match their parent's strategy, in that case, it is divided into two small populations according to the encountered opponents, and the player imitates the strategy of the small population with the highest expected benefit. For example, when a player encounters an opponent who chooses strategy L, he will choose strategy U, and the proportion is 2αy1(t)x1(t)x2(t). Similarly, if he encounters an opponent who chooses strategy R, he will choose strategy D, and the proportion is 2αy2(t)x1(t)x2(t).
With the above variation of strategies, the proportional update formula for the offspring selection strategy U of population 1 can be obtained as:
x1(t+1)=(1−α)x1(t)+α(x1(t)2+2y1(t)x1(t)x2(t)). | (2.1) |
For the population game model, {Γ,X,F}, Schlag [14] presents an alternative perspective, asserting that each player can observe the strategies and expected benefits of others within their population. This perspective eliminates the notion of parent and offspring from social learning theory. Schlag argues that the emergence of Nash equilibrium in population games hinges solely on the phenomenon of imitation. The rules of imitation, as defined by Schlag, are as follows:
1) Following imitative behavior, i.e., change behavior exclusively by imitating others.
2) Never imitate someone who performs worse than you do.
Rule 2) means that the players will only imitate those who exhibit superior expected benefits. This implies that players evaluate their strategies based on the expected benefit of other players. And players choose to imitate other players with superior expected benefits to improve their own benefit. The essence of the imitation rule is that players adjust their strategies based on the strategies and benefits of other players.
In 1995, inspired by the regularity of birds' flock feeding behavior, Kennedy and Eberhart [4,5] developed a simplified algorithm model, which later evolved into the particle swarm optimization (PSO) algorithm through subsequent enhancements. The idea of the PSO algorithm originated from studying the birds' flock feeding behavior, where the birds share information collectively so that the flock can find the optimal destination. In the PSO algorithm, the feasible solution of each optimization problem can be considered as a point in the d-dimensional search space. Let the position of the i-th particle be denoted as li=(li1,li2,...,lid), and the best position it has experienced is denoted as pi=(pi1,pi2,...,pid), and also known as pbest. The index number of the best position experienced by all particles is denoted by the symbol gbest. The velocity of the i-th particle is denoted as vi=(vi1,vi2,...,vid). For each iteration, the velocity and position of the particle change according to the following:
vk+1i=wvki+c1r1(pkbest(i)−lki)+c2r2(gkbest−lki), | (3.1) |
lk+1i=lki+vk+1i, | (3.2) |
where c1, c2 are learning factors; r1, r2 are random numbers varying within (0,1); w are inertia weights; k is the number of iterations.
The PGPSO algorithm builds upon the framework of the PSO algorithm to realize Nash equilibrium and find the realization path, which introduces social learning and population imitation theory into the PSO algorithm. In the PGPSO algorithm, each Nash equilibrium represents a solution to the problem, and each player in the population is considered a particle of the PSO algorithm. Players with different strategies reflect the differences in position among particles, and different benefits reflect the differences in expected benefits among particles, both of which constitute particle diversity.
According to population imitation theory, in the population updating process of particles (players), particles (players) with high expected benefits are learned. However, if all players with low benefits change their strategies by imitating in the first iteration, the algorithm stops directly, resulting in Nash equilibrium losing the opportunity to be learned. Therefore, this paper takes the introspection rate to the PSO algorithm. This serves two purposes: firstly, only some particles (players) choose to introspect, effectively maintaining the diversity of particles (players) in each iteration. Secondly, the introspection rate fits the lag of strategy update of the players in the actual game.
During each iteration, the player chooses a pure strategy, i.e., the position of the particles. Given the nature of the two-population game, the PGPSO algorithm accommodates two distinct particle populations. The benefit matrices for a two-population game are defined as follows:
A=(a11a12a21a22),B=(b11b12b21b22). |
At the k-th iteration, the expected benefit of the i-th player in population 1 is calculated as per references [33,34].
F1s(k)=(xi,1−xi)A(y1,1−y1)T=(a11−a12−a21+a22)xiy1+(a12−a22)xi+(a21−a22)y1+a22, | (3.3) |
where xi denotes the i-th player strategy choice in population 1, xi∈{0,1}. If xi=1, then the player has chosen the strategy 1; if xi=0, then the player has chosen the strategy 2. y1 indicates the proportion of players in population 2 who choose strategy 1.
The expected benefit of the j-th player in population 2 is
F2s(k)=(x1,1−x1)B(yj,1−yj)T=(b11−b12−b21+b22)x1yj+(b12−b22)x1+(b21−b22)yj+b22, | (3.4) |
where yi denotes the i-th player strategy choice in population 2, yi∈{0,1}. If yi=1, then the player has chosen the strategy 1; if yi=0, then the player has chosen the strategy 2. x1 denotes the proportion of players in population 1 who choose strategy 1.
The Nash equilibrium of the population game represents a state where all players maximize their benefits. The players are selfish and aim to maximize their benefits, so the benefits defined in the Eqs (3.3) and (3.4) are obtained based on that consideration. Since the benefits of players in the population who choose the same strategy are indistinguishable, it is assumed that determining the benefits corresponding to each strategy becomes an optimization problem. In this case, solving the Nash equilibrium is equivalent to finding the optimal solution of this optimization problem. The key difference is that an ordinary optimization problem is a single-player optimization problem. At the same time, the game studies a multi-player optimization problem, which is the essential distinction between the two.
In the iterative process of particles, population imitation theory guides introspective players to adopt the strategy associated with the highest expected benefit. In the k-th iteration of a population, the particle with the highest expected benefit is determined by the Eqs (3.3) and (3.4), denoted as ikbest, and the introspective particle changes its strategy to ikbest. In contrast, the non-introspective particle keeps its strategy unchanged. At the k-th iteration, suppose the set of all particles' ordinal numbers is Ik, and the set of introspective players' ordinal numbers is denoted as Ikα.
Take the Eqs (3.1) and (3.2) as the basis, the PGPSO algorithm iteration function is
vk+1i=ikbest−lki, | (3.5) |
lk+1i={lki+vk+1i,i∈Ikαlki,i∉Ikα. | (3.6) |
This is the iterative formulation of the PGPSO algorithm, which draws on the formula form of the Eqs (3.1) and (3.2). However, the idea is derived from the theory of population imitation and social learning, where players with relatively lower benefits adopt strategies observed from the players with the highest benefit.
The implementing steps of the PGPSO algorithm are as follows.
