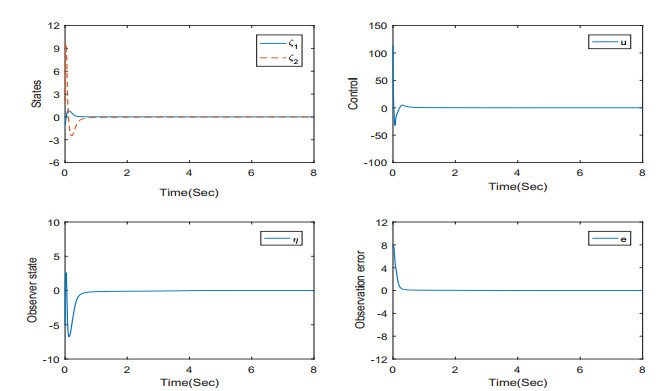
Citation: Rui Xie, Yuanyuan Zhao, Liming Chen. Structural path analysis and its applications: literature review[J]. National Accounting Review, 2020, 2(1): 83-94. doi: 10.3934/NAR.2020005
[1] | Jingya Wang, Ye Zhu . L2−L∞ control for memristive NNs with non-necessarily differentiable time-varying delay. Mathematical Biosciences and Engineering, 2023, 20(7): 13182-13199. doi: 10.3934/mbe.2023588 |
[2] | Kseniia Kravchuk, Alexander Vidybida . Non-Markovian spiking statistics of a neuron with delayed feedback in presence of refractoriness. Mathematical Biosciences and Engineering, 2014, 11(1): 81-104. doi: 10.3934/mbe.2014.11.81 |
[3] | Tianqi Yu, Lei Liu, Yan-Jun Liu . Observer-based adaptive fuzzy output feedback control for functional constraint systems with dead-zone input. Mathematical Biosciences and Engineering, 2023, 20(2): 2628-2650. doi: 10.3934/mbe.2023123 |
[4] | Gonzalo Robledo . Feedback stabilization for a chemostat with delayed output. Mathematical Biosciences and Engineering, 2009, 6(3): 629-647. doi: 10.3934/mbe.2009.6.629 |
[5] | Linni Li, Jin-E Zhang . Input-to-state stability of stochastic nonlinear system with delayed impulses. Mathematical Biosciences and Engineering, 2024, 21(2): 2233-2253. doi: 10.3934/mbe.2024098 |
[6] | Xiaoxiao Dong, Huan Qiao, Quanmin Zhu, Yufeng Yao . Event-triggered tracking control for switched nonlinear systems. Mathematical Biosciences and Engineering, 2023, 20(8): 14046-14060. doi: 10.3934/mbe.2023627 |
[7] | Tong Guo, Jing Han, Cancan Zhou, Jianping Zhou . Multi-leader-follower group consensus of stochastic time-delay multi-agent systems subject to Markov switching topology. Mathematical Biosciences and Engineering, 2022, 19(8): 7504-7520. doi: 10.3934/mbe.2022353 |
[8] | Dong Xu, Xinling Li, Weipeng Tai, Jianping Zhou . Event-triggered stabilization for networked control systems under random occurring deception attacks. Mathematical Biosciences and Engineering, 2023, 20(1): 859-878. doi: 10.3934/mbe.2023039 |
[9] | Yue Song, Yi Zhang, Song Yang, Na Li . Investigation on stability and controller design for singular bio-economic systems with stochastic fluctuations. Mathematical Biosciences and Engineering, 2021, 18(3): 2991-3005. doi: 10.3934/mbe.2021150 |
[10] | Xiaofeng Chen . Double-integrator consensus for a switching network without dwell time. Mathematical Biosciences and Engineering, 2023, 20(7): 11627-11643. doi: 10.3934/mbe.2023516 |
In many industrial applications, due to the ubiquity of stochastic noise and nonlinear [1,2], real systems are often modelled by stochastic differential equations, which attracts researchers to pay more and more attention to the control of stochastic systems. Using the state-feedback, a closed-loop pole can be arbitrarily configured to improve the performance of the control systems. Therefore, some scholars research the problem of state-feedback stabilization for stochastic systems, e.g., reference [3] focuses on the cooperative control problem of multiple nonlinear systems perturbed by second-order moment processes in a directed topology. Reference [4] considers the case where the diffusion term and the drift term are unknown parameters for stochastic systems with strict feedback. Reference [5] studies stochastic higher-order systems with state constraints and [6] discusses output constrained stochastic systems with low-order and high-order nonlinear and stochastic inverse dynamics. However, it is often difficult to obtain all the state variables of the system directly, it is unsuitable for direct measurement or the measurement equipment is limited in economy and practicality, so the physical implementation of state-feedback is difficult. One of the solutions to this difficulty is to reconstruct the state of the system. At this time, scholars use an observer to investigate the output-feedback stabilization, e.g., reference [7] investigates the prescribed-time stability problem of stochastic nonlinear strict-feedback systems. Reference [8] focuses on stochastic strict feedback systems with sensor uncertainties. In addition, based on output-feedback, for nonlinear multiagent systems, a distributed output-feedback tracking controller is proposed in [9].
