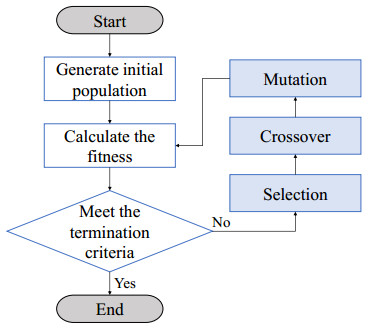
The neuropsychiatric systemic lupus erythematosus (NPSLE), a severe disease that can damage the heart, liver, kidney, and other vital organs, often involves the central nervous system and even leads to death. Magnetic resonance spectroscopy (MRS) is a brain functional imaging technology that can detect the concentration of metabolites in organs and tissues non-invasively. However, the performance of early diagnosis of NPSLE through conventional MRS analysis is still unsatisfactory. In this paper, we propose a novel method based on genetic algorithm (GA) and multi-agent reinforcement learning (MARL) to improve the performance of the NPSLE diagnosis model. Firstly, the proton magnetic resonance spectroscopy (1H-MRS) data from 23 NPSLE patients and 16 age-matched healthy controls (HC) were standardized before training. Secondly, we adopt MARL by assigning an agent to each feature to select the optimal feature subset. Thirdly, the parameter of SVM is optimized by GA. Our experiment shows that the SVM classifier optimized by feature selection and parameter optimization achieves 94.9% accuracy, 91.3% sensitivity, 100% specificity and 0.87 cross-validation score, which is the best score compared with other state-of-the-art machine learning algorithms. Furthermore, our method is even better than other dimension reduction ones, such as SVM based on principal component analysis (PCA) and variational autoencoder (VAE). By analyzing the metabolites obtained by MRS, we believe that this method can provide a reliable classification result for doctors and can be effectively used for the early diagnosis of this disease.
Citation: Guanru Tan, Boyu Huang, Zhihan Cui, Haowen Dou, Shiqiang Zheng, Teng Zhou. A noise-immune reinforcement learning method for early diagnosis of neuropsychiatric systemic lupus erythematosus[J]. Mathematical Biosciences and Engineering, 2022, 19(3): 2219-2239. doi: 10.3934/mbe.2022104
[1] | Ruiping Yuan, Jiangtao Dou, Juntao Li, Wei Wang, Yingfan Jiang . Multi-robot task allocation in e-commerce RMFS based on deep reinforcement learning. Mathematical Biosciences and Engineering, 2023, 20(2): 1903-1918. doi: 10.3934/mbe.2023087 |
[2] | Xiaobo Zhang, Donghai Zhai, Yan Yang, Yiling Zhang, Chunlin Wang . A novel semi-supervised multi-view clustering framework for screening Parkinson's disease. Mathematical Biosciences and Engineering, 2020, 17(4): 3395-3411. doi: 10.3934/mbe.2020192 |
[3] | Wei Kong, Feifan Xu, Shuaiqun Wang, Kai Wei, Gen Wen, Yaling Yu . Application of orthogonal sparse joint non-negative matrix factorization based on connectivity in Alzheimer's disease research. Mathematical Biosciences and Engineering, 2023, 20(6): 9923-9947. doi: 10.3934/mbe.2023435 |
[4] | Zichen Wang, Xin Wang . Fault-tolerant control for nonlinear systems with a dead zone: Reinforcement learning approach. Mathematical Biosciences and Engineering, 2023, 20(4): 6334-6357. doi: 10.3934/mbe.2023274 |
[5] | Yangjie Sun, Xiaoxi Che, Nan Zhang . 3D human pose detection using nano sensor and multi-agent deep reinforcement learning. Mathematical Biosciences and Engineering, 2023, 20(3): 4970-4987. doi: 10.3934/mbe.2023230 |
[6] | Feng Guo, Haiyu Xu, Peng Xu, Zhiwei Guo . Design of a reinforcement learning-based intelligent car transfer planning system for parking lots. Mathematical Biosciences and Engineering, 2024, 21(1): 1058-1081. doi: 10.3934/mbe.2024044 |
[7] | Koji Oshima, Daisuke Yamamoto, Atsuhiro Yumoto, Song-Ju Kim, Yusuke Ito, Mikio Hasegawa . Online machine learning algorithms to optimize performances of complex wireless communication systems. Mathematical Biosciences and Engineering, 2022, 19(2): 2056-2094. doi: 10.3934/mbe.2022097 |
[8] | Shixuan Yao, Xiaochen Liu, Yinghui Zhang, Ze Cui . An approach to solving optimal control problems of nonlinear systems by introducing detail-reward mechanism in deep reinforcement learning. Mathematical Biosciences and Engineering, 2022, 19(9): 9258-9290. doi: 10.3934/mbe.2022430 |
[9] | Chun Li, Ying Chen, Zhijin Zhao . Frequency hopping signal detection based on optimized generalized S transform and ResNet. Mathematical Biosciences and Engineering, 2023, 20(7): 12843-12863. doi: 10.3934/mbe.2023573 |
[10] | Qing Zou, Zachary Miller, Sanja Dzelebdzic, Maher Abadeer, Kevin M. Johnson, Tarique Hussain . Time-Resolved 3D cardiopulmonary MRI reconstruction using spatial transformer network. Mathematical Biosciences and Engineering, 2023, 20(9): 15982-15998. doi: 10.3934/mbe.2023712 |
The neuropsychiatric systemic lupus erythematosus (NPSLE), a severe disease that can damage the heart, liver, kidney, and other vital organs, often involves the central nervous system and even leads to death. Magnetic resonance spectroscopy (MRS) is a brain functional imaging technology that can detect the concentration of metabolites in organs and tissues non-invasively. However, the performance of early diagnosis of NPSLE through conventional MRS analysis is still unsatisfactory. In this paper, we propose a novel method based on genetic algorithm (GA) and multi-agent reinforcement learning (MARL) to improve the performance of the NPSLE diagnosis model. Firstly, the proton magnetic resonance spectroscopy (1H-MRS) data from 23 NPSLE patients and 16 age-matched healthy controls (HC) were standardized before training. Secondly, we adopt MARL by assigning an agent to each feature to select the optimal feature subset. Thirdly, the parameter of SVM is optimized by GA. Our experiment shows that the SVM classifier optimized by feature selection and parameter optimization achieves 94.9% accuracy, 91.3% sensitivity, 100% specificity and 0.87 cross-validation score, which is the best score compared with other state-of-the-art machine learning algorithms. Furthermore, our method is even better than other dimension reduction ones, such as SVM based on principal component analysis (PCA) and variational autoencoder (VAE). By analyzing the metabolites obtained by MRS, we believe that this method can provide a reliable classification result for doctors and can be effectively used for the early diagnosis of this disease.
Systemic lupus erythematosus (SLE) is an autoimmune disease that manifests remarkable clinical heterogeneity. This disease affects multiple organs with variable severity [1,2,3], including joints, lungs, heart, skin, and blood cells [4,5,6]. Furthermore, it may cause serious organ complications, such as lupus nephritis (LN) [7,8]. Neuropsychiatric systemic lupus erythematosus (NPSLE), as the most unknown manifestation of SLE, has severe effects on the central nervous system (CNS), peripheral nervous system (PNS), and autonomous nervous system (ANS) [9,10]. The nomenclature of NPSLE published by the American College of Rheumatology (ACR) includes 12 CNS and 7 PNS manifestations, which is widely used in clinical practice and facilitates research [11].
Although recent studies have made great progress in understanding the pathogenesis of NPSLE [12,13], the early diagnosis of NPSLE is still challenging due to the lack of gold standards. To date, researchers usually diagnosis NPSLE through clinical manifestations and a set of tests to make assessments, including brain magnetic resonance imaging (MRI) [2,14,15]. Due to MRI being widely used, it is the choice of clinical evaluation of the work-up of NPSLE, which can reveal lesions in the brain tissue of the patients [16]. However, almost half of the clinically active NPSLE patients with the absence of MRI abnormalities because of the lack of remarkable imaging manifestations, which shows the limitation of MRI in the NPSLE diagnosis [17].
