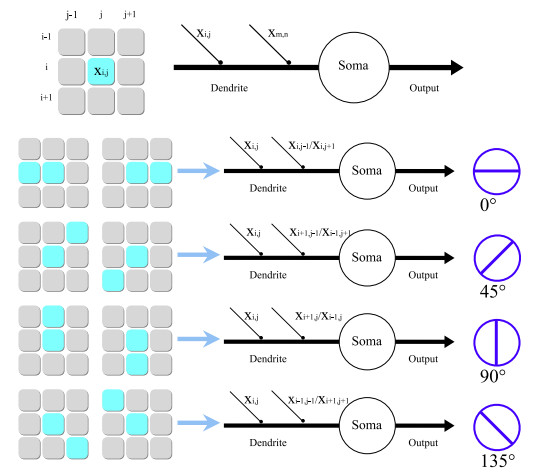
The interest in circular economy for the construction sector is constantly increasing, and Global Warming Potential (GWP) is often used to assess the carbon footprint of buildings and building materials. However, GWP presents some methodological challenges when assessing the environmental impacts of construction materials. Due to the long life of construction materials, GWP calculation should take into consideration also time-related aspects. However, in the current GWP, any temporal information is lost, making traditional static GWP better suited for retrospective assessment rather than forecasting purposes. Building on this need, this study uses a time-dependent GWP to assess the carbon footprint of two newly developed construction materials, produced through the recycling of industrial residues (stainless steel slag and industrial goethite). The results for both materials are further compared with the results of traditional ordinary Portland cement (OPC) based concrete, presenting similar characteristics. The results of the dynamic GWP (D_GWP) are also compared to the results of traditional static GWP (S_GWP), to see how the methodological development of D_GWP may influence the final environmental evaluation for construction materials. The results show the criticality of the recycling processes, especially in the case of goethite valorization. The analysis shows also that, although the D_GWP did not result in a shift in the ranking between the three materials compared with S_GWP, it provides a clearer picture of emission flows and their effect on climate change over time.
Citation: Andrea Di Maria, Annie Levasseur, Karel Van Acker. Assessing the long term effects on climate change of metallurgical slags valorization as construction material: a comparison between static and dynamic global warming impacts[J]. Clean Technologies and Recycling, 2021, 1(1): 88-111. doi: 10.3934/ctr.2021005
[1] | Eray Önler . Feature fusion based artificial neural network model for disease detection of bean leaves. Electronic Research Archive, 2023, 31(5): 2409-2427. doi: 10.3934/era.2023122 |
[2] | Chetan Swarup, Kamred Udham Singh, Ankit Kumar, Saroj Kumar Pandey, Neeraj varshney, Teekam Singh . Brain tumor detection using CNN, AlexNet & GoogLeNet ensembling learning approaches. Electronic Research Archive, 2023, 31(5): 2900-2924. doi: 10.3934/era.2023146 |
[3] | Kuntha Pin, Jung Woo Han, Yunyoung Nam . Retinal diseases classification based on hybrid ensemble deep learning and optical coherence tomography images. Electronic Research Archive, 2023, 31(8): 4843-4861. doi: 10.3934/era.2023248 |
[4] | Tej Bahadur Shahi, Cheng-Yuan Xu, Arjun Neupane, William Guo . Machine learning methods for precision agriculture with UAV imagery: a review. Electronic Research Archive, 2022, 30(12): 4277-4317. doi: 10.3934/era.2022218 |
[5] | Jing Lu, Longfei Pan, Jingli Deng, Hongjun Chai, Zhou Ren, Yu Shi . Deep learning for Flight Maneuver Recognition: A survey. Electronic Research Archive, 2023, 31(1): 75-102. doi: 10.3934/era.2023005 |
[6] | Hui Xu, Longtan Bai, Wei Huang . An optimization-inspired intrusion detection model for software-defined networking. Electronic Research Archive, 2025, 33(1): 231-254. doi: 10.3934/era.2025012 |
[7] | Yixin Sun, Lei Wu, Peng Chen, Feng Zhang, Lifeng Xu . Using deep learning in pathology image analysis: A novel active learning strategy based on latent representation. Electronic Research Archive, 2023, 31(9): 5340-5361. doi: 10.3934/era.2023271 |
[8] | Abul Bashar . Employing combined spatial and frequency domain image features for machine learning-based malware detection. Electronic Research Archive, 2024, 32(7): 4255-4290. doi: 10.3934/era.2024192 |
[9] | Huixia Liu, Zhihong Qin . Deep quantization network with visual-semantic alignment for zero-shot image retrieval. Electronic Research Archive, 2023, 31(7): 4232-4247. doi: 10.3934/era.2023215 |
[10] | Mashael S. Maashi, Yasser Ali Reyad Ali, Abdelwahed Motwakel, Amira Sayed A. Aziz, Manar Ahmed Hamza, Amgad Atta Abdelmageed . Anas platyrhynchos optimizer with deep transfer learning-based gastric cancer classification on endoscopic images. Electronic Research Archive, 2023, 31(6): 3200-3217. doi: 10.3934/era.2023162 |
The interest in circular economy for the construction sector is constantly increasing, and Global Warming Potential (GWP) is often used to assess the carbon footprint of buildings and building materials. However, GWP presents some methodological challenges when assessing the environmental impacts of construction materials. Due to the long life of construction materials, GWP calculation should take into consideration also time-related aspects. However, in the current GWP, any temporal information is lost, making traditional static GWP better suited for retrospective assessment rather than forecasting purposes. Building on this need, this study uses a time-dependent GWP to assess the carbon footprint of two newly developed construction materials, produced through the recycling of industrial residues (stainless steel slag and industrial goethite). The results for both materials are further compared with the results of traditional ordinary Portland cement (OPC) based concrete, presenting similar characteristics. The results of the dynamic GWP (D_GWP) are also compared to the results of traditional static GWP (S_GWP), to see how the methodological development of D_GWP may influence the final environmental evaluation for construction materials. The results show the criticality of the recycling processes, especially in the case of goethite valorization. The analysis shows also that, although the D_GWP did not result in a shift in the ranking between the three materials compared with S_GWP, it provides a clearer picture of emission flows and their effect on climate change over time.
The visual system is a component of the nervous system, which, as one of the most basic human sensory systems, gives humans the ability to visualize and perceive [1]. More than one-third of the human cerebral cortex is related to the visual system, and for humans, the vast majority of external information is obtained through vision. Thus, the visual system contributes to human cognition, decision-making, emotional behavior and other behaviors [2]. The complete visual system consists of the eye (especially the retina), the optic nerve, the optic cross, the visual tract, the lateral geniculate body, the visual cortex and the visual association cortex [3]. These structures are divided into the anterior and posterior visual pathways from the lateral geniculate body. The eye, as the first station in the anterior visual pathway, is responsible for the initial processing of visual information [4]. Light is refracted by the eye and then projected onto the retina. Photoreceptor cells in the retina convert light signals into electrical signals, transmit them to bipolar cells and ganglion cells and finally transmit action potential signals to the brain via the optic nerve [5]. Amacrine cells and horizontal cells are involved in lateral information transmission in the retina, thus forming various complex visual receptive fields, which are usually sensitive to certain specific features of visual information, such as color, size, distance and orientation [6]. Hence, visual information is usually initially processed in the retina into channels carrying various specific features [7]. Retinal cells that carry similar visual information features are classified under the same visual channel. To date, more than 30 visual channels have been verified by genetics and anatomy [8]. In the present study, we concentrate on the visual channels associated with orientation features.
Orientation-selective cells were first identified in the pigeon retina by Maturana and Frenk in 1963 [9], and Levick demonstrated similar orientation selectivity in the rabbit retina in 1967 [10]. Subsequent research was reported on the orientation selectivity of retinal cells in cats [11,12,13,14], turtles [15], mice [16,17,18], goldfish [19,20] and zebrafish [21,22]. The congruence between the functional roles of amacrine cells and retinal ganglion cells in orientation selection circuits was established by Paride Antinucci in 2013 [23]. The study of the cell-adhesion molecule Tenm3 further demonstrated that AC is a critical component of orientation selection in retinal ganglion cells [24]. In a study of rabbit retinal cells, researchers found that orientation-selective amacrine cells (OSACs) have radially symmetrical dendrites, and the receptive field of OSACs can be approximated as a circle [25,26]. A common feature evident in these vertebrate visual models is that an orientation-selective amacrine cell becomes an essential element in the visual circuitry for tuning neurons, and it is always present in the initial reception and transmission of visual information. In these models, the orientation tuning function exhibited by the amacrine cells tends to be determined more by their own morphological features than by the inhibition of the superficial cells. That is, the dendritic orientation of the amacrine cell itself determines the implementation of orientation-selective functions in initial visual information processing to a greater extent. OSACs are sensitive to orientation information stimuli that are consistent with the direction of dendrite growth and insensitive to orientation information stimuli that are inconsistent with the direction of dendrite growth; hence, OSACs have two types of expressions for orientation information stimuli: ON response and OFF response [27]. In essence, OSACs are activated only by stimuli from a specific orientation and do not respond to stimuli from other orientations.
There is great potential value in employing the biological properties and mechanisms of OSACs in the field of engineering. Accordingly, in this study, a perceptron-based orientation detection model (PODM) is proposed, and the effectiveness of the model for the orientation detection of objects in images is experimentally verified. As a single neuron is sensitive only to stimuli in a specific orientation, four neurons are inserted into the mechanism to detect information in four orientations. These neurons receive information in the receptive field and are activated by the corresponding information, in the same manner as OSACs receive information from photoreceptor cells and are sensitive to information in a specific orientation. This indicates that the PODM is highly compatible with the biological properties of the cell. The global orientation selection is dependent on the aggregation of neuronal activation in all receptive fields. To ensure the validity of the mechanism's orientation detection, the object features (e.g., color, location and shape) in the experimental dataset are randomly generated. The experimental results confirm that the PODM is always efficient in detecting the orientation of objects, regardless of changes in features such as the color, position and shape of the objects. Based on the experimental results, it is likely that more angles of orientation recognition can be achieved by adding more neurons to the model or by interacting information between a limited number of neurons. This may inspire new ideas to unravel the mysteries of the functioning of the visual system.
