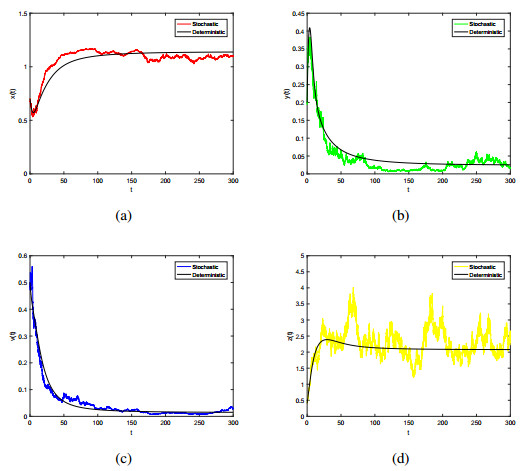
Citation: Xiaoqin Wang, Yiping Tan, Yongli Cai, Kaifa Wang, Weiming Wang. Dynamics of a stochastic HBV infection model with cell-to-cell transmission and immune response[J]. Mathematical Biosciences and Engineering, 2021, 18(1): 616-642. doi: 10.3934/mbe.2021034
[1] | Yiping Tan, Yongli Cai, Zhihang Peng, Kaifa Wang, Ruoxia Yao, Weiming Wang . Dynamics of a stochastic HBV infection model with drug therapy and immune response. Mathematical Biosciences and Engineering, 2022, 19(8): 7570-7585. doi: 10.3934/mbe.2022356 |
[2] | Ting Guo, Zhipeng Qiu . The effects of CTL immune response on HIV infection model with potent therapy, latently infected cells and cell-to-cell viral transmission. Mathematical Biosciences and Engineering, 2019, 16(6): 6822-6841. doi: 10.3934/mbe.2019341 |
[3] | Yan Wang, Tingting Zhao, Jun Liu . Viral dynamics of an HIV stochastic model with cell-to-cell infection, CTL immune response and distributed delays. Mathematical Biosciences and Engineering, 2019, 16(6): 7126-7154. doi: 10.3934/mbe.2019358 |
[4] | Jiazhe Lin, Rui Xu, Xiaohong Tian . Threshold dynamics of an HIV-1 model with both viral and cellular infections, cell-mediated and humoral immune responses. Mathematical Biosciences and Engineering, 2019, 16(1): 292-319. doi: 10.3934/mbe.2019015 |
[5] | Yan Wang, Minmin Lu, Daqing Jiang . Viral dynamics of a latent HIV infection model with Beddington-DeAngelis incidence function, B-cell immune response and multiple delays. Mathematical Biosciences and Engineering, 2021, 18(1): 274-299. doi: 10.3934/mbe.2021014 |
[6] | Ran Zhang, Shengqiang Liu . Global dynamics of an age-structured within-host viral infection model with cell-to-cell transmission and general humoral immunity response. Mathematical Biosciences and Engineering, 2020, 17(2): 1450-1478. doi: 10.3934/mbe.2020075 |
[7] | Khalid Hattaf, Noura Yousfi . Dynamics of SARS-CoV-2 infection model with two modes of transmission and immune response. Mathematical Biosciences and Engineering, 2020, 17(5): 5326-5340. doi: 10.3934/mbe.2020288 |
[8] | Jinhu Xu, Yicang Zhou . Bifurcation analysis of HIV-1 infection model with cell-to-cell transmission and immune response delay. Mathematical Biosciences and Engineering, 2016, 13(2): 343-367. doi: 10.3934/mbe.2015006 |
[9] | Cameron Browne . Immune response in virus model structured by cell infection-age. Mathematical Biosciences and Engineering, 2016, 13(5): 887-909. doi: 10.3934/mbe.2016022 |
[10] | Jiawei Deng, Ping Jiang, Hongying Shu . Viral infection dynamics with mitosis, intracellular delays and immune response. Mathematical Biosciences and Engineering, 2023, 20(2): 2937-2963. doi: 10.3934/mbe.2023139 |
Hepatitis B, including acute and chronic disease, is caused by the hepatitis B virus (HBV) infection and has become a major global health problem. World Health Organization (WHO) estimates that in 2019,325 million people globally live with hepatitis B and/or C, while in 2015,257 million people were living with chronic hepatitis B infection (defined as hepatitis B surface antigen positive), of them, 887,000 deaths, mostly from cirrhosis and hepatocellular carcinoma (i.e., primary liver cancer)[1].
Generally, cellular immunopathological reaction plays an important role in controlling HBV infection. For example, cytotoxic T lymphocytes (CTLs) can specifically attack the target infected host cells [2], which will induce hepatocyte damage. And more and more attentions have been paid to the study of virus dynamics within-host, which can provide insights into virus infection and dynamics, as well as to how an infection can be reduced or even eradicated, see [3,4,5,6,7] and the references therein.
Studies have shown that human immunodeficiency virus (HIV) and hepatitis C virus (HCV) can spread by two fundamental modes within-host, one by virus-to-cell infection through the extracellular space and the other by cell-to-cell transfer involving direct cell-to-cell contact [4,8,9,10,11,12,13,14,15,16]. Especially, Sourissea et al. [17] had reported that viral transfer in vitro via cell-to-cell contact is much more rapid and efficient than infection by free virus because it avoids several biophysical and kinetic barriers. Monel et al. [18] showed that direct cell-to-cell transmission in vivo is also more potent and more efficient. And Yang and co-authors [19] showed a previously unappreciated role of exosomes in HBV transmission and natural killer cells dysfunction during chronic hepatitis B (CHB) infection. These results showed that cell-to-cell transmission is reasonable apart from virus-to-cell mode in HBV infection because exosomes can transfer genetic material between cells. Therefore, during HBV infection, uninfected hepatocytes can be infected not only by newly released free virus, but also by contacting with infected hepatocytes. Combining with the CTLs population, to describe HBV from a single patient's point of view, we first propose the following HBV infection model:
{dxdt=λ1−d1x−β1xv−β2xy,dydt=β1xv+β2xy−ay−pyz,dvdt=ky−γv,dzdt=λ2−d2z+qyz, | (1) |
where x, y, v and z denote the total numbers of uninfected hepatocytes, infected hepatocytes, free virus and CTLs, respectively. It is assumed that normal hepatocytes are produced by bone marrow and other organs at a constant rate of λ1 and the natural death rate is d1x. β1 is the effective contact rate between uninfected hepatocytes and virus, β2 stands for the effective contact rate between uninfected and infected hepatocytes, a represents the natural death rate of the infected hepatocytes. Infected hepatocytes are eliminated by CTLs at rate pyz, free virus are produced from infected cells at rate ky and die at rate γv. CTLs are produced at a constant λ2 from the thymus, and at rate qyz due to the stimulation of infected cells [20], and die at rate d2z.
Note that there are inevitably random disturbances in the process of HBV infection within-host, such as temperature fluctuation, mood fluctuation and other physiological rhythm changes, which may affect the dynamics of HBV infection. Thus, in addition to the traditional ordinary differential equations (ODEs), such as model (1), more attention has been paid to the stochastic differential equations (SDEs) which takes Brownian motion into consideration [21,22,23,24,25,26,27,28,29,30,31] and the references therein). Concretely, [31] was about HIV infection and did not consider cell-to-cell transmission. Especially, references [5,26,32] investigated the stochastic dynamics of HBV infection. After assuming the total number of hepatocytes is constant, Bertacchi et al. [5] proposed a stochastic HBV model for the infection within a patient which was treated with two drugs, either sequentially or contemporaneously, developed a two-step mutation which was resistant to both drugs, and studied the deterministic approximation of the stochastic model and gave a biological interpretation of its asymptotic behaviour. Luzyanina and Bocharov [26] considered a reduced ODEs model which only describes the interaction between the HBV and the CTL response, and Xie et al. [32] investigated the stochastic HBV dynamics. Unfortunately, these studies had not considered the CTL response and cell-to-cell transmission in their SDE HBV model.
Thanks to the insightful work of Luzyanina and Bocharov [26], we know that the variations can affect either the replication of the virus or its elimination kinetics, e.g., via parameters β2, γ and d2, respectively. And we can explore their impacts on the dynamics of HBV infection could be the extension of the deterministic description of the virus—CTL interaction to include the stochastic forcing in a multiplicative way.
To investigate the influence of fluctuating (vs. constant) rates β2, γ and d2 on the model solutions, following [26], we randomize these parameters as follows.
