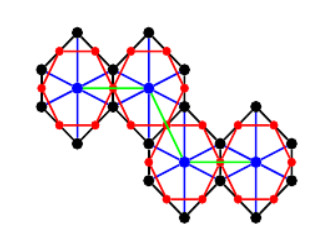
This study mainly used The Cancer Genome Atlas (TCGA) RNA sequencing dataset to screen prognostic snoRNAs of acute myeloid leukemia (AML), and used for the construction of prognostic snoRNAs signature for AML. A total of 130 AML patients with RNA sequencing dataset were used for prognostic snoRNAs screenning. SnoRNAs co-expressed genes and differentially expressed genes (DEGs) were used for functional annotation, as well as gene set enrichment analysis (GSEA). Connectivity Map (CMap) also used for potential targeted drugs screening. Through genome-wide screening, we identified 30 snoRNAs that were significantly associated with the prognosis of AML. Then we used the step function to screen a prognostic signature composed of 14 snoRNAs (SNORD72, SNORD38, U3, SNORA73B, SNORD79, SNORA73, SNORD12B, SNORA74, SNORD116-12, SNORA65, SNORA14, snoU13, SNORA75, SNORA31), which can significantly divide AML patients into high- and low-risk groups. Through GSEA, snoRNAs co-expressed genes and DEGs functional enrichment analysis, we screened a large number of potential functional mechanisms of this prognostic signature in AML, such as phosphatidylinositol 3-kinase-Akt, Wnt, epithelial to mesenchymal transition, T cell receptors, NF-kappa B, mTOR and other classic cancer-related signaling pathways. In the subsequent targeted drug screening using CMap, we also identified six drugs that can be used for AML targeted therapy, they were alimemazine, MG-262, fluoxetine, quipazine, naltrexone and oxybenzone. In conclusion, our current study was constructed an AML prognostic signature based on the 14 prognostic snoRNAs, which may serve as a novel prognostic biomarker for AML.
Citation: Rui Huang, Xiwen Liao, Qiaochuan Li. Integrative genomic analysis of a novel small nucleolar RNAs prognostic signature in patients with acute myelocytic leukemia[J]. Mathematical Biosciences and Engineering, 2022, 19(3): 2424-2452. doi: 10.3934/mbe.2022112
[1] | Rajish Kumar P, Sunil Jacob John . On redundancy, separation and connectedness in multiset topological spaces. AIMS Mathematics, 2020, 5(3): 2484-2499. doi: 10.3934/math.2020164 |
[2] | Seçil Çeken, Cem Yüksel . Generalizations of strongly hollow ideals and a corresponding topology. AIMS Mathematics, 2021, 6(12): 12986-13003. doi: 10.3934/math.2021751 |
[3] | Saad Ihsan Butt, Ahmet Ocak Akdemir, Muhammad Nadeem, Nabil Mlaiki, İşcan İmdat, Thabet Abdeljawad . (m,n)-Harmonically polynomial convex functions and some Hadamard type inequalities on the co-ordinates. AIMS Mathematics, 2021, 6(5): 4677-4690. doi: 10.3934/math.2021275 |
[4] | Qian Liu, Jianrui Xie, Ximeng Liu, Jian Zou . Further results on permutation polynomials and complete permutation polynomials over finite fields. AIMS Mathematics, 2021, 6(12): 13503-13514. doi: 10.3934/math.2021783 |
[5] | Usman Babar, Haidar Ali, Shahid Hussain Arshad, Umber Sheikh . Multiplicative topological properties of graphs derived from honeycomb structure. AIMS Mathematics, 2020, 5(2): 1562-1587. doi: 10.3934/math.2020107 |
[6] | Ninghe Yang . Exact wave patterns and chaotic dynamical behaviors of the extended (3+1)-dimensional NLSE. AIMS Mathematics, 2024, 9(11): 31274-31294. doi: 10.3934/math.20241508 |
[7] | Ali N. A. Koam, Ali Ahmad, Yasir Ahmad . Computation of reverse degree-based topological indices of hex-derived networks. AIMS Mathematics, 2021, 6(10): 11330-11345. doi: 10.3934/math.2021658 |
[8] | R. Aguilar-Sánchez, J. A. Mendez-Bermudez, José M. Rodríguez, José M. Sigarreta . Multiplicative topological indices: Analytical properties and application to random networks. AIMS Mathematics, 2024, 9(2): 3646-3670. doi: 10.3934/math.2024179 |
[9] | Muhammad Riaz, Khadija Akmal, Yahya Almalki, S. A. Alblowi . Cubic m-polar fuzzy topology with multi-criteria group decision-making. AIMS Mathematics, 2022, 7(7): 13019-13052. doi: 10.3934/math.2022721 |
[10] | Maryam Salem Alatawi, Ali Ahmad, Ali N. A. Koam, Sadia Husain, Muhammad Azeem . Computing vertex resolvability of benzenoid tripod structure. AIMS Mathematics, 2022, 7(4): 6971-6983. doi: 10.3934/math.2022387 |
This study mainly used The Cancer Genome Atlas (TCGA) RNA sequencing dataset to screen prognostic snoRNAs of acute myeloid leukemia (AML), and used for the construction of prognostic snoRNAs signature for AML. A total of 130 AML patients with RNA sequencing dataset were used for prognostic snoRNAs screenning. SnoRNAs co-expressed genes and differentially expressed genes (DEGs) were used for functional annotation, as well as gene set enrichment analysis (GSEA). Connectivity Map (CMap) also used for potential targeted drugs screening. Through genome-wide screening, we identified 30 snoRNAs that were significantly associated with the prognosis of AML. Then we used the step function to screen a prognostic signature composed of 14 snoRNAs (SNORD72, SNORD38, U3, SNORA73B, SNORD79, SNORA73, SNORD12B, SNORA74, SNORD116-12, SNORA65, SNORA14, snoU13, SNORA75, SNORA31), which can significantly divide AML patients into high- and low-risk groups. Through GSEA, snoRNAs co-expressed genes and DEGs functional enrichment analysis, we screened a large number of potential functional mechanisms of this prognostic signature in AML, such as phosphatidylinositol 3-kinase-Akt, Wnt, epithelial to mesenchymal transition, T cell receptors, NF-kappa B, mTOR and other classic cancer-related signaling pathways. In the subsequent targeted drug screening using CMap, we also identified six drugs that can be used for AML targeted therapy, they were alimemazine, MG-262, fluoxetine, quipazine, naltrexone and oxybenzone. In conclusion, our current study was constructed an AML prognostic signature based on the 14 prognostic snoRNAs, which may serve as a novel prognostic biomarker for AML.
In today's world mathematics is necessary in all fields. It is an essential instrument for comprehension around us. It covers all the facts of life. Mathematics is the branch of science that deals with the reasoning of figures, numbers and order. In our daily life, we use mathematics in our routine work in various forms. There are many branches of mathematics like algebra, geometry, arithmetic, trigonometry, analysis and many other theories. Graph theory is the study of mathematical objects known as graph, which consist of vertices connected by edges. It is the mathematical theory which deals with the properties and applications of graphs. When we apply graph theory on chemistry then it is called chemical graph theory. In mathematical models graph theory is used to get a deep understanding of the physical properties of these chemical compounds. Some physical properties such as boiling point, melting point, density are associated to geometrical structure of the compound. Now a days, several ways are used in mathematical chemistry to understand chemical structure which are existing behind the chemical concepts, to create and inquire novel mathematical representation. In complete history of chemistry certain scientist, usually contemplates connections between mathematics with chemistry and the possibility of using mathematics to analyze and predict new chemical concepts. Mufti defined sanskruti and harmonic indices of certain graph structure [25]. Babujee calculated topological indices and new graph structures in 2012 [5]. Farahani worked on a new version of zagreb index of circumcoronene series of benzenoid in 2013 [7]. Hayat defined some degree based topological indices of certain nanotubes [8]. Imran worked on topological indices of certain interconnection networks in 2014 [14]. In 2016, Siddiqui computed zagreb indices and zagreb polynomials of some nanostars dendrimers [27]. Saleem computed retractions and homomorphisms on some operations of graphs [28]. Iqbal calculated eccentricity based topological indices of some benzenoid structures [12]. Yang examined two-point resistances and random walks on stellated regular graphs [29]. Islam defined M-polynomial and entropy of paraline graph of Napthalene in 2019 [11]. Iqbal worked on topological indices of subdivided and line graph of subdivided friendship graph [13]. In 2020, Afzal examined M-polynomial and topological indices of zigzag edge coronoid fused by starphene [1]. Archdeacon defined the medial graph and voltage-current duality [2]. Munir computed M-polynomial and degree-based topological indices of polyhex nanotubes [22]. Iqbal calculated ve topological indices of tickysim spinnaker model [15]. Azhar examined a note on valency dependence invariants of L(G(K)) Graph [4]. Jamil defined the first general zagreb eccentricity index [18]. Iqbal defined ABC4 and GA5 index of subdivided and line graph of subdivided dutch windmill graph [16]. Maji worked on the first entire zagreb index of various corona products and their bounds [23]. Imran describe computation of topological indices of NEPS of graphs [17]. Hayat computed topological indices for networks derived by applying graph operations from honeycomb structures [9], based on this idea we have computed the topological indices for transformed structures. Next we have few definitions [3,9]:
Definition 1:
Let K be a simple connected graph its M-polynomial is defined as;
M(K;x,y)=∑S≤a≤b≤Tmab(K)xayb, (i) |
where: S = Min{dβ|β∈V(K)}, T = Max{dβ|β∈V(K)}, and mab(K) is the number of edges γβ∈E(K) such that {dγ, dβ} = {a,b}.
Definition 2:
When we place a new vertex in each face of a planar graph G, and attach that vertex with all the vertices of the respective face of G, we get the stellation of G and denote it as St(G).
Definition 3:
We introduce a node in each edge of the graph and join the nodes if their corresponding edges are adjacent and is denoted by Md(G).
Definition 4:
We introduce a node in each bounded faces of the graph and join the nodes by an edge if the faces share an edge in graph it is called bounded dual. It is represented as Bdu(G).