The perceptron was invented by Frank Rosenblatt in 1957 [28]. As a type of artificial neural network (ANN), it was designed from its inception to mimic the working mechanism of nerve cells. The state of a nerve cell depends on the strength of the information received from other nerve cells. When the strength of the information exceeds a certain threshold, the nerve cell is activated and generates action potentials, which are then transmitted to other neurons via synapses [29]. Corresponding underlying concepts in the perceptron include weight ω corresponding to synapses, bias b corresponding to thresholds and activation functions corresponding to cell bodies. The equation of the perceptron is shown below:
f(x)={1ifω⋅x+b>00else, | (2.1) |
where x is the input received by the current neuron. OSACs usually receive input from multiple photoreceptors to determine the orientation of the object comprehensively, rather than relying on a single photoreceptor [30]. In this work, the neuron is set to receive the grayscale values of two adjacent points to determine whether the neuron corresponding to the orientation where these two points are located is activated or not. When the grayscale values of the two adjacent points are equal, the object is considered to be in the orientation corresponding to the two points, and the corresponding neuron is activated. Considering that the human eye has a limited grayscale recognition rate, and the colors of real objects are not exactly the same, a threshold needs to be added to the mechanism to determine whether the grayscale values are the same (approximately) [31]. Since human individuals differ, the ability of the human eye to recognize the minimum grayscale difference varies. For our model, the threshold value, as a user-set parameter, is both a switch for the model to work properly and a fault tolerance for the model detection. The smaller that the threshold is set, the better the model will recognize the smallest grayscale difference, and the more it will be affected by the background color change; the larger that the threshold is set, the weaker the model will be at recognizing the smallest grayscale, and it is likely to lose the detection ability. So, we need to give the threshold a suitable value to give the model a certain error tolerance. During our experiment, we found that the model can maintain a more ideal working state when the threshold is set to 3, and thus we define the threshold as 3. When the difference between two points is less than or equal to the threshold value, the two points are considered to have the same (approximate) grayscale value, and the neurons of the corresponding orientation are activated; otherwise, the two points are regarded as having different grayscale values, and the neurons of the corresponding orientation are not activated. In the receptive field of neuronal cells, the central point is selected as the reference point, the grayscale values of eight adjacent points are compared with the reference point, and the neuronal cells of the corresponding orientation are activated if the difference in grayscale values is less than the threshold. Based on the aforementioned basic principles, we propose an equation to adapt our mechanism as shown below:
Response={ONif|x∗−xi|<thresholdOFFelse, | (2.2) |
where x∗ represents the grayscale value of the central reference point of the perceptual field, and the xi represents the grayscale value of the points adjacent to the reference point.
In this study, four neurons are set up in the mechanism to detect the four orientations of 0°, 45°, 90° and 135°, as shown in Figure 1. We define the photoreceptor cell in the center of the receptive field as the reference point with coordinates (i, j), and Xi,j represents the signal received from the photoreceptor cell by the amacrine cell. In such a receptive field, the horizontally oriented neuron (0 degrees) is activated when the signal received from the photoreceptor cell located at (i, j+1) or (i, j-1) is close to the signal at the reference point. A vertically oriented neuron (90 degrees) is activated when Xi+1,j or Xi−1,j is close to Xi,j. The neuron corresponding to 135 degrees is activated when the Xi+1,j+1 or Xi−1,j−1 is similar to Xi,j. The neuron corresponding to 45 degrees is activated when Xi−1,j+1 or Xi+1,j−1 is approaching Xi,j.
Neuronal cells based on this mechanism would be able to be activated by an object as small as 1*2 pixels. A simple demonstration is given in Figure 2. In the retina, photoreceptor cells receive light signals that are converted into electrical signals and then transmit information to cells in the posterior layer in turn. Two of the photoreceptor cells receive light signals, which are reflected in the current receptive field as the central point with the same signal as its horizontal neighbor, and activate the neuron in the amacrine cell layer in the corresponding horizontal orientation (0°).
When processing a complete image, it is necessary for the neurons to globally detect the image to detect the orientation of objects in the image. Therefore, the sliding window scanning mechanism from the convolutional neural network (CNN) [32] is utilized in this study. That is, the perceptual field slides sequentially from the beginning of the image to the next position in a fixed step, scanning over the whole image line by line to read the information of the whole image as an input to the model. The neurons corresponding to the four orientations are activated during the scanning process, the frequencies of activation are recorded and summarized at the same time, and the orientation detection results of the model are output after substitution into the activation function calculation. The equation of the activation function is shown below:
f(x)=eXi∑ni=1Xi | (2.3) |
where Xi represents the activation frequencies, and n equals 4, meaning that a total of four orientation-selective neurons are employed here. It is worth noting that the output here is the probability of selecting each orientation, and the sum of the probabilities of the four orientations being selected is 1. The final detection result of the model is taken from the one with the highest probability among the four orientations, i.e., the orientation with the highest activation frequency is considered to be the final detection result of the model. A simplified diagram of the model is shown in Figure 3. An example of the specific sliding window scanning mechanism is shown in Figure 4. Figure 4(a) shows an object which is sized at 1*3 and in the orientation of 135°, placed on an image with a gradient grayscale background. Figure 4(b) shows the whole process of receiving this image by the model. A receptive field of size 3*3 slides across the entire image from left to right line by line. In each receptive field, the neurons in the corresponding orientation are activated and recorded separately (activation is shown in highlighted blue). Figure 4(c) summarizes the result of the scan, and the direction with the highest number of neuron activations is considered to be the orientation of the object. It can be seen that the global detection result obtained by the four neurons matches the actual orientation of the object. Unlike the sliding window scanning in the CNN, the proposed neuron needs minimal information interaction to read the features of the whole picture completely, and thus using a 3*3 receptive field to scan the whole picture will cause unnecessary waste of computational resources. Therefore, the size of the receptive field is collapsed from 3*3 to 2*3, and the activation level of neurons in the corresponding orientation is weighted accordingly, as shown in Figure 5. The experimental results verify that this improvement saves approximately one-third of the computational resources while ensuring detection accuracy.
A series of experiments were conducted to evaluate the effectiveness of the suggested model. In the first subsection, the composition of the experimental dataset and the method it generates are described. In the second subsection, the mechanism proposed above is utilized to detect the orientation of each object in the dataset to validate the feasibility of the mechanism. In the third subsection, the PODM is compared with CNN to verify the robustness of the mechanism by detecting simulated realistic images and the detection accuracy when subjected to the same level of interference. The fourth subsection then compares the performances of the mechanism when different sizes of perceptual fields are applied.
All datasets were randomly generated and according to the following guidelines: Each dataset contains 2500 images, each image has a resolution of 100*100 pixels, the background color of the image is a randomly generated grayscale color, an object is placed on each image, and the orientation and color of the object are randomly generated. Figure 6 displays a partial sample of the dataset. The experiments are classified only according to the size of the object pixel values to check the accuracy and reliability of the model when dealing with objects of different sizes. Further experiments are applied to examine the ability of the proposed mechanism to cope with different backgrounds by replacing the filled form of the image background.
It is well known that a complete image is a combination of grayscale maps of multiple color channels. In this set of experiments, the validity of the proposed mechanism is verified in the monochromatic grayscale images. In this dataset, the background of the image and the color of the object in the image are each randomly generated monochromatic grayscale colors. The sizes of the objects in the images are divided into four categories: 50,100,500 and 1000 pixel values. The percentage of the object occupying the image ranges from 0.5 to 10%, which allows the tolerance of the proposed mechanism to object size to be fully investigated. Some samples from the monochrome grayscale image dataset are shown in Figure 6. It can be seen that the background of the image is a randomly generated monochrome grayscale, and the position of the object in the image, as well as its color, is random, while the aspect ratio (shape) of the object also has different variations. Figure 7 shows the detection result of the mechanism for one of the images in which the object has a size of approximately 500 pixels and an orientation of 135°. The activation intensity of neurons in the 135° orientation reaches 924, which is significantly higher than the activation intensity in the other three directions. The mechanism detection results point to 135°, which is consistent with the actual orientation of the object. It indicates that the proposed mechanism successfully detects the orientation of the object in that image. Table 1 presents the results obtained by the mechanism in this dataset. The mechanism had an accurate detection of the orientation of each object with a success rate of 100%, which demonstrates that the mechanism is always able to efficiently detect the orientation of the object in the images, regardless of the object's size, orientation, shape or color. Thus, the results indicate the mechanism has a stable recognition rate and good robustness in detecting the orientation of objects in monochrome grayscale images. Also, since monochrome grayscale images of multiple channels can be superimposed to form color images, the mechanism is likely also capable of detecting the orientation of objects in color images.
Object Size | Angle | 0° | 45° | 90° | 135° | Total |
50 | Number of images | 646 | 608 | 627 | 619 | 2500 |
Predicted number | 646 | 608 | 627 | 619 | 2500 | |
Accuracy rate | 100% | 100% | 100% | 100% | 100% | |
100 | Number of images | 641 | 589 | 644 | 626 | 2500 |
Predicted number | 641 | 589 | 644 | 626 | 2500 | |
Accuracy rate | 100% | 100% | 100% | 100% | 100% | |
500 | Number of images | 634 | 646 | 585 | 635 | 2500 |
Predicted number | 634 | 646 | 585 | 635 | 2500 | |
Accuracy rate | 100% | 100% | 100% | 100% | 100% | |
1000 | Number of images | 600 | 623 | 643 | 634 | 2500 |
Predicted number | 600 | 623 | 643 | 634 | 2500 | |
Accuracy rate | 100% | 100% | 100% | 100% | 100% |
The effectiveness of the mechanism in monochromatic grayscale images was verified in the previous set of experiments. However, ambient light in the real world often appears as gradient colors because the intensity of light is usually affected by many factors, such as temperature, humidity, light source proximity and irradiation angle. Therefore, in this set of experiments, the background of the images is replaced with a gradient grayscale color. It should be noted that to restore the background under various lighting conditions to the extent possible, the gradient direction of the grayscale background is random, and the grayscale value of the background is also random. An example is given in Figure 8. The background of this sample image is a grayscale color that fades to white (grayscale values become larger) from the upper left to the lower right, and there is an object of approximately 100 pixels in size and 45° orientation in the image. The detection result on the right side of the image shows that the activation intensity of neurons in the 45° direction reaches 336, which is stronger than the activation in other directions. Thus, the detection result of the mechanism is 45°, which matches with the actual orientation of the object. The results of this set of experiments are shown in Table 2, and it is clearly observed that the detection accuracy remains at 100% in all orientations. This indicates that even if the background is changed to a random gradient grayscale color, the proposed mechanism still recognizes the object independent of the background grayscale, object size, location, etc. It further supports the robustness and feasibility of the mechanism.
