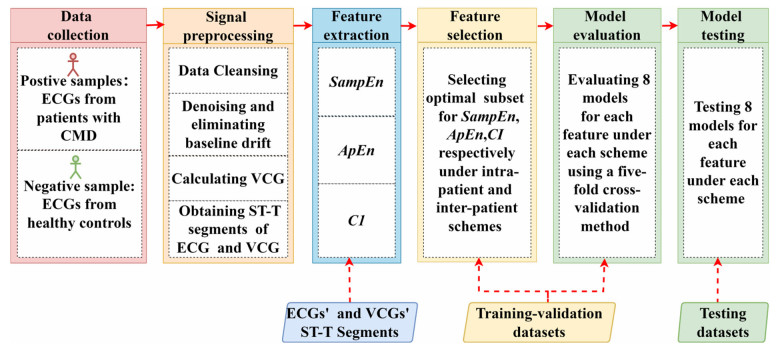
The COVID-19 pandemic has driven an upheaval of new research, providing key insights into the pathogenesis of this disease. Lymphocytopenia, hyper-inflammation and cardiac involvement are prominent features of the disease and have prognostic value. However, the mechanistic links among these phenomena are not well understood. Likewise, some COVID-19 patients exhibit multi-organ failure with diseases affecting the cardiac system, appearing to be an emerging feature of the COVID-19 pandemic. Neutrophil extracellular traps (NETs) have been frequently correlated with larger infarct sizes and can predict major adverse cardiac events. However, the exact mechanism behind this remains unknown. Although the excessive NET formation can drive inflammation, particularly endothelial and promote thrombosis, it is essential to normal immunity. In this paper, we postulate the role of NETs in cardiac disease by providing an overview of the relationship between NET and inflammasome activities in lung and liver diseases, speculating a link between these entities in cardiac diseases as well. Future research is required to specify the role of NETs in COVID-19, since this carries potential therapeutic significance, as inhibition of NETosis could alleviate symptoms of this disease. Knowledge gained from this could serve to inform the assessment and therapeutics of other hyper inflammatory diseases affecting the heart and vasculature alike.
Citation: Amal Feiroze Farouk, Areez Shafqat, Shameel Shafqat, Junaid Kashir, Khaled Alkattan, Ahmed Yaqinuddin. COVID-19 associated cardiac disease: Is there a role of neutrophil extracellular traps in pathogenesis?[J]. AIMS Molecular Science, 2021, 8(4): 275-290. doi: 10.3934/molsci.2021021
[1] | Jucheng Zhang, Minwen Ma, Huajun Li, Zhaoxia Pu, Haipeng Liu, Tianhai Huang, Huan Cheng, Yinglan Gong, Yonghua Chu, Zhikang Wang, Jun Jiang, Ling Xia . Early diagnosis of coronary microvascular dysfunction by myocardial contrast stress echocardiography. Mathematical Biosciences and Engineering, 2023, 20(5): 7845-7858. doi: 10.3934/mbe.2023339 |
[2] | Li Cai, Qian Zhong, Juan Xu, Yuan Huang, Hao Gao . A lumped parameter model for evaluating coronary artery blood supply capacity. Mathematical Biosciences and Engineering, 2024, 21(4): 5838-5862. doi: 10.3934/mbe.2024258 |
[3] | Xintong Wu, Yingyi Geng, Xinhong Wang, Jucheng Zhang, Ling Xia . Continuous extraction of coronary artery centerline from cardiac CTA images using a regression-based method. Mathematical Biosciences and Engineering, 2023, 20(3): 4988-5003. doi: 10.3934/mbe.2023231 |
[4] | Keyue Yan, Tengyue Li, João Alexandre Lobo Marques, Juntao Gao, Simon James Fong . A review on multimodal machine learning in medical diagnostics. Mathematical Biosciences and Engineering, 2023, 20(5): 8708-8726. doi: 10.3934/mbe.2023382 |
[5] | Honghui Zhang, Jun Xia, Yinlong Yang, Qingqing Yang, Hongfang Song, Jinjie Xie, Yue Ma, Yang Hou, Aike Qiao . Branch flow distribution approach and its application in the calculation of fractional flow reserve in stenotic coronary artery. Mathematical Biosciences and Engineering, 2021, 18(5): 5978-5994. doi: 10.3934/mbe.2021299 |
[6] | Huan Cheng, Jucheng Zhang, Yinglan Gong, Zhaoxia Pu, Jun Jiang, Yonghua Chu, Ling Xia . Semantic segmentation method for myocardial contrast echocardiogram based on DeepLabV3+ deep learning architecture. Mathematical Biosciences and Engineering, 2023, 20(2): 2081-2093. doi: 10.3934/mbe.2023096 |
[7] | Ziyu Jin, Ning Li . Diagnosis of each main coronary artery stenosis based on whale optimization algorithm and stacking model. Mathematical Biosciences and Engineering, 2022, 19(5): 4568-4591. doi: 10.3934/mbe.2022211 |
[8] | Yajing Zeng, Siyu Yang, Xiongkai Yu, Wenting Lin, Wei Wang, Jijun Tong, Shudong Xia . A multimodal parallel method for left ventricular dysfunction identification based on phonocardiogram and electrocardiogram signals synchronous analysis. Mathematical Biosciences and Engineering, 2022, 19(9): 9612-9635. doi: 10.3934/mbe.2022447 |
[9] | Ya'nan Wang, Sen Liu, Haijun Jia, Xintao Deng, Chunpu Li, Aiguo Wang, Cuiwei Yang . A two-step method for paroxysmal atrial fibrillation event detection based on machine learning. Mathematical Biosciences and Engineering, 2022, 19(10): 9877-9894. doi: 10.3934/mbe.2022460 |
[10] | Lili Jiang, Sirong Chen, Yuanhui Wu, Da Zhou, Lihua Duan . Prediction of coronary heart disease in gout patients using machine learning models. Mathematical Biosciences and Engineering, 2023, 20(3): 4574-4591. doi: 10.3934/mbe.2023212 |
The COVID-19 pandemic has driven an upheaval of new research, providing key insights into the pathogenesis of this disease. Lymphocytopenia, hyper-inflammation and cardiac involvement are prominent features of the disease and have prognostic value. However, the mechanistic links among these phenomena are not well understood. Likewise, some COVID-19 patients exhibit multi-organ failure with diseases affecting the cardiac system, appearing to be an emerging feature of the COVID-19 pandemic. Neutrophil extracellular traps (NETs) have been frequently correlated with larger infarct sizes and can predict major adverse cardiac events. However, the exact mechanism behind this remains unknown. Although the excessive NET formation can drive inflammation, particularly endothelial and promote thrombosis, it is essential to normal immunity. In this paper, we postulate the role of NETs in cardiac disease by providing an overview of the relationship between NET and inflammasome activities in lung and liver diseases, speculating a link between these entities in cardiac diseases as well. Future research is required to specify the role of NETs in COVID-19, since this carries potential therapeutic significance, as inhibition of NETosis could alleviate symptoms of this disease. Knowledge gained from this could serve to inform the assessment and therapeutics of other hyper inflammatory diseases affecting the heart and vasculature alike.
Clinically, myocardial ischemia refers to a condition in which the perfusion of heart muscle is insufficient, resulting in the reduction of oxygen supply to the heart, abnormal myocardial energy metabolism, and abnormal work of the heart [1]. It can increase cardiovascular events including sudden cardiac death and acute myocardial infarction [2,3,4], contributes 16% to the world's total deaths, and has been considered as the leading cause of mortality. Obstructive coronary artery disease (CAD), defined as severe stenosis in any epicardial coronary arteries (diameter ~ 5 mm) determined via coronary angiography (CAG, diameter severity [DS] ≥ 50%) or a fractional flow reserve (FFR < 0.8) [5], had been quantitatively investigated and well established as a main etiology of myocardial ischemia. However, up to 70% of patients undergoing CAG do not have obstructive CAD [6]. Patients with symptoms and signs of ischemic heart disease (IHD) but found to have nonobstructive coronary arteries (INOCA) are increasingly recognized [7]. Coronary microvascular dysfunction (CMD) has been recognized as a major cause of INOCA by the latest European Society of Cardiology guidelines [8,9]. Since the coronary microvasculature (diameter < 500 μm) has remained elusive to conventional medical images [10], the clinical significance of CMD has not been fully understood [11].
CMD refers to impaired blood flow in the coronary microcirculation [11,12]. CMD can be caused by structural or functional abnormalities in the coronary microcirculation, resulting in an inefficacy increase of myocardial blood supply in response to an increased myocardial oxygen demand or coronary microvascular spasm [8,13] and eventually leading to myocardial ischemia. Patients with CMD have higher risks of poor prognosis, re-hospitalization, adverse cardiovascular events, and mortality [9,14]. Recently, the functional assessment or detection of CMD has gained much popularity due to the substantial proportion of patients developing symptoms and signs of myocardial ischemia, in spite of the absence of obstructive CAD, or after the well-established treatment of obstructive CAD [5]. The prevalence of CMD has reported to be 50 to 65% of angina patients with INOCA [14], and more than 50% in patients with obstructive CAD [15]. CMD is common but easy to be ignored [16]. Therefore, making an early and accurate diagnosis of CMD plays an increasingly critical role in clinical practice. However, the natural course of CMD tends to be relatively long and asymptomatic, making early diagnosis difficult [17], and there is no reliable noninvasive test available.
Currently, the gold standard of diagnostic criteria for CMD is the invasive measurement of index of microcirculatory resistance (IMR) and FFR [6,18,19,20]. They are not applicable for the early detection of CMD, given that only a minority of inpatient with acute chest pain meets the clinical threshold that requires invasive testing. Besides the invasive measurement of IMR, methods have been developed for the non-invasive evaluation of cardiac microcirculation, including Doppler echocardiography, stress cardiac magnetic resonance imaging, and computational fluid simulation [13]. However, these techniques are not applied as an accessible tool for early detection of CMD in daily clinical practice due to their radiation, high cost, invasiveness, and complicated operation [21]. Since the 2010s, coronary microcirculatory dysfunction has been focused on as a main etiology of myocardial ischemia [20]. There is an increasing clinical demand for a non-invasive, accessible, and cost-efficient tool to recognize patients with a high likelihood of CMD who may subsequently be referred for invasive detection and further diagnosis [22].
