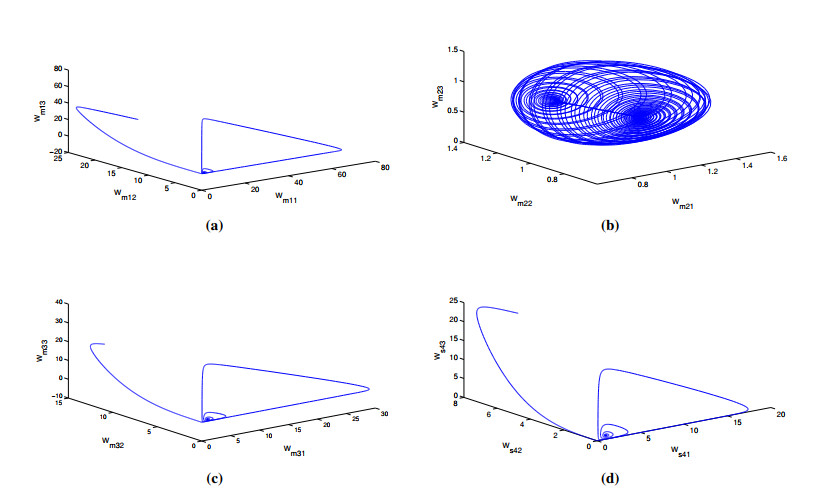
Numerous sustainability initiatives have been discussed and widely adopted in various sectors worldwide. This research aims to identify which essential parameters affect sustainable supply chain management (SSCM) practice through the case of the Taiwanese fresh-fruit sector. An industry has great importance to the households of indigenous people, significantly contributing to the sustainable development in rural areas of Taiwan. A total of twelve SSCM parameters have been verified, and then the authors conducted the TOPSIS approach process to determine the importance level of these SSCM parameters. The analysis results suggested that "Collaboration", "Distribution and Logistics service", "Customer" have supreme importance, respectively. On the contrary, at the bottom of the prioritized list is three variables consisting of "Warehouse/Storage", "Organizational social responsibilities", and "Processing and packaging". This is the unique study to consider SSCM practice through the case of the Taiwanese fresh-fruit chain; hence, these key findings could be a valuable reference for the top managers to make decisions.
Citation: Manh-Hoang Do, Yung-Fu Huang. Evaluation of parameters for the sustainable supply chain management: a Taiwanese fresh-fruit sector[J]. AIMS Environmental Science, 2022, 9(1): 16-32. doi: 10.3934/environsci.2022002
[1] | Honglei Wang, Wenliang Zeng, Xiaoling Huang, Zhaoyang Liu, Yanjing Sun, Lin Zhang . MTTLm6A: A multi-task transfer learning approach for base-resolution mRNA m6A site prediction based on an improved transformer. Mathematical Biosciences and Engineering, 2024, 21(1): 272-299. doi: 10.3934/mbe.2024013 |
[2] | Shanzheng Wang, Xinhui Xie, Chao Li, Jun Jia, Changhong Chen . Integrative network analysis of N6 methylation-related genes reveal potential therapeutic targets for spinal cord injury. Mathematical Biosciences and Engineering, 2021, 18(6): 8174-8187. doi: 10.3934/mbe.2021405 |
[3] | Pingping Sun, Yongbing Chen, Bo Liu, Yanxin Gao, Ye Han, Fei He, Jinchao Ji . DeepMRMP: A new predictor for multiple types of RNA modification sites using deep learning. Mathematical Biosciences and Engineering, 2019, 16(6): 6231-6241. doi: 10.3934/mbe.2019310 |
[4] | Yong Zhu, Zhipeng Jiang, Xiaohui Mo, Bo Zhang, Abdullah Al-Dhelaan, Fahad Al-Dhelaan . A study on the design methodology of TAC3 for edge computing. Mathematical Biosciences and Engineering, 2020, 17(5): 4406-4421. doi: 10.3934/mbe.2020243 |
[5] | Atefeh Afsar, Filipe Martins, Bruno M. P. M. Oliveira, Alberto A. Pinto . A fit of CD4+ T cell immune response to an infection by lymphocytic choriomeningitis virus. Mathematical Biosciences and Engineering, 2019, 16(6): 7009-7021. doi: 10.3934/mbe.2019352 |
[6] | Tamás Tekeli, Attila Dénes, Gergely Röst . Adaptive group testing in a compartmental model of COVID-19*. Mathematical Biosciences and Engineering, 2022, 19(11): 11018-11033. doi: 10.3934/mbe.2022513 |
[7] | Wenli Cheng, Jiajia Jiao . An adversarially consensus model of augmented unlabeled data for cardiac image segmentation (CAU+). Mathematical Biosciences and Engineering, 2023, 20(8): 13521-13541. doi: 10.3934/mbe.2023603 |
[8] | Tongmeng Jiang, Pan Jin, Guoxiu Huang, Shi-Cheng Li . The function of guanylate binding protein 3 (GBP3) in human cancers by pan-cancer bioinformatics. Mathematical Biosciences and Engineering, 2023, 20(5): 9511-9529. doi: 10.3934/mbe.2023418 |
[9] | Xin Yu, Jun Liu, Ruiwen Xie, Mengling Chang, Bichun Xu, Yangqing Zhu, Yuancai Xie, Shengli Yang . Construction of a prognostic model for lung squamous cell carcinoma based on seven N6-methylandenosine-related autophagy genes. Mathematical Biosciences and Engineering, 2021, 18(5): 6709-6723. doi: 10.3934/mbe.2021333 |
[10] | Tahir Rasheed, Faran Nabeel, Muhammad Bilal, Yuping Zhao, Muhammad Adeel, Hafiz. M. N. Iqbal . Aqueous monitoring of toxic mercury through a rhodamine-based fluorescent sensor. Mathematical Biosciences and Engineering, 2019, 16(4): 1861-1873. doi: 10.3934/mbe.2019090 |
Numerous sustainability initiatives have been discussed and widely adopted in various sectors worldwide. This research aims to identify which essential parameters affect sustainable supply chain management (SSCM) practice through the case of the Taiwanese fresh-fruit sector. An industry has great importance to the households of indigenous people, significantly contributing to the sustainable development in rural areas of Taiwan. A total of twelve SSCM parameters have been verified, and then the authors conducted the TOPSIS approach process to determine the importance level of these SSCM parameters. The analysis results suggested that "Collaboration", "Distribution and Logistics service", "Customer" have supreme importance, respectively. On the contrary, at the bottom of the prioritized list is three variables consisting of "Warehouse/Storage", "Organizational social responsibilities", and "Processing and packaging". This is the unique study to consider SSCM practice through the case of the Taiwanese fresh-fruit chain; hence, these key findings could be a valuable reference for the top managers to make decisions.