Object Size | Angle | 0° | 45° | 90° | 135° | Total |
50 | Number of images | 580 | 647 | 653 | 620 | 2500 |
Predicted number | 580 | 647 | 653 | 620 | 2500 | |
Accuracy rate | 100% | 100% | 100% | 100% | 100% | |
100 | Number of images | 611 | 635 | 648 | 606 | 2500 |
Predicted number | 611 | 635 | 648 | 606 | 2500 | |
Accuracy rate | 100% | 100% | 100% | 100% | 100% | |
500 | Number of images | 602 | 602 | 670 | 626 | 2500 |
Predicted numbers | 602 | 602 | 670 | 626 | 2500 | |
Accuracy rate | 100% | 100% | 100% | 100% | 100% | |
1000 | Number of images | 642 | 616 | 591 | 651 | 2500 |
Predicted number | 642 | 616 | 591 | 651 | 2500 | |
Accuracy rate | 100% | 100% | 100% | 100% | 100% |
Considering the excellent performance of CNNs in image processing, it is informative to compare the performance of the PODM with the CNN. The images for this set of experiments are still set to have gradient grayscale backgrounds, while, to increase the difficulty, different levels of salt-and-pepper noise are added to all of the images to test noise resistance. Salt-and-pepper noise, also known as impulse noise, is often observed in images. It is a random appearance of white or black dots, either as black pixels in bright areas or white pixels in dark areas (or both). The cause of such noise in an image is usually a sudden and strong disturbance of the transmitted signal. A partial failure of the sensor produces black dots (pepper) on the image, whereas an oversaturation of the sensor produces white dots (salt)[33]. Since the size of the largest object in the dataset in this study is about 1000 pixels, which occupies only 10% of the image size, a noise value is added in the range of 1–10% of the image size so that the maximum noise value is controlled to be no more than the size of the object. Two example sets of images with added noise are presented in Figure 10. The original image without noise is placed on the left, while the examples on the right present the actual image when the added noise is incremented from 1 to 10%. The added pepper noise is evenly and randomly distributed over the image, while the ratio of pepper noise to salt noise is 1:1. Figure 10(a) shows a horizontally placed object of size 50 pixels. When excessive noise is added, the shape of the object becomes greatly disturbed, offering a severe test for the detection mechanism. Figure 10(b) shows an object with an orientation of 135° and a size of 1000 pixels. Both sets of images are added with equal noise values, and it is obvious to the naked eye that the shapes of large objects are easier to distinguish compared with small objects. The detection results made by PODM for the orientation of the objects on these images are also similar to the perception of human eye observation, which to some extent indicates that PODM is built in accordance with the logic of human eye work.
The CNN is a feedforward neural network that includes convolutional computation and has a deep structure [34]. The "neocognitron" neural network proposed by Fukushima in 1980 is considered to be the inspiration for the CNN [35]. Alex formally proposed the first CNN called "time delay network" in 1987, which is mainly applied to the field of sound recognition [36]. In 1988, Wei Zhang proposed the first two-dimensional CNN [37]. Recently, the application area of CNNs has been extended to include portrait recognition [38] and gesture recognition [39]. An input layer, hidden layers and an output layer are the three components of a CNN. The hidden layer usually contains n convolutional and pooling layers and a fully connected layer. CNN usually consumes a large number of computational resources in the training process, and the more convolutional layers there are, the more computational resources are required. Therefore, to ensure the fairness of the experimental comparison, we allocate the computational resources of both methods using the time required to implement both methods on the same platform as a uniform metric (with an error of no more than 10%). In this experiment, only two convolutional layers are set, the size of the convolutional kernel is 3*3, the step size is 1, and the ratio of training set to test set is 7:3. A simplified diagram of the CNN for the present experiment is displayed in Figure 9. With such acceptable computational resource consumption, each set of experiments runs 30 times, and the results derived from the experiments will eventually be tested by hypothesis testing to verify whether the results are significantly different, as shown in Tables 3–6. These tables give the mean and standard deviation (SD) differences of the detection accuracies obtained from the two methods running 30 times each in the four sets of control experiments when adding different levels of noise to the images. Higher accuracy rates are bolded in the table. It is clear that PODM outperforms CNN in most instances at accurately detecting the orientation of an object. The detection accuracies of PODM and CNN are only comparable in images with large objects and high noise values. We employed a P-test to examine whether the accuracies of the two methods are fundamentally different. The P-test is a statistical method that is applied to examine the validity of a generally accepted hypothesis about the aggregate. The smaller the p-value is, the more evidence there is that the null hypothesis should be rejected, and the alternative hypothesis is more plausible [40]. In this set of experiments, the p-value is less than 0.05, which implies that there is a significant difference between the detection results of PODM and CNN. In other words, the detection results of PODM are significantly better than those of CNN. As can be seen from the results in the tables, all of the p-values are less than 0.05, which means there is a significant difference between the results obtained by the two methods. This indicates the PODM is always capable of achieving better detection results than those obtained by the CNN regardless of object size.
Noise | PODM | CNN | ||||
Mean(%) | ± | SD | Mean(%) | ± | SD | |
1% | 99.99 | ± | 0.0149 | 67.83 | ± | 0.1596 |
2% | 99.40 | ± | 0.1228 | 52.71 | ± | 0.1674 |
3% | 97.16 | ± | 0.3379 | 45.52 | ± | 0.1447 |
4% | 92.95 | ± | 0.4359 | 41.59 | ± | 0.1298 |
5% | 87.69 | ± | 0.7140 | 41.61 | ± | 0.1813 |
6% | 81.84 | ± | 0.5256 | 35.97 | ± | 0.1021 |
7% | 76.59 | ± | 0.7738 | 33.96 | ± | 0.1017 |
8% | 70.99 | ± | 0.7243 | 31.58 | ± | 0.1052 |
9% | 66.31 | ± | 0.8904 | 30.90 | ± | 0.0748 |
10% | 62.28 | ± | 0.7972 | 32.30 | ± | 0.0889 |
P-value | - | 8.64E-07 |
Noise | PODM | CNN | ||||
Mean(%) | ± | SD | Mean(%) | ± | SD | |
1% | 99.98 | ± | 0.0267 | 93.03 | ± | 0.0236 |
2% | 99.54 | ± | 0.1267 | 90.87 | ± | 0.0399 |
3% | 98.60 | ± | 0.2016 | 85.30 | ± | 0.1019 |
4% | 96.99 | ± | 0.2789 | 84.34 | ± | 0.1290 |
5% | 95.34 | ± | 0.3437 | 79.01 | ± | 0.1528 |
6% | 93.11 | ± | 0.4067 | 77.87 | ± | 0.1796 |
7% | 90.43 | ± | 0.4366 | 78.39 | ± | 0.1530 |
8% | 87.91 | ± | 0.6577 | 76.80 | ± | 0.1522 |
9% | 84.91 | ± | 0.5372 | 72.51 | ± | 0.1787 |
10% | 82.16 | ± | 0.7730 | 75.30 | ± | 0.1598 |
P-value | - | 9.53E-04 |
Noise | PODM | CNN | ||||
Mean(%) | ± | SD | Mean(%) | ± | SD | |
1% | 100.00 | ± | 0.0100 | 97.58 | ± | 0.0067 |
2% | 99.94 | ± | 0.0503 | 97.17 | ± | 0.0068 |
3% | 99.69 | ± | 0.1204 | 96.93 | ± | 0.0072 |
4% | 99.34 | ± | 0.1709 | 95.96 | ± | 0.0121 |
5% | 98.91 | ± | 0.1891 | 96.08 | ± | 0.0092 |
6% | 98.42 | ± | 0.2377 | 95.80 | ± | 0.0078 |
7% | 97.81 | ± | 0.3068 | 95.25 | ± | 0.0084 |
8% | 97.19 | ± | 0.3345 | 95.26 | ± | 0.0063 |
9% | 96.52 | ± | 0.2720 | 94.83 | ± | 0.0100 |
10% | 95.74 | ± | 0.3695 | 94.67 | ± | 0.0077 |
P-value | - | 5.13E-04 |
Noise | PODM | CNN | ||||
Mean(%) | ± | SD | Mean(%) | ± | SD | |
1% | 99.99 | ± | 0.0072 | 96.71 | ± | 0.0065 |
2% | 99.81 | ± | 2.8279 | 96.49 | ± | 0.0055 |
3% | 99.43 | ± | 0.7075 | 96.29 | ± | 0.0070 |
4% | 98.85 | ± | 0.1964 | 96.13 | ± | 0.0064 |
5% | 98.16 | ± | 0.1602 | 95.85 | ± | 0.0101 |
6% | 97.41 | ± | 0.2816 | 95.66 | ± | 0.0058 |
7% | 96.71 | ± | 0.3358 | 95.90 | ± | 0.0075 |
8% | 95.70 | ± | 0.3497 | 95.43 | ± | 0.0115 |
9% | 94.91 | ± | 0.3863 | 95.93 | ± | 0.0087 |
10% | 93.77 | ± | 0.3876 | 95.58 | ± | 0.0096 |
P-value | - | 4.76E-02 |
The results are also shown in the form of a line graph in Figure 11 for a more in-depth visual analysis of the differences between the two methods. It is obvious that the CNN responds more obviously to the effect of noise, and the obtained results are usually accompanied by certain volatility. This also indicates that the PODM has better noise tolerance than the CNN. Furthermore, in the recognition of small objects, especially when the noise value is larger than that of small objects, the recognition accuracy of the CNN decreases sharply, whereas the proposed PODM maintains acceptable accuracy. The results of this set of experiments reveal that the PODM has many advantages over the CNN, such as higher accuracy in detecting the orientation of objects (especially for small objects), better noise tolerance, less sensitivity to the influence of external factors and better robustness.
