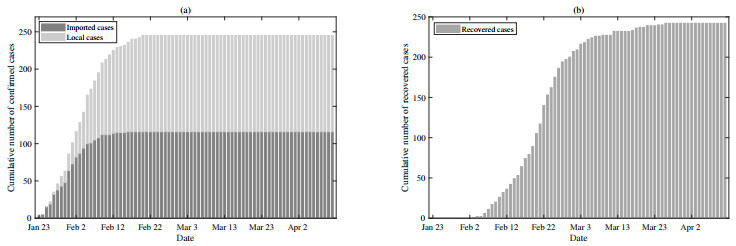
The large-scale infection of COVID-19 has led to a significant impact on lives and economies around the world and has had considerable impact on global public health. Social distancing, mask wearing and contact tracing have contributed to containing or at least mitigating the outbreak, but how public awareness influences the effectiveness and efficiency of such approaches remains unclear. In this study, we developed a discrete compartment dynamic model to mimic and explore how media reporting and the strengthening containment strategies can help curb the spread of COVID-19 using Shaanxi Province, China, as a case study. The targeted model is parameterized based on multi-source data, including the cumulative number of confirmed cases, recovered individuals, the daily number of media-reporting items and the imported cases from the rest of China outside Shaanxi from January 23 to April 11, 2020. We carried out a sensitivity analysis to investigate the effect of media reporting and imported cases on transmission. The results revealed that reducing the intensity of media reporting, which would result in a significant increasing of the contact rate and a sizable decreasing of the contact-tracing rate, could aggravate the outbreak severity by increasing the cumulative number of confirmed cases. It also demonstrated that diminishing the imported cases could alleviate the outbreak severity by reducing the length of the epidemic and the final size of the confirmed cases; conversely, delaying implementation of lockdown strategies could prolong the length of the epidemic and magnify the final size. These findings suggest that strengthening media coverage and timely implementing of lockdown measures can significantly reduce infection.
Citation: Jin Guo, Aili Wang, Weike Zhou, Yinjiao Gong, Stacey R. Smith?. Discrete epidemic modelling of COVID-19 transmission in Shaanxi Province with media reporting and imported cases[J]. Mathematical Biosciences and Engineering, 2022, 19(2): 1388-1410. doi: 10.3934/mbe.2022064
[1] | Weike Zhou, Aili Wang, Fan Xia, Yanni Xiao, Sanyi Tang . Effects of media reporting on mitigating spread of COVID-19 in the early phase of the outbreak. Mathematical Biosciences and Engineering, 2020, 17(3): 2693-2707. doi: 10.3934/mbe.2020147 |
[2] | Xiaojing Wang, Yu Liang, Jiahui Li, Maoxing Liu . Modeling COVID-19 transmission dynamics incorporating media coverage and vaccination. Mathematical Biosciences and Engineering, 2023, 20(6): 10392-10403. doi: 10.3934/mbe.2023456 |
[3] | Xinghua Chang, Maoxing Liu, Zhen Jin, Jianrong Wang . Studying on the impact of media coverage on the spread of COVID-19 in Hubei Province, China. Mathematical Biosciences and Engineering, 2020, 17(4): 3147-3159. doi: 10.3934/mbe.2020178 |
[4] | Ming-Tao Li, Gui-Quan Sun, Juan Zhang, Yu Zhao, Xin Pei, Li Li, Yong Wang, Wen-Yi Zhang, Zi-Ke Zhang, Zhen Jin . Analysis of COVID-19 transmission in Shanxi Province with discrete time imported cases. Mathematical Biosciences and Engineering, 2020, 17(4): 3710-3720. doi: 10.3934/mbe.2020208 |
[5] | Hao Wang, Di Zhu, Shiqi Li, Robert A. Cheke, Sanyi Tang, Weike Zhou . Home quarantine or centralized quarantine? A mathematical modelling study on the COVID-19 epidemic in Guangzhou in 2021. Mathematical Biosciences and Engineering, 2022, 19(9): 9060-9078. doi: 10.3934/mbe.2022421 |
[6] | Tianfang Hou, Guijie Lan, Sanling Yuan, Tonghua Zhang . Threshold dynamics of a stochastic SIHR epidemic model of COVID-19 with general population-size dependent contact rate. Mathematical Biosciences and Engineering, 2022, 19(4): 4217-4236. doi: 10.3934/mbe.2022195 |
[7] | Yue Deng, Siming Xing, Meixia Zhu, Jinzhi Lei . Impact of insufficient detection in COVID-19 outbreaks. Mathematical Biosciences and Engineering, 2021, 18(6): 9727-9742. doi: 10.3934/mbe.2021476 |
[8] | Quentin Griette, Jacques Demongeot, Pierre Magal . What can we learn from COVID-19 data by using epidemic models with unidentified infectious cases?. Mathematical Biosciences and Engineering, 2022, 19(1): 537-594. doi: 10.3934/mbe.2022025 |
[9] | Sha He, Sanyi Tang, Libin Rong . A discrete stochastic model of the COVID-19 outbreak: Forecast and control. Mathematical Biosciences and Engineering, 2020, 17(4): 2792-2804. doi: 10.3934/mbe.2020153 |
[10] | H. Swapnarekha, Janmenjoy Nayak, H. S. Behera, Pandit Byomakesha Dash, Danilo Pelusi . An optimistic firefly algorithm-based deep learning approach for sentiment analysis of COVID-19 tweets. Mathematical Biosciences and Engineering, 2023, 20(2): 2382-2407. doi: 10.3934/mbe.2023112 |
The large-scale infection of COVID-19 has led to a significant impact on lives and economies around the world and has had considerable impact on global public health. Social distancing, mask wearing and contact tracing have contributed to containing or at least mitigating the outbreak, but how public awareness influences the effectiveness and efficiency of such approaches remains unclear. In this study, we developed a discrete compartment dynamic model to mimic and explore how media reporting and the strengthening containment strategies can help curb the spread of COVID-19 using Shaanxi Province, China, as a case study. The targeted model is parameterized based on multi-source data, including the cumulative number of confirmed cases, recovered individuals, the daily number of media-reporting items and the imported cases from the rest of China outside Shaanxi from January 23 to April 11, 2020. We carried out a sensitivity analysis to investigate the effect of media reporting and imported cases on transmission. The results revealed that reducing the intensity of media reporting, which would result in a significant increasing of the contact rate and a sizable decreasing of the contact-tracing rate, could aggravate the outbreak severity by increasing the cumulative number of confirmed cases. It also demonstrated that diminishing the imported cases could alleviate the outbreak severity by reducing the length of the epidemic and the final size of the confirmed cases; conversely, delaying implementation of lockdown strategies could prolong the length of the epidemic and magnify the final size. These findings suggest that strengthening media coverage and timely implementing of lockdown measures can significantly reduce infection.
After being first identified in Wuhan, China, COVID-19 has spread to almost every country in the world [1,2,3]. On March 11 2020, the World Health Organization (WHO) made the assessment that COVID-19 could be characterized as a pandemic due to the alarming levels of spread, severity and inaction [4]. As of August 13, 2021, there were 204,644,849 cases, with 4,323,139 deaths [1]. The rapid increase in confirmed cases and deaths, along with emerging variants of SARS-CoV-2 and multiple waves or new outbreaks, has put a very considerable strain on public-health organizations. Global public authorities have primarily relied upon non-pharmaceutical interventions, such as social distancing, frequent hand-washing, face masks and lockdowns, in order to curb or at least mitigate the severity of the ongoing pandemic.
Disseminating information from governments and public-health authorities is often done through local and national media, which have the potential to drastically affect public awareness and behaviour [5,6,78]. There is evidence that educated women (resp. men) are 4.69 (or 77.73) times more aware of AIDS than uneducated women (resp. men) in Bangladesh, while the probability of being aware of AIDS in those watching TV regularly is 8.6 times higher than those who never watch TV [9]. Media also plays a vital role in combating COVID-19 by raising public awareness and helping curb transmission [10,11].