Magnetic resonance spectroscopy (MRS) is an alternative method for detecting the concentration of metabolites non-invasively in NPSLE. Although previous work based on MRS has devoted massive efforts, there is still a long way to improve the accuracy of early diagnosis of NPSLE. Firstly, due to the data collected by MRS usually being sample-limited, it is challenging to build a robust model in this condition. Besides, the distribution of samples on these metabolic characteristics is high dimensional and nonlinear, affecting the model's accuracy significantly. Recently, deep learning have been widely used in many domains, such as traffic flow forecasting [18,19], medical image processing [20,21], and intelligent systems [22,23]. Note that the deep learning technology requires high quality and a large amount of high-quality data [24]. In this paper, we employ multi-voxel proton MRS (MVS) instead of the standard single-voxel proton MRS (SVS) for more accurate diagnosis, considered 13 metabolites in 9 brain regions concurrently, and combined a total of 117 metabolic features in total. It is easy to fall into the local minimum value, so we believe this method does not apply to our dataset.
Support vector machine (SVM) [25] is naturally good at learning nonlinear dependencies with limited samples. Therefore, SVM is an ideal classifier to avoid the problem above. Unlike deep learning, SVM is more suitable for small and mid-sized datasets because its hyperparameters are determined by supported vectors. In theory, SVM can achieve the optimum solution in the global range. This algorithm has been adopted in many fields, such as computer vision and machine learning. Zhu et al. [26] combined SVM and YOLOv3 to grade food with an accuracy of 98.5% achieved by SVM while the accuracy of YOLOv3 is 85.7%, and the overall accuracy is 96.4%. Cai et al. [27] introduced a SVM-based ensemble learning framework for basketball outcomes prediction. Tan et al. [28] proposed a hybrid GA-SVR to effectively forecast the short-term traffic flow in a large scale. However, the application of SVM has shown that the appropriate selection of the kernel parameter γ and the penalty factor C is the key to improving the learning ability and generalization ability of the SVM. In this work, we employ the genetic algorithm (GA) to optimize the hyperparameters of SVM to learn the nonlinear dependencies in features. Although there are many optimization methods on SVM, such as Kouziokas et al. [29] introduced an SVM Kernel, which combined the neural network weight vector of particle swarm optimization (PSO) [30] with the SVM kernel of Bayesian Optimization for time series problems. Faris et al. [31] proposed an approach based on a nature-inspired metaheuristic called multi-verse optimizer (MVO) for selecting optimal features and optimizing the hyperparameters of SVM simultaneously. These works have been combined with parameter optimization, but few letters are available to analyze the NPSLE diagnosis.
In recent years, reinforcement learning (RL) has been widely used in the healthcare domain. Petersen et al. [32] used deep reinforcement learning (DRL) to find an adaptive and personalized treatment of multi-cytokine mediation sepsis. Yu et al. [33] studied the integration of causal factors into the RL process and proposed a causal policy gradient algorithm to obtain better dynamic treatment regimes (DTRs) in HIV management. Maicas et al. [34] proposed a method based on DRL to detect the breast lesions automatically from dynamic contrast-enhanced magnetic resonance volumes with high accuracy and less detection time. Inspired by the powerful capacity of RL, we explore the possibility of empowering RL to the early diagnosis of NPSLE to further improve the model's classification performance. In this paper, the multi-agent reinforcement learning (MARL) is used to find the optimal feature subsets from the subset space. The central idea is to "assign" an agent to each feature, which controls the individual features and decides whether the feature is included in the final feature subset. Furthermore, feature selection is another effective technique for improving SVM classification. Some of the features are noisy or irrelevant, which can increase the computation quantity or even reduce the performance of the classifier [35]. Therefore, effective feature selection is necessary to enhance generalization ability, avoid overfitting, and provide a better explanation [36].
Existing reports mainly focus on optimizing the hyperparameters of SVM, selecting feature subset, or applying it to other applications, for example, hospitalization expense modeling [37], land-cover classification [38] and Polystyrene binding peptides (PSBPs) identification [39]. In the sample-limited and high-dimensional situation, few studies related to the early diagnosis of NPSLE in the machine learning field. The objective of this letter is to optimize the feature subset and SVM hyperparameters. On the other hand, we explore whether the proposed method can improve the classification performance of NPSLE early diagnosis.
The main contribution of this paper is summarized as follows:
● We employ the multi-agent reinforcement learning to find the optimal feature subset.
● We propose a genetic algorithm optimized support vector machine classification model for early diagnosis of NPSLE.
● We conduct extensive experiments to demonstrate the feasibility of our model. The experimental results show the superior performance of the proposed model to state-of-the-art models.
The rest organization of this paper is as follows. Section 2 describes the dataset used and the background material related to this work. Next, we introduce and present the proposed model and its details in Section 3. The outcomes of the experiments are elucidated in Section 4. The discussion and conclusion are presented in Section 5.
In this section, we first explain the details of the dataset. Then, we briefly introduce the methods employed in this paper, including support vector machines (SVM), genetic algorithm (GA) and reinforcement learning (RL).
We analyzed proton magnetic resonance spectroscopy (1H-MRS) data from twenty-three NPSLE patients and nineteen age-matched volunteers as healthy controls (HCs) who were admitted to the 2nd Affiliated Hospital of Shantou University Medical College and Shantou Central Hospital. All volunteers had no history of hypertension or diabetes and no head trauma, neurological, psychiatric, metabolic diseases, or other systemic diseases that may affect the nervous system. All patients met the classification standard of American College of Rheumatology (ACR) criteria [3] SLE in 1997 and the 1999 ACR definitions for NPSLE. Because of the ethical implications, routine drug therapy for NPSLE patients was not discontinued in the case group during the study period. The mean age of NPSLE patients is 31.71 while the one of HC is 29.5; there is no statistical difference in age between the two groups (p=0.765). This study is reviewed and approved by the Ethics Committee of Shantou University Medical College. All subjects voluntarily participated in the study and signed the informed consent.
In order to evaluate the changes of metabolites in different brain regions of patients with NPSLE, a 3.0T MRI with MRS was performed in these subjects. Nine areas of metabolic data were collected, including right posterior cingulate gyrus (RPCG), left posterior cingulate gyrus (LPCG), right dorsal thalamus (RDT), left dorsal thalamus (LDT), right lentiform nucleus (RLN), left lentiform nucleus (LLN), right posterior paratrigonal white matter (RPWM), and left posterior paratrigonal white matter (LPWM), and right insula (RI) in all subjects. And obtained thirteen metabolic features from each brain region, include Creatine (Cr), Phosphocreatine (PCr), Cr + PCr, NAA, N-Acetylaspartylglutamate (NAAG), NAA + NAAG, NAA + NAAG / Cr + PCr ratio, myo-Inositol (mI), mI / Cr + PCr, Glycerophosphocholine (GPC / Cho) + Phosphocholine (Pch), Cho + Pch / Cr + PCr, Glutamate (Glu) + Glutamine (Gln), and Glu + Gln / Cr + PCr. Nine brain regions and thirteen metabolic characteristics were combined into 117 metabolic characteristics used as features in the dataset and set HC as 0 and NPSLE as 1 as the dataset's labels.
Support vector machine (SVM) is a powerful tool for binary and multi-class classification model developed by Vapnik and Cortes [25]. The learning strategy of SVM is to maximize the interval, which can be formalized as the regularized hinge loss function minimization problem. The basic idea of the SVM classifier is to find a hyperplane with the largest geometric interval between two groups that can correctly divide the data set. This is a small sample learning strategy with a solid theoretical foundation. The final decision function is only determined by a few support vectors. The computational complexity depends on the number of support vectors instead of the dimension of samples, which avoids the "curse of dimensionality" [40] in a sense.