T. Réti introduced First and Second Zagreb indices are [26],
M1(K)=∑γβ∈E(K)(dγ+dβ), | (1.1) |
M2(K)=∑γβ∈E(K)(dγdβ). | (1.2) |
Second modified Zagreb index is defined as [10],
mM2(k)=∑γβ∈E(K)(1dγdβ). | (1.3) |
Symmetric division and reciprocal general Randic index is [19],
SDD(K)=∑γβ∈E(K) {min(dγ,dβ)max(dγ,dβ)+max(dγ,dβ)min(dγ,dβ)}, | (1.4) |
RRα(K)=∑γβ∈E(K)1(dγdβ)α. | (1.5) |
Whereas, the General Randic index defined as [24],
Rα(K)=∑γβ∈E(K)(dγdβ)α, | (1.6) |
where α is an arbitrary real number.
In 1998, the Atomic Bond Connectivity Index is defined as [6],
ABC(K)=∑γβ∈E(K)√dγ+dβ−2dγdβ. | (1.7) |
Geometric-Arithmetic index is [30],
GA(K)=∑γβ∈E(K)2√dγdβdγ+dβ. | (1.8) |
In 2015, the General Version of Harmonic Index is defined [31],
Hk(K)=∑γβ∈E(K)(2dγ+dβ)k. | (1.9) |
First and Second Gourava Indices were introduced by Kalli which is defined as, respectively [20],
GO1(K)=∑γβ∈E(K)[(dγ+dβ)+(dγdβ)], | (1.10) |
GO2(K)=∑γβ∈E(K)[(dγ+dβ)(dγdβ)]. | (1.11) |
Kalli introduced the First and Second Hyper-Gourava Indices which is defined as, respectively [21],
HGO1(K)=∑γβ∈E(K)[(dγ+dβ)+(dγdβ)]2, | (1.12) |
HGO2(K)=∑γβ∈E(K)[(dγ+dβ)(dγdβ)]2. | (1.13) |
At first, we obtain transformed pattern of molecular structures zigzag and triangular benzenoid named as T1 and T2. Next we define M-polynomial and topological indices of these networks by applying the stellation, medial and bounded dual. Further we define edge partition depending upon degree based vertices of T1 and T2 and then calculate the indices.
Now we will construct the stellation, medial and bounded dual operations on simple and undirected zigzag benzenoid structure. We get a new transformed network (say) T1, shown in Figure 1 for n = 2. After this we will compute M-polynomial and degree based topological indices of our newly obtained network. Where, blue is stellation, red is medial, green is bounded dual operations applied on T1.
Following Table 3 shows the types of edges and their count for network T1; n≥2. Now, we will calculate the M-polynomial and vertex degree based topological indices namely first Zagreb index, second Zagreb index, second modified index, symmetric division index, general Randic index, reciprocal general Randic index, atomic bond connectivity index, geometric arithmetic index, general version of harmonic index, first and second gourava indices, first and second hyper gourava indices.
Let T1, be the transformed network then its M-polynomial is
M(T1;x,y)=(8n+8)x3y4+8x3y7+(4n−4)x3y8+(4n+4)x4y4+(8n−4)x4y5+(8n−4)x4y6+(4n−2)x5y6+4x5y7+(8n−8)x5y8+2x7y8+(2n−3)x8y8. |
Proof:
Using definition of M-polynomial and information from Table 3, we have
M(T1;x,y)=∑a≤bmabxayb=∑3≤4m34x3y4+∑3≤7m37x3y7+∑3≤8m38x3y8+∑4≤4m44x4y4+∑4≤5m45x4y5+∑4≤6m46x4y6+∑5≤6m56x5y6+∑5≤7m57x5y7+∑5≤8m58x5y8+∑7≤8m78x7y8+∑8≤8m88x8y8=|E3,4|x3y4+|E3,7|x3y7+|E3,8|x3y8+|E4,4|x4y4+|E4,5|x4y5+|E4,6|x4y6+|E5,6|x5y6+|E5,7|x5y7+|E5,8|x5y8+|E7,8|x7y8+|E8,8|x8y8=(8n+8)x3y4+8x3y7+(4n−4)x3y8+(4n+4)x4y4+(8n−4)x4y5+(8n−4)x4y6+(4n−2)x5y6+4x5y7+(8n−8)x5y8+2x7y8+(2n−3)x8y8. |
Let T1, be the transformed network and
M(T1;x,y)=(8n+8)x3y4+8x3y7+(4n−4)x3y8+(4n+4)x4y4+(8n−4)x4y5+(8n−4)x4y6+(4n−2)x5y6+4x5y7+(8n−8)x5y8+2x7y8+(2n−3)x8y8, |
be its M-polynomial. Then, the first Zagreb index M1(T1), the second Zagreb index M2(T1), the second modified Zagreb index mM2(T1), the general Randic index Rα(T1), where α ϵ N, reciprocal general Randic index RRα(T1), where α ϵ N, and the symmetric division degree index SDD(T1) obtained from M-polynomial are as follows:
M1(T1)=464n−48,M2(T1)=1176n−264,mM2(T1)=1112(n+1)+1130(n−1)+1330(2n−1)+164(2n−3)+223420,Rα(T1)=12α(8n+8)+21α(8)+24α(4n−4)+16α(4n+4)+20α(8n−4)+24α(8n−4)+30α(4n−2)+35α(4)+40α(8n−8)+56α(2)+64α(2n−3),RRα(T1)=812α(n+1)+821α+424α(n−1)+442α(n+1)+420α(2n−1)+424α(2n−1)+230α(2n−1)+435α+840α(n−1)+256α+164α(2n−3),SDD(T1)=743(n+1)+89930(n−1)+31415(2n−1)+2(2n−3)+14527420. |
Proof: Let f(x,y) = M(T1;x,y) be the M-polynomial of the transformed network T1. Then
M(T1;x,y)=(8n+8)x3y4+8x3y7+(4n−4)x3y8+(4n+4)x4y4+(8n−4)x4y5+(8n−4)x4y6+(4n−2)x5y6+4x5y7+(8n−8)x5y8+2x7y8+(2n−3)x8y8. |
Now, the required partial derivatives and integrals are obtained as:
By using the information given in Table 3 and formulas for Table 1;
Dxf(x,y)=3(8n+8)x3y4+24x3y7+3(4n−4)x3y8+4(4n+4)x4y4+4(8n−4)x4y5+4(8n−4)x4y6+5(4n−2)x5y6+20x5y7+5(8n−8)x5y8+14x7y8+8(2n−3)x8y8,Dyf(x,y)=4(8n+8)x3y4+56x3y7+8(4n−4)x3y8+4(4n+4)x4y4+5(8n−4)x4y5+6(8n−4)x4y6+6(4n−2)x5y6+28x5y7+8(8n−8)x5y8+16x7y8+8(2n−3)x8y8,DxDyf(x,y)=12(8n+8)x3y4+168x3y7+24(4n−4)x3y8+16(4n+4)x4y4+20(8n−4)x4y5+24(8n−4)x4y6+30(4n−2)x5y6+140x5y7+40(8n−8)x5y8+112x7y8+64(2n−3)x8y8,SxSyf(x,y)=23(n+1)x3y4+821x3y7+16(n−1)x3y8+14(n+1)x4y4+15(2n−1)x4y5+16(2n−1)x4y6+115(2n−1)x5y6+435x5y7+15(n−1)x5y8+128x7y8+164(2n−3)x8y8,DαxDαyf(x,y)=12α(8n+8)x3y4+21α(8)x3y7+24α(4n−4)x3y8+42α(4n+4)x4y4+20α(8n−4)x4y5+24α(8n−4)x4y6+30α(4n−2)x5y6+35α(4)x5y7+40α(8n−8)x5y8+56α(2)x7y8+46α(2n−3)x8y8,SαxSαyf(x,y)=812α(n+1)x3y4+821αx3y7+424α(n−1)x3y8+442α(n+1)x4y4+420α(2n−1)x4y5+424α(2n−1)x4y6+230α(2n−1)x5y6+435αx5y7+840α(n−1)x5y8+256αx7y8+164α(2n−3)x8y8,SyDxf(x,y)=6(n+1)x3y4+247x3y7+32(n−1)x3y8+4(n+1)x4y4+165(2n−1)x4y5+83(2n−1)x4y6+53(2n−1)x5y6+207x5y7+5(n−1)x5y8+74x7y8+(2n−3)x8y8,SxDyf(x,y)=323(n+1)x3y4+563x3y7+323(n−1)x3y8+4(n+1)x4y4+5(2n−1)x4y5+6(2n−1)x4y6+125(2n−1)x5y6+285x5y7+645(n−1)x5y8+167x7y8+(2n−3)x8y8. |
Topological Index | Derivation from M (K; x, y) | |
First Zagreb | (Dx+Dy)(M(K;x,y))|x=1=y | (ii) |
Second Zagreb | (DxDy)(M(K;x,y))|x=1=y | (iii) |
Second Modified Zagreb | (SxSy)(M(K;x,y))|x=1=y | (iv) |
general Randic | (DαxDαy)(M(K;x,y))|x=1=y | (v) |
Reciprocal general Randic | (SαxSαy)(M(K;x,y))|x=1=y | (vi) |
Symmetric Division Index | (SyDx)+(SxDy)(M(K;x,y))|x=1=y | (vii) |
Graph | Planar/ Non−Planar |
St(G1,G2) | Planar |
Md(G1,G2) | Planar |
Bdu(G1,G2) | Non−Planar |
T1 | Non−Planar |
T2 | Non−Planar |
Consequently,
M1(T1)=(Dx+Dy)f(x,y)|x=1=y=464n−48,M2(T1)=DxDyf(x,y)|x=1=y=1176n−264,mM2(T1)=SxSyf(x,y)|x=1=y=1112(n+1)+1130(n−1)+1330(2n−1)+164(2n−3)+223420,Rα(T1)=DαxDαyf(x,y)|x=1=y=12α(8n+8)+(21)α8+24α(4n−4)+16α(4n+4)+20α(8n−4)+24α(8n−4)+30α(4n−2)+(35)α4+40α(8n−8)+(56)α2+64α(2n−3),RRα(T1)=SαxSαyf(x,y)|x=1=y=812α(n+1)+821α+424α(n−1)+442α(n+1)+420α(2n−1)+424α(2n−1)+230α(2n−1)+435α+840α(n−1)+256α+164α(2n−3),SDD(T1)=(SyDx+SxDy)(x,y)|x=1=y=743(n+1)+89930(n−1)+31415(2n−1)+2(2n−3)+14527420. |
For T1, the Atomic Bond Connectivity, Geometric Arithematic Index and General Harmonic Index are as follows, respectively.