For this study, we focus on Shaanxi Province, located to the northwest of China and adjacent to Hubei in the south. To avoid greater infection, many districts and countries, including China, have implemented unprecedented and stringent interventions, including lockdown of cities, strict tracing and quarantine of contacts. In the time since the first three confirmed COVID-19 cases were reported on January 23, 2020, in Shaanxi, a total of 245 confirmed cases were reported until February 20, 2020, of which 116 were imported from the rest of China outside Shaanxi [12]. Thus, examining the impact of imported cases as well as media reporting can cast light on the future direction of the epidemic.
A significant number of mathematical models have assessed the COVID-19 transmission risk, estimated the peak time and peak size, explored the time for ending lockdowns and evaluated the effectiveness and implementation of control measures [13,14,15,16,17,18,19,20,21,22,23,24,25,26,27,2829]. Tang et al. [13,14] used a deterministic compartmental model in terms of the clinical progression of the disease and effects of interventions to determine the transmission risk, basic reproduction number R0, peak time, peak size and final scale of the infection. Zhao et al. [15,16,17] employed statistical methods including maximum likelihood estimation to estimate the basic reproduction number. Gatto et al. [18,19,20] revealed the critical role of non-pharmaceutical interventions in alleviating the severity of the epidemic. Laxminarayan et al. [21,22,23] explored the transmission dynamics and highlighted the key factors affect the contact patterns. He et al. [24] investigated the relative transmissibility of asymptomatic COVID-19 infections. Wang et al. [25] used a network model to explore the impact of ending lockdown in Wuhan and 15 surrounding cities on the risk of secondary outbreak. A number of papers [26,27,28] have analyzed the epidemic in Wuhan to determine the implementation of containment strategies for the ongoing transmission of COVID-19. Li et al. [29] formulated a difference model to assess the impact of imported cases on the local epidemic. A handful of studies have modelled the effect of media on the containment of COVID-19 in Wuhan [6,11]. However, to the best of our knowledge, no studies have examined how media reporting and imported cases interact to affect the transmission of COVID-19 in Shaanxi.
Using the example of Shaanxi, we integrated control measures, imported cases and media reports into classic infectious disease models to characterize the spread of COVID-19. Our main goal is to assess the effect of the media reports, imported cases and the strengthening of control measures in containing or mitigating the spread of COVID-19. Specifically, we aim to identify the key parameters affecting the peak time and peak size of the outbreak. Our findings may provide useful for policymakers to combat COVID-19 through enhancing media reports and limiting imported cases.
We obtained data of COVID-19 cases in Shaanxi Province from January 23 2020 to April 11 2020 from the National Health Commission of the People's Republic of China and Health Commission of Shaanxi Province [12]. The data include the reported cumulative number of confirmed cases and the cumulative number of recovered cases, as shown in Figure 1. Figure 1(a) shows the cumulative number of local cases as well as the imported cases, and Figure 1(b) shows the cumulative number of recovered individuals. COVID-19 in Shaanxi led to 245 infected cases as of April 11, of which 116 cases were imported from the rest of China.
A total of 242 infected individuals had recovered by March 26, and 3 people died of the virus. It is worth noting that the strict contact-tracing strategy in Shaanxi Province provides more detailed information for most confirmed cases, including illness onset, the first medical visit, laboratory confirmation and discharge. The date of arriving in Shaanxi for imported cases was also released. All the data were released anonymously.
We thus had detailed information on the number of infecteds without hospital visits, the number of quarantined susceptibles, the number of hospitalized but not confirmed individuals, the number of hospitalized and confirmed individuals and the number of recovered cases on the day the infection was firstly reported in Shaanxi, January 23, 2020. These correspond to initial values of I,Sq,H,Hc and R in our model. We also obtained the number of exposed, symptomatic-infected, hospitalized and confirmed-infected populations amongst those who have been imported into Shaanxi every day from January 23 to April 11, 2020. We obtained detailed information of the imported cases among confirmed individuals from January 23 to April 11, including when they arrived in Shaanxi, when they were exposed to the virus, when they visited the hospital and when they are confirmed. This allowed us to extrapolate the daily numbers of imported exposed, imported infected, imported hospitalized and imported confirmed individuals from January 23 to April 11. It is worth noting that most of the confirmed cases in Shaanxi were imported cases at the beginning of the epidemic, so we assumed that all the confirmed individuals among those coming to Shaanxi were infected in elsewhere in China. We thus ignore imported susceptible individuals. We calculated the average latent period as 5.3889 days and the period from illness onset to hospitalization as 2.4299 days.
We also collected the daily number of media reports on COVID-19 from the four most popular or government websites of Shaanxi Province, including sanqin.com, sxdaily.com.cn, cnwest.com and xiancity.cn by searching the key words "novel coronavirus", as shown in Figure 2(a).
In particular, we counted the number of those links matching the words "novel coronavirus". (Note that very few media reports on these four websites ever referred to COVID-19 as "coronavirus" alone.) Let xi(i=1,2,3,4) be the daily number of media reports collected in each website, where xi represents sanqin.com, sxdaily.com, cnwest.com.cn and xiancity.cn, respectively. We defined the average daily number of media reports x as
x=Σ4i=1xi4. |
We can easily observe from Figure 2(b) that the average daily number of media reports correlates strongly with the number of daily confirmed cases. We analyzed the relationship between the average daily number of media reports and the number of daily confirmed cases from January 23 to April 11, 2020 by conducting a cross-correlation analysis [30].
Based on the clinical progression of the disease and epidemiological status of the individuals, we stratify the population into eight compartments: susceptible (St), exposed (Et), infected without hospital visit (It), hospitalized but not confirmed (Ht), hospitalized and confirmed (Hct), recovered (Rt), quarantined susceptible (Sqt) and quarantined exposed (Eqt). For simplicity, in the rest of this work, we call the infected without hospital visit (It) infected, the hospitalized but not confirmed (Ht) hospitalized, and the hospitalized and confirmed (Hct) confirmed. Note that none of the 245 confirmed individuals reported in Shaanxi Province were asymptomatic, so we do not consider the asymptomatic infections in our model. Moreover, on the first day of the outbreak in Shaanxi, the lockdown policy was carried out throughout China; since then, people became so concerned about the COVID-19 epidemic that even those with mild symptoms went to the hospital. Medical resources were abundant in Shaanxi then, so all infected individuals, including those with mild symptoms, were hospitalized for treatment as soon as possible. We incorporated media as a separate class (Mt) into our targeted model to quantify the impact of media on the transmission, as shown in Figure 3.
Note that infected individuals cannot be confirmed as soon as they visit a hospital, as there exists an interval between an infected individual visiting hospital and being confirmed. Therefore, we differentiate those being admitted into hospital and those being confirmed to provide a detailed view into disease-transmission pathways in Shaanxi, and have two different classes in our model: hospitalized (Ht) and confirmed cases (Hct). Since the case numbers in Shaanxi Province are relatively small, a discrete model is adopted to mimic the transmission of COVID-19. Based on the flowcharts shown in Figure 3, we established the following model:
St+1=St−(c(Mt)β+q(Mt)c(Mt)(1−β))StItNt+(1−e−λ)Sq,t,Et+1=Et+(1−q(Mt))c(Mt)βStItNt−(1−e−σ)Et+ξˉet,It+1=It+(1−e−σ)Et−(1−e−αe−δI)It+ξˉit,Sq,t+1=Sq,t+q(Mt)c(Mt)(1−β)StItNt−(1−e−λ)Sq,t,Eq,t+1=Eq,t+q(Mt)c(Mt)βStItNt−(1−e−σ)Eq,t,Ht+1=Ht+e−α(1−e−δI)It+(1−e−σ)Eq,t−(1−e−δH)Ht+ξˉht,Hc,t+1=Hc,t+(1−e−δH)Ht−(1−e−γHe−α)Hc,t+ξˉhc,t,Rt+1=Rt+e−α(1−e−γH)Hc,t,Mt+1=Mt+η(Ht+Hct)−(1−e−μM)Mt, | (2.1) |
where ˉet,ˉit,ˉht,ˉhc,t represent the imported exposed, infected, hospital visited, confirmed individuals from the rest of China outside Shaanxi. The time unit is days.