In comparison, electrocardiogram (ECG)-based analysis is easy-to-use, low-cost, without the requirement of expertise or expensive devices. CMD can lead to ischemic ECG changes [22,23], which provides the possibility of ECG-based non-invasive detection of CMD. Specially, standard deviation of normal R-R intervals (SDNN) < 100 ms [24], ischemic ST-segment changes [25,26], the prolongation of the heart rate-corrected QT interval [27,28], and variations in T wave [22,27] have been validated as indicators of CMD in patients with INOCA. Sara et al. extracted three features from T wave and developed a linear discriminant classifier which yielded the sensitivity and specificity of 65.9 and 67.7% in detecting CMD [22]. These studies preliminarily validated the effectiveness of ECG-based features in detecting CMD, which provides the possibility of computer-aided CMD detection method using machine learning algorithm. Nevertheless, there are very few computer-aided diagnostic algorithms based on ECG-based features for CMD detection, given that previous studies mainly focused on detecting obstructive CAD [29].
Entropy is a common measure of complexity of time series [30]. Myocardial ischemia-induced variations in waveform and frequency components at certain ECG segments can be reflected in the changes of its entropy. Sample entropy (SampEn), approximate entropy (ApEn), and multiscale entropy (MSE) have been extracted from heart rate variability [31], ST-segments [32], or filtered 12-lead ECGs [33] for evaluating the changes of complexity in ECGs from patients with obstructive CAD [34]. However, the performances of these entropies in detecting CMD are yet to be explored. Therefore, we aim to investigate whether SampEn, ApEn, and MSE extracted from beat-to-beat ST-T segments of each lead in ECG and vectorcardiography (VCG) could reliably detect CMD, and develop an ECG-based CMD detection algorithm which will lay the groundwork for patient-specific, early, and non-invasive detection of CMD by comparing different entropy subsets and machine learning models.
Our work contains five parts: data collection, signal preprocessing, feature extraction, feature selection, and model evaluation and testing, as shown in Figure 1. Firstly, 12-lead ECGs from CMD patients and healthy controls were collected and these from healthy controls were split into 10-second (10 s) segments. After 10 s ECGs were cleansed and denoised, VCGs were synthesized from 12-lead ECGs mathematically. The ST-wave onset and T-wave offset were located on VCGs and ECGs employing a squeeze and regional approach respectively. Three entropy features, i.e., SampEn, ApEn, and MSE-derived complexity index (CI), were extracted from the time series obtained by splicing ST-T segments of each lead in ECGs and VCGs beat by beat. Subsequently, the most effective subset of each entropy feature for each model was determined using the sequential backward selection (SBS) algorithm under the intra-patient and inter-patient schemes. Under each scheme, eight different machine learning models for each entropy feature were comprehensively evaluated on a five-fold cross-validation method on the training-validation datasets at the very first place, and then tested on the corresponding testing datasets for every scenario. Finally, the best model for CMD detection was investigated from the three entropy-based models (i.e., SampEn-based, ApEn-based, and CI-based) under each scheme.
In our study, ECGs and the clinical characteristics of 177 subjects in two cohorts were collected. Regard to positive samples, 99 10 s, 12-lead resting ECG recordings of 70 patients with CMD were obtained from the Second Affiliated Hospital of Zhejiang University, Zhejiang, China, from June 2019 to September 2022 with approval from the local ethics committee for sharing and analyzing retrospective anonymised patient data with informed consent form waived.
Inclusion criteria were described as follows: 1) existence of stable or chronic symptoms of myocardial ischemia; 2) presence of coronary stenosis assessed by CAG but a FFR > 0.8; 3) presence of symptoms of chest pain or tightness but no obvious evidence of myocardial ischemia; 4) presence of objective evidence of myocardial ischemia.
Exclusion criteria applied to the study population were given by: 1) existence of unsuccessful measurement of FFR or IMR; 2) presence of myocardial infarction in the target vessel within 72 hours; 3) existence of isotonic creatinine > 150 µmol/L, glomerular filtration rate < 45 mL/kg/m2, or left ventricular ejection fraction < 30%; 4) existence of the following diseases: bundle branch blocks, atrial fibrillation, left ventricular hypertrophy, congestive heart failure, pulmonary arterial hypertension, or heart valve disease.
CMD was diagnosed according to the standardized diagnostic criteria proposed by COVADIS (Coronary Vasomotor Disorders International Study Group): IMR ≥ 25 in the absence of overt obstructive CAD (FFR > 0.80) [12,35], as illustrated in Figure 3. FFR and IMR were measured in the usual fashion by employing a coronary guidewire (St. Jude Medical Inc., PressureWireCertus, C12008) placed in target artery during CAG [35].
Finally, patients with CMD were selected whilst patients with CAD were ruled out, as shown in Figure 3. Totally, 99 10 s, 12-lead ECGs (sampling rate: 500 Hz; resolution: 16-bit with 4 μV/LSB) were recorded utilizing a commercially available electrocardiograph (Netherlands Philips Electronics Co. LTD, PageWriter TC30). The clinical characteristics of CMD patients are listed in Table 1.
Characteristics | Value* |
Age, years | 67.29 ± 9.23 |
Female, n (%) | 26/70 |
Hypertension, n (%) | 32/70 |
Diabetes mellitus, n (%) | 22/70 |
Heart rate, bpm | 70.24 ± 12.87 |
Systolic blood pressure, mmHg | 130.82 ± 15.34 |
Diastolic blood Pressure, mmHg | 73.43 ± 10.45 |
Smoking history, n (%) | 12/70 |
Family history of CAD, n (%) | 12/70 |
*The categorical data are provided as numbers and percentages, and all of numerical variables are afforded in the form mean ± standard deviation for normally distributed data. |
Regarding negative samples, 107 12-lead ECGs (duration: ⩾10 seconds; sampling rate: 500 Hz; resolution: 16-bit with 1 mV/LSB) were collected from 107 healthy controls (age: 36.3 ± 15.1 years, 73 females and 34 males) of China Physiological Signal Challenge in 2018 database [36].
After ECGs from healthy controls were segmented into 10 s ones, data cleansing was implemented to guarantee the quality of ECG signals. Low-quality ECGs resulted from the shedding of the leads or/and poor attachment between the lead and body surface were eliminated, as illustrated in Figure 4. After data cleansing, in total, 301 10 s, 12-lead ECGs (99 CMD, 202 controls) were finally selected for further analysis.
Then, the baseline drift and low-frequency fluctuations were removed using two moving median filters connected in cascade (with length 1.2 and 0.6 seconds respectively) to maximally avoid the deformation of ST segment in comparison with the classical high-pass filter. The output of first median filter was fed into the second one. High-frequency power-line interference and electromyogram noise were eliminated employing discrete wavelet transform (DWT). In detail, Coif4 determined as a wavelet basis function was adopted to decompose noise-containing ECGs up to four levels [37]. After the elimination of noise by an adaptive threshold, the processed signals were transformed back by utilizing the inverse of DWT [37].
Subsequently, all 10 s, 12-lead ECGs were standardized utilizing the clinical standard gain [37,38] and then transformed into VCGs [39], as shown in Figure 5(C−F).
[VxVyVz]=D⋅FT | (1) |
where D=[0.38−0.07−0.130.05−0.010.140.060.54−0.070.930.06−0.02−0.050.06−0.170.130.11−0.23−0.43−0.06−0.14−0.20−0.110.31],
F=[IIIV1V2V3V4V5V6]; I, II, V1, V2, V3, V4, V5, and V6 are leads of ECG; Vx, Vy, and Vz are leads of VCG.
Ultimately, the ST-segment onset and T-wave offset were simultaneously marked on the VCGs and ECGs employing a hybrid approach [40] to obtain ST-T segments for calculating entropies, as shown in Figure 5(B−E). ST-wave onsets were located using a squeeze algorithm, and T-wave offsets using a regional approach [40].
Sample entropy (SampEn), approximate entropy (ApEn), and multiscale entropy (MSE) have been demonstrated to be electrocardiographic indictors for evaluating the changes of complexity in ECGs from patients with obstructive CAD [33,34]. Similarly, SampEn, ApEn, and MSE-derived CI were calculated from ST-T segments of each VCG and ECG lead to assess the changes of complexity in ECGs induced by CMD.
For each lead in VCGs or ECGs, its time series, i.e., {t(l)}=t(1),t(2),...,t(L) was obtained by splicing ST-T segments beat by beat and standardized as follows:
t(l)=t(l)−μσ | (2) |
where L presents the length of the time series; σ and μ are the standard deviation and mean value of the time series.
SampEn is defined as the negative natural logarithm of a conditional probability [30] and calculated to measure the complexity of beat-to-beat ST-T segment in this work. For each VCG and ECG lead, SampEn (Si,i=Vx,Vy,Vz,I,II,III,avR,avL,avF,V1,V2,V3,V4,V5,V6) was calculated from the time series, i.e., {t(l)}=t(1),t(2),...,t(L) as follows [37,41]:
SampEn(L,m,ε)=−lnψm+1(ε)ψm(ε) | (3) |
where ψm(ε) and ψm+1(ε) are the average probability that two vectors coincide for m and m+1 points, respectively; ε denotes the tolerance for accepting matches, and m refers to embedded dimension [30].
In this work, the value of ε was set to 0.1, and that of m was set to 2 [30,37].
In our work, ApEn (Ai,i=Vx,Vy,Vz,I,II,III,avR,avL,avF,V1,V2,V3,V4,V5,V6) of time series {t(l)}=t(1),t(2),...,t(L) of each lead in ECGs and VCGs were calculated [30,41].
Firstly, the vectors Tm(1),...,Tm(L−m+1) and Tm+1(1),...,Tm+1(L−m) with a dimension of m and m+1 were formed to be Tm(z)={t(z),t(z+1),...,t(z+m−1)},1⩽z⩽L−m+1 and Tm+1(z)={t(z),t(z+1),...,t(z+m)},1⩽z⩽L−m.