It should be noted that all of the above results [7,8,9], Markovian switching is not considered in the design of output-feedback controller. However, as demonstrated by [10], switching system is a complex hybrid system, which consists of a series of subsystems and switching rules that coordinate the order of each subsystem. In real life, due to the aging of internal components, high temperature, sudden disturbance of external environment, operator error and other inevitable factors, the structure of many systems changes suddenly. Such systems can be reasonably modelled as differential equation with Markovian switching, see [11,12]. Recently, references [13] and [14] discuss the adaptive tracking problem and output tracking problem with Markovian switching respectively. Besides, as shown in [15], the power of the system changes because of factors such as the aging of the springs inside the boiler-turbine unit. Therefore, the research on the stability of stochastic nonlinear systems with time-varying powers has important practical significance. Reference [16] investigates the optimality and stability of high-order stochastic systems with time-varying powers. However, these results do not address the output-feedback stabilization for higher-order stochastic systems with both Markovian switching and time-varying powers.
Based on these discussions, we aim to resolve the output-feedback stabilization for higher-order stochastic nonlinear systems with both Markovian switching and time-varying powers. The main contributions and characteristics of this paper are two-fold:
1) The system model we take into account is more applicable than the existing results [7,8,9] and [12,13,14]. Different from the previous results [7,8,9], the stochastic system with Markovian switching is studied in this paper. Unlike previous studies in [12,13,14], we investigate the power is time-varying. The simultaneous existence of the Markov process and time-varying order makes the controller design process more complicated and difficult. More advanced stochastic analysis techniques are needed.
2) We propose a new observer. The existence of Markovian switching and nondifferentiable time-varying power makes the observer constructed in [7,8,9] invalid. We use the time-varying power's bounds to construct a new observer, which can effectively observe the unmeasurable state and can deal with the nonlinear growth rate, while the existing observer can only deal with constant growth rate.
The rest of this paper is listed as follows. The problem is formulated in Section 2. In Section 3, an output-feedback controller is designed. Section 4 is the stability analysis. A simulation is given in Section 5. The conclusions are collected in Section 6.
Notations: R2 denotes the 2-dimensional space and the set of nonnegative real numbers is represented by R+. X denotes the matrix or vector, its transpose is represented by XT. |X| denotes the Euclidean norm of a vector X. When X is square, Tr{X} denotes its trace. The set of all functions with continuous ith partial derivatives is represented by Ci. Let C2,1(R2×R+×S;R+) represent all nonnegative functions V on R2×R+×S which are C2 in x and C1 in t.
This paper studies the output-feedback stabilization for stochastic nonlinear systems with both Markovian switching and time-varying powers described by:
dζ1=[ζ2]m(t)dt,dζ2=[u]m(t)dt+fTγ(t)(ˉζ2)dω,y=ζ1, | (2.1) |
where ζ=ˉζ2=(ζ1,ζ2)T∈R2, y∈R and u∈R are the system state, control output and the input, respectively. The state ζ2 is unmeasurable. The function m(t):R+→R+ is continuous and bounded, which satisfies 1≤m_≤m(t)≤ˉm with m_ and ˉm being constants. The powers sign function [⋅]α is defined as [⋅]α:=sign(⋅)|⋅|α with α∈(0,+∞). The functions fγ(t) is assumed to be smooth, and for all t≥0, the locally Lipschitz continuous in x uniformly. fγ(t)(t,0)=0. ω is an r−dimensional standard Wiener process, which is defined on the complete probability space (Ω,F,Ft,P) with the filtration Ft satisfying the general conditions. γ(t) is a homogeneous Markov process on the probability space taking values in a space S={1,2,...,N}, which the generator Γ=(λij)N×N given by
Pij(t)=P{γ(t+s)=i|γ(s)=j}={λijt+o(t)ifi≠j,1+λijt+o(t)ifi=j, | (2.2) |
where λij>0 is the transition rate from i to j if i≠j while λii=−ΣNj=1,i≠jλij for any s,t≥0. Suppose the Markov process γ(t) is irrelevant to the ω(t).
To implement the controller design, we need the following assumption.
Assumption 2.1. There exists a non-negative smooth function ˜f(ζ1) such that
|fγ(t)(ˉζ2)|≤(|ζ1|m(t)+12+|ζ2|m(t)+12)˜f(ζ1). | (2.3) |
Remark 2.1. As we know, the existing results for stochastic systems with time-varying powers (e.g., [16]), neither the state-feedback control nor the output-feedback control, has considered Markovian switching. However, the structure of many physical systems in the actual system often mutates, which makes it necessary to study systems with both Markovain switching and time-varying powers. Therefore, compared with [16], the model we consider is more practical and more general.
Remark 2.2. In Assumption 2.1, we can see that the power m(t) is time-varying and the growth rate ˜f(ζ1) is a nonlinear function. When m(t)=1 and ˜f(ζ1) is a constant, Assumption 2.1 is a linear growth condition. However, we consider that ˜f(ζ1) is a nonlinear function, which includes the constant case as a special case. The growth condition of Assumption 2.1 is broader than the linear growth condition. The time-varying power m(t) makes the design in [7,8,9] for time-invariant power invalid. In addition, the nonlinear growth rate ˜f(ζ1) makes the design in [7,8,9,17,18] for constant growth rate fail. A new design scheme should be proposed.
In this section, we develop an output-feedback controller design for system (2.1). The process is divided into two steps:
∙ Firstly, we assume that all states are measurable and develop a state-feedback controller using backstepping technique.
∙ Secondly, we construct a reduced-order observer with a dynamic gain, and design an output-feedback controller.
In this part, under Assumption 2.1, our objective is to develop a state-feedback controller design for the system (2.1).