(a) The PGPSO algorithm initializes the parameter values. These include the introspection rates α, β, the lower bound of the search space popmin, the upper bound of the search space popmax, the population size m, n, and the number of iterations genmax.
(b) Two populations are created, each containing m and n particles, respectively. The algorithm randomly generates the strategy xi for population 1 of m particles, xi satisfies xi=0 or 1. Then the algorithm randomly generates the strategy yj for population 2 of n particles, yj satisfies yj=0 or 1.
(c) Expected benefits of all particles in both populations are computed using Eqs (3.3) and (3.4), and the particle strategy with the highest expected benefit is found, denoted as ibest1 and ibest2, respectively.
(d) All particles update their positions according to Eqs (3.5) and (3.6).
(e) Whether to end the iteration is determined according to the number of iterations genmax. If the iteration ends, the algorithm outputs the two populations' optimal benefits and particle position figures. Otherwise, the algorithm turns to (c).
In the PGPSO algorithm, the update rule of players' strategies is as follows: the players with α proportion choose to adjust the strategy in population 1, and the remaining players do not. The players with β proportion choose to adjust their strategy in population 2, and the remaining players do not. For the players who choose to adjust their strategies, they can observe the expected benefits of the players in their population, i.e., the benefits are derived from the Eqs (3.3) and (3.4). Subsequently, these players apply population imitation theory to select an optimal strategy. After the above adjustment, the Eq (2.1) is the basis. The updated formula for the proportion of two populations choosing strategy 1 is obtained as follows:
{x1(t+1)=(1−α)x1(t)+α[p1(t)x1+p2(t)(1−x1(t))]y1(t+1)=(1−β)y1(t)+β[q1(t)y1+q2(t)(1−y1(t))]. | (4.1) |
where x1(t+1), y1(t+1) is the proportion of players choosing strategy 1 at t+1 iterations for both populations, respectively x1(t+1),y1(t+1)∈[0,1]. If x1(t+1) and y1(t+1) are both 0, it implies that both populations choose strategy 2. According to the benefit matrices A and B, all players in the first population receive the benefit a22, and all players in the second population receive the benefit b22.
p1(t)={1,F11(t)≥F12(t)0,F11(t)<F12(t);p2(t)={1,F11(t)>F12(t)0,F11(t)≤F12(t); |
q1(t)={1,F21(t)≥F22(t)0,F21(t)<F22(t);q2(t)={1,F21(t)>F22(t)0,F21(t)≤F22(t). |
The updating Eq (4.1) essentially represents a discretized form of the differential Eqs (3.5) and (3.6). Compared with the Eq (2.1) in social learning theory, Eq (4.1) can be effectively applied to any two-population and two-strategy game model. Second, the social learning rule specifies the concepts of a parent, pending generation, and child, and the parent influences strategy updating. At the same time, the Eq (4.1) removes the concepts of a parent and pending generation, thereby reducing the dependency on heritability as a condition for strategy adaptation.
For the benefit matrices of the two-population game:
A=(a11a12a21a22),B=(b11b12b21b22) |
Let a1=a11−a21, a2=a22−a12, b1=b11−b12, b2=b22−b21.
The benefit matrices simplify to
A=(a100a2),B=(b100b2) |
where a1≠0,a2≠0,b1≠0,b2≠0.
Theorem 4.1. In a non-cooperative repeated two-population and two-strategy game with benefit matrices A and B, when each population updates its strategy following the Eq (4.1), the convergence outcome corresponds to either the Nash equilibrium or its stable limit ring.
Proof. The updating Eq (4.1) is transformed to continuous time, and the imitation dynamic equation is obtained using the difference method.
{dx1dt=−αx1+α[p1(t)x1+p2(t)(1−x1)]dy1dt=−βy1+β[q1(t)y1+q2(t)(1−y1)]. | (4.2) |
The two-population game is classified into three types according to their equilibria: one pure strategy Nash equilibrium, one mixed strategy Nash equilibrium, and three Nash equilibria (two pure strategy Nash equilibria, one mixed strategy Nash equilibrium). Next, we discuss the classification.
For the first type, if Nash equilibrium (ˉx,ˉy)=((1,0),(1,0)), then a1>0,a2<0,b1>0,b2<0, deducing F11=a1y1, F12=a2(1−y1), F21=b1x1 and F22=b2(1−x1), implying that F11>F12,F21>F22. From the Eq (4.2), we get dx1dt=−αx1+α≥0, dy1dt=−βy1+β≥0. The imitation dynamic Eq (4.2) converges to x1=y1=1. That is, it converges to Nash equilibrium ((1,0),(1,0)). When (ˉx,ˉy)=((1,0),(0,1)), (ˉx,ˉy)=((0,1),(1,0)) and (ˉx,ˉy)=((0,1),(0,1)), the analysis is similar. The Eq (4.2) converges to Nash equilibrium, which is proved.
For the second type, Nash equilibrium (ˉx,ˉy)=((b2b1+b2,b1b1+b2),(a2a1+a2,a1a1+a2)), then a1<0,a2<0,b1>0,b2>0. For the Eq (4.1), we get:
p1(t)={0,y1(t)>a2a1+a21,y1(t)≤a2a1+a2;p2(t)={0,y1(t)≥a2a1+a21,y1(t)<a2a1+a2; |
q1(t)={1,x1(t)≥b2b1+b20,x1(t)<b2b1+b2;q2(t)={1,x1(t)>b2b1+b20,x1(t)≤b2b1+b2. |
When x1(0)=b2b1+b2,y1(0)=a2a1+a2, dx1dt=dy1dt=0, i.e., the imitation dynamic Eq (4.2) will converge to Nash equilibrium ((b2b1+b2,b1b1+b2),(a2a1+a2,a1a1+a2)).
If the initial point is not Nash equilibrium, let b′=b2b1+b2, a′=a2a1+a2 and x1=x1−b2b1+b2, y1=y1−a2a1+a2, then we can obtain that the Eq (4.2) is a differential equation with zero solutions.
Then the Eq (4.2) becomes
{˙x1=αp1(t)(x1+b′)+αp2(t)(1−b′−x1)−α(x1+b′)˙y1=βq1(t)(y1+a′)+βq2(t)(1−a′−y1)−β(y1+a′). | (4.3) |
Taking the polar coordinates x1=rcosθ, y1=rsinθ, we can obtain that the Eq (4.3) is
˙r=α(p1(t)−p2(t)−1)rcos2θ+α(b′p1(t)−b′p2(t)−b′+p2(t))cosθ+β(q1(t)−q2(t)−1)rsin2θ+β(a′q1(t)−a′q2(t)−a′+q2(t))sinθ. | (4.4) |
Divide the Eq (4.4) into 0<θ<π2,π2<θ<π,π<θ<3π2,3π2<θ<2π and θ=0,π2,π,3π2,2π. These cases are classified and discussed.