1) ABC(T1)=2n√6+(n+1)4√153+(14n+11)√1456+(n−1)2√1105+(2n−1)(2√75+4√3+√65)+16√4221+√18214,2) GA(T1)=(n+1)(32√37+4)+(n−1)32√1013+(32n−21)8√655+(2n−1)(16√59+4√3011)+(2n−3)+8√215+2√353+8√1415,3) Hk(T1)=(8n+8)(27)k+(8n+4)(15)k+(4n−4)(211)k+(4n+4)(14)k+(8n−4)(29)k+(4n−2)(211)k+4(16)k+(8n−8)(213)k+2(215)k+(2n−3)(18)k. |
Proof:
1). According to Eq (1.7)
ABC(T1)=∑γβ∈E(K)√dγ+dβ−2dγdβ. |
By using the information given in Table 3.
=(8n+8)(√3+4−23×4)+8(√3+7−23×7)+(4n−4)(√3+8−23×8)+(4n+4)(√4+4−24×4)+(8n−4)(√4+5−24×5)+(8n−4)(√4+6−24×6)+(4n−2)(√5+6−25×6)+4(√5+7−25×7)+(8n−8)(√5+8−25×8)+2(√7+8−27×8)+(2n−3)(√8+8−28×8)=2n√6+(n+1)4√153+(14n+11)√1456+(n−1)2√1105+(2n−1)(2√75+4√3+√65)+16√4221+√18214. |
Types of edges | Count of edges |
(3,4) | 8n+8 |
(3,7) | 8 |
(3,8) | 4n−4 |
(4,4) | 4n+4 |
(4,5) | 8n−4 |
(4,6) | 8n−4 |
(5,6) | 4n−2 |
(5,7) | 4 |
(5,8) | 8n−8 |
(7,8) | 2 |
(8,8) | 2n−3 |
2). According to Eq (1.8)
GA(T1)=∑γβ∈E(K)2√dγdβdγ+dβ. |
By using the information given in Table 3.
=(8n+8)(2√3×43+4)+8(2√3×73+7)+(4n−4)(2√3×83+8)+(4n+4)(2√4×44+4)+(8n−4)(2√4×54+5)+(8n−4)(2√4×64+6)+(4n−2)(2√5×65+6)+4(2√5×75+7)+(8n−8)(2√5×85+8)+2(2√7×87+8)+(2n−3)(2√8×88+8)=(n+1)(32√37+4)+(n−1)32√1013+(32n−21)8√655+(2n−1)(16√59+4√3011)+(2n−3)+8√215+2√353+8√1415. |
3). According to Eq (1.9)
Hk(T1)=∑γβ∈E(K)(2dγ+dβ)k. |
By using the information given in Table 3.
=(8n+8)(23+4)k+8(23+7)k+(4n−4)(23+8)k+(4n+4)(24+4)k+(8n−4)(24+5)k+(8n−4)(24+6)k+(4n−2)(25+6)k+4(25+7)k+(8n−8)(25+8)k+2(27+8)k+(2n−3)(28+8)k=(8n+8)(27)k+(8n+4)(15)k+(4n−4)(211)k+(4n+4)(14)k+(8n−4)(29)k+(4n−2)(211)k+4(16)k+(8n−8)(213)k+2(215)k+(2n−3)(18)k. |
For T1, the First, Second Gourava Indices and the First, Second Hyper Gourava Indices are as follows, respectively.
1) GO1(T1)=1640n−312,2) GO2(T1)=13128n−4404,3) HGO1(T1)=68064n−26124,4) HGO2(T1)=5816720n−3573928. |
Proof:
1). According to Eq (1.10)
GO1(T1)=∑γβ∈E(K)[(dγ+dβ)+(dγdβ)]. |
By using the information given in Table 3.
=(8n+8)[(3+4)+(3×4)]+8[(3+7)+(3×7)]+(4n−4)[(3+8)+(3×8)]+(4n+4)[(4+4)+(4×4)]+(8n−4)[(4+5)+(4×5)]+(8n−4)[(4+6)+(4×6)]+(4n−2)[(5+6)+(5×6)]+4[(5+7)+(5×7)]+(8n−8)[(5+8)+(5×8)]+2[(7+8)+(7×8)]+(2n−3)[(8+8)+(8×8)]=1640n−312. |
2). According to Eq (1.11)
GO2(T1)=∑γβ∈E(K)[(dγ+dβ)(dγdβ)]. |
By using the information given in Table 3.
=(8n+8)[(3+4)(3×4)]+8[(3+7)(3×7)]+(4n−4)[(3+8)(3×8)]+(4n+4)[(4+4)(4×4)]+(8n−4)[(4+5)(4×5)]+(8n−4)[(4+6)(4×6)]+(4n−2)[(5+6)(5×6)]+4[(5+7)(5×7)]+(8n−8)[(5+8)(5×8)]+2[(7+8)(7×8)]+(2n−3)[(8+8)(8×8)]=13128n−4404. |
3). According to Eq (1.12)
HGO1(T1)=∑γβ∈E(K)[(dγ+dβ)+(dγdβ)]2. |
By using the information given in Table 3.
=(8n+8)[(3+4)+(3×4)]2+8[(3+7)+(3×7)]2+(4n−4)[(3+8)+(3×8)]2+(4n+4)[(4+4)+(4×4)]2+(8n−4)[(4+5)+(4×5)]2+(8n−4)[(4+6)+(4×6)]2+(4n−2)[(5+6)+(5×6)]2+4[(5+7)+(5×7)]2+(8n−8)[(5+8)+(5×8)]2+2[(7+8)+(7×8)]2+(2n−3)[(8+8)+(8×8)]2=68064n−26124. |
4). According to Eq (1.13)
HGO2(T1)=∑γβ∈E(K)[(dγ+dβ)(dγdβ)]2. |
By using the information given in Table 3.
=(8n+8)[(3+4)(3×4)]2+8[(3+7)(3×7)]2+(4n−4)[(3+8)(3×8)]2+(4n+4)[(4+4)(4×4)]2+(8n−4)[(4+5)(4×5)]2+(8n−4)[(4+6)(4×6)]2+(4n−2)[(5+6)(5×6)]2+4[(5+7)(5×7)]2+(8n−8)[(5+8)(5×8)]2+2[(7+8)(7×8)]2+(2n−3)[(8+8)(8×8)]2=5816720n−3573928. |
Now we will construct the stellation, medial and bounded dual operations on simple and undirected triangular benzenoid structure. We get a new transformed network (say) T2, shown in Figure 3 for n = 5. After this we will compute M-polynomial and degree based topological indices of our newly obtained network. Where, blue is stellation, red is medial, green is bounded dual operations applied on T2.
Following Table 4 shows the types of edges and their count for network T2; n≥5. Now, we will calculate the different M-polynomial and vertex degree based topological indices namely first Zagreb index, second Zagreb index, second modified index, symmetric division index, general Randic index, reciprocal general Randic index, atomic bond connectivity index, geometric arithmetic index, general version of harmonic index, first and second gourava indices, first and second hyper gourava indices.
Types of edges | Count of edges |
(3,4) | 6n+6 |
(3,8) | 9 |
(3,10) | 3n−6 |
(4,4) | 3n+3 |
(4,5) | 6n−6 |
(4,6) | 6n−6 |
(5,6) | 3n−3 |
(5,8) | 6 |
(5,10) | 6n−12 |
(6,6) | 6(n−1)2 |
(6,8) | 3 |
(6,10) | 9n−18 |
(6,12) | 6[(n−(n−1))+(n−(n−2))+... |
+(n−4)+(n−3)] | |
(8,10) | 6 |
(10,10) | 3n−6 |
(10,12) | 6n−18 |
(12,12) | 3[(n−4)+(n−5)+... |
+(n−(n−1))] |
Let T2, be the transformed network then its M-polynomial is
M(T2;x,y)=(6n+6)x3y4+9x3y8+(3n−6)x3y10+(3n+3)x4y4+(6n−6)x4y5+(6n−6)x4y6+(3n−3)x5y6+6x5y8+(6n−12)x5y10+6(n−1)2x6y6+3x6y8+(9n−18)x6y10+6[(n−(n−1))+(n−(n−2))+...+(n−4)+(n−3)]x6y12+6x8y10+(3n−6)x10y10+(6n−18)x10y12+3[(n−4)+(n−5)+...+(n−(n−1))]x12y12. |
Proof: Using definition of M-polynomial and information from Table 4, we have
M(T2;x,y)=∑a≤bmabxayb=∑3≤4m34x3y4+∑3≤8m38x3y8+∑3≤10m3 10x3y10+∑4≤4m44x4y4+∑4≤5m45x4y5+∑4≤6m46x4y6+∑5≤6m56x5y6+∑5≤8m58x5y8+∑5≤10m5 10x5y10+∑6≤6m66x6y6+∑6≤8m68x6y8+∑6≤10m6 10x6y10+∑6≤12m6 12x6y12+∑8≤10m8 10x8y10+∑10≤10m10 10x10y10+∑10≤12m10 12x10y12+∑12≤12m12 12x12y12=|E3,4|x3y4+|E3,8|x3y8+|E3,10|x3y10+|E4,4|x4y4+|E4,5|x4y5+|E4,6|x4y6+|E5,6|x5y6+|E5,8|x5y8+|E5,10|x5y10+|E6,6|x6y6+|E6,8|x6y8+|E6,10|x6y10+|E6,12|x6y12+|E8,10|x8y10+|E10,10|x10y10+|E10,12|x10y12+|E12,12|x12y12=(6n+6)x3y4+9x3y8+(3n−6)x3y10+(3n+3)x4y4+(6n−6)x4y5+(6n−6)x4y6+(3n−3)x5y6+6x5y8+(6n−12)x5y10+6(n−1)2x6y6+3x6y8+(9n−18)x6y10+6[(n−(n−1))+(n−(n−2))+...+(n−4)+(n−3)]x6y12+6x8y10+(3n−6)x10y10+(6n−18)x10y12+3[(n−4)+(n−5)+...+(n−(n−1))]x12y12. |
Let T2, be the transformed network and
M(T2;x,y)=(6n+6)x3y4+9x3y8+(3n−6)x3y10+(3n+3)x4y4+(6n−6)x4y5+(6n−6)x4y6+(3n−3)x5y6+6x5y8+(6n−12)x5y10+6(n−1)2x6y6+3x6y8+(9n−18)x6y10+6[(n−(n−1))+(n−(n−2))+...+(n−4)+(n−3)]x6y12+6x8y10+(3n−6)x10y10+(6n−18)x10y12+3[(n−4)+(n−5)+...+(n−(n−1))]x12y12, |
be its M-polynomial. Then, the first Zagreb index M1(T2), the second Zagreb index M2(T2), the second modified Zagreb mM2(T2), the general Randic index Rα(T2), where α ϵ N, reciprocal general Randic index RRα(T2), where α ϵ N, and the symmetric division degree index SDD(T2) obtained from M-polynomial are as follows:
M1(T2)=678n−816+72(n−1)2+108[(n−(n−1))+(n−(n−2))+...+(n−4)+(n−3)]+72[(n−4)+(n−5)+...+(n−(n−1))],M2(T2)=2424n−3774+216(n−1)2+432[(n−(n−1))+(n−(n−2))+...+(n−4)+(n−3)]+432[(n−4)+(n−5)+...+(n−(n−1))],mM2(T2)=1116(n+1)+25(n−2)+1320(n−1)+120(n−3)+5380+16(n−1)2+112[(n−(n−1))+(n−(n−2))+...+(n−4)+(n−3)]+148[(n−4)+(n−5)+...+(n−(n−1))],Rα(T2)=12α(6n+6)+24α(9)+30α(3n−6)+42α(3n+3)+20α(6n−6)+24α(6n−6)+30α(3n−3)+40α(6)+50α(6n−12)+6(n−1)2(36)α+48α(3)+60α(9n−18)+72α[(n−(n−1))+(n−(n−2))+...+(n−4)+(n−3)]6+80α(6)+100α(3n−6)+120α(6n−18)+144α[(n−4)+(n−5)+...+(n−(n−1))]3,RRα(T2)=612α(n+1)+924α+330α(n−2)+342α(n+1)+620α(n−1)+624α(n−1)+330α(n−1)+640α+650α(n−2)+636α(n−1)2+348α+960α(n−2)+680α+3100α(n−2)+172α[(n−(n−1))+(n−(n−2))+...+(n−4)+(n−3)]6+6120α(n−3)+1144α[(n−4)+(n−5)+...+(n−(n−1))]3,SDD(T2)=372(n+1)+1575(n−1)+52310(n−2)+615(n−3)+237140+12(n−1)2+15[(n−(n−1))+(n−(n−2))+...+(n−4)+(n−3)]+6[(n−4)+(n−5)+...+(n−(n−1))]. |
Proof: Let f(x,y) = M(T2;x,y) be the M-polynomial of the transformed network T2. Then
M(T2;x,y)=(6n+6)x3y4+9x3y8+(3n−6)x3y10+(3n+3)x4y4+(6n−6)x4y5+(6n−6)x4y6+(3n−3)x5y6+6x5y8+(6n−12)x5y10+6(n−1)2x6y6+3x6y8+(9n−18)x6y10+6[(n−(n−1))+(n−(n−2))+...+(n−4)+(n−3)]x6y12+6x8y10+(3n−6)x10y10+(6n−18)x10y12+3[(n−4)+(n−5)+...+(n−(n−1))]x12y12. |
Now, the required partial derivatives and integrals are obtained.