Step 1. Introducing the coordinate transformation ξ1=ζ1 and choosing V1=14ξ41, by using the infinitesimal generator defined in section 1.8 of [11], we have
LV1≤ξ31[ζ2]m(t)+IIV1≤ξ31([ζ2]m(t)−[ζ∗2]m(t))+ξ31[ζ∗2]m(t)+IIV1. | (3.1) |
If we choose ζ∗2 as
ζ∗2=−c1/m_1ξ1:=−α1ξ1, | (3.2) |
we get
ξ31[ζ∗2]m(t)=−αm(t)1ξm(t)+31≤−c1|ξ1|m(t)+3, | (3.3) |
where α1=c1/m_1≥1 is a constant with c1≥1 being a design parameter.
Substituting (3.3) into (3.1) yields
LV1≤−c1|ξ1|m(t)+3+ξ31([ζ2]m(t)−[ζ∗2]m(t))+IIV1. | (3.4) |
Step 2. Introducing the coordinate transformation ξ2=ζ2−ζ∗2, and using Itô's differentiation rule, we get
dξ2=([u]m(t)−∂ζ∗2∂ζ1[ζ2]m(t))dt+fTγ(t)(ˉζ2)dω. | (3.5) |
Choose V2=V1+14ξ42. From (3.4) and (3.5), we obtain
LV2≤−c1|ξ1|m(t)+3+ξ31([ζ2]m(t)−[ζ∗2]m(t))+ξ32[u]m(t)−ξ32∂ζ∗2∂ζ1[ζ2]m(t)+32ξ22|fTγ(t)(ˉζ2)|2+IIV2. | (3.6) |
By (3.2) and using Lemma 1 in [19], we have
ξ31([ζ2]m(t)−[ζ∗2]m(t))≤ˉm(2ˉm−2+2)(|ξ1|3|ξ2|m(t)+αˉm−11|ξ1|m(t)+2|ξ2|). | (3.7) |
By using Lemma 2.1 in [20], we get
ˉm(2+2ˉm−2)|ξ1|3|ξ2|m(t)≤16|ξ1|3+m(t)+β211|ξ2|3+m(t),ˉm(2+2ˉm−2)αˉm−11|ξ1|m(t)+2|ξ2|≤16|ξ1|3+m(t)+β212|ξ2|3+m(t), | (3.8) |
where
β211=ˉmm_+3(ˉm(2+2ˉm−2))3+ˉmm_(3+m_18)−3ˉm,β212=1m_+3(ˉm(2+2ˉm−2)αˉm−11)ˉm+3(m_+36(ˉm+2))−(ˉm+2). | (3.9) |
Substituting (3.8) into (3.7) yields
ξ31([ζ2]m(t)−[ζ∗2]m(t))≤13|ξ1|3+m(t)+β21|ξ2|3+m(t), | (3.10) |
where β21=β211+β212 is a positive constant.
By (3.2) and using Lemma 5 in [21], we get
|ζ2|m(t)=|ξ2+ζ∗2|m(t)≤(|ξ2|+|α1ξ1|)m(t)≤2ˉm−1(|ξ2|m(t)+|α1ξ1|m(t))≤2ˉm−1αˉm1(|ξ2|m(t)+|ξ1|m(t)), | (3.11) |
which means that
|ζ1|m(t)+|ζ2|m(t)≤φ1(|ξ2|m(t)+|ξ1|m(t)), | (3.12) |
where φ1=2ˉm−1αˉm1+1≥0 is a constant.
By (3.11) and using Lemma 1 in [19], we have
ξ32∂ζ∗2∂ζ1[ζ2]m(t)≤|ξ32||∂ζ∗2∂ζ1|2ˉm−1αˉm1(|ξ2|m(t)+|ξ1|m(t))≤2ˉm−1αˉm1|∂ζ∗2∂ζ1|(|ξ2|m(t)+3+|ξ32||ξ1|m(t)). | (3.13) |
By using Lemma 2.1 in [20], we get
2ˉm−1αˉm1|∂ζ∗2∂ζ1||ξ32||ξ1|m(t)≤13|ξ1|3+m(t)+β221(ζ1)|ξ2|3+m(t), | (3.14) |
where
β221(ζ1)=3m_+3(2ˉm−1αˉm1|∂ζ∗2∂ζ1|)ˉm+33(m_+33ˉm)−ˉm3. | (3.15) |
Substituting (3.14) into (3.13) yields
ξ32∂ζ∗2∂ζ1[ζ2]m(t)≤13|ξ1|3+m(t)+β22(ζ1)|ξ2|3+m(t), | (3.16) |
where β22(ζ1)=2ˉm−1αˉm1|∂ζ∗2∂ζ1|+β221(ζ1) is a smooth function irrelevant to m(t).
By (3.12), using Assumption 2.1 and Lemma 1 in [19], we get
32ξ22|fTγ(t)(ˉζ2)|2≤3˜f2(ζ1)|ξ2|2(|ζ1|m(t)+1+|ζ2|m(t)+1)≤3˜f2(ζ1)φ2(|ξ2|m(t)+3+|ξ2|2|ξ1|m(t)+1), | (3.17) |
where φ2=2ˉmαˉm+11+1≥0 is a constant.