Transmission can occur once a susceptible person is in contact with an infected person, so we denote the transmission probability and contact rate by β and c, respectively. With contact tracing, we assume a proportion q of individuals exposed to the virus have been contact traced and quarantined, and they move to either quarantined exposed compartment (Eq) or quarantined susceptible compartment (Sq) at a rate of βcq or (1−β)cq, depending on whether they are infected or not. The remaining proportion 1−q of those in contact with infected people consists of individuals exposed to the virus and missed from contact tracing and quarantine; they move to the exposed compartment E at a rate of βc(1−q) if they are infected or stay in the compartment S if they aren't infected. The detailed definitions and values of variables and parameters are listed in Table 1.
Variables | Description | Initial value | Std | Resource | |
St | Susceptible population | 38640000 | – | Data | |
Et | Exposed population | 52 | 0.0193 | MCMC | |
It | Infected population | 26 | – | Data | |
Sqt | Quarantined susceptible population | 52 | – | Data | |
Eqt | Isolated exposed population | 0 | 0.1736 | MCMC | |
Ht | Hospitalized population | 36 | – | Data | |
Hct | Confirmed population | 3 | – | Data | |
Rt | Recovered population | 0 | – | Data | |
Mt | Media reports | 39.3 | – | Data | |
ˉet | imported exposed population | 4 | – | Data | |
ˉit | imported infected population | 2 | – | Data | |
ˉht | imported hospitalized population | 1 | – | Data | |
ˉhc,t | imported confirmed population | 0 | – | Data | |
Parameters | Definition | Mean value | Std | Resource | |
c(Mt) | c0 | Contact rate without media impact | 10.1163 | 0.0217 | MCMC |
c1 | Minimum contact rate with media impact | 3.1459 | 0.0328 | MCMC | |
μ1 | Modification factor | 0.8961 | 0.0091 | MCMC | |
β | Probability of transmission from I to S per contact | 0.1068 | 0.0041 | MCMC | |
q(Mt) | q0 | Quarantined rate of exposed individuals without media impact | 0.0280 | 0.0024 | MCMC |
q1 | Maximum quarantined rate of exposed individuals with media impact | 0.9259 | 0.0041 | MCMC | |
μ2 | Modification factor | 0.8189 | 0.0076 | MCMC | |
λ | Releasing rate of quarantined individuals | 1/14 | – | Data | |
σ | Progression rate of exposed individuals to infectives | 1/5.3889 | – | Data | |
α | Disease-induced death rate | 1.1715×10−3 | 2.9413×10−5 | MCMC | |
δI | Progression rate of infective class to hospitalized class | 1/2.4299 | – | Data | |
δH | Progression rate of hospitalized class to confirmed class | 0.4646 | 0.0053 | MCMC | |
γH1 | Recovery rate of hospitalized individuals | 0.0874 | 0.0051 | MCMC | |
η | Media-reported rate of number of new hospital notifications | 0.6487 | 0.0004 | MCMC | |
μM | Media waning rate | 0.9821 | 0.0092 | MCMC | |
ξ | Proportion of imported cases involved in the transmission of the epidemic | 1 | – | Data |
If individuals (denoted as x(t)) in one compartment move to another compartment with proportion a, then we have dx(t)dt=−ax(t), which yields x(t)=x(0)e−at. Letting t=1 time unit, it follows that individuals stay in their own compartment with probability e−a and move to the other compartment with the probability 1−e−a. In model (2.1), 1−e−λ represents the probability by which quarantined susceptible individuals (Sq) are released; 1−e−σ represents the probability by which exposed individuals (E) progress to the infected compartment (I) or quarantined exposed individuals (Eq) are admitted to hospital; 1−e−αe−δI represents the probability by which infecteds (I) are neither admitted to hospital nor die; e−α(1−e−δI) represents the probability by which infecteds (I) are admitted to hospital but don't die; 1−e−δH represents the probability by which those admitted to hospital (H) are confirmed; 1−e−γHe−α represents the probability by which confirmed individuals (Hc) neither recover nor die; e−α(1−e−γH) represents the probability by which confirmed individuals (Hc) recover but don't die; and 1−e−μM represents the probability by which the media (M) wanes due to ineffectiveness and other problems. The parameter ξ represents the proportion of imported cases involved in the spread of the epidemic in Shaanxi, which takes the value of 1 in this epidemic. We will explore the impact of the variance of the proportion of imported cases on the epidemic in Shaanxi.
From Figure 2, the daily number of confirmed cases and average daily number of media reports are in a strong relationship, so we adopt a function dependent on the total number of hospitalized individuals (Ht) and hospitalized and confirmed individuals (Hct) to describe the daily number of media reports in our modeling. In particular, we use η(Ht+Hct) to represent the newly reported items on day t, where η is the intensity of media reporting on new case numbers.
It is worth emphasizing that all parameters except those related to the incidence rates appear in exponential forms. This is because we can calculate some rate parameters from the detailed case records in Shaanxi Province, from which we can derive the corresponding probability directly. For instance, we calculated that the average incubation period during the COVID-19 outbreak in Shaanxi was 5.3889 days, so the progression rate of exposed individuals to infectives is 1/5.3889. Hence, it is convenient to represent the probability by which exposed individuals progress to the infected compartment as 1−e−1/5.3889. Parameters related to incidence rates could be calculated directly from the data, so they remain the same form as in the continuous model.
Since the first confirmed cases were reported in Shaanxi, media reporting and other prevention strategies have been strengthened continuously. These had a profound impact on public perception and caused obvious changes in public behaviour; for instance, they diminished contact rates by social distancing, going out less, wearing masks, etc. We assume the contact rate c(Mt) and quarantine rate q(Mt) are functions of media reporting. The contact rate c(Mt) is a decreasing function with respect to media reporting Mt, which takes the form
c(Mt)=c1+c0−c11+μ1Mt, | (2.2) |
where c0 is the contact rate without media impact, c1 is the minimum contact rate with media impact and μ1 is the modification of the contact rate due to media reporting. Thus c1<c0 and
c(0)=c0,limMt→∞c(Mt)=c1. |
Similarly, we assume the contact tracing and quarantine rate is an increasing function of media-reporting items Mt, which is
q(Mt)=q1−q1−q01+μ2Mt, | (2.3) |
where q0 is the contact tracing and quarantine rate of those susceptibles who have contacted with infecteds without media impact, q1 is the maximum contact tracing and quarantine rate of these individuals with media impact, μ2 is the modification of contact tracing and quarantine due to media reporting. Thus q1>q0 and
q(0)=q0,limMt→∞q(Mt)=q1. |
Since the first recovered case was reported on February 3, 2020 [12], as shown in Figure 1(b), we formulated the recovery rate γH by a piecewise function, which is 0 before February 3, 2020 and is a constant since February 4, 2020; i.e.,
γH(t)={0, before 3 Feb,γH1,after 3 Feb. |
In order to examine the relationship between the daily number of media reports on each website and the daily number of confirmed cases of COVID-19 in Shaanxi Province, we computed and plotted the cross-correlation function (CCF) between them, as shown in Figure 4. We can observe from Figure 4 that statistically significant cross-correlation occurs between the media reports of the websites sanqin.com (x1), sxdaily.com.cn (x2), cnwest.com (x3), xiancity.cn (x4) and the daily number of confirmed cases (y). In particular, the local maximal cross correlation coefficient occurs at the time lag of −2,−3,0,0 days for sanqin.com, sxdaily.com.cn, cnwest.com and xiancity.cn, respectively, which suggests that the media reports of sanqin.com (resp. sxdaily.com.cn) has the strongest correlation with the confirmed cases two (resp. three) days prior. We can also observe that the significant cross-correlation exists at lags ranging from −10 to 6 days, −10 to 10 days, −10 to 10 days and −10 to 10 days, respectively.