1) When 0<θ<π2, we get x1(t),y1(t)>0, which is obtained from the Eq (4.4) as
˙r=−(αcos2θ+βsin2θ)r−αb′cosθ+β(1−a′)sinθ. |
From Eq (4.4), it follows that when r=−αb′cosθ+β(1−a′)sinθαcos2θ+βsin2θ, ˙r=0, i.e., there is a special solution
r=−αb′cosθ+β(1−a′)sinθαcos2θ+βsin2θ. |
The solution is a curve in the phase plane centered at the origin.
When r>−αb′cosθ+β(1−a′)sinθαcos2θ+βsin2θ, we get ˙r<0 from the Eq (4.4). That is, the trajectory converges to the curve from outside the curve. When r<−αb′cosθ+β(1−a′)sinθαcos2θ+βsin2θ, we get ˙r>0 from the Eq (4.4). That is, the trajectory converges to the curve from inside the curve. Therefore the system has a stable limit ring centered at the origin at 0<θ<π2. A stable limit ring is a periodic solution around a non-isolated equilibrium point. When the solution trajectory evolves from a point in the solution space, it converges to this limit ring and makes a periodic movement on this limit ring.
2) When π2<θ<π,π<θ<3π2,3π2<θ<2π, ˙r is respectively,
−(αcos2θ+βsin2θ)r−αb′cosθ−βa′sinθ, |
−(αcos2θ+βsin2θ)r+α(1−b′)cosθ−βa′sinθ, |
−(αcos2θ+βsin2θ)r+α(1−b′)cosθ+β(1−a′)sinθ. |
The analysis idea and result are similar to 0<θ<π2.
3) When θ=0,π2,π,3π2,2π, ˙r=0.
The polar differential Eq (4.4) has a stable limit ring centered at the origin. That is, the imitation dynamic Eq (4.2) has a stable limit ring centered at ((b2b1+b2,b1b1+b2),(a2a1+a2,a1a1+a2)). The imitation dynamic Eq (4.2) will converge to the Nash equilibrium or a stable limit ring centered on the Nash equilibrium.
After the above analysis, the second type proves to be completed.
For the third type, if Nash equilibrium set E(F)={((1,0),(1,0)),((0,1),(0,1)),((b2b1+b2,b1b1+b2),(a2a1+a2,a1a1+a2))}, then a1>0,a2>0,b1>0,b2>0. For the Eq (4.1), we get
p1(t)={1,y1(t)≥a2a1+a20,y1(t)<a2a1+a2;p2(t)={1,y1(t)≥a2a1+a20,y1(t)<a2a1+a2; |
q1(t)={1,x1(t)≥b2b1+b20,x1(t)<b2b1+b2;q2(t)={1,x1(t)>b2b1+b20,x1(t)≤b2b1+b2. |
According to the method of variation of parameters, the solution of the imitation dynamic Eq (4.2) is
{x1(t)=eα(p1(t)−p2(t)−1)t[α(p1(t)−p2(t)−1)x1(0)+αp2(t)]−αp2(t)α(p1(t)−p2(t)−1)y1(t)=eβ(q1(t)−q2(t)−1)t[β(q1(t)−q2(x1)−1)y1(0)+βq2(t)]−βq2(t)β(q1(t)−q2(t)−1). |
For ∀ε>0, there exists δ>0, when √(x1(0)−1)2+(y1(0)−1)2<δ, there is p1(t)=p2(t)=q1(t)=q2(t)=1. From the Eq (4.2), we get
limt→+∞√(x1(t)−1)2+(y1(t)−1)2=√(1−1)2+(1−1)2=0. |
This proves the asymptotic stability of ((1,0),(1,0)).
When √x1(0)2+y1(0)2<δ, we have p1(t)=p2(t)=q1(t)=q2(t)=0. From the Eq (4.2), we get
limt→+∞√x1(t)2+y1(t)2=√02+02=0. |
This proves the asymptotic stability of ((0,1),(0,1)).
If and only if x1(0)=b2b1+b2,y1(0)=a2a1+a2, we get limt→+∞x1(t)=b2b1+b2,limt→+∞y1(t)=a2a1+a2.
According to the above analysis, the solution trajectory of the Eq (4.2) starts from any position in the solution space. It will converge to an element of the Nash equilibrium set E(F).
When the Nash equilibrium set
E(F)={((1,0),(0,1)),((0,1),(1,0)),((b2b1+b2,b1b1+b2),(a2a1+a2,a1a1+a2))}, |
the analysis is similar. The Eq (4.2) converges to Nash equilibrium, which is proved.
Example 4.1. Take the prisoner's dilemma game with the following benefit matrices. We set the introspection rate α=0.1,β=0.2, the horizontal or vertical separation of the initial position is 0.2, and the arrows represent the direction of the solution trajectory. The solution trajectory is shown in Figure 1.
A1=(−50−8−1),B1=(−5−80−1) |
From the Figure 1, it can be seen that solution trajectories eventually converge to (1,1) for all initial points, i.e., the solution trajectories converge to Nash equilibrium (ˉx,ˉy)=((1,0),(1,0)).
Example 4.2. Take the coin-flip game with the following benefit matrices. We set the introspection rate α=0.1,β=0.2, the horizontal or vertical separation of the initial position is 0.25, and the arrow represents the direction of the solution trajectory. The solution trajectory is shown in Figure 2.
A2=(−111−1),B2=(1−1−11) |
From the Figure 2, we can see that when the initial point is (0.5,0.5), the solution trajectory converges to (0.5,0.5), i.e., Nash equilibrium (ˉx,ˉy)=((12,12),(12,12)); when the initial point is other than (0.5,0.5), the solution trajectory evolves counterclockwise to (0.5,0.5) and converges to the limit ring centered at (0.5,0.5), i.e., a stable limit ring centered at Nash equilibrium (ˉx,ˉy)=((12,12)).
Example 4.3. Take the coordination game with the following benefit matrices. We set the introspection rate α=0.1,β=0.2, the horizontal or vertical separation of the initial position is 16, and the arrow represents the direction of the solution trajectory. The solution trajectory is shown in Figure 3.