Let p∈[p1,p2] be a parameter being randomized and p1,p2 are its low and upper bounds. We assume that p varies randomly according to p(t)=˜p+σpξ(t), where ˜p is the value of p around which we randomize, ξ(t) is a standard Gaussian random variable for each t and σp>0 is the intensity of the noise. We adopt σp as
σp=min(˜p−p1,p2−˜p)3 |
to ensure that p remains in the interval [p1,p2] with probability 0.997. This implies that about 99.7% of values drawn from a normal distribution are within three standard deviations σp away from the mean.
More precisely, let
β2→β2+σ1dB1(t),γ→γ+σ2dB2(t),d2→d2+σ3dB3(t), |
then, corresponding to the deterministic model (1), we can further derive the following SDE HBV model:
{dx=(λ1−d1x−β1xv−β2xy)dt−σ1xydB1(t),dy=(β1xv+β2xy−ay−pyz)dt+σ1xydB1(t),dv=(ky−γv)dt−σ2vdB2(t),dz=(λ2−d2z+qyz)dt−σ3zdB3(t), | (2) |
where σi(i=1,2,3) represent the intensities of the white noises, and Bi(t)(i=1,2,3) are independent standard Brownian motions.
It is worthy to note that, if the number of individuals in the population gets very large, Kurtz [33,34] proved that if there is a density-dependent stochastic jump process (such as the number of viral particles, the infected/uninfected hepatocytes etc..), then the process that we obtain when rescaling by a constant and large quantity can be approximated by the solution of the corresponding ODE.
The purpose of the present study is to elaborate the virus infection dynamics to random perturbations in the virus replication and immune responses parameters. In the next section, we give the global dynamics of the deterministic HBV model (1), including the basic reproduction number and global stability of infection-free equilibrium and infection equilibrium. In Section 3, we show the existence and uniqueness of the global positive solutions of the SDE HBV model (2). In Section 4, we give the asymptotic property of the positive solution of model (2) around infection-free equilibrium. And in Section 5, the dynamics of the stochastic model around infection equilibrium is obtained. In Section 6, numerical simulations of model (2) illustrate the correctness of the theoretical results and the correlation between fluctuation amplitude and intensity of the white noise is further studied. In Section 7, we discuss our new findings in the view of epidemiological implications.
We are interested in the dynamics of viral infection rather than the initial processes of infection. The initial condition of model (1) is assumed as the form x(0)>0,y(0)>0,v(0)>0 and z(0)>0. Based on the initial conditions, it is clear that the solutions of model (1) are non-negative and ultimately bounded.
Model (1) always has an infection-free equilibrium E0=(x0,0,0,z0), where x0=λ1d1,z0=λ2d2. According to the definition and algorithm of the basic reproduction number of virus in [35], we can obtain the basic reproduction number of model (1) as follows:
R0:=λ1d2(kβ1γ+β2)d1(ad2+λ2p). | (3) |
Obviously, R0 increases as β1 and β2 increasing.
After some algebraic operations, it is easy to know that, if R0>1, model (1) has an infection equilibrium E∗=(x∗,y∗,v∗,z∗), where
x∗=λ1d1+βy∗,v∗=ky∗γ,z∗=λ1βp(d1+βy∗)−ap, |
and y∗ is the positive root of Eq (4) in (0,(R0−1)d1β):
aβqy2=[β(λ2p+ad2)+q(λ1β−ad1)]y−d1(ad2+λ2p)(R0−1). | (4) |
On the global stability of the equilibria of model (1), we have the following results.
Theorem 2.1. For model (1), if R0≤1, E0 is globally asymptotically stable. If R0>1, E∗ is globally asymptotically stable and E0 is unstable.
Proof. We first prove the global stability of the disease-free equilibrium E0.
Define a Lyapunov function
L1(x,y,v,z)=x−x0−x0lnxx0+y+mv+pq(z−z0−z0lnzz0), |
where m will be determined below.
Taking the time derivative of L1(x,y,v,z) with respect to the solution of model (1), we can obtain:
L′1|(1)=x−x0x(λ1−d1x−β1xv−β2xy)+β1xv+β2xy−ay−pyz+m(ky−γv)+p(z−z0)qz(λ2−d2z+qyz)=(x−x0)(λ1(1x−1x0)−β1v−β2y)+pλ2q(z−z0)(1z−1z0)+m(ky−γv)+py(z−z0)+(β1(x−x0)v+β2(x−x0)y−py(z−z0)+(β2x0−a−pz0)y)+β1x0v=λ1(1x−1x0)(x−x0)+pλ2q(z−z0)(1z−1z0)+(β2x0−a−pz0+mk)y+(β1x0−mγ)v. |
When R0≤1, we obtain β1x0γ≤a+pz0−β2x0k, so there exists m satisfying
β1x0γ≤m≤a+pz0−β2x0k, |
such that
β2x0−a−pz0+mk≤0,β1x0−mγ≤0. |
Then
L′1|(1)≤λ1(1x−1x0)(x−x0)+pλ2q(z−z0)(1z−1z0)≤0. |
Let M1={(x,y,v,z)∣L′1|(1)}. We can know that L′1|(1)=0 if and only if x=x0, y=0, v=0, z=z0. Hence, M1={E0}. Therefore it follows from Lyapunov-Lasalle invariance principle that E0 is globally asymptotically stable when R0≤1.
Next, we prove the global stability of the positive equilibrium E∗.
Consider the following Lyapunov function:
L2(x,y,v,z)=x−x∗−x∗lnxx∗+(y−y∗−y∗lnyy∗)+β1x∗v∗ky∗(v−v∗−v∗lnvv∗)+pq(z−z∗−z∗lnzz∗). |
At the infection equilibrium E∗, we can get:
λ1=d1x∗+β1x∗v∗+β2x∗y∗,a=β1x∗v∗y∗+β2x∗−pz∗,γ=ky∗v∗,d2=λ2z∗+qy∗. | (5) |
Taking the time derivation of L2(x,y,v,z) along with the solution of model (1), and considering Eq (5), we can obtain:
L′2|(1)=(1−x∗x)(−d1(x−x∗)−β1(xv−x∗v∗)−β2(xy−x∗y∗))+(y−y∗)(β1(xvy−x∗v∗y∗)+β2(x−x∗)−p(z−z∗))+β1x∗v∗y∗(v−v∗)(yv−y∗v∗)+pq(z−z∗)(λ2(1z−1z∗)+q(y−y∗)). |
Let x1=xx∗,y1=yy∗,v1=vv∗,z1=zz∗, then
L′2|(1)=−d1x∗(x1−1)(1−1x1)−β1x∗v∗(x1v1−1)(1−1x1)−β2x∗y∗(x1y1−1)(1−1x1)+β1x∗v∗(y1−1)(x1v1y1−1)+β1x∗v∗(v1−1)(y1v1−1)+β2x∗y∗(y1−1)(x1−1)+pλ2q(z1−1)(1z1−1)=(β2x∗y∗+d1x∗)(2−x1−1x1)+pλ2q(2−z1−1z1)+β1x∗v∗(3−1x1−x1v1y1−y1v1). |
Since the arithmetical mean m+n2 of m and n is greater than or equal to their geometrical mean √mn, it follows that L′2|(1)≤0. Let M∗={(x,y,v,z)∣L′2|(1)=0}. We note that L′2|(1)=0 if and only if x1=1, y1=v1, z1=1, i.e., x=x∗, yy∗=vv∗, z=z∗. Easy to know, y=y∗, v=v∗. So the largest invariant set of model (1) on the set M∗ is the singleton {E∗}. By the Lasalle principle, when R0>1, the infection equilibrium E∗ is globally asymptotically stable.
The proof is completed.
Remark 1. From Theorem 2.1, we know that R0 can be used to govern the threshold dynamics of deterministic HBV model (1). That is, if R0≤1, the HBV infection will go to extinction; while if R0>1, the HBV infection will persist.