Then, the distances between any Tm(z) and Tm(p), and between any Tm+1(z) and Tm+1(p) were defined as:
D[Tm(z),Tm(p)]=max0⩽q⩽m−1(|t(z+q)−t(p+q)|),1⩽z,p⩽L−m+1 | (4) |
D[Tm+1(z),Tm+1(p)]=max0⩽q⩽m(|t(z+q)−t(p+q)|),1⩽z,p⩽L−m | (5) |
Subsequently, Cmj(ε) and Cm+1j(ε) were given by:
Cmz(ε)=umz(ε)L−m+1,z=1,⋯,L−m+1 | (6) |
Cm+1z(ε)=um+1z(ε)L−m,z=1,⋯,L−m | (7) |
where umz(ε) denotes the number that the distance between any vector Tm(z) and vector Tm(p) is within ε, and similarly um+1z(ε) is the number that the distance between any vector Tm+1(z) and vector Tm+1(p) is within ε. ε denotes the tolerance for accepting matches [30].
θm(ε)=L−m+1∑z=1logCmz(ε)L−m+1 | (8) |
θm+1(ε)=L−m∑z=1logCm+1z(ε)L−m | (9) |
where θm(ε) is the average probability that two any vectors coincide for m+1 points, and θm+1(ε) is the average probability that two any vectors coincide for m+1 points.
Ultimately, ApEn was calculated as follows:
ApEn(ε,m)=θm(ε)−θm+1(ε) | (10) |
In this work, the value of ε was set to 0.1 and that of m was set to 2 [30,37].
MSE builds on SampEn algorithm by integrating a coarse-graining procedure and provides insight into the point-to-point fluctuation across multiple time scales [42]. The kth coarse-grained time series for each time scale of τ, y(τ)={y(τ)1,y(τ)2,⋯,y(τ)p}, was defined as [42]:
y(τ)k=1τkτ−1∑l=(k−1)τ+1t(l),1⩽k⩽Lτ | (11) |
where p=Lτ, τ is the time scale of interest, L is the length of the time series, and t(k) is a data point in the time series, i.e., {t(l)}=t(1),t(2),...,t(L), of each ECG and VCG lead.
SampEn algorithm was employed to obtain an entropy value at each time scale.
MSE(τ,m,ε)=SampEn(y(τ),m,ε),1⩽τ⩽M | (12) |
where M is the maximum time scale; the value of ε was set to 0.1 and that of m set to 2 [30,37].
A major concern when employing MSE algorithm is to guarantee that the data length of the coarse-grained time series is long enough at the maximum time scale for the reason that too short time series at any time scale associates with inconsistent probabilities from SampEn. More than 200 data points per time scale has been recommended to elicit consistent SampEn values [43]. Since the data length of time series is between 900 and 1200, the maximum time scale was set to 3. The values of τ were set to 1, 2, 3 [30,43].
Once all parameters and MSE have been determined, the area under the MSE vs. time scale curve, entitled as CI [42] of ECGs' and VCGs' ST-T segments were calculated as follows:
CI=M∑τ=1MSE(τ) | (13) |
where MSE(τ) is the MSE value at time scale factor of τ.
Eight common machine learning models [29] i.e., support vector machine (SVM), multilayer perceptron (MLP), gradient boosting [44], bagging ensemble (Bagging), random forest, ada boost, decision tree, and K-nearest neighbors (KNN) were conducted to distinguish between healthy controls and patients with CMD in this work. All machine learning models were implemented in Python 3.7 employing open-source libraries: Scikit-learn (0.20.1, http://scikit-learn.org/stable).
The entropy subsets selected by utilizing the SBS algorithm were fed into each framework to identify the subjects (i.e., CMD patients vs. healthy controls). Regard to the model design, SampEn-based model denoted each framework fed with VCGs' and ECGs' SampEn only. Similarly, ApEn-based and CI-based models presented respectively the frameworks utilizing ApEn and CI only.
Feature selection is widely implemented to determine the optimal feature subset from a large initial set of features thus improving generalization of the machine learning model and reducing the computational demand. To achieve the most effective entropy subset, SBS algorithm [45,46] has been conducted, as presented in Figure 6.
First, a collection of candidate entropy subset for each model was created on the training-validation dataset, as illustrated in Figure 6A. For each algorithm, a baseline model was constructed using each entropy feature separately, i.e., 15 SampEn, 15 ApEn, or 15 CI calculated from ST-T segments of 3-lead VCGs and 12-lead ECGs. The iteration variable n was initially set as 15. Each subset with n-1 entropies was trained and evaluated by employing a five-fold cross-validation to select the one with the highest average F1 score, which takes into account precision and recall, and is not sensitive to the class-imbalance [47] into next iteration where subsets with n-2 entropies derived from the selected n-1 element subset were evaluated and compared similarly. The iteration continued until n = 1. Finally, 15 candidate subsets with different numbers of entropies (i.e., 1, 2, …, 15) were selected by the iterative SBS algorithm.
Second, the optimal entropy subset, which had maximal five-fold cross-validation average F1 score, was identified from the SBS-generated collection of the candidate entropy subsets achieved above, as illustrated in Figure 6B.
Finally, the optimal entropy subset was separately determined from the total 15 SampEn for each SampEn-based model. Similarly, optimal entropy subsets for ApEn-based and CI-based models were determined.
The utility of the constructed models was evaluated by accuracy, specificity, sensitivity, and F1 score [37]. Additionally, the geometric mean (G-mean) and an area under the curve for precision/recall (PR-AUC) were considered as evaluation metrics. The geometric mean (G-mean) is the geometric mean of sensitivity and specificity [48]. PR-AUC is a metric commonly used in highly imbalanced datasets [49].
Both intra-patient and inter-patient schemes were implemented to verify the effectiveness of our proposed modes. Under the intra-patient scheme, positive and negative ECGs were randomly split in 80% for training and validation, and 20% for testing at the same time. Regarding the inter-patient scheme, patients were randomly separated in the proportion of 4:1 for training-validation and testing, and the corresponding ECGs formed the training-validation and testing datasets. Under both scheme, a five-fold cross-validation approach was adopted in the training-validation dataset to optimize model parameters and check evaluation metrics (i.e., sensitivity, accuracy, specificity, F1 score, G-mean, and PR-AUC) on the training-validation dataset for each model at the very first place. Then, each trained model was tested on the testing dataset to check whether the selected model always performed best under each scheme for every scenario.
In this work, the signal preprocessing and feature extraction were developed utilizing MATLAB (R2021; The MathWorks Inc. Natick, USA). All machine learning models were established, evaluated, and tested in Python 3.7 as well as feature selection.
The evaluation metrics of SampEn-based, ApEn-based, and CI-based models on the validation dataset are illustrated in first, second, and third columns of Figure 7 respectively. It can be observed from the first column that SVM model outperforms other ApEn-based models in response to the major evaluation metrics. Therefore, SVM was determined as the candidate one for SampEn-based model. Similarly, MLP and SVM models were selected as the candidates for SampEn-based and CI-based models respectively. As listed in Table 2, the testing evaluation metrics of ApEn-based SVM model are higher than those of other ApEn-based models. Similarly, SampEn-based MLP and CI-based SVM models provide the highest the testing evaluation metrics across all SampEn-based and CI-based ones respectively.
Models | Accuracy | Specificity | Sensitivity | F1 score | PR-AUC | G-mean |
ApEn-based SVM | 0.898 | 0.925 | 0.842 | 0.842 | 0.868 | 0.883 |
ApEn-based MLP | 0.881 | 0.925 | 0.789 | 0.811 | 0.845 | 0.855 |
ApEn-based Gradient Boost | 0.729 | 0.750 | 0.684 | 0.619 | 0.676 | 0.716 |
ApEn-based Bagging | 0.881 | 0.950 | 0.737 | 0.800 | 0.848 | 0.837 |
ApEn-based Random Forest | 0.847 | 0.950 | 0.632 | 0.727 | 0.804 | 0.775 |
ApEn-based Ada Boost | 0.814 | 0.900 | 0.632 | 0.686 | 0.750 | 0.754 |
ApEn-based Decision Tree | 0.847 | 0.900 | 0.737 | 0.757 | 0.800 | 0.814 |
ApEn-based KNN | 0.831 | 0.925 | 0.632 | 0.706 | 0.775 | 0.764 |
SampEn-based SVM | 0.831 | 0.825 | 0.842 | 0.762 | 0.794 | 0.833 |
SampEn-based MLP | 0.881 | 0.925 | 0.789 | 0.811 | 0.845 | 0.855 |
SampEn-based Gradient Boost | 0.814 | 0.850 | 0.737 | 0.718 | 0.761 | 0.791 |
SampEn-based Bagging | 0.847 | 0.900 | 0.737 | 0.757 | 0.800 | 0.814 |
SampEn-based Random Forest | 0.814 | 0.875 | 0.684 | 0.703 | 0.754 | 0.774 |
SampEn-based Ada Boost | 0.847 | 0.900 | 0.737 | 0.757 | 0.800 | 0.814 |
SampEn-based Decision Tree | 0.746 | 0.750 | 0.737 | 0.651 | 0.702 | 0.743 |
SampEn-based KNN | 0.712 | 0.825 | 0.476 | 0.514 | 0.603 | 0.625 |
CI-based SVM | 0.847 | 0.875 | 0.789 | 0.769 | 0.804 | 0.831 |
CI-based MLP | 0.831 | 0.875 | 0.737 | 0.737 | 0.779 | 0.803 |
CI-based Gradient Boost | 0.746 | 0.775 | 0.684 | 0.634 | 0.688 | 0.728 |
CI-based Bagging | 0.780 | 0.825 | 0.684 | 0.667 | 0.718 | 0.751 |
CI-based Random Forest | 0.814 | 0.875 | 0.684 | 0.703 | 0.754 | 0.773 |
CI-based Ada Boost | 0.746 | 0.800 | 0.632 | 0.615 | 0.675 | 0.711 |
CI-based Decision Tree | 0.746 | 0.750 | 0.737 | 0.651 | 0.702 | 0.743 |
CI-based KNN | 0.712 | 0.825 | 0.474 | 0.514 | 0.603 | 0.625 |
Note: Best performance for ApEn-based, SampEn-based, and CI-based models is highlighted in bold. |
Subsequently, the comparisons among the three candidate models (i.e., ApEn-based SVM, SampEn-based MLP, and CI-based SVM models) show that ApEn-based SVM model affords the highest evaluation metrics on the validation dataset, as shown in Figure 7. Furthermore, the classification performances of the three candidate models on the testing dataset present that ApEn-based SVM model is superior to the other two models (i.e., SampEn-based MLP and CI-based SVM models) with all evaluation metrics higher than 0.8, as listed in Table 2. Thus, ApEn-based SVM model was validated as the optimal one in detecting CMD under the intra-patient scheme.