The constituent members in a system mainly found in nature can be interacting with each other through cooperation and competition. Demonstrations for such systems involve biological species, countries, businesses, and many more. It's very much intriguing to investigate in a comprehensive manner numerous social as well as biological interactions existent in dissimilar species/entities utilizing mathematical modeling. The predation and the competition species are the most famous interactions among all such types of interactions. Importantly, Lotka [1] and Volterra [2] in the 1920s have announced individually the classic equations portraying population dynamics. Such illustrious equations are notably described as predator-prey (PP) equations or Lotka-Volterra (LV) equations. In this structure, PP/LV model represents the most influential model for interacting populations. The interplay between prey and predator together with additional factors has been a prominent topic in mathematical ecology for a long period. Arneodo et al. [3] have established in 1980 that a generalized Lotka-Volterra biological system (GLVBS) would depict chaos phenomena in an ecosystem for some explicitly selected system parameters and initial conditions. Additionally, Samardzija and Greller [4] demonstrated in 1988 that GLVBS would procure chaotic reign from the stabled state via rising fractal torus. LV model was initially developed as a biological concept, yet it is utilized in enormous diversified branches for research [5,6,7,8]. Synchronization essentially is a methodology of having different chaotic systems (non-identical or identical) following exactly a similar trajectory, i.e., the dynamical attributes of the slave system are locked finally into the master system. Specifically, synchronization and control have a wide spectrum for applications in engineering and science, namely, secure communication [9], encryption [10,11], ecological model [12], robotics [13], neural network [14], etc. Recently, numerous types of secure communication approaches have been explored [15,16,17,18] such as chaos modulation [18,19,20,21], chaos shift keying [22,23] and chaos masking [9,17,20,24]. In chaos communication schemes, the typical key idea for transmitting a message through chaotic/hyperchaotic models is that a message signal is nested in the transmitter system/model which originates a chaotic/ disturbed signal. Afterwards, this disturbed signal has been emitted to the receiver through a universal channel. The message signal would finally be recovered by the receiver. A chaotic model has been intrinsically employed both as receiver and transmitter. Consequently, this area of chaotic synchronization & control has sought remarkable considerations among differential research fields.
Most prominently, synchronization theory has been in existence for over 30 years due to the phenomenal research of Pecora and Carroll [25] established in 1990 using drive-response/master-slave/leader-follower configuration. Consequently, many authors and researchers have started introducing and studying numerous control and synchronization methods [9,26,27,28,29,30,31,32,33,34,35,36] etc. to achieve stabilized chaotic systems for possessing stability. In [37], researchers discussed optimal synchronization issues in similar GLVBSs via optimal control methodology. In [38,39], the researchers studied the adaptive control method (ACM) to synchronize chaotic GLVBSs. Also, researchers [40] introduced a combination difference anti-synchronization scheme in similar chaotic GLVBSs via ACM. In addition, authors [41] investigated a combination synchronization scheme to control chaos existing in GLVBSs using active control strategy (ACS). Bai and Lonngren [42] first proposed ACS in 1997 for synchronizing and controlling chaos found in nonlinear dynamical systems. Furthermore, compound synchronization using ACS was first advocated by Sun et al. [43] in 2013. In [44], authors discussed compound difference anti-synchronization scheme in four chaotic systems out of which two chaotic systems are considered as GLVBSs using ACS and ACM along with applications in secure communications of chaos masking type in 2019. Some further research works [45,46] based on ACS have been reported in this direction. The considered chaotic GLVBS offers a generalization that allows higher-order biological terms. As a result, it may be of interest in cases where biological systems experience cataclysmic changes. Unfortunately, some species will be under competitive pressure in the coming years and decades. This work may be comprised as a step toward preserving as many currently living species as possible by using the proposed synchronization approach which is based on master-slave configuration and Lyapunov stability analysis.