Theorem 3.1. For any initial value X(0)=(x(0),y(0),v(0),z(0))∈R4+, there exist positive constants N1 and N2 such that the set Ω is almost surely positively invariant of the SDE HBV model (2), where
Ω={X(t)∈R4+:0<x(t)+y(t)≤Λ,0<v(t)≤N1,0<x(t)+y(t)+pqz(t)≤N2} |
and
Λ:=λ1min{a,d1}. |
Proof. It is clear that all solutions of model (2) starting from R4+={(x,y,v,z):x(0)>0,y(0)>0,v(0)>0,z(0)>0} are non-negative. From the first two equations of model (2), we have
d(x+y)≤(λ1−d1x−ay)dt≤(λ1−min{a,d1}(x+y))dt, |
which implies
lim supt→+∞(x(t)+y(t))≤λ1min{a,d1}:=Λ. |
From the third equation of model (2), we have
dv≤(kΛ−γv)dt−σ2vdB2(t). |
It follows that
v(t)≤kΛγ+(v(0)−kΛγ)e−γt−σ2∫t0e−γ(t−s)v(s)dB2(s):=X1(t), |
where
X1(t)=v(0)+A1(t)−C1(t)+ˆM1(t), |
and
A1(t)=kΛγ(1−e−γt),C1(t)=v(0)(1−e−γt),ˆM1(t)=−σ2∫t0e−γ(t−s)v(s)dB2(s). |
Clearly, A1(t) and C1(t) are continuous adapted increasing process on t≥0 with A1(0)=C1(0)=0. By [36,Theorem 1.3.9,p.14], we can obtain
limt→+∞X1(t)<∞a.s. |
That is, limt→+∞v(t)<∞a.s., which means that there is a positive constant N1 such that
v(t)≤N1,∀t≥0a.s. |
From the first two and the fourth equations, we have
d(x+y+pqz)=(λ1−d1x−ay+pλ2q−pd2qz)dt−pσ3qzdB3(t)≤(λ1+pλ2q−min{a,d1,d2}(x+y+pqz))dt−pσ3qzdB3(t). |
It follows that
x(t)+y(t)+pqz(t)≤λ1+pλ2qmin{a,d1,d2}+(x(0)+y(0)+pqz(0)−λ1+pλ2qmin{a,d1,d2})e−min{a,d1,d2}t−pσ3q∫t0e−min{a,d1,d2}(t−s)z(s)dB3(s):=X2(t), |
where
X2(t)=x(0)+y(0)+pqz(0)+A2(t)−C2(t)+ˆM2(t), |
and
A2(t)=λ1+pλ2qmin{a,d1,d2}(1−e−min{a,d1,d2}t),C2(t)=(x(0)+y(0)+pqz(0))(1−e−min{a,d1,d2}t),ˆM2(t)=−pσ3q∫t0e−min{a,d1,d2}(t−s)z(s)dB3(s). |
Clearly, A2(t) and C2(t) are continuous adapted increasing process on t≥0 with A2(0)=C2(0)=0. By [36,Theorem 1.3.9,p.14], we can obtain
limt→+∞X2(t)<∞a.s. |
That is, limt→+∞(x(t)+y(t)+pqz(t))<∞a.s., which means that there is a positive constant N2 such that
x(t)+y(t)+pqz(t)≤N2,∀t≥0a.s. |
Summarize the discussions above, we can conclude that the invariant set of model (2) is given as follows:
Ω={X(t)∈R4+:0<x(t)+y(t)≤Λ,0<v(t)≤N1,0<x(t)+y(t)+pqz(t)≤N2}. | (6) |
The proof is completed.
Theorem 3.2. For any initial value X(0)=(x(0),y(0),v(0),z(0))∈R4+, model (2) has a unique globally positive solution X(t)=(x(t),y(t),v(t),z(t)),t≥0, and the solution will remain in R4+ with probability one.
Proof. It is obvious that the coefficients of model (2) satisfy the local Lipschitz conditions. Then, there is a unique local solution X(t) on (t∈[0,τe)) for any given initial value X(0)∈R4+, where τe is the explosion time. In order to show this solution is global, we next prove τe=∞a.s.
Set n0>0 large enough for any initial value (x(0),y(0),v(0),z(0)) lying within the interval [1n0,n0]. Define the following stopping time
τk=inf{t∈[0,τe):x(t)∉(1/n,n),y(t)∉(1/n,n),v(t)∉(1/n,n),z(t)∉(1/n,n)}, |
where n≥n0. Set infϕ=∞, clearly, when n→∞, τk is increasing. Let τ∞=limk→∞τk, we can get τ∞≤τea.s. If there is τ∞=∞a.s., we can complete the proof at τe=∞a.s. Let us put it in another way, we can prove (x(t),y(t),v(t),z(t))∈R4+,(t≥0)a.s.
Suppose there are constants T>0 and ε∈(0,1) such that P{τ∞≤T}>ε. So, if n1≥n0 exists, when n≥n1, we have P{τn≤T}≥ε.
Define a C2-function V: R4+→R+:
V(x,y,v,z)=(x−a1−a1lnxa1)+(y−a2−a2lnya2)+c1(v−1−lnv)+c2(z−1−lnz), |
where a1,a2,c1,c2 are positive constants to be determined.
Using the Itô's formula, we get:
dV(x,y,v,z)=LV(x,y,v,z)dt−σ1y(x−a1)dB1(t)+σ1x(y−a2)dB1(t)−c1σ2(v−1)dB2(t)−c2σ3(z−1)dB3(t), |
where
LV(x,y,v,z)=(1−a1x)(λ1−d1x−β1xv−β2xy)+(1−a2y)(β1xv+β2xy−ay−pyz)+c1(1−1v)(ky−γv)+12σ22c1+12σ23c2+c2(1−1z)(λ2−d2z+qyz)+12σ21a1y2+12σ21a2x2=λ1−d1x−β1xv−β2xy−a1λ1x+a1d1+a1β1v+a1β2y+β1xv+β2xy−ay−pyz−a2β1xvy−a2β2x+aa2+a2pz+c1ky−c1γv−c1kyv+c1γ+c2λ2−c2d2z+c2qyz−c2λ2z+c2d2−c2qy+12σ22c1+12σ23c2+12σ21a1y2+12σ21a2x2≤(λ1+a1d1+aa2+c1γ+c2λ2+c2d2+12σ22c1+12σ23c2)+(−d1−a2β2)x−a1λ1x+(a1β2−a+c1k−c2q)y−a2β1xvy+(a1β1−c1γ)v−c1kyv+(a2p−c2d2)z−c2λ2z+(c2q−p)yz+12σ21a1Λ2+12σ21a2Λ2. |
Choose a1=(a+p)γβ2γ+β1k,a2=d2q,c1=(a+p)β1β2γ+β1k and c2=pq such that
a1β2−a+c1k−c2q=0,a1β1−c1γ=0,a2p−c2d2=0,c2q−p=0. |
Then,
LV(x,y,v,z)≤(λ1+a1d1+aa2+c1γ+c2λ2+c2d2+12σ22c1+12σ23c2+12σ21a1Λ2+12σ21a2Λ2)−(d1+a2β2)x−a1λ1x−a2β1xvy−c1kyv−c2λ2z≤λ1+a1d1+aa2+c1γ+c2λ2+c2d2+12σ22c1+12σ23c2+12σ21a1Λ2+12σ21a2Λ2≤K′. |
where K′ is a positive constant. Therefore, we have
dV(x,y,v,z)≤K′dt−σ1y(x−a1)dB1(t)+σ1x(y−a2)dB1(t)−c1σ2(v−1)dB2(t)−c2σ3(z−1)dB3(t). |
Thus,
∫τn∧T0dV(x,y,v,z)≤∫τn∧T0K′dt−∫τn∧T0σ1y(x−a1)dB1(t)+∫τn∧T0σ1x(y−a2)dB1(t)−∫τn∧T0c1σ2(v−1)dB2(t)−∫τn∧T0c2σ3(z−1)dB3(t). |
Then taking the expectation on both sides, we obtain
E[V(x(τn∧T),y(τn∧T),v(τn∧T),z(τn∧T))]≤V(x(0),y(0),v(0),z(0))+K′E(τn∧T)≤V(x(0),y(0),v(0),z(0))+K′T. |
Let Ωn={τn≤T}, where n≥n1, then P(Ωn)≥ε. Note that ∀ω∈Ωn. By the definition of stopping time, there is at least one of (x(τn,ω), y(τn,ω), v(τn,ω) and z(τn,ω)) equal to n or 1n. Therefore,
V(x(τn,ω),y(τn,ω),v(τn,ω),z(τn,ω))≥(n−a1−a1lnna1)∧(1n−a1+a1ln(a1n))∧(n−a2−a2lnna2)∧(1n−a2+a2ln(a2n))∧c1(n−1−lnn)∧c1(1n−1+lnn)∧c2(n−1−lnn)∧c2(1n−1+lnn):=h(n). |
It then follows that
V(x(0),y(0),v(0),z(0))+K′T≥E[V(x(τn∧T),y(τn∧T),v(τn∧T),z(τn∧T))]≥E[1Ωn(ω)V(x(τn),y(τn),v(τn),z(τn))]≥εh(n), |
where 1Ωn(ω) is the indicator function of Ωn. Letting n→∞ leads to the contradiction ∞>V(x(0),y(0),v(0),z(0))+K′T=∞, so we can conclude that τ∞=∞, which completes the proof.