By using the information given in Table 4 and formulas for Table 1:
Dxf(x,y)=3(6n+6)x3y4+27x3y8+3(3n−6)x3y10+4(3n+3)x4y4+4(6n−6)x4y5+4(6n−6)x4y6+5(3n−3)x5y6+30x5y8+5(6n−12)x5y10+36(n−1)2x6y6+18x6y8+6(9n−18)x6y10+36[(n−(n−1))+(n−(n−2))+...+(n−4)+(n−3)]x6y12+48x8y10+10(3n−6)x10y10+10(6n−18)x10y12+36[(n−4)+(n−5)+...+(n−(n−1))]x12y12,Dyf(x,y)=4(6n+6)x3y4+72x3y8+10(3n−6)x3y10+4(3n+3)x4y4+5(6n−6)x4y5+6(6n−6)x4y6+6(3n−3)x5y6+48x5y8+10(6n−12)x5y10+36(n−1)2x6y6+24x6y8+10(9n−18)x6y10+72[(n−(n−1))+(n−(n−2))+...+(n−4)+(n−3)]x6y12+60x8y10+10(3n−6)x10y10+12(6n−18)x10y12+36[(n−4)+(n−5)+...+(n−(n−1))]x12y12,DxDyf(x,y)=12(6n+6)x3y4+216x3y8+30(3n−6)x3y10+16(3n+3)x4y4+20(6n−6)x4y5+24(6n−6)x4y6+30(3n−3)x5y6+240x5y8+50(6n−12)x5y10+216(n−1)2x6y6+144x6y8+60(9n−18)x6y10+432[(n−(n−1))+(n−(n−2))+...+(n−4)+(n−3)]x6y12+480x8y10+100(3n−6)x10y10+120(6n−18)x10y12+432[(n−4)+(n−5)+...+(n−(n−1))]x12y12,SxSyf(x,y)=12(n+1)x3y4+38x3y8+110(n−2)x3y10+316(n+1)x4y4+310(n−1)x4y5+14(n−1)x4y6+110(n−1)x5y6+320x5y8+325(n−2)x5y10+16(n−1)2x6y6+116x6y8+320(n−2)x6y10+112[(n−(n−1))+(n−(n−2))+...+(n−4)+(n−3)]x6y12+340x8y10+3100(n−2)x10y10+120(n−3)x10y12+148[(n−4)+(n−5)+...+(n−(n−1))]x12y12,DαxDαyf(x,y)=12α(6n+6)x3y4+24α(9)x3y8+30α(3n−6)x3y10+42α(3n+3)x4y4+20α(6n−6)x4y5+24α(6n−6)x4y6+30α(3n−3)x5y6+40α(6)x5y8+50α(6n−12)x5y10+(36)α(n−1)26x6y6+48α(3)x6y8+60α(9n−18)x6y10+72α[(n−(n−1))+(n−(n−2))+...+(n−4)+(n−3)]6x6y12+80α(6)x8y10+100α(3n−6)x10y10+120α(6n−18)x10y12+144α[(n−4)+(n−5)+...+(n−(n−1))]3x12y12,SαxSαyf(x,y)=612α(n+1)x3y4+924αx3y8+330α(n−2)x3y10+342α(n+1)x4y4+620α(n−1)x4y5+624α(n−1)x4y6+330α(n−1(n−1)x5y6+640αx5y8+650α(n−2)x5y10+636α(n−1)2x6y6+348αx6y8+960α(n−2)x6y10+172α[(n−(n−1))+(n−(n−2))+...+(n−4)+(n−3)]6x6y12+680αx8y10+3100α(n−2)x10y10+6120α(n−3)x10y12+1144α[(n−4)+(n−5)+...+(n−(n−1))]3x12y12,SyDxf(x,y)=92(n+1)x3y4+278x3y8+910(n−2)x3y10+3(n+1)x4y4+245(n−1)x4y5+4(n−1)x4y6+52(n−1)x5y6+154x5y8+3(n−2)x5y10+6(n−1)2x6y6+94x6y8+275(n−2)x6y10+3[(n−(n−1))+(n−(n−2))+...+(n−4)+(n−3)]x6y12+245x8y10+3(n−2)x10y10+5(n−3)x10y12+3[(n−4)+(n−5)+...+(n−(n−1))]x12y12,SxDyf(x,y)=8(n+1)x3y4+24x3y8+10(n−2)x3y10+3(n+1)x4y4+152(n−1)x4y5+9(n−1)x4y6+185(n−1)x5y6+485x5y8+12(n−2)x5y10+6(n−1)2x6y6+4x6y8+15(n−2)x6y10+12[(n−(n−1))+(n−(n−2))+...+(n−4)+(n−3)]x6y12+152x8y10+3(n−2)x10y10+365(n−3)x10y12+3[(n−4)+(n−5)+...+(n−(n−1))]x12y12. |
Consequently,
M1(T2)=(Dx+Dy)f(x,y)|x=1=y=678n−816+72(n−1)2+108[(n−(n−1))+(n−(n−2))+...+(n−4)+(n−3)]+72[(n−4)+(n−5)+...+(n−(n−1))],M2(T2)=DxDyf(x,y)|x=1=y=2424n−3774+216(n−1)2+432[(n−(n−1))+(n−(n−2))+...+(n−4)+(n−3)]+432[(n−4)+(n−5)+...+(n−(n−1))],mM2(T2)=SxSyf(x,y)|x=1=y=1116(n+1)+25(n−2)+1320(n−1)+120(n−3)+710+16(n−1)2+112[(n−(n−1))+(n−(n−2))+...+(n−4)+(n−3)]+148[(n−4)+(n−5)+...+(n−(n−1))],Rα(T2)=DαxDαyf(x,y)|x=1=y=12α(6n+6)+24α(9)+30α(3n−6)+42α(3n+3)+20α(6n−6)+24α(6n−6)+30α(3n−3)+40α(6)+50α(6n−12)+6(n−1)2(36)α+48α(3)+60α(9n−18)+72α[(n−(n−1))+(n−(n−2))+...+(n−4)+(n−3)]6+80α(6)+100α(3n−6)+120α(6n−18)+144α[(n−4)+(n−5)+...+(n−(n−1))]3,RRα(T2)=SαxSαyf(x,y)|x=1=y=612α(n+1)+924α+330α(n−2)+342α(n+1)+620α(n−1)+624α(n−1)+330α(n−1)+640α+650α(n−2)+636α(n−1)2+348α+960α(n−2)+680α+3100α(n−2)+172α[(n−(n−1))+(n−(n−2))+...+(n−4)+(n−3)]6+6120α(n−3)+1144α[(n−4)+(n−5)+...+(n−(n−1))]3,SDD(T2)=(SyDx+SxDy)(x,y)|x=1=y=372(n+1)+1575(n−1)+52310(n−2)+615(n−3)+237140+12(n−1)2+15[(n−(n−1))+(n−(n−2))+...+(n−4)+(n−3)]+6[(n−4)+(n−5)+...+(n−(n−1))]. |
For T2, the Atomic Bond Connectivity, Geometric Arithmetic Index and General Harmonic Index are as follows, respectively.