In consideration of the aforementioned discussions and observations, our primary focus here is to develop a systematic approach for investigating compound difference anti-synchronization (CDAS) approach in 4 similar chaotic GLVBSs via ACS. The considered ACS is a very efficient yet theoretically rigorous approach for controlling chaos found in GLVBSs. Additionally, in view of widely known Lyapunov stability analysis (LSA) [47], we discuss actively designed biological control law & convergence for synchronization errors to attain CDAS synchronized states.
The major attributes for our proposed research in the present manuscript are:
● The proposed CDAS methodology considers four chaotic GLVBSs.
● It outlines a robust CDAS approach based active controller to achieve compound difference anti-synchronization in discussed GLVBSs & conducts oscillation in synchronization errors along with extremely fast convergence.
● The construction of the active control inputs has been executed in a much simplified fashion utilizing LSA & master-salve/ drive-response configuration.
● The proposed CDAS approach in four identical chaotic GLVBSs of integer order utilizing ACS has not yet been analyzed up to now. This depicts the novelty of our proposed research work.
This manuscript is outlined as follows: Section 2 presents the problem formulation of the CDAS scheme. Section 3 designs comprehensively the CDAS scheme using ACS. Section 4 consists of a few structural characteristics of considered GLVBS on which CDAS is investigated. Furthermore, the proper active controllers having nonlinear terms are designed to achieve the proposed CDAS strategy. Moreover, in view of Lyapunov's stability analysis (LSA), we have examined comprehensively the biological controlling laws for achieving global asymptotical stability of the error dynamics for the discussed model. In Section 5, numerical simulations through MATLAB are performed for the illustration of the efficacy and superiority of the given scheme. Lastly, we also have presented some conclusions and the future prospects of the discussed research work in Section 6.
We here formulate a methodology to examine compound difference anti-synchronization (CDAS) scheme viewing master-slave framework in four chaotic systems which would be utilized in the coming up sections.
Let the scaling master system be
˙wm1= f1(wm1), | (2.1) |
and the base second master systems be
˙wm2= f2(wm2), | (2.2) |
˙wm3= f3(wm3). | (2.3) |
Corresponding to the aforementioned master systems, let the slave system be
˙ws4= f4(ws4)+U(wm1,wm2,wm3,ws4), | (2.4) |
where wm1=(wm11,wm12,...,wm1n)T∈Rn, wm2=(wm21,wm22,...,wm2n)T∈Rn, wm3=(wm31,wm32,...,wm3n)T∈Rn, ws4=(ws41,ws42,...,ws4n)T∈Rn are the state variables of the respective chaotic systems (2.1)–(2.4), f1,f2,f3,f4:Rn→Rn are four continuous vector functions, U=(U1,U2,...,Un)T:Rn×Rn×Rn×Rn→Rn are appropriately constructed active controllers.
Compound difference anti-synchronization error (CDAS) is defined as
E=Sws4+Pwm1(Rwm3−Qwm2), |
where P=diag(p1,p2,.....,pn),Q=diag(q1,q2,.....,qn),R=diag(r1,r2,.....,rn),S=diag(s1,s2,.....,sn) and S≠0.
Definition: The master chaotic systems (2.1)–(2.3) are said to achieve CDAS with slave chaotic system (2.4) if
limt→∞‖E(t)‖=limt→∞‖Sws4(t)+Pwm1(t)(Rwm3(t)−Qwm2(t))‖=0. |
We now present our proposed CDAS approach in three master systems (2.1)–(2.3) and one slave system (2.4). We next construct the controllers based on CDAS approach by
Ui= ηisi−(f4)i−KiEisi, | (3.1) |
where ηi=pi(f1)i(riwm3i−qiwm2i)+piwm1i(ri(f3)i−qi(f2)i), for i=1,2,...,n.
Theorem: The systems (2.1)–(2.4) will attain the investigated CDAS approach globally and asymptotically if the active control functions are constructed in accordance with (3.1).