In this subsection, a comparison between the original PODM with a 3*3 receptive field and the evolved PODM with a 2*3 receptive field is performed. Gradient grayscale images make up this dataset for the comparative experiment. The size of the detected objects in the images is still divided into four categories, and the images are also added with different levels of noise. The results are shown in Tables 7–10. As can be seen from these tables, the detection accuracies achieved by both models with the same noise impact are very close, regardless of how the size of the object varies. The p-values obtained from the statistical hypothesis tests are all greater than 0.05, which indicates there is no difference in the final results between the two mechanisms with different receptive fields. Unlike the differences between the objects compared in the previous section, neither mechanism in this group imposes a high computational load on the computer, so the length of time taken is the only criterion for comparing the two mechanisms. The time recorded at the bottom of the table clearly shows that the mechanism with a perceptual field of 3*3 takes a significantly longer time. Furthermore, the reduced perceptual field of the mechanism does not reduce the accuracy of recognition. In other words, the improved PODM reduces the reuse rate of information while still maintaining the efficiency and robustness of orientation detection. This indicates that it is necessary and beneficial for the model to change the shape of the receptive field from 3*3 to 2*3.
Receptive field | 2*3 | 3*3 | ||||
Noise | Mean(%) | ± | SD | Mean(%) | ± | SD |
1% | 99.98 | ± | 0.0246 | 100.00 | ± | 0.0147 |
2% | 99.17 | ± | 0.1496 | 99.36 | ± | 0.1210 |
3% | 96.42 | ± | 0.3577 | 96.96 | ± | 0.3363 |
4% | 92.11 | ± | 0.6329 | 92.80 | ± | 0.4348 |
5% | 86.64 | ± | 0.5642 | 88.00 | ± | 0.7038 |
6% | 80.74 | ± | 0.9089 | 81.68 | ± | 0.5230 |
7% | 75.04 | ± | 0.8508 | 77.48 | ± | 0.7652 |
8% | 70.24 | ± | 0.8117 | 71.56 | ± | 0.7256 |
9% | 65.31 | ± | 0.8048 | 66.24 | ± | 0.8806 |
10% | 61.68 | ± | 0.9211 | 62.28 | ± | 0.7914 |
P-value | - | 8.84E-01 | ||||
Time cost | 2193.02 s | 3378.61 s |
Receptive field | 2*3 | 3*3 | ||||
Noise | Mean(%) | ± | SD | Mean(%) | ± | SD |
1% | 99.95 | ± | 0.0377 | 99.96 | ± | 0.0266 |
2% | 99.39 | ± | 0.1232 | 99.72 | ± | 0.1251 |
3% | 98.23 | ± | 0.2248 | 98.68 | ± | 0.1983 |
4% | 96.71 | ± | 0.3103 | 97.00 | ± | 0.2752 |
5% | 94.97 | ± | 0.3515 | 95.08 | ± | 0.3446 |
6% | 92.70 | ± | 0.4397 | 92.88 | ± | 0.4001 |
7% | 90.19 | ± | 0.4157 | 90.44 | ± | 0.4311 |
8% | 87.31 | ± | 0.6059 | 87.44 | ± | 0.6692 |
9% | 84.57 | ± | 0.5317 | 84.36 | ± | 0.5287 |
10% | 81.49 | ± | 0.7801 | 82.12 | ± | 0.7805 |
P-value | - | 9.40E-01 | ||||
Time cost | 2195.05 s | 3312.37 s |
Receptive field | 2*3 | 3*3 | ||||
Noise | Mean(%) | ± | SD | Mean(%) | ± | SD |
1% | 99.99 | ± | 0.0149 | 100.00 | ± | 0.0098 |
2% | 99.91 | ± | 0.0572 | 99.84 | ± | 0.0507 |
3% | 99.65 | ± | 0.1274 | 99.72 | ± | 0.1222 |
4% | 99.27 | ± | 0.1609 | 99.40 | ± | 0.1699 |
5% | 98.78 | ± | 0.2088 | 98.84 | ± | 0.1891 |
6% | 98.32 | ± | 0.2011 | 98.20 | ± | 0.2344 |
7% | 97.63 | ± | 0.1843 | 97.64 | ± | 0.3094 |
8% | 97.04 | ± | 0.2397 | 97.56 | ± | 0.3291 |
9% | 96.41 | ± | 0.3563 | 96.56 | ± | 0.2679 |
10% | 95.69 | ± | 0.3067 | 95.72 | ± | 0.3724 |
P-value | - | 9.06E-01 | ||||
Time cost | 2214.19 s | 3379.97 s |
Receptive field | 2*3 | 3*3 | ||||
Noise | Mean(%) | ± | SD | Mean(%) | ± | SD |
1% | 99.99 | ± | 0.0181 | 100.00 | ± | 0.0071 |
2% | 99.81 | ± | 0.0696 | 99.84 | ± | 2.7832 |
3% | 99.43 | ± | 0.1429 | 99.56 | ± | 0.6968 |
4% | 98.85 | ± | 0.1799 | 99.00 | ± | 0.1933 |
5% | 98.16 | ± | 0.2300 | 98.24 | ± | 0.1582 |
6% | 97.41 | ± | 0.3432 | 97.32 | ± | 0.2833 |
7% | 96.71 | ± | 0.3401 | 96.36 | ± | 0.3413 |
8% | 95.70 | ± | 0.3142 | 95.60 | ± | 0.3461 |
9% | 94.91 | ± | 0.3150 | 95.08 | ± | 0.3803 |
10% | 93.77 | ± | 0.3777 | 94.00 | ± | 0.3815 |
P-value | - | 9.84E-01 | ||||
Time cost | 2335.16 s | 3583.72 s |
A set of experiments on the orientation detection of natural objects is added in this section to further validate the confidence level of the PODM. This dataset contains 50 images of size 100*100 with objects such as pens of various colors, contrails of airplanes, elongated stars, elongated drops of water, etc. The orientation of these objects in the images also varies, and there are various light disturbances in the background of the images because of the shooting angle. This imposes some demands on the ability of the PODM to detect the orientation. Meanwhile, to further evaluate the performance of the model, we conducted a downsampling operation on all the images in this dataset, and the size of the images after the operation became 50*50. The image downsampling operation usually reduces the image quality while decreasing the image size, so it can perfectly reproduce the actual quality reduction process of the image delivery. Some of the examples are shown in Figure 12, and the detection results on both the original images and downsampled images are displayed in Table 11. It can be seen that the PODM still achieves 100% correctness in dealing with these practical problems, which proves that the proposed model is also effective in dealing with practical problems and that the stability of getting correct results can be trusted.
Angle | 0° | 45° | 90° | 135° | Total |
Original images | 16 | 10 | 13 | 11 | 50 |
Predicted number | 16 | 10 | 13 | 11 | 50 |
Accuracy rate | 100% | 100% | 100% | 100% | 100% |
Downsampled images | 16 | 10 | 13 | 11 | 50 |
Predicted number | 16 | 10 | 13 | 11 | 50 |
Accuracy rate | 100% | 100% | 100% | 100% | 100% |
The motivation of this study was to propose a perceptron-based orientation detection mechanism (PODM) inspired by the working mechanism of amacrine cells and to verify the effectiveness of the mechanism through a series of experiments. As color images are usually superimposed by multiple channels of grayscale images, it is reasonable to believe that the successful orientation detection of the classical monochrome grayscale image means the mechanism will also be effective for object orientation detection in color images. Images with gradient grayscale backgrounds can restore the state of actual objects to some extent, and the successful detection of object orientation in such images verifies that the mechanism is also competent for actual object orientation recognition. To further corroborate the effectiveness of the mechanism, it is compared with CNN, the state-of-the-art method in image recognition processing. Different levels of noise are added to the dataset to compare the accuracy of both methods for object orientation recognition under the same interference conditions. The results also confirm that the PODM is superior to the CNN in terms of accuracy, noise tolerance and robustness. Because each feature of the object in the dataset is randomly generated, it can be concluded that the orientation detection of the object by the PODM is always efficient and consistent, regardless of the color, size or shape of the object. The detection results of PODM for noise-affected images closely resemble the perception of the human eye, and we are confident that this model can account for the functioning of the human visual system. In other words, it can give biologists a fresh perspective when conducting research on the visual system.
The authors declare there is no conflict of interest.