In this section, in order to investigate whether the HBV infection is stochastically extinct, we study the dynamics around E0.
Theorem 4.1. For any given initial value X(0)=(x(0),y(0),v(0),z(0))∈Ω, if σ21<2d14Λ2+Λ22(d1λ1+1Λ)(1+λ1d1),σ22<2γ,σ23<d2, and R0≤1, then the solution of model (2) has the following property:
lim supt→+∞1tE∫t0((x−λ1d1)2+y2+v2+(z−λ2d2)2)dr≤M1ξ, | (7) |
where
M1:=4λ21σ21Λ2d21+2σ23λ22p2q2d22+12d1(d1λ1+1Λ)Λ2σ21λ1+12d21(d1λ1+1Λ)Λ2σ21λ21,ξ:=min{2d1−(Λ2σ212(d1λ1+1Λ)(1+λ1d1)+4σ21Λ2),aΛ,a2k2(2γ−σ22),2p2q2(d2−σ23)}. | (8) |
Proof. Set ω=x−λ1d1,Q=z−λ2d2, then model (2) can be rewritten as:
{dω=(−d1ω−β1v(ω+λ1d1)−β2y(ω+λ1d1))dt−σ1y(ω+λ1d1)dB1(t),dy=(β1v(ω+λ1d1)+β2y(ω+λ1d1)−ay−py(Q+λ2d2))dt+σ1y(ω+λ1d1)dB1(t),dv=(ky−γv)dt−σ2vdB2(t),dQ=(−d2Q+qy(Q+λ2d2))dt−σ3(Q+λ2d2)dB3(t). | (9) |
Let
V1(ω,y,v,Q)=(ω+y+akv+pqQ)2. |
By the Itô's formula, we get:
LV1=2(ω+y+akv+pqQ)(−d1ω−β1v(ω+λ1d1)−β2y(ω+λ1d1)+β1v(ω+λ1d1)+β2y(ω+λ1d1)−ay−py(Q+λ2d2)+ay−aγkv−pd2qQ+py(Q+λ2d2))+a2σ22k2v2+p2σ23q2(Q+λ2d2)2+2σ21y2(ω+λ1d1)2≤2(ω+y+akv+pqQ)(−d1ω−aγkv−pd2qQ)+a2σ22k2v2+p2σ23q2(Q2+2λ2d2Q+λ22d22)+2σ21Λ2(ω2+2λ1d1ω+λ21d21)≤−2d1ω2−2p2d2q2Q2−2a2γk2v2+a2σ22k2v2+p2σ23q2Q2+2λ2p2σ23d2q2Q+σ23p2λ22q2d22+2Λ2σ21ω2+4Λ2λ1σ21d1ω+2λ21Λ2d21σ21≤(2σ21Λ2−2d1)ω2+p2q2(σ23−2d2)Q2+a2k2(σ22−2γ)v2+2λ2σ23p2d2q2Q+σ23p2λ22q2d22+4Λ2λ1σ21d1ω+2λ21Λ2d21σ21. |
Noting that
4σ21λ1Λ2d1ω=4σ21Λ2(ω⋅λ1d1)≤2σ21ω2Λ2+2λ21σ21Λ2d21,2σ23λ2p2d2q2Q=2σ23p2q2(Q⋅λ2d2)≤σ23p2q2Q2+λ22σ23p2q2d22, |
then we can obtain:
LV1≤(4σ21Λ2−2d1)ω2+2p2q2(σ23−d2)Q2+a2k2(σ22−2γ)v2+2σ23λ22p2q2d22+4σ21λ21Λ2d21. |
Consider a new Lyapunov function
V2(ω,y,v,Q)=ω+y+nv+d12λ1ω2+12Λy2, |
where n is positive constant, we compute
LV2=−d1ω−β1v(ω+λ1d1)−β2y(ω+λ1d1)+(β1v(ω+λ1d1)+β2y(ω+λ1d1)−ay−py(Q+λ2d2))+n(ky−γv)+d1ωλ1(−d1ω−β1v(ω+λ1d1)−β2y(ω+λ1d1))+yΛ(β1v(ω+λ1d1)+β2y(ω+λ1d1)−ay−py(Q+λ2d2))+12(d1λ1+1Λ)σ21y2(ω+λ1d1)2≤(β2λ1d1−a−pλ2d2+nk)y+(β1λ1d1−nγ)v−aΛy2+12(d1λ1+1Λ)Λ2σ21ω2+12d1(d1λ1+1Λ)Λ2σ21λ1ω2+12d1(d1λ1+1Λ)Λ2σ21λ1+12d21(d1λ1+1Λ)Λ2σ21λ21. |
When R0≤1, we get
β1λ1d1γ≤a+pλ2d2−β2λ1d1k. |
We choose n satisfying
β1λ1d1γ≤n≤a+pλ2d2−β2λ1d1k. |
Then,
LV2≤−aΛy2+12(d1λ1+1Λ)Λ2σ21ω2+12d1(d1λ1+1Λ)Λ2σ21λ1ω2+12d1(d1λ1+1Λ)Λ2σ21λ1+12d21(d1λ1+1Λ)Λ2σ21λ21. |
Define V=V1+V2, then
LV≤(12(d1λ1+1Λ)Λ2σ21(1+λ1d1)+4σ21Λ2−2d1)ω2+2p2q2(σ23−d2)Q2−aΛy2+4λ21σ21Λ2d21+a2k2(σ22−2γ)v2+2σ23λ22p2q2d22+12d1(d1λ1+1Λ)Λ2σ21λ1+12d21(d1λ1+1Λ)Λ2σ21λ21. |
Let
M1=4λ21σ21Λ2d21+2σ23λ22p2q2d22+12d1(d1λ1+1Λ)Λ2σ21λ1+12d21(d1λ1+1Λ)Λ2σ21λ21. |
Consequently,
0≤E(V(ω(t),y(t),v(t),Q(t)))≤V(ω(0),y(0),v(0),Q(0))+E∫t0(−aΛy2+(12(d1λ1+1Λ)Λ2σ21(1+λ1d1)+4σ21Λ2−2d1)ω2+2p2q2(σ23−d2)Q2+a2k2(σ22−2γ)v2+M1)dr. |
Then
0≤E∫t0((2d1−(12(d1λ1+1Λ)Λ2σ21(1+λ1d1)+4σ21Λ2))ω2+aΛy2+a2k2(2γ−σ22)v2+2p2q2(d2−σ23)Q2)dr≤V(ω(0),y(0),v(0),Q(0))+M1t. |
So we obtain
lim supt→+∞1tE∫t0((2d1−(12(d1λ1+1Λ)Λ2σ21(1+λ1d1)+4σ21Λ2))ω2+aΛy2+a2k2(2γ−σ22)v2+2p2q2(d2−σ23)Q2)dr≤M1. |
Considering the definition of ξ in Eq (8), we obtain the conclusion (7). This ends the proof.
Remark 2. From Theorem 4.1, we know that, when R0≤1, if the white noise intensity is small and satisfies
σ21<2d14Λ2+Λ22(d1λ1+1Λ)(1+λ1d1),σ22<2γ,σ23<d2, |
the solution of the stochastic model (2) is fluctuating around the disease-free equilibrium point E0 of model (1). Epidemiologically speaking, the HBV infection dies out with probability one.
In this section, we discuss the stochastic dynamics around the positive equilibrium E∗ of the ODE HBV model (1) when R0>1.