Proof. Considering the error as
Ei= siws4i+piwm1i(riwm3i−qiwm2i),fori=1,2,3,.....,n. |
Error dynamical system takes the form
˙Ei= si˙ws4i+pi˙wm1i(riwm3i−qiwm2i)+piwm1i(ri˙wm3i−qi˙wm2i)= si((f4)i+Ui)+pi(f1)i(riwm3i−qiwm2i)+piwm1i(ri(f3)i−qi(f2)i)= si((f4)i+Ui)+ηi, |
where ηi=pi(f1)i(riwm3i−qiwm2i)+piwm1i(ri(f3)i−qi(f2)i), i=1,2,3,....,n. This implies that
˙Ei= si((f4)i−ηisi−(f4)i−KiEisi)+ηi= −KiEi | (3.2) |
The classic Lyapunov function V(E(t)) is described by
V(E(t))= 12ETE= 12ΣE2i |
Differentiation of V(E(t)) gives
˙V(E(t))=ΣEi˙Ei |
Using Eq (3.2), one finds that
˙V(E(t))=ΣEi(−KiEi)= −ΣKiE2i). | (3.3) |
An appropriate selection of (K1,K1,.......,Kn) makes ˙V(E(t)) of eq (3.3), a negative definite. Consequently, by LSA [47], we obtain
limt→∞Ei(t)=0,(i=1,2,3). |
Hence, the master systems (2.1)–(2.3) and slave system (2.4) have attained desired CDAS strategy.
We now describe GLVBS as the scaling master system:
{˙wm11=wm11−wm11wm12+b3w2m11−b1w2m11wm13,˙wm12=−wm12+wm11wm12,˙wm13=b2wm13+b1w2m11wm13, | (4.1) |
where (wm11,wm12,wm13)T∈R3 is state vector of (4.1). Also, wm11 represents the prey population and wm12, wm13 denote the predator populations. For parameters b1=2.9851, b2=3, b3=2 and initial conditions (27.5,23.1,11.4), scaling master GLVBS displays chaotic/disturbed behaviour as depicted in Figure 1(a).
The base master systems are the identical chaotic GLVBSs prescribed respectively as:
{˙wm21=wm21−wm21wm22+b3w2m21−b1w2m21wm23,˙wm22=−wm22+wm21wm22,˙wm23=b2wm23+b1w2m21wm23, | (4.2) |
where (wm21,wm22,wm23)T∈R3 is state vector of (4.2). For parameter values b1=2.9851, b2=3, b3=2, this base master GLVBS shows chaotic/disturbed behaviour for initial conditions (1.2,1.2,1.2) as displayed in Figure 1(b).
{˙wm31=wm31−wm31wm32+b3w2m31−b1w2m31wm33,˙wm32=−wm32+wm31wm32,˙wm33=b2wm33+b1w2m31wm33, | (4.3) |
where (wm31,wm32,wm33)T∈R3 is state vector of (4.3). For parameters b1=2.9851, b2=3, b3=2, this second base master GLVBS displays chaotic/disturbed behaviour for initial conditions (2.9,12.8,20.3) as shown in Figure 1(c).
The slave system, represented by similar GLVBS, is presented by
{˙ws41=ws41−ws41ws42+b3w2s41−b1w2s41ws43+U1,˙ws42=−ws42+ws41ws42+U2,˙ws43=b2ws43+b1w2s41ws43+U3, | (4.4) |
where (ws41,ws42,ws43)T∈R3 is state vector of (4.4). For parameter values, b1=2.9851, b2=3, b3=2 and initial conditions (5.1,7.4,20.8), the slave GLVBS exhibits chaotic/disturbed behaviour as mentioned in Figure 1(d).
Moreover, the detailed theoretical study for (4.1)–(4.4) can be found in [4]. Further, U1, U2 and U3 are controllers to be determined.
Next, the CDAS technique has been discussed for synchronizing the states of chaotic GLVBS. Also, LSA-based ACS is explored & the necessary stability criterion is established.
Here, we assume P=diag(p1,p2,p3), Q=diag(q1,q2,q3), R=diag(r1,r2,r3), S=diag(s1,s2,s3). The scaling factors pi,qi,ri,si for i=1,2,3 are selected as required and can assume the same or different values.
The error functions (E1,E2,E3) are defined as:
{E1=s1ws41+p1wm11(r1wm31−q1wm21),E2=s2ws42+p2wm12(r2wm32−q2wm22),E3=s3ws43+p3wm13(r3wm33−q3wm23). | (4.5) |
The major objective of the given work is the designing of active control functions Ui,(i=1,2,3) ensuring that the error functions represented in (4.5) must satisfy
limt→∞Ei(t)=0for(i=1,2,3). |
Therefore, subsequent error dynamics become
{˙E1=s1˙ws41+p1˙wm11(r1wm31−q1wm21)+p1wm11(r1˙wm31−q1˙wm21),˙E2=s2˙ws42+p2˙wm12(r2wm32−q2wm22)+p2wm12(r2˙wm32−q2˙wm22),˙E3=s3˙ws43+p3˙wm13(r3wm33−q3wm23)+p3wm13(r3˙wm33−q3˙wm23). | (4.6) |
Using (4.1), (4.2), (4.3), and (4.5) in (4.6), the error dynamics simplifies to
{˙E1=s1(ws41−ws41ws42+b3w2s41−b1w2s41ws43+U1)+p1(wm11−wm11wm12+b3w2m11−b1w2m11wm13)(r1wm31−q1wm21)+p1wm11(r1(wm31−wm31wm32+b3w2m31−b1w2m31wm33)−q1(wm21−wm21wm22+b3w2m21−b1w2m21wm23),˙E2=s2(−ws42+ws41ws42+U2)+p2(−wm12+wm11wm12)(r2wm32−q2wm22)+p2wm12(r2(−wm32+wm31wm32)−q2(−wm22+wm21wm22)),˙E3=s3(b2ws43+b1w2s41ws43+U3)+p3(b2wm13+b1w2m11wm13)(r3wm33−q3wm23)+p3wm13(r3(b2wm33+b1w2m31wm33)−q3(b2wm23+b1w2m21wm23)). | (4.7) |
Let us now choose the active controllers:
U1= η1s1−(f4)1−K1E1s1, | (4.8) |
where η1=p1(f1)1(r1wm31−q1wm21)+p1wm11(r1(f3)1−q1(f2)1), as described in (3.1).