In this paper, we present the dataset illustrated in Section 2.1 by D={(xi,yi)}i=1,…,N, where xi∈RM, RM is the sample space, yi∈{0,1} are the corresponding labels. N, and M are the number of samples and features, respectively. For each groundtruth yi, SVM model is to predict the corresponding result ^yi=f(xi) according to xi. f(x) is the classification function of SVM, which is defined as Eq (2.1).
f(x)=ωT⋅x+b, | (2.1) |
where ωT is the weight vector of nonlinear mapped metabolic characteristics, and b is a constant bias term. The target of SVM is to find the optimal hyperplane that is explained in Eq (2.1). The objective function is shown in Eq (2.2).
minω,b12||ω||2+CN∑i=1ξis.t.{yi(ωTxi+b)≥1−ξi,i=1,2,⋯,Nξi≥0, | (2.2) |
where 12||ω||2 is the regularization term that minimizes the L2 norm of the weight vector ω, and C∑Ni=1ξi is the training loss. Sepcifically, C is a penalty parameter of the training loss, ξi=max(0,1−yi(ω⋅xi+b)) is the slack variable.
The dataset we produced is high-dimensional (117 features) with limited samples (39 samples). SVM is good at handling small and medium-sized, nonlinear, and high-dimensional classification and regression problems and has succeeded in many practical applications. To this end, a reliable classification model based on SVM is naturally used to diagnose NPSLE.
In order to map our nonlinear dataset to high-dimensional space, we use kernel functions. In this letter, the radial basis kernel function (RBF) kernel is selected because it can classify data with high dimensions, unlike the linear kernel. Besides, the RBF kernel function has fewer parameters to define than the sigmoid kernel and the polynomial kernel. Specifically, it only has one hyperparameter γ that affects the "spread" of the kernel [38].
The Genetic Algorithm (GA) is to find the global optimal solution in the search space of complex problems, which is a computational model simulating the natural selection and genetic mechanism of Darwin's theory of biological evolution [41]. The genetic algorithm is simple to implement without a complex problem-solving process. Figure 1 shows the process of the genetic algorithm.
Firstly, designing a scheme to encode the given information into the specific bit string encoding and setting the random initial starting point as the "parents". The encoding improves the convergence speed of the algorithm. Then, constructing the fitness function and obtaining each individual's fitness. Thirdly, creating the next generation through genetic operations, including natural selection, crossover, and mutation, as shown in Figure 2. After a certain amount of reproduction, GA can get acceptable individuals that satisfy the termination criteria. Due to the survival struggle, the best choice of high probability population will reach the overall optimal level [42].
The basic model of reinforcement learning (RL) is the interaction between individual and environment [43], as shown in Figure 3. The individual/agent is the part that can take a series of actions and expect to get a higher reward r. The other part related to this called the environment. The whole process is divided into different time steps. At each moment, the environment and the agent will have the corresponding interaction. The agent can take certain actions, which are imposed on the environment. After receiving an agent's action, the environment will feedback the current state of the agent's environment and the reward generated by the previous action.
The goal of RL is to get more rewards in the long term. There is a mapping between state and action, generally expressed by π; that is, a=π(s), which means a state s can correspond to an action a. The agent executes the action at in the state st and then transfers to the next state st+1 with the probability T(st,at,st+1). At this moment, it receives the reward rt from the environment with a discount coefficient γ. So the sum of reward from the beginning of t time to the training end T is Rt=∑Tt′=tγt′−trt′. γ∈[0,1] is the discount coefficient, representing every moment's reward obtained in the future will be reduced. In order to estimate the cumulative reward Rπ obtained by strategy π in the future, the value functions including state value function V(s) and state-action pair value function Q(s,a) are used to quantify the cumulative reward in reinforcement learning.
Vπ(s)=E[Rt|st=s,π], | (2.3) |
Qπ(s,a)=E[Rt|st=s,at=a,π], | (2.4) |
if the expected reward of a strategy is greater than or equal to the expected reward of other strategies, then it is the optimal strategy π∗. The optimal state value function V∗(s) and the optimal state-action pair value function Q∗(s,a) can be defined according to the optimal strategy π∗ as follows:
V∗(s)=maxπE[Rt|st=s,π], | (2.5) |
Q∗(s,a)=maxπE[Rt|st=s,at=a,π], | (2.6) |
in the process of RL training, the optimal strategy π∗ is found by solving the value functions V∗(s) and Q∗(s,a).
First, we design MARL to select the optimal feature subset and then use the selected features to train the classifier optimized by GA. In general, GA for parameter optimization is related to chromosome design, fitness function construction, and system processing. GA designs a suitable chromosome through binary encoding, then computes the fitness value and evaluates it by the system architecture.
We transfer the feature selection into multi-agent reinforcement learning by assigning an agent to each feature. Figure 4 illustrates the structure of feature selection based on MARL. The structure consists of agents, environment, actions, state, reward and reward assignment strategy.
● Agents. For the dataset described in Section 2.1 with 117 features, we create 117 feature agents that decide to discard or select the corresponding feature.
● Environment. We regard the selected features, that is, feature subset, as the environment.
● Actions. Action "0" means that the feature is disabled and will be excluded in the feature subset while action "1" means the feature is included in the feature subset. Note that we employ the ϵ-greedy [44] to balance the exploration and exploitation. Specifically, the agent chooses the best action based on what it already knows at most of the time while it moves randomly with the probability ϵ.
● State. We extract the mutual-statistic information as the state of the environment. Assuming that the dataset after feature selection is the matrix F=(Fij)N×n, n is the length of the selected features, and N is the number of samples in our dataset, here is 39. We first extract the statistical information of F, including mean, median, standard deviation, maximum, and minimum, to construct matrix Fs=(Fsij)5×n. Next, we extract five types of statistical representation of each line in Fs, obtaining the mutual-statistic information Fms. The dimension of the Fms is 5×5.
● Reward. For our task, it is essential to boost the model's classification performance, here is accuracy Acc, and decrease the number of the feature subset n. To solve this issue, we define the overall reward function as follows:
R(a)={Accif n≤bAcc⋅b/nif n>b, | (3.1) |
where b is the upper bound of the size of selected features, and a is the joint action. The above global reward function balance the size of the selected subset and the performance of the model.
● Reward allocation. In this paper, the problem extends to multiple agents, where each agent acts simultaneously. We need to coordinate the cooperation and competition between agents [45]. The communication between features is planned by reward allocation. Specifically, if an agent i does not change its action at the current iteration, it will be excluded when allocating reward. The reward R is assigned equally to the agent that participates in the current iteration.
Q-learning is a prevalent reinforcement learning algorithm. At the time stamp t, an agent performs an action at in the state st, then the state of the environment turns into st+1, the corresponding reward rt is sent to this agent. In Q-learning, Q-value Q(st,at) is the maximum long-term reward after acting at in the state st, which observes the following rules:
Q(st,at)=rt+γmaxQ(st+1,at+1), | (3.2) |
where γ∈[0,1] is the discount coefficient. The pseudocode of feature selection based on MARL is detailed in Algorithm 1. After that, we obtain the feature subset F that be sent to GA-optimized SVM.
Algorithm 1: The pseudocode for feature selection based on MARL. |
Data: threshold ϵ, ϵ decay rate rϵ, upper bound of the feature subset b, discount coefficient γ, max iteration Imax, raw dataset D=(Dij)N×M
Result: feature subset F 1 Iteration I=0 2 while I≤Imax do ![]() 25 end 26 return F; |
The first step of GA is individual gene designing and coding. In optimizing the combination of the two SVM hyperparameters, the kernel function parameter C and the error penalty factor γ are real numbers. We adopt binary coding to transfer them into bit strings, encoding the binary string of l1 and l2 bits. The gene string structure is shown in Figure 5. By combining the binary code of l1 and l2 bits, the gene string is obtained.
Because the data input to SVM should be decimal, we need to decode it into a phenotype according to Eq 3.3
p=minp+maxp−minp2m−1dv, | (3.3) |
where p is the bit string, minp and maxp are the minima and maximum values of the parameter respectively, dv is the decimal value of the bit string and m is the length of the bit string.