Figure 8 illustrates the comparisons of evaluation metrics among ApEn-based, SampEn-based, and CI-based models on the validation dataset for detecting CMD. From the first column, SVM model yields the highest classification performance across all ApEn-based models. Therefore, it was verified as the candidate one for ApEn-based model. Similarly, Bagging and SVM models were determined as the candidates for ApEn-based and CI-based models respectively. Regard to the classification performances on the testing dataset, ApEn-based SVM model outperforms other ApEn-based ones, as listed in Table 3. Similarly, SampEn-based Bagging and CI-based SVM models perform best across all SampEn-based and CI-based ones respectively.
Models | Accuracy | Specificity | Sensitivity | F1 score | PR-AUC | G-mean |
ApEn-based SVM | 0.881 | 0.925 | 0.789 | 0.811 | 0.845 | 0.855 |
ApEn-based MLP | 0.814 | 0.775 | 0.895 | 0.756 | 0.791 | 0.833 |
ApEn-based Gradient Boost | 0.797 | 0.850 | 0.684 | 0.684 | 0.735 | 0.763 |
ApEn-based Bagging | 0.864 | 0.925 | 0.737 | 0.778 | 0.823 | 0.826 |
ApEn-based Random Forest | 0.847 | 0.925 | 0.684 | 0.743 | 0.799 | 0.796 |
ApEn-based Ada Boost | 0.847 | 0.925 | 0.684 | 0.743 | 0.799 | 0.796 |
ApEn-based Decision Tree | 0.847 | 0.925 | 0.684 | 0.743 | 0.799 | 0.796 |
ApEn-based KNN | 0.831 | 0.925 | 0.632 | 0.706 | 0.775 | 0.764 |
SampEn-based SVM | 0.847 | 0.900 | 0.737 | 0.757 | 0.800 | 0.814 |
SampEn-based MLP | 0.814 | 0.850 | 0.737 | 0.718 | 0.761 | 0.791 |
SampEn-based Gradient Boost | 0.864 | 0.900 | 0.789 | 0.789 | 0.823 | 0.843 |
SampEn-based Bagging | 0.864 | 0.925 | 0.737 | 0.778 | 0.823 | 0.826 |
SampEn-based Random Forest | 0.864 | 0.925 | 0.737 | 0.778 | 0.823 | 0.826 |
SampEn-based Ada Boost | 0.831 | 0.925 | 0.632 | 0.706 | 0.775 | 0.764 |
SampEn-based Decision Tree | 0.797 | 0.850 | 0.684 | 0.684 | 0.735 | 0.763 |
SampEn-based KNN | 0.814 | 0.900 | 0.632 | 0.686 | 0.750 | 0.754 |
CI-based SVM | 0.831 | 0.875 | 0.737 | 0.737 | 0.779 | 0.803 |
CI-based MLP | 0.729 | 0.750 | 0.684 | 0.619 | 0.676 | 0.716 |
CI-based Gradient Boost | 0.763 | 0.825 | 0.632 | 0.632 | 0.691 | 0.722 |
CI-based Bagging | 0.763 | 0.825 | 0.632 | 0.632 | 0.691 | 0.722 |
CI-based Random Forest | 0.729 | 0.825 | 0.526 | 0.556 | 0.633 | 0.659 |
CI-based Ada Boost | 0.695 | 0.750 | 0.579 | 0.550 | 0.619 | 0.659 |
CI-based Decision Tree | 0.678 | 0.675 | 0.684 | 0.578 | 0.643 | 0.680 |
CI-based KNN | 0.780 | 0.950 | 0.421 | 0.552 | 0.704 | 0.632 |
Note: Best performance for ApEn-based, SampEn-based, and CI-based models is highlighted in bold. |
Then, the classification performances of SampEn-based Bagging, ApEn-based SVM, and CI-based SVM models were compared to select the optimal one. It can be observed from Figure 8 that ApEn-based SVM model has superiority to the other two models except the sensitivity for SampEn-based Bagging model. Moreover, the comparisons of the three candidate models on the testing dataset demonstrate that ApEn-based SVM model has all testing evaluation metrics higher than SampEn-based Bagging and CI-based SVM models, as listed in Table 3. Hence, ApEn-based SVM model was verified as the optimal one for CMD detection under the inter-patient scheme.
The ApEn-based SVM models were verified to be the optimal ones for CMD detection, with all and major testing evaluation metrics over 0.8 under the intra-patient and inter-patient schemes respectively, indicating the feasibility of entropy-based early detection of CMD using machine learning algorithm.
Obstructive CAD and CMD are recognized as two major causes of myocardial ischemia. Since obstructive CAD had been considered as the leading cause of myocardial ischemia, previous studies of ECG-based machine learning algorithms mainly focused on obstructive CAD detection [29,50], with a lack of in-depth investigation in patients with CMD. An advantage of the ECG-based method is its interpretability. Some mathematical models disclosed the pathology of CMD from cell levels. Some critical electrophysiological phenomena and parameters, e.g., T wave alternans, can be directly reflected on ECG and VCG, providing the possibility of fine-grained classification and stratification of CMD [51,52]. To detect CMD non-invasively, electrocardiographic indictors., i.e., SDNN < 100 ms [24], ischemic ST-T segment changes [22,25,26,27], and the prolongation of the heart rate-corrected QT interval [27,28] have been investigated. Specially, SARA et al. combined three features, i.e., T-wave area, T Peak-T end, and Y-center of gravity as inputs of a linear discriminant analysis and recognized the existence presence of an abnormal coronary flow reserve (CFR) with the highest accuracy of 66.5 ± 0.3% and 74 ± 2% for females and males [22]. Since CFR assesses the entire coronary bed including both epicardial and microvascular districts, the authors utilized an abnormal CFR and DS < 50% as the standard for selecting CMD patients to exclude the influence of obstructive CAD. In contrast, IMR used in our work is not influenced by epicardial artery stenosis and can accurately reflect the microvascular flow resistance [18,21].
As far as we known, this is the first machine learning model employing entropy features of ECG and VCG to detect CMD based on reliable clinical diagnosis derived from patient specific FFR and IMR values.
CMD is believed to result from inadequate relaxation of vascular smooth muscle, which mediates myocardial ischemia [1]. During myocardial ischemia, the decrease of conduction velocity and the duration of action potential lead to the heterogeneous repolarization process [53]. Further, ischemia-induced repolarization dispersion causes ischemic changes in ECG and VCG signals. Regarding ECG, the variations in ST segment include ST-segment depression or elevation, and those in T wave include high-tip T wave, biphasic T wave or inverted T wave [51,52], as illustrated in Figure 1. As for VCG, the changes exist in the QRS loop, the spatial orientations and magnitude of ST vectors [51,54], the T-loop morphology, and the T-vector angle [55]. The beat-to-beat variations in T-loop roundness present intrinsic measures of beat-to-beat repolarization ability [55]. Therefore, the variations in ST-T segments of VCGs and ECGs can be considered as indicators of myocardial ischemia [37,51,55].
The ischemic variations have been demonstrated to afford detectable morphology variability in beat-to-beat ST-T segments, while the morphology shows a consistent pattern in normal subjects [56]. CMD can lead to changes in ST segment or/and T wave [22]. The complexity resulting from the morphology variations can be measured by entropy.
For the first time, we observed that CMD-induced complexity changes of beat-to-beat ST-T segments can be reliably detected by SampEn, ApEn, and MSE-derived CI (Tables 2 and 3), which provided reference for other ECG-based CMD detection algorithms. Specially, ApEn outperforms SampEn and CI in detecting CMD under both intra-patient and inter-patient schemes. ApEn values extracted from pathologic time series are different from those obtained from free running physiologic systems under healthy condition [57]. In this work, ApEn could be used to effectively measure the changed of the complexity induced by CMD.
An SVM model has been widely applied in the detection of myocardial ischemia location and multiclass myocardial infraction classification [50]. The other seven models also have been applied for CAD detection and yield excellent classification performance [29].
We evaluated and tested these common machine learning models to determine the optimal one for CMD detection. Our results present that an SVM model yields the best classification performance across all models under both schemes. Moreover, compared with MLP, gradient boosting, Bagging, and Ada boost models, an SVM model does not require extensive computational resources to determine the classification results. Therefore, it is more accomplishable on wearable devices, given that their computational resources are restricted.
We observed a difference between entropy features in the performance of SVM algorithm in detecting CMD. Under the intra-patient scheme, ApEn-based SVM model employing (AI, AII, AavR, AavL, AavF, AV4, AV5, AV6, AVx, AVz) was demonstrated to surpass the other models. Under the inter-patient scheme, ApEn-based SVM model utilizing (AI, AII, AIII, AV4, AV5, AV6, AVx, AVy) provided the best performance across all models. The patient-specific algorithm optimization deserves further investigation.
The results in this work have demonstrated that SampEn, ApEn, and CI calculated from ST-T segments of ECGs and VCGs could be useful features to recognize a patient with CMD for the first time. Furthermore, our proposed models afford the possibility of noninvasive and cost-effective detection of CMD. It could be easily deployed on conventional ECG acquisition equipment, given that VCG can be calculated from 12-lead ECG by employing a Kors transformation matrix [39]. Additionally, it is based on the 10 s ECG which has been widely accepted as the first-line diagnostic technique in assessing patients with suspected myocardial ischemia due to its non-invasiveness, non-radiation, and low cost [58]. The data length of the time series consisting of beat-to-beat ST-T segments in each lead is between 900 and 1200 and longer than 200 which is suggested for calculating ApEn and SampEn [43]. The minimum data length is more than 300 with the maximal time scale factor of τ = 3 regarding MSE. The commonly used 10 s ECG is available for calculating reliably the three entropy features. Our algorithm meets the clinical needs with no extra workload for operators, given that our algorithms are fully automatic. Hence, our results open a new pathway towards non-invasive, low-cost, and operator-friendly detection of CMD in various application scenarios using wearable ECG sensors. Therefore, the proposed method may provide an approach for large-scale screening of CMD in populations, especially in low-resource areas.