[1] |
Kylili A, Fokaides PA (2017) Policy trends for the sustainability assessment of construction materials: A review. Sustain Cities Soc 35: 280–288. doi: 10.1016/j.scs.2017.08.013
![]() |
[2] |
Häfliger IF, John V, Passer A, et al. (2017) Buildings environmental impacts' sensitivity related to LCA modelling choices of construction materials. J Clean Prod 156: 805–816. doi: 10.1016/j.jclepro.2017.04.052
![]() |
[3] | Pontikes Y, Snellings R (2014) Chapter 16 - Cementitious Binders Incorporating Residues, Boston, Elsevier, 219–229. |
[4] | Panesar DK (2019) Supplementary cementing materials, Developments in the Formulation and Reinforcement of Concrete, Elsevier, 55–85. |
[5] |
Di Filippo J, Karpman J, DeShazo JR (2019) The impacts of policies to reduce CO2 emissions within the concrete supply chain. Cem Concr Compos 101: 67–82. doi: 10.1016/j.cemconcomp.2018.08.003
![]() |
[6] | Myhre G, Shindell D, Bréon FM, et al. (2013) Anthropogenic and Natural Radiative Forcing, In: Stocker TF, Qin D, Plattner G-K, et al. (Eds.), Climate Change 2013: The Physical Science Basis. Contribution of Working Group I to the Fifth Assessment Report of the Intergovernmental Panel on Climate Change, Cambridge, UK, Cambridge University Press, 659–740. |
[7] |
Allen MR, Shine KP, Fuglestvedt JS, et al. (2018) A solution to the misrepresentations of CO2-equivalent emissions of short-lived climate pollutants under ambitious mitigation. Npj Clim Atmos Sci 1: 16. doi: 10.1038/s41612-018-0026-8
![]() |
[8] |
Dyckhoff H, Kasah T (2014) Time Horizon and Dominance in Dynamic Life Cycle Assessment. J Ind Ecol 18: 799–808. doi: 10.1111/jiec.12131
![]() |
[9] |
Pigné Y, Navarrete Gutiérrez T, Gibon T, et al. (2020) LCI METHODOLOGY AND DATABASES A tool to operationalize dynamic LCA, including time differentiation on the complete background database. Int J Life Cycle Assess 25: 267–279. doi: 10.1007/s11367-019-01696-6
![]() |
[10] |
Reap J, Roman F, Duncan S, et al. (2008) A survey of unresolved problems in life cycle assessment. Int J Life Cycle Assess 13: 374. doi: 10.1007/s11367-008-0009-9
![]() |
[11] |
Shimako AH, Tiruta-Barna L, Bisinella de Faria AB, et al. (2018) Sensitivity analysis of temporal parameters in a dynamic LCA framework. Sci Total Environ 624: 1250–1262. doi: 10.1016/j.scitotenv.2017.12.220
![]() |
[12] |
Levasseur A, Lesage P, Margni M, et al. (2010) Considering Time in LCA: Dynamic LCA and Its Application to Global Warming Impact Assessments. Environ Sci Technol 44: 3169–3174. doi: 10.1021/es9030003
![]() |
[13] |
Levasseur A, Lesage P, Margni M, et al. (2013) Biogenic Carbon and Temporary Storage Addressed with Dynamic Life Cycle Assessment. J Ind Ecol 17: 117–128. doi: 10.1111/j.1530-9290.2012.00503.x
![]() |
[14] |
Pasupathy K, Berndt M, Castel A, et al. (2016) Carbonation of a blended slag-fly ash geopolymer concrete in field conditions after 8 years. Constr Build Mater 125: 661–669. doi: 10.1016/j.conbuildmat.2016.08.078
![]() |
[15] |
Collinge WO, Landis AE, Jones AK, et al. (2013) Dynamic life cycle assessment: framework and application to an institutional building. Int J Life Cycle Assess 18: 538–552. doi: 10.1007/s11367-012-0528-2
![]() |
[16] |
Su S, Li X, Zhu Y, et al. (2017) Dynamic LCA Framework for Environmental Impact Assessment of Buildings. Energy Build 149: 310–320. doi: 10.1016/j.enbuild.2017.05.042
![]() |
[17] |
Hu M (2018) Dynamic life cycle assessment integrating value choice and temporal factors—A case study of an elementary school. Energy Build 158: 1087–1096. doi: 10.1016/j.enbuild.2017.10.043
![]() |
[18] |
Negishi K, Lebert A, Almeida D, et al. (2019) Evaluating climate change pathways through a building's lifecycle based on Dynamic Life Cycle Assessment. Build Environ 164: 106377. doi: 10.1016/j.buildenv.2019.106377
![]() |
[19] |
Resch E, Andresen I, Cherubini F, et al. (2021) Estimating dynamic climate change effects of material use in buildings—Timing, uncertainty, and emission sources. Build Environ 187: 107399. doi: 10.1016/j.buildenv.2020.107399
![]() |
[20] |
Mastrucci A, Marvuglia A, Benetto E, et al. (2020) A spatio-temporal life cycle assessment framework for building renovation scenarios at the urban scale. Renew Sustain Energy Rev 126: 109834. doi: 10.1016/j.rser.2020.109834
![]() |
[21] |
Fouquet M, Levasseur A, Margni M, et al. (2015) Methodological challenges and developments in LCA of low energy buildings: Application to biogenic carbon and global warming assessment. Build Environ 90: 51–59. doi: 10.1016/j.buildenv.2015.03.022
![]() |
[22] |
Tiruta-Barna L, Pigné Y, Navarrete Gutiérrez T, et al. (2016) Framework and computational tool for the consideration of time dependency in Life Cycle Inventory: proof of concept. J Clean Prod 116: 198–206. doi: 10.1016/j.jclepro.2015.12.049
![]() |
[23] |
Fouquet M, Levasseur A, Margni M, et al. (2015) Methodological challenges and developments in LCA of low energy buildings: Application to biogenic carbon and global warming assessment. Build Environ 90: 51–59. doi: 10.1016/j.buildenv.2015.03.022
![]() |
[24] |
Su S, Zhang H, Zuo J, et al. (2021) Assessment models and dynamic variables for dynamic life cycle assessment of buildings: a review. Environ Sci Pollut Res 28: 26199–26214. doi: 10.1007/s11356-021-13614-1
![]() |
[25] |
Beloin-Saint-Pierre D, Albers A, Hélias A, et al. (2020) Addressing temporal considerations in life cycle assessment. Sci Total Environ 743: 140700. doi: 10.1016/j.scitotenv.2020.140700
![]() |
[26] | IPCC (2014) AR5 Climate Change 2014: Mitigation of Climate Change — IPCC, 2014. Available from: https://www.ipcc.ch/report/ar5/wg3/. |
[27] |
Shine KP (2009) The global warming potential—the need for an interdisciplinary retrial. Clim Change 96: 467–472. doi: 10.1007/s10584-009-9647-6
![]() |
[28] | IPCC (2013) Climate Change 2013 The Physical Science Basis Working Group I Contribution To The Fifth Assessment Report of The Intergovernmental Panel On Climate Change Wg I In T Ergov Ernmenta L Pa Nel On climate change, United Kingdom and New York, NY, USA. |
[29] |
Ismael MR, Carvalho JM (2003) Iron recovery from sulphate leach liquors in zinc hydrometallurgy. Miner Eng 16: 31–39. doi: 10.1016/S0892-6875(02)00310-2
![]() |
[30] |
Yue T, Xu Z, Hu Y, et al. (2018) Magnetic Separation and Recycling of Goethite and Calcium Sulfate in Zinc Hydrometallurgy in the Presence of Maghemite Fine Particles. ACS Sustainable Chem Eng 6: 1532–1538. doi: 10.1021/acssuschemeng.7b03856
![]() |
[31] |
Di Maria A, Van Acker K (2018) Turning Industrial Residues into Resources: An Environmental Impact Assessment of Goethite Valorization. Engineering 4: 421–429. doi: 10.1016/j.eng.2018.05.008
![]() |
[32] |
Yue T, Niu Z, Tao H, et al. (2019) Green Recycling of Goethite and Gypsum Residues in Hydrometallurgy with α-Fe 3 O 4 and γ-Fe 2 O 3 Nanoparticles: Application, Characterization, and DFT Calculation. ACS Sustainable Chem Eng 7: 6821–6829. doi: 10.1021/acssuschemeng.8b06142
![]() |
[33] |
Van Roosendael S, Roosen J, Banerjee D, et al. (2019) Selective recovery of germanium from iron-rich solutions using a supported ionic liquid phase (SILP). Sep Purif Technol 221: 83–92. doi: 10.1016/j.seppur.2019.03.068