U2= η2s2−(f4)2−K2E2s2, | (4.9) |
where η2=p2(f1)2(r2wm32−q2wm22)+p2wm12(r2(f3)2−q2(f2)2).
U3= η3s3−(f4)3−K3E3s3, | (4.10) |
where η3=p3(f1)3(r3wm33−q3wm23)+p3wm13(r3(f3)3−q3(f2)3) and K1>0,K2>0,K3>0 are gaining constants.
By substituting the controllers (4.8), (4.9) and (4.10) in (4.7), we obtain
{˙E1=−K1E1,˙E2=−K2E2,˙E3=−K3E3. | (4.11) |
Lyapunov function V(E(t)) is now described by
V(E(t))= 12[E21+E22+E23]. | (4.12) |
Obviously, the Lyapunov function V(E(t)) is +ve definite in R3. Therefore, the derivative of V(E(t)) as given in (4.12) can be formulated as:
˙V(E(t))= E1˙E1+E2˙E2+E3˙E3. | (4.13) |
Using (4.11) in (4.13), one finds that
˙V(E(t))= −K1E21−K2E22−K3E23<0, |
which displays that ˙V(E(t)) is -ve definite.
In view of LSA [47], we, therefore, understand that CDAS error dynamics is globally as well as asymptotically stable, i.e., CDAS error E(t)→0 asymptotically for t→∞ to each initial value E(0)∈R3.
This section conducts a few simulation results for illustrating the efficacy of the investigated CDAS scheme in identical chaotic GLVBSs using ACS. We use 4th order Runge-Kutta algorithm for solving the considered ordinary differential equations. Initial conditions for three master systems (4.1)–(4.3) and slave system (4.4) are (27.5,23.1,11.4), (1.2,1.2,1.2), (2.9,12.8,20.3) and (14.5,3.4,10.1) respectively. We attain the CDAS technique among three masters (4.1)–(4.3) and corresponding one slave system (4.4) by taking pi=qi=ri=si=1, which implies that the slave system would be entirely anti-synchronized with the compound of three master models for i=1,2,3. In addition, the control gains (K1,K2,K3) are taken as 2. Also, Figure 2(a)–(c) indicates the CDAS synchronized trajectories of three master (4.1)–(4.3) & one slave system (4.4) respectively. Moreover, synchronization error functions (E1,E2,E3)=(51.85,275.36,238.54) approach 0 as t tends to infinity which is exhibited via Figure 2(d). Hence, the proposed CDAS strategy in three masters and one slave models/systems has been demonstrated computationally.
In this work, the investigated CDAS approach in similar four chaotic GLVBSs using ACS has been analyzed. Lyapunov's stability analysis has been used to construct proper active nonlinear controllers. The considered error system, on the evolution of time, converges to zero globally & asymptotically via our appropriately designed simple active controllers. Additionally, numerical simulations via MATLAB suggest that the newly described nonlinear control functions are immensely efficient in synchronizing the chaotic regime found in GLVBSs to fitting set points which exhibit the efficacy and supremacy of our proposed CDAS strategy. Exceptionally, both analytic theory and computational results are in complete agreement. Our proposed approach is simple yet analytically precise. The control and synchronization among the complex GLVBSs with the complex dynamical network would be an open research problem. Also, in this direction, we may extend the considered CDAS technique on chaotic systems that interfered with model uncertainties as well as external disturbances.
The authors gratefully acknowledge Qassim University, represented by the Deanship of Scientific Research, on the financial support for this research under the number 10163-qec-2020-1-3-I during the academic year 1441 AH/2020 AD.
The authors declare there is no conflict of interest.