From Lemma 2.1 in [20], we obtain
3˜f2(ζ1)φ2|ξ2|2|ξ1|m(t)+1≤13|ξ1|m(t)+3+β231(ζ1)|ξ2|m(t)+3, | (3.18) |
where
β231(ζ1)=2m_+3(3˜f2(ζ1)φ2)ˉm+32(m_+33(ˉm+1))−ˉm+12. | (3.19) |
Substituting (3.18) into (3.17) yields
32ξ22|fTγ(t)(ˉζ2)|2≤13|ξ1|m(t)+3+β23(ζ1)|ξ2|m(t)+3, | (3.20) |
where β23(ζ1)=3˜f2(ζ1)φ2+β231(ζ1)≥0 is a smooth function irrelevant to m(t).
By using (3.6), (3.10), (3.16) and (3.20), we obtain
LV2≤−(c1−1)|ξ1|m(t)+3+ξ32([u]m(t)−[x∗3]m(t))+ξ32[x∗3]m(t)+β2(ζ1)|ξ2|m(t)+3+IIV2, | (3.21) |
where β2(ζ1)=β21(ζ1)+β22(ζ1)+β23(ζ1) is a smooth function irrelevant to m(t).
Constructing the virtual controller as
x∗3=−(c2+β2(ζ1))1m_:=−α2(ζ1)ξ2, | (3.22) |
we have
ξ32[x∗3]m(t)=−αm(t)2(ζ1)ξm(t)+32≤−(c2+β2(ζ1))ξm(t)+32, | (3.23) |
where c2>0 is a constant and α2(ζ1)≥0 is a smooth function irrelevant to m(t).
Substituting (3.23) into (3.21) yields
LV2≤−(c1−1)|ξ1|m(t)+3−c2|ξ2|m(t)+3+ξ32([u]m(t)−[x∗3]m(t))+IIV2. | (3.24) |
In this part, we first design a reduced-order observer with a dynamic gain, then we design an output-feedback controller.
Since ζ2 are unmeasurable, we construct the following observer
dη=([u]m(t)−∂L(ζ1)∂ζ1[η+L(ζ1)]m(t))dt, | (3.25) |
where L(ζ1) is a smooth function, and ∂L(ζ1)∂ζ1>0 is irrelevant to m(t).
Defining e=ζ2−L(ζ1)−η and by the construction of the observer, we have
de=∂L(ζ1)∂ζ1([η+L(ζ1)]m(t)−[ζ2]m(t))dt+fTγ(t)(ˉζ2)dω. | (3.26) |
Choose U=14e4. From (3.26), we get
LU=e3∂L(ζ1)∂ζ1([η+L(ζ1)]m(t)−[ζ2]m(t))+32e2|fTγ(t)(ˉζ2)|2+IIU. | (3.27) |
By definition of e and lemma 2.2 in [22], we have
e3∂L(ζ1)∂ζ1([η+L(ζ1)]m(t)−[ζ2]m(t))≤−12ˉm−1∂L(ζ1)∂ζ1em(t)+3. | (3.28) |
From (3.12), (3.17) and Assumption 2.1, we get
32e2|fTγ(t)(ˉζ2)|2≤3˜f2(ζ1)|e|2(|ζ1|m(t)+1+|ζ2|m(t)+1)≤3˜f2(ζ1)φ2(|e|2|ξ2|m(t)+1+|e|2|ξ1|m(t)+1). | (3.29) |
By using Lemma 2.1 in [20], we have
3˜f2(ζ1)φ2|e|2|ξ1|1+m(t)≤|ξ1|3+m(t)+β31(ζ1)|e|3+m(t),3˜f2(ζ1)φ2|e|2|ξ2|1+m(t)≤12|ξ2|3+m(t)+β32(ζ1)|e|3+m(t), | (3.30) |
where
β31(ζ1)=2m_+3(3˜f2(ζ1)φ2)ˉm+32(m_+3ˉm+1)−ˉm+12,β32(ζ1)=2m_+3(3˜f2(ζ1)φ2)ˉm+32(3+m_2(1+ˉm))−1+ˉm2. | (3.31) |
Substituting (3.30) into (3.29) yields
32e2|fTγ(t)(ˉζ2)|2≤|ξ1|m(t)+3+12|ξ2|m(t)+3+β3(ζ1)|e|m(t)+3, | (3.32) |
where β3(ζ1)=β31(ζ1)+β32(ζ1)≥0 is a smooth function irrelevant to m(t).
Substituting (3.28), (3.32) into (3.27) yields
LU≤|ξ1|m(t)+3+12|ξ2|m(t)+3−(12ˉm−1∂L(ζ1)∂ζ1−β3(ζ1))|e|m(t)+3+IIU. | (3.33) |
Since ζ2 is unmeasurable, replace ζ2 in virtual controller x∗3 with η+L(ζ1), and we can get the controller as follows
u=−α2(ζ1)(η+L(ζ1)+α1ζ1). | (3.34) |
By (3.22), (3.24) and (3.34), we obtain
LV2≤−(c1−1)|ξ1|m(t)+3−c2|ξ2|m(t)+3+ξ32αˉm2(ζ1)([ξ2]m(t)−[ξ2−e]m(t))+IIV2. | (3.35) |
By using Lemma 1 in [19], we have
ξ32αˉm2(ζ1)([ξ2]m(t)−[ξ2−e]m(t))≤αˉm2(ζ1)ˉm(2ˉm−2+2)(|ξ2|3|e|m(t)+|e||ξ2|m(t)+2). | (3.36) |
By using Lemma 2.1 in [20], we get
αˉm2(ζ1)ˉm(2ˉm−2+2)|ξ2|3|e|m(t)≤14|ξ2|3+m(t)+β41(ζ1)|e|3+m(t),αˉm2(ζ1)ˉm(2ˉm−2+2)|e||ξ2|2+m(t)≤14|ξ2|3+m(t)+β42(ζ1)|e|3+m(t), | (3.37) |
where
β41(ζ1)=ˉmm_+3(αˉm2(ζ1)ˉm(2ˉm−2+2))ˉm+3m_(m_+312)−3ˉm,β42(ζ1)=1m_+3(αˉm2(ζ1)ˉm(2ˉm−2+2))ˉm+3(m_+34(ˉm+2))−(ˉm+2). | (3.38) |
Substituting (3.37) into (3.36) yields
ξ32αˉm2(ζ1)([ξ2]m(t)−[ξ2−e]m(t))≤12|ξ2|3+m(t)+β4(ζ1)|e|3+m(t), | (3.39) |
where β4(ζ1)=β41(ζ1)+β42(ζ1)≥0 is a smooth function irrelevant to m(t).