![]() |
[34] |
Szewczuk-Karpisz K, Krasucka P, Boguta P, et al. (2019) Anionic polyacrylamide efficiency in goethite removal from aqueous solutions: goethite suspension destabilization by PAM. Int J Environ Sci Technol 16: 3145–3154. doi: 10.1007/s13762-018-2064-5
![]() |
[35] |
Van Roosendael S, Regadío M, Roosen J, et al. (2019) Selective recovery of indium from iron-rich solutions using an Aliquat 336 iodide supported ionic liquid phase (SILP). Sep Purif Technol 212: 843–853. doi: 10.1016/j.seppur.2018.11.092
![]() |
[36] |
Rodriguez Rodriguez N, Onghena B, Binnemans K (2019) Recovery of Lead and Silver from Zinc Leaching Residue Using Methanesulfonic Acid. ACS Sustainable Chem Eng 7: 19807–19815. doi: 10.1021/acssuschemeng.9b05116
![]() |
[37] |
Rodriguez Rodriguez N, Machiels L, Onghena B, et al. (2020) Selective recovery of zinc from goethite residue in the zinc industry using deep-eutectic solvents. RSC Adv 10: 7328–7335. doi: 10.1039/D0RA00277A
![]() |
[38] |
Abo Atia T, Spooren J (2020) Microwave assisted alkaline roasting-water leaching for the valorisation of goethite sludge from zinc refining process. Hydrometallurgy 191: 105235. doi: 10.1016/j.hydromet.2019.105235
![]() |
[39] |
Wang Z, Liu Y, Qu Z, et al. (2021) In situ conversion of goethite to erdite nanorods to improve the performance of doxycycline hydrochloride adsorption. Colloids Surfaces A Physicochem Eng Asp 614: 126132. doi: 10.1016/j.colsurfa.2021.126132
![]() |
[40] |
Huda N, Naser J, Brooks G, et al. (2012) Computational Fluid Dynamic Modeling of Zinc Slag Fuming Process in Top-Submerged Lance Smelting Furnace. Metall Mater Trans B 43: 39–55. doi: 10.1007/s11663-011-9558-6
![]() |
[41] | Nagraj S, Chintinne M, Guo M, et al. (2020) A Dynamic Model of a Submerged Plasma Slag Fuming Process, In: Minerals, Metals and Materials Series, Springer, 237–245. |
[42] | Verscheure K, Van Camp M, Blanpain B, et al. (2007) Continuous Fuming of Zinc-Bearing Residues: Part I. Model Development. Metall Mater Trans B 38: 13–20. |
[43] | Verscheure K, Camp M Van, Blanpain B, et al. (2007) Continuous Fuming of Zinc-Bearing Residues: Part II. The Submerged-Plasma Zinc-Fuming Process. Metall Mater Trans B 38: 21–33. |
[44] |
Alemán JV, Chadwick AV, He J, et al. (2009) Definitions of terms relating to the structure and processing of sols, gels, networks, and inorganic-organic hybrid materials (IUPAC Recommendations 2007). Pure Appl Chem 79: 1801. doi: 10.1351/pac200779101801
![]() |
[45] |
van Deventer JSJ, Provis JL, Duxson P, et al. (2010) Chemical Research and Climate Change as Drivers in the Commercial Adoption of Alkali Activated Materials. Waste Biomass Valorization 1: 145–155. doi: 10.1007/s12649-010-9015-9
![]() |
[46] |
Sofilić T, Rastovčan-Mioč A, Cerjan-Stefanović Š, et al. (2004) Characterization of steel mill electric-arc furnace dust. J Hazard Mater 109: 59–70. doi: 10.1016/j.jhazmat.2004.02.032
![]() |
[47] | Rosales J, Agrela F, Entrenas JA, et al. (2020) Potential of stainless steel slag waste in manufacturing self-compacting concrete. Materials (Basel) 13. |
[48] |
Wang X, Geysen D, Van Gerven T, et al. (2017) Characterization of landfilled stainless steel slags in view of metal recovery. Front Chem Sci Eng 11: 353–362. doi: 10.1007/s11705-017-1656-9
![]() |
[49] |
Adamczyk B, Brenneis R, Adam C, et al. (2010) Recovery of Chromium from AOD-Converter Slags. Steel Res Int 81: 1078–1083. doi: 10.1002/srin.201000193
![]() |
[50] |
Durinck D, Engström F, Arnout S, et al. (2008) Hot stage processing of metallurgical slags. Resour Conserv Recycl 52: 1121–1131. doi: 10.1016/j.resconrec.2008.07.001
![]() |
[51] |
Durinck D, Arnout S, Mertens G, et al. (2008) Borate Distribution in Stabilized Stainless-Steel Slag. J Am Ceram Soc 91: 548–554. doi: 10.1111/j.1551-2916.2007.02147.x
![]() |
[52] |
Salman M, Cizer Ö, Pontikes Y, et al. (2014) Effect of accelerated carbonation on AOD stainless steel slag for its valorisation as a CO2-sequestering construction material. Chem Eng J 246: 39–52. doi: 10.1016/j.cej.2014.02.051
![]() |
[53] |
Salman M, Dubois M, Di Maria A, et al. (2016) Construction Materials from Stainless Steel Slags: Technical Aspects, Environmental Benefits, and Economic Opportunities. J Ind Ecol 20: 854–866. doi: 10.1111/jiec.12314
![]() |
[54] | Di Maria A, Salman M, Dubois M, et al. (2018) Life cycle assessment to evaluate the environmental performance of new construction material from stainless steel slag. Int J Life Cycle Assess 1–19. |
[55] |
Iacobescu RI, Angelopoulos GN, Jones PT, et al. (2016) Ladle metallurgy stainless steel slag as a raw material in Ordinary Portland Cement production: a possibility for industrial symbiosis. J Clean Prod 112: 872–881. doi: 10.1016/j.jclepro.2015.06.006
![]() |
[56] | Provis J, van Deventer J (2014) Alkali Activated Materials - State-of-the-Art Report, RILEM TC, John Provis, Springer. |
[57] | Pommer K, Pade C (2006) Guidelines-uptake of carbon dioxide in the life cycle inventory of concrete, Oslo, Norway, Dansk Teknologisk Institut, Nordic Innovation Centre. |
[58] | European Commision (2015) Screening template for Construction and Demolition Waste management in Belgium. |
[59] |
Di Maria A, Eyckmans J, Van Acker K (2018) Downcycling versus recycling of construction and demolition waste: Combining LCA and LCC to support sustainable policy making. Waste Manag 75: 3–21. doi: 10.1016/j.wasman.2018.01.028
![]() |
[60] | Martaud T (2008) Evaluation environnementale de la production de granulats en exploitation de carrières. Indicateurs, Modèles et Outils, Géologie appliquée, Université d'Orléans. |
[61] | Mroueh U-M, Eskola P, Laine-Ylijoki J, et al. (2000) Life cycle assessment of road construction, Helsinki, Finland. |
[62] | Dewar J (2003) Concrete mix design, In: Newman JB, Choo BS (Eds.), Advanced concrete technology, Oxford, Butterworth-Heinemann, 3–40. |
[63] | Kjellsen KO, Guimaraes M, Nilsson A (2005) The CO2 Balance of Concrete in a Life Cycle Perspective, Oslo, Norway. |
[64] | Lagerblad B (2006) CO2 uptake during concrete life cycle- state of the art, Oslo, Norway. |
[65] | Pommer K, Pade C (2006) Guidelines-uptake of carbon dioxide in the life cycle inventory of concrete, Oslo, Norway. |
[66] | Kellemberg D, Althaus HJ, Kunninger T, et al. (2007) Life Cycle Inventories of Building Products. Ecoinvent report No. 7, Dübendorf. |
[67] |
Castellote M, Fernandez L, Andrade C, et al. (2009) Chemical changes and phase analysis of OPC pastes carbonated at different CO2 concentrations. Mater Struct 42: 515–525. doi: 10.1617/s11527-008-9399-1
![]() |
[68] |
Van Deventer JSJ, Provis JL, Duxson P (2012) Technical and commercial progress in the adoption of geopolymer cement. Miner Eng 29: 89–104. doi: 10.1016/j.mineng.2011.09.009
![]() |
[69] |
Bernal SA, Provis JL, Brice DG, et al. (2012) Accelerated carbonation testing of alkali-activated binders significantly underestimates service life: The role of pore solution chemistry.Cem Concr Res 42: 1317–1326. doi: 10.1016/j.cemconres.2012.07.002
![]() |
[70] |
Gruskovnjak A, Lothenbach B, Holzer L, et al. (2006) Hydration of alkali-activated slag: comparison with ordinary Portland cement. Adv Cem Res 18: 119–128. doi: 10.1680/adcr.2006.18.3.119