The average daily number of media reports is denoted by x, which is formulated in Section 2. We also conducted the cross-correlation analysis between x and y (the daily number of confirmed cases), which is summarized in Figure 5. The result shows a statistically significant cross correlation between x and y at lags from −10 to 10 days, which demonstrates a notable effect of the daily number of confirmed cases on the daily number of media reports.
We used data extracted from Shaanxi Provincial Health Commission of the People's Republic of China from Januaryv to April 11, 2020, to study the impact of imported cases and media reporting on COVID-19 transmission. In the initial stage of the COVID-19 epidemic in Shaanxi, almost everyone was susceptible to the virus, so we set S(0)=38,640,000 according to the population of Shaanxi [31]. Quarantined individuals are required to isolate for 14 days, so we set λ=1/14 and the probability by which quarantined susceptible individuals are released is then 1−e−114 [29,32]. Specifically, if the number of quarantined individuals is Nsq, whose release rate per day is λ, then, since all quarantined individuals can be released in 14 days, we easily get 14λNsq=Nsq, which yields λ=1/14. We computed the average incubation period 1/σ=5.3889 days, which fell within the period 3–7 days reported by Chinese Center for Disease Control and Prevention [33]. The average period from symptom onset to hospitalization for those infected was computed as 1/δI=2.4299 days. The recovery rate of the confirmed population was set to be γH1=0 before February 3.
We derived other unknown parameter values by fitting our targeted model (2.1) to the collected multi-source data, including the cumulative number of confirmed cases, cumulative number of recovered and average daily number of media reports from January 23 to April 11, 2020, using the Markov Chain Monte Carlo Method (MCMC) [34]. Consider the cumulative number of confirmed cases, cumulative number of recovered cases and average daily number of media reports on day t (i.e., Ct,Rt,Mt) subject to Poisson distributions with the corresponding real data on day t (i.e., ct,rt,mt). The statistic data is a Poisson process such that Ci and Cj, Ri and Rj, Mi and Mj(i≠j) are independent of each other. To perform the MCMC procedure, we adopted an adaptive Metropolis–Hastings (M–H) algorithm, which was run for 1,000,000 iterations with a burn-in of the first 5000 iterations. Let Θ be the parameter vector, which we estimate from the initial conditions and the multi-source data. A Markov chain of Θ, whose smooth distribution is a posterior distribution, was generated by the M–H algorithm according to a jump rule of Markov chain transfer. To this end, the random walk sampling is selected to update the parameter vector; i.e.,
Θ′=Θ+ω, |
where Θ represents the value of the parameter vector in the present state, Θ′ represents the randomly generated candidate vector of the parameters and ω is a random variable. The reception probability of the parameter vector is defined as
α=min{1,exp(Nlik−Olik)}, |
with Olik and Nlik being the log-likelihood function values calculated before and after the update of the parameter vector, respectively; i.e., they were calculated at Θ and Θ′. The log-likelihood function took the form
L(Θ)=n∑t=1[ctlnCt−Ct−lnΓ(ct+1)+rtlnRt−Rt−lnΓ(rt+1)+mtlnMt−Mt−lnΓ(mt+1)], |
where n=80 was the length of the epidemic data, since the timeline we used was from January 23 to April 11. The dynamics of Ct, Rt and Mt are determined by the following model
Ct=Ct−1+(1−e−δH)Ht−1Rt=Rt−1+e−α(1−e−γH)Hc,t−1Mt=Mt−1+η(Ht−1+Hc,t−1)−(1−e−μM)Mt−1. |
The estimated initial/mean values of variables/parameters are listed in Table 1. From Table 1, the contact rate c0 (resp. contact-tracing rate q0) without media impact is estimated as 10.1163 (resp. 0.028), which is around 3 times (resp., 1/33) its counterpart c1 (resp. q1) with media effect. It was worth noting that the initial value of susceptibles should be the difference between the population of Shaanxi and the sum of initial conditions of all other compartments according to Table 1; i.e., S(0)=38,639,792, which is actually 99.9995% of the value we used for fitting. Thus it is reasonable to use the population of Shaanxi as S(0).
The mean estimated values of cumulative number of confirmed individuals, recovered individuals and daily number of media reports are plotted in black asterisks in Figure 6, showing that the fitted model captures the data well, while the red asterisks are the data from January 23 to April 11, 2020. After 500 stochastic fittings of model (2.1), we derived 95% upper confidence limits, 95% lower confidence limits and 95% confidence intervals of the cumulative number of confirmed individuals, recovered individuals and daily number of media reports, which are shown by light grey curves, dark grey curves and grey regions, respectively, in Figure 6. It is worth noting that data on the cumulative number of recovered individuals in the early stage were not all within the 95% confidence interval, which was also in line with the situation of slow reporting of early treatment of COVID-19 in Shaanxi, as shown in Figure 6(b).
The basic reproduction number, R0, which is defined as the average number of new infected individuals generated by one infected individual in the population, is adopted to measure the transmissibility of a virus at the initial stage of an epidemic [35]. Given the targeted structure with media effect and contact tracing, we calculate the control reproduction number for model (2.1) using the next-generation matrix [36]. Denoting X≡(E,I,Eq,H,Hc), we have ˙X=G(X). Let G(X)=F+V where
F=[(1−q(Mt))c(Mt)βStItNt0q(Mt)c(Mt)βStItNt00],V=[Et−(1−e−σ)EtIt+(1−e−σ)Et−(1−e−αe−δI)ItEqt−(1−e−σ)EqtHt+e−α(1−e−δI)It+(1−e−σ)Eqt−(1−e−δH)HtHct+(1−e−δH)Ht−(1−e−γHe−α)Hct]. |
Here F stands for the vector of new infections and V is the vector of all other transitions. Differentiating F and V with respect to X and evaluating them at the DFE yields
F=[0βc0(1−q0)000000000βc0q00000000000000],V=[e−σ00001−e−σe−αe−δI00000e−σ000e−α(1−e−δI)1−e−σe−δH00001−e−δHe−γHe−α]. |
We thus derive the control reproduction number for model (2.1) as
Rc=ρ(F(I−V)−1)=c0(1−q0)β1−e−σe−δI, |
where ρ(F(I−V)−1) denotes the spectral radius of the matrix F(I−V)−1. According to the estimated values of parameters reported in Table 1, we estimated the mean control reproduction number as Rc=3.1056.
We used partial rank correlation coefficients (PRCCs) and Latin hypercube sampling (LHS) to examine the sensitivity of the peak time and peak size of daily number of confirmed cases to model parameters. PRCCs, calculated using the rank-transformed LHS matrix and the output matrix, allow us to assess whether one parameter has significant influence on the peak time/size of the daily number of confirmed cases over a whole time interval during the progression of the model dynamics. Latin Hypercube Sampling was done with 5000 bins and 500 simulations per sampling. The results are shown in Figure 7. We considered absolute values of PRCCs greater than 0.5 as indicating significant correlations between the parameters and the peak time/size, values between 0.2 and 0.5 as moderate correlations, and values less than 0.2 as not significant. It follows from Figure 7 that the transmission probability β, the proportion of imported cases ξ and the maximum contact rate c0 have significant influence on both the peak time and peak size. In particular, Figure 7 shows that the peak time could be brought forward and lowered in magnitude if reductions occurred in the transmission probability β, the proportion of imported cases ξ or the maximum contact rate c0.