The goal of GA is to optimize the performance of SVM, which helps SVM get better generalization ability. The training samples are divided into two parts, training data, and testing data. Because our data samples are very scarce and difficult to collect, we use k-fold cross-validation to prevent over-fitting. We choose the mean score of cross-validation as the objective function to be optimized, using Eq 3.4
CV(k)=1kn∑i=1MSEi, | (3.4) |
where k=10. Mean squared error (MSE) can evaluate the change degree of the data. The smaller the MSE value is, the better the accuracy of the prediction model to describe the experimental data is, which can be calculated by Eq 3.5
MSE=SSEn=1nm∑i=1wi(yi−ˆy)2, | (3.5) |
where y is the groundtruth, and ˆy is the predicted label.
To ensure the evolution to the direction of optimization, we use the wheel-roulette selection operator, which is based on an individual's fitness value. The higher fitness it has, the higher probability it can be selected.
The crossover operator is used to select individuals selected by the selection operator to reproduce the next generation. Two different chromosomes exchange genes at the exact location to create a new chromosome. The crossover operator includes two steps: random pairing and crossover. This paper uses a two-point crossover method; two crossover points are set in a single code string, and then partial gene exchange is performed. Take the second coding bit in the gene string as the first crossover point. Then, randomly generate a crossover point in the remaining binary code part, and exchange the corresponding gene fragments between the two crossover points. Figure 6 is an example describes the two-point crossover.
The mutation operator increases the ability of the genetic algorithm to find the global optimal solution. The mutation operator randomly changes the value of a position in the string with a certain probability. Specifically, converting the value of the binary-coded gene string from 0 to 1, or 1 to 0.
The structure of the SVM classifier based on MARL and GA is presented in Figure 7, and the detailed steps are described below:
1) Assign a feature for each agent and perform action "save" or "discard" through executing the optimal policy to select the optimal feature subset;
2) Initialize the population to generate a number of individuals, including the SVM hyperparameters (C, γ);
3) The two hyperparameters (C, γ) are substituted into SVM to train classifier;
4) Compute the fitness of each individual. The average score of cross-validation is taken as the objective function to be optimized, and C and γ are taken as decision variables;
5) Judge whether the ending condition is met. If the termination condition is met, exit the cycle and finish the genetic optimization to get the optimized hyperparameter combination(C, γ). Otherwise, go to step 6;
6) The individual with a high fitness will be chosen and maintained in the next generation;
7) After the selection, crossover, and mutation operator are executed to form a new generation of individuals, return to step 3 to continue.
Furthermore, to reduce the influence of large numerical features on the experiment, we scale the data before the first step, or the model may perform poorly. Here we use the robustScaler, which is defined as follows
v′i=vi−medianIQR, | (3.6) |
where vi is a sample value, median is the sample's median, and IQR is the interquartile distance of samples. To overcome the disadvantage of limited samples, we use stratified sampling five times. Compared with K-Fold, it can ensure that the proportion of samples in the training set and test set is the same as that in the original data set.
We use MARL for feature selection to eliminate features that may be irrelevant or redundant, then we obtain 27 features, as shown in Table 1. Table 2 lists the initial parameters of the GA. Choosing these parameter values is based on the highest fitness value of the population.
Brain region | Metabolite |
LDT | Cr |
LDT | PCr |
LDT | NAA |
LDT | Ins/Cr + PCr |
LDT | GPC + PCh |
LDT | GPC + PCh/Cr + PCr |
RDT | Ins |
LPCG | Cr |
LPCG | PCr |
LPCG | NAA + NAAG |
LPCG | Ins/Cr + PCr |
RPCG | Cr |
RPCG | PCr |
RPCG | GPC + PCh |
RPCG | Glu + Gln/Cr + PCr |
RPCG | NAA + NAAG/Cr + PCr |
LLN | Ins |
LLN | NAA + NAAG/Cr + PCr |
RLN | NAAG |
RLN | GPC + PCh |
LFWM | Ins |
LFWM | Cr + PCr |
LFWM | Ins/Cr + PCr |
RFWM | NAAG |
RFWM | NAA + NAAG |
RFWM | NAA + NAAG/Cr + PCr |
RI | Glu + Gln/Cr + PCr |
GA Parameters | Setting |
C | 2−7 |
γ | 29 |
Generations Number | 100 |
Population Size | 40 |
Selection Type | Wheel-roulette |
Crossover Type | Two-point Crossover |
Crossover Rate | 0.7 |
Mutation Rate | 0.02 |
Except for cross-validation score, sensitivity, specificity, and accuracy are used to evaluate the performance of these classifiers, which are defined in Table 3. We compare the performance of the SVM and other models, including Decision Tree, Bagging, AdaBoost, SVM and their combinations with PCA, SVM based on VAE, SVM based on GA, and the combination of SVM, MARL and GA. As shown in Table 4, the sensitivity, specificity, accuracy, and cross-validation scores of SVM are 73.9%, 75.0%, 74.4% and 0.754, respectively, while the corresponding metrics of our proposed method are 91.3%, 100%, 94.9% and 0.870, respectively, which are much higher than others and achieve the best results. In addition, Figure 8 presents the receiver operating characteristic (ROC) curves of different methods. In the case of SVM based on GA and MARL, the area under the curve (AUC) is 0.969, which is much higher than the AUC of other models, including other deep learning methods, such as the combination of VAE and SVM. The AUC of SVM and SVM based on PCA are only 0.854 and 0.867, respectively.
Measure | Formula |
Sensitivity | Sensitivity=TPTP+FN |
Specificity | Specificity=TNTN+FP |
Accuracy | Accuracy=TP+TNTP+TN+FP+FN |
Algorithms | Sensitivity | Specificity | Accuracy | Cross-validation score |
Bagging | 69.6% | 43.8% | 59.0% | 0.587 |
Decision Tree | 73.9% | 50.0% | 64.1% | 0.643 |
SVM | 73.9% | 75.0% | 74.4% | 0.754 |
AdaBoost | 87.0% | 56.3% | 74.4% | 0.761 |
PCA+AdaBoost | 78.3% | 75% | 76.9% | 0.763 |
VAE+SVM | 82.6% | 75% | 79.5% | 0.798 |
PCA+Decision Tree | 82.6% | 81.3% | 82.1% | 0.827 |
PCA+Bagging | 87.0% | 81.3% | 84.6% | 0.849 |
PCA+SVM | 78.3% | 93.8% | 84.6% | 0.852 |
GA+SVM | 91.3% | 93.8% | 92.3% | 0.864 |
MARL+GA+SVM | 91.3% | 100.0% | 94.9% | 0.870 |
Figure 9 shows the changes of optimal individual's fitness and the average fitness of the whole population. At the 18th generation, the population stops evolution because the optimal individual reaches the judgment threshold that stops the algorithm. In the final evaluation phase, the fitness value of the optimal individual has risen to 0.87.
In addition, we compare genetic algorithm with grid search, a popular parameter selection method. Through GA, we get the optimal value of C, and γ are 240.37, 0.0078, respectively. Therefore, we set the values of C to be selected are [240.30,240.37,240.40], and the ones of γ are [0.0075,0.0078,0.0080]. We explore the optimal parameter combination through grid search, and the result displayed in Table 5.
C | γ | Sensitivity | Specificity | Accuracy | Cross-validation score |
240.30 | 0.0080 | 82.6% | 81.3% | 82.1% | 0.827 |
0.0078 | 87.0% | 81.3% | 87.2% | 0.852 | |
0.0080 | 91.3% | 87.5% | 89.7% | 0.859 | |
240.37 | 0.0075 | 91.3% | 93.8% | 92.3% | 0.864 |
0.0078 | 91.3% | 100.0% | 94.9% | 0.870 | |
0.0075 | 87.0% | 93.8% | 92.3% | 0.862 | |
240.40 | 0.0075 | 87.0% | 93.8% | 89.7% | 0.859 |
0.0078 | 82.6% | 93.8% | 87.2% | 0.854 | |
0.0080 | 82.6% | 87.5% | 84.6% | 0.850 |
By taking different values of C and γ, we can explore the optimal combination of hyperparameters. However, this method can be time-consuming, especially when there are many hyperparameters that need to search. Besides, we set the value of C and γ above in the condition that we get the optimal hyperparameters through GA. If the range of values we set manually is unreasonable or the interval between different values is too large, it will be challenging to find the optimal value. By contrast, GA can search the optimal parameters automatically.