Theorem 5.1. For any given initial value X(0)=(x(0),y(0),v(0),z(0))∈Ω, if σ21<d12Λ2, σ22<γ, σ23<d22 and R0>1, then the solution of model (2) has the following property:
lim supt→+∞1tE∫t0((x−x∗)2+(y−y∗)2+(v−v∗)2+(z−z∗)2)ds≤M2η, |
where
M2:=2σ21(x∗)2Λ2+a(γ−σ22)2k2σ22(v∗)2+p2q2σ23(z∗)2+p(a+d2)2q2z∗σ23+h(12σ21x∗Λ2+12σ21y∗Λ2+σ22β1x∗(v∗)22ky∗+σ23pz∗2q),h:=Λd1((a+d1)2a+(d1+d2)22d2),η:=min{d1−2σ21Λ2,a2,a(γ−σ22)24k2,p2q2(d22−σ23)}. |
Proof. Let
V1(x,y,v,z)=12(x−x∗+y−y∗+pq(z−z∗))2+p(a+d2)q2(z−z∗−z∗lnzz∗). |
By using Itô's formula, we can obtain:
LV1=(x−x∗+y−y∗+pq(z−z∗))(λ1−d1x−ay+pλ2q−pd2qz)+p2σ232q2z2+p(a+d2)q2(z−z∗)(λ2z−d2+qy)+p(a+d2)2q2z∗σ23+σ21x2y2=(x−x∗+y−y∗+pq(z−z∗))(d1x∗+ay∗+pd2qz∗−d1x−ay−pd2qz)+p2σ232q2z2+p(a+d2)q2(z−z∗)(λ2(1z−1z∗)+q(y−y∗))+p(a+d2)2q2z∗σ23+σ21x2y2=(x−x∗+y−y∗+pq(z−z∗))(−d1(x−x∗)−a(y−y∗)−pd2q(z−z∗))+p2σ232q2z2+p(a+d2)q2λ2(2−zz∗−z∗z)+p(a+d2)q(y−y∗)(z−z∗)+p(a+d2)2q2z∗σ23+σ21x2y2≤−d1(x−x∗)2−a(y−y∗)2−p2d2q2(z−z∗)2−(a+d1)(x−x∗)(y−y∗)−pq(d1+d2)(x−x∗)(z−z∗)+p2σ232q2z2+p(a+d2)2q2z∗σ23+σ21x2y2. |
Consider the following Lyapunov function
V2(x,y,v,z)=12(v−v∗)2. |
By Itô's formula, when σ22<γ, we can obtain:
LV2=(v−v∗)(ky−γv)+12σ22v2=(v−v∗)(ky−γv−ky∗+γv∗)+12σ22v2≤(v−v∗)(k(y−y∗)−γ(v−v∗))+σ22(v−v∗)2+σ22(v∗)2≤γ−σ222(v−v∗)2+k22(γ−σ22)(y−y∗)2−(γ−σ22)(v−v∗)2+σ22(v∗)2≤k22(γ−σ22)(y−y∗)2−γ−σ222(v−v∗)2+σ22(v∗)2 |
Define W1=V1+a(γ−σ22)2k2V2, then
LW1≤−d1(x−x∗)2−a(y−y∗)2−a(γ−σ22)24k2(v−v∗)2−p2d2q2(z−z∗)2+a4(y−y∗)2−(a+d1)(x−x∗)(y−y∗)+a(γ−σ22)2k2σ22(v∗)2−pq(d1+d2)(x−x∗)(z−z∗)+p22q2σ23z2+p(a+d2)2q2z∗σ23+σ21x2y2≤−d1(x−x∗)2−a(y−y∗)2−a(γ−σ22)24k2(v−v∗)2−p2d2q2(z−z∗)2+a4(y−y∗)2+(a+d1)2a(x−x∗)2+a4(y−y∗)2+(d1+d2)22d2(x−x∗)2+p2d22q2(z−z∗)2+a(γ−σ22)2k2σ22(v∗)2+p22q2σ23z2+p(a+d2)2q2z∗σ23+σ21x2y2≤((a+d1)2a+(d1+d2)22d2−d1)(x−x∗)2−a2(y−y∗)2+p22q2σ23z2−a(γ−σ22)24k2(v−v∗)2−p2d22q2(z−z∗)2+a(γ−σ22)2k2σ22(v∗)2+p(a+d2)2q2z∗σ23+σ21x2y2. |
Consider a new Lyapunov function
V3(x,y,v,z)=x−x∗−x∗lnxx∗+(y−y∗−y∗lnyy∗)+β1x∗v∗ky∗(v−v∗−v∗lnvv∗)+pq(z−z∗−z∗lnzz∗). |
Applying Itô's formula, we get:
dV3(x,y,v,z)=LV3(x,y,v,z)dt−σ1y(x−x∗)dB1(t)+σ1x(y−y∗)dB1(t)−σ2β1x∗v∗ky∗(v−v∗)dB2(t)−pσ3q(z−z∗)dB3(t), |
where
LV3=(1−x∗x)(λ1−d1x−β1xv−β2xy)+(1−y∗y)(β1xv+β2xy−ay−pyz)+β1x∗v∗ky∗(1−v∗v)(ky−γv)+pq(1−z∗z)(λ2−d2z+qyz)+σ22β1x∗(v∗)22ky∗+σ23pz∗2q+12σ21x∗y2+12σ21y∗x2=(1−x∗x)(−d1(x−x∗)−β1(xv−x∗v∗)−β2(xy−x∗y∗))+σ23pz∗2q+β1x∗v∗y∗(v−v∗)(yv−y∗v∗)+(y−y∗)(β1(xvy−x∗v∗y∗)+β2(x−x∗)−p(z−z∗))+σ22β1x∗(v∗)22ky∗+pq(z−z∗)(λ2(1z−1z∗)+q(y−y∗))+12σ21x∗y2+12σ21y∗x2=−d1x∗(x∗x+xx∗−2)+pλ2q(2−zz∗−z∗z)−β2x∗y∗(x∗x+xx∗−2)+β1x∗v∗(3−x∗x−v∗yy∗v−y∗xvx∗v∗y)+σ22β1x∗(v∗)22ky∗+σ23pz∗2q+12σ21x∗y2+12σ21y∗x2≤−d1x∗(x∗x+xx∗−2)−pλ2q(zz∗+z∗z−2)+σ22β1x∗(v∗)22ky∗+σ23pz∗2q+12σ21x∗y2+12σ21y∗x2≤−d1Λ(x−x∗)2+σ22β1x∗(v∗)22ky∗+σ23pz∗2q+12σ21x∗Λ2+12σ21y∗Λ2. |
Define W2=W1+hV3, then
LW2≤((a+d1)2a+(d1+d2)22d2−d1hΛ−d1)(x−x∗)2−a2(y−y∗)2−a(γ−σ22)24k2(v−v∗)2−p2d22q2(z−z∗)2+a(γ−σ22)2k2σ22(v∗)2+p22q2σ23z2+h(12σ21x∗Λ2+12σ21y∗Λ2+σ22β1x∗(v∗)22ky∗+σ23pz∗2q)+p(a+d2)2q2z∗σ23+σ21x2y2. |
Choosing
h=Λd1((a+d1)2a+(d1+d2)22d2) |
such that (a+d1)2a+(d1+d2)22d2=d1hΛ, we have:
LW2≤−d1(x−x∗)2−a2(y−y∗)2−a(γ−σ22)24k2(v−v∗)2−p2d22q2(z−z∗)2+a(γ−σ22)2k2σ22(v∗)2+p22q2σ23z2+σ21Λ2x2+p(a+d2)2q2z∗σ23+h(12σ21x∗Λ2+12σ21y∗Λ2+σ22β1x∗(v∗)22ky∗+σ23pz∗2q). |
Because a2≤2(a−b)2+2b2, we can get:
LW2≤(2σ21Λ2−d1)(x−x∗)2−a2(y−y∗)2−a(γ−σ22)24k2(v−v∗)2+p2q2(σ23−d22)(z−z∗)2+2σ21(x∗)2Λ2+a(γ−σ22)2k2σ22(v∗)2+p2q2σ23(z∗)2+h(12σ21x∗Λ2+12σ21y∗Λ2+σ22β1x∗(v∗)22ky∗+σ23pz∗2q)+p(a+d2)2q2z∗σ23. |
Let
M2=2σ21(x∗)2Λ2+a(γ−σ22)2k2σ22(v∗)2+p2q2σ23(z∗)2+p(a+d2)2q2z∗σ23+h(12σ21x∗Λ2+12σ21y∗Λ2+σ22β1x∗(v∗)22ky∗+σ23pz∗2q), |
and we can derive that
LW2≤(2σ21Λ2−d1)(x−x∗)2−a2(y−y∗)2−a(γ−σ22)24k2(v−v∗)2+p2q2(σ23−d22)(z−z∗)2+M2. |
As a result,
dW2≤LW2dt−pq(x−x∗+y−y∗+pq(z−z∗))σ3zdB3(t)−a(γ−σ22)2k2(v−v∗)σ2vdB2(t)−hβ1x∗v∗ky∗(v−v∗)σ2dB2(t)−hpq(z−z∗)σ3dB3(t)−pσ3(a+d2)q2(z−z∗)dB3(t)−h(x−x∗)σ1ydB1(t)+h(y−y∗)σ1xdB1(t). |
By integrating both sides from 0 to t and then taking the expectation on both sides, it yields
0≤E(W2(x(t),y(t),v(t),z(t)))≤W2(x(0),y(0),v(0),z(0))+E∫t0((2σ21Λ2−d1)(x−x∗)2−a2(y−y∗)2−a(γ−σ22)24k2(v−v∗)2+p2q2(σ23−d22)(z−z∗)2+M2)ds. |
Then, we can get
E∫t0((d1−2σ21Λ2)(x−x∗)2+a2(y−y∗)2+a(γ−σ22)24k2(v−v∗)2+p2q2(d22−σ23)(z−z∗)2)ds≤W2(x(0),y(0),v(0),z(0))+M2t. |
And hence, there is
lim supt→+∞1tE∫t0((d1−2σ21Λ2)(x−x∗)2+a2(y−y∗)2+a(γ−σ22)24k2(v−v∗)2+p2q2(d22−σ23)(z−z∗)2)ds≤M2. |
Let
η=min{d1−2σ21Λ2,a2,a(γ−σ22)24k2,p2q2(d22−σ23)}, |
then
lim supt→+∞1tE∫t0((x−x∗)2+(y−y∗)2+(v−v∗)2+(z−z∗)2)ds≤M2η. |
Remark 3. From Theorem 5.1, we know that, when R0>1, if the noise intensity is small and satisfies
σ21<d12Λ2,σ22<γ,σ23<d22, | (10) |
then the solution of the stochastic model (2) fluctuates around the infection equilibrium E∗ of the deterministic model (1). Epidemiologically, the HBV infection persist with probability one.