However, some limitations exist in our work. First, the sample size is small, especially the positive cases. The sample size is limited by the number of patients diagnosed with CMD who underwent full examination. The scarce of CMD patients' data is a common limitation of existing studies (e.g., 41 in [24]; 20, in [59]). Especially, the in vivo measurement of IMR is performed invasively by using an intravascular guide wire with the injection of saline into coronary artery multiple times and is expensive. Therefore, it is not widely used in clinical practice and only performed for the patients with obvious symptoms of CMD, which limited the data size to develop highly reliable algorithms that suit different cohorts. Secondly, the positive (i.e., CMD patients) and negative (i.e., healthy controls) samples were collected from different cohorts. Hence, the difference in physiological characteristics may influence the classification performance. Finally, only ECG-based features were selected as inputs, whereas clinical characteristic and other medical images were not integrated in our algorithms.
In future studies, multicenter large-scale studies can extend the positive sample size and further verify our results, optimize the algorithm, and improve the classification performance by considering the difference in physiological features among different cohorts. Based on large datasets, the combination between ECG-based features and clinic-characteristics could be considered to boost the improvement in the classification performance. The fine-grained classification of CMD based on ECG-VCG features also deserves further exploration.
In conclusion, SampEn, ApEn, and CI extracted from the beat-to-beat ST-T segment of ECGs and VCGs could be useful entropy features to recognize electrocardiographic signatures of CMD under both intra-patient and inter-patient schemes. Our proposed models may provide the possibility of an ECG-based tool for non-invasive detection of CMD.
The authors would like to appreciate all participants for data collection and providing constructive comments. This word was funded by the Natural Science Foundation of Ningxia Province (No. 2022AAC03242), the North Minzu University Scientific Research Projects (No. 2021JCYJ10), the Natural Science Foundation of China (NSFC) (No. 62171408), the Major Scientific Project of Zhejiang Lab (No. 2020ND8AD01), the Key Research and Development Program of Zhejiang Province (No. 2020C03016), Ningxia First-Class Discipline and Scientific Research Projects (Electronic Science and Technology) (No. NXYLXK2017A07), Innovation Team of Lidar Atmosphere Remote Sensing of Ningxia Province, the high level talent selection and training plan of North Minzu University, and Plan for Leading Talents of the State Ethnic Affairs Commission of the People's Republic of China.
The authors declare there is no conflict of interest.
[1] |
Gupta A, Madhavan MV, Sehgal K, et al. (2020) Extrapulmonary manifestations of COVID-19. Nat Med 26: 1017-1032. doi: 10.1038/s41591-020-0968-3
![]() |
[2] |
Ramos-Casals M, Brito-Zerón P, Mariette X (2021) Systemic and organ-specific immune-related manifestations of COVID-19. Nat Rev Rheumatol 17: 315-332. doi: 10.1038/s41584-021-00608-z
![]() |
[3] |
Akhmerov A, Marbán E (2020) COVID-19 and the Heart. Circ Res 126: 1443-1455. doi: 10.1161/CIRCRESAHA.120.317055
![]() |
[4] |
Guo T, Fan Y, Chen M, et al. (2020) Cardiovascular Implications of Fatal Outcomes of Patients With Coronavirus Disease 2019 (COVID-19). JAMA Cardiol 5: 811-818. doi: 10.1001/jamacardio.2020.1017
![]() |
[5] |
Hilscher MB, Shah VH (2020) Neutrophil Extracellular Traps and Liver Disease. Semin Liver Dis 40: 171-179. doi: 10.1055/s-0039-3399562
![]() |
[6] |
El Kazzi M, Rayner BS, Chami B, et al. (2020) Neutrophil-Mediated Cardiac Damage After Acute Myocardial Infarction: Significance of Defining a New Target Cell Type for Developing Cardioprotective Drugs. Antioxid Redox Signal 33: 689-712. doi: 10.1089/ars.2019.7928
![]() |
[7] |
Yaqinuddin A, Kashir J (2020) Innate immunity in COVID-19 patients mediated by NKG2A receptors, and potential treatment using Monalizumab, Cholroquine, and antiviral agents. Med Hypotheses 140: 109777. doi: 10.1016/j.mehy.2020.109777
![]() |
[8] |
Yaqinuddin A, Kashir J (2020) Novel therapeutic targets for SARS-CoV-2-induced acute lung injury: Targeting a potential IL-1β/neutrophil extracellular traps feedback loop. Med Hypotheses 143: 109906. doi: 10.1016/j.mehy.2020.109906
![]() |
[9] |
Yaqinuddin A, Kvietys P, Kashir J (2020) COVID-19: Role of neutrophil extracellular traps in acute lung injury. Respir Investig 58: 419-420. doi: 10.1016/j.resinv.2020.06.001
![]() |
[10] |
Lee C, Choi WJ (2021) Overview of COVID-19 inflammatory pathogenesis from the therapeutic perspective. Arch Pharm Res 44: 99-116. doi: 10.1007/s12272-020-01301-7
![]() |
[11] |
Liao M, Liu Y, Yuan J, et al. (2020) Single-cell landscape of bronchoalveolar immune cells in patients with COVID-19. Nat Med 26: 842-844. doi: 10.1038/s41591-020-0901-9
![]() |
[12] |
Kvietys PR, Fakhoury HMA, Kadan S, et al. (2021) COVID-19: Lung-Centric Immunothrombosis. Front Cell Infect Microbiol 11: 679878. doi: 10.3389/fcimb.2021.679878
![]() |
[13] | Yaqinuddin A, Kashir J (2020) The central role of neutrophil extracellular traps in SARS-CoV-2-induced thrombogenesis and vasculitis. J Afr J Respir Med 15: 5. |
[14] |
Ackermann M, Verleden SE, Kuehnel M, et al. (2020) Pulmonary Vascular Endothelialitis, Thrombosis, and Angiogenesis in Covid-19. N Engl J Med 383: 120-128. doi: 10.1056/NEJMoa2015432
![]() |
[15] |
Vasquez-Bonilla WO, Orozco R, Argueta V, et al. (2020) A review of the main histopathological findings in coronavirus disease 2019. Hum Pathol 105: 74-83. doi: 10.1016/j.humpath.2020.07.023
![]() |
[16] |
Rapkiewicz AV, Mai X, Carsons SE, et al. (2020) Megakaryocytes and platelet-fibrin thrombi characterize multi-organ thrombosis at autopsy in COVID-19: A case series. EClinicalMedicine 24: 100434. doi: 10.1016/j.eclinm.2020.100434
![]() |
[17] |
Agrati C, Sacchi A, Bordoni V, et al. (2020) Expansion of myeloid-derived suppressor cells in patients with severe coronavirus disease (COVID-19). Cell Death Differ 27: 3196-3207. doi: 10.1038/s41418-020-0572-6
![]() |
[18] |
Carissimo G, Xu W, Kwok I, et al. (2020) Whole blood immunophenotyping uncovers immature neutrophil-to-VD2 T-cell ratio as an early marker for severe COVID-19. Nat Commun 11: 5243. doi: 10.1038/s41467-020-19080-6
![]() |
[19] |
Mohamed Khosroshahi L, Rezaei N (2021) Dysregulation of the immune response in coronavirus disease 2019. Cell Biol Int 45: 702-707. doi: 10.1002/cbin.11517
![]() |
[20] | Luo XH, Zhu Y, Mao J, et al. (2021) T cell immunobiology and cytokine storm of COVID-19. Scand J Immunol 93: e12989. |
[21] |
Wilk AJ, Rustagi A, Zhao NQ, et al. (2020) A single-cell atlas of the peripheral immune response in patients with severe COVID-19. Nat Med 26: 1070-1076. doi: 10.1038/s41591-020-0944-y
![]() |
[22] |
Ackermann M, Anders HJ, Bilyy R, et al. (2021) Patients with COVID-19: in the dark-NETs of neutrophils. Cell Death Differ 28: 3125-3139. doi: 10.1038/s41418-021-00805-z
![]() |
[23] |
Gollomp K, Kim M, Johnston I, et al. (2018) Neutrophil accumulation and NET release contribute to thrombosis in HIT. JCI Insight 3: e99445. doi: 10.1172/jci.insight.99445
![]() |
[24] |
Arcanjo A, Logullo J, Menezes CCB, et al. (2020) The emerging role of neutrophil extracellular traps in severe acute respiratory syndrome coronavirus 2 (COVID-19). Sci Rep 10: 19630. doi: 10.1038/s41598-020-76781-0
![]() |
[25] |
Ouwendijk WJD, Raadsen MP, van Kampen JJA, et al. (2021) High Levels of Neutrophil Extracellular Traps Persist in the Lower Respiratory Tract of Critically Ill Patients With Coronavirus Disease 2019. J Infect Dis 223: 1512-1521. doi: 10.1093/infdis/jiab050