By using (3.39) and (3.35), we have
LV2≤−(c1−1)|ξ1|m(t)+3−(c2−12)|ξ2|m(t)+3+β4(ζ1)|e|m(t)+3+IIV2. | (3.40) |
Choosing V(ξ1,ξ2,e)=V2(ξ1,ξ2)+U(e), by (3.33) and (3.40), we obtain
LV≤−(c1−2)|ξ1|m(t)+3−(c2−1)|ξ2|m(t)+3−(12ˉm−1∂L(ζ1)∂ζ1−β3(ζ1)−β4(ζ1))|e|m(t)+3+IIV. | (3.41) |
Let
L(ζ1)=12ˉm−1(c3ζ1+∫ζ10(β3(s)+β4(s))ds), | (3.42) |
and the controller as
u=−α2(ζ1)(η+12ˉm−1(c3ζ1+∫ζ10(β3(s)+β4(s))ds)+α1ζ1), | (3.43) |
where c3>0 is a design parameter.
By using (3.41) and (3.42), we can obtain
LV≤−(c1−2)|ξ1|m(t)+3−(c2−1)|ξ2|m(t)+3−c3|e|m(t)+3+IIV. | (3.44) |
Remark 3.1. If m(t) is time-invariant and the growth rate is a constant rather than a smooth function, such as those in [7,8,9], from (3.32) and (3.39), β3 and β4 are constants irrelevant to ζ1. Then, the dynamic gain L(ζ) is a linear function of ζ1. We can design L(ζ1)=cζ1 by choosing the right parameter c to make LV in (3.41) negative definite. However, in this paper, the growth rate ˜f(ζ1) is a nonnegative smooth function and the m(t) is time-varying and non-differentiable, which makes the deducing of the dynamic gain much more difficult. To solve this problem, we introduce two constants m_ and ˉm, which are reasonably used in the design process, see (3.7) and (3.11). In this way, the dynamic gain (3.42) can be designed irrelevant to m(t), which is crucial to assure the effectiveness of the observer and controller. This is one of the main innovations of this paper.
In this section, for the closed-loop system (2.1), (3.25) and (3.43), we first give a lemma, which is useful to prove the system has a unique solution. Then, we present the main results of the stability analysis.
Lemma 4.1. For ζ∈R, the function g(ζ)=[ζ]m(t) satisfies the locally Lipschitz condition.
Proof. If ζ=0, we can get
h′+(0)=limζ→0+h(ζ)−h(0)ζ=0,h′−(0)=limζ→0−h(ζ)−h(0)ζ=0. | (4.1) |
Then, we have
dhdζ|ζ=0=h′+(0)=h′−(0)=0, | (4.2) |
thus, h(ζ) is differentiable function in ζ=0 and so meets the locally Lipschitz condition in ζ=0.
As ζ>0, we get
h(ζ)=[ζ]m(t)=ζm(t). | (4.3) |
For m(t)≥1, h(ζ) is differentiable function in ζ>0, so meets the locally Lipschitz condition in ζ>0. Similarly, as ζ<0, the conclusion is valid.
Therefore, the conclusion holds for ζ∈R.
Next, we give the stability results.
Theorem 4.1. Under Assumption 2.1, for the system (2.1), using the observer (3.25) and controller (3.43) with
ci>3−i,i=1,2,3, | (4.4) |
we can get
1) For each ζ(t0)=ζ0∈R2 and γ(t0)=i0∈S, the closed-loop system has an almost surely unique solution on [0,+∞);
2) For any ζ0∈R2 and i0∈S, the closed-loop system is almost surely regulated to the equilibrium at the origin.
Proof. By (2.1), (3.25), (3.43) and using Lemma 4.1, we can conclude that the closed-loop system satisfies the locally Lipschitz condition. By (3.2), (3.22), (3.25) and (3.42), we can get that ξ1,ξ2,η are bounded, which implies that ζ1 is bounded, which means that
VR=inft≥t0,|ζ|>RV(ζ(t))→∞⟺R→∞. | (4.5) |
Through the verification of the controller development process, we choose appropriate design parameters ci to satisfy (4.4), and we can get IIV=0. For each l>0, the first exit time is defined as
σl=inf{t:t≥t0,|ζ(t)|≥l}. | (4.6) |
When t≥t0, choose tl=min{σl,t}. We can obtain that bounded |ζ(t)| on interval [t0,tl] a.s., which means that V(ζ) is bounded in the interval [t0,tl] a.s. By using (3.44), we can get that LV is bounded in the interval [t0,tl] a.s. By using Lemma 1.9 in [11], (3.44) and (4.4), we can obtain
EV(ζ(tl))≤EV(ζ(t0)). | (4.7) |
By (4.5), (4.7) and using Lemma 1 in [23], we can obtain conclusion (1).