[1] |
Panigrahi SS, Bahinipati B, Jain V (2019) Sustainable supply chain management: A review of literature and implications for future research. Manag Environ Qual An Int J 30: 1001–1049. https://doi.org/10.1108/MEQ-01-2018-0003 doi: 10.1108/MEQ-01-2018-0003
![]() |
[2] |
Zimon D, Tyan J, Sroufe R (2020) Drivers of sustainable supply chain management: Practices to alignment with un sustainable development goals. Int J Qual Res 14: 219–236. https://doi.org/10.24874/IJQR14.01-14 doi: 10.24874/IJQR14.01-14
![]() |
[3] | Darbari JD, Agarwal V, Sharma R, et al. (2018) Analysis of Impediments to Sustainability in the Food Supply Chain: An Interpretive Structural Modeling Approach, Quality, IT and Business Operations, Springer Nature Singapore Pte Ltd., 57–68. https://doi.org/10.1007/978-981-10-5577-5_5 |
[4] |
Do TN, Kumar V, Do MH (2020) Prioritize the key parameters of Vietnamese coffee industries for sustainability. Int J Product Perform Manag 69: 1153–1176. https://doi.org/10.1108/IJPPM-06-2019-0282 doi: 10.1108/IJPPM-06-2019-0282
![]() |
[5] | Dania WAP, Xing K, Amer Y (2020) The assessment of collaboration quality: a case of sugar supply chain in Indonesia. Int J Product Perform Manag In press. https://doi.org/10.1108/IJPPM-11-2019-0527 |
[6] |
Santos RR dos, Guarnieri P (2021) Social gains for artisanal agroindustrial producers induced by cooperation and collaboration in agri-food supply chain. Soc Responsib J 17: 1131–1149. https://doi.org/10.1108/SRJ-09-2019-0323 doi: 10.1108/SRJ-09-2019-0323
![]() |
[7] |
Narimissa O, Kangarani-Farahani A, Molla-Alizadeh-Zavardehi S (2020) Drivers and barriers for implementation and improvement of Sustainable Supply Chain Management. Sustain Dev 28: 247–258. https://doi.org/10.1002/sd.1998 doi: 10.1002/sd.1998
![]() |
[8] |
Menon RR, Ravi V (2021) An analysis of barriers affecting implementation of sustainable supply chain management in electronics industry: a Grey-DEMATEL approach. J Model Manag. https://doi.org/10.1108/JM2-02-2021-0042 doi: 10.1108/JM2-02-2021-0042
![]() |
[9] |
Pandey N, Bhatnagar M, Ghosh D (2021) An analysis of critical success factors towards sustainable supply chain management–in the context of an engine manufacturing industry. Int J Sustain Eng 0: 1–13. https://doi.org/10.1080/19397038.2021.1966128 doi: 10.1080/19397038.2021.1966128
![]() |
[10] |
Dahooie JH, Babgohari AZ, Meidutė-Kavaliauskienė I, et al. (2021) Prioritising sustainable supply chain management practices by their impact on multiple interacting barriers. Int J Sustain Dev World Ecol 28: 267–290. https://doi.org/10.1080/13504509.2020.1795004 doi: 10.1080/13504509.2020.1795004
![]() |
[11] |
Khan SA, Agyemang M, Ishizaka A, et al. (2021) Barriers and overcoming strategies to multi-tier sustainable supply chain management: an explorative study in an emerging economy. Int J Sustain Eng 00: 1–12. https://doi.org/10.1080/19397038.2021.1986595 doi: 10.1080/19397038.2021.1986595
![]() |
[12] |
Chaudhuri A, Srivastava SK, Srivastava RK, et al. (2016) Risk propagation and its impact on performance in food processing supply chain: A fuzzy interpretive structural modeling based approach. J Model Manag 11: 660–693. https://doi.org/10.1108/JM2-08-2014-0065 doi: 10.1108/JM2-08-2014-0065
![]() |
[13] |
Zimon D, Tyan J, Sroufe R (2019) Implementing sustainable supply chain management: Reactive, cooperative, and dynamic models. Sustain 11. https://doi.org/10.3390/su11247227 doi: 10.3390/su11247227
![]() |
[14] |
Huang YC, Huang CH (2021) Examining the antecedents and consequences of sustainable green supply chain management from the perspective of ecological modernization: evidence from Taiwan's high-tech sector. J Environ Plan Manag 0: 1–32. https://doi.org/10.1080/09640568.2021.1941809 doi: 10.1080/09640568.2021.1941809
![]() |
[15] |
Ba QX, Lu DJ, Kuo WHJ, et al. (2018) Traditional farming and sustainable development of an indigenous community in the mountain area-a case study of Wutai Village in Taiwan. Sustain 10: 1–16. https://doi.org/10.3390/su10103370 doi: 10.3390/su10103370
![]() |
[16] |
Chen KL, Kong WH, Chen CC, et al. (2021) Evaluating benefits of eco-agriculture: The cases of farms along taiwan's east coast in yilan and hualien. Sustain 13: 1–23. https://doi.org/10.3390/su131910889 doi: 10.3390/su131910889
![]() |