In this paper, we propose a method that combines the feature selection based on MARL and parameter optimization based on GA to improve the early diagnosis of NPSLE. The data obtained by multivoxel proton magnetic resonance spectroscopy is high dimensional, sample-limited, and non-linear. It is hard to meet the model's requirements through traditional modeling methods or linear dimensionality reduction. SVM is an ideal classifier for our study, which has an advantage at dealing with small samples [46,47] and high-dimensional datasets. Even if a limited training set produces some bias, the model still has good generalization if we select appropriate parameters [48]. Besides, since the original data contains a lot of redundant information, it is essential to select the proper subset of features to avoid reducing the classifier's performance and increasing the computation quantity.
The proposed approach results were compared with Bagging, Decision Tree, AdaBoost and SVM without optimization to determine GA's ability of optimizing SVM hyperparameters. Experimental results show that the GA-SVM is significantly better than those classifiers just mentioned, achieving satisfactory sensitivity, specificity, and accuracy as 91.3%, 93.8% and 92.3%, respectively, while the SVM with default parameters optimization only achieves 73.9% sensitivity, 75.0% specificity and 74.4% accuracy. We select the optimal feature subset of the data through MARL and optimize SVM by GA. More remarkably, this method is compared with other dimension reduction ones, such as PCA and the encoder of the VAE. Specifically, the SVM optimized by GA and MARL reached the 100% specificity, 91.3% sensitivity, 94.9% accuracy and 0.870 cross-validation score, achieving the best performance. In summary, the proposed method can improve the NPSLE diagnosis efficiently. In the future, we plan to apply this method to practical medical diagnosis. In the application scenario, we will evaluate the risk of information systems using this technology to prevent some incidents and ensure patient safety [49]. Since this technology involves critical infrastructure (healthcare), it is necessary to be regulated.
However, our study still has some limits. On the one hand, further studies and comparisons are needed in patients with HCs, non-NPSLE and NPSLE. Previous studies have found that the cerebral perfusion patterns of NPSLE and non NPSLE patients are different [50]. Besides, NPSLE is significantly associated with CII and depression, fatigue and pain [51], while non-NPSLE patients and controls don't have it. On the other hand, we do not have sufficient data. We know the importance of abundant labeled data for obtaining good fitting of classifier models [52], but the data collection process is complex, and the patients are limited. To address this issue, we use stratified sampling to minimize sampling error and improve the model's generalization ability. By contrast, K-fold cross-validation does not consider the problem of label distribution. Furthermore, to effectively use limited data and overcome overfitting, we use the stratified sampling strategy five times so that the evaluation results can be as close as possible to the model's performance on the test set. The results show that the proposed model can perform well on the unknown data.
In conclusion, our model can perform well in the condition of limited samples with missing values of metabolic features, proving its robustness. Therefore, the proposed algorithm provides a new perspective for early diagnosis and intervention for the NPSLE and reduces the deviation caused by manual screening with more space for its potential development. For the next step, we prepare to collect more samples and related data, including clinical case data and its corresponding medical images, to develop a multimodal machine learning model.
This work was supported by the NSFC (No. 61902232), the 2022 Guangdong Basic and Applied Basic Research Foundation grant to Dr. Teng Zhou, the STU Incubation Project for the Research of Digital Humanities and New Liberal Arts (No. 2021DH-3), the STU Scientific Research Foundation for Talents (No. NTF18006), the 2020 Li Ka Shing Foundation Cross-Disciplinary Research Grant (No. 2020LKSFG05D), and the Education Science Planning Project of Guangdong Province (No. 2018GXJK048).
The authors declare that they have no conflict of interest.
[1] |
M. Aringer, S. R. Johnson, Classifying and diagnosing systemic lupus erythematosus in the 21st century, Rheumatology, 59 (2020), v4–v11. http://doi.org/10.1093/rheumatology/keaa379 doi: 10.1093/rheumatology/keaa379
![]() |
[2] |
G. Ruiz-Irastorza, G. Bertsias, Treating systemic lupus erythematosus in the 21st century: new drugs and new perspectives on old drugs, Rheumatology, 59 (2020), v69–v81. http://doi.org/10.1093/rheumatology/keaa403 doi: 10.1093/rheumatology/keaa403
![]() |
[3] |
P. M. van der Meulen, A. M. Barendregt, E. Cuadrado, C. Magro-Checa, G. M. Steup-Beekman, D. Schonenberg-Meinema, et al., Protein array autoantibody profiles to determine diagnostic markers for neuropsychiatric systemic lupus erythematosus, Rheumatology, 56 (2017), 1407–1416. http://doi.org/10.1093/rheumatology/kex073 doi: 10.1093/rheumatology/kex073
![]() |
[4] |
M. E. Kathleen, A. Janice, H. Margaret, B. Jane, L. Peter, R. Anisur, et al., Flares in patients with systemic lupus erythematosus, Rheumatology, 60 (2021), 3262–3267. http://doi.org/10.1093/rheumatology/keaa777 doi: 10.1093/rheumatology/keaa777
![]() |
[5] |
A. Kernder, E. Elefante, G. Chehab, C. Tani, M. Mosca, M. Schneider, The patient's perspective: are quality of life and disease burden a possible treatment target in systemic lupus erythematosus?, Rheumatology, 59 (2020), v63–v68. http://doi.org/10.1093/rheumatology/keaa427 doi: 10.1093/rheumatology/keaa427
![]() |
[6] |
M. Bruschi, G. Moroni, R. A. Sinico, F. Franceschini, M. Fredi, A. Vaglio, et al., Serum igg2 antibody multi-composition in systemic lupus erythematosus and in lupus nephritis (part 2): prospective study, Rheumatology, 60 (2021), 3388–3397. http://doi.org/10.1093/rheumatology/keaa793 doi: 10.1093/rheumatology/keaa793
![]() |
[7] |