![]() |
[26] |
Teluguakula N (2021) Neutrophils Set Extracellular Traps to Injure Lungs in Coronavirus Disease 2019. J Infect Dis 223: 1503-1505. doi: 10.1093/infdis/jiab053
![]() |
[27] |
Middleton EA, He XY, Denorme F, et al. (2020) Neutrophil extracellular traps contribute to immunothrombosis in COVID-19 acute respiratory distress syndrome. Blood 136: 1169-1179. doi: 10.1182/blood.2020007008
![]() |
[28] |
Schurink B, Roos E, Radonic T, et al. (2020) Viral presence and immunopathology in patients with lethal COVID-19: a prospective autopsy cohort study. Lancet Microbe 1: e290-e299. doi: 10.1016/S2666-5247(20)30144-0
![]() |
[29] |
Veras FP, Pontelli MC, Silva CM, et al. (2020) SARS-CoV-2-triggered neutrophil extracellular traps mediate COVID-19 pathology. J Exp Med 217: e20201129. doi: 10.1084/jem.20201129
![]() |
[30] |
Youn YJ, Lee YB, Kim SH, et al. (2021) Nucleocapsid and Spike Proteins of SARS-CoV-2 Drive Neutrophil Extracellular Trap Formation. Immune Netw 21: e16. doi: 10.4110/in.2021.21.e16
![]() |
[31] |
Iliadi V, Konstantinidou I, Aftzoglou K, et al. (2021) The Emerging Role of Neutrophils in the Pathogenesis of Thrombosis in COVID-19. Int J Mol Sci 22: 5368. doi: 10.3390/ijms22105368
![]() |
[32] |
Janiuk K, Jabłońska E, Garley M (2021) Significance of NETs Formation in COVID-19. Cells 10: 151. doi: 10.3390/cells10010151
![]() |
[33] |
Leshner M, Wang S, Lewis C, et al. (2012) PAD4 mediated histone hypercitrullination induces heterochromatin decondensation and chromatin unfolding to form neutrophil extracellular trap-like structures. Front Immunol 3: 307. doi: 10.3389/fimmu.2012.00307
![]() |
[34] |
Yipp BG, Petri B, Salina D, et al. (2012) Infection-induced NETosis is a dynamic process involving neutrophil multitasking in vivo. Nat Med 18: 1386-1393. doi: 10.1038/nm.2847
![]() |
[35] |
Leppkes M, Maueröder C, Hirth S, et al. (2016) Externalized decondensed neutrophil chromatin occludes pancreatic ducts and drives pancreatitis. Nat Commun 7: 10973. doi: 10.1038/ncomms10973
![]() |
[36] |
Giustino G, Croft LB, Stefanini GG, et al. (2020) Characterization of Myocardial Injury in Patients With COVID-19. J Am Coll Cardiol 76: 2043-2055. doi: 10.1016/j.jacc.2020.08.069
![]() |
[37] |
Fuchs TA, Brill A, Duerschmied D, et al. (2010) Extracellular DNA traps promote thrombosis. Proc Natl Acad Sci U S A 107: 15880-15885. doi: 10.1073/pnas.1005743107
![]() |
[38] |
Semeraro F, Ammollo CT, Morrissey JH, et al. (2011) Extracellular histones promote thrombin generation through platelet-dependent mechanisms: involvement of platelet TLR2 and TLR4. Blood 118: 1952-1961. doi: 10.1182/blood-2011-03-343061
![]() |
[39] |
Martinod K, Wagner DD (2014) Thrombosis: tangled up in NETs. Blood 123: 2768-2776. doi: 10.1182/blood-2013-10-463646
![]() |
[40] |
Fuchs TA, Bhandari AA, Wagner DD (2011) Histones induce rapid and profound thrombocytopenia in mice. Blood 118: 3708-3714. doi: 10.1182/blood-2011-01-332676
![]() |
[41] |
Xu J, Zhang X, Pelayo R, et al. (2009) Extracellular histones are major mediators of death in sepsis. Nat Med 15: 1318-1321. doi: 10.1038/nm.2053
![]() |
[42] |
Etulain J, Martinod K, Wong SL, et al. (2015) P-selectin promotes neutrophil extracellular trap formation in mice. Blood 126: 242-246. doi: 10.1182/blood-2015-01-624023
![]() |
[43] |
Maugeri N, Campana L, Gavina M, et al. (2014) Activated platelets present high mobility group box 1 to neutrophils, inducing autophagy and promoting the extrusion of neutrophil extracellular traps. J Thromb Haemost 12: 2074-2088. doi: 10.1111/jth.12710
![]() |
[44] |
Thålin C, Hisada Y, Lundström S, et al. (2019) Neutrophil Extracellular Traps: Villains and Targets in Arterial, Venous, and Cancer-Associated Thrombosis. Arterioscler Thromb Vasc Biol 39: 1724-1738. doi: 10.1161/ATVBAHA.119.312463
![]() |
[45] |
Boeltz S, Amini P, Anders HJ, et al. (2019) To NET or not to NET:current opinions and state of the science regarding the formation of neutrophil extracellular traps. Cell Death Differ 26: 395-408. doi: 10.1038/s41418-018-0261-x
![]() |
[46] |
Klopf J, Brostjan C, Eilenberg W, et al. (2021) Neutrophil Extracellular Traps and Their Implications in Cardiovascular and Inflammatory Disease. Int J Mol Sci 22: 559. doi: 10.3390/ijms22020559
![]() |
[47] |
Massberg S, Grahl L, von Bruehl ML, et al. (2010) Reciprocal coupling of coagulation and innate immunity via neutrophil serine proteases. Nat Med 16: 887-896. doi: 10.1038/nm.2184
![]() |
[48] |
Ammollo CT, Semeraro F, Xu J, et al. (2011) Extracellular histones increase plasma thrombin generation by impairing thrombomodulin-dependent protein C activation. J Thromb Haemost 9: 1795-1803. doi: 10.1111/j.1538-7836.2011.04422.x
![]() |
[49] |
Kang Y, Chen T, Mui D, et al. (2020) Cardiovascular manifestations and treatment considerations in COVID-19. Heart 106: 1132-1141. doi: 10.1136/heartjnl-2020-317056
![]() |
[50] |
Bearse M, Hung YP, Krauson AJ, et al. (2021) Factors associated with myocardial SARS-CoV-2 infection, myocarditis, and cardiac inflammation in patients with COVID-19. Mod Pathol 34: 1345-1357. doi: 10.1038/s41379-021-00790-1
![]() |
[51] |
Lindner D, Fitzek A, Bräuninger H, et al. (2020) Association of Cardiac Infection With SARS-CoV-2 in Confirmed COVID-19 Autopsy Cases. JAMA Cardiol 5: 1281-1285. doi: 10.1001/jamacardio.2020.3551
![]() |
[52] |
Van Linthout S, Miteva K, Tschöpe C (2014) Crosstalk between fibroblasts and inflammatory cells. Cardiovasc Res 102: 258-269. doi: 10.1093/cvr/cvu062
![]() |
[53] |
Lippi G, Plebani M (2020) Laboratory abnormalities in patients with COVID-2019 infection. Clin Chem Lab Med 58: 1131-1134. doi: 10.1515/cclm-2020-0198
![]() |
[54] | Blasco A, Coronado MJ, Hernández-Terciado F, et al. (2020) Assessment of Neutrophil Extracellular Traps in Coronary Thrombus of a Case Series of Patients With COVID-19 and Myocardial Infarction. JAMA Cardiol 6: 1-6. |
[55] |
Henry BM, Aggarwal G, Wong J, et al. (2020) Lactate dehydrogenase levels predict coronavirus disease 2019 (COVID-19) severity and mortality: A pooled analysis. Am J Emerg Med 38: 1722-1726. doi: 10.1016/j.ajem.2020.05.073
![]() |
[56] |
Henry BM, de Oliveira MHS, Benoit S, et al. (2020) Hematologic, biochemical and immune biomarker abnormalities associated with severe illness and mortality in coronavirus disease 2019 (COVID-19): a meta-analysis. Clin Chem Lab Med 58: 1021-1028. doi: 10.1515/cclm-2020-0369
![]() |
[57] |
Szarpak L, Ruetzler K, Safiejko K, et al. (2021) Lactate dehydrogenase level as a COVID-19 severity marker. Am J Emerg Med 45: 638-639. doi: 10.1016/j.ajem.2020.11.025
![]() |
[58] |
Bonow RO, Fonarow GC, O'Gara PT, et al. (2020) Association of Coronavirus Disease 2019 (COVID-19) With Myocardial Injury and Mortality. JAMA Cardiol 5: 751-753. doi: 10.1001/jamacardio.2020.1105
![]() |
[59] |
Muraro SP, De Souza GF, Gallo SW, et al. (2018) Respiratory Syncytial Virus induces the classical ROS-dependent NETosis through PAD-4 and necroptosis pathways activation. Sci Rep 8: 14166. doi: 10.1038/s41598-018-32576-y
![]() |
[60] |
López-Reyes A, Martinez-Armenta C, Espinosa-Velázquez R, et al. (2020) NLRP3 Inflammasome: The Stormy Link Between Obesity and COVID-19. Front Immunol 11: 570251. doi: 10.3389/fimmu.2020.570251
![]() |
[61] |
Yang Y, Wang H, Kouadir M, et al. (2019) Recent advances in the mechanisms of NLRP3 inflammasome activation and its inhibitors. Cell Death Dis 10: 128. doi: 10.1038/s41419-019-1413-8
![]() |
[62] |
Lara PC, Macías-Verde D, Burgos-Burgos J (2020) Age-induced NLRP3 Inflammasome Over-activation Increases Lethality of SARS-CoV-2 Pneumonia in Elderly Patients. Aging Dis 11: 756-762. doi: 10.14336/AD.2020.0601
![]() |
[63] |