Shortening the time to hospitalization for infected individuals with symptoms (1/δI) could advance the peak time. Improving the maximum contact-tracing rate with media effect (q1) would lead to an increase of the peak size and the advance of the peak time. Shortening the incubation period (1/σ) would result in an increase of the peak size, while reducing the maximum contact rate with media effect (c1) could decrease the peak size. Aside from these significant parameters, there are some moderately sensitive parameters. Shortening the confirmation time (1/δH) would advance the peak time and raise the peak size. Enhancing the response intensity of awareness programs on the number of newly confirmed cases (η) and the discharging rate of confirmed individuals (γH2) could bring forward the peak time and lower the peak size.
It is worth emphasizing that 47.35% (i.e., 116 cases) of the final size of the epidemic in Shaanxi Province (i.e., 245 cases) were imported from the rest of China outside Shaanxi, which suggests an indispensable role of the imported cases during the transmission of the epidemic. Media reports were shown to interrelate strongly with the daily number of confirmed cases in Figures 2(b), 4 and 5. Hence, in the following, we quantify how the imported cases and the media reports affect the spread of COVID-19 in Shaanxi. To this end, we choose the proportion of the imported cases ξ, the minimum contact rate with media effect c1 and the maximum contact-tracing rate with media effect q1 and explore how they affect the spread of the epidemic. Although strengthening media coverage or reducing imported cases cannot completely eliminate the epidemic, it is nevertheless desirable to lower the peak size of infections and/or diminish the cumulative number of confirmed cases. We first used contour plots to explore the dependence of the peak time and peak size of the daily number of confirmed individuals on q1 and c1 (Figure 8), ξ and c1 (Figure 9) and ξ and q1 (Figure 10).
These results demonstrate that reducing the maximum contact-tracing rate q1 while increasing the minimum contact rate c1 would delay the peak time of the number of newly confirmed cases (Figure 8(a)), and the peak size of the number of newly confirmed cases would be raised substantially. In particular, if the maximum contact-tracing rate was reduced to 50% of its baseline value and the minimum contact rate was increased to 6.5 from the baseline value 3.24, the peak time would be delayed from 10 to 20 days, and the peak size would be raised to 17 from 11 cases.
It follows from Figure 9 that increasing the proportion of imported cases ξ and the minimum contact rate c1 would lead to a delay in the peak time (Figure 9(a)) and an increase in the peak size (Figure 9(b)). Similarly, increasing the proportion of imported cases ξ while diminishing the maximum contact-tracing rate q1 could result in a delay in the peak time (Figure 10(a)) and an increase in the peak size (Figure 10(b)). The values fitting the data were close to the minimum of c1 and the maximum of q1. This was in line with the fact that the control measures in Shaanxi were so stringent that the contact rate was reduced to low levels, while the contact-tracing rate was significantly increased. Another point we should note was that the final size of confirmed cases in Shaanxi (245 cases) was comparatively small, with a very short epidemic duration (29 days), so the difference between the peak times with different values of c1,q1 and ξ were on the order of days.
To further examine the impact of media reports on the transmission of COVID-19 in Shaanxi Province, we analyzed how the cumulative number of confirmed cases varied as the media reporting M changed. We explored what would happen if the media reporting were reduced to 50% and 20% of the baseline value, as shown in Figure 11(a). In Figure 11, we plotted the cumulative number of confirmed cases over time as the media reports varied. The results show that diminishing the intensity of media reporting would result in an increase in confirmed cases, which would trigger severe outbreaks of COVID-19 in Shaanxi. In particular, the final confirmed cases would climb up to 268 cases (resp. 305 cases) if the media reports decreased to 50% (resp. 20%) of the baseline values, as shown in Figure 11(a). We also investigated what would happen without media reporting, as shown in Figure 11(b). Without media coverage, the cumulative number of infections would climb up to 1833 cases by 21 February, compared to 245 cases in reality. (February 21 was the last day when new confirmed cases were reported during the COVID-19 outbreak in Shaanxi.) Without media reporting, the cumulative number of confirmed cases would be more than seven times of the corresponding number in reality, continue to increase into April. This further confirms the vital role of media reporting in combating COVID-19.
Aside from media reporting, the imported cases from the rest of China outside Shaanxi had a significant impact on the spread of the COVID-19 in Shaanxi. Next, we conducted a sensitivity analysis to address what would happen if the number of imported cases decreased to 80%, 50%, 20% and 0% of the baseline value, with all other parameters fixed. Table 2 shows that the peak time would be brought forward 0, 2, 4 and 5 days (from 10 days to 10, 8, 6 and 5 days), the peak size would reduce by 0.75, 2.62, 5.24 and 7.07 cases (from 10.89 cases to 10.14, 8.27, 5.65 and 3.82 cases) and the length of the epidemic would decrease by 2, 6, 10 and 15 days (from 32 days to 30, 26, 22 and 17 days) for ξ=0.8,0.5,0.2 and 0, respectively.
Parameters | Peak time | Peak size | Length of the epidemic | Final confirmed cases | |
Baseline values | 10 | 10.89 | 32 | 245.89 | |
Reduce imported cases | 0.8ξ | 10 | 10.14 | 30 | 210.86 |
0.5ξ | 8 | 8.27 | 26 | 151.76 | |
0.2ξ | 6 | 5.65 | 22 | 86.35 | |
0ξ | 5 | 3.82 | 17 | 61.19 | |
Delay lockdown until | Jan 30 | 13 | 26.93 | 38 | 442.39 |
Feb 6 | 16 | 41.42 | 41 | 639.87 |
When ξ=0.8, the peak size would vary little, while the peak time would remain the same, but the final number of confirmed cases would vary from 246 cases to 211 cases. When ξ=0.5 and ξ=0.2, the peak size would be reduced by 24.06% and 48.12%, respectively; the final confirmed cases would be reduced by 38.28% and 64.88%, respectively; and the length of the epidemic would be shortened by 18.75% and 31.25%, respectively. When ξ=0 (i.e., no imported cases from the rest of China outside Shaanxi), the peak size, final size and length of the epidemic would be decreased by 64.92%, 75.11% and 46.88%, respectively. As a result, the imported cases delayed the peak time of the epidemic in Shaanxi Province, magnified the peak size of the epidemic, prolonged the duration of the epidemic and increased the final number of confirmed cases significantly. Table 2 and Figure 12(a) show that the cumulative number of confirmed cases would reduce to 210.86,151.76, 86.35 and 61.19 if the imported cases were reduced to 80%, 50%, 20% and 0% of the baseline value, respectively.
Next, we evaluated the impact of the time of implementing lockdown strategy on the spread of COVID-19 in Shaanxi. We investigated how the peak time, peak size, length of the epidemic and final number of confirmed cases varies if the lockdown measure was delayed 1 week (starting January 30, 2020) and 2 weeks (starting February 6, 2020). We used the Baidu Migration Index of Shaanxi Province to fit the number of imported cases from the rest of China outside Shaanxi before the lockdown of the city in Shaanxi Province. The function we adopted to fit is
y=p1x+p2, | (3.1) |
where x represents the Baidu Migration Index and y represents the number of imported cases at the corresponding time. The fitting parameters were reported in Table 3.
Coefficients | Value | 95% confidence bound | Resource |
p1 | 7.351 | (5.115, 9.586) | Estimate |
p2 | −34.74 | (−47.48,−22) | Estimate |
The goodness of the fitting result was shown in Figure 12(b). With the fitting result, Eq (3.1) and the Baidu Migration Index of the corresponding time in 2019, we inferred the number of imported cases in Shaanxi Province without lockdown measures.