From (3.44), (4.5), by using Theorem 2.1 in [24], we can prove conclusion (2).
In this section, a simulation example is given to show the availability of the control method.
Study the stabilization for system with two modes. The Markov process γ(t) belongs to the space S={1,2} with generator Γ=(λij)2×2 given by λ11=2,λ12=−2,λ21=−1 and λ22=1. We have π1=13,π2=23. When γ(t)=1, the systems can be written as
dζ1=[ζ2]32+12sintdt,dζ2=[u]32+12sintdt+ζ1sinζ2dω,y=ζ1, | (5.1) |
where m(t)=32+12sint,m_=1,ˉm=2. When γ(t)=2, the systems are described by
dζ1=[ζ2]2+sintdt,dζ2=[u]2+sintdt+12ζ21sinζ2dω,y=ζ1, | (5.2) |
where m(t)=2+sint,m_=1,ˉm=3. Clearly, system (5.1) and (5.2) satisfy Assumption 2.1.
According to the above design process, when γ(t)=1, the observer is constructed as
dη=([u]32+12sint−∂L(ζ1)∂ζ1[η+L(ζ1)]32+12sint)dt, | (5.3) |
and the control is
u=−(c2+4ζ21)(η+L(ζ1)+c1ζ1), | (5.4) |
where L(ζ1)=12(c3ζ1+6ζ21).
When γ(t)=2, the observer is constructed as
dη=([u]2+sint−∂L(ζ1)∂ζ1[η+L(ζ1)]2+sint)dt, | (5.5) |
and the control is
u=−(c2+4ζ1+12ζ21)(η+L(ζ1)+c1ζ1), | (5.6) |
where L(ζ1)=14(c3ζ1+20ζ1+4ζ21).
For simulation, we select c1=6,c2=6,c3=5, and the initial conditions as ζ1(0)=−1,ζ2(0)=2,η(0)=−5. We can obtain Figure 1, which illustrates that the signals of the closed-loop system (ζ1,ζ2,u,η,e) converge to zero. Specifically, the states and controller of the closed-loop system converge to zero. The observation error also converges to zero, which means that our constructed observer and controller are efficient. Figure 2 illustrates the jump of Markov process γ(t) in 1 and 2.
Remark 5.1. It can be observed from the example that there are time-varying powers and Markovian switching in systems (5.1) and (5.2). For the output-feedback control of the system (5.1) and (5.2), the method in [7,8,9] fails since they can only deal with time-invariant powers without Markovian switching. To solve the difficulties caused by time-varying powers, we introduce constants 1, 2, and 1, 3 so that the design of the observer and controller is irrelevant to the power. This is one of the characteristics of our controller and observer design scheme (5.3)–(5.6).
We investigate the output-feedback stabilization for stochastic nonlinear systems with both Markovian switching and time-varying powers in this paper. Compared with existing work, the system model considered in this paper is more general because it studies the time-varying power and Markovian switching, simultaneously. To achieve stabilization, we first design a state observer with a dynamic gain and an output-feedback controller, then use advanced stochastic analysis techniques to prove that the closed-loop system has an almost surely unique solution and the states are regulated to the origin almost surely. Even though there is no Markovian switching, the results in this paper are also new in the sense that we consider nonlinear growth rate, which is much more general than constant growth rate cases in [7,8,9].
There are many related problems to be considered, such as how to extend the result to impulsive systems [25,26,27] and systems with arbitrary order.
This work is funded by Shandong Province Higher Educational Excellent Youth Innovation team, China (No. 2019KJN017), and Shandong Provincial Natural Science Foundation for Distinguished Young Scholars, China (No. ZR2019JQ22).
The authors declare there is no conflict of interest.