[17] | Statista (2021) Statista Inc., Fresh fruit market size in Taiwan 2016-2020, 2021. Available from: https://www.statista.com/statistics/947290/taiwan-fresh-fruit-market-size/. |
[18] |
Kumar V, Verma P, Jha A, et al. (2020) Dynamics of a medium value consumer apparel supply chain key parameters. Int J Product Perform Manag 1–32. https://doi.org/10.1108/IJPPM-10-2019-0501 doi: 10.1108/IJPPM-10-2019-0501
![]() |
[19] |
Yu VF, Tseng LC (2014) Measuring social compliance performance in the global sustainable supply chain: an AHP approach. J Inf Optim Sci 35: 47–72. https://doi.org/10.1080/02522667.2013.876777 doi: 10.1080/02522667.2013.876777
![]() |
[20] |
Negi S, Anand N (2019) Wholesalers perspectives on mango supply chain efficiency in India. J Agribus Dev Emerg Econ 9: 175–200. https://doi.org/10.1108/JADEE-02-2018-0032 doi: 10.1108/JADEE-02-2018-0032
![]() |
[21] |
Raut R, Gardas BB (2018) Sustainable logistics barriers of fruits and vegetables: An interpretive structural modeling approach. Benchmarking An Int J 25: 2589–2610. https://doi.org/10.1108/BIJ-07-2017-0166 doi: 10.1108/BIJ-07-2017-0166
![]() |
[22] |
Accorsi R, Cholette S, Manzini R, et al. (2018) A hierarchical data architecture for sustainable food supply chain management and planning. J Clean Prod 203: 1039–1054. https://doi.org/10.1016/j.jclepro.2018.08.275 doi: 10.1016/j.jclepro.2018.08.275
![]() |
[23] |
Parajuli R, Thoma G, Matlock MD (2019) Environmental sustainability of fruit and vegetable production supply chains in the face of climate change: A review. Sci Total Environ 650: 2863–2879. https://doi.org/10.1016/j.scitotenv.2018.10.019 doi: 10.1016/j.scitotenv.2018.10.019
![]() |
[24] |
Peano C, Girgenti V, Baudino C, et al. (2017) Blueberry supply chain in Italy: Management, innovation and sustainability. Sustain 9: 1–17. https://doi.org/10.3390/su9020261 doi: 10.3390/su9020261
![]() |
[25] |
Bourlakis M, Maglaras G, Aktas E, et al. (2014) Firm size and sustainable performance in food supply chains: Insights from Greek SMEs. Int J Prod Econ 152: 112–130. https://doi.org/10.1016/j.ijpe.2013.12.029 doi: 10.1016/j.ijpe.2013.12.029
![]() |
[26] | Ramirez MJ, Roman IE, Ramos E, et al. (2020) The value of supply chain integration in the Latin American agri-food industry: trust, commitment and performance outcomes. Int J Logist Manag In press. https://doi.org/10.1108/IJLM-02-2020-0097 |
[27] |
Krishnan R, Agarwal R, Bajada C, et al. (2020) Redesigning a food supply chain for environmental sustainability – An analysis of resource use and recovery. J Clean Prod 242: 118374. https://doi.org/10.1016/j.jclepro.2019.118374 doi: 10.1016/j.jclepro.2019.118374
![]() |
[28] |
Petway JR, Lin YP, Wunderlich RF (2019) Analyzing opinions on sustainable agriculture: Toward increasing farmer knowledge of organic practices in Taiwan-Yuanli township. Sustain 11: 1–27. https://doi.org/10.3390/su11143843 doi: 10.3390/su11143843
![]() |
[29] |
Kumar V, Purbey SK, Anal AKD (2016) Losses in litchi at various stages of supply chain and changes in fruit quality parameters. Crop Prot 79: 97–104. https://doi.org/10.1016/j.cropro.2015.10.014 doi: 10.1016/j.cropro.2015.10.014
![]() |
[30] |
Gardas BB, Raut RD, Narkhede B (2018) Evaluating critical causal factors for post-harvest losses (PHL) in the fruit and vegetables supply chain in India using the DEMATEL approach. J Clean Prod 199: 47–61. https://doi.org/10.1016/j.jclepro.2018.07.153 doi: 10.1016/j.jclepro.2018.07.153
![]() |
[31] |
Sharma J, Tyagi M, Bhardwaj A (2020) Parametric review of food supply chain performance implications under different aspects. J Adv Manag Res 17: 421–453. https://doi.org/10.1108/JAMR-10-2019-0193 doi: 10.1108/JAMR-10-2019-0193
![]() |
[32] |
Wu PJ, Huang PC (2018) Business analytics for systematically investigating sustainable food supply chains. J Clean Prod 203: 968–976. https://doi.org/10.1016/j.jclepro.2018.08.178 doi: 10.1016/j.jclepro.2018.08.178
![]() |
[33] |
Raut RD, Gardas BB, Narwane VS, et al. (2019) Improvement in the food losses in fruits and vegetable supply chain - a perspective of cold third-party logistics approach. Oper Res Perspect 6: 100117. https://doi.org/10.1016/j.orp.2019.100117 doi: 10.1016/j.orp.2019.100117
![]() |
[34] |
Dhaoui O, Nikolaou K, Mattas K, et al. (2020) Consumers' attitude towards alternative distribution channels of fresh fruits and vegetables in Crete. Br Food J 122: 2823–2840. https://doi.org/10.1108/BFJ-05-2019-0342 doi: 10.1108/BFJ-05-2019-0342