L. Arnaud, M. G. Tektonidou, Long-term outcomes in systemic lupus erythematosus: trends over time and major contributors, Rheumatology, 59 (2020), 29–38. http://doi.org/10.1093/rheumatology/keaa382 doi: 10.1093/rheumatology/keaa382
![]() |
[8] |
N. Sarbu, F. Alobeidi, P. Toledano, G. Espinosa, I. Giles, A. Rahman, et al., Brain abnormalities in newly diagnosed neuropsychiatric lupus: systematic mri approach and correlation with clinical and laboratory data in a large multicenter cohort, Autoimmun. Rev., 14 (2015), 153–159. http://doi.org/10.1016/j.autrev.2014.11.001 doi: 10.1016/j.autrev.2014.11.001
![]() |
[9] |
J. A. Mikdashi, Altered functional neuronal activity in neuropsychiatric lupus: a systematic review of the fmri investigations, Semin. Arthritis Rheum., 45 (2016), 455–462. http://doi.org/10.1016/j.semarthrit.2015.08.002 doi: 10.1016/j.semarthrit.2015.08.002
![]() |
[10] |
M. Govoni, J. G. Hanly, The management of neuropsychiatric lupus in the 21st century: still so many unmet needs?, Rheumatology, 59 (2020), v52–v62. http://doi.org/10.1093/rheumatology/keaa404 doi: 10.1093/rheumatology/keaa404
![]() |
[11] |
M. H. Liang, M. Corzillius, S. C. Bae, R. A. Lew, P. R. Fortin, C. Gordon, et al., The american college of rheumatology nomenclature and case definitions for neuropsychiatric lupus syndromes, Arthritis Rheum., 42 (1999), 599–608. http://doi.org/10.1002/1529-0131(199904)42:4 < 599::AID-ANR2 > 3.0.CO; 2-F doi: 10.1002/1529-0131(199904)42:4 < 599::AID-ANR2 > 3.0.CO; 2-F
![]() |
[12] |
E. Moore, M. W. Huang, C. Putterman, Advances in the diagnosis, pathogenesis and treatment of neuropsychiatric systemic lupus erythematosus, Curr. Opin. Rheumatol., 32 (2020), 152–158. http://doi.org/10.1097/BOR.0000000000000682 doi: 10.1097/BOR.0000000000000682
![]() |
[13] |
H. Jeltsch-David, S. Muller, Neuropsychiatric systemic lupus erythematosus: pathogenesis and biomarkers, Nat. Rev. Neurol., 10 (2014), 579–596. http://doi.org/10.1038/nrneurol.2014.148 doi: 10.1038/nrneurol.2014.148
![]() |
[14] |
C. Magro-Checa, E. J. Zirkzee, L. J. Beaart-van de Voorde, H. A. Middelkoop, N. J. van der Wee, M. V. Huisman, et al., Value of multidisciplinary reassessment in attribution of neuropsychiatric events to systemic lupus erythematosus: prospective data from the leiden npsle cohort, Rheumatology, 56 (2017), 1676–1683. http://doi.org/10.1093/rheumatology/kex019 doi: 10.1093/rheumatology/kex019
![]() |
[15] |
A. N. Culshaw, D. B. Roychowdhury, P80 value of the clinical nurse specialist role in the care of patients with systemic lupus erythematosus: the patient experience, Rheumatology, 59 (2020), keaa111.078. http://doi.org/10.1093/rheumatology/keaa111.078 doi: 10.1093/rheumatology/keaa111.078
![]() |
[16] |
Y. Cheng, A. Cheng, Y. Jia, L. Yang, Y. Ning, L. Xu, et al., ph-responsive multifunctional theranostic rapamycin-loaded nanoparticles for imaging and treatment of acute ischemic stroke, ACS Appl. Mater. Interfaces, 13 (2021), 56909–56922. http://doi.org/10.1021/acsami.1c16530 doi: 10.1021/acsami.1c16530
![]() |
[17] |
J. Luyendijk, S. Steens, W. Ouwendijk, G. Steup-Beekman, E. Bollen, J. Van Der Grond, et al., Neuropsychiatric systemic lupus erythematosus: lessons learned from magnetic resonance imaging, Arthritis Rheum., 63 (2011), 722–732. http://doi.org/10.1002/art.30157 doi: 10.1002/art.30157
![]() |
[18] |
H. Lu, Z. Ge, Y. Song, D. Jiang, T. Zhou, J. Qin, A temporal-aware lstm enhanced by loss-switch mechanism for traffic flow forecasting, Neurocomputing, 427 (2021), 169–178. http://doi.org/10.1016/j.neucom.2020.11.026 doi: 10.1016/j.neucom.2020.11.026
![]() |
[19] |
H. Lu, D. Huang, S. Youyi, D. Jiang, T. Zhou, J. Qin, St-trafficnet: A spatial-temporal deep learning network for traffic forecasting, Electronics, 9 (2020), 1–17. http://doi.org/10.3390/electronics9091474 doi: 10.3390/electronics9091474
![]() |
[20] | Y. Song, Z. Yu, T. Zhou, J. Y. C. Teoh, B. Lei, C. Kup-Sze, et al., Cnn in ct image segmentation: Beyond loss function for exploiting ground truth images, in 2020 IEEE International Symposium on Biomedical Imaging (ISBI), (2020), 325–328. http://doi.org/10.1109/ISBI45749.2020.9098488 |
[21] |
T. Zhou, G. Han, B. N. Li, Z. Lin, E. J. Ciaccio, P. H. Green, et al., Quantitative analysis of patients with celiac disease by video capsule endoscopy: A deep learning method, Comput. Biol. Med., 85 (2017), 1–6. http://doi.org/10.1016/j.compbiomed.2017.03.031 doi: 10.1016/j.compbiomed.2017.03.031
![]() |
[22] |
G. Tu, J. Wen, H. Liu, S. Chen, L. Zheng, D. Jiang, Exploration meets exploitation: Multitask learning for emotion recognition based on discrete and dimensional models, Knowl. Based Syst., 235 (2022), 107598. http://doi.org/10.1016/j.knosys.2021.107598 doi: 10.1016/j.knosys.2021.107598
![]() |
[23] |
W. Fang, W. Zhuo, J. Yan, Y. Song, D. Jiang, T. Zhou, Attention meets long short-term memory: A deep learning network for traffic flow forecasting, Phys. A, 587 (2022), 126485. http://doi.org/10.1016/j.physa.2021.126485 doi: 10.1016/j.physa.2021.126485
![]() |
[24] | Y. LeCun, Y. Bengio, G. Hinton, Deep learning, Nature, 521 (2015), 436–444. http://doi.org/10.1038/nature14539 |
[25] | C. Cortes, V. Vapnik, Support-vector networks, Mach. Learn., 20 (1995), 273–297. http://doi.org/10.1007/BF00994018 |
[26] |
L. Zhu, P. Spachos, Support vector machine and yolo for a mobile food grading system, Internet Things, 13 (2021), 100359. http://doi.org/10.1016/j.iot.2021.100359 doi: 10.1016/j.iot.2021.100359
![]() |
[27] |
W. Cai, D. Yu, Z. Wu, X. Du, T. Zhou, A hybrid ensemble learning framework for basketball outcomes prediction, Phys. A, 528 (2019), 1–8. http://doi.org/10.1016/j.physa.2019.121461 doi: 10.1016/j.physa.2019.121461
![]() |
[28] | G. Tan, S. Zheng, B. Huang, Z. Cui, H. Dou, X. Yang, et al., Hybrid ga-svr: An effective way to predict short-term traffic flow, in 21st International Conference on Algorithms and Architectures for Parallel Processing (ICA3PP 2021), (2021), 1–11. |
[29] |
G. N. Kouziokas, Svm kernel based on particle swarm optimized vector and bayesian optimized svm in atmospheric particulate matter forecasting, Appl. Soft Comput., 93 (2020), 106410. http://doi.org/10.1016/j.asoc.2020.106410 doi: 10.1016/j.asoc.2020.106410
![]() |
[30] |