1) ABC(T2)=(7n)√64+(n+1)√15+(n−1)(3√75+2√3+3√310)(n−2)(√33010+3√265+3√21010+9√210)+3√11010+32+6√5+(n−1)2√10+[(n−(n−1))+(n−(n−2))+...+(n−4)+(n−3)]2√2+[(n−4)+(n−5)+...+(n−(n−1))]√224,2) GA(T2)=(24n+36)√37+(132n+48)√655+(50n−113)6√30143+3(n+1)+(8n)√53+(n−2)(4√2+9√154+3)+24√1013+6(n−1)2+[(n−(n−1))+(n−(n−2))+...+(n−4)+(n−3)]4√2+3[(n−4)+(n−5)+...+(n−(n−1))],3) Hk(T2)=(6n+6)(27)k+9(211)k+(3n−6)(213)k+(3n+3)(14)k+(6n−6)(29)k+(6n−6)(15)k+(3n−3)(211)k+6(213)k+(6n−12)(215)k+6(n−1)2)(16)k+3(17)k+(9n−18)(18)k+6[(n−(n−1))+(n−(n−2))+...+(n−4)+(n−3)](19)k+6(19)k+(3n−6)(110)k+(6n−18)(111)k+3[(n−4)+(n−5)+...+(n−(n−1))](112)k. |
Proof: Using Eqs 1.7–1.9 and Table 4, we get the results 1–3.
For T2, the First, Second Gourava Indices and the First, Second Hyper Gourava Indices are as follows, respectively.
1) GO1(T2)=3102n−4590+288(n−1)2+540[(n−(n−1))+(n−(n−2))+...+(n−4)+(n−3)]+504[(n−4)+(n−5)+...+(n−(n−1))],2) GO2(T2)=40548n−74610+2592(n−1)2+7776[(n−(n−1))+(n−(n−2))+...+(n−4)+(n−3)]+10368[(n−4)+(n−5)+...+(n−(n−1))],3) HGO1(T2)=267984n−531210+13824(n−1)2+48600[(n−(n−1))+(n−(n−2))+...+(n−4)+(n−3)]+84672[(n−4)+(n−5)+...+(n−(n−1))],4) HGO2(T2)=66901488n−158433396+1119744(n−1)2+10077696[(n−(n−1))+(n−(n−2))+...+(n−4)+(n−3)]+35831808[(n−4)+(n−5)+...+(n−(n−1))]. |
Proof: Using Eqs 1.10–1.13 and Table 4 we get the above results 1–4.
In this paper, we defined M-polynomial of the transformed zigzag benzenoid and transformed triangular benzenoid structures by applying stellation, medial and bounded dual operations to get networks named as T1 and T2. With the help of M-polynomial, we computed certain degree-based topological indices such as first Zagreb index, second Zagreb index, second modified Zagreb index, general Randic index, reciprocal general Randic index, symmetric division degree index. We also computed atomic bound connectivity index, geometric arithmetic index, general harmonic index, first and second gourava indices, first and second hyper gourava indices. M-polynomial is used to calculate the certain degree based topological indices as a latest developed instrument in the chemical graph theory. In future, we can compute other indices on these structures and some additional transformed structures can be studied for a variety of topological indices to have an insight about their properties.
This work was supported by the Key teaching research project of quality engineering in Colleges and universities in Anhui Province. Anhui Vocational college of Electronics and Information Technology Guangzhou Zhuoya Education Investment Co., Ltd. Practical Education Base (subject No: 2020sjjd093). The authors are very thankful for this research funding. We are also very grateful to the reviewers for their valuable suggestions.
The authors declare no conflict of interest.
[1] |
A. Khwaja, M. Bjorkholm, R. E. Gale, R. L. Levine, C. T. Jordan, G. Ehninger, et al., Acute myeloid leukaemia, Nat. Rev. Dis. Primers, 2 (2016), 16010. https://doi.org/10.1038/nrdp.2016.10 doi: 10.1038/nrdp.2016.10
![]() |
[2] |
E. Estey, H. Dohner, Acute myeloid leukaemia, Lancet, 368 (2006), 1894-1907. https://doi.org/10.1016/S0140-6736(06)69780-8 doi: 10.1016/S0140-6736(06)69780-8
![]() |
[3] |
L. Bullinger, K. Dohner, E. Bair, S. Frohling, R. F. Schlenk, R. Tibshirani, et al., Use of gene-expression profiling to identify prognostic subclasses in adult acute myeloid leukemia, N. Eng. J. Med., 350 (2004), 1605-1616. https://doi.org/10.1056/NEJMoa031046 doi: 10.1056/NEJMoa031046
![]() |
[4] |
E. Papaemmanuil, M. Gerstung, L. Bullinger, V. I. Gaidzik, P. Paschka, N. D. Roberts, et al., Genomic classification and prognosis in acute myeloid leukemia, N. Eng. J. Med., 374 (2016), 2209-2221. https://doi.org/10.1056/NEJMoa1516192 doi: 10.1056/NEJMoa1516192
![]() |
[5] |
C. C. Coombs, M. S. Tallman, R. L. Levine, Molecular therapy for acute myeloid leukaemia, Nat. Rev. Clin. Oncol., 13 (2016), 305-318. https://doi.org/10.1038/nrclinonc.2015.210 doi: 10.1038/nrclinonc.2015.210
![]() |
[6] |
J. W. Tyner, C. E. Tognon, D. Bottomly, B. Wilmot, S. E. Kurtz, S. L. Savage, et al., Functional genomic landscape of acute myeloid leukaemia, Nature, 562 (2018), 526-531. https://doi.org/10.1038/s41586-018-0623-z doi: 10.1038/s41586-018-0623-z
![]() |
[7] |
S. Abelson, G. Collord, S. W. K. Ng, O. Weissbrod, N. M. Cohen, E. Niemeyer, et al., Prediction of acute myeloid leukaemia risk in healthy individuals, Nature, 559 (2018), 400-404. https://doi.org/10.1038/s41586-018-0317-6 doi: 10.1038/s41586-018-0317-6
![]() |
[8] |
S. C. Meyer, R. L. Levine, Translational implications of somatic genomics in acute myeloid leukaemia, Lancet Oncol., 15 (2014), e382-394. https://doi.org/10.1016/S1470-2045(14)70008-7 doi: 10.1016/S1470-2045(14)70008-7
![]() |
[9] |
T. Bratkovic, J. Bozic, B. Rogelj, Functional diversity of small nucleolar RNAs, Nucleic Acids Res., 48 (2020), 1627-1651. https://doi.org/10.1093/nar/gkz1140 doi: 10.1093/nar/gkz1140
![]() |
[10] |
J. Ni, A. L. Tien, M. J. Fournier, Small nucleolar RNAs direct site-specific synthesis of pseudouridine in ribosomal RNA, Cell, 89 (1997), 565-573. https://doi.org/10.1016/s0092-8674(00)80238-x doi: 10.1016/s0092-8674(00)80238-x
![]() |
[11] |
V. Chikne, K. S. Rajan, M. Shalev-Benami, K. Decker, S. Cohen-Chalamish, H. Madmoni, et al., Small nucleolar RNAs controlling rRNA processing in Trypanosoma brucei, Nucleic Acids Res., 47 (2019), 2609-2629. https://doi.org/10.1093/nar/gky1287 doi: 10.1093/nar/gky1287
![]() |
[12] |
L. Xing, X. Zhang, X. Zhang, D. Tong, Expression scoring of a small-nucleolar-RNA signature identified by machine learning serves as a prognostic predictor for head and neck cancer, J. Cell Phys., 235 (2020), 8071-8084. https://doi.org/10.1002/jcp.29462 doi: 10.1002/jcp.29462
![]() |
[13] |
Y. Zhao, Y. Yan, R. Ma, X. Lv, L. Zhang, J. Wang, et al., Expression signature of six-snoRNA serves as novel non-invasive biomarker for diagnosis and prognosis prediction of renal clear cell carcinoma, J. Cell Mol. Med., 24 (2020), 2215-2228. https://doi.org/10.1111/jcmm.14886 doi: 10.1111/jcmm.14886
![]() |
[14] | L. Huang, X. Z. Liang, Y. Deng, Y. B. Liang, X. Zhu, X. Y. Liang, et al., Prognostic value of small nucleolar RNAs (snoRNAs) for colon adenocarcinoma based on RNA sequencing data, Pathol. Res. Pract., 216 (2020), 152937. https: //doi.org/10.1016/j.prp.2020.152937 |
[15] |
J. Gong, Y. Li, C. J. Liu, Y. Xiang, C. Li, Y. Ye, et al., A pan-cancer analysis of the expression and clinical relevance of small nucleolar RNAs in human cancer, Cell Rep., 21 (2017), 1968-1981. https://doi.org/10.1016/j.celrep.2017.10.070 doi: 10.1016/j.celrep.2017.10.070
![]() |
[16] | The Cancer Genome Atlas Research Network, Genomic and epigenomic landscapes of adult de novo acute myeloid leukemia, N. Eng. J. Med., 368 (2013), 2059-2074. https://doi.org/10.1056/NEJMoa1301689 |
[17] |
M. D. Robinson, D. J. McCarthy, G. K. Smyth, edgeR: a Bioconductor package for differential expression analysis of digital gene expression data, Bioinformatics, 26 (2010), 139-140. https://doi.org/10.1093/bioinformatics/btp616 doi: 10.1093/bioinformatics/btp616
![]() |
[18] |
R. Huang, X. Liao, Q. Li, Identification and validation of potential prognostic gene biomarkers for predicting survival in patients with acute myeloid leukemia, Oncol. Targets Ther., 10 (2017), 5243-5254. https://doi.org/10.2147/OTT.S147717 doi: 10.2147/OTT.S147717
![]() |
[19] |
X. Liao, X. Wang, K. Huang, C. Yang, T. Yu, C. Han, et al., Genome-scale analysis to identify prognostic microRNA biomarkers in patients with early stage pancreatic ductal adenocarcinoma after pancreaticoduodenectomy, Cancer Manage. Res., 10 (2018), 2537-2551. https://doi.org/10.2147/CMAR.S168351 doi: 10.2147/CMAR.S168351