We further inferred the numbers of exposed individuals, infected individuals with symptoms, hospitalized individuals and confirmed individuals in the imported cases, based on the proportions of the above four population in the imported cases before the lockdown. The result was shown in Figure 12(c). Then we inferred the cumulative number of confirmed cases if the lockdown measure was delayed one week (i.e., starting 30 January) and two weeks (i.e., starting 6 February). The result was reported in Table 2 and shown in Figure 12(d). The results showed that if the lockdown strategy was implemented one week later or two weeks later, the peak time would be delayed 3 or 6 days (from 10 days to 13 or 16 days), the peak size would increase by 16.04 or 30.53 cases (from 10.89 cases to 26.93 or 41.42 cases), the length of the epidemic would be prolonged 6 or 9 days (from 32 days to 38 or 41 days) and the final confirmed cases would increase by 196.5 or 393.98 cases (from 245.89 cases to 442.39 or 639.87 cases) of the baseline values, respectively. It is worth emphasizing that the final confirmed cases would increase 79.91% and 160.24% of the baseline values if the lockdown strategy were delayed 1 week or 2 weeks, respectively, both of which could aggravate the severity of outbreaks of COVID-19 in Shaanxi.
We formulated a deterministic dynamic model based on the control policies in China to examine the COVID-19 transmission pathways in Shaanxi and the effects of interventions. In view of the fact that the number of COVID-19 confirmed cases in Shaanxi Province was relatively small (245 cases), imported cases accounted for 47% of the total confirmed cases (116 imported cases in total). The Shaanxi Provincial Health Commission reported the specific time when each imported case arrived in Shaanxi, broken down into disease onset, first hospital admission and confirmed, so our model framework is based on a discrete system incorporating imported cases. We also took into account the effect of media reporting in combating of the virus by incorporating media as a separate class M in the targeted model. We adopted two saturated functions in terms of M to describe the effect of media on the contact rate and the contact tracing and quarantine rate, which made our targeted model distinct from earlier work [6,11]. They either did not take media as a separate compartment [6] or did not address the media effect on the quarantine rate, instead addressing the media effect on the contact rate with a distinct function [11].
The control reproductive number of the disease is estimated as 3.1056 in this paper, which is close to the value of 2.79 in Liu et al. [37]. It indicates that COVID-19 is more contagious than Middle East respiratory syndrome (MERS) and severe acute respiratory syndrome (SARS). However, it has been effectively controlled with the strict control measures in China.
Our sensitivity analysis showed that decreasing the transmission probability β, the proportion of imported cases ξ and the maximum contact rate c0 could all bring the peak time forward and lower the peak size. These outcomes could also be achieved by enhancing the response intensity of awareness programs on the number of newly confirmed cases η. The sensitivity analysis further indicated that increasing the proportion of the imported cases ξ or the minimum contact rate with media effect c1, or decreasing the maximum contact tracing rate q1, could trigger a more severe outbreak of COVID-19 in Shaanxi Province by raising the peak size and delaying the peak time. Table 2 indicated that if no infected cases were imported into Shaanxi, the peak size would decline by 7 cases and the final confirmed cases would be reduced by 185, while the peak time would be advanced and the length of the epidemic would be shortened significantly. The results also demonstrated that postponing lockdown measures by one or two weeks could result in 197 and 394 more infections, respectively. These findings suggest that media coverage and the timing of lockdown play a vital role in mitigating the virus.
It is important to emphasize that eradicating the COVID-19 epidemic completely is likely impossible, so minimising the case numbers and peak size of infections is of major importance. Our study suggested that strengthening the response rate of media reports and advancing travel restrictions can greatly alleviate the outbreak of COVID-19 by lowering the peak size and decreasing the cumulative number of infected individuals.
Our modelling has some limitations, which should be acknowledged. We took the initial condition as the entire population of Shaanxi when fitting. That was because the final size of confirmed cases in Shaanxi was so small that the initial conditions of all other compartments were ignored, which cannot be neglected when the size of confirmed cases is large compared to the total population (for example, India). We focused on the situation in Shaanxi Province, China, as an example, but the model can be extended to mimic the outbreak in other countries or other infectious diseases in the future.
In conclusion, we have conducted a retrospective study by proposing a discrete dynamic model to fit the multi-source data, including the epidemic data and media-reporting data. Our study revealed how media reporting helped mitigate the spread of COVID-19 in Shaanxi. Our results suggest that imported cases played a vital role in aggravating the severity of the epidemic in Shaanxi. The results support the importance of timely implementation of lockdown measures. Our findings could provide useful information for policy-makers on combating COVID-19 and other epidemics.
For citation purposes, note that the question mark in "Smith?" is a part of her name.
This research was funded by the National Natural Science Foundation of China (Grant number: 11801013 (AW), 12001349(WZ)), the innovative research projects for graduate students (Grant number: 12305 (JG)), special scientific research project of emergency public health security of education department of Shaanxi Province (Grant number: 20JG002) and by NSERC Discovery and Alliance Grants in Canada (SRS?).
The authors declare there is no conflict of interest.
[1] | World Health Organization (WHO), Coronavirus disease (COVID-19) pandemic. Available from: https://www.who.int/emergencies/diseases/novel-coronavirus-2019. |
[2] |
P. Zhou, X. Yang, X. Wang, B. Hu, L. Zhang, W. Zhang, et al., A pneumonia outbreak associated with a new coronavirus of probable bat origin, Nature, 579 (2020), 270–273. doi: 10.1038/s41586-020-2012-7. doi: 10.1038/s41586-020-2012-7
![]() |
[3] |
C. Huang, Y. Wang, X. Li, L. Ren, J. Zhao, Y. Hu et al., Clinical features of patients infected with 2019 novel coronavirus in Wuhan, China, Lancet, 395 (2020), 497–506. doi: 10.1016/S0140-6736(20)30183-5. doi: 10.1016/S0140-6736(20)30183-5
![]() |