W. Cai, J. Yang, Y. Yu, Y. Song, T. Zhou, J. Qin, Pso-elm: A hybrid learning model for short-term traffic flow forecasting, IEEE Access, 8 (2020), 6505–6514. http://doi.org/10.1109/ACCESS.2019.2963784 doi: 10.1109/ACCESS.2019.2963784
![]() |
[31] |
H. Faris, M. A. Hassonah, A. Z. Ala'M, S. Mirjalili, I. Aljarah, A multi-verse optimizer approach for feature selection and optimizing svm parameters based on a robust system architecture, Neural Comput. Appl., 30 (2018), 2355–2369. http://doi.org/10.1007/s00521-016-2818-2 doi: 10.1007/s00521-016-2818-2
![]() |
[32] | B. K. Petersen, J. Yang, W. S. Grathwohl, C. Cockrell, C. Santiago, G. An, et al., Precision medicine as a control problem: Using simulation and deep reinforcement learning to discover adaptive, personalized multi-cytokine therapy for sepsis, preprint, arXiv: 1802.10440. |
[33] |
C. Yu, Y. Dong, J. Liu, G. Ren, Incorporating causal factors into reinforcement learning for dynamic treatment regimes in hiv, BMC Med. Inf. Decis. Making, 19 (2019), 19–29. http://doi.org/10.1186/s12911-019-0755-6 doi: 10.1186/s12911-019-0755-6
![]() |
[34] | G. Maicas, G. Carneiro, A. P. Bradley, J. C. Nascimento, I. Reid, Deep reinforcement learning for active breast lesion detection from dce-mri, in International conference on medical image computing and computer-assisted intervention, (2017), 665–673. |
[35] | H. Dou, J. Ji, H. Wei, F. Wang, J. Wang, T. Zhou, Transfer inhibitory potency prediction to binary classification: A model only needs a small training set, Comput. Methods Programs Biomed.. |
[36] | K. Liu, Y. Fu, P. Wang, L. Wu, R. Bo, X. Li, Automating feature subspace exploration via multi-agent reinforcement learning, in Proceedings of the 25th ACM SIGKDD International Conference on Knowledge Discovery and Data Mining, (2019), 207–215. http://doi.org/10.1145/3292500.3330868 |
[37] |
Z. Tao, L. Huiling, W. Wenwen, Y. Xia, Ga-svm based feature selection and parameter optimization in hospitalization expense modeling, Appl. Soft Comput., 75 (2019), 323–332. http://doi.org/10.1016/j.asoc.2018.11.001 doi: 10.1016/j.asoc.2018.11.001
![]() |
[38] |
C. Sukawattanavijit, J. Chen, H. Zhang, Ga-svm algorithm for improving land-cover classification using sar and optical remote sensing data, IEEE Geosci. Remote Sens. Lett., 14 (2017), 284–288. http://doi.org/10.1109/LGRS.2016.2628406 doi: 10.1109/LGRS.2016.2628406
![]() |
[39] |
C. Meng, Y. Hu, Y. Zhang, F. Guo, Psbp-svm: A machine learning-based computational identifier for predicting polystyrene binding peptides, Frontiers in bioengineering and biotechnology, 8 (2020), 245. http://doi.org/10.3389/fbioe.2020.00245 doi: 10.3389/fbioe.2020.00245
![]() |
[40] |
L. Cai, Q. Chen, W. Cai, X. Xu, T. Zhou, J. Qin, Svrgsa: a hybrid learning based model for short-term traffic flow forecasting, IET Intell. Transp. Syst., 13 (2019), 1348–1355. http://doi.org/10.1049/iet-its.2018.5315 doi: 10.1049/iet-its.2018.5315
![]() |
[41] |
S. Katoch, S. S. Chauhan, V. Kumar, A review on genetic algorithm: past, present, and future, Multimedia Tools Appl., 80 (2021), 8091–8126. http://doi.org/10.1007/s11042-020-10139-6 doi: 10.1007/s11042-020-10139-6
![]() |
[42] |
M. A. Ehyaei, A. Ahmadi, M. A. Rosen, A. Davarpanah, Thermodynamic optimization of a geothermal power plant with a genetic algorithm in two stages, Processes, 8 (2020), 1277. http://doi.org/10.3390/pr8101277 doi: 10.3390/pr8101277
![]() |
[43] | R. S. Sutton, A. G. Barto, Reinforcement learning: An introduction, MIT press, (2018), http://doi.org/10.1109/TNN.1998.712192 |
[44] |
V. François-Lavet, P. Henderson, R. Islam, M. G. Bellemare, J. Pineau, An introduction to deep reinforcement learning, Found. Trends Mach. Learn., 11 (2018), 219–354. http://doi.org/10.1561/2200000071 doi: 10.1561/2200000071
![]() |
[45] | S. Gronauer, K. Diepold, Multi-agent deep reinforcement learning: a survey, Artif. Intell. Rev., 1–49. http://doi.org/10.1007/s10462-021-09996-w |
[46] |
Z. Yin, J. Hou, Recent advances on svm based fault diagnosis and process monitoring in complicated industrial processes, Neurocomputing, 174 (2016), 643–650. http://doi.org/10.1016/j.neucom.2015.09.081 doi: 10.1016/j.neucom.2015.09.081
![]() |
[47] |
Z. Liu, L. Wang, Y. Zhang, C. P. Chen, A svm controller for the stable walking of biped robots based on small sample sizes, Appl. Soft Comput., 38 (2016), 738–753. http://doi.org/10.1016/j.asoc.2015.10.029 doi: 10.1016/j.asoc.2015.10.029
![]() |
[48] |
S. M. Erfani, S. Rajasegarar, S. Karunasekera, C. Leckie, High-dimensional and large-scale anomaly detection using a linear one-class svm with deep learning, Pattern Recognit., 58 (2016), 121–134. http://doi.org/10.1016/j.patcog.2016.03.028 doi: 10.1016/j.patcog.2016.03.028
![]() |
[49] | A. Coronato, A. Cuzzocrea, An innovative risk assessment methodology for medical information systems, IEEE Trans. Knowl. Data Eng., (2020). http://doi.org/10.1109/TKDE.2020.3023553 |
[50] |
Z. Zhuo, L. Su, Y. Duan, J. Huang, X. Qiu, S. Haller, et al., Different patterns of cerebral perfusion in sle patients with and without neuropsychiatric manifestations, Hum. brain Mapp., 41 (2020), 755–766. http://doi.org/10.1002/hbm.24837 doi: 10.1002/hbm.24837
![]() |
[51] |
E. Kozora, M. C. Ellison, S. West, Depression, fatigue, and pain in systemic lupus erythematosus (sle): relationship to the american college of rheumatology sle neuropsychological battery, Arthritis Rheum., 55 (2006), 628–635. http://doi.org/10.1002/art.22101 doi: 10.1002/art.22101
![]() |
[52] | A. Anaby-Tavor, B. Carmeli, E. Goldbraich, A. Kantor, G. Kour, S. Shlomov, et al., Do not have enough data? deep learning to the rescue!, in Proceedings of the AAAI Conference on Artificial Intelligence, 34 (2020), 7383–7390. http://doi.org/10.1609/aaai.v34i05.6233 |
1. | Zhaoshun Jiang, Yuxi Cai, Songbin Liu, Pei Ye, Yifeng Yang, Guangwu Lin, Shihong Li, Yan Xu, Yangjing Zheng, Zhijun Bao, Shengdong Nie, Weidong Gu, Decreased default mode network functional connectivity with visual processing regions as potential biomarkers for delayed neurocognitive recovery: A resting-state fMRI study and machine-learning analysis, 2023, 14, 1663-4365, 10.3389/fnagi.2022.1109485 | |
2. | Katherine Nay Yaung, Joo Guan Yeo, Pavanish Kumar, Martin Wasser, Marvin Chew, Angelo Ravelli, Annie Hui Nee Law, Thaschawee Arkachaisri, Alberto Martini, David S Pisetsky, Salvatore Albani, Artificial intelligence and high-dimensional technologies in the theragnosis of systemic lupus erythematosus, 2023, 5, 26659913, e151, 10.1016/S2665-9913(23)00010-3 | |