A3=(2001),B3=(2001) |
From the Figure 3, when the initial point is (13,13), the solution trajectory converges to (13,13), i.e., Nash equilibrium (ˉx,ˉy)=((13,23),(13,23)); when the initial point is to the upper right of (13,13), the solution trajectory converges to (1,1), i.e., Nash equilibrium ((1,0),(1,0)). When the initial point is to the lower left of (13,13), the solution trajectory converges to (0,0), i.e., Nash equilibrium ((0,1),(0,1)).
Research in the realm of realizing or computing Nash equilibrium through algorithmic approaches has led to various contributions. Zhang et al. [35] proposed the PMR-IGA algorithm, and Zhang et al. [36] proposed the SA-IGA algorithm. Both algorithms are based on the reinforcement learning theory, striving to guide individuals within a population through iterative processes that ultimately lead to Nash equilibrium convergence. For the computation of Nash equilibrium, Li et al. [37] proposed the GPDEPSO algorithm to compute the Nash equilibrium of a finite non-cooperative game. This approach equates solving the Nash equilibrium to solving an optimization problem and considers the algorithmic process stochastic. Stochastic generalized function theory is adopted to prove the convergence to the Nash equilibrium.
On the other hand, the PGPSO algorithm's inspiration differs from the above-mentioned approaches, as it is based on the theory of social learning and population imitation. Its convergence is demonstrated by transforming the iterative process into differential equations. It proves the pure Nash equilibrium strategy's asymptotic stability from an equation-driven standpoint. In this case, the unique mixed strategy Nash equilibrium is the center of the stable limit ring in the solution space, and the solution trajectories from any point will converge to that limit ring.
In this section, we study the Nash equilibrium realizations of the prisoner's dilemma game, the coin-flip game, and the hawk-dove game using the PGPSO algorithm, respectively. The prisoner's dilemma game has only one pure strategy Nash equilibrium, the coin-flip game has only one mixed strategy Nash equilibrium, and the hawk-dove game has three Nash equilibria, i.e., two pure strategy Nash equilibria and one mixed strategy Nash equilibrium.
The prisoner's dilemma game, typically a two-person game, has been employed by Wang [38] to corroborate the presence of the "baiting effect" within social populations. Notably, the benefit matrices characteristic of the inter-player game can be seamlessly applied to the population game model. Therefore, this game model can be used as an example of the population game. The prisoner's dilemma game has only one pure-strategy Nash equilibrium. Both populations choose strategy 1, i.e., (ˉx,ˉy)=((1,0),(1,0)). The following are the benefit matrices and expected benefits of the prisoner's dilemma game:
A1=(−50−8−1),B1=(−5−80−1), |
F1=2xiy1+xi−7y1−1;F2=2x1yj−7x1+yj−1. |
In the PGPSO algorithm of the prisoner's dilemma game, we set the introspection rate α=β={112,k≤25124,k>25, the population size m=n=48, the search space range from popmin=0 to popmax=1, and the number of iterations genmax=50. The initial states of the populations are chosen randomly by the system. The two populations' optimal benefit and location figures are shown in Figure 4.
From the Figure 4(a), (b) we can see that all the particles of population 1 converge to x1=1, i.e., all players of population 1 choose the strategy 1, and the best benefit of population 1 converges to −5. All particles of population 2 converge to y1=1, i.e., all players of population 2 choose strategy 1, and the best benefit of population 2 converges to −5. This outcome is consistent with the Nash equilibrium ((1,0),(1,0)) of the prisoner's dilemma game. Therefore, the PGPSO algorithm accurately finds the particles' positions and benefits corresponding to its Nash equilibrium strategy. It completely records the path to the Nash equilibrium of the prisoner's dilemma game.
Consider the coin-flip game as a population game, where two populations play against each other according to the benefit matrices of the coin-flip game. The coin-flip game has a mixed-strategy Nash equilibrium, i.e., (ˉx,ˉy)=((12,12),(12,12)). The following are the benefit matrices and expected benefits of the coin-flip game:
A2=(−111−1),B2=(1−1−11), |
F1=−4xiy1+2xi+2y1−1;F2=4x1yj−2x1−2yj+1. |
In the PGPSO algorithm of the coin-flip game, we set the introspection rate α=β={112,k≤25124,k>25, the population size m=n=48, the search space range from popmin=0 to popmax=1 and the number of iterations genmax=50. The initial states of the populations are chosen randomly by the system. The two populations' optimal benefit and location figures are shown in Figure 5.
From the Figure 5(a), (b), we can see that all particles of population 1 converge cyclically to x1=12, i.e., nearly half of the players choose the strategy 1 in population 1. The optimal benefit of population 1 converges cyclically at 0.025. All particles of population 2 converge cyclically to y1=12, i.e., nearly half of the players choose strategy 1 in population 2. The optimal benefit of population 2 converges cyclically to 0.025. All particles converge cyclically to ((12,12),(12,12)). It represents the evolution of the two populations toward Nash equilibrium, which eventually converges to a neighborhood centered on Nash equilibrium. Corresponding to the mixed-strategy Nash equilibrium is a stable limit ring in the imitation dynamic equation Eq (4.2). And from the Figure 5(c), (d), we can see that when the initial strategy distribution is Nash equilibrium for the two populations, all particles of population 1 converge to x1=12. The optimal benefit of population 2 converges to 0. All particles of population 2 converge to y1=12, and the optimal benefit of population 2 converges to 0. This outcome is consistent with the Nash equilibrium ((12,12),(12,12)) of the coin-flip game. Therefore, it can be seen that the PGPSO algorithm accurately finds the particle positions and benefits corresponding to its Nash equilibrium strategy. It completely records the path to the Nash equilibrium of the coin-flip game.
The hawk-dove game is an important population game model in evolutionary game theory. The model has three Nash equilibria, and the set of Nash equilibria is E(F)={((1,0),(0,1)),((0,1),(1,0)),((23,13),(23,13))}. The following are the benefit matrices and expected benefits of the hawk-dove game:
A4=(−1402),B4=(−1042), |
F1=−3xiy1+2xi−2y1+2;F2=−3x1yj−2x1+2yj+2. |
In the PGPSO algorithm of the hawk-dove game, we set the introspection rate α=β={112,k≤25124,k>25, the population size m=n=48, the search space range from popmin=0 to popmax=1, and the number of iterations genmax=50. The initial states of the populations are chosen randomly by the system. The two populations' optimal benefit and location figures are shown in Figure 6.