[1] |
Acquaye AA, Wiedmann T, Feng K, et al. (2011) Identification of 'carbon hot-spots' and quantification of GHG intensities in the biodiesel supply chain using hybrid LCA and structural path analysis. Environ Sci Technol 45: 2471-2478. doi: 10.1021/es103410q
![]() |
[2] |
Aroche-Reyes F (2003) A qualitative input-output method to find basic economic structures. Pap Regional Sci 82: 581-590. doi: 10.1007/s10110-003-0149-z
![]() |
[3] | Castaño A, Lufin M, Atienza M (2019) A structural path analysis of Chilean mining linkages between 1995 and 2011. What are the channels through which extractive activity affects the economy? Resour Policy 60: 106-117. |
[4] |
Defourny J, Thorbecke E (1984) Structural path analysis and multiplier decomposition within a social accounting matrix framework. Econ J 94: 111-136. doi: 10.2307/2232220
![]() |
[5] |
do Amaral JF, Dias J, Lopes JC (2007) Complexity as interdependence in input-output systems. Environ Planning A 39: 1770-1782. doi: 10.1068/a38214
![]() |
[6] |
Gui S, Mu H, Li N (2014) Analysis of impact factors on China's CO2 emissions from the view of supply chain paths. Energy 74: 405-416. doi: 10.1016/j.energy.2014.06.116
![]() |
[7] |
Gunluk-Senesen G, Kaya T, Senesen U (2018) Promoting investment in the Turkish construction sector: a structural path analysis. Econ Syst Res 30:422-438. doi: 10.1080/09535314.2018.1477739
![]() |
[8] |
Hong J, Shen GQ, Li CZ, et al. (2018) An integrated framework for embodied energy quantification of buildings in China: A multi-regional perspective. Resour Conserv Recycl 138: 183-193. doi: 10.1016/j.resconrec.2018.06.016
![]() |
[9] |
Hong J, Shen Q, Xue F (2016) A multi-regional structural path analysis of the energy supply chain in China's construction industry. Energy Policy 92: 56-68. doi: 10.1016/j.enpol.2016.01.017
![]() |
[10] |
Huang YA, Lenzen M, Weber CL, et al. (2009) The role of input-output analysis for the screening of corporate carbon footprints. Econ Syst Res 21: 217-242. doi: 10.1080/09535310903541348
![]() |
[11] |
Itoh H (2016) Understanding of economic spillover mechanism by structural path analysis: a case study of interregional social accounting matrix focused on institutional sectors in Japan. J Econ Struct 5: 22. doi: 10.1186/s40008-016-0052-9
![]() |
[12] |
Kanemoto K, Moran D, Lenzen M, et al. (2014) International trade undermines national emission reduction targets: New evidence from air pollution. Global Environ Change 24: 52-59. doi: 10.1016/j.gloenvcha.2013.09.008
![]() |
[13] |
Lenzen M (2002) A guide for compiling inventories in hybrid life-cycle assessments: some Australian results. J Cleaner Prod 10: 545-572. doi: 10.1016/S0959-6526(02)00007-0
![]() |
[14] |
Lenzen M (2003) Environmentally important paths, linkages and key sectors in the Australian economy. Struct Change Econ Dyn 14: 1-34. doi: 10.1016/S0954-349X(02)00025-5
![]() |
[15] |
Lenzen M (2007) Structural path analysis of ecosystem networks. Ecol Model 200: 334-342. doi: 10.1016/j.ecolmodel.2006.07.041
![]() |
[16] |
Lenzen M, Murray J (2010) Conceptualising environmental responsibility. Ecol Econ 70: 261-270. doi: 10.1016/j.ecolecon.2010.04.005
![]() |
[17] |
Li Y, Su B, Dasgupta S (2018) Structural path analysis of India's carbon emissions using input-output and social accounting matrix frameworks. Energy Econ 76: 457-469. doi: 10.1016/j.eneco.2018.10.029
![]() |
[18] |
Liang S, Qu S, Xu M (2016) Betweenness-based method to identify critical transmission sectors for supply chain environmental pressure mitigation. Environ Sci Technol 50: 1330-1337. doi: 10.1021/acs.est.5b04855
![]() |
[19] |
Liang S, Wang Y, Zhang T, et al. (2017) Structural analysis of material flows in China based on physical and monetary input-output models. J Cleaner Prod 158: 209-217. doi: 10.1016/j.jclepro.2017.04.171
![]() |
[20] |
Llop M, Ponce-Alifonso X (2015) Identifying the role of final consumption in structural path analysis: an application to water uses. Ecol Econ 109: 203-210. doi: 10.1016/j.ecolecon.2014.11.011
![]() |
[21] | Mattila T (2012) Any sustainable decoupling in the Finnish economy? A comparison of the pathways and sensitivities of GDP and ecological footprint 2002-2005. Ecol Indic 16: 128-134. |
[22] | Meng J, Liu J, Xu Y, et al. (2015) Tracing Primary PM2.5 emissions via Chinese supply chains. Environ Res Lett 10: 054005. |
[23] |
Mo W, Zhang Q, Mihelcic JR, et al. (2011) Embodied energy comparison of surface water and groundwater supply options. Water Res 45: 5577-5586. doi: 10.1016/j.watres.2011.08.016
![]() |
[24] |
Muñiz ASG (2013) Input-output research in structural equivalence: Extracting paths and similarities. Econ Model 31: 796-803. doi: 10.1016/j.econmod.2013.01.016
![]() |
[25] | Ngandu S, Garcia AF, Arndt C (2010) The economic influence of infrastructural expenditure in South Africa: A multiplier and structural path analysis. |
[26] |
Oshita Y (2012) Identifying critical supply chain paths that drive changes in CO2 emissions. Energy Econ 34: 1041-1050. doi: 10.1016/j.eneco.2011.08.013
![]() |
[27] | Oshita Y, Kikuchi Y, OSHITA Y (2014) Flow analysis on products of agriculture, forestry, fisheries industry using structural path analysis. In Proc. 22nd Int. Input-Output Conference. |