![]() |
[71] | Adam A (2009) Strength and durability properties of alkali activated slag and fly ash-based geopolymer concrete, Australia, RMIT University Melbourne. |
[72] |
Bakharev T, Sanjayan JG, Cheng YB (2001) Resistance of alkali-activated slag concrete to carbonation. Cem Concr Res 31: 1277–1283. doi: 10.1016/S0008-8846(01)00574-9
![]() |
[73] |
Ul Haq E, Padmanabhan SK, Licciulli A (2014) In-situ carbonation of alkali activated fly ash geopolymer. Constr Build Mater 66: 781–786. doi: 10.1016/j.conbuildmat.2014.06.012
![]() |
1. | Ratnesh Kumar Dubey, Dilip Kumar Choubey, 2024, Chapter 34, 978-981-97-2613-4, 485, 10.1007/978-981-97-2614-1_34 | |
2. | Syed Khasim, Irfan Sadiq Rahat, Hritwik Ghosh, Kareemulla Shaik, Sujit Kumar Panda, Using Deep Learning and Machine Learning: Real-Time Discernment and Diagnostics of Rice-Leaf Diseases in Bangladesh, 2023, 10, 2414-1399, 10.4108/eetiot.4579 | |
3. | Hussain. A, Balaji Srikaanth. P, 2023, VGG19 Enhanced Convolutional Neural Network for Paddy Leaf Disease Detection, 979-8-3503-2284-2, 840, 10.1109/ICPCSN58827.2023.00144 | |
4. | R. Senthil, Ravi Khatwal, An Effective Disease Detection Analysis on Rice Leaves Using Hybrid MCSVM-DNN Predictor Architecture, 2024, 2520-8195, 10.1007/s41976-024-00175-3 | |
5. | Yasmin M. Alsakar, Nehal A. Sakr, Mohammed Elmogy, An enhanced classification system of various rice plant diseases based on multi-level handcrafted feature extraction technique, 2024, 14, 2045-2322, 10.1038/s41598-024-81143-1 |
Object Size | Angle | 0° | 45° | 90° | 135° | Total |
50 | Number of images | 646 | 608 | 627 | 619 | 2500 |
Predicted number | 646 | 608 | 627 | 619 | 2500 | |
Accuracy rate | 100% | 100% | 100% | 100% | 100% | |
100 | Number of images | 641 | 589 | 644 | 626 | 2500 |
Predicted number | 641 | 589 | 644 | 626 | 2500 | |
Accuracy rate | 100% | 100% | 100% | 100% | 100% | |
500 | Number of images | 634 | 646 | 585 | 635 | 2500 |
Predicted number | 634 | 646 | 585 | 635 | 2500 | |
Accuracy rate | 100% | 100% | 100% | 100% | 100% | |
1000 | Number of images | 600 | 623 | 643 | 634 | 2500 |
Predicted number | 600 | 623 | 643 | 634 | 2500 | |
Accuracy rate | 100% | 100% | 100% | 100% | 100% |
Object Size | Angle | 0° | 45° | 90° | 135° | Total |
50 | Number of images | 580 | 647 | 653 | 620 | 2500 |
Predicted number | 580 | 647 | 653 | 620 | 2500 | |
Accuracy rate | 100% | 100% | 100% | 100% | 100% | |
100 | Number of images | 611 | 635 | 648 | 606 | 2500 |
Predicted number | 611 | 635 | 648 | 606 | 2500 | |
Accuracy rate | 100% | 100% | 100% | 100% | 100% | |
500 | Number of images | 602 | 602 | 670 | 626 | 2500 |
Predicted numbers | 602 | 602 | 670 | 626 | 2500 | |
Accuracy rate | 100% | 100% | 100% | 100% | 100% | |
1000 | Number of images | 642 | 616 | 591 | 651 | 2500 |
Predicted number | 642 | 616 | 591 | 651 | 2500 | |
Accuracy rate | 100% | 100% | 100% | 100% | 100% |
Noise | PODM | CNN | ||||
Mean(%) | ± | SD | Mean(%) | ± | SD | |
1% | 99.99 | ± | 0.0149 | 67.83 | ± | 0.1596 |
2% | 99.40 | ± | 0.1228 | 52.71 | ± | 0.1674 |
3% | 97.16 | ± | 0.3379 | 45.52 | ± | 0.1447 |
4% | 92.95 | ± | 0.4359 | 41.59 | ± | 0.1298 |
5% | 87.69 | ± | 0.7140 | 41.61 | ± | 0.1813 |
6% | 81.84 | ± | 0.5256 | 35.97 | ± | 0.1021 |
7% | 76.59 | ± | 0.7738 | 33.96 | ± | 0.1017 |
8% | 70.99 | ± | 0.7243 | 31.58 | ± | 0.1052 |
9% | 66.31 | ± | 0.8904 | 30.90 | ± | 0.0748 |
10% | 62.28 | ± | 0.7972 | 32.30 | ± | 0.0889 |
P-value | - | 8.64E-07 |
Noise | PODM | CNN | ||||
Mean(%) | ± | SD | Mean(%) | ± | SD | |
1% | 99.98 | ± | 0.0267 | 93.03 | ± | 0.0236 |
2% | 99.54 | ± | 0.1267 | 90.87 | ± | 0.0399 |
3% | 98.60 | ± | 0.2016 | 85.30 | ± | 0.1019 |
4% | 96.99 | ± | 0.2789 | 84.34 | ± | 0.1290 |
5% | 95.34 | ± | 0.3437 | 79.01 | ± | 0.1528 |
6% | 93.11 | ± | 0.4067 | 77.87 | ± | 0.1796 |
7% | 90.43 | ± | 0.4366 | 78.39 | ± | 0.1530 |
8% | 87.91 | ± | 0.6577 | 76.80 | ± | 0.1522 |
9% | 84.91 | ± | 0.5372 | 72.51 | ± | 0.1787 |
10% | 82.16 | ± | 0.7730 | 75.30 | ± | 0.1598 |
P-value | - | 9.53E-04 |
Noise | PODM | CNN | ||||
Mean(%) | ± | SD | Mean(%) | ± | SD | |
1% | 100.00 | ± | 0.0100 | 97.58 | ± | 0.0067 |
2% | 99.94 | ± | 0.0503 | 97.17 | ± | 0.0068 |
3% | 99.69 | ± | 0.1204 | 96.93 | ± | 0.0072 |
4% | 99.34 | ± | 0.1709 | 95.96 | ± | 0.0121 |
5% | 98.91 | ± | 0.1891 | 96.08 | ± | 0.0092 |
6% | 98.42 | ± | 0.2377 | 95.80 | ± | 0.0078 |
7% | 97.81 | ± | 0.3068 | 95.25 | ± | 0.0084 |
8% | 97.19 | ± | 0.3345 | 95.26 | ± | 0.0063 |
9% | 96.52 | ± | 0.2720 | 94.83 | ± | 0.0100 |
10% | 95.74 | ± | 0.3695 | 94.67 | ± | 0.0077 |
P-value | - | 5.13E-04 |
Noise | PODM | CNN | ||||
Mean(%) | ± | SD | Mean(%) | ± | SD | |
1% | 99.99 | ± | 0.0072 | 96.71 | ± | 0.0065 |
2% | 99.81 | ± | 2.8279 | 96.49 | ± | 0.0055 |
3% | 99.43 | ± | 0.7075 | 96.29 | ± | 0.0070 |
4% | 98.85 | ± | 0.1964 | 96.13 | ± | 0.0064 |
5% | 98.16 | ± | 0.1602 | 95.85 | ± | 0.0101 |
6% | 97.41 | ± | 0.2816 | 95.66 | ± | 0.0058 |
7% | 96.71 | ± | 0.3358 | 95.90 | ± | 0.0075 |
8% | 95.70 | ± | 0.3497 | 95.43 | ± | 0.0115 |
9% | 94.91 | ± | 0.3863 | 95.93 | ± | 0.0087 |
10% | 93.77 | ± | 0.3876 | 95.58 | ± | 0.0096 |
P-value | - | 4.76E-02 |
Receptive field | 2*3 | 3*3 | ||||
Noise | Mean(%) | ± | SD | Mean(%) | ± | SD |
1% | 99.98 | ± | 0.0246 | 100.00 | ± | 0.0147 |
2% | 99.17 | ± | 0.1496 | 99.36 | ± | 0.1210 |
3% | 96.42 | ± | 0.3577 | 96.96 | ± | 0.3363 |
4% | 92.11 | ± | 0.6329 | 92.80 | ± | 0.4348 |
5% | 86.64 | ± | 0.5642 | 88.00 | ± | 0.7038 |
6% | 80.74 | ± | 0.9089 | 81.68 | ± | 0.5230 |
7% | 75.04 | ± | 0.8508 | 77.48 | ± | 0.7652 |
8% | 70.24 | ± | 0.8117 | 71.56 | ± | 0.7256 |
9% | 65.31 | ± | 0.8048 | 66.24 | ± | 0.8806 |
10% | 61.68 | ± | 0.9211 | 62.28 | ± | 0.7914 |
P-value | - | 8.84E-01 | ||||
Time cost | 2193.02 s | 3378.61 s |
Receptive field | 2*3 | 3*3 | ||||
Noise | Mean(%) | ± | SD | Mean(%) | ± | SD |
1% | 99.95 | ± | 0.0377 | 99.96 | ± | 0.0266 |
2% | 99.39 | ± | 0.1232 | 99.72 | ± | 0.1251 |
3% | 98.23 | ± | 0.2248 | 98.68 | ± | 0.1983 |
4% | 96.71 | ± | 0.3103 | 97.00 | ± | 0.2752 |
5% | 94.97 | ± | 0.3515 | 95.08 | ± | 0.3446 |
6% | 92.70 | ± | 0.4397 | 92.88 | ± | 0.4001 |
7% | 90.19 | ± | 0.4157 | 90.44 | ± | 0.4311 |
8% | 87.31 | ± | 0.6059 | 87.44 | ± | 0.6692 |
9% | 84.57 | ± | 0.5317 | 84.36 | ± | 0.5287 |
10% | 81.49 | ± | 0.7801 | 82.12 | ± | 0.7805 |
P-value | - | 9.40E-01 | ||||
Time cost | 2195.05 s | 3312.37 s |
Receptive field | 2*3 | 3*3 | ||||
Noise | Mean(%) | ± | SD | Mean(%) | ± | SD |
1% | 99.99 | ± | 0.0149 | 100.00 | ± | 0.0098 |
2% | 99.91 | ± | 0.0572 | 99.84 | ± | 0.0507 |
3% | 99.65 | ± | 0.1274 | 99.72 | ± | 0.1222 |
4% | 99.27 | ± | 0.1609 | 99.40 | ± | 0.1699 |
5% | 98.78 | ± | 0.2088 | 98.84 | ± | 0.1891 |
6% | 98.32 | ± | 0.2011 | 98.20 | ± | 0.2344 |
7% | 97.63 | ± | 0.1843 | 97.64 | ± | 0.3094 |
8% | 97.04 | ± | 0.2397 | 97.56 | ± | 0.3291 |
9% | 96.41 | ± | 0.3563 | 96.56 | ± | 0.2679 |
10% | 95.69 | ± | 0.3067 | 95.72 | ± | 0.3724 |
P-value | - | 9.06E-01 | ||||
Time cost | 2214.19 s | 3379.97 s |
Receptive field | 2*3 | 3*3 | ||||
Noise | Mean(%) | ± | SD | Mean(%) | ± | SD |
1% | 99.99 | ± | 0.0181 | 100.00 | ± | 0.0071 |
2% | 99.81 | ± | 0.0696 | 99.84 | ± | 2.7832 |