From the Figure 6(a), (b), it can be seen that all particles of population 1 converge to x1=1, and the optimal benefit converges to 4. All particles of population 2 converge to y1=0, and the optimal benefit converge to 0. From the Figure 6(c), (d), all particles of population 1 converge to x1=0, and the optimal benefit converges to 0; all particles of population 2 converge to y1=1, and the optimal benefit converges to 4. From the Figure 6(e), (f), when the initial strategy distribution of two populations is Nash equilibria ((23,13),(23,13)), all particles of population 1 converge to x1=23, and the optimal benefit converges to 23. All particles of population 2 converge to y1=23, and the optimal benefit converges to 23. This outcome is consistent with the three Nash equilibria of the hawk-dove game, E(F)={((1,0),(0,1)),((0,1),(1,0)),((23,13)),(23,13))}. Therefore, it can be seen that the PGPSO algorithm accurately finds the particle positions and benefits corresponding to its Nash equilibrium strategy. It completely records the path to the Nash equilibrium of the hawk-dove game.
In the PGPSO algorithm with introspection rate sensitivity of the three games, we set the introspection rate α=β, the population size m=n=48, the search space range from popmin=0 to popmax=1, and the number of iterations genmax=20. The initial states of the prisoner's dilemma and the hawk-dove game are x1(0)=y1(0)=0.5, and the initial states of the coin-flip game are x1(0)=y1(0)=0.4. The position figures of the two populations are shown in Figure 7.
From the Figure 7(a), (c), it can be seen that in the prisoner's dilemma and the hawk-dove game, for the two populations, the number of players converging to Nash equilibrium decreases as the introspection rate α increases, representing that the increase of the introspection rate α speeds up the evolution of Nash equilibrium realization. From the Figure 7(b), it can be seen that in the coin-flip game, the magnitude of the cycle convergence increases with the increase of the introspection rate α, which means that the increase of the introspection rate α expands the range of cycle fluctuations.
In the PGPSO algorithm for the initial state sensitivity of the three games, we set the introspection rate α=β={112,k≤25124,k>25, the population size m=n=48, the search space range from popmin=0 to popmax=1 and the number of iterations genmax=50. For the prisoner's dilemma and the hawk-dove game, the initial state range of population 1 is 0.1–0.9, and the positions are chosen every 0.1. The initial state range of population 2 is chosen with the same rules as population 1. A total of 81 parameter configurations are generated by combining the two populations. For each parameter configuration, a series of 50 experiments are conducted to observe the system's steady state. Two initial states (0.1, 0.1) and (0.9, 0.9) are selected for the coin-flip game. The location figures of the two populations are shown in Figure 8.
From Figure 8(a), it can be seen that the populations start from any initial state in the prisoner's dilemma game. The two populations converge to the Nash equilibrium ((1,0),(1,0)), which means that the change of initial state can't affect the Nash equilibrium realization. From Figure 8(b), it can be seen that the population starts from two initial states in the coin-flip game. The two populations converge cyclically to the mixed strategy Nash equilibrium ((12,12),(12,12)), representing that the initial state changes can't affect the Nash equilibrium realization. From Figure 8(c), it can be seen that in the hawk-dove game, the two populations converge to the different Nash equilibrium from the different initial states. Specifically, when the initial state is on the right side of the diagonal with x=y, the two populations converge to the Nash equilibrium ((1,0),(0,1)). Conversely, when the initial state is on the left side of this diagonal, the two populations converge to Nash equilibrium ((0,1),(1,0)). Subtle nuances emerge when considering different positions along the diagonal. Specifically, for the first five positions, the populations converge to ((0,1),(1,0)), while the last four positions lead to ((0,1),(1,0)). These results represent that the initial state will affect the Nash equilibrium realization.
Taking the welfare game in [31] as an example, for finding its mixed-strategy Nash equilibrium realization path, we compare the difference between the PGPSO algorithm and the Meta Equilibrium Q-learning algorithm.
Example 5.1. This game model has a mixed-strategy Nash equilibrium, i.e., (ˉx,ˉy)=((12,12),(14,34)), and the followings are the benefit matrices and expected benefits of the welfare game:
A5=(3−1−10),B5=(2310), |
F1=5xiy1−xi−y1;F2=−2x1yj+3x1+yj. |
The Meta Equilibrium Q-learning algorithm represents an enhancement over the Nash Q-learning algorithm. The rationale behind this improvement stems from the Nash Q-learning algorithm's limitation C specifically, its inability to devise a pathway to realize a mixed-strategy Nash equilibrium when each player opts for a pure strategy. In order to address this problem, the Meta Equilibrium Q-learning algorithm transforms the welfare game into a meta-game. It uses the pure strategy meta-equilibrium to represent the mixed-strategy Nash equilibrium in the welfare game. The meta-equilibrium's realization path replaces the mixed strategy's realization path. Thus, we can obtain the path to find the mixed-strategy Nash equilibrium. However, it is important to note that this transformation comes at the expense of increased complexity in locating the realization path for the mixed-strategy Nash equilibrium.
In the PGPSO algorithm of the welfare game, we set the introspection rate α=β={112,k≤25124,k>25, the population size m=n=48, the search space range from popmin=0 to popmax=1 and the number of iterations genmax=50. The computer system randomly selects the initial states of the populations. The position figures of the two populations are shown in Figure 9.
From Figure 9(a), it can be seen that after 25 iterations, all particles of population 1 converge cyclically to x1=12 and all particle of population 2 converge cyclically to y1=14. The difference between the maximum and minimum values of the cycle convergence is 0.0625. From the Figure 9(b), when the initial strategy distribution of the two populations is Nash equilibrium, all particles of population 1 converge to x1=12, and all particles of population 2 converge to y1=14. This outcome is consistent with the Nash equilibrium of the welfare game. Therefore, for players who choose a pure strategy in the welfare game, the PGPSO algorithm converges to the mixed strategy Nash equilibrium and finds the path to realize the equilibrium.
In the welfare game, for the problem of finding the realization path of the mixed-strategy Nash equilibrium, the Meta Equilibrium Q-learning algorithm transforms the welfare game into a meta-game. The meta-equilibrium's realization path replaces the mixed strategy's realization path. From Figure 9(a), it can be seen that the PGPSO algorithm converges the stable limit ring centered on the mixed-strategy Nash equilibrium. The PGPSO algorithm directly finds the realization path, which means that the algorithm reduces the complexity by eliminating the operation of transforming the welfare game into a meta-game.
The population's Nash equilibrium exists commonly in human societies and biological populations. Its realization is crucial from the perspective of individual players within a population. In a population of finite players, players can optimize their strategies by imitating other players with higher benefits, depending on their knowledge of their strategic environment. So far, the rule of imitation has been widely studied in different game models. In this paper, we combine social learning theory and population imitation theory, develop the PGPSO algorithm, and apply it to the Nash equilibrium realization of three two-population game models.