In this section, we continue to study the SDE model (2) through a numerical approach. We aim to investigate how environmental fluctuations affect disease spreading on the HBV dynamics.
We employ the Milstein's method [37] to simulate the SDE model (2), whose numerical scheme for the SDE model (2) is given by
{xi+1=xi+(λ1−d1xi−β1xivi−β2xiyi)Δt−σ1xiyi√Δtςi−σ212xiyi(Δtς2i−Δt),yi+1=yi+(β1xivi+β2xiyi−ayi−pyizi)Δt+σ1xiyi√Δtςi+σ212xiyi(Δtς2i−Δt),vi+1=vi+(kyi−γvi)Δt−σ2vi√Δtζi−σ222vi(Δtζ2i−Δt),zi+1=zi+(λ2−d2zi+qyizi)Δt−σ3zi√Δtϑi−σ232zi(Δtϑ2i−Δt), |
where ςi,ζi,ϑi,i=1,2,...,n are Gaussian random variables. We choose Δt=0.01.
As an example, the parameters are taken as follows:
λ1=0.3,d1=0.25,β1=0.4,a=0.2,p=0.2,k=0.06,γ=0.1,q=0.15,d2=0.1,β2=0.3,λ2=0.2. | (11) |
Then we obtain
R0=λ1d2(kβ1γ+β2)d1(ad2+λ2p)=1.08>1, |
and the corresponding deterministic model (1) has a disease-free equilibrium E0=(1.2,0,0,2.0) and a unique epidemic equilibrium E∗=(1.140,0.025,0.015,2.077), which is globally asymptotically stable according to Theorem 2.1.
Next, we use different values of σi(i=1,2,3) to understand the role of the noise strength in the resulting HBV dynamics for the SDE model (2).
Example 1: We adopt (σ1,σ2,σ3)=(0.15,0.08,0.1) and (0.2,0.1,0.15), respectively, then simple computations show that σ21<0.056,σ22<0.100,σ23<0.050, hence the conditions in Theorem 5.1 hold. From Theorem 5.1, we know that the HBV persist with probability one. The numerical results are shown in Figures 1 and 2, we know that, after some initial transients the solutions x(t),y(t),v(t),z(t) of the SDE model (2) fluctuation around the endemic equilibrium E∗=(1.140,0.025,0.015,2.077) of the deterministic model (1). Comparing Figures 1 and 2, we can see that, if the noise is lower, the amplitude of fluctuation is small (Figure 1); while if the noise is higher, the amplitude of fluctuation is big (Figure 2).
For the sake of learning the impact of random perturbation on the HBV disease dynamics, we have repeated the simulation 10000 times keeping all parameters fixed and never observed any extinction scenario up to t=100. And we give the histogram of the approximate stationary distributions of x(t) (a), y(t) (b), v(t) (c) and z(t) (d) at t=100 for the stochastic model (2) with σ1=0.15,σ2=0.08 and σ3=0.1 (Figure 3) and σ1=0.2,σ2=0.1 and σ3=0.15 (Figure 4), respectively (the numerical method can be found in [23]). One can find that x(t),y(t),v(t) and z(t) distributes normally, and also the solution to the SDE model (2) suggests for lower σi(i=1,2,3), the amplitude of fluctuation is slight and the oscillations are more symmetrically distributed (Figure 3); while for higher σi that the amplitude of fluctuation is remarkable and the distribution of the solution is skewed (Figure 4).
Example 2: If we adopt (σ1,σ2,σ3)=(0.8,0.65,0.6), then σ21>0.056,σ22>0.100,σ23>0.050, obviously, the conditions of Theorem 5.1 are not satisfied. The numerical results are shown in Figure 5, which suggest that {the HBV infection dies out} with probability one. That is, in the case of R0>1, if the environmental forcing intensity σi(i=1,2,3) is so large that the conditions of Theorem 5.1 are not satisfied, the HBV could die out with probability one. Obviously, the extinction of the HBV is induced by the noise.
Form Example 2, we know that in the case of R0>1, there exists extinction of the HBV infection in the SDE model (2). Next, we will focus on the extinction time of the HBV infection in the stochastic model (2).
Example 3: We adopt (σ2,σ3)=(0.65,0.6), then σ22−γ=0.32, σ23−d22=0.31. Obviously, the conditions of Theorem 5.1 are not satisfied. And we repeat 10,000 simulations with the same parameters as in Figure 5, we can calculate the average extinction time of y(t) with σ1, and the results are shown in Figure 6. For example, when σ1=0.4, the average extinction time for y(t) is 196.6; when σ1=0.8, it is 79.6. We can conclude that the average extinction time decreases with the increase of noise intensity σ1.
Finally, we will investigate the effect of the effective contact rate β2 on the HBV dynamics of the SDE HBV model (2) with fixed forcing intensity.
Example 4: We fix (σ1,σ2,σ3)=(0.1,0.1,0.1), and choose β2=0.15,0.2,0.25,0.35,0.4,0.45, other parameters are taken as in parameter set (11). In this case, we can obtain
σ21−2d14Λ2+Λ22(d1λ1+1Λ)(1+λ1d1)=−0.03<0,σ22−2γ=−0.19<0,σ23−d2=−0.09<0,σ21−d12Λ2=−0.05<0,σ22−γ=−0.09<0,σ23−d22=−0.04<0 |
and the values of R0 are given in Table 1. Obviously, the cases of β2=0.15,0.2,0.25 satisfy the conditions of Theorem 4.1, and the cases of β2=0.35,0.4,0.45 satisfy the conditions of Theorem 5.1. The numerical results of the stochastic extinction and persistence of model (2) are shown in Figure 7.
parameter | values | |||||
β2 | 0.15 | 0.2 | 0.25 | 0.35 | 0.4 | 0.45 |
R0 | 0.78 | 0.88 | 0.98 | 1.18 | 1.28 | 1.38 |
For the study of an HBV infection model, much attention is usually paid to the persistence and extinction of HBV infection. In the deterministic HBV model, the value of the basic reproductive number is usually the key to determining the persistent and extinction of the HBV. When R0≤1, the HBV will die out; when R0>1, the HBV will persist (see Theorem 2.1). But the SDE HBV model, such as model (2), does not have any equilibrium, so we have to study the asymptotic behavior of the solution of the SDE model around the equilibrium of the deterministic HBV model to reflect the trend of the HBV infection to some extent.