![]() |
[20] |
X. Liao, X. Wang, K. Huang, C. Han, J. Deng, T. Yu, et al., Integrated analysis of competing endogenous RNA network revealing potential prognostic biomarkers of hepatocellular carcinoma, J. Cancer, 10 (2019), 3267-3283. https://doi.org/10.7150/jca.29986 doi: 10.7150/jca.29986
![]() |
[21] |
W. H. Da, B. T. Sherman, R. A. Lempicki, Systematic and integrative analysis of large gene lists using DAVID bioinformatics resources, Nat. Protoc., 4 (2009), 44-57. https://doi.org/10.1038/nprot.2008.211 doi: 10.1038/nprot.2008.211
![]() |
[22] |
V. K. Mootha, C. M. Lindgren, K. F. Eriksson, A. Subramanian, S. Sihag, J. Lehar, et al., PGC-1alpha-responsive genes involved in oxidative phosphorylation are coordinately downregulated in human diabetes, Nat. Genet., 34 (2003), 267-273. https://doi.org/10.1038/ng1180 doi: 10.1038/ng1180
![]() |
[23] |
A. Subramanian, P. Tamayo, V. K. Mootha, S. Mukherjee, B. L. Ebert, M. A. Gillette, et al., Gene set enrichment analysis: a knowledge-based approach for interpreting genome-wide expression profiles, Proc. Nat. Acad. Sci. U. S. A., 102 (2005), 15545-15550. https://doi.org/10.1073/pnas.0506580102 doi: 10.1073/pnas.0506580102
![]() |
[24] |
A. Liberzon, C. Birger, H. Thorvaldsdottir, M. Ghandi, J. P. Mesirov, P. Tamayo, The molecular signatures database (MSigDB) hallmark gene set collection, Cell Syst., 1 (2015), 417-425. https://doi.org/10.1016/j.cels.2015.12.004 doi: 10.1016/j.cels.2015.12.004
![]() |
[25] |
A. Liberzon, A. Subramanian, R. Pinchback, H. Thorvaldsdottir, P. Tamayo, J. P. Mesirov, Molecular signatures database (MSigDB) 3.0, Bioinformatics, 27 (2011), 1739-1740. https://doi.org/10.1093/bioinformatics/btr260 doi: 10.1093/bioinformatics/btr260
![]() |
[26] |
J. Lamb, The connectivity map: a new tool for biomedical research, Nat. Rev. Cancer, 7 (2007), 54-60. https://doi.org/10.1038/nrc2044 doi: 10.1038/nrc2044
![]() |
[27] |
J. Lamb, E. D. Crawford, D. Peck, J. W. Modell, I. C. Blat, M. J. Wrobel, et al., The connectivity map: using gene-expression signatures to connect small molecules, genes, and disease, Science, 313 (2006), 1929-1935. https://doi.org/10.1126/science.1132939 doi: 10.1126/science.1132939
![]() |
[28] | E. W. Sayers, J. Beck, J. R. Brister, E. E. Bolton, K. Canese, D. C. Comeau, et al., Database resources of the national center for biotechnology information, Nucleic Acids Res., 48 (2020), D9-D16. https://doi.org/10.1093/nar/gkz899 |
[29] | S. Kim, J. Chen, T. Cheng, A. Gindulyte, J. He, S. He, et al., PubChem 2019 update: improved access to chemical data, Nucleic Acids Res., 47 (2019), D1102-D1109. https://doi.org/10.1093/nar/gky1033 |
[30] | M. Kuhn, D. Szklarczyk, A. Franceschini, M. Campillos, C. V. Mering, L. J. Jensen, et al., STITCH 2: an interaction network database for small molecules and proteins, Nucleic Acids Res., 38 (2010), D552-556. https://doi.org/10.1093/nar/gkp937 |
[31] | M. Kuhn, C. V. Mering, M. Campillos, L. J. Jensen, P. Bork, STITCH: interaction networks of chemicals and proteins, Nucleic Acids Res., 36 (2008), D684-688. https://doi.org/10.1093/nar/gkm795 |
[32] | D. Szklarczyk, A. Santos, C. V. Mering, L. J. Jensen, P. Bork, M. Kuhn, STITCH 5: augmenting protein-chemical interaction networks with tissue and affinity data, Nucleic Acids Res., 44 (2016), D380-384. https://doi.org/10.1093/nar/gkv1277 |
[33] | K. Yoshihara, M. Shahmoradgoli, E. Martinez, R. Vegesna, H. Kim, W. Torres-Garcia, et al., Inferring tumour purity and stromal and immune cell admixture from expression data, Nat. Commun., 4 (2013), 2612. https://doi.org/10.1038/ncomms3612 |
[34] |
B. Chen, M. S. Khodadoust, C. L. Liu, A. M. Newman, A. A. Alizadeh, Profiling tumor infiltrating immune cells with CIBERSORT, Methods Mol. Biol., 1711 (2018), 243-259. https://doi.org/10.1007/978-1-4939-7493-1_12 doi: 10.1007/978-1-4939-7493-1_12
![]() |
[35] |
Y. Benjamini, D. Drai, G. Elmer, N. Kafkafi, I. Golani, Controlling the false discovery rate in behavior genetics research, Behav. Brain Res., 125 (2001), 279-284. https://doi.org/10.1016/s0166-4328(01)00297-2 doi: 10.1016/s0166-4328(01)00297-2
![]() |
[36] |
P. Shannon, A. Markiel, O. Ozier, N. S. Baliga, J. T. Wang, D. Ramage, et al., Cytoscape: a software environment for integrated models of biomolecular interaction networks, Genome Res., 13 (2003), 2498-2504. https://doi.org/10.1101/gr.1239303 doi: 10.1101/gr.1239303
![]() |
[37] | D. Otasek, J. H. Morris, J. Boucas, A. R. Pico, B. Demchak, Cytoscape automation: empowering workflow-based network analysis, Genome Biol., 20 (2019), 185. https://doi.org/10.1186/s13059-019-1758-4 |
[38] | D. Ronchetti, L. Mosca, G. Cutrona, G. Tuana, M. Gentile, S. Fabris, et al., Small nucleolar RNAs as new biomarkers in chronic lymphocytic leukemia, BMC Med. Genomics, 6 (2013), 27. https://doi.org/10.1186/1755-8794-6-27 |
[39] |
E. Bignotti, S. Calza, R. A. Tassi, L. Zanotti, E. Bandiera, E. Sartori, et al., Identification of stably expressed reference small non-coding RNAs for microRNA quantification in high-grade serous ovarian carcinoma tissues, J. Cell Mol. Med., 20 (2016), 2341-2348. https://doi.org/10.1111/jcmm.12927 doi: 10.1111/jcmm.12927
![]() |
[40] |
L. H. Mao, S. Y. Chen, X. Q. Li, F. Xu, J. Lei, Q. L. Wang, et al., LncRNA-LALR1 upregulates small nucleolar RNA SNORD72 to promote growth and invasion of hepatocellular carcinoma, Aging (Albany NY), 12 (2020), 4527-4546. https://doi.org/10.18632/aging.102907 doi: 10.18632/aging.102907
![]() |
[41] |
F. G. Lafaille, O. Harschnitz, Y. S. Lee, P. Zhang, M. L. Hasek, G. Kerner, et al., Human SNORA31 variations impair cortical neuron-intrinsic immunity to HSV-1 and underlie herpes simplex encephalitis, Nat. Med., 25 (2019), 1873-1884. https://doi.org/10.1038/s41591-019-0672-3 doi: 10.1038/s41591-019-0672-3
![]() |
[42] |
H. Davanian, A. Balasiddaiah, R. Heymann, M. Sundstrom, P. Redenstrom, M. Silfverberg, et al., Ameloblastoma RNA profiling uncovers a distinct non-coding RNA signature, Oncotarget, 8 (2017), 4530-4542. https://doi.org/10.18632/oncotarget.13889 doi: 10.18632/oncotarget.13889
![]() |
[43] | I. Nepstad, K. J. Hatfield, I. S. Gronningsaeter, H. Reikvam, The PI3K-Akt-mTOR signaling pathway in human acute myeloid leukemia (AML) cells, Int. J. Mol. Sci., 21 (2020), 2907. https://doi.org/10.3390/ijms21082907 |
[44] |
L. Herschbein, J. L. Liesveld, Dueling for dual inhibition: Means to enhance effectiveness of PI3K/Akt/mTOR inhibitors in AML, Blood Rev., 32 (2018), 235-248. https://doi.org/10.1016/j.blre.2017.11.006 doi: 10.1016/j.blre.2017.11.006
![]() |
[45] |
J. Bertacchini, N. Heidari, L. Mediani, S. Capitani, M. Shahjahani, A. Ahmadzadeh, et al., Targeting PI3K/AKT/mTOR network for treatment of leukemia, Cell Mol. Life Sci., 72 (2015), 2337-2347. https://doi.org/10.1007/s00018-015-1867-5 doi: 10.1007/s00018-015-1867-5
![]() |
[46] |
Y. Su, X. Li, J. Ma, J. Zhao, S. Liu, G. Wang, et al., Targeting PI3K, mTOR, ERK and Bcl-2 signaling network shows superior antileukemic activity against AML ex vivo, Biochem. Pharmacol., 148 (2018), 13-26. https://doi.org/10.1016/j.bcp.2017.11.022 doi: 10.1016/j.bcp.2017.11.022
![]() |
[47] |
Y. Tabe, A. Tafuri, K. Sekihara, H. Yang, M. Konopleva, Inhibition of mTOR kinase as a therapeutic target for acute myeloid leukemia, Expert Opin. Ther. Targets, 21 (2017), 705-714. https://doi.org/10.1080/14728222.2017.1333600 doi: 10.1080/14728222.2017.1333600
![]() |
[48] |
N. Guo, M. Azadniv, M. Coppage, M. Nemer, J. Mendler, M. Becker, et al., Effects of neddylation and mTOR inhibition in acute myelogenous leukemia, Transl. Oncol., 12 (2019), 602-613. https://doi.org/10.1016/j.tranon.2019.01.001 doi: 10.1016/j.tranon.2019.01.001
![]() |
[49] |