The motivation for studying the imitation learning rule is to explore a problem: whether imitation learning rules can realize the Nash equilibrium realization of population games. Specifically, the new learning rule is transformed into a swarm intelligence algorithm, which is used to simulate the behavioral dynamics of the players in the game. For the PGPSO algorithm iterative formulation, the convergence analysis is performed from the perspective of differential equations. The result is that the solution trajectory of differential equations converges completely to the pure strategy Nash equilibrium. The solution trajectory will converge completely to the mixed strategy Nash equilibrium when the initial position is the mixed strategy Nash equilibrium. Also, in the coin-flip game, the mixed-strategy Nash equilibrium is the center of a stable limit ring of the differential equation. When the initial position is not on the mixed strategy Nash equilibrium, all initial points converge to this limit ring.
Using the PGPSO algorithm, we simulate the Nash equilibrium realization process for three two-population games. Simulation outcomes demonstrate that the PGPSO algorithm successfully realizes Nash equilibrium realization. Meanwhile, the PGPSO algorithm clearly shows the path of realizing Nash equilibrium. According to the analysis of the effect of introspection rate and initial state on the realization of Nash equilibrium, the increase of introspection rate accelerates the evolution of pure strategy Nash equilibrium realization. However, it expands the range of cycle fluctuations of mixed strategy Nash equilibrium. The change in the initial state can't affect the Nash equilibrium realization of the prisoner's dilemma and the coin-flip game, but it causes the hawk-dove game to converge to the different Nash equilibrium.
The following abbreviations are used in this manuscript.
PSO | Particle swarm optimization |
EWA | Experience-adding weight affinity |
IGA | Infinitesimal gradient algorithm |
GIGA | Generalized infinitesimal gradient algorithm |
PGPSO | Population game particle swarm optimization |
The authors declare they have not used Artificial Intelligence (AI) tools in the creation of this article.
This work was supported by the National Natural Science Foundation of China (Grant No. 71961003) and Science and Technology Program of Guizhou Province (Grant No. 7223), and the Doctoral Foundation Project of Guizhou University (Grant No. 49).
The authors declare there is no conflicts of interest.
[1] |
P. Büttner, L. Feistner, P. Lurz, H. Thiele, J. D. Hutcheson, F. Schlotter, Dissecting calcific aortic valve disease—The role, etiology, and drivers of valvular fibrosis, Front Cardiovasc. Med., 10 (2021), 660797. https://doi.org/10.3389/fcvm.2021.660797 doi: 10.3389/fcvm.2021.660797
![]() |
[2] |
B. Alushi, L. Curini, M. R. Christopher, H. Grubitzch, U. Landmesser, A. Amedei, et al., Calcific aortic valve disease-natural history and future therapeutic strategies, Front Pharmacol., 13 (2020), 685. https://doi.org/10.3389/fphar.2020.00685 doi: 10.3389/fphar.2020.00685
![]() |
[3] |
A. D. Vito, A. Donato, I. Presta, T. Mancuso, F. S. Brunetti, P. Mastroroberto, et al., Extracellular matrix in calcific aortic valve disease: Architecture, dynamic and perspectives, Int. J. Mol. Sci., 22 (2021), 913. https://doi.org/10.3390/ijms22020913 doi: 10.3390/ijms22020913
![]() |
[4] |
J. Rysä, Novel insights into the molecular basis of calcific aortic valve disease, J. Thorac. Dis., 12 (2020), 6419–6421. https://doi.org/10.21037/jtd-20-1669 doi: 10.21037/jtd-20-1669
![]() |
[5] |
A. Kapelouzou, C. Kontogiannis, D. I. Tsilimigras, G. Georgiopoulos, L. Kaklamanis, L. Tsourelis, et al., Differential expression patterns of Toll Like Receptors and Interleukin-37 between calcific aortic and mitral valve cusps in humans, Cytokine, 116 (2019), 150–160. https://doi.org/10.1016/j.cyto.2019.01.009 doi: 10.1016/j.cyto.2019.01.009
![]() |
[6] | J. Podolec, J. Baran, M. Siedlinski, M. Urbanczyk, M. Krupinski, K. Bartus, et al., Serum rantes, transforming growth factor-β1 and interleukin-6 levels correlate with cardiac muscle fibrosis in patients with aortic valve stenosis, J. Physiol. Pharmacol., 69 (2018), 615–623. |
[7] |
J. Weisell, P. Ohukainen, J. Näpänkangas, S. Ohlmeier, U. Bergmann, T. Peltonen, et al., Heat shock protein 90 is downregulated in calcific aortic valve disease, BMC Cardiovasc. Disord., 19 (2019), 306. https://doi.org/10.1186/s12872-019-01294-2 doi: 10.1186/s12872-019-01294-2
![]() |
[8] |
G. Karadimou, O. Plunde, S. Pawelzik, M. Carracedo, P. Eriksson, A. Franco-Cereceda, et al., TLR7 Expression Is Associated with M2 Macrophage Subset in Calcific Aortic Valve Stenosis, Cells, 9 (2020), 9071710. https://doi.org/10.3390/cells9071710 doi: 10.3390/cells9071710
![]() |
[9] |
G. Li, W. Qiao, W. Zhang, F. Li, J. Shi, N. Dong, The shift of macrophages toward M1 phenotype promotes aortic valvular calcification, J. Thorac. Cardiovasc. Surg., 153 (2017), 1318–1327. https://doi.org/10.1016/j.jtcvs.2017.01.052 doi: 10.1016/j.jtcvs.2017.01.052
![]() |
[10] |
M. A. Raddatz, T. Huffstater, M. R. Bersi, B. I. Reinfeld, M. Z. Madden, S. E. Booton, et al., Macrophages promote aortic valve cell calcification and alter STAT3 splicing, Arterioscler., Thromb., Vasc. Biol., 40 (2020), e153–e165. https://doi.org/10.1161/ATVBAHA.120.314360 doi: 10.1161/ATVBAHA.120.314360