Gedefaw L, Ullah S, Leung PHM, et al. (2021) Inflammasome Activation-Induced Hypercoagulopathy: Impact on Cardiovascular Dysfunction Triggered in COVID-19 Patients. Cells 10: 916. doi: 10.3390/cells10040916
![]() |
[64] |
Saeedi-Boroujeni A, Mahmoudian-Sani MR, Nashibi R, et al. (2021) Tranilast: a potential anti-Inflammatory and NLRP3 inflammasome inhibitor drug for COVID-19. Immunopharmacol Immunotoxicol 43: 247-258. doi: 10.1080/08923973.2021.1925293
![]() |
[65] |
Ng H, Havervall S, Rosell A, et al. (2021) Circulating Markers of Neutrophil Extracellular Traps Are of Prognostic Value in Patients With COVID-19. Arterioscler Thromb Vasc Biol 41: 988-994. doi: 10.1161/ATVBAHA.120.315267
![]() |
[66] |
Bonaventura A, Vecchié A, Abbate A, et al. (2020) Neutrophil Extracellular Traps and Cardiovascular Diseases: An Update. Cells 9: 231. doi: 10.3390/cells9010231
![]() |
[67] |
Distelmaier K, Winter MP, Dragschitz F, et al. (2014) Prognostic value of culprit site neutrophils in acute coronary syndrome. Eur J Clin Invest 44: 257-265. doi: 10.1111/eci.12228
![]() |
[68] |
Ge L, Zhou X, Ji WJ, et al. (2015) Neutrophil extracellular traps in ischemia-reperfusion injury-induced myocardial no-reflow: therapeutic potential of DNase-based reperfusion strategy. Am J Physiol Heart Circ Physiol 308: H500-509. doi: 10.1152/ajpheart.00381.2014
![]() |
[69] |
Huang H, Tohme S, Al-Khafaji AB, et al. (2015) Damage-associated molecular pattern-activated neutrophil extracellular trap exacerbates sterile inflammatory liver injury. Hepatology 62: 600-614. doi: 10.1002/hep.27841
![]() |
[70] |
Kawaguchi M, Takahashi M, Hata T, et al. (2011) Inflammasome activation of cardiac fibroblasts is essential for myocardial ischemia/reperfusion injury. Circulation 123: 594-604. doi: 10.1161/CIRCULATIONAHA.110.982777
![]() |
[71] |
Giannopoulos G, Vrachatis DA, Deftereos SG (2020) Myocardial Injury in COVID-19-Can We Successfully Target Inflammation? JAMA Cardiol 5: 1069-1070. doi: 10.1001/jamacardio.2020.2569
![]() |
[72] |
Liu J, Li J, Arnold K, et al. (2020) Using heparin molecules to manage COVID-2019. Res Pract Thromb Haemost 4: 518-523. doi: 10.1002/rth2.12353
![]() |
[73] |
Horby P, Lim WS, Emberson JR, et al. (2021) Dexamethasone in Hospitalized Patients with Covid-19. N Engl J Med 384: 693-704. doi: 10.1056/NEJMoa2021436
![]() |
[74] |
Boulware DR, Pullen MF, Bangdiwala AS, et al. (2020) A Randomized Trial of Hydroxychloroquine as Postexposure Prophylaxis for Covid-19. N Engl J Med 383: 517-525. doi: 10.1056/NEJMoa2016638
![]() |
[75] |
Sun Y, Chen C, Zhang X, et al. (2020) Heparin improves alveolarization and vascular development in hyperoxia-induced bronchopulmonary dysplasia by inhibiting neutrophil extracellular traps. Biochem Biophys Res Commun 522: 33-39. doi: 10.1016/j.bbrc.2019.11.041
![]() |
[76] |
Santocki M, Kolaczkowska E (2020) On Neutrophil Extracellular Trap (NET) Removal: What We Know Thus Far and Why So Little. Cells 9: 2079. doi: 10.3390/cells9092079
![]() |
[77] | Kashir J, Ambia AR, Shafqat A, et al. (2021) Scientific premise for the involvement of neutrophil extracellular traps (NETs) in vaccine-induced thrombotic thrombocytopenia (VITT). J Leukoc Biol . |
[78] |
Weber AG, Chau AS, Egeblad M, et al. (2020) Nebulized in-line endotracheal dornase alfa and albuterol administered to mechanically ventilated COVID-19 patients: a case series. Mol Med 26: 91. doi: 10.1186/s10020-020-00215-w
![]() |
[79] |
Laridan E, Denorme F, Desender L, et al. (2017) Neutrophil extracellular traps in ischemic stroke thrombi. Ann Neurol 82: 223-232. doi: 10.1002/ana.24993
![]() |
[80] |
Bikdeli B, Madhavan MV, Gupta A, et al. (2020) Pharmacological Agents Targeting Thromboinflammation in COVID-19: Review and Implications for Future Research. Thromb Haemost 120: 1004-1024. doi: 10.1055/s-0040-1713152
![]() |
[81] |
Simka M (2021) Is digital necrosis in COVID-19 caused by neutrophil extracellular traps: Potential therapeutic strategies. Med Hypotheses 156: 110684. doi: 10.1016/j.mehy.2021.110684
![]() |
[82] |
Gillot C, Favresse J, Mullier F, et al. (2021) NETosis and the Immune System in COVID-19: Mechanisms and Potential Treatments. Front Pharmacol 12: 708302. doi: 10.3389/fphar.2021.708302
![]() |
[83] |
Okubo K, Kamiya M, Urano Y, et al. (2016) Lactoferrin Suppresses Neutrophil Extracellular Traps Release in Inflammation. EBioMedicine 10: 204-215. doi: 10.1016/j.ebiom.2016.07.012
![]() |
[84] |
Liu X, Li Z, Liu S, et al. (2020) Potential therapeutic effects of dipyridamole in the severely ill patients with COVID-19. Acta Pharm Sin B 10: 1205-1215. doi: 10.1016/j.apsb.2020.04.008
![]() |
[85] |
Ali RA, Gandhi AA, Meng H, et al. (2019) Adenosine receptor agonism protects against NETosis and thrombosis in antiphospholipid syndrome. Nat Commun 10: 1916. doi: 10.1038/s41467-019-09801-x
![]() |
[86] |
Hazeldine J, Lord JM (2021) Neutrophils and COVID-19: Active Participants and Rational Therapeutic Targets. Front Immunol 12: 680134. doi: 10.3389/fimmu.2021.680134
![]() |
[87] |
Kyriazopoulou E, Poulakou G, Milionis H, et al. (2021) Early treatment of COVID-19 with anakinra guided by soluble urokinase plasminogen receptor plasma levels: a double-blind, randomized controlled phase 3 trial. Nat Med 27: 1752-1760. doi: 10.1038/s41591-021-01499-z
![]() |
[88] |
Barnes BJ, Adrover JM, Baxter-Stoltzfus A, et al. (2020) Targeting potential drivers of COVID-19: Neutrophil extracellular traps. J Exp Med 217: e20200652. doi: 10.1084/jem.20200652
![]() |
[89] |
Pal D, Goyal J, Sharma U, et al. (2021) Mesenchymal stem cells in SARS-CoV-2 infection: A hype or hope. Life Sci 284: 119901. doi: 10.1016/j.lfs.2021.119901
![]() |
[90] |
Klok FA, Kruip M, van der Meer NJM, et al. (2020) Incidence of thrombotic complications in critically ill ICU patients with COVID-19. Thromb Res 191: 145-147. doi: 10.1016/j.thromres.2020.04.013
![]() |
1. | Xiaoye Zhao, Yinglan Gong, Jucheng Zhang, Haipeng Liu, Tianhai Huang, Haicheng Wei, Ling Xia, Jiandong Mao, Prognostic significance of global electrical heterogeneity (GEH) parameters between coronary microvascular dysfunction (CMD) and obstructive coronary artery disease (OCAD): a retrospective cohort study, 2025, 100, 17468094, 106952, 10.1016/j.bspc.2024.106952 | |
2. | Xiaoye Zhao, Yinglan Gong, Jucheng Zhang, Haipeng Liu, Tianhai Huang, Jun Jiang, Ling Xia, Jiandong Mao, 2024, A Multiplayer Perceptron Algorithm Based on Global Electrical Heterogeneity and Cardiodynamicsgram for Non-Invasive Detection of Coronary Microvascular Dysfunction, 979-8-3503-5359-4, 504, 10.1109/EEI63073.2024.10696636 | |
3. | Visweswar Sirish Parupudi, Amit Kumar Panda, Rajesh Kumar Tripathy, A Smartphone-Enabled Deep Learning Approach for Myocardial Infarction Detection Using ECG Traces for IoT-Based Healthcare Applications, 2023, 7, 2475-1472, 1, 10.1109/LSENS.2023.3328593 | |
4. | Yun Tang, Xuan Ma, Zhixiang Dong, Xingrui Chen, Shujuan Yang, Xiuyu Chen, Kai Yang, Shihua Zhao, Cardiac Magnetic Resonance Visualization of the Myocardial Microstructure in Non-Ischemic Cardiomyopathies, 2024, 9, 2009-8618, 10.15212/CVIA.2024.0042 |
Characteristics | Value* |
Age, years | 67.29 ± 9.23 |
Female, n (%) | 26/70 |
Hypertension, n (%) | 32/70 |
Diabetes mellitus, n (%) | 22/70 |
Heart rate, bpm | 70.24 ± 12.87 |
Systolic blood pressure, mmHg | 130.82 ± 15.34 |
Diastolic blood Pressure, mmHg | 73.43 ± 10.45 |
Smoking history, n (%) | 12/70 |
Family history of CAD, n (%) | 12/70 |
*The categorical data are provided as numbers and percentages, and all of numerical variables are afforded in the form mean ± standard deviation for normally distributed data. |
Models | Accuracy | Specificity | Sensitivity | F1 score | PR-AUC | G-mean |
ApEn-based SVM | 0.898 | 0.925 | 0.842 | 0.842 | 0.868 | 0.883 |