3% | 99.43 | ± | 0.1429 | 99.56 | ± | 0.6968 |
4% | 98.85 | ± | 0.1799 | 99.00 | ± | 0.1933 |
5% | 98.16 | ± | 0.2300 | 98.24 | ± | 0.1582 |
6% | 97.41 | ± | 0.3432 | 97.32 | ± | 0.2833 |
7% | 96.71 | ± | 0.3401 | 96.36 | ± | 0.3413 |
8% | 95.70 | ± | 0.3142 | 95.60 | ± | 0.3461 |
9% | 94.91 | ± | 0.3150 | 95.08 | ± | 0.3803 |
10% | 93.77 | ± | 0.3777 | 94.00 | ± | 0.3815 |
P-value | - | 9.84E-01 | ||||
Time cost | 2335.16 s | 3583.72 s |
Angle | 0° | 45° | 90° | 135° | Total |
Original images | 16 | 10 | 13 | 11 | 50 |
Predicted number | 16 | 10 | 13 | 11 | 50 |
Accuracy rate | 100% | 100% | 100% | 100% | 100% |
Downsampled images | 16 | 10 | 13 | 11 | 50 |
Predicted number | 16 | 10 | 13 | 11 | 50 |
Accuracy rate | 100% | 100% | 100% | 100% | 100% |
Object Size | Angle | 0° | 45° | 90° | 135° | Total |
50 | Number of images | 646 | 608 | 627 | 619 | 2500 |
Predicted number | 646 | 608 | 627 | 619 | 2500 | |
Accuracy rate | 100% | 100% | 100% | 100% | 100% | |
100 | Number of images | 641 | 589 | 644 | 626 | 2500 |
Predicted number | 641 | 589 | 644 | 626 | 2500 | |
Accuracy rate | 100% | 100% | 100% | 100% | 100% | |
500 | Number of images | 634 | 646 | 585 | 635 | 2500 |
Predicted number | 634 | 646 | 585 | 635 | 2500 | |
Accuracy rate | 100% | 100% | 100% | 100% | 100% | |
1000 | Number of images | 600 | 623 | 643 | 634 | 2500 |
Predicted number | 600 | 623 | 643 | 634 | 2500 | |
Accuracy rate | 100% | 100% | 100% | 100% | 100% |
Object Size | Angle | 0° | 45° | 90° | 135° | Total |
50 | Number of images | 580 | 647 | 653 | 620 | 2500 |
Predicted number | 580 | 647 | 653 | 620 | 2500 | |
Accuracy rate | 100% | 100% | 100% | 100% | 100% | |
100 | Number of images | 611 | 635 | 648 | 606 | 2500 |
Predicted number | 611 | 635 | 648 | 606 | 2500 | |
Accuracy rate | 100% | 100% | 100% | 100% | 100% | |
500 | Number of images | 602 | 602 | 670 | 626 | 2500 |
Predicted numbers | 602 | 602 | 670 | 626 | 2500 | |
Accuracy rate | 100% | 100% | 100% | 100% | 100% | |
1000 | Number of images | 642 | 616 | 591 | 651 | 2500 |
Predicted number | 642 | 616 | 591 | 651 | 2500 | |
Accuracy rate | 100% | 100% | 100% | 100% | 100% |
Noise | PODM | CNN | ||||
Mean(%) | ± | SD | Mean(%) | ± | SD | |
1% | 99.99 | ± | 0.0149 | 67.83 | ± | 0.1596 |
2% | 99.40 | ± | 0.1228 | 52.71 | ± | 0.1674 |
3% | 97.16 | ± | 0.3379 | 45.52 | ± | 0.1447 |
4% | 92.95 | ± | 0.4359 | 41.59 | ± | 0.1298 |
5% | 87.69 | ± | 0.7140 | 41.61 | ± | 0.1813 |
6% | 81.84 | ± | 0.5256 | 35.97 | ± | 0.1021 |
7% | 76.59 | ± | 0.7738 | 33.96 | ± | 0.1017 |
8% | 70.99 | ± | 0.7243 | 31.58 | ± | 0.1052 |
9% | 66.31 | ± | 0.8904 | 30.90 | ± | 0.0748 |
10% | 62.28 | ± | 0.7972 | 32.30 | ± | 0.0889 |
P-value | - | 8.64E-07 |
Noise | PODM | CNN | ||||
Mean(%) | ± | SD | Mean(%) | ± | SD | |
1% | 99.98 | ± | 0.0267 | 93.03 | ± | 0.0236 |
2% | 99.54 | ± | 0.1267 | 90.87 | ± | 0.0399 |
3% | 98.60 | ± | 0.2016 | 85.30 | ± | 0.1019 |
4% | 96.99 | ± | 0.2789 | 84.34 | ± | 0.1290 |
5% | 95.34 | ± | 0.3437 | 79.01 | ± | 0.1528 |
6% | 93.11 | ± | 0.4067 | 77.87 | ± | 0.1796 |
7% | 90.43 | ± | 0.4366 | 78.39 | ± | 0.1530 |
8% | 87.91 | ± | 0.6577 | 76.80 | ± | 0.1522 |
9% | 84.91 | ± | 0.5372 | 72.51 | ± | 0.1787 |
10% | 82.16 | ± | 0.7730 | 75.30 | ± | 0.1598 |
P-value | - | 9.53E-04 |
Noise | PODM | CNN | ||||
Mean(%) | ± | SD | Mean(%) | ± | SD | |
1% | 100.00 | ± | 0.0100 | 97.58 | ± | 0.0067 |
2% | 99.94 | ± | 0.0503 | 97.17 | ± | 0.0068 |
3% | 99.69 | ± | 0.1204 | 96.93 | ± | 0.0072 |
4% | 99.34 | ± | 0.1709 | 95.96 | ± | 0.0121 |
5% | 98.91 | ± | 0.1891 | 96.08 | ± | 0.0092 |
6% | 98.42 | ± | 0.2377 | 95.80 | ± | 0.0078 |
7% | 97.81 | ± | 0.3068 | 95.25 | ± | 0.0084 |
8% | 97.19 | ± | 0.3345 | 95.26 | ± | 0.0063 |
9% | 96.52 | ± | 0.2720 | 94.83 | ± | 0.0100 |
10% | 95.74 | ± | 0.3695 | 94.67 | ± | 0.0077 |
P-value | - | 5.13E-04 |
Noise | PODM | CNN | ||||
Mean(%) | ± | SD | Mean(%) | ± | SD | |
1% | 99.99 | ± | 0.0072 | 96.71 | ± | 0.0065 |
2% | 99.81 | ± | 2.8279 | 96.49 | ± | 0.0055 |
3% | 99.43 | ± | 0.7075 | 96.29 | ± | 0.0070 |
4% | 98.85 | ± | 0.1964 | 96.13 | ± | 0.0064 |
5% | 98.16 | ± | 0.1602 | 95.85 | ± | 0.0101 |
6% | 97.41 | ± | 0.2816 | 95.66 | ± | 0.0058 |
7% | 96.71 | ± | 0.3358 | 95.90 | ± | 0.0075 |
8% | 95.70 | ± | 0.3497 | 95.43 | ± | 0.0115 |
9% | 94.91 | ± | 0.3863 | 95.93 | ± | 0.0087 |
10% | 93.77 | ± | 0.3876 | 95.58 | ± | 0.0096 |
P-value | - | 4.76E-02 |
Receptive field | 2*3 | 3*3 | ||||
Noise | Mean(%) | ± | SD | Mean(%) | ± | SD |
1% | 99.98 | ± | 0.0246 | 100.00 | ± | 0.0147 |
2% | 99.17 | ± | 0.1496 | 99.36 | ± | 0.1210 |
3% | 96.42 | ± | 0.3577 | 96.96 | ± | 0.3363 |
4% | 92.11 | ± | 0.6329 | 92.80 | ± | 0.4348 |
5% | 86.64 | ± | 0.5642 | 88.00 | ± | 0.7038 |
6% | 80.74 | ± | 0.9089 | 81.68 | ± | 0.5230 |
7% | 75.04 | ± | 0.8508 | 77.48 | ± | 0.7652 |
8% | 70.24 | ± | 0.8117 | 71.56 | ± | 0.7256 |
9% | 65.31 | ± | 0.8048 | 66.24 | ± | 0.8806 |
10% | 61.68 | ± | 0.9211 | 62.28 | ± | 0.7914 |
P-value | - | 8.84E-01 | ||||
Time cost | 2193.02 s | 3378.61 s |
Receptive field | 2*3 | 3*3 | ||||
Noise | Mean(%) | ± | SD | Mean(%) | ± | SD |
1% | 99.95 | ± | 0.0377 | 99.96 | ± | 0.0266 |
2% | 99.39 | ± | 0.1232 | 99.72 | ± | 0.1251 |
3% | 98.23 | ± | 0.2248 | 98.68 | ± | 0.1983 |
4% | 96.71 | ± | 0.3103 | 97.00 | ± | 0.2752 |
5% | 94.97 | ± | 0.3515 | 95.08 | ± | 0.3446 |
6% | 92.70 | ± | 0.4397 | 92.88 | ± | 0.4001 |
7% | 90.19 | ± | 0.4157 | 90.44 | ± | 0.4311 |
8% | 87.31 | ± | 0.6059 | 87.44 | ± | 0.6692 |
9% | 84.57 | ± | 0.5317 | 84.36 | ± | 0.5287 |
10% | 81.49 | ± | 0.7801 | 82.12 | ± | 0.7805 |
P-value | - | 9.40E-01 | ||||
Time cost | 2195.05 s | 3312.37 s |
Receptive field | 2*3 | 3*3 | ||||
Noise | Mean(%) | ± | SD | Mean(%) | ± | SD |
1% | 99.99 | ± | 0.0149 | 100.00 | ± | 0.0098 |
2% | 99.91 | ± | 0.0572 | 99.84 | ± | 0.0507 |
3% | 99.65 | ± | 0.1274 | 99.72 | ± | 0.1222 |
4% | 99.27 | ± | 0.1609 | 99.40 | ± | 0.1699 |
5% | 98.78 | ± | 0.2088 | 98.84 | ± | 0.1891 |
6% | 98.32 | ± | 0.2011 | 98.20 | ± | 0.2344 |
7% | 97.63 | ± | 0.1843 | 97.64 | ± | 0.3094 |
8% | 97.04 | ± | 0.2397 | 97.56 | ± | 0.3291 |
9% | 96.41 | ± | 0.3563 | 96.56 | ± | 0.2679 |
10% | 95.69 | ± | 0.3067 | 95.72 | ± | 0.3724 |
P-value | - | 9.06E-01 | ||||
Time cost | 2214.19 s | 3379.97 s |
Receptive field | 2*3 | 3*3 | ||||
Noise | Mean(%) | ± | SD | Mean(%) | ± | SD |
1% | 99.99 | ± | 0.0181 | 100.00 | ± | 0.0071 |
2% | 99.81 | ± | 0.0696 | 99.84 | ± | 2.7832 |
3% | 99.43 | ± | 0.1429 | 99.56 | ± | 0.6968 |
4% | 98.85 | ± | 0.1799 | 99.00 | ± | 0.1933 |
5% | 98.16 | ± | 0.2300 | 98.24 | ± | 0.1582 |
6% | 97.41 | ± | 0.3432 | 97.32 | ± | 0.2833 |
7% | 96.71 | ± | 0.3401 | 96.36 | ± | 0.3413 |
8% | 95.70 | ± | 0.3142 | 95.60 | ± | 0.3461 |
9% | 94.91 | ± | 0.3150 | 95.08 | ± | 0.3803 |
10% | 93.77 | ± | 0.3777 | 94.00 | ± | 0.3815 |
P-value | - | 9.84E-01 | ||||
Time cost | 2335.16 s | 3583.72 s |
Angle | 0° | 45° | 90° | 135° | Total |
Original images | 16 | 10 | 13 | 11 | 50 |
Predicted number | 16 | 10 | 13 | 11 | 50 |
Accuracy rate | 100% | 100% | 100% | 100% | 100% |
Downsampled images | 16 | 10 | 13 | 11 | 50 |
Predicted number | 16 | 10 | 13 | 11 | 50 |
Accuracy rate | 100% | 100% | 100% | 100% | 100% |