[4] | World Health Organization(WHO), Timeline: WHO's COVID-19 response. Available from: https://www.who.int/emergencies/diseases/novel-coronavirus-2019/interactive-timeline. |
[5] | J. M. Tchuenche, N. Dube, C. P. Bhunu, R. J. Smith?, C. T. Bauch, The impact of media coverage on the transmission dynamics of human influenza, BMC Public Health, 11 (2011), 1–4. doi: 0.1186/1471-2458-11-S1-S5. |
[6] |
Q. Yan, Y. Tang, D. Yan, J. Wang, L. Yang, X. Yang, et al., Impact of media reports on the early spread of COVID-19 epidemic, J. Theor. Biol., 502 (2020), 110385. doi: 10.1016/j.jtbi.2020.110385. doi: 10.1016/j.jtbi.2020.110385
![]() |
[7] |
Y. Xiao, S. Tang, J. Wu, Media impact switching surface during an infectious disease outbreak, Sci. Rep., 5 (2015), 7838. doi: 10.1038/srep07838. doi: 10.1038/srep07838
![]() |
[8] |
P. Song, Y. Xiao, Analysis of an epidemic system with two response delays in media impact function, Bull. Math. Biol., 81 (2019), 1582–1612. doi: 10.1007/s11538-019-00586-0. doi: 10.1007/s11538-019-00586-0
![]() |
[9] |
M. S. Rahman, M. L. Rahman, Media and education play a tremendous role in mounting AIDS awareness among married couples in Bangladesh, AIDS Res. Ther., 4 (2007), 10. doi: 10.1186/1742-6405-4-10. doi: 10.1186/1742-6405-4-10
![]() |
[10] |
M. Cinelli, W. Quattrociocchi, A. Galeazzi, The COVID-19 social media infodemic, Sci. Rep., 10 2020, 16598. doi: 10.1038/s41598-020-73510-5. doi: 10.1038/s41598-020-73510-5
![]() |
[11] |
W. Zhou, A. Wang, F. Xia, Y. Xiao, S. Tang, Effects of media reporting on mitigating spread of COVID-19 in the early phase of the outbreak, Math. Biosci. Eng., 17 (2020), 2673–2707. doi: 10.3934/mbe.2020147. doi: 10.3934/mbe.2020147
![]() |
[12] | Shaanxi Provincial Health Commission. Available from: http://sxwjw.shaanxi.gov.cn/sy/ztzl/fyfkzt/gzdt_2232/202004/t20200411_2118054.html. |
[13] |
B. Tang, X. Wang, Q. Li, N. L. Bragazzi, S. Tang, Y. Xiao, et al., Estimation of the transmission risk of the 2019- nCoV and its implication for public health interventions, J. Clin. Med., 9 (2020), 462. doi: 10.3390/jcm9020462. doi: 10.3390/jcm9020462
![]() |
[14] |
B. Tang, F. Xia, S. Tang, N. L. Bragazzi, Q. Li, X. Sun, et al., The effectiveness of quarantine and isolation determine the trend of the COVID-19 epidemics in the final phase of the current outbreak in China, Int. J. Infect. Dis., 96 (2020), 636–647. doi: 10.1016/j.ijid.2020.03.018. doi: 10.1016/j.ijid.2020.03.018
![]() |
[15] |
S. Zhao, S. S. Musa, Q. Lin, J. Ran, G. Yang, W. Wang, et al., Estimating the unreported number of novel coronavirus (2019-nCoV) cases in China in the first half of January 2020: a data-driven modelling analysis of the early outbreak, J. Clin. Med., 9 (2020), 388. doi: 10.3390/jcm9020388. doi: 10.3390/jcm9020388
![]() |
[16] |
S. Zhao, Q. Lin, J. Ran, S. S. Musa, G. Yang, W. Wang, et al., Preliminary estimation of the basic reproduction number of novel coronavirus (2019-nCoV) in China, from 2019 to 2020: A data-driven analysis in the early phase of the outbreak, Int. J. Infect. Dis., 92 (2020), 214–217. doi: 10.1016/j.ijid.2020.01.050. doi: 10.1016/j.ijid.2020.01.050
![]() |
[17] |
B. Tang, N. L. Bragazzi, Q. Li, S. Tang, Y. Xiao, J. Wu, An updated estimation of the risk of transmission of the novel coronavirus (2019-nCov), Infect. Dis. Modell., 5 (2020), 248–255. doi: 10.1016/j.idm.2020.02.001. doi: 10.1016/j.idm.2020.02.001
![]() |
[18] |
M. Gatto, E. Bertuzzo, L. Mari, S. Miccoli, L. Carraro, R. Casagrandi, A. Rinaldo, Spread and dynamics of the COVID-19 epidemic in Italy: Effects of emergency containment measures, Proc. Nat. Acad. Sci., 117 (2020), 10484–10491. doi: 10.1073/pnas.2004978117. doi: 10.1073/pnas.2004978117
![]() |
[19] |
C. C. Kerr, R. M. Stuart, D. Mistry, R. G. Abeysuriya, K. Rosenfeld, G. R. Hart, et al., Covasim: an agent-based model of COVID-19 dynamics and interventions, PLOS Comput. Biol., 17 (2021), e1009149. doi: 10.1371/journal.pcbi.1009149. doi: 10.1371/journal.pcbi.1009149
![]() |
[20] |
R. E. Baker, S. W. Park, W. Yang, G. A. Vecchi, C. J. E. Metcalf, B. T. Grenfell, The impact of COVID-19 nonpharmaceutical interventions on the future dynamics of endemic infections, Proc. Nat. Acad. Sci., 117 (2020), 30547–30553. doi: 10.1073/pnas.2013182117. doi: 10.1073/pnas.2013182117
![]() |
[21] |
R. Laxminarayan, B. Wahl, S. R. Dudala, K. Gopal, B. C. Mohan, S. Neelima, Epidemiology and transmission dynamics of COVID-19 in two Indian states, Science, 370 (2020), 691–697. doi: 10.1126/science.abd7672. doi: 10.1126/science.abd7672
![]() |
[22] |
J. Zhang, M. Litvinova, Y. Liang, Y. Wang, W. Wang, S. Zhao, et al., Changes in contact patterns shape the dynamics of the COVID-19 outbreak in China, Science, 368 (2020), 1481–1486. doi: 10.1126/science.abb8001. doi: 10.1126/science.abb8001
![]() |
[23] |
X. Hao, S. Cheng, D. Wu, T. Wu, X. Lin, C. Wang, Reconstruction of the full transmission dynamics of COVID-19 in Wuhan, Nature, 584 (2020), 420–424. doi: 10.1038/s41586-020-2554-8. doi: 10.1038/s41586-020-2554-8
![]() |
[24] |
D. He, S. Zhao, Q. Lin, Z. Zhuang, P. Cao, M. H. Wang, et al., The relative transmissibility of asymptomatic COVID-19 infections among close contacts, Int. J. Infect. Dis., 94 (2020), 145–147. doi: 10.1016/j.ijid.2020.04.034. doi: 10.1016/j.ijid.2020.04.034
![]() |
[25] | X. Wang, S. Tang, Y. Chen, X. Feng, Y. Xiao, Z. Xu, When will be the resumption of work in Wuhan and its surrounding areas during COVID-19 epidemic? A data-driven network modeling analysis, Sci. Sinica, 50 (2020), 969–978. |
[26] |
B. Maier, D. Brockmann, Effective containment explains subexponential growth in recent confirmed COVID-19 cases in China, Science, 368 (2020), 742–746. doi: 10.1126/science.abb4557. doi: 10.1126/science.abb4557
![]() |
[27] |
J. Li, P. Yuan, J. Heffernan, T. Zheng, N. Ogden, B. Sander, et al., Fangcang shelter hospitals during the COVID-19 epidemic, Wuhan, China, Bull. World Health Organ., 98 (2020), 830–841. doi: 10.2471/BLT.20.258152. doi: 10.2471/BLT.20.258152
![]() |
[28] |
Q. Li, X. Guan, P. Wu, X. Wang, L. Zhou, Y. Tong, et al., Early transmission dynamics in Wuhan, China, of novel coronavirus–infected pneumonia, N. Engl. J. Med., 382 (2020). doi: 10.1056/NEJMoa2001316. doi: 10.1056/NEJMoa2001316
![]() |
[29] |
M. Li, G. Sun, J. Zhang, Y. Zhao, X. Pei, L. Li, et al., Analysis of COVID-19 transmission in Shanxi Province with discrete time imported cases, Math. Biosci. Eng., 17 (2020), 3710–3720. doi: 10.3934/mbe.2020208. doi: 10.3934/mbe.2020208
![]() |
[30] |
F. Huang, S. Zhou, S. Zhang, H. Wang, L. Tang, Temporal correlation analysis between malaria and meteorological factors in Motuo County, Tibet, Malar. J., 10 (2011), 54. doi: 10.1186/1475-2875-10-54. doi: 10.1186/1475-2875-10-54
![]() |
[31] | Shaanxi Provincial Bureau Of Statistics. Available from: http://tjj.shaanxi.gov.cn/upload/2020/pro/3sxtjnj/zk/indexch.html. |
[32] |