![]() |
[11] |
B. Erkhem-Ochir, W. Tatsuishi, T. Yokobori, T. Ohno, K. Hatori, T. Handa, et al., Inflammatory and immune checkpoint markers are associated with the severity of aortic stenosis, JTCVS Open, 5 (2021), 1–12. https://doi.org/10.1016/j.xjon.2020.11.007 doi: 10.1016/j.xjon.2020.11.007
![]() |
[12] |
S. H. Lee, J. Choi, Involvement of inflammatory responses in the early development of calcific aortic valve disease: lessons from statin therapy, Anim. Cells Syst., 22 (2018), 390–399. https://doi.org/10.1080/19768354.2018.1528175 doi: 10.1080/19768354.2018.1528175
![]() |
[13] |
N. Venardos, X. Deng, Q. Yao, M. J. Weyant, T. B. Reece, X. Meng, et al., Simvastatin reduces the TLR4-induced inflammatory response in human aortic valve interstitial cells, J. Surg. Res., 230 (2018), 101–109. https://doi.org/10.1016/j.jss.2018.04.054 doi: 10.1016/j.jss.2018.04.054
![]() |
[14] |
P. Sarajlic, O. Plunde, A. Franco-Cereceda, M. Bäck, Artificial intelligence models reveal sex-specific gene expression in aortic valve calcification, JACC Basic Transl. Sci., 6 (2021), 403–412. https://doi.org/10.1016/j.jacbts.2021.02.005 doi: 10.1016/j.jacbts.2021.02.005
![]() |
[15] |
J. Qiu, B. Peng, Y. Tang, Y. Qian, Pi Guo, M. Li, et al., CpG methylation signature predicts recurrence in early-stage hepatocellular carcinoma: Results from a multicenter study, J. Clin. Oncol., 35 (2017), 734–742. https://doi.org/10.1200/JCO.2016.68.2153 doi: 10.1200/JCO.2016.68.2153
![]() |
[16] |
E. Zhao, H. Xie, Y. Zhang, Predicting diagnostic gene biomarkers associated with immune infiltration in patients with acute myocardial infarction, Front. Cardiovasc. Med., 7 (2020), 586871. https://doi.org/10.3389/fcvm.2020.586871 doi: 10.3389/fcvm.2020.586871
![]() |
[17] |
X. Zheng, F. Wang, J. Zhang, X. Cui, F. Jiang, N. Chen, et al., Using machine learning to predict atrial fibrillation diagnosed after ischemic stroke, Int. J. Cardiol., 347 (2022), 21–27. https://doi.org/10.1016/j.ijcard.2021.11.005 doi: 10.1016/j.ijcard.2021.11.005
![]() |
[18] |
D. Lambrechts, E. Wauters, B. Boeckx, S. Aibar, D. Nittner, O. Burton, et al., Phenotype molding of stromal cells in the lung tumor microenvironment, Nat. Med., 24 (2018), 1277–1289. https://doi.org/10.1038/s41591-018-0096-5 doi: 10.1038/s41591-018-0096-5
![]() |
[19] |
M. E. Ritchie, B. Phipson, D. Wu, Y. Hu, C. W. Law, W. Shi, et al., limma powers differential expression analyses for RNA-sequencing and microarray studies, Nucleic Acids Res., 43 (2015), e47. https://doi.org/10.1093/nar/gkv007 doi: 10.1093/nar/gkv007
![]() |
[20] |
C. Ginestet, ggplot2: Elegant graphics for data analysis, J. R. Stat. Soc. Ser. A, 174 (2011), 245–245. https://doi.org/10.1111/j.1467-985X.2010.00676_9.x doi: 10.1111/j.1467-985X.2010.00676_9.x
![]() |
[21] |
A. Liberzon, A. Subramanian, R. Pinchback, H. Thorvaldsdóttir, P. Tamayo, J. P. Mesirov, Molecular signatures database (MSigDB) 3.0, Bioinformatics, 27 (2011), 1739–1740. https://doi.org/10.1093/bioinformatics/btr260 doi: 10.1093/bioinformatics/btr260
![]() |
[22] |
P. Ghosh, S. Azam, M. Jonkman, A. Karim, F. M. J. M. Shamrat, E. Ignatious, et al., Efficient prediction of cardiovascular disease using machine learning algorithms with relief and LASSO feature selection techniques, IEEE Access, 9 (2021), 19304–19326. https://doi.org/10.1109/ACCESS.2021.3053759 doi: 10.1109/ACCESS.2021.3053759
![]() |
[23] |
B. Richhariya, M. Tanveer, A. H. Rashid, Diagnosis of Alzheimer's disease using universum support vector machine based recursive feature elimination (USVM-RFE), Biomed. Signal Process. Control, 59 (2020), 101903. https://doi.org/10.1016/j.bspc.2020.101903 doi: 10.1016/j.bspc.2020.101903
![]() |
[24] |
J. Friedman, T. Hastie, R. Tibshirani, Regularization paths for generalized linear models via coordinate descent, J. Stat. Softw., 33 (2010), 1–22. https://doi.org/10.18637/jss.v033.i01 doi: 10.18637/jss.v033.i01
![]() |
[25] |
M. Huang, Y. Hung, W. M. Lee, R. K. Li, B. Jiang, SVM-RFE based feature selection and Taguchi parameters optimization for multiclass SVM classifier, Sci. World J., 2014 (2014), 795624. https://doi.org/10.1155/2014/795624 doi: 10.1155/2014/795624
![]() |
[26] |
B. Xiao, L. Liu, A. Li, C. Xiang, P. Wang, H. Li, et al., Identification and verification of immune-related gene prognostic signature based on ssGSEA for osteosarcoma, Front. Oncol., 10 (2020), 607622. https://doi.org/10.3389/fonc.2020.607622 doi: 10.3389/fonc.2020.607622
![]() |
[27] |
J. Kawada, S. Takeuchi, H. Imai, T. Okumura, K. Horiba, T. Suzuki, et al., Immune cell infiltration landscapes in pediatric acute myocarditis analyzed by CIBERSORT, J. Cardiol., 77 (2021), 174–178. https://doi.org/10.1016/j.jjcc.2020.08.004 doi: 10.1016/j.jjcc.2020.08.004
![]() |
[28] |
M. Friendly, Corrgrams: Exploratory displays for correlation matrices, Am. Stat., 56 (2002), 316–324. https://doi.org/10.1198/000313002533 doi: 10.1198/000313002533
![]() |
[29] |
A. L. Dailey, Metabolomic bioinformatic analysis, Methods Mol. Biol., 1606 (2017), 341–352. https://doi.org/10.1007/978-1-4939-6990-6_22 doi: 10.1007/978-1-4939-6990-6_22
![]() |
[30] |