3. | Ye Yuan, Tianhong Quan, Youyi Song, Jitian Guan, Teng Zhou, Renhua Wu, Noise-Immune Extreme Ensemble Learning for Early Diagnosis of Neuropsychiatric Systemic Lupus Erythematosus, 2022, 26, 2168-2194, 3495, 10.1109/JBHI.2022.3164937 | |
4. | Fulvia Ceccarelli, Francesco Natalucci, Licia Picciariello, Claudia Ciancarella, Giulio Dolcini, Angelica Gattamelata, Cristiano Alessandri, Fabrizio Conti, Application of Machine Learning Models in Systemic Lupus Erythematosus, 2023, 24, 1422-0067, 4514, 10.3390/ijms24054514 | |
5. | Yuan Zhou, Meng Wang, Shasha Zhao, Yan Yan, Muhammad Ahmad, Machine Learning for Diagnosis of Systemic Lupus Erythematosus: A Systematic Review and Meta-Analysis, 2022, 2022, 1687-5273, 1, 10.1155/2022/7167066 | |
6. | Guanru Tan, Teng Zhou, Boyu Huang, Haowen Dou, Youyi Song, Zhizhe Lin, A noise-immune and attention-based multi-modal framework for short-term traffic flow forecasting, 2024, 28, 1432-7643, 4775, 10.1007/s00500-023-09173-x | |
7. | Zienab Shahada, Naram Khalalyli, Leen Allahham, Maysoun Kudsi, Acute psychosis as the presenting manifestation of lupus: case reports, 2023, 6, 2576-3342, 10.1097/GH9.0000000000000384 | |
8. | Kevin Zhan, Katherine A Buhler, Irene Y Chen, Marvin J Fritzler, May Y Choi, Systemic lupus in the era of machine learning medicine, 2024, 11, 2053-8790, e001140, 10.1136/lupus-2023-001140 | |
9. | Maria Giovanna Danieli, Silvia Brunetto, Luca Gammeri, Davide Palmeri, Ilaria Claudi, Yehuda Shoenfeld, Sebastiano Gangemi, Machine learning application in autoimmune diseases: State of art and future prospectives, 2024, 23, 15689972, 103496, 10.1016/j.autrev.2023.103496 | |
10. | M. J. W. Prior, 2022, 978-1-83916-706-5, 271, 10.1039/9781839167690-00271 |
Algorithm 1: The pseudocode for feature selection based on MARL. |
Data: threshold ϵ, ϵ decay rate rϵ, upper bound of the feature subset b, discount coefficient γ, max iteration Imax, raw dataset D=(Dij)N×M
Result: feature subset F 1 Iteration I=0 2 while I≤Imax do ![]() 25 end 26 return F; |
Brain region | Metabolite |
LDT | Cr |
LDT | PCr |
LDT | NAA |
LDT | Ins/Cr + PCr |
LDT | GPC + PCh |
LDT | GPC + PCh/Cr + PCr |
RDT | Ins |
LPCG | Cr |
LPCG | PCr |
LPCG | NAA + NAAG |
LPCG | Ins/Cr + PCr |
RPCG | Cr |
RPCG | PCr |
RPCG | GPC + PCh |
RPCG | Glu + Gln/Cr + PCr |
RPCG | NAA + NAAG/Cr + PCr |
LLN | Ins |
LLN | NAA + NAAG/Cr + PCr |
RLN | NAAG |
RLN | GPC + PCh |
LFWM | Ins |
LFWM | Cr + PCr |
LFWM | Ins/Cr + PCr |
RFWM | NAAG |
RFWM | NAA + NAAG |
RFWM | NAA + NAAG/Cr + PCr |
RI | Glu + Gln/Cr + PCr |
GA Parameters | Setting |
C | 2−7 |
γ | 29 |
Generations Number | 100 |
Population Size | 40 |
Selection Type | Wheel-roulette |
Crossover Type | Two-point Crossover |
Crossover Rate | 0.7 |
Mutation Rate | 0.02 |
Measure | Formula |
Sensitivity | Sensitivity=TPTP+FN |
Specificity | Specificity=TNTN+FP |
Accuracy | Accuracy=TP+TNTP+TN+FP+FN |
Algorithms | Sensitivity | Specificity | Accuracy | Cross-validation score |
Bagging | 69.6% | 43.8% | 59.0% | 0.587 |
Decision Tree | 73.9% | 50.0% | 64.1% | 0.643 |
SVM | 73.9% | 75.0% | 74.4% | 0.754 |
AdaBoost | 87.0% | 56.3% | 74.4% | 0.761 |
PCA+AdaBoost | 78.3% | 75% | 76.9% | 0.763 |
VAE+SVM | 82.6% | 75% | 79.5% | 0.798 |
PCA+Decision Tree | 82.6% | 81.3% | 82.1% | 0.827 |
PCA+Bagging | 87.0% | 81.3% | 84.6% | 0.849 |
PCA+SVM | 78.3% | 93.8% | 84.6% | 0.852 |
GA+SVM | 91.3% | 93.8% | 92.3% | 0.864 |
MARL+GA+SVM | 91.3% | 100.0% | 94.9% | 0.870 |
C | γ | Sensitivity | Specificity | Accuracy | Cross-validation score |
240.30 | 0.0080 | 82.6% | 81.3% | 82.1% | 0.827 |
0.0078 | 87.0% | 81.3% | 87.2% | 0.852 | |
0.0080 | 91.3% | 87.5% | 89.7% | 0.859 | |
240.37 | 0.0075 | 91.3% | 93.8% | 92.3% | 0.864 |
0.0078 | 91.3% | 100.0% | 94.9% | 0.870 | |
0.0075 | 87.0% | 93.8% | 92.3% | 0.862 | |
240.40 | 0.0075 | 87.0% | 93.8% | 89.7% | 0.859 |
0.0078 | 82.6% | 93.8% | 87.2% | 0.854 | |
0.0080 | 82.6% | 87.5% | 84.6% | 0.850 |
Algorithm 1: The pseudocode for feature selection based on MARL. |
Data: threshold ϵ, ϵ decay rate rϵ, upper bound of the feature subset b, discount coefficient γ, max iteration Imax, raw dataset D=(Dij)N×M
Result: feature subset F 1 Iteration I=0 2 while I≤Imax do ![]() 25 end 26 return F; |
Brain region | Metabolite |
LDT | Cr |
LDT | PCr |
LDT | NAA |
LDT | Ins/Cr + PCr |
LDT | GPC + PCh |
LDT | GPC + PCh/Cr + PCr |
RDT | Ins |
LPCG | Cr |
LPCG | PCr |
LPCG | NAA + NAAG |
LPCG | Ins/Cr + PCr |
RPCG | Cr |
RPCG | PCr |
RPCG | GPC + PCh |
RPCG | Glu + Gln/Cr + PCr |
RPCG | NAA + NAAG/Cr + PCr |
LLN | Ins |
LLN | NAA + NAAG/Cr + PCr |
RLN | NAAG |
RLN | GPC + PCh |
LFWM | Ins |
LFWM | Cr + PCr |
LFWM | Ins/Cr + PCr |
RFWM | NAAG |
RFWM | NAA + NAAG |
RFWM | NAA + NAAG/Cr + PCr |
RI | Glu + Gln/Cr + PCr |
GA Parameters | Setting |
C | 2−7 |
γ | 29 |
Generations Number | 100 |
Population Size | 40 |
Selection Type | Wheel-roulette |
Crossover Type | Two-point Crossover |
Crossover Rate | 0.7 |
Mutation Rate | 0.02 |
Measure | Formula |
Sensitivity | Sensitivity=TPTP+FN |
Specificity | Specificity=TNTN+FP |
Accuracy | Accuracy=TP+TNTP+TN+FP+FN |
Algorithms | Sensitivity | Specificity | Accuracy | Cross-validation score |
Bagging | 69.6% | 43.8% | 59.0% | 0.587 |
Decision Tree | 73.9% | 50.0% | 64.1% | 0.643 |
SVM | 73.9% | 75.0% | 74.4% | 0.754 |
AdaBoost | 87.0% | 56.3% | 74.4% | 0.761 |
PCA+AdaBoost | 78.3% | 75% | 76.9% | 0.763 |
VAE+SVM | 82.6% | 75% | 79.5% | 0.798 |
PCA+Decision Tree | 82.6% | 81.3% | 82.1% | 0.827 |
PCA+Bagging | 87.0% | 81.3% | 84.6% | 0.849 |
PCA+SVM | 78.3% | 93.8% | 84.6% | 0.852 |
GA+SVM | 91.3% | 93.8% | 92.3% | 0.864 |
MARL+GA+SVM | 91.3% | 100.0% | 94.9% | 0.870 |
C | γ | Sensitivity | Specificity | Accuracy | Cross-validation score |
240.30 | 0.0080 | 82.6% | 81.3% | 82.1% | 0.827 |
0.0078 | 87.0% | 81.3% | 87.2% | 0.852 | |
0.0080 | 91.3% | 87.5% | 89.7% | 0.859 | |
240.37 | 0.0075 | 91.3% | 93.8% | 92.3% | 0.864 |
0.0078 | 91.3% | 100.0% | 94.9% | 0.870 | |
0.0075 | 87.0% | 93.8% | 92.3% | 0.862 | |
240.40 | 0.0075 | 87.0% | 93.8% | 89.7% | 0.859 |
0.0078 | 82.6% | 93.8% | 87.2% | 0.854 | |
0.0080 | 82.6% | 87.5% | 84.6% | 0.850 |