In the present paper, we investigate the stochastic dynamics of an HBV infection model with cell-to-cell transmission and CTL immune response, and analyze the stochastic fluctuation of positive solutions of SDE model (2) around the infection-free equilibrium E0 (Figures 7(a)–7(c)) and the infection equilibrium E∗ (Figures 1, 2 and Figure 7(d)–7(f)) of the deterministic model (1), respectively, which reveals that the influence of the white noise on HBV dynamics. More precisely, when the deterministic model (1) is disturbed by small white noise σi(i=1,2,3), the {HBV infection} is extinct (see Theorem 4.1 and Remark 2) or persist (see Theorem 5.1 and Remark 3), and the solutions of model (2) will fluctuate around the infection equilibrium E∗ of the deterministic model (1). Furthermore, via numerical simulations, we find that the fluctuation amplitude is positively related to the intensity of the white noise, which means, epidemiologically, as the intensity of white noise decreases, the fluctuation of the solution of model (2) around the equilibrium of the corresponding deterministic model (1) becomes smaller and smaller (Figures 1–4). In these cases, to a great extent, we can ignore the noise and use the deterministic model (2) to describe the HBV infection dynamics. However, when the noise σi(i=1,2,3) is sufficiently large, the infected hepatocytes y(t) on relatively large disturbance of white noise goes to extinction, and the uninfected hepatocytes x(t) generates persistence (Figure 5). In these cases, we cannot ignore the effect of noise, that is, we should use the stochastic HBV model (2) rather than the deterministic model (1) to describe the HBV infection dynamics. In addition, it should be pointed out that the increase in β2 results in the corresponding increase in R0. From the biological point of view, one can find that under certain forcing intensity, reducing the value of the effective contact rate β2 so that the value of R0 is less than unity could lead to the stochastically extinction of the HBV infection. These results can provide us with some useful control strategies to regulate HBV infection dynamics.
The authors would like to thank the anonymous referees for very helpful suggestions and comments which led to improvements of our original manuscript. This research was supported by the National Natural Science Foundation of China (Grant numbers 61672013, 11601179 and 12071173), and the Huaian Key Laboratory for Infectious Diseases Control and Prevention (HAP201704). K.F. Wang was supported by the National Natural Science Foundation of China (Grant number 11771448).
The authors declare that they have no competing interests.
[1] | World Health Organization, Hepatitis B, Accessed 12 Nov. 2020. Available from: https://www.who.int/health-topics/hepatitis#tab=tab_1. |
[2] |
M. Nowak, R. M. May, Population dynamics of immune responses to persistent viruses, Science, 272 (1996), 74–79. doi: 10.1126/science.272.5258.74
![]() |
[3] |
R. A. Arnaout, M. A. Nowak, Competitive coexistence in antiviral immunity, J. Theor. Biol., 204 (2000), 431–441. doi: 10.1006/jtbi.2000.2027
![]() |
[4] |
R. V. Culshaw, S. Ruan, R. J. Spiteri, Optimal HIV treatment by maximising immune response, J. Math. Biol., 48 (2004), 545–562. doi: 10.1007/s00285-003-0245-3
![]() |
[5] | D. Bertacchi, F. Zucca, S. Foresti, D. Mangioni, A. Gori, Combination versus sequential monotherapy in chronic HBV infection: a mathematical approach, Math. Med. Biol., 32 (2015), 383–403. |
[6] | A. U. Neumann, Hepatitis B viral kinetics: A dynamic puzzle still to be resolved, Hepatology, 42 (2005), 249–254. |
[7] | M. Nowak, R. M. May, Virus Dynamics: Mathematical Principles of Immunology and Virology: Mathematical Principles of Immunology and Virology, Oxford University Press, UK, 2000. |
[8] |
J. Li, K. Men, Y. Yang, D. Li, Dynamical analysis on a chronic hepatitis C virus infection model with immune response, J. Theor. Biol., 365 (2015), 337–346. doi: 10.1016/j.jtbi.2014.10.039
![]() |
[9] |
M. Li, J. Zu, The review of differential equation models of HBV infection dynamics, J. Virol. Meth., 266 (2019), 103–113. doi: 10.1016/j.jviromet.2019.01.014
![]() |
[10] | A. Mojaver, H. Kheiri, Dynamical analysis of a class of hepatitis C virus infection models with application of optimal control, Inter. J. Biomath., 9 (2016), 1650038. |
[11] | W. Mothes, N. M. Sherer, J. Jin, P. Zhong, Virus cell-to-cell transmission, J. Virol., 84 (2010), 8360–8368. |
[12] |
S. Pan, S. P. Chakrabarty, Threshold dynamics of HCV model with cell-to-cell transmission and a non-cytolytic cure in the presence of humoral immunity, Commun. Nonlinear Sci., 61 (2018), 180–197. doi: 10.1016/j.cnsns.2018.02.010
![]() |
[13] | K. Razali, H. Thein, J. Bell, M. Cooper-Stanbury, K. Dolan, G. Dore, et al., Modelling the hepatitis C virus epidemic in Australia, Drug Alcohol Depen., 91 (2007), 228–235. |
[14] | B. Roe, W. W. Hall, Cellular and molecular interactions in coinfection with hepatitis C virus and human immunodeficiency virus, Expert Rev. Mol. Med., 10 (2008), e30. |
[15] |
F. Zhang, J. Li, C. Zheng, L. Wang, Dynamics of an HBV/HCV infection model with intracellular delay and cell proliferation, Commun. Nonlinear Sci., 42 (2017), 464–476. doi: 10.1016/j.cnsns.2016.06.009
![]() |
[16] | P. Zhong, L. M. Agosto, J. B. Munro, W. Mothes, Cell-to-cell transmission of viruses, Curr. Opin. Virol., 3 (2013), 44–50. |
[17] | M. Sourisseau, N. Sol-Foulon, F. Porrot, F. Blanchet, O. Schwartz, Inefficient human immunodeficiency virus replication in mobile lymphocytes, J. Virol., 81 (2006), 1000–1012. |
[18] |
B. Monel, E. Beaumont, D. Vendrame, O. Schwartz, D. Brand, Hiv cell-to-cell transmission requires the production of infectious virus particles and does not proceed through env-mediated fusion pores, J. Virology, 86 (2012), 3924–3933. doi: 10.1128/JVI.06478-11
![]() |
[19] |
Y. Yang, Q. Han, Z. Hou, C. Zhang, Z. Tian, J. Zhang, Exosomes mediate hepatitis B virus (HBV) transmission and NK-cell dysfunction, Cell. Mol. Immunol., 14 (2017), 465–475. doi: 10.1038/cmi.2016.24
![]() |
[20] | G. Bocharov, B. Ludewig, A. Bertoletti, P. Klenerman, T. Junt, P. Krebs, et al., Underwhelming the immune response: effect of slow virus growth on CD8+-T-lymphocyte responses, J. Virol., 78 (2004), 2247–2254. |
[21] |
S. Cai, Y. Cai, X. Mao, A stochastic differential equation SIS epidemic model with two independent brownian motions, J. Math. Anal. Appl., 474 (2019), 1536–1550. doi: 10.1016/j.jmaa.2019.02.039
![]() |
[22] |
Y. Cai, J. Jiao, Z. Gui, Y. Liu, W. M. Wang, Environmental variability in a stochastic epidemic model, Appl. Math. Comp., 329 (2018), 210–226. doi: 10.1016/j.amc.2018.02.009
![]() |
[23] |
Y. Cai, Y. Kang, M. Banerjee, W.M. Wang, A stochastic SIRS epidemic model with infectious force under intervention strategies, J. Differ. Equations, 259 (2015), 7463–7502. doi: 10.1016/j.jde.2015.08.024
![]() |
[24] |