ApEn-based MLP | 0.881 | 0.925 | 0.789 | 0.811 | 0.845 | 0.855 |
ApEn-based Gradient Boost | 0.729 | 0.750 | 0.684 | 0.619 | 0.676 | 0.716 |
ApEn-based Bagging | 0.881 | 0.950 | 0.737 | 0.800 | 0.848 | 0.837 |
ApEn-based Random Forest | 0.847 | 0.950 | 0.632 | 0.727 | 0.804 | 0.775 |
ApEn-based Ada Boost | 0.814 | 0.900 | 0.632 | 0.686 | 0.750 | 0.754 |
ApEn-based Decision Tree | 0.847 | 0.900 | 0.737 | 0.757 | 0.800 | 0.814 |
ApEn-based KNN | 0.831 | 0.925 | 0.632 | 0.706 | 0.775 | 0.764 |
SampEn-based SVM | 0.831 | 0.825 | 0.842 | 0.762 | 0.794 | 0.833 |
SampEn-based MLP | 0.881 | 0.925 | 0.789 | 0.811 | 0.845 | 0.855 |
SampEn-based Gradient Boost | 0.814 | 0.850 | 0.737 | 0.718 | 0.761 | 0.791 |
SampEn-based Bagging | 0.847 | 0.900 | 0.737 | 0.757 | 0.800 | 0.814 |
SampEn-based Random Forest | 0.814 | 0.875 | 0.684 | 0.703 | 0.754 | 0.774 |
SampEn-based Ada Boost | 0.847 | 0.900 | 0.737 | 0.757 | 0.800 | 0.814 |
SampEn-based Decision Tree | 0.746 | 0.750 | 0.737 | 0.651 | 0.702 | 0.743 |
SampEn-based KNN | 0.712 | 0.825 | 0.476 | 0.514 | 0.603 | 0.625 |
CI-based SVM | 0.847 | 0.875 | 0.789 | 0.769 | 0.804 | 0.831 |
CI-based MLP | 0.831 | 0.875 | 0.737 | 0.737 | 0.779 | 0.803 |
CI-based Gradient Boost | 0.746 | 0.775 | 0.684 | 0.634 | 0.688 | 0.728 |
CI-based Bagging | 0.780 | 0.825 | 0.684 | 0.667 | 0.718 | 0.751 |
CI-based Random Forest | 0.814 | 0.875 | 0.684 | 0.703 | 0.754 | 0.773 |
CI-based Ada Boost | 0.746 | 0.800 | 0.632 | 0.615 | 0.675 | 0.711 |
CI-based Decision Tree | 0.746 | 0.750 | 0.737 | 0.651 | 0.702 | 0.743 |
CI-based KNN | 0.712 | 0.825 | 0.474 | 0.514 | 0.603 | 0.625 |
Note: Best performance for ApEn-based, SampEn-based, and CI-based models is highlighted in bold. |
Models | Accuracy | Specificity | Sensitivity | F1 score | PR-AUC | G-mean |
ApEn-based SVM | 0.881 | 0.925 | 0.789 | 0.811 | 0.845 | 0.855 |
ApEn-based MLP | 0.814 | 0.775 | 0.895 | 0.756 | 0.791 | 0.833 |
ApEn-based Gradient Boost | 0.797 | 0.850 | 0.684 | 0.684 | 0.735 | 0.763 |
ApEn-based Bagging | 0.864 | 0.925 | 0.737 | 0.778 | 0.823 | 0.826 |
ApEn-based Random Forest | 0.847 | 0.925 | 0.684 | 0.743 | 0.799 | 0.796 |
ApEn-based Ada Boost | 0.847 | 0.925 | 0.684 | 0.743 | 0.799 | 0.796 |
ApEn-based Decision Tree | 0.847 | 0.925 | 0.684 | 0.743 | 0.799 | 0.796 |
ApEn-based KNN | 0.831 | 0.925 | 0.632 | 0.706 | 0.775 | 0.764 |
SampEn-based SVM | 0.847 | 0.900 | 0.737 | 0.757 | 0.800 | 0.814 |
SampEn-based MLP | 0.814 | 0.850 | 0.737 | 0.718 | 0.761 | 0.791 |
SampEn-based Gradient Boost | 0.864 | 0.900 | 0.789 | 0.789 | 0.823 | 0.843 |
SampEn-based Bagging | 0.864 | 0.925 | 0.737 | 0.778 | 0.823 | 0.826 |
SampEn-based Random Forest | 0.864 | 0.925 | 0.737 | 0.778 | 0.823 | 0.826 |
SampEn-based Ada Boost | 0.831 | 0.925 | 0.632 | 0.706 | 0.775 | 0.764 |
SampEn-based Decision Tree | 0.797 | 0.850 | 0.684 | 0.684 | 0.735 | 0.763 |
SampEn-based KNN | 0.814 | 0.900 | 0.632 | 0.686 | 0.750 | 0.754 |
CI-based SVM | 0.831 | 0.875 | 0.737 | 0.737 | 0.779 | 0.803 |
CI-based MLP | 0.729 | 0.750 | 0.684 | 0.619 | 0.676 | 0.716 |
CI-based Gradient Boost | 0.763 | 0.825 | 0.632 | 0.632 | 0.691 | 0.722 |
CI-based Bagging | 0.763 | 0.825 | 0.632 | 0.632 | 0.691 | 0.722 |
CI-based Random Forest | 0.729 | 0.825 | 0.526 | 0.556 | 0.633 | 0.659 |
CI-based Ada Boost | 0.695 | 0.750 | 0.579 | 0.550 | 0.619 | 0.659 |
CI-based Decision Tree | 0.678 | 0.675 | 0.684 | 0.578 | 0.643 | 0.680 |
CI-based KNN | 0.780 | 0.950 | 0.421 | 0.552 | 0.704 | 0.632 |
Note: Best performance for ApEn-based, SampEn-based, and CI-based models is highlighted in bold. |
Characteristics | Value* |
Age, years | 67.29 ± 9.23 |
Female, n (%) | 26/70 |
Hypertension, n (%) | 32/70 |
Diabetes mellitus, n (%) | 22/70 |
Heart rate, bpm | 70.24 ± 12.87 |
Systolic blood pressure, mmHg | 130.82 ± 15.34 |
Diastolic blood Pressure, mmHg | 73.43 ± 10.45 |
Smoking history, n (%) | 12/70 |
Family history of CAD, n (%) | 12/70 |
*The categorical data are provided as numbers and percentages, and all of numerical variables are afforded in the form mean ± standard deviation for normally distributed data. |
Models | Accuracy | Specificity | Sensitivity | F1 score | PR-AUC | G-mean |
ApEn-based SVM | 0.898 | 0.925 | 0.842 | 0.842 | 0.868 | 0.883 |
ApEn-based MLP | 0.881 | 0.925 | 0.789 | 0.811 | 0.845 | 0.855 |
ApEn-based Gradient Boost | 0.729 | 0.750 | 0.684 | 0.619 | 0.676 | 0.716 |
ApEn-based Bagging | 0.881 | 0.950 | 0.737 | 0.800 | 0.848 | 0.837 |
ApEn-based Random Forest | 0.847 | 0.950 | 0.632 | 0.727 | 0.804 | 0.775 |
ApEn-based Ada Boost | 0.814 | 0.900 | 0.632 | 0.686 | 0.750 | 0.754 |
ApEn-based Decision Tree | 0.847 | 0.900 | 0.737 | 0.757 | 0.800 | 0.814 |
ApEn-based KNN | 0.831 | 0.925 | 0.632 | 0.706 | 0.775 | 0.764 |
SampEn-based SVM | 0.831 | 0.825 | 0.842 | 0.762 | 0.794 | 0.833 |
SampEn-based MLP | 0.881 | 0.925 | 0.789 | 0.811 | 0.845 | 0.855 |
SampEn-based Gradient Boost | 0.814 | 0.850 | 0.737 | 0.718 | 0.761 | 0.791 |
SampEn-based Bagging | 0.847 | 0.900 | 0.737 | 0.757 | 0.800 | 0.814 |
SampEn-based Random Forest | 0.814 | 0.875 | 0.684 | 0.703 | 0.754 | 0.774 |
SampEn-based Ada Boost | 0.847 | 0.900 | 0.737 | 0.757 | 0.800 | 0.814 |
SampEn-based Decision Tree | 0.746 | 0.750 | 0.737 | 0.651 | 0.702 | 0.743 |
SampEn-based KNN | 0.712 | 0.825 | 0.476 | 0.514 | 0.603 | 0.625 |
CI-based SVM | 0.847 | 0.875 | 0.789 | 0.769 | 0.804 | 0.831 |
CI-based MLP | 0.831 | 0.875 | 0.737 | 0.737 | 0.779 | 0.803 |
CI-based Gradient Boost | 0.746 | 0.775 | 0.684 | 0.634 | 0.688 | 0.728 |
CI-based Bagging | 0.780 | 0.825 | 0.684 | 0.667 | 0.718 | 0.751 |
CI-based Random Forest | 0.814 | 0.875 | 0.684 | 0.703 | 0.754 | 0.773 |
CI-based Ada Boost | 0.746 | 0.800 | 0.632 | 0.615 | 0.675 | 0.711 |
CI-based Decision Tree | 0.746 | 0.750 | 0.737 | 0.651 | 0.702 | 0.743 |
CI-based KNN | 0.712 | 0.825 | 0.474 | 0.514 | 0.603 | 0.625 |
Note: Best performance for ApEn-based, SampEn-based, and CI-based models is highlighted in bold. |
Models | Accuracy | Specificity | Sensitivity | F1 score | PR-AUC | G-mean |
ApEn-based SVM | 0.881 | 0.925 | 0.789 | 0.811 | 0.845 | 0.855 |
ApEn-based MLP | 0.814 | 0.775 | 0.895 | 0.756 | 0.791 | 0.833 |
ApEn-based Gradient Boost | 0.797 | 0.850 | 0.684 | 0.684 | 0.735 | 0.763 |
ApEn-based Bagging | 0.864 | 0.925 | 0.737 | 0.778 | 0.823 | 0.826 |
ApEn-based Random Forest | 0.847 | 0.925 | 0.684 | 0.743 | 0.799 | 0.796 |
ApEn-based Ada Boost | 0.847 | 0.925 | 0.684 | 0.743 | 0.799 | 0.796 |
ApEn-based Decision Tree | 0.847 | 0.925 | 0.684 | 0.743 | 0.799 | 0.796 |
ApEn-based KNN | 0.831 | 0.925 | 0.632 | 0.706 | 0.775 | 0.764 |
SampEn-based SVM | 0.847 | 0.900 | 0.737 | 0.757 | 0.800 | 0.814 |
SampEn-based MLP | 0.814 | 0.850 | 0.737 | 0.718 | 0.761 | 0.791 |
SampEn-based Gradient Boost | 0.864 | 0.900 | 0.789 | 0.789 | 0.823 | 0.843 |
SampEn-based Bagging | 0.864 | 0.925 | 0.737 | 0.778 | 0.823 | 0.826 |
SampEn-based Random Forest | 0.864 | 0.925 | 0.737 | 0.778 | 0.823 | 0.826 |
SampEn-based Ada Boost | 0.831 | 0.925 | 0.632 | 0.706 | 0.775 | 0.764 |
SampEn-based Decision Tree | 0.797 | 0.850 | 0.684 | 0.684 | 0.735 | 0.763 |
SampEn-based KNN | 0.814 | 0.900 | 0.632 | 0.686 | 0.750 | 0.754 |
CI-based SVM | 0.831 | 0.875 | 0.737 | 0.737 | 0.779 | 0.803 |
CI-based MLP | 0.729 | 0.750 | 0.684 | 0.619 | 0.676 | 0.716 |
CI-based Gradient Boost | 0.763 | 0.825 | 0.632 | 0.632 | 0.691 | 0.722 |
CI-based Bagging | 0.763 | 0.825 | 0.632 | 0.632 | 0.691 | 0.722 |
CI-based Random Forest | 0.729 | 0.825 | 0.526 | 0.556 | 0.633 | 0.659 |
CI-based Ada Boost | 0.695 | 0.750 | 0.579 | 0.550 | 0.619 | 0.659 |
CI-based Decision Tree | 0.678 | 0.675 | 0.684 | 0.578 | 0.643 | 0.680 |
CI-based KNN | 0.780 | 0.950 | 0.421 | 0.552 | 0.704 | 0.632 |
Note: Best performance for ApEn-based, SampEn-based, and CI-based models is highlighted in bold. |