S. He, S. Tang, L. Rong, A discrete stochastic model of the COVID-19 outbreak: Forecast and control, Math. Biosci. Eng., 17 (2020), 2792–2804. doi: 10.3934/mbe.2020153. doi: 10.3934/mbe.2020153
![]() |
[33] | Chinese Center for Disease Control and Prevention, Report about 2019-nCov, Available from: http://www.chinacdc.cn/yyrdgz/202001/P020200128523354919292.pdf. |
[34] |
A. Morton, B. F. Finkenstädt, Discrete time modelling of disease incidence time series by using Markov chain Monte Carlo methods, J. R. Stat. Soc. Ser. C Appl. Stat., 54 (2010), 575–594. doi: 10.1111/j.1467-9876.2005.05366.x. doi: 10.1111/j.1467-9876.2005.05366.x
![]() |
[35] |
J. M. Heffernan, R. J. Smith?, L. M. Wahl, Perspectives on the basic reproduction ratio, J. R. Soc. Interface, 2 (2005), 281–283. doi: 10.1098/rsif.2005.0042. doi: 10.1098/rsif.2005.0042
![]() |
[36] |
L. J. S. Allen, P. van den Driessche, The basic reproduction number in some discrete-time epidemic models, J. Differ. Equations Appl., 14 (2008), 1127–1147. doi: 10.1080/10236190802332308. doi: 10.1080/10236190802332308
![]() |
[37] |
Y. Liu, A. A. Gayle, A. Wilder-Smith, J. Rocklöv, The reproductive number of COVID-19 is higher compared to SARS coronavirus, J. Travel Med., 27 (2020), 1–4. doi: 10.1093/jtm/taaa021. doi: 10.1093/jtm/taaa021
![]() |
![]() |
![]() |
1. | Yinjiao Gong, Aili Wang, Jin Guo, Stacey R. Smith?, Jorge E. Macias-Diaz, Modelling the Impact of Media-Induced Social Distancing on the Containment of COVID-19 in Beijing, 2022, 2022, 1607-887X, 1, 10.1155/2022/3954598 | |
2. | Erik A. Vázquez Jiménez, Jesús Martínez Martínez, Leonardo D. Herrera Zuniga, J. Guadalupe Reyes Victoria, Discrete models for analyzing the behavior of COVID-19 pandemic in the State of Mexico, Mexico, 2022, 20, 1551-0018, 296, 10.3934/mbe.2023014 |
Variables | Description | Initial value | Std | Resource | |
St | Susceptible population | 38640000 | – | Data | |
Et | Exposed population | 52 | 0.0193 | MCMC | |
It | Infected population | 26 | – | Data | |
Sqt | Quarantined susceptible population | 52 | – | Data | |
Eqt | Isolated exposed population | 0 | 0.1736 | MCMC | |
Ht | Hospitalized population | 36 | – | Data | |
Hct | Confirmed population | 3 | – | Data | |
Rt | Recovered population | 0 | – | Data | |
Mt | Media reports | 39.3 | – | Data | |
ˉet | imported exposed population | 4 | – | Data | |
ˉit | imported infected population | 2 | – | Data | |
ˉht | imported hospitalized population | 1 | – | Data | |
ˉhc,t | imported confirmed population | 0 | – | Data | |
Parameters | Definition | Mean value | Std | Resource | |
c(Mt) | c0 | Contact rate without media impact | 10.1163 | 0.0217 | MCMC |
c1 | Minimum contact rate with media impact | 3.1459 | 0.0328 | MCMC | |
μ1 | Modification factor | 0.8961 | 0.0091 | MCMC | |
β | Probability of transmission from I to S per contact | 0.1068 | 0.0041 | MCMC | |
q(Mt) | q0 | Quarantined rate of exposed individuals without media impact | 0.0280 | 0.0024 | MCMC |
q1 | Maximum quarantined rate of exposed individuals with media impact | 0.9259 | 0.0041 | MCMC | |
μ2 | Modification factor | 0.8189 | 0.0076 | MCMC | |
λ | Releasing rate of quarantined individuals | 1/14 | – | Data | |
σ | Progression rate of exposed individuals to infectives | 1/5.3889 | – | Data | |
α | Disease-induced death rate | 1.1715×10−3 | 2.9413×10−5 | MCMC | |
δI | Progression rate of infective class to hospitalized class | 1/2.4299 | – | Data | |
δH | Progression rate of hospitalized class to confirmed class | 0.4646 | 0.0053 | MCMC | |
γH1 | Recovery rate of hospitalized individuals | 0.0874 | 0.0051 | MCMC | |
η | Media-reported rate of number of new hospital notifications | 0.6487 | 0.0004 | MCMC | |
μM | Media waning rate | 0.9821 | 0.0092 | MCMC | |
ξ | Proportion of imported cases involved in the transmission of the epidemic | 1 | – | Data |
Parameters | Peak time | Peak size | Length of the epidemic | Final confirmed cases | |
Baseline values | 10 | 10.89 | 32 | 245.89 | |
Reduce imported cases | 0.8ξ | 10 | 10.14 | 30 | 210.86 |
0.5ξ | 8 | 8.27 | 26 | 151.76 | |
0.2ξ | 6 | 5.65 | 22 | 86.35 | |
0ξ | 5 | 3.82 | 17 | 61.19 | |
Delay lockdown until | Jan 30 | 13 | 26.93 | 38 | 442.39 |
Feb 6 | 16 | 41.42 | 41 | 639.87 |
Coefficients | Value | 95% confidence bound | Resource |
p1 | 7.351 | (5.115, 9.586) | Estimate |
p2 | −34.74 | (−47.48,−22) | Estimate |
Variables | Description | Initial value | Std | Resource | |
St | Susceptible population | 38640000 | – | Data | |
Et | Exposed population | 52 | 0.0193 | MCMC | |
It | Infected population | 26 | – | Data | |
Sqt | Quarantined susceptible population | 52 | – | Data | |
Eqt | Isolated exposed population | 0 | 0.1736 | MCMC | |
Ht | Hospitalized population | 36 | – | Data | |
Hct | Confirmed population | 3 | – | Data | |
Rt | Recovered population | 0 | – | Data | |
Mt | Media reports | 39.3 | – | Data | |
ˉet | imported exposed population | 4 | – | Data | |
ˉit | imported infected population | 2 | – | Data | |
ˉht | imported hospitalized population | 1 | – | Data | |
ˉhc,t | imported confirmed population | 0 | – | Data | |
Parameters | Definition | Mean value | Std | Resource | |
c(Mt) | c0 | Contact rate without media impact | 10.1163 | 0.0217 | MCMC |
c1 | Minimum contact rate with media impact | 3.1459 | 0.0328 | MCMC | |
μ1 | Modification factor | 0.8961 | 0.0091 | MCMC | |
β | Probability of transmission from I to S per contact | 0.1068 | 0.0041 | MCMC | |
q(Mt) | q0 | Quarantined rate of exposed individuals without media impact | 0.0280 | 0.0024 | MCMC |
q1 | Maximum quarantined rate of exposed individuals with media impact | 0.9259 | 0.0041 | MCMC | |
μ2 | Modification factor | 0.8189 | 0.0076 | MCMC | |
λ | Releasing rate of quarantined individuals | 1/14 | – | Data | |
σ | Progression rate of exposed individuals to infectives | 1/5.3889 | – | Data | |
α | Disease-induced death rate | 1.1715×10−3 | 2.9413×10−5 | MCMC | |
δI | Progression rate of infective class to hospitalized class | 1/2.4299 | – | Data | |
δH | Progression rate of hospitalized class to confirmed class | 0.4646 | 0.0053 | MCMC | |
γH1 | Recovery rate of hospitalized individuals | 0.0874 | 0.0051 | MCMC | |
η | Media-reported rate of number of new hospital notifications | 0.6487 | 0.0004 | MCMC | |
μM | Media waning rate | 0.9821 | 0.0092 | MCMC | |
ξ | Proportion of imported cases involved in the transmission of the epidemic | 1 | – | Data |
Parameters | Peak time | Peak size | Length of the epidemic | Final confirmed cases | |
Baseline values | 10 | 10.89 | 32 | 245.89 | |
Reduce imported cases | 0.8ξ | 10 | 10.14 | 30 | 210.86 |
0.5ξ | 8 | 8.27 | 26 | 151.76 | |
0.2ξ | 6 | 5.65 | 22 | 86.35 | |
0ξ | 5 | 3.82 | 17 | 61.19 | |
Delay lockdown until | Jan 30 | 13 | 26.93 | 38 | 442.39 |
Feb 6 | 16 | 41.42 | 41 | 639.87 |
Coefficients | Value | 95% confidence bound | Resource |
p1 | 7.351 | (5.115, 9.586) | Estimate |
p2 | −34.74 | (−47.48,−22) | Estimate |