Y. Cai, Y. Kang, W. M. Wang, A stochastic SIRS epidemic model with nonlinear incidence rate, Appl. Math. Comp., 305 (2017), 221–240. doi: 10.1016/j.amc.2017.02.003
![]() |
[25] | C. Ji, D. Jiang, Dynamics of an HIV-1 infection model with cell-mediated immune response and stochastic perturbation, Inter. J. Biomath., 5 (2012), 1250039. |
[26] |
T. Luzyanina, G. Bocharov, Stochastic modeling of the impact of random forcing on persistent hepatitis B virus infection, Math. Comp. Simul., 96 (2014), 54–65. doi: 10.1016/j.matcom.2011.10.002
![]() |
[27] | F. A. Rihan, H. J. Alsakaji, C. Rajivganthi, Stochastic SIRC epidemic model with time-delay for covid-19, Adv. Differ. Equ., 2020 (2020), 502. |
[28] | Y. Wang, D. Jiang, Stationary distribution and extinction of a stochastic viral infection model, Discrete Dyn. Nat. Soc., 2017 (2017), 1–13. |
[29] |
Y. Wang, D. Jiang, A. Alsaedi, T. Hayat, Modelling a stochastic HIV model with logistic target cell growth and nonlinear immune response function, Phys. A, 501 (2018), 276–292. doi: 10.1016/j.physa.2018.02.040
![]() |
[30] |
Y. Wang, D. Jiang, T. Hayat, B. Ahmad, A stochastic HIV infection model with T-cell proliferation and CTL immune response, Appl. Math. Comp., 315 (2017), 477–493. doi: 10.1016/j.amc.2017.07.062
![]() |
[31] |
Y. Yuan, L. J. S. Allen, Stochastic models for virus and immune system dynamics, Math. Biosci., 234 (2011), 84–94. doi: 10.1016/j.mbs.2011.08.007
![]() |
[32] |
F. Xie, M. Shan, X. Lian, W. M. Wang, Periodic solution of a stochastic HBV infection model with logistic hepatocyte growth, Appl. Math. Comp., 293 (2017), 630–641. doi: 10.1016/j.amc.2016.06.028
![]() |
[33] |
T. G. Kurtz, Solutions of ordinary differential equations as limits of pure jump Markov processes, J. Appl. Probab., 7 (1970), 49–58. doi: 10.2307/3212147
![]() |
[34] |
T. G. Kurtz, Limit theorems for sequences of jump Markov processes approximating ordinary differential processes, J. Appl. Probab., 8 (1971), 344–356. doi: 10.2307/3211904
![]() |
[35] |
P. Van den Driessche, J. Watmough, Reproduction numbers and sub-threshold endemic equilibria for compartmental models of disease transmission, Math. Biosci., 180 (2002), 29–48. doi: 10.1016/S0025-5564(02)00108-6
![]() |
[36] | X. Mao, Stochastic Differential Equations and Applications, Elsevier, 2007. |
[37] |
D. J. Higham, An algorithmic introduction to numerical simulations of stochastic differentila equations, SIAM Rev., 43 (2001), 525–546. doi: 10.1137/S0036144500378302
![]() |
1. | Tengfei Wang, Shaoli Wang, Fei Xu, Bistability and Robustness for Virus Infection Models with Nonmonotonic Immune Responses in Viral Infection Systems, 2022, 10, 2227-7390, 2139, 10.3390/math10122139 | |
2. | Yucong Dai, Baoquan Zhou, Daqing Jiang, Tasawar Hayat, Stationary distribution and density function analysis of stochastic susceptible‐vaccinated‐infected‐recovered (SVIR) epidemic model with vaccination of newborns, 2022, 45, 0170-4214, 3401, 10.1002/mma.7986 | |
3. | Yousef Alnafisah, Moustafa El-Shahed, Deterministic and stochastic model for the hepatitis C with different types of virus genome, 2022, 7, 2473-6988, 11905, 10.3934/math.2022664 | |
4. | Yiping Tan, Yongli Cai, Xiaodan Sun, Kai Wang, Ruoxia Yao, Weiming Wang, Zhihang Peng, A stochastic SICA model for HIV/AIDS transmission, 2022, 165, 09600779, 112768, 10.1016/j.chaos.2022.112768 | |
5. | Fathalla A. Rihan, Hebatallah J. Alsakaji, Analysis of a stochastic HBV infection model with delayed immune response, 2021, 18, 1551-0018, 5194, 10.3934/mbe.2021264 | |
6. | Jiying Ma, Shasha Ma, Dynamics of a stochastic hepatitis B virus transmission model with media coverage and a case study of China, 2022, 20, 1551-0018, 3070, 10.3934/mbe.2023145 | |
7. | Yiping Tan, Yongli Cai, Zhihang Peng, Kaifa Wang, Ruoxia Yao, Weiming Wang, Dynamics of a stochastic HBV infection model with drug therapy and immune response, 2022, 19, 1551-0018, 7570, 10.3934/mbe.2022356 | |
8. | Maysaa Al Qurashi, Saima Rashid, Fahd Jarad, A computational study of a stochastic fractal-fractional hepatitis B virus infection incorporating delayed immune reactions via the exponential decay, 2022, 19, 1551-0018, 12950, 10.3934/mbe.2022605 | |
9. | Yousef Alnafisah, Moustafa El-Shahed, Stochastic Analysis of a Hantavirus Infection Model, 2022, 10, 2227-7390, 3756, 10.3390/math10203756 | |
10. | Zakaria Yaagoub, Karam Allali, Fractional HBV infection model with both cell-to-cell and virus-to-cell transmissions and adaptive immunity, 2022, 165, 09600779, 112855, 10.1016/j.chaos.2022.112855 | |
11. | Moustafa El-Shahed, Asma Al-Nujiban, Nagdy F. Abdel-Baky, Stochastic Modelling of Red Palm Weevil Using Chemical Injection and Pheromone Traps, 2022, 11, 2075-1680, 334, 10.3390/axioms11070334 | |
12. | Selma El Messaoudi, Annabelle Lemenuel‐Diot, Antonio Gonçalves, Jérémie Guedj, A Semi‐mechanistic Model to Characterize the Long‐Term Dynamics of Hepatitis B Virus Markers During Treatment With Lamivudine and Pegylated Interferon, 2023, 113, 0009-9236, 390, 10.1002/cpt.2798 | |
13. | Xinxin Su, Xinhong Zhang, Daqing Jiang, Dynamics of a stochastic HBV infection model with general incidence rate, cell-to-cell transmission, immune response and Ornstein–Uhlenbeck process, 2024, 186, 09600779, 115208, 10.1016/j.chaos.2024.115208 | |
14. | Yuequn Gao, Ning Li, Fractional order PD control of the Hopf bifurcation of HBV viral systems with multiple time delays, 2023, 83, 11100168, 1, 10.1016/j.aej.2023.10.032 | |
15. | Z. Yaagoub, K. Allali, Fractional HCV infection model with adaptive immunity and treatment, 2023, 10, 23129794, 995, 10.23939/mmc2023.04.995 | |
16. | Yuwen Wang, Jiachen Li, Jianing Li, Wenjie Li, Chun Yang, Xiaoyang Liu, Wei Wang, Patient visit behaviour shapes the virus infection dynamics in hosts, 2024, 527, 03759601, 129985, 10.1016/j.physleta.2024.129985 | |
17. | Jialiang Huang, Xianlong Fu, Asymptotic analysis on a new stochastic epidemic model involving isolation mechanism, 2024, 34, 1054-1500, 10.1063/5.0151930 | |
18. | Chong Chen, Yinggao Zhou, Zhijian Ye, Mengze Gu, Stability and Hopf bifurcation of a HBV infection model with capsids and CTL immune response delay, 2024, 139, 2190-5444, 10.1140/epjp/s13360-024-05764-1 | |
19. | A.M. Elaiw, GH.S. Alsaadi, A.A. Raezah, A.D. Hobiny, Co-dynamics of hepatitis B and C viruses under the influence of CTL immunity, 2025, 119, 11100168, 285, 10.1016/j.aej.2025.01.029 | |
20. | Ahmed M. Kareem, 2025, 3264, 0094-243X, 050105, 10.1063/5.0258458 | |
21. | Jialiang Huang, Xianlong Fu, Asymptotic properties and optimal control of rainfall models involving forest-lake effects, 2025, 20, 0973-5348, 8, 10.1051/mmnp/2025005 | |
22. | Jialiang Huang, Xianlong Fu, Asymptotical Analysis on Deterministic and Stochastic Climate Models of Vegetation‐CO2$$ {\mathrm{CO}}_2 $$‐Temperature, 2025, 0170-4214, 10.1002/mma.10950 |
parameter | values | |||||
β2 | 0.15 | 0.2 | 0.25 | 0.35 | 0.4 | 0.45 |
R0 | 0.78 | 0.88 | 0.98 | 1.18 | 1.28 | 1.38 |