![]() |
[35] |
Pérez-Mesa JC, Piedra-Muñoz L, García-Barranco MC, et al. (2019) Response of fresh food suppliers to sustainable supply chain management of large European retailers. Sustain 11: 1–24. https://doi.org/10.3390/su11143885 doi: 10.3390/su11143885
![]() |
[36] |
Manzini R, Accorsi R, Ayyad Z, et al. (2014) Sustainability and quality in the food supply chain. A case study of shipment of edible oils. Br Food J 116: 2069–2090. https://doi.org/10.1108/BFJ-11-2013-0338 doi: 10.1108/BFJ-11-2013-0338
![]() |
[37] |
Shen B, Choi TM, Minner S (2019) A review on supply chain contracting with information considerations: information updating and information asymmetry. Int J Prod Res 57: 4898–4936. https://doi.org/10.1080/00207543.2018.1467062 doi: 10.1080/00207543.2018.1467062
![]() |
[38] |
Xiao Q, Chen L, Xie M, et al. (2020) Optimal contract design in sustainable supply chain: Interactive impacts of fairness concern and overconfidence. J Oper Res Soc 72: 1–20. https://doi.org/10.1080/01605682.2020.1727784 doi: 10.1080/01605682.2020.1727784
![]() |
[39] |
Mangla SK, Luthra S, Rich N, et al. (2018) Enablers to implement sustainable initiatives in agri-food supply chains. Int J Prod Econ 203: 379–393. https://doi.org/10.1016/j.ijpe.2018.07.012 doi: 10.1016/j.ijpe.2018.07.012
![]() |
[40] |
Gokarn S, Kuthambalayan TS (2017) Analysis of challenges inhibiting the reduction of waste in food supply chain. J Clean Prod 168: 595–604. https://doi.org/10.1016/j.jclepro.2017.09.028 doi: 10.1016/j.jclepro.2017.09.028
![]() |
[41] |
Cappellesso G, Thomé KM (2019) Technological innovation in food supply chains: systematic literature review. Br Food J 121: 2413–2428. https://doi.org/10.1108/BFJ-03-2019-0160 doi: 10.1108/BFJ-03-2019-0160
![]() |
[42] |
Azmi FR, Abdullah A, Musa H, et al. (2019) Perception of food manufacturers towards adoption of halal food supply chain in Malaysia: Exploratory factor analysis. J Islam Mark 11: 571–589. https://doi.org/10.1108/JIMA-12-2018-0236 doi: 10.1108/JIMA-12-2018-0236
![]() |
[43] |
Lin PC, Wu LS (2011) How supermarket chains in Taiwan select suppliers of fresh fruit and vegetables via direct purchasing. Serv Ind J 31: 1237–1255. https://doi.org/10.1080/02642060903437568 doi: 10.1080/02642060903437568
![]() |
[44] |
Coppola A, Ianuario S, Romano S, et al. (2020) Corporate social responsibility in agri-food firms: The relationship between CSR actions and firm's performance. AIMS Environ Sci 7: 542–558. https://doi.org/10.3934/environsci.2020034 doi: 10.3934/environsci.2020034
![]() |
[45] |
Kumar G, Goswami M (2019) Sustainable supply chain performance, its practice and impact on barriers to collaboration. Int J Product Perform Manag 68: 1434–1456. https://doi.org/10.1108/IJPPM-12-2018-0425 doi: 10.1108/IJPPM-12-2018-0425
![]() |
[46] |
Zaridis A, Vlachos I, Bourlakis M (2021) SMEs strategy and scale constraints impact on agri-food supply chain collaboration and firm performance. Prod Plan Control 32: 1165–1178. https://doi.org/10.1080/09537287.2020.1796136 doi: 10.1080/09537287.2020.1796136
![]() |
[47] |
Canto NR do, Bossle MB, Vieira LM, et al. (2021) Supply chain collaboration for sustainability: a qualitative investigation of food supply chains in Brazil. Manag Environ Qual An Int J 32: 1210–1232. https://doi.org/10.1108/MEQ-12-2019-0275 doi: 10.1108/MEQ-12-2019-0275
![]() |
[48] |
Kuo TC, Chen GYH, Hsiao YL, et al. (2017) Investigating the Influential Factors of Sustainable Supply Chain Management, Using Two Asian Countries as Examples. Sustain Dev 25: 559–579. https://doi.org/10.1002/sd.1678 doi: 10.1002/sd.1678
![]() |
[49] |
Song W, Ming X, Liu HC (2017) Identifying critical risk factors of sustainable supply chain management: A rough strength-relation analysis method. J Clean Prod 143: 100–115. https://doi.org/10.1016/j.jclepro.2016.12.145 doi: 10.1016/j.jclepro.2016.12.145
![]() |
[50] |
Prasad DS, Pradhan RP, Gaurav K, et al. (2018) Analysing the critical success factors for implementation of sustainable supply chain management: An Indian case study. Decision 45: 3–25. https://doi.org/10.1007/s40622-017-0171-7 doi: 10.1007/s40622-017-0171-7
![]() |
1. | Muhammad Zubair Mehboob, Arslan Hamid, Jeevotham Senthil Kumar, Xia Lei, Comprehensive characterization of pathogenic missense CTRP6 variants and their association with cancer, 2025, 25, 1471-2407, 10.1186/s12885-025-13685-0 |