[28] |
Owen A, Scott K, Barrett J (2018) Identifying critical supply chains and final products: An input-output approach to exploring the energy-water-food nexus. Appl Energy 210: 632-642. doi: 10.1016/j.apenergy.2017.09.069
![]() |
[29] |
Owen A, Wood R, Barrett J, et al. (2016) Explaining value chain differences in MRIO databases through structural path decomposition. Econ Syst Res 28: 243-272. doi: 10.1080/09535314.2015.1135309
![]() |
[30] |
Peng J, Xie R, Lai M (2018) Energy-related CO2 emissions in the China's iron and steel industry: a global supply chain analysis. Resour Conserv Recycl 129: 392-401. doi: 10.1016/j.resconrec.2016.09.019
![]() |
[31] |
Peters GP, Hertwich EG (2006) Structural analysis of international trade: Environmental impacts of Norway. Econ Syst Res 18: 155-181. doi: 10.1080/09535310600653008
![]() |
[32] | Qu X, Meng J, Sun X, et al. (2017) Demand-driven primary energy requirements by Chinese economy 2012. In 8th International conference on applied energy (ICAE2016), Elsevier, 105: 3132-3137. |
[33] |
Seung CK (2016) Identifying channels of economic impacts: An inter-regional structural path analysis for Alaska fisheries. Marine Policy 66: 39-49. doi: 10.1016/j.marpol.2016.01.015
![]() |
[34] |
Shao L, Li Y, Feng K, et al. (2018) Carbon emission imbalances and the structural paths of Chinese regions. Appl Energy 215: 396-404. doi: 10.1016/j.apenergy.2018.01.090
![]() |
[35] |
Sonis M, Hewings GJ (1998) Economic complexity as network complication: Multiregional input-output structural path analysis. Ann Reg Sci 32: 407-436. doi: 10.1007/s001680050081
![]() |
[36] |
Sonis M, Hewings GJ, Sulistyowati S (1997) Block structural path analysis: applications to structural changes in the Indonesian economy. Econ Syst Res 9: 265-280. doi: 10.1080/09535319700000020
![]() |
[37] | Thorbecke E (2017) Social accounting matrices and social accounting analysis, In Methods interregional and regional analysis, Routledge, 281-332. |
[38] |
Tian Y, Xiong S, Ma X, et al. (2018) Structural path decomposition of carbon emission: A study of China's manufacturing industry. J Cleaner Prod 193: 563-574. doi: 10.1016/j.jclepro.2018.05.047
![]() |
[39] |
Treloar GJ (1997) Extracting embodied energy paths from input-output tables: towards an input-output-based hybrid energy analysis method. Econ Syst Res 9: 375-391. doi: 10.1080/09535319700000032
![]() |
[40] |
Wang J, Du T, Wang H, et al. (2019) Identifying critical sectors and supply chain paths for the consumption of domestic resource extraction in China. Jo Cleaner Prod 208: 1577-1586. doi: 10.1016/j.jclepro.2018.10.151
![]() |
[41] |
Wang Z, Cui C, Peng S (2018) Critical sectors and paths for climate change mitigation within supply chain networks. J Environ Manage 226: 30-36. doi: 10.1016/j.jenvman.2018.08.018
![]() |
[42] |
Wang Z, Wei L, Niu B, et al. (2017) Controlling embedded carbon emissions of sectors along the supply chains: A perspective of the power-of-pull approach. Appl Energy 206: 1544-1551. doi: 10.1016/j.apenergy.2017.09.108
![]() |
[43] |
Wilting HC, van Oorschot MM (2017) Quantifying biodiversity footprints of Dutch economic sectors: A global supply-chain analysis. J Cleaner Prod 156: 194-202. doi: 10.1016/j.jclepro.2017.04.066
![]() |
[44] | Wood R (2008) Spatial structural path analysis: Analysing the greenhouse impacts of trade substitution. In International Input-Output Meeting on Managing the Environment, 9-11. |
[45] |
Wood R, Lenzen M (2003) An application of a modified ecological footprint method and structural path analysis in a comparative institutional study. Local Environ 8: 365-386. doi: 10.1080/13549830306670
![]() |
[46] |
Wood R, Lenzen M (2009) Structural path decomposition. Energy Econ 31: 335-341. doi: 10.1016/j.eneco.2008.11.003
![]() |
[47] |
Wu F, Sun Z, Wang F, et al. (2018) Identification of the critical transmission sectors and typology of industrial water use for supply-chain water pressure mitigation. Resour Conserv Recycl 131: 305-312. doi: 10.1016/j.resconrec.2017.10.024
![]() |
[48] |
Yang X, Zhang W, Fan J, et al. (2018) The temporal variation of SO2 emissions embodied in Chinese supply chains, 2002-2012. Environ Pollut 241: 172-181. doi: 10.1016/j.envpol.2018.05.052
![]() |
[49] |
Yang Z, Dong W, Xiu J, et al. (2015) Structural path analysis of fossil fuel based CO2 emissions: a case study for China. PloS one 10: e0135727. doi: 10.1371/journal.pone.0135727
![]() |
[50] |
Zhang B, Guan S, Wu X, et al. (2018) Tracing natural resource uses via China's supply chains. J Cleaner Product 196: 880-888. doi: 10.1016/j.jclepro.2018.06.109
![]() |
[51] |
Zhang B, Qu X, Meng J, et al. (2017) Identifying primary energy requirements in structural path analysis: a case study of China 2012. Appl Energy 191: 425-435. doi: 10.1016/j.apenergy.2017.01.066
![]() |
1. | Xinling Li, Xueli Qin, Zhiwei Wan, Weipeng Tai, Chaos synchronization of stochastic time-delay Lur'e systems: An asynchronous and adaptive event-triggered control approach, 2023, 31, 2688-1594, 5589, 10.3934/era.2023284 | |
2. | Jingya Wang, Ye Zhu, L2−L∞ control for memristive NNs with non-necessarily differentiable time-varying delay, 2023, 20, 1551-0018, 13182, 10.3934/mbe.2023588 |