J. Wu, G. Hu, Y. Dong, R. Ma, Z. Yu, S. Jiang, et al., Matrine induces Akt/mTOR signalling inhibition-mediated autophagy and apoptosis in acute myeloid leukaemia cells, J. Cell Mol. Med., 21 (2017), 1171-1181. https://doi.org/10.1111/jcmm.13049 doi: 10.1111/jcmm.13049
![]() |
[50] | Y. Feng, L. Wu, mTOR up-regulation of PFKFB3 is essential for acute myeloid leukemia cell survival, Biochem. Biophys. Res. Commun., 483 (2017), 897-903. |
[51] |
J. Bertacchini, C. Frasson, F. Chiarini, D. D'Avella, B. Accordi, L. Anselmi, et al., Dual inhibition of PI3K/mTOR signaling in chemoresistant AML primary cells, Adv. Biol. Regul., 68 (2018), 2-9. https://doi.org/10.1016/j.jbior.2018.03.001 doi: 10.1016/j.jbior.2018.03.001
![]() |
[52] |
V. Stavropoulou, S. Kaspar, L. Brault, M. A. Sanders, S. Juge, S. Morettini, et al., MLL-AF9 expression in hematopoietic stem cells drives a highly invasive AML expressing EMT-related genes linked to poor outcome, Cancer Cell, 30 (2016), 43-58. https://doi.org/10.1016/j.ccell.2016.05.011 doi: 10.1016/j.ccell.2016.05.011
![]() |
[53] |
T. J. Zhang, J. D. Zhou, J. C. Ma, Z. Q. Deng, Z. Qian, D. M. Yao, et al., CDH1 (E-cadherin) expression independently affects clinical outcome in acute myeloid leukemia with normal cytogenetics, Clin. Chem. Lab. Med., 55 (2017), 123-131. https://doi.org/10.1515/cclm-2016-0205 doi: 10.1515/cclm-2016-0205
![]() |
[54] | S. Wu, Y. Du, J. Beckford, H. Alachkar, Upregulation of the EMT marker vimentin is associated with poor clinical outcome in acute myeloid leukemia, J. Transl. Med., 16 (2018), 170. https://doi.org/10.1186/s12967-018-1539-y |
[55] |
L. Zhong, J. Chen, X. Huang, Y. Li, T. Jiang, Monitoring immunoglobulin heavy chain and T-cell receptor gene rearrangement in cfDNA as minimal residual disease detection for patients with acute myeloid leukemia, Oncol. Lett., 16 (2018), 2279-2288. https://doi.org/10.3892/ol.2018.8966 doi: 10.3892/ol.2018.8966
![]() |
[56] |
A. G. Chapuis, D. N. Egan, M. Bar, T. M. Schmitt, M. S. McAfee, K. G. Paulson, et al., T cell receptor gene therapy targeting WT1 prevents acute myeloid leukemia relapse post-transplant, Nat. Med., 25 (2019), 1064-1072. https://doi.org/10.1038/s41591-019-0472-9 doi: 10.1038/s41591-019-0472-9
![]() |
[57] |
H. J. Stauss, S. Thomas, M. Cesco-Gaspere, D. P. Hart, S. A. Xue, A. Holler, et al., WT1-specific T cell receptor gene therapy: improving TCR function in transduced T cells, Blood Cells Mol. Dis., 40 (2008), 113-116. https://doi.org/10.1016/j.bcmd.2007.06.018 doi: 10.1016/j.bcmd.2007.06.018
![]() |
[58] |
Y. Wang, A. V. Krivtsov, A. U. Sinha, T. E. North, W. Goessling, Z. Feng, et al., The Wnt/beta-catenin pathway is required for the development of leukemia stem cells in AML, Science, 327 (2010), 1650-1653. https://doi.org/10.1126/science.1186624 doi: 10.1126/science.1186624
![]() |
[59] | A. M. Gruszka, D. Valli, M. Alcalay, Wnt signalling in acute myeloid leukaemia, Cells, 8 (2019), 1403. https://doi.org/10.3390/cells8111403 |
[60] | F. J. Staal, F. Famili, L. G. Perez, K. Pike-Overzet, Aberrant Wnt signaling in leukemia, Cancers (Basel), 8 (2016), 78. https://doi.org/10.3390/cancers8090078 |
[61] |
A. Valencia, J. Roman-Gomez, J. Cervera, E. Such, E. Barragan, P. Bolufer, et al., Wnt signaling pathway is epigenetically regulated by methylation of Wnt antagonists in acute myeloid leukemia, Leukemia, 23 (2009), 1658-1666. https://doi.org/10.1038/leu.2009.86 doi: 10.1038/leu.2009.86
![]() |
[62] |
E. A. Griffiths, S. D. Gore, C. Hooker, M. A. McDevitt, J. E. Karp, B. D. Smith, et al., Acute myeloid leukemia is characterized by Wnt pathway inhibitor promoter hypermethylation, Leuk. Lymphoma, 51 (2010), 1711-1719. https://doi.org/10.3109/10428194.2010.496505 doi: 10.3109/10428194.2010.496505
![]() |
[63] |
C. Gasparini, C. Celeghini, L. Monasta, G. Zauli, NF-kappaB pathways in hematological malignancies, Cell Mol. Life Sci., 71 (2014), 2083-2102. https://doi.org/10.1007/s00018-013-1545-4 doi: 10.1007/s00018-013-1545-4
![]() |
[64] |
M. Breccia, G. Alimena, NF-kappaB as a potential therapeutic target in myelodysplastic syndromes and acute myeloid leukemia, Expert Opin. Ther. Targets, 14 (2010), 1157-1176. https://doi.org/10.1517/14728222.2010.522570 doi: 10.1517/14728222.2010.522570
![]() |
[65] |
M. C. Bosman, J. J. Schuringa, E. Vellenga, Constitutive NF-kappaB activation in AML: Causes and treatment strategies, Crit. Rev. Oncol. Hematol., 98 (2016), 35-44. https://doi.org/10.1016/j.critrevonc.2015.10.001 doi: 10.1016/j.critrevonc.2015.10.001
![]() |
[66] |
J. Zhou, Y. Q. Ching, W. J. Chng, Aberrant nuclear factor-kappa B activity in acute myeloid leukemia: from molecular pathogenesis to therapeutic target, Oncotarget, 6 (2015), 5490-5500. https://doi.org/10.18632/oncotarget.3545 doi: 10.18632/oncotarget.3545
![]() |
[67] |
C. H. Choi, H. Xu, H. Bark, T. B. Lee, J. Yun, S. I. Kang, et al., Balance of NF-kappaB and p38 MAPK is a determinant of radiosensitivity of the AML-2 and its doxorubicin-resistant cell lines, Leuk. Res., 31 (2007), 1267-1276. https://doi.org/10.1016/j.leukres.2006.11.006 doi: 10.1016/j.leukres.2006.11.006
![]() |
[68] |
A. Volk, J. Li, J. Xin, D. You, J. Zhang, X. Liu, et al., Co-inhibition of NF-kappaB and JNK is synergistic in TNF-expressing human AML, J. Exp. Med., 211 (2014), 1093-1108. https://doi.org/10.1084/jem.20130990 doi: 10.1084/jem.20130990
![]() |
[69] |
M. C. Bosman, H. Schepers, J. Jaques, A. Z. Brouwers-Vos, W. J. Quax, J. J. Schuringa, et al., The TAK1-NF-kappaB axis as therapeutic target for AML, Blood, 124 (2014), 3130-3140. https://doi.org/10.1182/blood-2014-04-569780 doi: 10.1182/blood-2014-04-569780
![]() |
[70] | M. Ma, X. Wang, N. Liu, F. Shan, Y. Feng, Low-dose naltrexone inhibits colorectal cancer progression and promotes apoptosis by increasing M1-type macrophages and activating the Bax/Bcl-2/caspase-3/PARP pathway, Int. Immunopharmacol., 83 (2020), 106388. https://doi.org/10.1016/j.intimp.2020.106388 |
[71] | N. Liu, M. Ma, N. Qu, R. Wang, H. Chen, F. Hu, et al., Low-dose naltrexone inhibits the epithelial-mesenchymal transition of cervical cancer cells in vitro and effects indirectly on tumor-associated macrophages in vivo, Int. Immunopharmacol., 86 (2020), 106718. https://doi.org/10.1016/j.intimp.2020.106718 |
[72] |
A. C. Menezes, M. Carvalheiro, J. M. P. F. de Oliveira, A. Ascenso, H. Oliveira, Cytotoxic effect of the serotonergic drug 1-(1-Naphthyl)piperazine against melanoma cells, Toxicol. Int. Vitro, 47 (2018), 72-78. https://doi.org/10.1016/j.tiv.2017.11.011 doi: 10.1016/j.tiv.2017.11.011
![]() |
[73] | G. G. Wei, L. Gao, Z. Y. Tang, P. Lin, L. B. Liang, J. J. Zeng, et al., Drug repositioning in head and neck squamous cell carcinoma: An integrated pathway analysis based on connectivity map and differential gene expression, Pathol. Res. Pract., 215 (2019), 152378. https://doi.org/10.1016/j.prp.2019.03.007 |
[74] |
J. Takezawa, Y. Ishimi, K. Yamada, Proteasome inhibitors remarkably prevent translesion replication in cancer cells but not normal cells, Cancer Sci., 99 (2008), 863-871. https://doi.org/10.1111/j.1349-7006.2008.00764.x doi: 10.1111/j.1349-7006.2008.00764.x
![]() |
[75] |
P. G. Richardson, C. Mitsiades, T. Hideshima, K. C. Anderson, Bortezomib: proteasome inhibition as an effective anticancer therapy, Annu. Rev. Med., 57 (2006), 33-47. https://doi.org/10.1146/annurev.med.57.042905.122625 doi: 10.1146/annurev.med.57.042905.122625
![]() |
[76] |
I. Zavrski, C. Naujokat, K. Niemoller, C. Jakob, U. Heider, C. Langelotz, et al., Proteasome inhibitors induce growth inhibition and apoptosis in myeloma cell lines and in human bone marrow myeloma cells irrespective of chromosome 13 deletion, J. Cancer Res. Clin. Oncol., 129 (2003), 383-391. https://doi.org/10.1007/s00432-003-0454-6 doi: 10.1007/s00432-003-0454-6