K. Hu, Become competent within one day in generating boxplots and violin plots for a novice without prior r experience, Methods Protoc., 3 (2020), E64. https://doi.org/10.3390/mps3040064 doi: 10.3390/mps3040064
![]() |
[31] |
O. Kwon, H. Lee, H. Kong, E. Kwon, J. E. Park, W. Lee, et al., Connectivity map-based drug repositioning of bortezomib to reverse the metastatic effect of GALNT14 in lung cancer, Oncogene, 39 (2020), 4567–4580. https://doi.org/10.1038/s41388-020-1316-2 doi: 10.1038/s41388-020-1316-2
![]() |
[32] |
F. E. C. M. Peeters, S. J. R. Meex, M. R. Dweck, E. Aikawa, H. J. G. M. Crijns, L. J. Schurgers, et al., Calcific aortic valve stenosis: hard disease in the heart: A biomolecular approach towards diagnosis and treatment, Eur. Heart J., 39 (2018), 2618–2624. https://doi.org/10.1093/eurheartj/ehx653 doi: 10.1093/eurheartj/ehx653
![]() |
[33] |
K. I. Cho, I. Sakuma, I. Sohn, S. Jo, K. K. Koh, Inflammatory and metabolic mechanisms underlying the calcific aortic valve disease, Atherosclerosis, 277 (2018), 60–65. https://doi.org/10.1016/j.atherosclerosis.2018.08.029 doi: 10.1016/j.atherosclerosis.2018.08.029
![]() |
[34] |
M. Erdoğan, S. Öztürk, B. Kardeşler, M. Yiğitbaşı, H. A. Kasapkara, S. Baştuğ, et al., The relationship between calcific severe aortic stenosis and systemic immune-inflammation index, Echocardiography, 38 (2021), 737–744. https://doi.org/10.1111/echo.15044 doi: 10.1111/echo.15044
![]() |
[35] |
A. G. Kutikhin, A. E. Yuzhalin, E. B. Brusina, A. V. Ponasenko, A. S. Golovkin, O. L. Barbarash, et al., Genetic predisposition to calcific aortic stenosis and mitral annular calcification, Mol. Biol. Rep., 41 (2014), 5645–5663. https://doi.org/10.1007/s11033-014-3434-9 doi: 10.1007/s11033-014-3434-9
![]() |
[36] |
M. Azova, K. Timizheva, A. A. Aissa, M. Blagonravov, O. Gigani, A. Aghajanyan, et al., Gene polymorphisms of the renin-angiotensin-aldosterone system as risk factors for the development of in-stent restenosis in patients with stable coronary artery disease, Biomolecules, 11 (2021), 763. https://doi.org/10.3390/biom11050763 doi: 10.3390/biom11050763
![]() |
[37] |
B. Saravi, Z. Li, C. N. Lang, B. Schmid, F. K. Lang, S. Grad, et al., The tissue renin-angiotensin system and its role in the pathogenesis of major human diseases: Quo vadis?, Cells, 10 (2021), 650. https://doi.org/10.3390/cells10030650 doi: 10.3390/cells10030650
![]() |
[38] |
P. J. Pussinen, M. Jauhiainen, J. Metso, L. E. Pyle, Y. L. Marcel, N. H. Fidge, et al., Binding of phospholipid transfer protein (PLTP) to apolipoproteins A-I and A-II: location of a PLTP binding domain in the amino terminal region of apoA-I, J. Lipid Res., 39 (1998), 152–161. https://doi.org/10.1016/S0022-2275(20)34211-5 doi: 10.1016/S0022-2275(20)34211-5
![]() |
[39] |
J. I. Lommi, P. T. Kovanen, M. Jauhiainen, M. Lee-Rueckert, M. Kupari, S. Helske, High-density lipoproteins (HDL) are present in stenotic aortic valves and may interfere with the mechanisms of valvular calcification, Atherosclerosis, 219 (2011), 538–544. https://doi.org/10.1016/j.atherosclerosis.2011.08.027 doi: 10.1016/j.atherosclerosis.2011.08.027
![]() |
[40] |
M. A. Heuschkel, N. T. Skenteris, J. D. Hutcheson, D. D. Valk, J. Bremer, P. Goody, et al., Integrative multi-omics analysis in calcific aortic valve disease reveals a link to the formation of amyloid-like deposits, Cells, 9 (2020), E2164. https://doi.org/10.3390/cells9102164 doi: 10.3390/cells9102164
![]() |
[41] |
F. Schlotter, R. C. C. Freitas, M. A. Rogers, M. C. Blaser, P. Wu, H. Higashi, et al., ApoC-III is a novel inducer of calcification in human aortic valves, J. Biol. Chem., 296 (2020), 100193. https://doi.org/10.1074/jbc.RA120.015700 doi: 10.1074/jbc.RA120.015700
![]() |
[42] |
K. Zhang, J. Zheng, Y. Chen, J. Dong, Z. Li, Y. Chiang, et al., Inducible phospholipid transfer protein deficiency ameliorates atherosclerosis, Atherosclerosis, 324 (2021), 9–17. https://doi.org/10.1016/j.atherosclerosis.2021.03.011 doi: 10.1016/j.atherosclerosis.2021.03.011
![]() |
[43] |
N. Biswas, E. Curello, D. T. O'Connor, S. K. Mahata, Chromogranin/secretogranin proteins in murine heart: myocardial production of chromogranin A fragment catestatin (Chga364–384), Cell Tissue Res., 342 (2010), 353–361. https://doi.org/10.1007/s00441-010-1059-4 doi: 10.1007/s00441-010-1059-4
![]() |
[44] |
M. Liu, M. Luo, H. Sun, B. Ni, Y. Shao, Integrated bioinformatics analysis predicts the key genes involved in aortic valve calcification: From hemodynamic changes to extracellular remodeling, Tohoku J. Exp. Med., 243 (2017), 263–273. https://doi.org/10.1620/tjem.243.263 doi: 10.1620/tjem.243.263
![]() |
![]() ![]() |
![]() |
1. | Qianxi Yang, Yanlong Yang, Effects of an update mechanism based on combinatorial memory and high-reputation learning objects on the evolution of cooperation, 2025, 495, 00963003, 129309, 10.1016/j.amc.2025.129309 | |
2. | Meng Zhou, Yanlong Yang, Shuwen Xiang, Effect of community learning mechanism on cooperation in conflict societies, 2025, 192, 09600779, 116046, 10.1016/j.chaos.2025.116046 |
PSO | Particle swarm optimization |
EWA | Experience-adding weight affinity |
IGA | Infinitesimal gradient algorithm |
GIGA | Generalized infinitesimal gradient algorithm |
PGPSO | Population game particle swarm optimization |