![]() |
[77] | W. X. Wang, B. H. Kong, P. Li, K. Song, X. Qu, B. X. Cui, et al., Effect of extracellular signal regulated kinase signal pathway on apoptosis induced by MG262 in ovarian cancer cells, Zhonghua Fu Chan Ke Za Zhi, 43 (2008), 690-694 |
[78] |
J. Y. Wu, S. S. Lin, F. T. Hsu, J. G. Chung, Fluoxetine inhibits DNA repair and NF-kB-modulated metastatic potential in non-small cell lung cancer, Anticancer Res., 38 (2018), 5201-5210. https://doi.org/10.21873/anticanres.12843 doi: 10.21873/anticanres.12843
![]() |
[79] | L. C. Hsu, H. F. Tu, F. T. Hsu, P. F. Yueh, I. T. Chiang, Beneficial effect of fluoxetine on anti-tumor progression on hepatocellular carcinoma and non-small cell lung cancer bearing animal model, Biomed. Pharmacother., 126 (2020), 110054. https://doi.org/10.1016/j.biopha.2020.110054 |
[80] | A. R. Mun, S. J. Lee, G. B. Kim, H. S. Kang, J. S. Kim, S. J. Kim, Fluoxetine-induced apoptosis in hepatocellular carcinoma cells, Anticancer Res., 33 (2013), 3691-3697 |
[81] | D. Sun, L. Zhu, Y. Zhao, Y. Jiang, L. Chen, Y. Yu, et al., Fluoxetine induces autophagic cell death via eEF2K-AMPK-mTOR-ULK complex axis in triple negative breast cancer, Cell Prolif., 51 (2018), e12402. https://doi.org/10.1111/cpr.12402 |
[82] |
A. M. Kabel, A. A. Elkhoely, Ameliorative potential of fluoxetine/raloxifene combination on experimentally induced breast cancer, Tissue Cell, 48 (2016), 89-95. https://doi.org/10.1016/j.tice.2016.02.002 doi: 10.1016/j.tice.2016.02.002
![]() |
[83] |
M. Bowie, P. Pilie, J. Wulfkuhle, S. Lem, A. Hoffman, S. Desai, et al., Fluoxetine induces cytotoxic endoplasmic reticulum stress and autophagy in triple negative breast cancer, World J. Clin. Oncol., 6 (2015), 299-311. https://doi.org/10.5306/wjco.v6.i6.299 doi: 10.5306/wjco.v6.i6.299
![]() |
[84] |
T. M. Khing, W. W. Po, U. D. Sohn, Fluoxetine enhances anti-tumor activity of paclitaxel in gastric adenocarcinoma cells by triggering apoptosis and necroptosis, Anticancer Res., 39 (2019), 6155-6163. https://doi.org/10.21873/anticanres.13823 doi: 10.21873/anticanres.13823
![]() |
[85] |
P. P. Khin, W. W. Po, W. Thein, U. D. Sohn, Apoptotic effect of fluoxetine through the endoplasmic reticulum stress pathway in the human gastric cancer cell line AGS, Naunyn Schmiedebergs Arch. Pharmacol., 393 (2020), 537-549. https://doi.org/10.1007/s00210-019-01739-7 doi: 10.1007/s00210-019-01739-7
![]() |
[86] | M. Marcinkute, S. Afshinjavid, A. A. Fatokun, F. A. Javid, Fluoxetine selectively induces p53-independent apoptosis in human colorectal cancer cells, Eur. J. Pharmacol., 857 (2019), 172441. https://doi.org/10.1016/j.ejphar.2019.172441 |
[87] |
V. Kannen, S. B. Garcia, W. A. Silva, M. Gasser, R. Monch, E. J. Alho, et al., Oncostatic effects of fluoxetine in experimental colon cancer models, Cell Signal, 27 (2015), 1781-1788. https://doi.org/10.1016/j.cellsig.2015.05.008 doi: 10.1016/j.cellsig.2015.05.008
![]() |
[88] | V. Kannen, H. Hintzsche, D. L. Zanette, W. A. Silva, S. B. Garcia, A. M. Waaga-Gasser, et al., Antiproliferative effects of fluoxetine on colon cancer cells and in a colonic carcinogen mouse model, PLoS One, 7 (2012), e50043. https://doi.org/10.1371/journal.pone.0050043 |
[89] |
H. Stopper, S. B. Garcia, A. M. Waaga-Gasser, V. Kannen, Antidepressant fluoxetine and its potential against colon tumors, World J. Gastrointest. Oncol., 6 (2014), 11-21. https://doi.org/10.4251/wjgo.v6.i1.11 doi: 10.4251/wjgo.v6.i1.11
![]() |
[90] | S. J. Koh, J. M. Kim, I. K. Kim, N. Kim, H. C. Jung, I. S. Song, et al., Fluoxetine inhibits NF-kappaB signaling in intestinal epithelial cells and ameliorates experimental colitis and colitis-associated colon cancer in mice, Am. J. Physiol. Gastrointest. Liver Phys., 301 (2011), G9-19. https://doi.org/10.1152/ajpgi.00267.2010 |
[91] |
K. H. Liu, S. T. Yang, Y. K. Lin, J. W. Lin, Y. H. Lee, J. Y. Wang, et al., Fluoxetine, an antidepressant, suppresses glioblastoma by evoking AMPAR-mediated calcium-dependent apoptosis, Oncotarget, 6 (2015), 5088-5101. https://doi.org/10.18632/oncotarget.3243 doi: 10.18632/oncotarget.3243
![]() |
[92] |
J. Ma, Y. R. Yang, W. Chen, M. H. Chen, H. Wang, X. D. Wang, et al., Fluoxetine synergizes with temozolomide to induce the CHOP-dependent endoplasmic reticulum stress-related apoptosis pathway in glioma cells, Oncol. Rep., 36 (2016), 676-684. https://doi.org/10.3892/or.2016.4860 doi: 10.3892/or.2016.4860
![]() |
![]() |
![]() |
1. | Deeba Afzal, Farkhanda Afzal, Sabir Hussain, Faryal Chaudhry, Dhana Kumari Thapa, Gohar Ali, Investigation on Boron Alpha Nanotube by Studying Their M-Polynomial and Topological Indices, 2022, 2022, 2314-4785, 1, 10.1155/2022/6136168 | |
2. | Jia-Bao Liu, Hifza Iqbal, Khurram Shahzad, Topological Properties of Concealed Non-Kekulean Benzenoid Hydrocarbon, 2023, 43, 1040-6638, 1776, 10.1080/10406638.2022.2039230 | |
3. | Kamel Jebreen, Hifza Iqbal, Muhammad Haroon Aftab, Iram Yaqoob, Mohammed Issa Sowaity, Amjad Barham, Study of eccentricity based topological indices for benzenoid structure, 2023, 45, 10269185, 221, 10.1016/j.sajce.2023.05.010 | |
4. | Claudio Rocco, Jose A. Moronta, A Novel Perspective for Hosoya and M Polynomials for Analyzing Electric Power Network Topology, 2024, 1556-5068, 10.2139/ssrn.4806323 | |
5. | Hifza Iqbal, Muhammad Haroon Aftab, Ali Akgul, Zeeshan Saleem Mufti, Iram Yaqoob, Mustafa Bayram, Muhammad Bilal Riaz, Further study of eccentricity based indices for benzenoid hourglass network, 2023, 9, 24058440, e16956, 10.1016/j.heliyon.2023.e16956 |
Topological Index | Derivation from M (K; x, y) | |
First Zagreb | (Dx+Dy)(M(K;x,y))|x=1=y | (ii) |
Second Zagreb | (DxDy)(M(K;x,y))|x=1=y | (iii) |
Second Modified Zagreb | (SxSy)(M(K;x,y))|x=1=y | (iv) |
general Randic | (DαxDαy)(M(K;x,y))|x=1=y | (v) |
Reciprocal general Randic | (SαxSαy)(M(K;x,y))|x=1=y | (vi) |
Symmetric Division Index | (SyDx)+(SxDy)(M(K;x,y))|x=1=y | (vii) |
Graph | Planar/ Non−Planar |
St(G1,G2) | Planar |
Md(G1,G2) | Planar |
Bdu(G1,G2) | Non−Planar |
T1 | Non−Planar |
T2 | Non−Planar |
Types of edges | Count of edges |
(3,4) | 8n+8 |
(3,7) | 8 |
(3,8) | 4n−4 |
(4,4) | 4n+4 |
(4,5) | 8n−4 |
(4,6) | 8n−4 |
(5,6) | 4n−2 |
(5,7) | 4 |
(5,8) | 8n−8 |
(7,8) | 2 |
(8,8) | 2n−3 |
Types of edges | Count of edges |
(3,4) | 6n+6 |
(3,8) | 9 |
(3,10) | 3n−6 |
(4,4) | 3n+3 |
(4,5) | 6n−6 |
(4,6) | 6n−6 |
(5,6) | 3n−3 |
(5,8) | 6 |
(5,10) | 6n−12 |
(6,6) | 6(n−1)2 |
(6,8) | 3 |
(6,10) | 9n−18 |
(6,12) | 6[(n−(n−1))+(n−(n−2))+... |
+(n−4)+(n−3)] | |
(8,10) | 6 |
(10,10) | 3n−6 |
(10,12) | 6n−18 |
(12,12) | 3[(n−4)+(n−5)+... |
+(n−(n−1))] |
Topological Index | Derivation from M (K; x, y) | |
First Zagreb | (Dx+Dy)(M(K;x,y))|x=1=y | (ii) |
Second Zagreb | (DxDy)(M(K;x,y))|x=1=y | (iii) |
Second Modified Zagreb | (SxSy)(M(K;x,y))|x=1=y | (iv) |
general Randic | (DαxDαy)(M(K;x,y))|x=1=y | (v) |
Reciprocal general Randic | (SαxSαy)(M(K;x,y))|x=1=y | (vi) |
Symmetric Division Index | (SyDx)+(SxDy)(M(K;x,y))|x=1=y | (vii) |
Graph | Planar/ Non−Planar |
St(G1,G2) | Planar |
Md(G1,G2) | Planar |
Bdu(G1,G2) | Non−Planar |
T1 | Non−Planar |
T2 | Non−Planar |
Types of edges | Count of edges |
(3,4) | 8n+8 |
(3,7) | 8 |
(3,8) | 4n−4 |
(4,4) | 4n+4 |
(4,5) | 8n−4 |
(4,6) | 8n−4 |
(5,6) | 4n−2 |
(5,7) | 4 |
(5,8) | 8n−8 |
(7,8) | 2 |
(8,8) | 2n−3 |
Types of edges | Count of edges |
(3,4) | 6n+6 |
(3,8) | 9 |
(3,10) | 3n−6 |
(4,4) | 3n+3 |
(4,5) | 6n−6 |
(4,6) | 6n−6 |
(5,6) | 3n−3 |
(5,8) | 6 |
(5,10) | 6n−12 |
(6,6) | 6(n−1)2 |
(6,8) | 3 |
(6,10) | 9n−18 |
(6,12) | 6[(n−(n−1))+(n−(n−2))+... |
+(n−4)+(n−3)] | |
(8,10) | 6 |
(10,10) | 3n−6 |
(10,12) | 6n−18 |
(12,12) | 3[(n−4)+(n−5)+... |
+(n−(n−1))] |